CodeFatherTech
Learn to Code. Shape Your Future

How To Write a Unit Test in Python: A Simple Guide
Knowing how to write a unit test in Python is critical for developers. Just writing your application code is not enough, writing tests is a must.
Unit tests allow you to test self-contained units of your code independently from each other. Python provides the unittest framework that helps write unit tests following a pre-defined format. To test your code with the unittest framework you create test classes and test methods within each test class.
In this tutorial, you will write unit tests for a simple class that represents a user in a video game.
Let’s learn how to write and run tests in Python!
Why Should You Write Tests in Python?
When you write code you might assume that your code works fine just based on quick manual tests you execute after writing that code.
But, will you run the same tests manually every time you change your code? Unlikely!
To test a piece of code you have to make sure to cover all the different ways that code can be used in your program (e.g. passing different types of values for arguments if you are testing a Python function ).
Also, you want to make sure the tests you execute the first time after writing the code are always executed automatically in the future when you change that code. This is why you create a set of tests for your code.
Unit tests are one of the types of tests you can write to test your code. Every unit test covers a specific use case for that code and can be executed every time you modify your code.
Why is it best practice to always implement unit tests and execute unit tests when changing your code?
Executing unit tests helps you make sure not to break the existing logic in your code when you make changes to it (e.g. to provide a new feature). With unit tests, you test code continuously and hence you notice immediately, while writing your code, if you are breaking existing functionality. This allows you to fix errors straight away and keep your Python source code stable.
The Class We Will Write Unit Tests For
The following class represents a user who plays a video game. This class has the following functionalities:
- Activate the user account.
- Check if the user account is active.
- Add points to the user.
- Retrieve points assigned to the user.
- Get the level the user has reached in the game (it depends on the number of points).
The only class attribute is the profile dictionary that stores all the details related to the user.
Let’s create an instance of this class and run some manual tests to make sure it works as expected.
What is Manual Testing in Python?
Manual testing is the process of testing the functionality of your application by going through use cases one by one.
Think about it as a list of tests you manually run against your application to make sure it behaves as expected. This is also called exploratory testing .
Here is an example…
We will test three different use cases for our class. The first step before doing that is to create an instance of your class :
As you can see the profile of the user has been initialised correctly.
1st Use Case: User state is active after completing the activation – SUCCESS
2nd Use Case: User points are incremented correctly – SUCCESS
3rd Use Case: User level changes from 1 to 2 when the number of points is greater than 200 – SUCCESS
These tests give us some confirmation that the code does what it was designed for.
However, the problem is that you would have to run each single test manually every time the code changes considering that any change could break the existing code.
This is not a great approach, these are just three tests, imagine if you had to run hundreds of tests every time your code changes.
That’s why unit tests are important as a form of automated testing .
How To Write a Unit Test For a Class in Python
Now we will see how to use the Python unittest framework to write the three tests executed in the previous section.
Firstly, let’s assume that the main application code is in the file user.py . You will write your unit tests in a file called test_user.py .
The common naming convention for unit tests: the name of the file used for unit tests simply prepends “test_” to the .py file where the Python code to be tested is.
To use the unittest framework we have to do the following:
- import the unittest module
- create a test class that inherits unittest.TestCase . We will call it TestUser.
- add one method for each test.
- add an entry point to execute the tests from the command line using unittest.main .
Here is a Python unittest example:
You have created the structure of the test class. Before adding the implementation to each unit test class method let’s try to execute the tests to see what happens.
To run unit tests in Python you can use the following syntax:
The value of __name__ is checked when you execute the test_user.py file via the command line.
Note : the version of Python we are using in all the examples is Python 3.
How Do You Write a Unit Test in Python?
Now that we have the structure of our test class we can implement each test method.
Unit tests have this name because they test units of your Python code, in this case, the behavior of the methods in the class User.
Each unit test should be designed to verify that the behavior of our class is correct when a specific sequence of events occurs. As part of each unit test, you provide a set of inputs and then verify the output is the same as you expected using the concept of assertions.
In other words, each unit test automates the manual tests we have executed previously.
Technically, you could use the assert statement to verify the value returned by methods of our User class.
In practice, the unittest framework provides its assertion methods. We will use the following in our tests:
- assertEqual
Let’s start with the first test case…
…actually before doing that we need to be able to see the User class from our test class.
How can we do that?
This is the content of the current directory:
To use the User class in our tests add the following import after the unittest import in test_user.py :
And now let’s implement three unit tests.
1st Use Case: User state is active after activation has been completed
In this test, we activate the user and then assert that the is_active() method returns True.
2nd Use Case: User points are incremented correctly
This time instead of using assertTrue we have used assertEqual to verify the number of points assigned to the user.
3rd Use Case: User level changes from 1 to 2 when the number of points is greater than 200
The implementation of this unit test is similar to the previous one with the only difference being that we are asserting the value of the level for the user.
And now it’s the moment to run our tests…
All the tests are successful!
An Example of Unit Test Failure
Before completing this tutorial I want to show you what would happen if one of the tests fails.
First of all, let’s assume that there is a typo in the is_active() method:
I have replaced the attribute active of the user profile with active_user which doesn’t exist in the profile dictionary.
Now, run the tests again…
In the first line of the test execution you can see:
Each character represents the execution of a test. E indicates an error while a dot indicates a success.
This means that the first test in the test class has failed and the other two are successful.
The output of the test runner also tells us that the error is caused by the assertTrue part of the test_user_activation method.
This helps you identify what’s wrong with the code and fix it.
That was an interesting journey through unit testing in Python.
We have seen how to use unittest, one of the test frameworks provided by Python, to write unit tests for your programs.
Now you have all you need to start writing tests in Python for your application if you haven’t done it before 🙂
Related article : To bring your knowledge of Python testing to the next level have a look at the CodeFatherTech tutorial about creating mocks in Python .
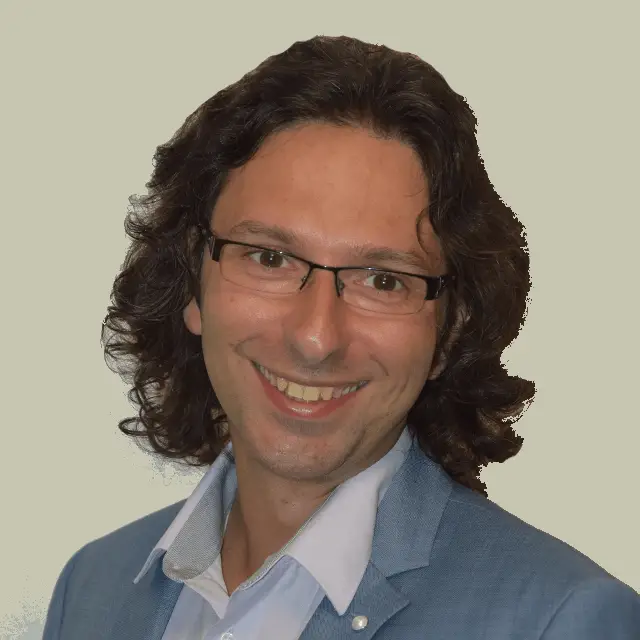
Claudio Sabato is an IT expert with over 15 years of professional experience in Python programming, Linux Systems Administration, Bash programming, and IT Systems Design. He is a professional certified by the Linux Professional Institute .
With a Master’s degree in Computer Science, he has a strong foundation in Software Engineering and a passion for robotics with Raspberry Pi.
Related posts:
- Python Assert Statement: Learn It in 7 Steps
- Mastering Python Mock and Patch: Mocking For Unit Testing
1 thought on “How To Write a Unit Test in Python: A Simple Guide”
Excellent write up with clear and helpful examples – thank you!
Leave a Comment Cancel reply
Save my name, email, and website in this browser for the next time I comment.

- Privacy Overview
- Strictly Necessary Cookies
This website uses cookies so that we can provide you with the best user experience possible. Cookie information is stored in your browser and performs functions such as recognising you when you return to our website and helping our team to understand which sections of the website you find most interesting and useful.
Strictly Necessary Cookie should be enabled at all times so that we can save your preferences for cookie settings.
If you disable this cookie, we will not be able to save your preferences. This means that every time you visit this website you will need to enable or disable cookies again.
We're sorry, but something went wrong
We've been notified about the issue and we'll do our best to fix it as soon as possible. You can return to our home page , or drop us a line if you need help.
- Python »
- 3.12.3 Documentation »
- The Python Standard Library »
- Development Tools »
- unittest — Unit testing framework
- Theme Auto Light Dark |
unittest — Unit testing framework ¶
Source code: Lib/unittest/__init__.py
(If you are already familiar with the basic concepts of testing, you might want to skip to the list of assert methods .)
The unittest unit testing framework was originally inspired by JUnit and has a similar flavor as major unit testing frameworks in other languages. It supports test automation, sharing of setup and shutdown code for tests, aggregation of tests into collections, and independence of the tests from the reporting framework.
To achieve this, unittest supports some important concepts in an object-oriented way:
A test fixture represents the preparation needed to perform one or more tests, and any associated cleanup actions. This may involve, for example, creating temporary or proxy databases, directories, or starting a server process.
A test case is the individual unit of testing. It checks for a specific response to a particular set of inputs. unittest provides a base class, TestCase , which may be used to create new test cases.
A test suite is a collection of test cases, test suites, or both. It is used to aggregate tests that should be executed together.
A test runner is a component which orchestrates the execution of tests and provides the outcome to the user. The runner may use a graphical interface, a textual interface, or return a special value to indicate the results of executing the tests.
Another test-support module with a very different flavor.
Kent Beck’s original paper on testing frameworks using the pattern shared by unittest .
Third-party unittest framework with a lighter-weight syntax for writing tests. For example, assert func(10) == 42 .
An extensive list of Python testing tools including functional testing frameworks and mock object libraries.
A special-interest-group for discussion of testing, and testing tools, in Python.
The script Tools/unittestgui/unittestgui.py in the Python source distribution is a GUI tool for test discovery and execution. This is intended largely for ease of use for those new to unit testing. For production environments it is recommended that tests be driven by a continuous integration system such as Buildbot , Jenkins , GitHub Actions , or AppVeyor .
Basic example ¶
The unittest module provides a rich set of tools for constructing and running tests. This section demonstrates that a small subset of the tools suffice to meet the needs of most users.
Here is a short script to test three string methods:
A testcase is created by subclassing unittest.TestCase . The three individual tests are defined with methods whose names start with the letters test . This naming convention informs the test runner about which methods represent tests.
The crux of each test is a call to assertEqual() to check for an expected result; assertTrue() or assertFalse() to verify a condition; or assertRaises() to verify that a specific exception gets raised. These methods are used instead of the assert statement so the test runner can accumulate all test results and produce a report.
The setUp() and tearDown() methods allow you to define instructions that will be executed before and after each test method. They are covered in more detail in the section Organizing test code .
The final block shows a simple way to run the tests. unittest.main() provides a command-line interface to the test script. When run from the command line, the above script produces an output that looks like this:
Passing the -v option to your test script will instruct unittest.main() to enable a higher level of verbosity, and produce the following output:
The above examples show the most commonly used unittest features which are sufficient to meet many everyday testing needs. The remainder of the documentation explores the full feature set from first principles.
Changed in version 3.11: The behavior of returning a value from a test method (other than the default None value), is now deprecated.
Command-Line Interface ¶
The unittest module can be used from the command line to run tests from modules, classes or even individual test methods:
You can pass in a list with any combination of module names, and fully qualified class or method names.
Test modules can be specified by file path as well:
This allows you to use the shell filename completion to specify the test module. The file specified must still be importable as a module. The path is converted to a module name by removing the ‘.py’ and converting path separators into ‘.’. If you want to execute a test file that isn’t importable as a module you should execute the file directly instead.
You can run tests with more detail (higher verbosity) by passing in the -v flag:
When executed without arguments Test Discovery is started:
For a list of all the command-line options:
Changed in version 3.2: In earlier versions it was only possible to run individual test methods and not modules or classes.
Command-line options ¶
unittest supports these command-line options:
The standard output and standard error streams are buffered during the test run. Output during a passing test is discarded. Output is echoed normally on test fail or error and is added to the failure messages.
Control - C during the test run waits for the current test to end and then reports all the results so far. A second Control - C raises the normal KeyboardInterrupt exception.
See Signal Handling for the functions that provide this functionality.
Stop the test run on the first error or failure.
Only run test methods and classes that match the pattern or substring. This option may be used multiple times, in which case all test cases that match any of the given patterns are included.
Patterns that contain a wildcard character ( * ) are matched against the test name using fnmatch.fnmatchcase() ; otherwise simple case-sensitive substring matching is used.
Patterns are matched against the fully qualified test method name as imported by the test loader.
For example, -k foo matches foo_tests.SomeTest.test_something , bar_tests.SomeTest.test_foo , but not bar_tests.FooTest.test_something .
Show local variables in tracebacks.
Show the N slowest test cases (N=0 for all).
Added in version 3.2: The command-line options -b , -c and -f were added.
Added in version 3.5: The command-line option --locals .
Added in version 3.7: The command-line option -k .
Added in version 3.12: The command-line option --durations .
The command line can also be used for test discovery, for running all of the tests in a project or just a subset.
Test Discovery ¶
Added in version 3.2.
Unittest supports simple test discovery. In order to be compatible with test discovery, all of the test files must be modules or packages importable from the top-level directory of the project (this means that their filenames must be valid identifiers ).
Test discovery is implemented in TestLoader.discover() , but can also be used from the command line. The basic command-line usage is:
As a shortcut, python -m unittest is the equivalent of python -m unittest discover . If you want to pass arguments to test discovery the discover sub-command must be used explicitly.
The discover sub-command has the following options:
Verbose output
Directory to start discovery ( . default)
Pattern to match test files ( test*.py default)
Top level directory of project (defaults to start directory)
The -s , -p , and -t options can be passed in as positional arguments in that order. The following two command lines are equivalent:
As well as being a path it is possible to pass a package name, for example myproject.subpackage.test , as the start directory. The package name you supply will then be imported and its location on the filesystem will be used as the start directory.
Test discovery loads tests by importing them. Once test discovery has found all the test files from the start directory you specify it turns the paths into package names to import. For example foo/bar/baz.py will be imported as foo.bar.baz .
If you have a package installed globally and attempt test discovery on a different copy of the package then the import could happen from the wrong place. If this happens test discovery will warn you and exit.
If you supply the start directory as a package name rather than a path to a directory then discover assumes that whichever location it imports from is the location you intended, so you will not get the warning.
Test modules and packages can customize test loading and discovery by through the load_tests protocol .
Changed in version 3.4: Test discovery supports namespace packages for the start directory. Note that you need to specify the top level directory too (e.g. python -m unittest discover -s root/namespace -t root ).
Changed in version 3.11: unittest dropped the namespace packages support in Python 3.11. It has been broken since Python 3.7. Start directory and subdirectories containing tests must be regular package that have __init__.py file.
Directories containing start directory still can be a namespace package. In this case, you need to specify start directory as dotted package name, and target directory explicitly. For example:
Organizing test code ¶
The basic building blocks of unit testing are test cases — single scenarios that must be set up and checked for correctness. In unittest , test cases are represented by unittest.TestCase instances. To make your own test cases you must write subclasses of TestCase or use FunctionTestCase .
The testing code of a TestCase instance should be entirely self contained, such that it can be run either in isolation or in arbitrary combination with any number of other test cases.
The simplest TestCase subclass will simply implement a test method (i.e. a method whose name starts with test ) in order to perform specific testing code:
Note that in order to test something, we use one of the assert* methods provided by the TestCase base class. If the test fails, an exception will be raised with an explanatory message, and unittest will identify the test case as a failure . Any other exceptions will be treated as errors .
Tests can be numerous, and their set-up can be repetitive. Luckily, we can factor out set-up code by implementing a method called setUp() , which the testing framework will automatically call for every single test we run:
The order in which the various tests will be run is determined by sorting the test method names with respect to the built-in ordering for strings.
If the setUp() method raises an exception while the test is running, the framework will consider the test to have suffered an error, and the test method will not be executed.
Similarly, we can provide a tearDown() method that tidies up after the test method has been run:
If setUp() succeeded, tearDown() will be run whether the test method succeeded or not.
Such a working environment for the testing code is called a test fixture . A new TestCase instance is created as a unique test fixture used to execute each individual test method. Thus setUp() , tearDown() , and __init__() will be called once per test.
It is recommended that you use TestCase implementations to group tests together according to the features they test. unittest provides a mechanism for this: the test suite , represented by unittest ’s TestSuite class. In most cases, calling unittest.main() will do the right thing and collect all the module’s test cases for you and execute them.
However, should you want to customize the building of your test suite, you can do it yourself:
You can place the definitions of test cases and test suites in the same modules as the code they are to test (such as widget.py ), but there are several advantages to placing the test code in a separate module, such as test_widget.py :
The test module can be run standalone from the command line.
The test code can more easily be separated from shipped code.
There is less temptation to change test code to fit the code it tests without a good reason.
Test code should be modified much less frequently than the code it tests.
Tested code can be refactored more easily.
Tests for modules written in C must be in separate modules anyway, so why not be consistent?
If the testing strategy changes, there is no need to change the source code.
Re-using old test code ¶
Some users will find that they have existing test code that they would like to run from unittest , without converting every old test function to a TestCase subclass.
For this reason, unittest provides a FunctionTestCase class. This subclass of TestCase can be used to wrap an existing test function. Set-up and tear-down functions can also be provided.
Given the following test function:
one can create an equivalent test case instance as follows, with optional set-up and tear-down methods:
Even though FunctionTestCase can be used to quickly convert an existing test base over to a unittest -based system, this approach is not recommended. Taking the time to set up proper TestCase subclasses will make future test refactorings infinitely easier.
In some cases, the existing tests may have been written using the doctest module. If so, doctest provides a DocTestSuite class that can automatically build unittest.TestSuite instances from the existing doctest -based tests.
Skipping tests and expected failures ¶
Added in version 3.1.
Unittest supports skipping individual test methods and even whole classes of tests. In addition, it supports marking a test as an “expected failure,” a test that is broken and will fail, but shouldn’t be counted as a failure on a TestResult .
Skipping a test is simply a matter of using the skip() decorator or one of its conditional variants, calling TestCase.skipTest() within a setUp() or test method, or raising SkipTest directly.
Basic skipping looks like this:
This is the output of running the example above in verbose mode:
Classes can be skipped just like methods:
TestCase.setUp() can also skip the test. This is useful when a resource that needs to be set up is not available.
Expected failures use the expectedFailure() decorator.
It’s easy to roll your own skipping decorators by making a decorator that calls skip() on the test when it wants it to be skipped. This decorator skips the test unless the passed object has a certain attribute:
The following decorators and exception implement test skipping and expected failures:
Unconditionally skip the decorated test. reason should describe why the test is being skipped.
Skip the decorated test if condition is true.
Skip the decorated test unless condition is true.
Mark the test as an expected failure or error. If the test fails or errors in the test function itself (rather than in one of the test fixture methods) then it will be considered a success. If the test passes, it will be considered a failure.
This exception is raised to skip a test.
Usually you can use TestCase.skipTest() or one of the skipping decorators instead of raising this directly.
Skipped tests will not have setUp() or tearDown() run around them. Skipped classes will not have setUpClass() or tearDownClass() run. Skipped modules will not have setUpModule() or tearDownModule() run.
Distinguishing test iterations using subtests ¶
Added in version 3.4.
When there are very small differences among your tests, for instance some parameters, unittest allows you to distinguish them inside the body of a test method using the subTest() context manager.
For example, the following test:
will produce the following output:
Without using a subtest, execution would stop after the first failure, and the error would be less easy to diagnose because the value of i wouldn’t be displayed:
Classes and functions ¶
This section describes in depth the API of unittest .
Test cases ¶
Instances of the TestCase class represent the logical test units in the unittest universe. This class is intended to be used as a base class, with specific tests being implemented by concrete subclasses. This class implements the interface needed by the test runner to allow it to drive the tests, and methods that the test code can use to check for and report various kinds of failure.
Each instance of TestCase will run a single base method: the method named methodName . In most uses of TestCase , you will neither change the methodName nor reimplement the default runTest() method.
Changed in version 3.2: TestCase can be instantiated successfully without providing a methodName . This makes it easier to experiment with TestCase from the interactive interpreter.
TestCase instances provide three groups of methods: one group used to run the test, another used by the test implementation to check conditions and report failures, and some inquiry methods allowing information about the test itself to be gathered.
Methods in the first group (running the test) are:
Method called to prepare the test fixture. This is called immediately before calling the test method; other than AssertionError or SkipTest , any exception raised by this method will be considered an error rather than a test failure. The default implementation does nothing.
Method called immediately after the test method has been called and the result recorded. This is called even if the test method raised an exception, so the implementation in subclasses may need to be particularly careful about checking internal state. Any exception, other than AssertionError or SkipTest , raised by this method will be considered an additional error rather than a test failure (thus increasing the total number of reported errors). This method will only be called if the setUp() succeeds, regardless of the outcome of the test method. The default implementation does nothing.
A class method called before tests in an individual class are run. setUpClass is called with the class as the only argument and must be decorated as a classmethod() :
See Class and Module Fixtures for more details.
A class method called after tests in an individual class have run. tearDownClass is called with the class as the only argument and must be decorated as a classmethod() :
Run the test, collecting the result into the TestResult object passed as result . If result is omitted or None , a temporary result object is created (by calling the defaultTestResult() method) and used. The result object is returned to run() ’s caller.
The same effect may be had by simply calling the TestCase instance.
Changed in version 3.3: Previous versions of run did not return the result. Neither did calling an instance.
Calling this during a test method or setUp() skips the current test. See Skipping tests and expected failures for more information.
Return a context manager which executes the enclosed code block as a subtest. msg and params are optional, arbitrary values which are displayed whenever a subtest fails, allowing you to identify them clearly.
A test case can contain any number of subtest declarations, and they can be arbitrarily nested.
See Distinguishing test iterations using subtests for more information.
Run the test without collecting the result. This allows exceptions raised by the test to be propagated to the caller, and can be used to support running tests under a debugger.
The TestCase class provides several assert methods to check for and report failures. The following table lists the most commonly used methods (see the tables below for more assert methods):
All the assert methods accept a msg argument that, if specified, is used as the error message on failure (see also longMessage ). Note that the msg keyword argument can be passed to assertRaises() , assertRaisesRegex() , assertWarns() , assertWarnsRegex() only when they are used as a context manager.
Test that first and second are equal. If the values do not compare equal, the test will fail.
In addition, if first and second are the exact same type and one of list, tuple, dict, set, frozenset or str or any type that a subclass registers with addTypeEqualityFunc() the type-specific equality function will be called in order to generate a more useful default error message (see also the list of type-specific methods ).
Changed in version 3.1: Added the automatic calling of type-specific equality function.
Changed in version 3.2: assertMultiLineEqual() added as the default type equality function for comparing strings.
Test that first and second are not equal. If the values do compare equal, the test will fail.
Test that expr is true (or false).
Note that this is equivalent to bool(expr) is True and not to expr is True (use assertIs(expr, True) for the latter). This method should also be avoided when more specific methods are available (e.g. assertEqual(a, b) instead of assertTrue(a == b) ), because they provide a better error message in case of failure.
Test that first and second are (or are not) the same object.
Test that expr is (or is not) None .
Test that member is (or is not) in container .
Test that obj is (or is not) an instance of cls (which can be a class or a tuple of classes, as supported by isinstance() ). To check for the exact type, use assertIs(type(obj), cls) .
It is also possible to check the production of exceptions, warnings, and log messages using the following methods:
Test that an exception is raised when callable is called with any positional or keyword arguments that are also passed to assertRaises() . The test passes if exception is raised, is an error if another exception is raised, or fails if no exception is raised. To catch any of a group of exceptions, a tuple containing the exception classes may be passed as exception .
If only the exception and possibly the msg arguments are given, return a context manager so that the code under test can be written inline rather than as a function:
When used as a context manager, assertRaises() accepts the additional keyword argument msg .
The context manager will store the caught exception object in its exception attribute. This can be useful if the intention is to perform additional checks on the exception raised:
Changed in version 3.1: Added the ability to use assertRaises() as a context manager.
Changed in version 3.2: Added the exception attribute.
Changed in version 3.3: Added the msg keyword argument when used as a context manager.
Like assertRaises() but also tests that regex matches on the string representation of the raised exception. regex may be a regular expression object or a string containing a regular expression suitable for use by re.search() . Examples:
Added in version 3.1: Added under the name assertRaisesRegexp .
Changed in version 3.2: Renamed to assertRaisesRegex() .
Test that a warning is triggered when callable is called with any positional or keyword arguments that are also passed to assertWarns() . The test passes if warning is triggered and fails if it isn’t. Any exception is an error. To catch any of a group of warnings, a tuple containing the warning classes may be passed as warnings .
If only the warning and possibly the msg arguments are given, return a context manager so that the code under test can be written inline rather than as a function:
When used as a context manager, assertWarns() accepts the additional keyword argument msg .
The context manager will store the caught warning object in its warning attribute, and the source line which triggered the warnings in the filename and lineno attributes. This can be useful if the intention is to perform additional checks on the warning caught:
This method works regardless of the warning filters in place when it is called.
Like assertWarns() but also tests that regex matches on the message of the triggered warning. regex may be a regular expression object or a string containing a regular expression suitable for use by re.search() . Example:
A context manager to test that at least one message is logged on the logger or one of its children, with at least the given level .
If given, logger should be a logging.Logger object or a str giving the name of a logger. The default is the root logger, which will catch all messages that were not blocked by a non-propagating descendent logger.
If given, level should be either a numeric logging level or its string equivalent (for example either "ERROR" or logging.ERROR ). The default is logging.INFO .
The test passes if at least one message emitted inside the with block matches the logger and level conditions, otherwise it fails.
The object returned by the context manager is a recording helper which keeps tracks of the matching log messages. It has two attributes:
A list of logging.LogRecord objects of the matching log messages.
A list of str objects with the formatted output of matching messages.
A context manager to test that no messages are logged on the logger or one of its children, with at least the given level .
If given, logger should be a logging.Logger object or a str giving the name of a logger. The default is the root logger, which will catch all messages.
Unlike assertLogs() , nothing will be returned by the context manager.
Added in version 3.10.
There are also other methods used to perform more specific checks, such as:
Test that first and second are approximately (or not approximately) equal by computing the difference, rounding to the given number of decimal places (default 7), and comparing to zero. Note that these methods round the values to the given number of decimal places (i.e. like the round() function) and not significant digits .
If delta is supplied instead of places then the difference between first and second must be less or equal to (or greater than) delta .
Supplying both delta and places raises a TypeError .
Changed in version 3.2: assertAlmostEqual() automatically considers almost equal objects that compare equal. assertNotAlmostEqual() automatically fails if the objects compare equal. Added the delta keyword argument.
Test that first is respectively >, >=, < or <= than second depending on the method name. If not, the test will fail:
Test that a regex search matches (or does not match) text . In case of failure, the error message will include the pattern and the text (or the pattern and the part of text that unexpectedly matched). regex may be a regular expression object or a string containing a regular expression suitable for use by re.search() .
Added in version 3.1: Added under the name assertRegexpMatches .
Changed in version 3.2: The method assertRegexpMatches() has been renamed to assertRegex() .
Added in version 3.2: assertNotRegex() .
Test that sequence first contains the same elements as second , regardless of their order. When they don’t, an error message listing the differences between the sequences will be generated.
Duplicate elements are not ignored when comparing first and second . It verifies whether each element has the same count in both sequences. Equivalent to: assertEqual(Counter(list(first)), Counter(list(second))) but works with sequences of unhashable objects as well.
The assertEqual() method dispatches the equality check for objects of the same type to different type-specific methods. These methods are already implemented for most of the built-in types, but it’s also possible to register new methods using addTypeEqualityFunc() :
Registers a type-specific method called by assertEqual() to check if two objects of exactly the same typeobj (not subclasses) compare equal. function must take two positional arguments and a third msg=None keyword argument just as assertEqual() does. It must raise self.failureException(msg) when inequality between the first two parameters is detected – possibly providing useful information and explaining the inequalities in details in the error message.
The list of type-specific methods automatically used by assertEqual() are summarized in the following table. Note that it’s usually not necessary to invoke these methods directly.
Test that the multiline string first is equal to the string second . When not equal a diff of the two strings highlighting the differences will be included in the error message. This method is used by default when comparing strings with assertEqual() .
Tests that two sequences are equal. If a seq_type is supplied, both first and second must be instances of seq_type or a failure will be raised. If the sequences are different an error message is constructed that shows the difference between the two.
This method is not called directly by assertEqual() , but it’s used to implement assertListEqual() and assertTupleEqual() .
Tests that two lists or tuples are equal. If not, an error message is constructed that shows only the differences between the two. An error is also raised if either of the parameters are of the wrong type. These methods are used by default when comparing lists or tuples with assertEqual() .
Tests that two sets are equal. If not, an error message is constructed that lists the differences between the sets. This method is used by default when comparing sets or frozensets with assertEqual() .
Fails if either of first or second does not have a set.difference() method.
Test that two dictionaries are equal. If not, an error message is constructed that shows the differences in the dictionaries. This method will be used by default to compare dictionaries in calls to assertEqual() .
Finally the TestCase provides the following methods and attributes:
Signals a test failure unconditionally, with msg or None for the error message.
This class attribute gives the exception raised by the test method. If a test framework needs to use a specialized exception, possibly to carry additional information, it must subclass this exception in order to “play fair” with the framework. The initial value of this attribute is AssertionError .
This class attribute determines what happens when a custom failure message is passed as the msg argument to an assertXYY call that fails. True is the default value. In this case, the custom message is appended to the end of the standard failure message. When set to False , the custom message replaces the standard message.
The class setting can be overridden in individual test methods by assigning an instance attribute, self.longMessage, to True or False before calling the assert methods.
The class setting gets reset before each test call.
This attribute controls the maximum length of diffs output by assert methods that report diffs on failure. It defaults to 80*8 characters. Assert methods affected by this attribute are assertSequenceEqual() (including all the sequence comparison methods that delegate to it), assertDictEqual() and assertMultiLineEqual() .
Setting maxDiff to None means that there is no maximum length of diffs.
Testing frameworks can use the following methods to collect information on the test:
Return the number of tests represented by this test object. For TestCase instances, this will always be 1 .
Return an instance of the test result class that should be used for this test case class (if no other result instance is provided to the run() method).
For TestCase instances, this will always be an instance of TestResult ; subclasses of TestCase should override this as necessary.
Return a string identifying the specific test case. This is usually the full name of the test method, including the module and class name.
Returns a description of the test, or None if no description has been provided. The default implementation of this method returns the first line of the test method’s docstring, if available, or None .
Changed in version 3.1: In 3.1 this was changed to add the test name to the short description even in the presence of a docstring. This caused compatibility issues with unittest extensions and adding the test name was moved to the TextTestResult in Python 3.2.
Add a function to be called after tearDown() to cleanup resources used during the test. Functions will be called in reverse order to the order they are added ( LIFO ). They are called with any arguments and keyword arguments passed into addCleanup() when they are added.
If setUp() fails, meaning that tearDown() is not called, then any cleanup functions added will still be called.
Enter the supplied context manager . If successful, also add its __exit__() method as a cleanup function by addCleanup() and return the result of the __enter__() method.
Added in version 3.11.
This method is called unconditionally after tearDown() , or after setUp() if setUp() raises an exception.
It is responsible for calling all the cleanup functions added by addCleanup() . If you need cleanup functions to be called prior to tearDown() then you can call doCleanups() yourself.
doCleanups() pops methods off the stack of cleanup functions one at a time, so it can be called at any time.
Add a function to be called after tearDownClass() to cleanup resources used during the test class. Functions will be called in reverse order to the order they are added ( LIFO ). They are called with any arguments and keyword arguments passed into addClassCleanup() when they are added.
If setUpClass() fails, meaning that tearDownClass() is not called, then any cleanup functions added will still be called.
Added in version 3.8.
Enter the supplied context manager . If successful, also add its __exit__() method as a cleanup function by addClassCleanup() and return the result of the __enter__() method.
This method is called unconditionally after tearDownClass() , or after setUpClass() if setUpClass() raises an exception.
It is responsible for calling all the cleanup functions added by addClassCleanup() . If you need cleanup functions to be called prior to tearDownClass() then you can call doClassCleanups() yourself.
doClassCleanups() pops methods off the stack of cleanup functions one at a time, so it can be called at any time.
This class provides an API similar to TestCase and also accepts coroutines as test functions.
Method called to prepare the test fixture. This is called after setUp() . This is called immediately before calling the test method; other than AssertionError or SkipTest , any exception raised by this method will be considered an error rather than a test failure. The default implementation does nothing.
Method called immediately after the test method has been called and the result recorded. This is called before tearDown() . This is called even if the test method raised an exception, so the implementation in subclasses may need to be particularly careful about checking internal state. Any exception, other than AssertionError or SkipTest , raised by this method will be considered an additional error rather than a test failure (thus increasing the total number of reported errors). This method will only be called if the asyncSetUp() succeeds, regardless of the outcome of the test method. The default implementation does nothing.
This method accepts a coroutine that can be used as a cleanup function.
Enter the supplied asynchronous context manager . If successful, also add its __aexit__() method as a cleanup function by addAsyncCleanup() and return the result of the __aenter__() method.
Sets up a new event loop to run the test, collecting the result into the TestResult object passed as result . If result is omitted or None , a temporary result object is created (by calling the defaultTestResult() method) and used. The result object is returned to run() ’s caller. At the end of the test all the tasks in the event loop are cancelled.
An example illustrating the order:
After running the test, events would contain ["setUp", "asyncSetUp", "test_response", "asyncTearDown", "tearDown", "cleanup"] .
This class implements the portion of the TestCase interface which allows the test runner to drive the test, but does not provide the methods which test code can use to check and report errors. This is used to create test cases using legacy test code, allowing it to be integrated into a unittest -based test framework.
Grouping tests ¶
This class represents an aggregation of individual test cases and test suites. The class presents the interface needed by the test runner to allow it to be run as any other test case. Running a TestSuite instance is the same as iterating over the suite, running each test individually.
If tests is given, it must be an iterable of individual test cases or other test suites that will be used to build the suite initially. Additional methods are provided to add test cases and suites to the collection later on.
TestSuite objects behave much like TestCase objects, except they do not actually implement a test. Instead, they are used to aggregate tests into groups of tests that should be run together. Some additional methods are available to add tests to TestSuite instances:
Add a TestCase or TestSuite to the suite.
Add all the tests from an iterable of TestCase and TestSuite instances to this test suite.
This is equivalent to iterating over tests , calling addTest() for each element.
TestSuite shares the following methods with TestCase :
Run the tests associated with this suite, collecting the result into the test result object passed as result . Note that unlike TestCase.run() , TestSuite.run() requires the result object to be passed in.
Run the tests associated with this suite without collecting the result. This allows exceptions raised by the test to be propagated to the caller and can be used to support running tests under a debugger.
Return the number of tests represented by this test object, including all individual tests and sub-suites.
Tests grouped by a TestSuite are always accessed by iteration. Subclasses can lazily provide tests by overriding __iter__() . Note that this method may be called several times on a single suite (for example when counting tests or comparing for equality) so the tests returned by repeated iterations before TestSuite.run() must be the same for each call iteration. After TestSuite.run() , callers should not rely on the tests returned by this method unless the caller uses a subclass that overrides TestSuite._removeTestAtIndex() to preserve test references.
Changed in version 3.2: In earlier versions the TestSuite accessed tests directly rather than through iteration, so overriding __iter__() wasn’t sufficient for providing tests.
Changed in version 3.4: In earlier versions the TestSuite held references to each TestCase after TestSuite.run() . Subclasses can restore that behavior by overriding TestSuite._removeTestAtIndex() .
In the typical usage of a TestSuite object, the run() method is invoked by a TestRunner rather than by the end-user test harness.
Loading and running tests ¶
The TestLoader class is used to create test suites from classes and modules. Normally, there is no need to create an instance of this class; the unittest module provides an instance that can be shared as unittest.defaultTestLoader . Using a subclass or instance, however, allows customization of some configurable properties.
TestLoader objects have the following attributes:
A list of the non-fatal errors encountered while loading tests. Not reset by the loader at any point. Fatal errors are signalled by the relevant method raising an exception to the caller. Non-fatal errors are also indicated by a synthetic test that will raise the original error when run.
Added in version 3.5.
TestLoader objects have the following methods:
Return a suite of all test cases contained in the TestCase -derived testCaseClass .
A test case instance is created for each method named by getTestCaseNames() . By default these are the method names beginning with test . If getTestCaseNames() returns no methods, but the runTest() method is implemented, a single test case is created for that method instead.
Return a suite of all test cases contained in the given module. This method searches module for classes derived from TestCase and creates an instance of the class for each test method defined for the class.
While using a hierarchy of TestCase -derived classes can be convenient in sharing fixtures and helper functions, defining test methods on base classes that are not intended to be instantiated directly does not play well with this method. Doing so, however, can be useful when the fixtures are different and defined in subclasses.
If a module provides a load_tests function it will be called to load the tests. This allows modules to customize test loading. This is the load_tests protocol . The pattern argument is passed as the third argument to load_tests .
Changed in version 3.2: Support for load_tests added.
Changed in version 3.5: Support for a keyword-only argument pattern has been added.
Changed in version 3.12: The undocumented and unofficial use_load_tests parameter has been removed.
Return a suite of all test cases given a string specifier.
The specifier name is a “dotted name” that may resolve either to a module, a test case class, a test method within a test case class, a TestSuite instance, or a callable object which returns a TestCase or TestSuite instance. These checks are applied in the order listed here; that is, a method on a possible test case class will be picked up as “a test method within a test case class”, rather than “a callable object”.
For example, if you have a module SampleTests containing a TestCase -derived class SampleTestCase with three test methods ( test_one() , test_two() , and test_three() ), the specifier 'SampleTests.SampleTestCase' would cause this method to return a suite which will run all three test methods. Using the specifier 'SampleTests.SampleTestCase.test_two' would cause it to return a test suite which will run only the test_two() test method. The specifier can refer to modules and packages which have not been imported; they will be imported as a side-effect.
The method optionally resolves name relative to the given module .
Changed in version 3.5: If an ImportError or AttributeError occurs while traversing name then a synthetic test that raises that error when run will be returned. These errors are included in the errors accumulated by self.errors.
Similar to loadTestsFromName() , but takes a sequence of names rather than a single name. The return value is a test suite which supports all the tests defined for each name.
Return a sorted sequence of method names found within testCaseClass ; this should be a subclass of TestCase .
Find all the test modules by recursing into subdirectories from the specified start directory, and return a TestSuite object containing them. Only test files that match pattern will be loaded. (Using shell style pattern matching.) Only module names that are importable (i.e. are valid Python identifiers) will be loaded.
All test modules must be importable from the top level of the project. If the start directory is not the top level directory then top_level_dir must be specified separately.
If importing a module fails, for example due to a syntax error, then this will be recorded as a single error and discovery will continue. If the import failure is due to SkipTest being raised, it will be recorded as a skip instead of an error.
If a package (a directory containing a file named __init__.py ) is found, the package will be checked for a load_tests function. If this exists then it will be called package.load_tests(loader, tests, pattern) . Test discovery takes care to ensure that a package is only checked for tests once during an invocation, even if the load_tests function itself calls loader.discover .
If load_tests exists then discovery does not recurse into the package, load_tests is responsible for loading all tests in the package.
The pattern is deliberately not stored as a loader attribute so that packages can continue discovery themselves.
top_level_dir is stored internally, and used as a default to any nested calls to discover() . That is, if a package’s load_tests calls loader.discover() , it does not need to pass this argument.
start_dir can be a dotted module name as well as a directory.
Changed in version 3.4: Modules that raise SkipTest on import are recorded as skips, not errors.
Changed in version 3.4: start_dir can be a namespace packages .
Changed in version 3.4: Paths are sorted before being imported so that execution order is the same even if the underlying file system’s ordering is not dependent on file name.
Changed in version 3.5: Found packages are now checked for load_tests regardless of whether their path matches pattern , because it is impossible for a package name to match the default pattern.
Changed in version 3.11: start_dir can not be a namespace packages . It has been broken since Python 3.7 and Python 3.11 officially remove it.
Changed in version 3.12.4: top_level_dir is only stored for the duration of discover call.
The following attributes of a TestLoader can be configured either by subclassing or assignment on an instance:
String giving the prefix of method names which will be interpreted as test methods. The default value is 'test' .
This affects getTestCaseNames() and all the loadTestsFrom* methods.
Function to be used to compare method names when sorting them in getTestCaseNames() and all the loadTestsFrom* methods.
Callable object that constructs a test suite from a list of tests. No methods on the resulting object are needed. The default value is the TestSuite class.
This affects all the loadTestsFrom* methods.
List of Unix shell-style wildcard test name patterns that test methods have to match to be included in test suites (see -k option).
If this attribute is not None (the default), all test methods to be included in test suites must match one of the patterns in this list. Note that matches are always performed using fnmatch.fnmatchcase() , so unlike patterns passed to the -k option, simple substring patterns will have to be converted using * wildcards.
Added in version 3.7.
This class is used to compile information about which tests have succeeded and which have failed.
A TestResult object stores the results of a set of tests. The TestCase and TestSuite classes ensure that results are properly recorded; test authors do not need to worry about recording the outcome of tests.
Testing frameworks built on top of unittest may want access to the TestResult object generated by running a set of tests for reporting purposes; a TestResult instance is returned by the TestRunner.run() method for this purpose.
TestResult instances have the following attributes that will be of interest when inspecting the results of running a set of tests:
A list containing 2-tuples of TestCase instances and strings holding formatted tracebacks. Each tuple represents a test which raised an unexpected exception.
A list containing 2-tuples of TestCase instances and strings holding formatted tracebacks. Each tuple represents a test where a failure was explicitly signalled using the assert* methods .
A list containing 2-tuples of TestCase instances and strings holding the reason for skipping the test.
A list containing 2-tuples of TestCase instances and strings holding formatted tracebacks. Each tuple represents an expected failure or error of the test case.
A list containing TestCase instances that were marked as expected failures, but succeeded.
A list containing 2-tuples of test case names and floats representing the elapsed time of each test which was run.
Added in version 3.12.
Set to True when the execution of tests should stop by stop() .
The total number of tests run so far.
If set to true, sys.stdout and sys.stderr will be buffered in between startTest() and stopTest() being called. Collected output will only be echoed onto the real sys.stdout and sys.stderr if the test fails or errors. Any output is also attached to the failure / error message.
If set to true stop() will be called on the first failure or error, halting the test run.
If set to true then local variables will be shown in tracebacks.
Return True if all tests run so far have passed, otherwise returns False .
Changed in version 3.4: Returns False if there were any unexpectedSuccesses from tests marked with the expectedFailure() decorator.
This method can be called to signal that the set of tests being run should be aborted by setting the shouldStop attribute to True . TestRunner objects should respect this flag and return without running any additional tests.
For example, this feature is used by the TextTestRunner class to stop the test framework when the user signals an interrupt from the keyboard. Interactive tools which provide TestRunner implementations can use this in a similar manner.
The following methods of the TestResult class are used to maintain the internal data structures, and may be extended in subclasses to support additional reporting requirements. This is particularly useful in building tools which support interactive reporting while tests are being run.
Called when the test case test is about to be run.
Called after the test case test has been executed, regardless of the outcome.
Called once before any tests are executed.
Called once after all tests are executed.
Called when the test case test raises an unexpected exception. err is a tuple of the form returned by sys.exc_info() : (type, value, traceback) .
The default implementation appends a tuple (test, formatted_err) to the instance’s errors attribute, where formatted_err is a formatted traceback derived from err .
Called when the test case test signals a failure. err is a tuple of the form returned by sys.exc_info() : (type, value, traceback) .
The default implementation appends a tuple (test, formatted_err) to the instance’s failures attribute, where formatted_err is a formatted traceback derived from err .
Called when the test case test succeeds.
The default implementation does nothing.
Called when the test case test is skipped. reason is the reason the test gave for skipping.
The default implementation appends a tuple (test, reason) to the instance’s skipped attribute.
Called when the test case test fails or errors, but was marked with the expectedFailure() decorator.
The default implementation appends a tuple (test, formatted_err) to the instance’s expectedFailures attribute, where formatted_err is a formatted traceback derived from err .
Called when the test case test was marked with the expectedFailure() decorator, but succeeded.
The default implementation appends the test to the instance’s unexpectedSuccesses attribute.
Called when a subtest finishes. test is the test case corresponding to the test method. subtest is a custom TestCase instance describing the subtest.
If outcome is None , the subtest succeeded. Otherwise, it failed with an exception where outcome is a tuple of the form returned by sys.exc_info() : (type, value, traceback) .
The default implementation does nothing when the outcome is a success, and records subtest failures as normal failures.
Called when the test case finishes. elapsed is the time represented in seconds, and it includes the execution of cleanup functions.
A concrete implementation of TestResult used by the TextTestRunner . Subclasses should accept **kwargs to ensure compatibility as the interface changes.
Changed in version 3.12: Added the durations keyword parameter.
Instance of the TestLoader class intended to be shared. If no customization of the TestLoader is needed, this instance can be used instead of repeatedly creating new instances.
A basic test runner implementation that outputs results to a stream. If stream is None , the default, sys.stderr is used as the output stream. This class has a few configurable parameters, but is essentially very simple. Graphical applications which run test suites should provide alternate implementations. Such implementations should accept **kwargs as the interface to construct runners changes when features are added to unittest.
By default this runner shows DeprecationWarning , PendingDeprecationWarning , ResourceWarning and ImportWarning even if they are ignored by default . This behavior can be overridden using Python’s -Wd or -Wa options (see Warning control ) and leaving warnings to None .
Changed in version 3.2: Added the warnings parameter.
Changed in version 3.2: The default stream is set to sys.stderr at instantiation time rather than import time.
Changed in version 3.5: Added the tb_locals parameter.
Changed in version 3.12: Added the durations parameter.
This method returns the instance of TestResult used by run() . It is not intended to be called directly, but can be overridden in subclasses to provide a custom TestResult .
_makeResult() instantiates the class or callable passed in the TextTestRunner constructor as the resultclass argument. It defaults to TextTestResult if no resultclass is provided. The result class is instantiated with the following arguments:
This method is the main public interface to the TextTestRunner . This method takes a TestSuite or TestCase instance. A TestResult is created by calling _makeResult() and the test(s) are run and the results printed to stdout.
A command-line program that loads a set of tests from module and runs them; this is primarily for making test modules conveniently executable. The simplest use for this function is to include the following line at the end of a test script:
You can run tests with more detailed information by passing in the verbosity argument:
The defaultTest argument is either the name of a single test or an iterable of test names to run if no test names are specified via argv . If not specified or None and no test names are provided via argv , all tests found in module are run.
The argv argument can be a list of options passed to the program, with the first element being the program name. If not specified or None , the values of sys.argv are used.
The testRunner argument can either be a test runner class or an already created instance of it. By default main calls sys.exit() with an exit code indicating success (0) or failure (1) of the tests run. An exit code of 5 indicates that no tests were run or skipped.
The testLoader argument has to be a TestLoader instance, and defaults to defaultTestLoader .
main supports being used from the interactive interpreter by passing in the argument exit=False . This displays the result on standard output without calling sys.exit() :
The failfast , catchbreak and buffer parameters have the same effect as the same-name command-line options .
The warnings argument specifies the warning filter that should be used while running the tests. If it’s not specified, it will remain None if a -W option is passed to python (see Warning control ), otherwise it will be set to 'default' .
Calling main actually returns an instance of the TestProgram class. This stores the result of the tests run as the result attribute.
Changed in version 3.1: The exit parameter was added.
Changed in version 3.2: The verbosity , failfast , catchbreak , buffer and warnings parameters were added.
Changed in version 3.4: The defaultTest parameter was changed to also accept an iterable of test names.
load_tests Protocol ¶
Modules or packages can customize how tests are loaded from them during normal test runs or test discovery by implementing a function called load_tests .
If a test module defines load_tests it will be called by TestLoader.loadTestsFromModule() with the following arguments:
where pattern is passed straight through from loadTestsFromModule . It defaults to None .
It should return a TestSuite .
loader is the instance of TestLoader doing the loading. standard_tests are the tests that would be loaded by default from the module. It is common for test modules to only want to add or remove tests from the standard set of tests. The third argument is used when loading packages as part of test discovery.
A typical load_tests function that loads tests from a specific set of TestCase classes may look like:
If discovery is started in a directory containing a package, either from the command line or by calling TestLoader.discover() , then the package __init__.py will be checked for load_tests . If that function does not exist, discovery will recurse into the package as though it were just another directory. Otherwise, discovery of the package’s tests will be left up to load_tests which is called with the following arguments:
This should return a TestSuite representing all the tests from the package. ( standard_tests will only contain tests collected from __init__.py .)
Because the pattern is passed into load_tests the package is free to continue (and potentially modify) test discovery. A ‘do nothing’ load_tests function for a test package would look like:
Changed in version 3.5: Discovery no longer checks package names for matching pattern due to the impossibility of package names matching the default pattern.
Class and Module Fixtures ¶
Class and module level fixtures are implemented in TestSuite . When the test suite encounters a test from a new class then tearDownClass() from the previous class (if there is one) is called, followed by setUpClass() from the new class.
Similarly if a test is from a different module from the previous test then tearDownModule from the previous module is run, followed by setUpModule from the new module.
After all the tests have run the final tearDownClass and tearDownModule are run.
Note that shared fixtures do not play well with [potential] features like test parallelization and they break test isolation. They should be used with care.
The default ordering of tests created by the unittest test loaders is to group all tests from the same modules and classes together. This will lead to setUpClass / setUpModule (etc) being called exactly once per class and module. If you randomize the order, so that tests from different modules and classes are adjacent to each other, then these shared fixture functions may be called multiple times in a single test run.
Shared fixtures are not intended to work with suites with non-standard ordering. A BaseTestSuite still exists for frameworks that don’t want to support shared fixtures.
If there are any exceptions raised during one of the shared fixture functions the test is reported as an error. Because there is no corresponding test instance an _ErrorHolder object (that has the same interface as a TestCase ) is created to represent the error. If you are just using the standard unittest test runner then this detail doesn’t matter, but if you are a framework author it may be relevant.
setUpClass and tearDownClass ¶
These must be implemented as class methods:
If you want the setUpClass and tearDownClass on base classes called then you must call up to them yourself. The implementations in TestCase are empty.
If an exception is raised during a setUpClass then the tests in the class are not run and the tearDownClass is not run. Skipped classes will not have setUpClass or tearDownClass run. If the exception is a SkipTest exception then the class will be reported as having been skipped instead of as an error.
setUpModule and tearDownModule ¶
These should be implemented as functions:
If an exception is raised in a setUpModule then none of the tests in the module will be run and the tearDownModule will not be run. If the exception is a SkipTest exception then the module will be reported as having been skipped instead of as an error.
To add cleanup code that must be run even in the case of an exception, use addModuleCleanup :
Add a function to be called after tearDownModule() to cleanup resources used during the test class. Functions will be called in reverse order to the order they are added ( LIFO ). They are called with any arguments and keyword arguments passed into addModuleCleanup() when they are added.
If setUpModule() fails, meaning that tearDownModule() is not called, then any cleanup functions added will still be called.
Enter the supplied context manager . If successful, also add its __exit__() method as a cleanup function by addModuleCleanup() and return the result of the __enter__() method.
This function is called unconditionally after tearDownModule() , or after setUpModule() if setUpModule() raises an exception.
It is responsible for calling all the cleanup functions added by addModuleCleanup() . If you need cleanup functions to be called prior to tearDownModule() then you can call doModuleCleanups() yourself.
doModuleCleanups() pops methods off the stack of cleanup functions one at a time, so it can be called at any time.
Signal Handling ¶
The -c/--catch command-line option to unittest, along with the catchbreak parameter to unittest.main() , provide more friendly handling of control-C during a test run. With catch break behavior enabled control-C will allow the currently running test to complete, and the test run will then end and report all the results so far. A second control-c will raise a KeyboardInterrupt in the usual way.
The control-c handling signal handler attempts to remain compatible with code or tests that install their own signal.SIGINT handler. If the unittest handler is called but isn’t the installed signal.SIGINT handler, i.e. it has been replaced by the system under test and delegated to, then it calls the default handler. This will normally be the expected behavior by code that replaces an installed handler and delegates to it. For individual tests that need unittest control-c handling disabled the removeHandler() decorator can be used.
There are a few utility functions for framework authors to enable control-c handling functionality within test frameworks.
Install the control-c handler. When a signal.SIGINT is received (usually in response to the user pressing control-c) all registered results have stop() called.
Register a TestResult object for control-c handling. Registering a result stores a weak reference to it, so it doesn’t prevent the result from being garbage collected.
Registering a TestResult object has no side-effects if control-c handling is not enabled, so test frameworks can unconditionally register all results they create independently of whether or not handling is enabled.
Remove a registered result. Once a result has been removed then stop() will no longer be called on that result object in response to a control-c.
When called without arguments this function removes the control-c handler if it has been installed. This function can also be used as a test decorator to temporarily remove the handler while the test is being executed:
Table of Contents
- Basic example
- Command-line options
- Test Discovery
- Organizing test code
- Re-using old test code
- Skipping tests and expected failures
- Distinguishing test iterations using subtests
- Grouping tests
- load_tests Protocol
- setUpClass and tearDownClass
- setUpModule and tearDownModule
- Signal Handling
Previous topic
doctest — Test interactive Python examples
unittest.mock — mock object library
- Report a Bug
- Show Source
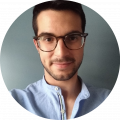
An Introduction to Python Unit Testing with unittest and pytest
Share this article
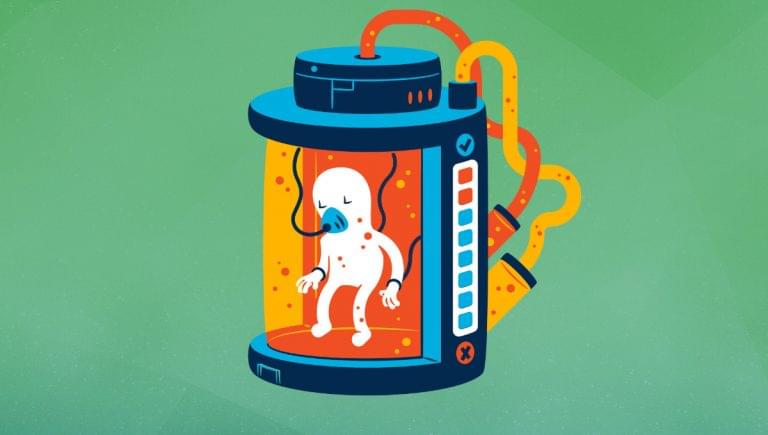

Introduction to Software Testing
Designing a test strategy, unit testing in python: unittest or pytest, faqs about python unit testing.
In this article, we’ll look at what software testing is, and why you should care about it. We’ll learn how to design unit tests and how to write Python unit tests. In particular, we’ll look at two of the most used unit testing frameworks in Python, unittest and pytest .
Software testing is the process of examining the behavior of a software product to evaluate and verify that it’s coherent with the specifications. Software products can have thousands of lines of code, and hundreds of components that work together. If a single line doesn’t work properly, the bug can propagate and cause other errors. So, to be sure that a program acts as it’s supposed to, it has to be tested.
Since modern software can be quite complicated, there are multiple levels of testing that evaluate different aspects of correctness. As stated by the ISTQB Certified Test Foundation Level syllabus , there are four levels of software testing:
- Unit testing , which tests specific lines of code
- Integration testing , which tests the integration between many units
- System testing , which tests the entire system
- Acceptance testing , which checks the compliance with business goals
In this article, we’ll talk about unit testing, but before we dig deep into that, I’d like to introduce an important principle in software testing.
Testing shows the presence of defects, not their absence. — ISTQB CTFL Syllabus 2018
In other words, even if all the tests you run don’t show any failure, this doesn’t prove that your software system is bug-free, or that another test case won’t find a defect in the behavior of your software.
What is Unit Testing?
This is the first level of testing, also called component testing . In this part, the single software components are tested. Depending on the programming language, the software unit might be a class, a function, or a method. For example, if you have a Java class called ArithmeticOperations that has multiply and divide methods, unit tests for the ArithmeticOperations class will need to test both the correct behavior of the multiply and divide methods.
Unit tests are usually performed by software testers. To run unit tests, software testers (or developers) need access to the source code, because the source code itself is the object under test. For this reason, this approach to software testing that tests the source code directly is called white-box testing .
You might be wondering why you should worry about software testing, and whether it’s worth it or not. In the next section, we’ll analyze the motivation behind testing your software system.
Why you should do unit testing
The main advantage of software testing is that it improves software quality. Software quality is crucial, especially in a world where software handles a wide variety of our everyday activities. Improving the quality of the software is still too vague a goal. Let’s try to specify better what we mean by quality of software. According to the ISO/IEC Standard 9126-1 ISO 9126 , software quality includes these factors:
- reliability
- functionality
- maintainability
- portability
If you own a company, software testing is an activity that you should consider carefully, because it can have an impact on your business. For example, in May 2022, Tesla recalled 130,000 cars due to an issue in vehicles’ infotainment systems. This issue was then fixed with a software update distributed “over the air”. These failures cost time and money to the company, and they also caused problems for the customers, because they couldn’t use their cars for a while. Testing software indeed costs money, but it’s also true that companies can save millions in technical support.
Unit testing focuses on checking whether or not the software is behaving correctly, which means checking that the mapping between the inputs and the outputs are all done correctly. Being a low-level testing activity, unit testing helps in the early identification of bugs so that they aren’t propagated to higher levels of the software system.
Other advantages of unit testing include:
- Simplifying integration : by ensuring that all the components work well individually, it’s easier to solve integration problems.
- Minimizing code regression : with a good amount of test cases, if some modifications to the source code in the future will cause problems, it’s easier to locate the issue.
- Providing documentation : by testing the correct mapping between input and output, unit tests provide documentation on how the method or class under test works.
Let’s now look at how to design a testing strategy.
Definition of test scope
Before starting to plan a test strategy, there’s an important question to answer. What parts of your software system do you want to test?
This is a crucial question, because exhaustive testing is impossible. For this reason, you can’t test every possible input and output, but you should prioritize your tests based on the risks involved.
Many factors need to be taken into account when defining your test scope:
- Risk : what business consequences would there be if a bug were to affect this component?
- Time : how soon do you want your software product to be ready? Do you have a deadline?
- Budget : how much money are you willing to invest in the testing activity?
Once you define the testing scope, which specifies what you should test and what you shouldn’t test, you’re ready to talk about the qualities that a good unit test should have.
Qualities of a unit test
- Fast . Unit tests are mostly executed automatically, which means they must be fast. Slow unit tests are more likely to be skipped by developers because they don’t provide instant feedback.
- Isolated . Unit tests are standalone by definition. They test the individual unit of code, and they don’t depend on anything external (like a file or a network resource).
- Repeatable . Unit tests are executed repeatedly, and the result must be consistent over time.
- Reliable . Unit tests will fail only if there’s a bug in the system under test. The environment or the order of execution of the tests shouldn’t matter.
- Named properly . The name of the test should provide relevant information about the test itself.
There’s one last step missing before diving deep into unit testing in Python. How do we organize our tests to make them clean and easy to read? We use a pattern called Arrange, Act and Assert (AAA).
The AAA pattern
The Arrange, Act and Assert pattern is a common strategy used to write and organize unit tests. It works in the following way:
- During the Arrange phase, all the objects and variables needed for the test are set.
- Next, during the Act phase, the function/method/class under test is called.
- In the end, during the Assert phase, we verify the outcome of the test.
This strategy provides a clean approach to organizing unit tests by separating all the main parts of a test: setup, execution and verification. Plus, unit tests are easier to read, because they all follow the same structure.
We’ll now talk about two different unit testing frameworks in Python. The two frameworks are unittest and pytest .
Introduction to unittest
The Python standard library includes the unittest unit testing framework. This framework is inspired by JUnit, which is a unit testing framework in Java.
As stated in the official documentation, unittest supports a few important concepts that we will mention in this article:
- test case , which is the single unit of testing
- test suite , which is a group of test cases that are executed together
- test runner , which is the component that will handle the execution and the result of all the test cases
unittest has its way to write tests. In particular, we need to:
- write our tests as methods of a class that subclasses unittest.TestCase
- use special assertion methods
Since unittest is already installed, we’re ready to write our first unit test!
Writing unit tests using unittest
Let’s say that we have the BankAccount class:
We can’t withdraw more money than the deposit availability, so let’s test that this scenario is handled correctly by our source code.
In the same Python file, we can add the following code:
We’re creating a class called TestBankOperations that’s a subclass of unittest.TestCase . In this way, we’re creating a new test case.
Inside this class, we define a single test function with a method that starts with test . This is important, because every test method must start with the word test .
We expect this test method to return False , which means that the operation failed. To assert the result, we use a special assertion method called assertFalse() .
We’re ready to execute the test. Let’s run this command on the command line:
Here, example.py is the name of the file containing all the source code. The output should look something like this:
Good! This means that our test was successful. Let’s see now how the output looks when there’s a failure. We add a new test to the previous class. Let’s try to deposit a negative amount of money, which of course isn’t possible. Will our code handle this scenario?
This is our new test method:
We can use the verbose mode of unittest to execute this test by putting the -v flag:
And the output is now different:
In this case, the verbose flag gives us more information. We know that the test_negative_deposit failed. In particular, the AssertionError tells us that the expected outcome was supposed to be false but True is not false , which means that the method returned True .
The unittest framework provides different assertion methods, based on our needs:
- assertEqual(x,y) , which tests whether x == y
- assertRaises(exception_type) , which checks if a specific exception is raised
- assertIsNone(x) , which tests if x is None
- assertIn(x,y) , which tests if x in y
Now that we have a basic understanding of how to write unit tests using the unittest framework, let’s have a look at the other Python framework called pytest .
Introduction to pytest
The pytest framework is a Python unit testing framework that has a few relevant features:
- it allows complex testing using less code
- it supports unittest test suites
- it offers more than 800 external plugins
Since pytest isn’t installed by default, we have to install it first. Note that pytest requires Python 3.7+ .
Installing pytest
Installing pytest is quite easy. You just have to run this command:
Then check that everything has been installed correctly by typing this:
The output should look something like this:
Good! Let’s write the first test using pytest .
Writing unit tests using pytest
We’ll use the BankAccount class written before, and we’ll test the same methods as before. In this way, it’s easier to compare the effort needed to write tests using the two frameworks.
To test with pytest we need to:
- Create a directory and put our test files inside it.
- Write our tests in files whose names start with test_ or end with _test.py . pytest will look for those files in the current directory and its subdirectories.
So, we create a file called test_bank.py and we put it into a folder. This is what our first test function looks like:
As you have noticed, the only thing that changed with respect to the unittest version is the assert section. Here we use plain Python assertion methods.
And now we can have a look at the test_bank.py file:
To run this test, let’s open a command prompt inside the folder where the test_bank.py file is located. Then, run this:
The output will be something like this:
In this case, we can see how easy it is to write and execute a test. Also, we can see that we wrote less code compared to unittest . The result of the test is also quite easy to understand.
Let’s move on to see a failed test!
We use the second method we wrote before, which is called test_negative_deposit . We refactor the assert section, and this is the result:
We run the test in the same way as before, and this should be the output:
By parsing the output, we can read collected 2 items , which means that two tests have been executed. Scrolling down, we can read that a failure occurred while testing the test_negative_deposit method. In particular, the error occurred when evaluating the assertion. Plus, the report also says that the value of the outcome variable is True , so this means that the deposit method contains an error.
Since pytest uses the default Python assertion keyword, we can compare any output we get with another variable that stores the expected outcome. All of this without using special assertion methods.
To wrap it up, in this article we covered the basics of software testing. We discovered why software testing is essential and why everyone should test their code. We talked about unit testing, and how to design and implement simple unit tests in Python.
We used two Python frameworks called unittest and pytest . Both have useful features, and they’re two of the most-used frameworks for Python unit testing.
In the end, we saw two basic test cases to give you an idea of how tests are written following the Arrange, Act and Assert pattern.
I hope I’ve convinced you of the importance of software testing. Choose a framework such as unittest or pytest , and start testing — because it’s worth the extra effort!
If you enjoyed this article, you might also find the following useful:
- Cypress Testing: A Guide to Running Web Application Tests
- How to Test React Components Using Jest
- Learn End-to-end Testing with Puppeteer
- 3 Methods for Hands-free Continuous Testing
- Re-Introducing Jenkins: Automated Testing with Pipelines
Unit testing in Python is a software testing technique where individual units or components of a program are tested in isolation to ensure that each unit works as intended.
Unit testing helps ensure the correctness of individual components in a Python program. It facilitates early detection of bugs, provides a safety net for code changes, and supports code maintainability.
Unit tests in Python are typically written using the built-in unittest module. You create test classes that inherit from unittest.TestCase and write test methods within these classes. Test methods usually start with the word “test.”
Yes, besides unittest , there are other popular testing frameworks for Python, such as pytest and nose2 . These frameworks offer different features and syntax, providing developers with flexibility in choosing the one that best fits their needs.
Fixtures are a way to set up preconditions and clean up after tests in Python. They help ensure that tests are isolated and can be run independently.
Test coverage measures the percentage of your codebase that is exercised by your tests. It helps identify untested code and ensures that your tests are comprehensive, reducing the likelihood of undiscovered bugs.
Yes, some best practices include writing independent and isolated tests, using descriptive test method names, and testing boundary cases. Additionally, strive for good test coverage and run tests frequently.
I am a computer science student fond of asking questions and learning new things. Traveller, musician and occasional writer. Check me out on my website .
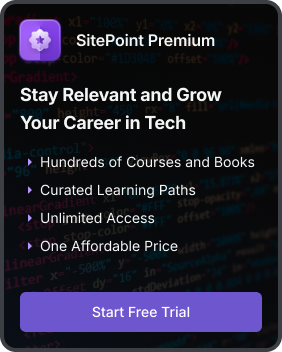
Testing Your Code ¶
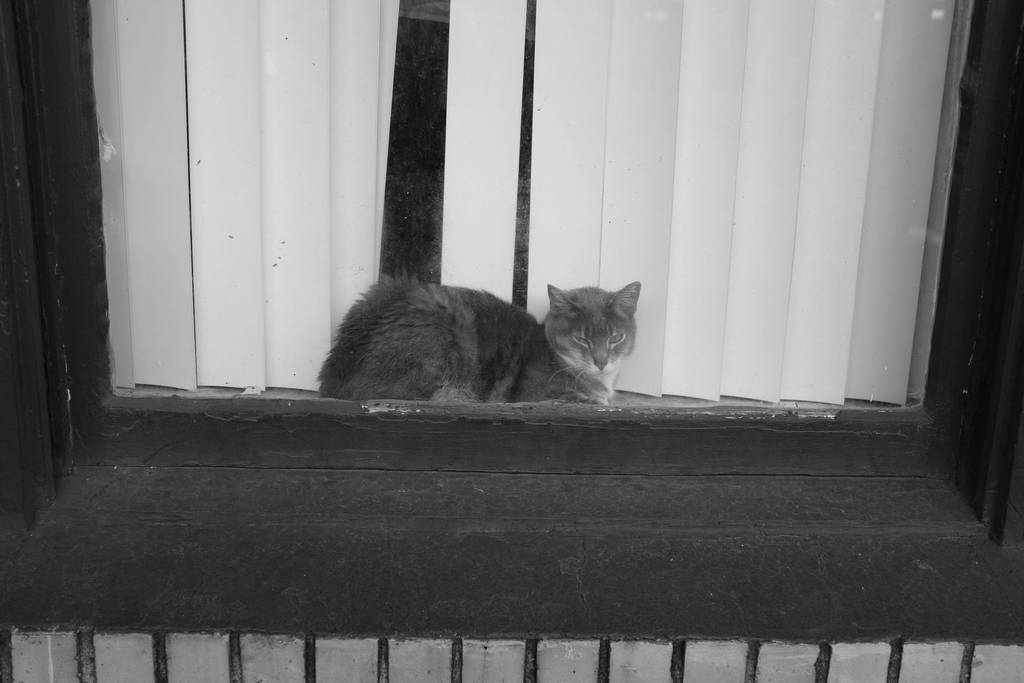
Testing your code is very important.
Getting used to writing testing code and running this code in parallel is now considered a good habit. Used wisely, this method helps to define your code’s intent more precisely and have a more decoupled architecture.
Some general rules of testing:
- A testing unit should focus on one tiny bit of functionality and prove it correct.
- Each test unit must be fully independent. Each test must be able to run alone, and also within the test suite, regardless of the order that they are called. The implication of this rule is that each test must be loaded with a fresh dataset and may have to do some cleanup afterwards. This is usually handled by setUp() and tearDown() methods.
- Try hard to make tests that run fast. If one single test needs more than a few milliseconds to run, development will be slowed down or the tests will not be run as often as is desirable. In some cases, tests can’t be fast because they need a complex data structure to work on, and this data structure must be loaded every time the test runs. Keep these heavier tests in a separate test suite that is run by some scheduled task, and run all other tests as often as needed.
- Learn your tools and learn how to run a single test or a test case. Then, when developing a function inside a module, run this function’s tests frequently, ideally automatically when you save the code.
- Always run the full test suite before a coding session, and run it again after. This will give you more confidence that you did not break anything in the rest of the code.
- It is a good idea to implement a hook that runs all tests before pushing code to a shared repository.
- If you are in the middle of a development session and have to interrupt your work, it is a good idea to write a broken unit test about what you want to develop next. When coming back to work, you will have a pointer to where you were and get back on track faster.
- The first step when you are debugging your code is to write a new test pinpointing the bug. While it is not always possible to do, those bug catching tests are among the most valuable pieces of code in your project.
- Use long and descriptive names for testing functions. The style guide here is slightly different than that of running code, where short names are often preferred. The reason is testing functions are never called explicitly. square() or even sqr() is ok in running code, but in testing code you would have names such as test_square_of_number_2() , test_square_negative_number() . These function names are displayed when a test fails, and should be as descriptive as possible.
- When something goes wrong or has to be changed, and if your code has a good set of tests, you or other maintainers will rely largely on the testing suite to fix the problem or modify a given behavior. Therefore the testing code will be read as much as or even more than the running code. A unit test whose purpose is unclear is not very helpful in this case.
- Another use of the testing code is as an introduction to new developers. When someone will have to work on the code base, running and reading the related testing code is often the best thing that they can do to start. They will or should discover the hot spots, where most difficulties arise, and the corner cases. If they have to add some functionality, the first step should be to add a test to ensure that the new functionality is not already a working path that has not been plugged into the interface.
The Basics ¶
unittest is the batteries-included test module in the Python standard library. Its API will be familiar to anyone who has used any of the JUnit/nUnit/CppUnit series of tools.
Creating test cases is accomplished by subclassing unittest.TestCase .
As of Python 2.7 unittest also includes its own test discovery mechanisms.
unittest in the standard library documentation
The doctest module searches for pieces of text that look like interactive Python sessions in docstrings, and then executes those sessions to verify that they work exactly as shown.
Doctests have a different use case than proper unit tests: they are usually less detailed and don’t catch special cases or obscure regression bugs. They are useful as an expressive documentation of the main use cases of a module and its components. However, doctests should run automatically each time the full test suite runs.
A simple doctest in a function:
When running this module from the command line as in python module.py , the doctests will run and complain if anything is not behaving as described in the docstrings.
py.test is a no-boilerplate alternative to Python’s standard unittest module.
Despite being a fully-featured and extensible test tool, it boasts a simple syntax. Creating a test suite is as easy as writing a module with a couple of functions:
and then running the py.test command:
is far less work than would be required for the equivalent functionality with the unittest module!
Hypothesis ¶
Hypothesis is a library which lets you write tests that are parameterized by a source of examples. It then generates simple and comprehensible examples that make your tests fail, letting you find more bugs with less work.
For example, testing lists of floats will try many examples, but report the minimal example of each bug (distinguished exception type and location):
Hypothesis is practical as well as very powerful and will often find bugs that escaped all other forms of testing. It integrates well with py.test, and has a strong focus on usability in both simple and advanced scenarios.
tox is a tool for automating test environment management and testing against multiple interpreter configurations.
tox allows you to configure complicated multi-parameter test matrices via a simple INI-style configuration file.
unittest.mock is a library for testing in Python. As of Python 3.3, it is available in the standard library .
For older versions of Python:
It allows you to replace parts of your system under test with mock objects and make assertions about how they have been used.
For example, you can monkey-patch a method:
To mock classes or objects in a module under test, use the patch decorator. In the example below, an external search system is replaced with a mock that always returns the same result (but only for the duration of the test).
Mock has many other ways with which you can configure and control its behaviour.
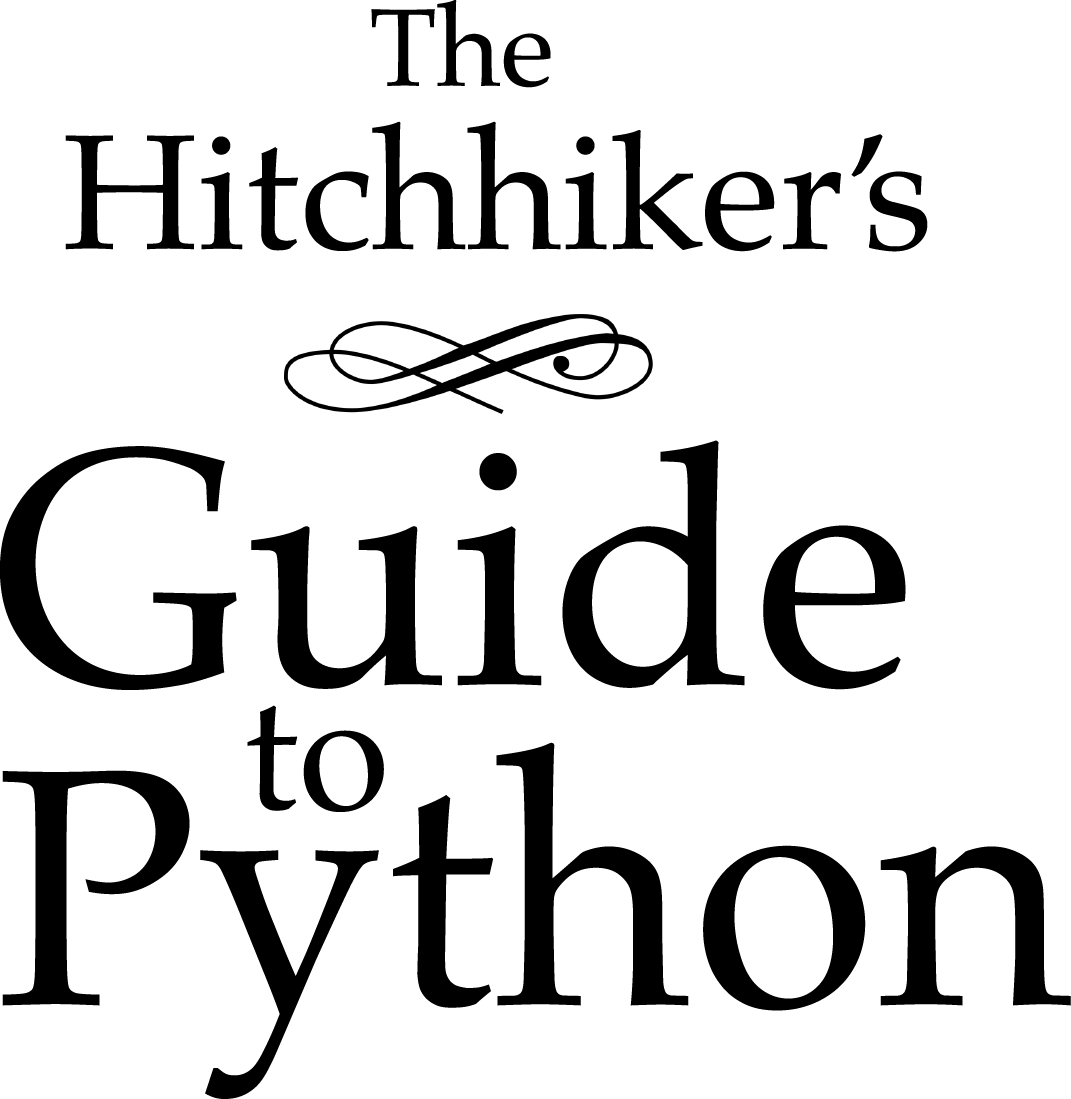
This opinionated guide exists to provide both novice and expert Python developers a best practice handbook to the installation, configuration, and usage of Python on a daily basis.
O'Reilly Book
This guide is now available in tangible book form!
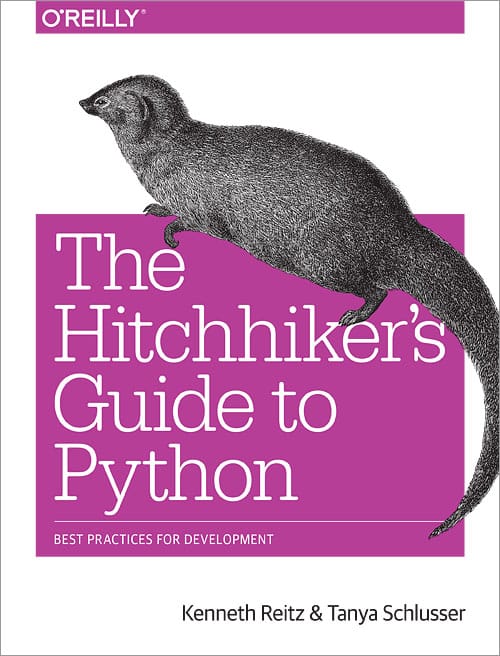
All proceeds are being directly donated to the DjangoGirls organization.
Translations
- Brazilian Portuguese
Table Of Contents
Related topics.
- Previous: Documentation
- Next: Logging
Quick search
©2011-2023 Kenneth Reitz & Real Python . CC BY-NC-SA 3.0
How to Write Unit Tests for Instance Methods in Python
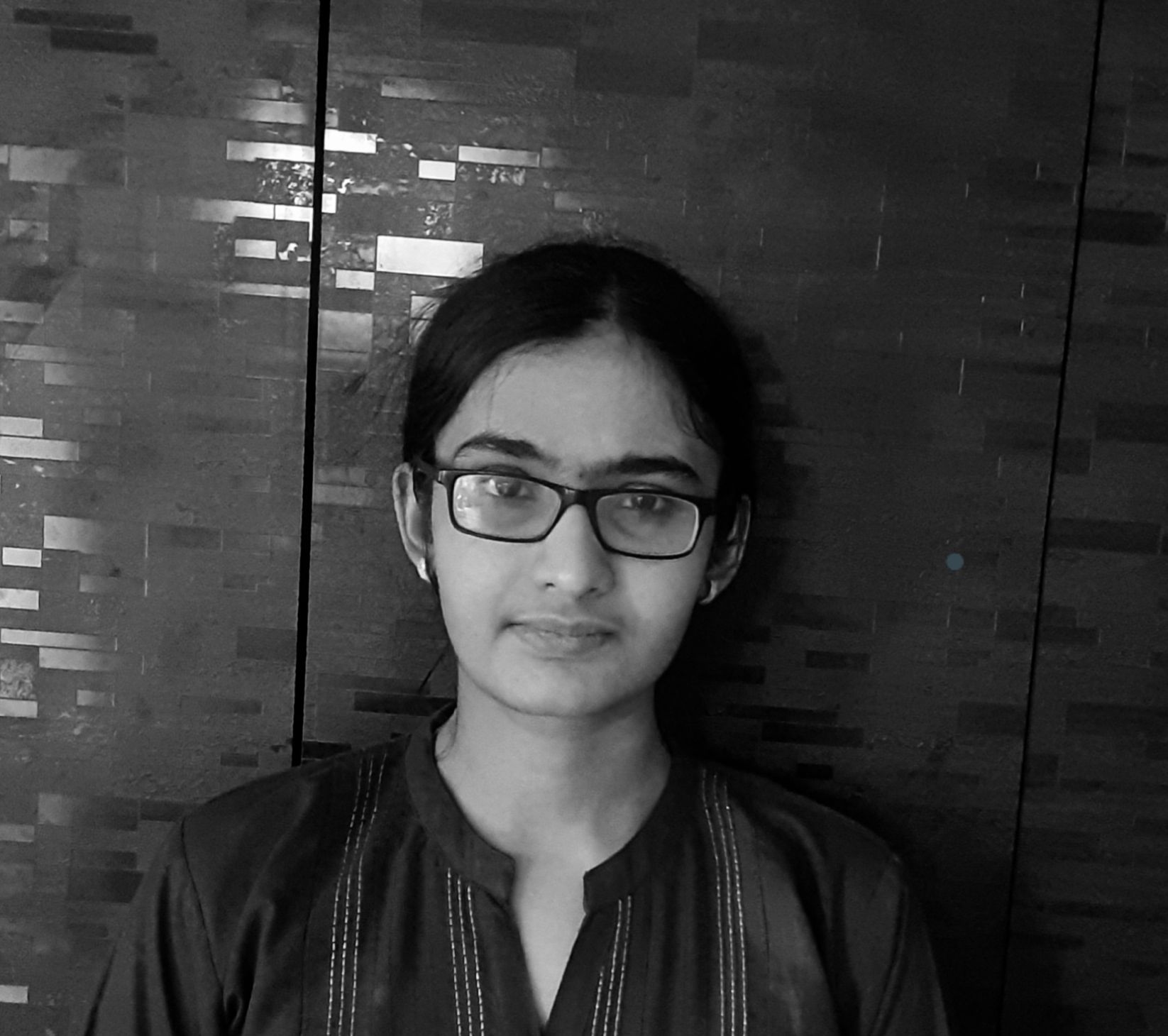
This tutorial will teach you how to write unit tests for instance methods in Python.
In a previous tutorial , we covered how to write unit tests for Python functions. While unit testing for instance methods works similarly, you may have some challenges creating and managing objects (instances of a class).
This tutorial will teach you how to use such methods for setting up and tearing down resources efficiently.
Let's begin!
Classes and Objects in Python – A Quick Review
If you're familiar with object-oriented programming, you know that classes let you group related data and behavior together. You can then use these classes as templates to create instances of the class. If a Python class is a cookie-cutter, then each instance is a cookie. 🍪
The data and behavior are described by the attributes and methods in the definition of the class, respectively. We'll look at an example to understad these better.
I know this section should have been named classes and objects explained for the impatient. 🙂 To brush up your Python OOP skills, you may check out this course on freeCodeCamp's YouTube channel.
How to Test the Instance Methods of a Python Class
Now, we'll learn how to set up unit tests for instances of Python classes. We'll write unit tests to check the functionality of the Book class shown below:
The Book class serves as a template or a blueprint with attributes: title, author, pages, price, and discount. get_reading_time() and apply_discount() are the instance methods that use the above attributes.
So we can create book objects from the Book class, each with their own attributes.
To test the instance methods get_reading_time() and apply_discount() , we can create two instances of the Book class inside the test methods. We can use the assertion method assertEqual() to check if the return values of the instance methods are correct.
How to Set Up and Tear Down Resources During Unit Tests
When setting up tests for instance methods, we instantiate two book objects, then verify if the instance methods work as expected. And we need to do this for each of the methods.
But this is repetitive and suboptimal when you need to test a large number of instance methods.
In this case, it'll be more convenient if we can define a method that instantiates these objects for us before running each test. This is where the setUp() method comes into play.
How the setUp() and tearDown() Methods Work
The setUp() and tearDown() methods are typically used for the complementary tasks of allocating and deallocating resources, before and after every unit test, respectively.
- The setUp() method runs before every test, and
- The tearDown() method runs after every test.
Here, we can use the setUp() method to instantiate the book objects. Sometimes, you'll need to use the tearDown() method as well.
For example, if each test adds files to a directory or creates multiple objects in memory, you may want to free up the directory and delete the created objects after each test. We'll add the tearDown() method to verify that it runs after each test.
To understand this better, let's add explanatory print() statements, as shown in the code below:
Now, run the test_book module. Here's the output:
How to Use the setUpClass() and tearDownClass() Methods
In addition to the above methods, you can also use the class methods: setUpClass() and tearDownClass() .
In Python, class methods bind to a class and not to a particular instance. To define a method as a class method, you can use the @classmethod decorator.
So when should we use these class methods?
Instantiating objects, as in the above example, is a simple task and not computationally expensive. But you may sometimes have tasks that are too expensive to be performed before every test: for instance, spinning up an in-memory database.
If all subsequent tests in the test class only read in some data from the database, we can use the setUpClass() class method to spin up the database and the tearDownClass() class method to tear down the database after all tests have run.
Putting it all together:
- The setUpClass() class method is executed before any of the tests are run.
- The tearDownClass() class method is executed after all the tests have run.
- The setUp() and tearDown() methods are executed before and after each test, respectively.
Let’s add the setUpClass() and tearDownClass() class methods to the TestBook class.
Now, rerun test_book.py .
From the output below, we see that the setUpClass() and tearDownClass() methods run before and after all the tests, respectively.
I hope this tutorial helped you learn how to set up unit tests for instance methods in Python.
If you're interested in learning more about the need for unit testing with a focus on doing it for Python functions, consider reading the article How to Write Unit Tests for Python Functions .
Happy coding!
I am a developer and technical writer from India. I write tutorials on all things programming and machine learning.
If you read this far, thank the author to show them you care. Say Thanks
Learn to code for free. freeCodeCamp's open source curriculum has helped more than 40,000 people get jobs as developers. Get started
- Python Basics
- Interview Questions
- Python Quiz
- Popular Packages
- Python Projects
- Practice Python
- AI With Python
- Learn Python3
- Python Automation
- Python Web Dev
- DSA with Python
- Python OOPs
- Dictionaries
- Getting Started With Testing in Python
- Getting Started with Python Programming
- Getting started with Python for Automated Trading
- Automated software testing with Python
- Unit Testing in Python - Unittest
- Unit Testing Jinja Templates with Flask
- Python | Unit Test Objects Patching | Set-1
- Python | Unit Test Objects Patching | Set-2
- Python | Testing Output to stdout
- Getting Started with Competitive Programming in Python
- How to split data into training and testing in Python without sklearn
- Welch's t-Test in Python
- Grouping the Tests in Pytest
- Test Internet Speed using Python
- Python | Exceptional Conditions Testing in Unit Tests
- Automated Browser Testing with Edge and Selenium in Python
- Thread Testing in Software Engineering
- Why is Unit Testing Harder in OOP?
- How to write Test Cases - Software Testing
- Unit Testing in R Programming
Getting Started With Unit Testing in Python
In Python, unit tests are the segments of codes that are written to test other modules and files that we refer to as a unit. Python Unit Testing is a very important part of the software development process that helps us to ensure that the code works properly without any errors. In this article, we will learn about Python Testing with the help of examples and proper explanations.
Table of Content
What is Python Unit Testing?
The assert statement.
- Implementing Unit Tests | Unittest
Implementing Unit Tests | Pytest
Implementing unit tests | nose, implementing unit tests | doctest, how to write assertions in python testing, more advanced testing scenarios, automating the execution of tests.
Python Unit testing is a software testing technique where individual units or components of a software application are tested in isolation from the rest of the system. The primary goal of Python unit testing is checking the validation that each unit of the software performs as designed, according to its specifications. Each unit test looks at a small part of the code, making sure it works even when things get a little tricky.
By testing each piece alone, programmers can find and fix mistakes early on, just like finding a little hole in a sock before it gets bigger, also developers can identify and fix bugs early in the development cycle, leading to better code quality and more robust software.
The main idea with unit testing is to make sure the code works well and won’t cause problems later on. So, by doing these tests as they’re building the program, developers can make sure it’s strong and won’t break when people use it.
Types of Python Testing Frameworks
Python has two main frameworks to make unit testing easier:
- Nose or nose2
Before diving to the testing framework, Let’s understand the assert statement, It is commonly used in Python unit testing frameworks like unittest and pytest to verify the correctness of code during testing. The assert statement in Python is used to check whether a given condition is true or false. It’s primarily used for debugging and testing purposes to verify assumptions made by the programmer during the development of the code.
Syntax of the assert statement
Here’s the syntax of the assert statement in Python :
- condition : The expression to be evaluated. If it evaluates to True, the program continues execution normally. If it evaluates to False, an AssertionError exception is raised.
- message (optional): An optional message that can be provided to provide additional context when the assertion fails. This message is displayed along with the traceback when an assertion fails.
Implementing Unit Tests | unittest
The unittest is built into the standard Python library used for testing with Python. import unittest should be the starting line of code for using it. Depends upon the python version, it should differ as later versions of Python supports unittest and earlier versions supported unittest2.
In this example, a unit test class that checks if the sum of a list of numbers matches an expected value using the unittest framework in Python.
Pytest is the built-in library provided by Python for testing in Python. It simplifies the process of writing and executing tests. Pytest supports unittest test cases execution. It has benefits like supporting built in assert statement, filtering of test cases, returning from last failing test etc.
We can install Pytest by writing the following command in the command prompt:
Let’s start with a simple example. Here, we will make a file named as math_functions.py.
Now, we will make another file named as test_math_functions.py . We are making this file to perform testing using pytest using sample inputs.
To run these tests using pytest, navigate to the directory containing these files in your terminal and run:
.png)
“Nose” is a popular testing framework in Python, primarily used for writing unit tests. Nose is a test runner that extends the capabilities of Python’s built-in unittest module. It provides a simpler syntax for writing tests and offers additional features for test discovery, parallel test execution, and plugin support.
Installing Nose
Now, to perform unit tests using nose, we will make a file named as main.py and write the following test code.
To run this program, we will write the following command:

Python Unit Testing Using nose
Doctest Module is used to write tests in the form of interactive Python sessions within docstrings and then automatically run those tests to verify that the code behaves as expected. Doctest Module finds patterns in the docstring that looks like interactive shell commands.
Now, we will create a file named as main.py and we will create a test code using docstring.
To run the program, write the following command in your terminal in the same directory as main.py file.
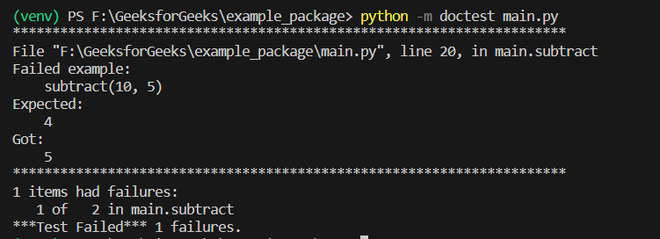
Python Unit Testing Using doctest
Assertion is nothing but validating the output against a known response. i.e. in above code, we have passed the list containing 3 values namely 10, 20 and 30, and we know that its multiplication result is 6000. So as a last step in code, we will be writing assertion code and above code comes up with assertEqual and surely it will give the output as 6000 and hence the testcase passes.
unittest has lots of methods to assert on the values, types, and existence of variables. Below are some of the methods that are commonly used to write assertions.
- Fixture is used which is nothing but the data that is created as an input and reusing them.
- Parameterization can be followed which is nothing but running the same test several times by passing different values and expecting the same result.
- Need to cover different scenarios like handling expected failures, writing integration tests, testing in multiple environments etc.
Below example shows how to write test for bad data type:
Continuous Integration/Continuous Deployment tools are available. They help to run tests, compile, publish and also deploy into production. https://travis-ci.com/ is one among them which works well with Python and it is an open source project. Login into the site and create. travis.yml with the below contents:
language: python python: <Include the versions as 2.7 or 3.7 whichever required> install: – pip install -r <your requirements file> script: – python -m unittest discover
Above file instructs “Travis CI” to look into this file and for given Python versions, the test cases are getting tested by installing the necessary packages as given in the requirements file and finally run below command to run the test.
Results are on the website against your credential.
Please Login to comment...
Similar reads.
- python-utility
Improve your Coding Skills with Practice
What kind of Experience do you want to share?

IMAGES
VIDEO
COMMENTS
The Python standard library ships with a testing framework named unittest, which you can use to write automated tests for your code.The unittest package has an object-oriented approach where test cases derive from a base class, which has several useful methods.. The framework supports many features that will help you write consistent unit tests for your code.
In this article, we'll cover the writing of unit tests in Python, from understanding the assert statement to using a framework designed specifically for this kind of task — and following Python unit testing best practices. Python has two main frameworks to make unit testing easier: unittest and PyTest. The first one has been part of Python's ...
You will write your unit tests in a file called test_user.py. The common naming convention for unit tests: the name of the file used for unit tests simply prepends "test_" to the .py file where the Python code to be tested is. To use the unittest framework we have to do the following: import the unittest module.
To focus on how to set up unit tests, we'll consider a simple function is_prime(), that takes in a number and checks whether or not it is prime. import math. def is_prime(num): '''Check if num is prime or not.''' for i in range(2,int(math.sqrt(num))+1): if num%i==0: return False return True. Let's start a Python REPL, call the function is ...
Here are some tips for writing good unit tests in Python: Keep tests atomic and independent: Each test should test a single unit of code in isolation and should not depend on the state of any ...
Tests can be written using functions or classes, providing flexibility. This tutorial will guide you through writing unit tests with Pytest. Prerequisites. Before proceeding with this tutorial, ensure that you have a recent version of Python installed, and a basic understanding of writing Python programs. Step 1 — Setting up the project directory
First you need to create a test file. Then import the unittest module, define the testing class that inherits from unittest.TestCase, and lastly, write a series of methods to test all the cases of your function's behavior. There's a line by line explanation below the following code: import unittest.
class unittest.TextTestRunner(stream=None, descriptions=True, verbosity=1, failfast=False, buffer=False, resultclass=None, warnings=None, *, tb_locals=False, durations=None) ¶. A basic test runner implementation that outputs results to a stream. If stream is None, the default, sys.stderr is used as the output stream.
Python Unit Testing. Though writing manual tests for your code is definitely a tedious and time-consuming task, Python's built-in unit testing framework has made life a lot easier. ... One good exercise for you would be to write a unit test module for any of your past projects. This will give you a good hands-on experience with writing unit ...
Is Python Good for Unit Testing? Python is well-suited for unit testing. Python provides several built-in libraries and frameworks, such as unittest, doctest, and PyTest, that make it easy to write and execute unit tests. These tools offer robust features and functionalities to streamline the testing process. 1.
The main test type that you'll come across is the unit test. This is a test of a specific unit or component — most commonly a function. Integration testing is testing how all these components fit together. The following examples will focus on unit testing. So, let's start with a basic example of a standalone unit test.
Let's see how this Python testing framework we've been going on about works. The first step is installing the package, which can be done with a simple pip command. Note: The creators of pytest recommend you use venv for development and pip for installing your application, dependencies, and pytest itself.
A Gentle Introduction to Unit Testing in Python. Unit testing is a method for testing software that looks at the smallest testable pieces of code, called units, which are tested for correct operation. By doing unit testing, we can verify that each part of the code, including helper functions that may not be exposed to the user, works correctly ...
To test with pytest we need to: Create a directory and put our test files inside it. Write our tests in files whose names start with test_ or end with _test.py. pytest will look for those files in ...
As a general rule test files are stored in a directory called "tests" inside the project directory. Now, let's create a python file called "test_customer.py". When naming your test files ...
Despite being a fully-featured and extensible test tool, it boasts a simple syntax. Creating a test suite is as easy as writing a module with a couple of functions: # content of test_sample.py def func(x): return x + 1 def test_answer(): assert func(3) == 5. and then running the py.test command: $ py.test.
74. The free Python book Dive Into Python has a chapter on unit testing that you might find useful. If you follow modern practices you should probably write the tests while you are writing your project, and not wait until your project is nearly finished. Bit late now, but now you know for next time. :)
How to Test the Instance Methods of a Python Class. Now, we'll learn how to set up unit tests for instances of Python classes. We'll write unit tests to check the functionality of the Book class shown below: class Book: def __init__(self,title,author,pages,price,discount): self.title = title.
pip install nose. Now, to perform unit tests using nose, we will make a file named as main.py and write the following test code. main.py. Python. def test_addition(): assert 1 + 1 == 2 def test_subtraction(): assert 3 - 1 == 2. To run this program, we will write the following command: nosetests main.py. Output:
Perhaps the issue isn't in how best to write test docstrings, but how to write the tests themselves? Refactoring tests in such a way that they're self documenting can go a long way, and your docstring won't go stale when the code changes. There's a few things you can do to make the tests clearer:
With doctest, you can write code that provides examples of how your code works, simulating REPL sessions. These examples work as documentation and also as test cases or unit tests. Each doctest test needs a statement or expression and an expected result.. You can use ChatGPT to help you out with writing doctest tests. First, you need some code to test.
A unit test should: test one method. provide some specific arguments to that method. test that the result is as expected. It should not look inside the method to see what it is doing, so changing the internals should not cause the test to fail. You should not directly test that private methods are being called.