Jump to navigation


Cochrane Training
Chapter 15: interpreting results and drawing conclusions.
Holger J Schünemann, Gunn E Vist, Julian PT Higgins, Nancy Santesso, Jonathan J Deeks, Paul Glasziou, Elie A Akl, Gordon H Guyatt; on behalf of the Cochrane GRADEing Methods Group
Key Points:
- This chapter provides guidance on interpreting the results of synthesis in order to communicate the conclusions of the review effectively.
- Methods are presented for computing, presenting and interpreting relative and absolute effects for dichotomous outcome data, including the number needed to treat (NNT).
- For continuous outcome measures, review authors can present summary results for studies using natural units of measurement or as minimal important differences when all studies use the same scale. When studies measure the same construct but with different scales, review authors will need to find a way to interpret the standardized mean difference, or to use an alternative effect measure for the meta-analysis such as the ratio of means.
- Review authors should not describe results as ‘statistically significant’, ‘not statistically significant’ or ‘non-significant’ or unduly rely on thresholds for P values, but report the confidence interval together with the exact P value.
- Review authors should not make recommendations about healthcare decisions, but they can – after describing the certainty of evidence and the balance of benefits and harms – highlight different actions that might be consistent with particular patterns of values and preferences and other factors that determine a decision such as cost.
Cite this chapter as: Schünemann HJ, Vist GE, Higgins JPT, Santesso N, Deeks JJ, Glasziou P, Akl EA, Guyatt GH. Chapter 15: Interpreting results and drawing conclusions. In: Higgins JPT, Thomas J, Chandler J, Cumpston M, Li T, Page MJ, Welch VA (editors). Cochrane Handbook for Systematic Reviews of Interventions version 6.4 (updated August 2023). Cochrane, 2023. Available from www.training.cochrane.org/handbook .
15.1 Introduction
The purpose of Cochrane Reviews is to facilitate healthcare decisions by patients and the general public, clinicians, guideline developers, administrators and policy makers. They also inform future research. A clear statement of findings, a considered discussion and a clear presentation of the authors’ conclusions are, therefore, important parts of the review. In particular, the following issues can help people make better informed decisions and increase the usability of Cochrane Reviews:
- information on all important outcomes, including adverse outcomes;
- the certainty of the evidence for each of these outcomes, as it applies to specific populations and specific interventions; and
- clarification of the manner in which particular values and preferences may bear on the desirable and undesirable consequences of the intervention.
A ‘Summary of findings’ table, described in Chapter 14 , Section 14.1 , provides key pieces of information about health benefits and harms in a quick and accessible format. It is highly desirable that review authors include a ‘Summary of findings’ table in Cochrane Reviews alongside a sufficient description of the studies and meta-analyses to support its contents. This description includes the rating of the certainty of evidence, also called the quality of the evidence or confidence in the estimates of the effects, which is expected in all Cochrane Reviews.
‘Summary of findings’ tables are usually supported by full evidence profiles which include the detailed ratings of the evidence (Guyatt et al 2011a, Guyatt et al 2013a, Guyatt et al 2013b, Santesso et al 2016). The Discussion section of the text of the review provides space to reflect and consider the implications of these aspects of the review’s findings. Cochrane Reviews include five standard subheadings to ensure the Discussion section places the review in an appropriate context: ‘Summary of main results (benefits and harms)’; ‘Potential biases in the review process’; ‘Overall completeness and applicability of evidence’; ‘Certainty of the evidence’; and ‘Agreements and disagreements with other studies or reviews’. Following the Discussion, the Authors’ conclusions section is divided into two standard subsections: ‘Implications for practice’ and ‘Implications for research’. The assessment of the certainty of evidence facilitates a structured description of the implications for practice and research.
Because Cochrane Reviews have an international audience, the Discussion and Authors’ conclusions should, so far as possible, assume a broad international perspective and provide guidance for how the results could be applied in different settings, rather than being restricted to specific national or local circumstances. Cultural differences and economic differences may both play an important role in determining the best course of action based on the results of a Cochrane Review. Furthermore, individuals within societies have widely varying values and preferences regarding health states, and use of societal resources to achieve particular health states. For all these reasons, and because information that goes beyond that included in a Cochrane Review is required to make fully informed decisions, different people will often make different decisions based on the same evidence presented in a review.
Thus, review authors should avoid specific recommendations that inevitably depend on assumptions about available resources, values and preferences, and other factors such as equity considerations, feasibility and acceptability of an intervention. The purpose of the review should be to present information and aid interpretation rather than to offer recommendations. The discussion and conclusions should help people understand the implications of the evidence in relation to practical decisions and apply the results to their specific situation. Review authors can aid this understanding of the implications by laying out different scenarios that describe certain value structures.
In this chapter, we address first one of the key aspects of interpreting findings that is also fundamental in completing a ‘Summary of findings’ table: the certainty of evidence related to each of the outcomes. We then provide a more detailed consideration of issues around applicability and around interpretation of numerical results, and provide suggestions for presenting authors’ conclusions.
15.2 Issues of indirectness and applicability
15.2.1 the role of the review author.
“A leap of faith is always required when applying any study findings to the population at large” or to a specific person. “In making that jump, one must always strike a balance between making justifiable broad generalizations and being too conservative in one’s conclusions” (Friedman et al 1985). In addition to issues about risk of bias and other domains determining the certainty of evidence, this leap of faith is related to how well the identified body of evidence matches the posed PICO ( Population, Intervention, Comparator(s) and Outcome ) question. As to the population, no individual can be entirely matched to the population included in research studies. At the time of decision, there will always be differences between the study population and the person or population to whom the evidence is applied; sometimes these differences are slight, sometimes large.
The terms applicability, generalizability, external validity and transferability are related, sometimes used interchangeably and have in common that they lack a clear and consistent definition in the classic epidemiological literature (Schünemann et al 2013). However, all of the terms describe one overarching theme: whether or not available research evidence can be directly used to answer the health and healthcare question at hand, ideally supported by a judgement about the degree of confidence in this use (Schünemann et al 2013). GRADE’s certainty domains include a judgement about ‘indirectness’ to describe all of these aspects including the concept of direct versus indirect comparisons of different interventions (Atkins et al 2004, Guyatt et al 2008, Guyatt et al 2011b).
To address adequately the extent to which a review is relevant for the purpose to which it is being put, there are certain things the review author must do, and certain things the user of the review must do to assess the degree of indirectness. Cochrane and the GRADE Working Group suggest using a very structured framework to address indirectness. We discuss here and in Chapter 14 what the review author can do to help the user. Cochrane Review authors must be extremely clear on the population, intervention and outcomes that they intend to address. Chapter 14, Section 14.1.2 , also emphasizes a crucial step: the specification of all patient-important outcomes relevant to the intervention strategies under comparison.
In considering whether the effect of an intervention applies equally to all participants, and whether different variations on the intervention have similar effects, review authors need to make a priori hypotheses about possible effect modifiers, and then examine those hypotheses (see Chapter 10, Section 10.10 and Section 10.11 ). If they find apparent subgroup effects, they must ultimately decide whether or not these effects are credible (Sun et al 2012). Differences between subgroups, particularly those that correspond to differences between studies, should be interpreted cautiously. Some chance variation between subgroups is inevitable so, unless there is good reason to believe that there is an interaction, review authors should not assume that the subgroup effect exists. If, despite due caution, review authors judge subgroup effects in terms of relative effect estimates as credible (i.e. the effects differ credibly), they should conduct separate meta-analyses for the relevant subgroups, and produce separate ‘Summary of findings’ tables for those subgroups.
The user of the review will be challenged with ‘individualization’ of the findings, whether they seek to apply the findings to an individual patient or a policy decision in a specific context. For example, even if relative effects are similar across subgroups, absolute effects will differ according to baseline risk. Review authors can help provide this information by identifying identifiable groups of people with varying baseline risks in the ‘Summary of findings’ tables, as discussed in Chapter 14, Section 14.1.3 . Users can then identify their specific case or population as belonging to a particular risk group, if relevant, and assess their likely magnitude of benefit or harm accordingly. A description of the identifying prognostic or baseline risk factors in a brief scenario (e.g. age or gender) will help users of a review further.
Another decision users must make is whether their individual case or population of interest is so different from those included in the studies that they cannot use the results of the systematic review and meta-analysis at all. Rather than rigidly applying the inclusion and exclusion criteria of studies, it is better to ask whether or not there are compelling reasons why the evidence should not be applied to a particular patient. Review authors can sometimes help decision makers by identifying important variation where divergence might limit the applicability of results (Rothwell 2005, Schünemann et al 2006, Guyatt et al 2011b, Schünemann et al 2013), including biologic and cultural variation, and variation in adherence to an intervention.
In addressing these issues, review authors cannot be aware of, or address, the myriad of differences in circumstances around the world. They can, however, address differences of known importance to many people and, importantly, they should avoid assuming that other people’s circumstances are the same as their own in discussing the results and drawing conclusions.
15.2.2 Biological variation
Issues of biological variation that may affect the applicability of a result to a reader or population include divergence in pathophysiology (e.g. biological differences between women and men that may affect responsiveness to an intervention) and divergence in a causative agent (e.g. for infectious diseases such as malaria, which may be caused by several different parasites). The discussion of the results in the review should make clear whether the included studies addressed all or only some of these groups, and whether any important subgroup effects were found.
15.2.3 Variation in context
Some interventions, particularly non-pharmacological interventions, may work in some contexts but not in others; the situation has been described as program by context interaction (Hawe et al 2004). Contextual factors might pertain to the host organization in which an intervention is offered, such as the expertise, experience and morale of the staff expected to carry out the intervention, the competing priorities for the clinician’s or staff’s attention, the local resources such as service and facilities made available to the program and the status or importance given to the program by the host organization. Broader context issues might include aspects of the system within which the host organization operates, such as the fee or payment structure for healthcare providers and the local insurance system. Some interventions, in particular complex interventions (see Chapter 17 ), can be only partially implemented in some contexts, and this requires judgements about indirectness of the intervention and its components for readers in that context (Schünemann 2013).
Contextual factors may also pertain to the characteristics of the target group or population, such as cultural and linguistic diversity, socio-economic position, rural/urban setting. These factors may mean that a particular style of care or relationship evolves between service providers and consumers that may or may not match the values and technology of the program.
For many years these aspects have been acknowledged when decision makers have argued that results of evidence reviews from other countries do not apply in their own country or setting. Whilst some programmes/interventions have been successfully transferred from one context to another, others have not (Resnicow et al 1993, Lumley et al 2004, Coleman et al 2015). Review authors should be cautious when making generalizations from one context to another. They should report on the presence (or otherwise) of context-related information in intervention studies, where this information is available.
15.2.4 Variation in adherence
Variation in the adherence of the recipients and providers of care can limit the certainty in the applicability of results. Predictable differences in adherence can be due to divergence in how recipients of care perceive the intervention (e.g. the importance of side effects), economic conditions or attitudes that make some forms of care inaccessible in some settings, such as in low-income countries (Dans et al 2007). It should not be assumed that high levels of adherence in closely monitored randomized trials will translate into similar levels of adherence in normal practice.
15.2.5 Variation in values and preferences
Decisions about healthcare management strategies and options involve trading off health benefits and harms. The right choice may differ for people with different values and preferences (i.e. the importance people place on the outcomes and interventions), and it is important that decision makers ensure that decisions are consistent with a patient or population’s values and preferences. The importance placed on outcomes, together with other factors, will influence whether the recipients of care will or will not accept an option that is offered (Alonso-Coello et al 2016) and, thus, can be one factor influencing adherence. In Section 15.6 , we describe how the review author can help this process and the limits of supporting decision making based on intervention reviews.
15.3 Interpreting results of statistical analyses
15.3.1 confidence intervals.
Results for both individual studies and meta-analyses are reported with a point estimate together with an associated confidence interval. For example, ‘The odds ratio was 0.75 with a 95% confidence interval of 0.70 to 0.80’. The point estimate (0.75) is the best estimate of the magnitude and direction of the experimental intervention’s effect compared with the comparator intervention. The confidence interval describes the uncertainty inherent in any estimate, and describes a range of values within which we can be reasonably sure that the true effect actually lies. If the confidence interval is relatively narrow (e.g. 0.70 to 0.80), the effect size is known precisely. If the interval is wider (e.g. 0.60 to 0.93) the uncertainty is greater, although there may still be enough precision to make decisions about the utility of the intervention. Intervals that are very wide (e.g. 0.50 to 1.10) indicate that we have little knowledge about the effect and this imprecision affects our certainty in the evidence, and that further information would be needed before we could draw a more certain conclusion.
A 95% confidence interval is often interpreted as indicating a range within which we can be 95% certain that the true effect lies. This statement is a loose interpretation, but is useful as a rough guide. The strictly correct interpretation of a confidence interval is based on the hypothetical notion of considering the results that would be obtained if the study were repeated many times. If a study were repeated infinitely often, and on each occasion a 95% confidence interval calculated, then 95% of these intervals would contain the true effect (see Section 15.3.3 for further explanation).
The width of the confidence interval for an individual study depends to a large extent on the sample size. Larger studies tend to give more precise estimates of effects (and hence have narrower confidence intervals) than smaller studies. For continuous outcomes, precision depends also on the variability in the outcome measurements (i.e. how widely individual results vary between people in the study, measured as the standard deviation); for dichotomous outcomes it depends on the risk of the event (more frequent events allow more precision, and narrower confidence intervals), and for time-to-event outcomes it also depends on the number of events observed. All these quantities are used in computation of the standard errors of effect estimates from which the confidence interval is derived.
The width of a confidence interval for a meta-analysis depends on the precision of the individual study estimates and on the number of studies combined. In addition, for random-effects models, precision will decrease with increasing heterogeneity and confidence intervals will widen correspondingly (see Chapter 10, Section 10.10.4 ). As more studies are added to a meta-analysis the width of the confidence interval usually decreases. However, if the additional studies increase the heterogeneity in the meta-analysis and a random-effects model is used, it is possible that the confidence interval width will increase.
Confidence intervals and point estimates have different interpretations in fixed-effect and random-effects models. While the fixed-effect estimate and its confidence interval address the question ‘what is the best (single) estimate of the effect?’, the random-effects estimate assumes there to be a distribution of effects, and the estimate and its confidence interval address the question ‘what is the best estimate of the average effect?’ A confidence interval may be reported for any level of confidence (although they are most commonly reported for 95%, and sometimes 90% or 99%). For example, the odds ratio of 0.80 could be reported with an 80% confidence interval of 0.73 to 0.88; a 90% interval of 0.72 to 0.89; and a 95% interval of 0.70 to 0.92. As the confidence level increases, the confidence interval widens.
There is logical correspondence between the confidence interval and the P value (see Section 15.3.3 ). The 95% confidence interval for an effect will exclude the null value (such as an odds ratio of 1.0 or a risk difference of 0) if and only if the test of significance yields a P value of less than 0.05. If the P value is exactly 0.05, then either the upper or lower limit of the 95% confidence interval will be at the null value. Similarly, the 99% confidence interval will exclude the null if and only if the test of significance yields a P value of less than 0.01.
Together, the point estimate and confidence interval provide information to assess the effects of the intervention on the outcome. For example, suppose that we are evaluating an intervention that reduces the risk of an event and we decide that it would be useful only if it reduced the risk of an event from 30% by at least 5 percentage points to 25% (these values will depend on the specific clinical scenario and outcomes, including the anticipated harms). If the meta-analysis yielded an effect estimate of a reduction of 10 percentage points with a tight 95% confidence interval, say, from 7% to 13%, we would be able to conclude that the intervention was useful since both the point estimate and the entire range of the interval exceed our criterion of a reduction of 5% for net health benefit. However, if the meta-analysis reported the same risk reduction of 10% but with a wider interval, say, from 2% to 18%, although we would still conclude that our best estimate of the intervention effect is that it provides net benefit, we could not be so confident as we still entertain the possibility that the effect could be between 2% and 5%. If the confidence interval was wider still, and included the null value of a difference of 0%, we would still consider the possibility that the intervention has no effect on the outcome whatsoever, and would need to be even more sceptical in our conclusions.
Review authors may use the same general approach to conclude that an intervention is not useful. Continuing with the above example where the criterion for an important difference that should be achieved to provide more benefit than harm is a 5% risk difference, an effect estimate of 2% with a 95% confidence interval of 1% to 4% suggests that the intervention does not provide net health benefit.
15.3.2 P values and statistical significance
A P value is the standard result of a statistical test, and is the probability of obtaining the observed effect (or larger) under a ‘null hypothesis’. In the context of Cochrane Reviews there are two commonly used statistical tests. The first is a test of overall effect (a Z-test), and its null hypothesis is that there is no overall effect of the experimental intervention compared with the comparator on the outcome of interest. The second is the (Chi 2 ) test for heterogeneity, and its null hypothesis is that there are no differences in the intervention effects across studies.
A P value that is very small indicates that the observed effect is very unlikely to have arisen purely by chance, and therefore provides evidence against the null hypothesis. It has been common practice to interpret a P value by examining whether it is smaller than particular threshold values. In particular, P values less than 0.05 are often reported as ‘statistically significant’, and interpreted as being small enough to justify rejection of the null hypothesis. However, the 0.05 threshold is an arbitrary one that became commonly used in medical and psychological research largely because P values were determined by comparing the test statistic against tabulations of specific percentage points of statistical distributions. If review authors decide to present a P value with the results of a meta-analysis, they should report a precise P value (as calculated by most statistical software), together with the 95% confidence interval. Review authors should not describe results as ‘statistically significant’, ‘not statistically significant’ or ‘non-significant’ or unduly rely on thresholds for P values , but report the confidence interval together with the exact P value (see MECIR Box 15.3.a ).
We discuss interpretation of the test for heterogeneity in Chapter 10, Section 10.10.2 ; the remainder of this section refers mainly to tests for an overall effect. For tests of an overall effect, the computation of P involves both the effect estimate and precision of the effect estimate (driven largely by sample size). As precision increases, the range of plausible effects that could occur by chance is reduced. Correspondingly, the statistical significance of an effect of a particular magnitude will usually be greater (the P value will be smaller) in a larger study than in a smaller study.
P values are commonly misinterpreted in two ways. First, a moderate or large P value (e.g. greater than 0.05) may be misinterpreted as evidence that the intervention has no effect on the outcome. There is an important difference between this statement and the correct interpretation that there is a high probability that the observed effect on the outcome is due to chance alone. To avoid such a misinterpretation, review authors should always examine the effect estimate and its 95% confidence interval.
The second misinterpretation is to assume that a result with a small P value for the summary effect estimate implies that an experimental intervention has an important benefit. Such a misinterpretation is more likely to occur in large studies and meta-analyses that accumulate data over dozens of studies and thousands of participants. The P value addresses the question of whether the experimental intervention effect is precisely nil; it does not examine whether the effect is of a magnitude of importance to potential recipients of the intervention. In a large study, a small P value may represent the detection of a trivial effect that may not lead to net health benefit when compared with the potential harms (i.e. harmful effects on other important outcomes). Again, inspection of the point estimate and confidence interval helps correct interpretations (see Section 15.3.1 ).
MECIR Box 15.3.a Relevant expectations for conduct of intervention reviews
15.3.3 Relation between confidence intervals, statistical significance and certainty of evidence
The confidence interval (and imprecision) is only one domain that influences overall uncertainty about effect estimates. Uncertainty resulting from imprecision (i.e. statistical uncertainty) may be no less important than uncertainty from indirectness, or any other GRADE domain, in the context of decision making (Schünemann 2016). Thus, the extent to which interpretations of the confidence interval described in Sections 15.3.1 and 15.3.2 correspond to conclusions about overall certainty of the evidence for the outcome of interest depends on these other domains. If there are no concerns about other domains that determine the certainty of the evidence (i.e. risk of bias, inconsistency, indirectness or publication bias), then the interpretation in Sections 15.3.1 and 15.3.2 . about the relation of the confidence interval to the true effect may be carried forward to the overall certainty. However, if there are concerns about the other domains that affect the certainty of the evidence, the interpretation about the true effect needs to be seen in the context of further uncertainty resulting from those concerns.
For example, nine randomized controlled trials in almost 6000 cancer patients indicated that the administration of heparin reduces the risk of venous thromboembolism (VTE), with a risk ratio of 43% (95% CI 19% to 60%) (Akl et al 2011a). For patients with a plausible baseline risk of approximately 4.6% per year, this relative effect suggests that heparin leads to an absolute risk reduction of 20 fewer VTEs (95% CI 9 fewer to 27 fewer) per 1000 people per year (Akl et al 2011a). Now consider that the review authors or those applying the evidence in a guideline have lowered the certainty in the evidence as a result of indirectness. While the confidence intervals would remain unchanged, the certainty in that confidence interval and in the point estimate as reflecting the truth for the question of interest will be lowered. In fact, the certainty range will have unknown width so there will be unknown likelihood of a result within that range because of this indirectness. The lower the certainty in the evidence, the less we know about the width of the certainty range, although methods for quantifying risk of bias and understanding potential direction of bias may offer insight when lowered certainty is due to risk of bias. Nevertheless, decision makers must consider this uncertainty, and must do so in relation to the effect measure that is being evaluated (e.g. a relative or absolute measure). We will describe the impact on interpretations for dichotomous outcomes in Section 15.4 .
15.4 Interpreting results from dichotomous outcomes (including numbers needed to treat)
15.4.1 relative and absolute risk reductions.
Clinicians may be more inclined to prescribe an intervention that reduces the relative risk of death by 25% than one that reduces the risk of death by 1 percentage point, although both presentations of the evidence may relate to the same benefit (i.e. a reduction in risk from 4% to 3%). The former refers to the relative reduction in risk and the latter to the absolute reduction in risk. As described in Chapter 6, Section 6.4.1 , there are several measures for comparing dichotomous outcomes in two groups. Meta-analyses are usually undertaken using risk ratios (RR), odds ratios (OR) or risk differences (RD), but there are several alternative ways of expressing results.
Relative risk reduction (RRR) is a convenient way of re-expressing a risk ratio as a percentage reduction:

For example, a risk ratio of 0.75 translates to a relative risk reduction of 25%, as in the example above.
The risk difference is often referred to as the absolute risk reduction (ARR) or absolute risk increase (ARI), and may be presented as a percentage (e.g. 1%), as a decimal (e.g. 0.01), or as account (e.g. 10 out of 1000). We consider different choices for presenting absolute effects in Section 15.4.3 . We then describe computations for obtaining these numbers from the results of individual studies and of meta-analyses in Section 15.4.4 .
15.4.2 Number needed to treat (NNT)
The number needed to treat (NNT) is a common alternative way of presenting information on the effect of an intervention. The NNT is defined as the expected number of people who need to receive the experimental rather than the comparator intervention for one additional person to either incur or avoid an event (depending on the direction of the result) in a given time frame. Thus, for example, an NNT of 10 can be interpreted as ‘it is expected that one additional (or less) person will incur an event for every 10 participants receiving the experimental intervention rather than comparator over a given time frame’. It is important to be clear that:
- since the NNT is derived from the risk difference, it is still a comparative measure of effect (experimental versus a specific comparator) and not a general property of a single intervention; and
- the NNT gives an ‘expected value’. For example, NNT = 10 does not imply that one additional event will occur in each and every group of 10 people.
NNTs can be computed for both beneficial and detrimental events, and for interventions that cause both improvements and deteriorations in outcomes. In all instances NNTs are expressed as positive whole numbers. Some authors use the term ‘number needed to harm’ (NNH) when an intervention leads to an adverse outcome, or a decrease in a positive outcome, rather than improvement. However, this phrase can be misleading (most notably, it can easily be read to imply the number of people who will experience a harmful outcome if given the intervention), and it is strongly recommended that ‘number needed to harm’ and ‘NNH’ are avoided. The preferred alternative is to use phrases such as ‘number needed to treat for an additional beneficial outcome’ (NNTB) and ‘number needed to treat for an additional harmful outcome’ (NNTH) to indicate direction of effect.
As NNTs refer to events, their interpretation needs to be worded carefully when the binary outcome is a dichotomization of a scale-based outcome. For example, if the outcome is pain measured on a ‘none, mild, moderate or severe’ scale it may have been dichotomized as ‘none or mild’ versus ‘moderate or severe’. It would be inappropriate for an NNT from these data to be referred to as an ‘NNT for pain’. It is an ‘NNT for moderate or severe pain’.
We consider different choices for presenting absolute effects in Section 15.4.3 . We then describe computations for obtaining these numbers from the results of individual studies and of meta-analyses in Section 15.4.4 .
15.4.3 Expressing risk differences
Users of reviews are liable to be influenced by the choice of statistical presentations of the evidence. Hoffrage and colleagues suggest that physicians’ inferences about statistical outcomes are more appropriate when they deal with ‘natural frequencies’ – whole numbers of people, both treated and untreated (e.g. treatment results in a drop from 20 out of 1000 to 10 out of 1000 women having breast cancer) – than when effects are presented as percentages (e.g. 1% absolute reduction in breast cancer risk) (Hoffrage et al 2000). Probabilities may be more difficult to understand than frequencies, particularly when events are rare. While standardization may be important in improving the presentation of research evidence (and participation in healthcare decisions), current evidence suggests that the presentation of natural frequencies for expressing differences in absolute risk is best understood by consumers of healthcare information (Akl et al 2011b). This evidence provides the rationale for presenting absolute risks in ‘Summary of findings’ tables as numbers of people with events per 1000 people receiving the intervention (see Chapter 14 ).
RRs and RRRs remain crucial because relative effects tend to be substantially more stable across risk groups than absolute effects (see Chapter 10, Section 10.4.3 ). Review authors can use their own data to study this consistency (Cates 1999, Smeeth et al 1999). Risk differences from studies are least likely to be consistent across baseline event rates; thus, they are rarely appropriate for computing numbers needed to treat in systematic reviews. If a relative effect measure (OR or RR) is chosen for meta-analysis, then a comparator group risk needs to be specified as part of the calculation of an RD or NNT. In addition, if there are several different groups of participants with different levels of risk, it is crucial to express absolute benefit for each clinically identifiable risk group, clarifying the time period to which this applies. Studies in patients with differing severity of disease, or studies with different lengths of follow-up will almost certainly have different comparator group risks. In these cases, different comparator group risks lead to different RDs and NNTs (except when the intervention has no effect). A recommended approach is to re-express an odds ratio or a risk ratio as a variety of RD or NNTs across a range of assumed comparator risks (ACRs) (McQuay and Moore 1997, Smeeth et al 1999). Review authors should bear these considerations in mind not only when constructing their ‘Summary of findings’ table, but also in the text of their review.
For example, a review of oral anticoagulants to prevent stroke presented information to users by describing absolute benefits for various baseline risks (Aguilar and Hart 2005, Aguilar et al 2007). They presented their principal findings as “The inherent risk of stroke should be considered in the decision to use oral anticoagulants in atrial fibrillation patients, selecting those who stand to benefit most for this therapy” (Aguilar and Hart 2005). Among high-risk atrial fibrillation patients with prior stroke or transient ischaemic attack who have stroke rates of about 12% (120 per 1000) per year, warfarin prevents about 70 strokes yearly per 1000 patients, whereas for low-risk atrial fibrillation patients (with a stroke rate of about 2% per year or 20 per 1000), warfarin prevents only 12 strokes. This presentation helps users to understand the important impact that typical baseline risks have on the absolute benefit that they can expect.
15.4.4 Computations
Direct computation of risk difference (RD) or a number needed to treat (NNT) depends on the summary statistic (odds ratio, risk ratio or risk differences) available from the study or meta-analysis. When expressing results of meta-analyses, review authors should use, in the computations, whatever statistic they determined to be the most appropriate summary for meta-analysis (see Chapter 10, Section 10.4.3 ). Here we present calculations to obtain RD as a reduction in the number of participants per 1000. For example, a risk difference of –0.133 corresponds to 133 fewer participants with the event per 1000.
RDs and NNTs should not be computed from the aggregated total numbers of participants and events across the trials. This approach ignores the randomization within studies, and may produce seriously misleading results if there is unbalanced randomization in any of the studies. Using the pooled result of a meta-analysis is more appropriate. When computing NNTs, the values obtained are by convention always rounded up to the next whole number.
15.4.4.1 Computing NNT from a risk difference (RD)
A NNT may be computed from a risk difference as

where the vertical bars (‘absolute value of’) in the denominator indicate that any minus sign should be ignored. It is convention to round the NNT up to the nearest whole number. For example, if the risk difference is –0.12 the NNT is 9; if the risk difference is –0.22 the NNT is 5. Cochrane Review authors should qualify the NNT as referring to benefit (improvement) or harm by denoting the NNT as NNTB or NNTH. Note that this approach, although feasible, should be used only for the results of a meta-analysis of risk differences. In most cases meta-analyses will be undertaken using a relative measure of effect (RR or OR), and those statistics should be used to calculate the NNT (see Section 15.4.4.2 and 15.4.4.3 ).
15.4.4.2 Computing risk differences or NNT from a risk ratio
To aid interpretation of the results of a meta-analysis of risk ratios, review authors may compute an absolute risk reduction or NNT. In order to do this, an assumed comparator risk (ACR) (otherwise known as a baseline risk, or risk that the outcome of interest would occur with the comparator intervention) is required. It will usually be appropriate to do this for a range of different ACRs. The computation proceeds as follows:

As an example, suppose the risk ratio is RR = 0.92, and an ACR = 0.3 (300 per 1000) is assumed. Then the effect on risk is 24 fewer per 1000:

The NNT is 42:

15.4.4.3 Computing risk differences or NNT from an odds ratio
Review authors may wish to compute a risk difference or NNT from the results of a meta-analysis of odds ratios. In order to do this, an ACR is required. It will usually be appropriate to do this for a range of different ACRs. The computation proceeds as follows:

As an example, suppose the odds ratio is OR = 0.73, and a comparator risk of ACR = 0.3 is assumed. Then the effect on risk is 62 fewer per 1000:

The NNT is 17:
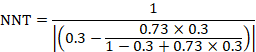
15.4.4.4 Computing risk ratio from an odds ratio
Because risk ratios are easier to interpret than odds ratios, but odds ratios have favourable mathematical properties, a review author may decide to undertake a meta-analysis based on odds ratios, but to express the result as a summary risk ratio (or relative risk reduction). This requires an ACR. Then
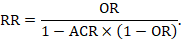
It will often be reasonable to perform this transformation using the median comparator group risk from the studies in the meta-analysis.
15.4.4.5 Computing confidence limits
Confidence limits for RDs and NNTs may be calculated by applying the above formulae to the upper and lower confidence limits for the summary statistic (RD, RR or OR) (Altman 1998). Note that this confidence interval does not incorporate uncertainty around the ACR.
If the 95% confidence interval of OR or RR includes the value 1, one of the confidence limits will indicate benefit and the other harm. Thus, appropriate use of the words ‘fewer’ and ‘more’ is required for each limit when presenting results in terms of events. For NNTs, the two confidence limits should be labelled as NNTB and NNTH to indicate the direction of effect in each case. The confidence interval for the NNT will include a ‘discontinuity’, because increasingly smaller risk differences that approach zero will lead to NNTs approaching infinity. Thus, the confidence interval will include both an infinitely large NNTB and an infinitely large NNTH.
15.5 Interpreting results from continuous outcomes (including standardized mean differences)
15.5.1 meta-analyses with continuous outcomes.
Review authors should describe in the study protocol how they plan to interpret results for continuous outcomes. When outcomes are continuous, review authors have a number of options to present summary results. These options differ if studies report the same measure that is familiar to the target audiences, studies report the same or very similar measures that are less familiar to the target audiences, or studies report different measures.
15.5.2 Meta-analyses with continuous outcomes using the same measure
If all studies have used the same familiar units, for instance, results are expressed as durations of events, such as symptoms for conditions including diarrhoea, sore throat, otitis media, influenza or duration of hospitalization, a meta-analysis may generate a summary estimate in those units, as a difference in mean response (see, for instance, the row summarizing results for duration of diarrhoea in Chapter 14, Figure 14.1.b and the row summarizing oedema in Chapter 14, Figure 14.1.a ). For such outcomes, the ‘Summary of findings’ table should include a difference of means between the two interventions. However, when units of such outcomes may be difficult to interpret, particularly when they relate to rating scales (again, see the oedema row of Chapter 14, Figure 14.1.a ). ‘Summary of findings’ tables should include the minimum and maximum of the scale of measurement, and the direction. Knowledge of the smallest change in instrument score that patients perceive is important – the minimal important difference (MID) – and can greatly facilitate the interpretation of results (Guyatt et al 1998, Schünemann and Guyatt 2005). Knowing the MID allows review authors and users to place results in context. Review authors should state the MID – if known – in the Comments column of their ‘Summary of findings’ table. For example, the chronic respiratory questionnaire has possible scores in health-related quality of life ranging from 1 to 7 and 0.5 represents a well-established MID (Jaeschke et al 1989, Schünemann et al 2005).
15.5.3 Meta-analyses with continuous outcomes using different measures
When studies have used different instruments to measure the same construct, a standardized mean difference (SMD) may be used in meta-analysis for combining continuous data. Without guidance, clinicians and patients may have little idea how to interpret results presented as SMDs. Review authors should therefore consider issues of interpretability when planning their analysis at the protocol stage and should consider whether there will be suitable ways to re-express the SMD or whether alternative effect measures, such as a ratio of means, or possibly as minimal important difference units (Guyatt et al 2013b) should be used. Table 15.5.a and the following sections describe these options.
Table 15.5.a Approaches and their implications to presenting results of continuous variables when primary studies have used different instruments to measure the same construct. Adapted from Guyatt et al (2013b)
15.5.3.1 Presenting and interpreting SMDs using generic effect size estimates
The SMD expresses the intervention effect in standard units rather than the original units of measurement. The SMD is the difference in mean effects between the experimental and comparator groups divided by the pooled standard deviation of participants’ outcomes, or external SDs when studies are very small (see Chapter 6, Section 6.5.1.2 ). The value of a SMD thus depends on both the size of the effect (the difference between means) and the standard deviation of the outcomes (the inherent variability among participants or based on an external SD).
If review authors use the SMD, they might choose to present the results directly as SMDs (row 1a, Table 15.5.a and Table 15.5.b ). However, absolute values of the intervention and comparison groups are typically not useful because studies have used different measurement instruments with different units. Guiding rules for interpreting SMDs (or ‘Cohen’s effect sizes’) exist, and have arisen mainly from researchers in the social sciences (Cohen 1988). One example is as follows: 0.2 represents a small effect, 0.5 a moderate effect and 0.8 a large effect (Cohen 1988). Variations exist (e.g. <0.40=small, 0.40 to 0.70=moderate, >0.70=large). Review authors might consider including such a guiding rule in interpreting the SMD in the text of the review, and in summary versions such as the Comments column of a ‘Summary of findings’ table. However, some methodologists believe that such interpretations are problematic because patient importance of a finding is context-dependent and not amenable to generic statements.
15.5.3.2 Re-expressing SMDs using a familiar instrument
The second possibility for interpreting the SMD is to express it in the units of one or more of the specific measurement instruments used by the included studies (row 1b, Table 15.5.a and Table 15.5.b ). The approach is to calculate an absolute difference in means by multiplying the SMD by an estimate of the SD associated with the most familiar instrument. To obtain this SD, a reasonable option is to calculate a weighted average across all intervention groups of all studies that used the selected instrument (preferably a pre-intervention or post-intervention SD as discussed in Chapter 10, Section 10.5.2 ). To better reflect among-person variation in practice, or to use an instrument not represented in the meta-analysis, it may be preferable to use a standard deviation from a representative observational study. The summary effect is thus re-expressed in the original units of that particular instrument and the clinical relevance and impact of the intervention effect can be interpreted using that familiar instrument.
The same approach of re-expressing the results for a familiar instrument can also be used for other standardized effect measures such as when standardizing by MIDs (Guyatt et al 2013b): see Section 15.5.3.5 .
Table 15.5.b Application of approaches when studies have used different measures: effects of dexamethasone for pain after laparoscopic cholecystectomy (Karanicolas et al 2008). Reproduced with permission of Wolters Kluwer
1 Certainty rated according to GRADE from very low to high certainty. 2 Substantial unexplained heterogeneity in study results. 3 Imprecision due to wide confidence intervals. 4 The 20% comes from the proportion in the control group requiring rescue analgesia. 5 Crude (arithmetic) means of the post-operative pain mean responses across all five trials when transformed to a 100-point scale.
15.5.3.3 Re-expressing SMDs through dichotomization and transformation to relative and absolute measures
A third approach (row 1c, Table 15.5.a and Table 15.5.b ) relies on converting the continuous measure into a dichotomy and thus allows calculation of relative and absolute effects on a binary scale. A transformation of a SMD to a (log) odds ratio is available, based on the assumption that an underlying continuous variable has a logistic distribution with equal standard deviation in the two intervention groups, as discussed in Chapter 10, Section 10.6 (Furukawa 1999, Guyatt et al 2013b). The assumption is unlikely to hold exactly and the results must be regarded as an approximation. The log odds ratio is estimated as
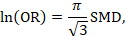
(or approximately 1.81✕SMD). The resulting odds ratio can then be presented as normal, and in a ‘Summary of findings’ table, combined with an assumed comparator group risk to be expressed as an absolute risk difference. The comparator group risk in this case would refer to the proportion of people who have achieved a specific value of the continuous outcome. In randomized trials this can be interpreted as the proportion who have improved by some (specified) amount (responders), for instance by 5 points on a 0 to 100 scale. Table 15.5.c shows some illustrative results from this method. The risk differences can then be converted to NNTs or to people per thousand using methods described in Section 15.4.4 .
Table 15.5.c Risk difference derived for specific SMDs for various given ‘proportions improved’ in the comparator group (Furukawa 1999, Guyatt et al 2013b). Reproduced with permission of Elsevier
15.5.3.4 Ratio of means
A more frequently used approach is based on calculation of a ratio of means between the intervention and comparator groups (Friedrich et al 2008) as discussed in Chapter 6, Section 6.5.1.3 . Interpretational advantages of this approach include the ability to pool studies with outcomes expressed in different units directly, to avoid the vulnerability of heterogeneous populations that limits approaches that rely on SD units, and for ease of clinical interpretation (row 2, Table 15.5.a and Table 15.5.b ). This method is currently designed for post-intervention scores only. However, it is possible to calculate a ratio of change scores if both intervention and comparator groups change in the same direction in each relevant study, and this ratio may sometimes be informative.
Limitations to this approach include its limited applicability to change scores (since it is unlikely that both intervention and comparator group changes are in the same direction in all studies) and the possibility of misleading results if the comparator group mean is very small, in which case even a modest difference from the intervention group will yield a large and therefore misleading ratio of means. It also requires that separate ratios of means be calculated for each included study, and then entered into a generic inverse variance meta-analysis (see Chapter 10, Section 10.3 ).
The ratio of means approach illustrated in Table 15.5.b suggests a relative reduction in pain of only 13%, meaning that those receiving steroids have a pain severity 87% of those in the comparator group, an effect that might be considered modest.
15.5.3.5 Presenting continuous results as minimally important difference units
To express results in MID units, review authors have two options. First, they can be combined across studies in the same way as the SMD, but instead of dividing the mean difference of each study by its SD, review authors divide by the MID associated with that outcome (Johnston et al 2010, Guyatt et al 2013b). Instead of SD units, the pooled results represent MID units (row 3, Table 15.5.a and Table 15.5.b ), and may be more easily interpretable. This approach avoids the problem of varying SDs across studies that may distort estimates of effect in approaches that rely on the SMD. The approach, however, relies on having well-established MIDs. The approach is also risky in that a difference less than the MID may be interpreted as trivial when a substantial proportion of patients may have achieved an important benefit.
The other approach makes a simple conversion (not shown in Table 15.5.b ), before undertaking the meta-analysis, of the means and SDs from each study to means and SDs on the scale of a particular familiar instrument whose MID is known. For example, one can rescale the mean and SD of other chronic respiratory disease instruments (e.g. rescaling a 0 to 100 score of an instrument) to a the 1 to 7 score in Chronic Respiratory Disease Questionnaire (CRQ) units (by assuming 0 equals 1 and 100 equals 7 on the CRQ). Given the MID of the CRQ of 0.5, a mean difference in change of 0.71 after rescaling of all studies suggests a substantial effect of the intervention (Guyatt et al 2013b). This approach, presenting in units of the most familiar instrument, may be the most desirable when the target audiences have extensive experience with that instrument, particularly if the MID is well established.
15.6 Drawing conclusions
15.6.1 conclusions sections of a cochrane review.
Authors’ conclusions in a Cochrane Review are divided into implications for practice and implications for research. While Cochrane Reviews about interventions can provide meaningful information and guidance for practice, decisions about the desirable and undesirable consequences of healthcare options require evidence and judgements for criteria that most Cochrane Reviews do not provide (Alonso-Coello et al 2016). In describing the implications for practice and the development of recommendations, however, review authors may consider the certainty of the evidence, the balance of benefits and harms, and assumed values and preferences.
15.6.2 Implications for practice
Drawing conclusions about the practical usefulness of an intervention entails making trade-offs, either implicitly or explicitly, between the estimated benefits, harms and the values and preferences. Making such trade-offs, and thus making specific recommendations for an action in a specific context, goes beyond a Cochrane Review and requires additional evidence and informed judgements that most Cochrane Reviews do not provide (Alonso-Coello et al 2016). Such judgements are typically the domain of clinical practice guideline developers for which Cochrane Reviews will provide crucial information (Graham et al 2011, Schünemann et al 2014, Zhang et al 2018a). Thus, authors of Cochrane Reviews should not make recommendations.
If review authors feel compelled to lay out actions that clinicians and patients could take, they should – after describing the certainty of evidence and the balance of benefits and harms – highlight different actions that might be consistent with particular patterns of values and preferences. Other factors that might influence a decision should also be highlighted, including any known factors that would be expected to modify the effects of the intervention, the baseline risk or status of the patient, costs and who bears those costs, and the availability of resources. Review authors should ensure they consider all patient-important outcomes, including those for which limited data may be available. In the context of public health reviews the focus may be on population-important outcomes as the target may be an entire (non-diseased) population and include outcomes that are not measured in the population receiving an intervention (e.g. a reduction of transmission of infections from those receiving an intervention). This process implies a high level of explicitness in judgements about values or preferences attached to different outcomes and the certainty of the related evidence (Zhang et al 2018b, Zhang et al 2018c); this and a full cost-effectiveness analysis is beyond the scope of most Cochrane Reviews (although they might well be used for such analyses; see Chapter 20 ).
A review on the use of anticoagulation in cancer patients to increase survival (Akl et al 2011a) provides an example for laying out clinical implications for situations where there are important trade-offs between desirable and undesirable effects of the intervention: “The decision for a patient with cancer to start heparin therapy for survival benefit should balance the benefits and downsides and integrate the patient’s values and preferences. Patients with a high preference for a potential survival prolongation, limited aversion to potential bleeding, and who do not consider heparin (both UFH or LMWH) therapy a burden may opt to use heparin, while those with aversion to bleeding may not.”
15.6.3 Implications for research
The second category for authors’ conclusions in a Cochrane Review is implications for research. To help people make well-informed decisions about future healthcare research, the ‘Implications for research’ section should comment on the need for further research, and the nature of the further research that would be most desirable. It is helpful to consider the population, intervention, comparison and outcomes that could be addressed, or addressed more effectively in the future, in the context of the certainty of the evidence in the current review (Brown et al 2006):
- P (Population): diagnosis, disease stage, comorbidity, risk factor, sex, age, ethnic group, specific inclusion or exclusion criteria, clinical setting;
- I (Intervention): type, frequency, dose, duration, prognostic factor;
- C (Comparison): placebo, routine care, alternative treatment/management;
- O (Outcome): which clinical or patient-related outcomes will the researcher need to measure, improve, influence or accomplish? Which methods of measurement should be used?
While Cochrane Review authors will find the PICO domains helpful, the domains of the GRADE certainty framework further support understanding and describing what additional research will improve the certainty in the available evidence. Note that as the certainty of the evidence is likely to vary by outcome, these implications will be specific to certain outcomes in the review. Table 15.6.a shows how review authors may be aided in their interpretation of the body of evidence and drawing conclusions about future research and practice.
Table 15.6.a Implications for research and practice suggested by individual GRADE domains
The review of compression stockings for prevention of deep vein thrombosis (DVT) in airline passengers described in Chapter 14 provides an example where there is some convincing evidence of a benefit of the intervention: “This review shows that the question of the effects on symptomless DVT of wearing versus not wearing compression stockings in the types of people studied in these trials should now be regarded as answered. Further research may be justified to investigate the relative effects of different strengths of stockings or of stockings compared to other preventative strategies. Further randomised trials to address the remaining uncertainty about the effects of wearing versus not wearing compression stockings on outcomes such as death, pulmonary embolism and symptomatic DVT would need to be large.” (Clarke et al 2016).
A review of therapeutic touch for anxiety disorder provides an example of the implications for research when no eligible studies had been found: “This review highlights the need for randomized controlled trials to evaluate the effectiveness of therapeutic touch in reducing anxiety symptoms in people diagnosed with anxiety disorders. Future trials need to be rigorous in design and delivery, with subsequent reporting to include high quality descriptions of all aspects of methodology to enable appraisal and interpretation of results.” (Robinson et al 2007).
15.6.4 Reaching conclusions
A common mistake is to confuse ‘no evidence of an effect’ with ‘evidence of no effect’. When the confidence intervals are too wide (e.g. including no effect), it is wrong to claim that the experimental intervention has ‘no effect’ or is ‘no different’ from the comparator intervention. Review authors may also incorrectly ‘positively’ frame results for some effects but not others. For example, when the effect estimate is positive for a beneficial outcome but confidence intervals are wide, review authors may describe the effect as promising. However, when the effect estimate is negative for an outcome that is considered harmful but the confidence intervals include no effect, review authors report no effect. Another mistake is to frame the conclusion in wishful terms. For example, review authors might write, “there were too few people in the analysis to detect a reduction in mortality” when the included studies showed a reduction or even increase in mortality that was not ‘statistically significant’. One way of avoiding errors such as these is to consider the results blinded; that is, consider how the results would be presented and framed in the conclusions if the direction of the results was reversed. If the confidence interval for the estimate of the difference in the effects of the interventions overlaps with no effect, the analysis is compatible with both a true beneficial effect and a true harmful effect. If one of the possibilities is mentioned in the conclusion, the other possibility should be mentioned as well. Table 15.6.b suggests narrative statements for drawing conclusions based on the effect estimate from the meta-analysis and the certainty of the evidence.
Table 15.6.b Suggested narrative statements for phrasing conclusions
Another common mistake is to reach conclusions that go beyond the evidence. Often this is done implicitly, without referring to the additional information or judgements that are used in reaching conclusions about the implications of a review for practice. Even when additional information and explicit judgements support conclusions about the implications of a review for practice, review authors rarely conduct systematic reviews of the additional information. Furthermore, implications for practice are often dependent on specific circumstances and values that must be taken into consideration. As we have noted, review authors should always be cautious when drawing conclusions about implications for practice and they should not make recommendations.
15.7 Chapter information
Authors: Holger J Schünemann, Gunn E Vist, Julian PT Higgins, Nancy Santesso, Jonathan J Deeks, Paul Glasziou, Elie Akl, Gordon H Guyatt; on behalf of the Cochrane GRADEing Methods Group
Acknowledgements: Andrew Oxman, Jonathan Sterne, Michael Borenstein and Rob Scholten contributed text to earlier versions of this chapter.
Funding: This work was in part supported by funding from the Michael G DeGroote Cochrane Canada Centre and the Ontario Ministry of Health. JJD receives support from the National Institute for Health Research (NIHR) Birmingham Biomedical Research Centre at the University Hospitals Birmingham NHS Foundation Trust and the University of Birmingham. JPTH receives support from the NIHR Biomedical Research Centre at University Hospitals Bristol NHS Foundation Trust and the University of Bristol. The views expressed are those of the author(s) and not necessarily those of the NHS, the NIHR or the Department of Health.
15.8 References
Aguilar MI, Hart R. Oral anticoagulants for preventing stroke in patients with non-valvular atrial fibrillation and no previous history of stroke or transient ischemic attacks. Cochrane Database of Systematic Reviews 2005; 3 : CD001927.
Aguilar MI, Hart R, Pearce LA. Oral anticoagulants versus antiplatelet therapy for preventing stroke in patients with non-valvular atrial fibrillation and no history of stroke or transient ischemic attacks. Cochrane Database of Systematic Reviews 2007; 3 : CD006186.
Akl EA, Gunukula S, Barba M, Yosuico VE, van Doormaal FF, Kuipers S, Middeldorp S, Dickinson HO, Bryant A, Schünemann H. Parenteral anticoagulation in patients with cancer who have no therapeutic or prophylactic indication for anticoagulation. Cochrane Database of Systematic Reviews 2011a; 1 : CD006652.
Akl EA, Oxman AD, Herrin J, Vist GE, Terrenato I, Sperati F, Costiniuk C, Blank D, Schünemann H. Using alternative statistical formats for presenting risks and risk reductions. Cochrane Database of Systematic Reviews 2011b; 3 : CD006776.
Alonso-Coello P, Schünemann HJ, Moberg J, Brignardello-Petersen R, Akl EA, Davoli M, Treweek S, Mustafa RA, Rada G, Rosenbaum S, Morelli A, Guyatt GH, Oxman AD, Group GW. GRADE Evidence to Decision (EtD) frameworks: a systematic and transparent approach to making well informed healthcare choices. 1: Introduction. BMJ 2016; 353 : i2016.
Altman DG. Confidence intervals for the number needed to treat. BMJ 1998; 317 : 1309-1312.
Atkins D, Best D, Briss PA, Eccles M, Falck-Ytter Y, Flottorp S, Guyatt GH, Harbour RT, Haugh MC, Henry D, Hill S, Jaeschke R, Leng G, Liberati A, Magrini N, Mason J, Middleton P, Mrukowicz J, O'Connell D, Oxman AD, Phillips B, Schünemann HJ, Edejer TT, Varonen H, Vist GE, Williams JW, Jr., Zaza S. Grading quality of evidence and strength of recommendations. BMJ 2004; 328 : 1490.
Brown P, Brunnhuber K, Chalkidou K, Chalmers I, Clarke M, Fenton M, Forbes C, Glanville J, Hicks NJ, Moody J, Twaddle S, Timimi H, Young P. How to formulate research recommendations. BMJ 2006; 333 : 804-806.
Cates C. Confidence intervals for the number needed to treat: Pooling numbers needed to treat may not be reliable. BMJ 1999; 318 : 1764-1765.
Clarke MJ, Broderick C, Hopewell S, Juszczak E, Eisinga A. Compression stockings for preventing deep vein thrombosis in airline passengers. Cochrane Database of Systematic Reviews 2016; 9 : CD004002.
Cohen J. Statistical Power Analysis in the Behavioral Sciences . 2nd edition ed. Hillsdale (NJ): Lawrence Erlbaum Associates, Inc.; 1988.
Coleman T, Chamberlain C, Davey MA, Cooper SE, Leonardi-Bee J. Pharmacological interventions for promoting smoking cessation during pregnancy. Cochrane Database of Systematic Reviews 2015; 12 : CD010078.
Dans AM, Dans L, Oxman AD, Robinson V, Acuin J, Tugwell P, Dennis R, Kang D. Assessing equity in clinical practice guidelines. Journal of Clinical Epidemiology 2007; 60 : 540-546.
Friedman LM, Furberg CD, DeMets DL. Fundamentals of Clinical Trials . 2nd edition ed. Littleton (MA): John Wright PSG, Inc.; 1985.
Friedrich JO, Adhikari NK, Beyene J. The ratio of means method as an alternative to mean differences for analyzing continuous outcome variables in meta-analysis: a simulation study. BMC Medical Research Methodology 2008; 8 : 32.
Furukawa T. From effect size into number needed to treat. Lancet 1999; 353 : 1680.
Graham R, Mancher M, Wolman DM, Greenfield S, Steinberg E. Committee on Standards for Developing Trustworthy Clinical Practice Guidelines, Board on Health Care Services: Clinical Practice Guidelines We Can Trust. Washington, DC: National Academies Press; 2011.
Guyatt G, Oxman AD, Akl EA, Kunz R, Vist G, Brozek J, Norris S, Falck-Ytter Y, Glasziou P, DeBeer H, Jaeschke R, Rind D, Meerpohl J, Dahm P, Schünemann HJ. GRADE guidelines: 1. Introduction-GRADE evidence profiles and summary of findings tables. Journal of Clinical Epidemiology 2011a; 64 : 383-394.
Guyatt GH, Juniper EF, Walter SD, Griffith LE, Goldstein RS. Interpreting treatment effects in randomised trials. BMJ 1998; 316 : 690-693.
Guyatt GH, Oxman AD, Vist GE, Kunz R, Falck-Ytter Y, Alonso-Coello P, Schünemann HJ. GRADE: an emerging consensus on rating quality of evidence and strength of recommendations. BMJ 2008; 336 : 924-926.
Guyatt GH, Oxman AD, Kunz R, Woodcock J, Brozek J, Helfand M, Alonso-Coello P, Falck-Ytter Y, Jaeschke R, Vist G, Akl EA, Post PN, Norris S, Meerpohl J, Shukla VK, Nasser M, Schünemann HJ. GRADE guidelines: 8. Rating the quality of evidence--indirectness. Journal of Clinical Epidemiology 2011b; 64 : 1303-1310.
Guyatt GH, Oxman AD, Santesso N, Helfand M, Vist G, Kunz R, Brozek J, Norris S, Meerpohl J, Djulbegovic B, Alonso-Coello P, Post PN, Busse JW, Glasziou P, Christensen R, Schünemann HJ. GRADE guidelines: 12. Preparing summary of findings tables-binary outcomes. Journal of Clinical Epidemiology 2013a; 66 : 158-172.
Guyatt GH, Thorlund K, Oxman AD, Walter SD, Patrick D, Furukawa TA, Johnston BC, Karanicolas P, Akl EA, Vist G, Kunz R, Brozek J, Kupper LL, Martin SL, Meerpohl JJ, Alonso-Coello P, Christensen R, Schünemann HJ. GRADE guidelines: 13. Preparing summary of findings tables and evidence profiles-continuous outcomes. Journal of Clinical Epidemiology 2013b; 66 : 173-183.
Hawe P, Shiell A, Riley T, Gold L. Methods for exploring implementation variation and local context within a cluster randomised community intervention trial. Journal of Epidemiology and Community Health 2004; 58 : 788-793.
Hoffrage U, Lindsey S, Hertwig R, Gigerenzer G. Medicine. Communicating statistical information. Science 2000; 290 : 2261-2262.
Jaeschke R, Singer J, Guyatt GH. Measurement of health status. Ascertaining the minimal clinically important difference. Controlled Clinical Trials 1989; 10 : 407-415.
Johnston B, Thorlund K, Schünemann H, Xie F, Murad M, Montori V, Guyatt G. Improving the interpretation of health-related quality of life evidence in meta-analysis: The application of minimal important difference units. . Health Outcomes and Qualithy of Life 2010; 11 : 116.
Karanicolas PJ, Smith SE, Kanbur B, Davies E, Guyatt GH. The impact of prophylactic dexamethasone on nausea and vomiting after laparoscopic cholecystectomy: a systematic review and meta-analysis. Annals of Surgery 2008; 248 : 751-762.
Lumley J, Oliver SS, Chamberlain C, Oakley L. Interventions for promoting smoking cessation during pregnancy. Cochrane Database of Systematic Reviews 2004; 4 : CD001055.
McQuay HJ, Moore RA. Using numerical results from systematic reviews in clinical practice. Annals of Internal Medicine 1997; 126 : 712-720.
Resnicow K, Cross D, Wynder E. The Know Your Body program: a review of evaluation studies. Bulletin of the New York Academy of Medicine 1993; 70 : 188-207.
Robinson J, Biley FC, Dolk H. Therapeutic touch for anxiety disorders. Cochrane Database of Systematic Reviews 2007; 3 : CD006240.
Rothwell PM. External validity of randomised controlled trials: "to whom do the results of this trial apply?". Lancet 2005; 365 : 82-93.
Santesso N, Carrasco-Labra A, Langendam M, Brignardello-Petersen R, Mustafa RA, Heus P, Lasserson T, Opiyo N, Kunnamo I, Sinclair D, Garner P, Treweek S, Tovey D, Akl EA, Tugwell P, Brozek JL, Guyatt G, Schünemann HJ. Improving GRADE evidence tables part 3: detailed guidance for explanatory footnotes supports creating and understanding GRADE certainty in the evidence judgments. Journal of Clinical Epidemiology 2016; 74 : 28-39.
Schünemann HJ, Puhan M, Goldstein R, Jaeschke R, Guyatt GH. Measurement properties and interpretability of the Chronic respiratory disease questionnaire (CRQ). COPD: Journal of Chronic Obstructive Pulmonary Disease 2005; 2 : 81-89.
Schünemann HJ, Guyatt GH. Commentary--goodbye M(C)ID! Hello MID, where do you come from? Health Services Research 2005; 40 : 593-597.
Schünemann HJ, Fretheim A, Oxman AD. Improving the use of research evidence in guideline development: 13. Applicability, transferability and adaptation. Health Research Policy and Systems 2006; 4 : 25.
Schünemann HJ. Methodological idiosyncracies, frameworks and challenges of non-pharmaceutical and non-technical treatment interventions. Zeitschrift für Evidenz, Fortbildung und Qualität im Gesundheitswesen 2013; 107 : 214-220.
Schünemann HJ, Tugwell P, Reeves BC, Akl EA, Santesso N, Spencer FA, Shea B, Wells G, Helfand M. Non-randomized studies as a source of complementary, sequential or replacement evidence for randomized controlled trials in systematic reviews on the effects of interventions. Research Synthesis Methods 2013; 4 : 49-62.
Schünemann HJ, Wiercioch W, Etxeandia I, Falavigna M, Santesso N, Mustafa R, Ventresca M, Brignardello-Petersen R, Laisaar KT, Kowalski S, Baldeh T, Zhang Y, Raid U, Neumann I, Norris SL, Thornton J, Harbour R, Treweek S, Guyatt G, Alonso-Coello P, Reinap M, Brozek J, Oxman A, Akl EA. Guidelines 2.0: systematic development of a comprehensive checklist for a successful guideline enterprise. CMAJ: Canadian Medical Association Journal 2014; 186 : E123-142.
Schünemann HJ. Interpreting GRADE's levels of certainty or quality of the evidence: GRADE for statisticians, considering review information size or less emphasis on imprecision? Journal of Clinical Epidemiology 2016; 75 : 6-15.
Smeeth L, Haines A, Ebrahim S. Numbers needed to treat derived from meta-analyses--sometimes informative, usually misleading. BMJ 1999; 318 : 1548-1551.
Sun X, Briel M, Busse JW, You JJ, Akl EA, Mejza F, Bala MM, Bassler D, Mertz D, Diaz-Granados N, Vandvik PO, Malaga G, Srinathan SK, Dahm P, Johnston BC, Alonso-Coello P, Hassouneh B, Walter SD, Heels-Ansdell D, Bhatnagar N, Altman DG, Guyatt GH. Credibility of claims of subgroup effects in randomised controlled trials: systematic review. BMJ 2012; 344 : e1553.
Zhang Y, Akl EA, Schünemann HJ. Using systematic reviews in guideline development: the GRADE approach. Research Synthesis Methods 2018a: doi: 10.1002/jrsm.1313.
Zhang Y, Alonso-Coello P, Guyatt GH, Yepes-Nunez JJ, Akl EA, Hazlewood G, Pardo-Hernandez H, Etxeandia-Ikobaltzeta I, Qaseem A, Williams JW, Jr., Tugwell P, Flottorp S, Chang Y, Zhang Y, Mustafa RA, Rojas MX, Schünemann HJ. GRADE Guidelines: 19. Assessing the certainty of evidence in the importance of outcomes or values and preferences-Risk of bias and indirectness. Journal of Clinical Epidemiology 2018b: doi: 10.1016/j.jclinepi.2018.1001.1013.
Zhang Y, Alonso Coello P, Guyatt G, Yepes-Nunez JJ, Akl EA, Hazlewood G, Pardo-Hernandez H, Etxeandia-Ikobaltzeta I, Qaseem A, Williams JW, Jr., Tugwell P, Flottorp S, Chang Y, Zhang Y, Mustafa RA, Rojas MX, Xie F, Schünemann HJ. GRADE Guidelines: 20. Assessing the certainty of evidence in the importance of outcomes or values and preferences - Inconsistency, Imprecision, and other Domains. Journal of Clinical Epidemiology 2018c: doi: 10.1016/j.jclinepi.2018.1005.1011.
For permission to re-use material from the Handbook (either academic or commercial), please see here for full details.
Generalizability and Transferability
In this chapter, we discuss generalizabililty, transferability, and the interrelationship between the two. We also explain how these two aspects of research operate in different methodologies, demonstrating how researchers may apply these concepts throughout the research process.
Generalizability Overview
Generalizability is applied by researchers in an academic setting. It can be defined as the extension of research findings and conclusions from a study conducted on a sample population to the population at large. While the dependability of this extension is not absolute, it is statistically probable. Because sound generalizability requires data on large populations, quantitative research -- experimental for instance -- provides the best foundation for producing broad generalizability. The larger the sample population, the more one can generalize the results. For example, a comprehensive study of the role computers play in the writing process might reveal that it is statistically probable that students who do most of their composing on a computer will move chunks of text around more than students who do not compose on a computer.
Transferability Overview
Transferability is applied by the readers of research. Although generalizability usually applies only to certain types of quantitative methods, transferability can apply in varying degrees to most types of research . Unlike generalizability, transferability does not involve broad claims, but invites readers of research to make connections between elements of a study and their own experience. For instance, teachers at the high school level might selectively apply to their own classrooms results from a study demonstrating that heuristic writing exercises help students at the college level.
Interrelationships
Generalizability and transferability are important elements of any research methodology, but they are not mutually exclusive: generalizability, to varying degrees, rests on the transferability of research findings. It is important for researchers to understand the implications of these twin aspects of research before designing a study. Researchers who intend to make a generalizable claim must carefully examine the variables involved in the study. Among these are the sample of the population used and the mechanisms behind formulating a causal model. Furthermore, if researchers desire to make the results of their study transferable to another context, they must keep a detailed account of the environment surrounding their research, and include a rich description of that environment in their final report. Armed with the knowledge that the sample population was large and varied, as well as with detailed information about the study itself, readers of research can more confidently generalize and transfer the findings to other situations.
Generalizability
Generalizability is not only common to research, but to everyday life as well. In this section, we establish a practical working definition of generalizability as it is applied within and outside of academic research. We also define and consider three different types of generalizability and some of their probable applications. Finally, we discuss some of the possible shortcomings and limitations of generalizability that researchers must be aware of when constructing a study they hope will yield potentially generalizable results.
In many ways, generalizability amounts to nothing more than making predictions based on a recurring experience. If something occurs frequently, we expect that it will continue to do so in the future. Researchers use the same type of reasoning when generalizing about the findings of their studies. Once researchers have collected sufficient data to support a hypothesis, a premise regarding the behavior of that data can be formulated, making it generalizable to similar circumstances. Because of its foundation in probability, however, such a generalization cannot be regarded as conclusive or exhaustive.
While generalizability can occur in informal, nonacademic settings, it is usually applied only to certain research methods in academic studies. Quantitative methods allow some generalizability. Experimental research, for example, often produces generalizable results. However, such experimentation must be rigorous in order for generalizable results to be found.
An example of generalizability in everyday life involves driving. Operating an automobile in traffic requires that drivers make assumptions about the likely outcome of certain actions. When approaching an intersection where one driver is preparing to turn left, the driver going straight through the intersection assumes that the left-turning driver will yield the right of way before turning. The driver passing through the intersection applies this assumption cautiously, recognizing the possibility that the other driver might turn prematurely.
American drivers also generalize that everyone will drive on the right hand side of the road. Yet if we try to generalize this assumption to other settings, such as England, we will be making a potentially disastrous mistake. Thus, it is obvious that generalizing is necessary for forming coherent interpretations in many different situations, but we do not expect our generalizations to operate the same way in every circumstance. With enough evidence we can make predictions about human behavior, yet we must simultaneously recognize that our assumptions are based on statistical probability.
Consider this example of generalizable research in the field of English studies. A study on undergraduate instructor evaluations of composition instructors might reveal that there is a strong correlation between the grade students are expecting to earn in a course and whether they give their instructor high marks. The study might discover that 95% of students who expect to receive a "C" or lower in their class give their instructor a rating of "average" or below. Therefore, there would be a high probability that future students expecting a "C" or lower would not give their instructor high marks. However, the results would not necessarily be conclusive. Some students might defy the trend. In addition, a number of different variables could also influence students' evaluations of an instructor, including instructor experience, class size, and relative interest in a particular subject. These variables -- and others -- would have to be addressed in order for the study to yield potentially valid results. However, even if virtually all variables were isolated, results of the study would not be 100% conclusive. At best, researchers can make educated predictions of future events or behaviors, not guarantee the prediction in every case. Thus, before generalizing, findings must be tested through rigorous experimentation, which enables researchers to confirm or reject the premises governing their data set.
Considerations
There are three types of generalizability that interact to produce probabilistic models. All of them involve generalizing a treatment or measurement to a population outside of the original study. Researchers who wish to generalize their claims should try to apply all three forms to their research, or the strength of their claims will be weakened (Runkel & McGrath, 1972).
In one type of generalizability, researchers determine whether a specific treatment will produce the same results in different circumstances. To do this, they must decide if an aspect within the original environment, a factor beyond the treatment, generated the particular result. This will establish how flexibly the treatment adapts to new situations. Higher adaptability means that the treatment is generalizable to a greater variety of situations. For example, imagine that a new set of heuristic prewriting questions designed to encourage freshman college students to consider audience more fully works so well that the students write thoroughly developed rhetorical analyses of their target audiences. To responsibly generalize that this heuristic is effective, a researcher would need to test the same prewriting exercise in a variety of educational settings at the college level, using different teachers, students, and environments. If the same positive results are produced, the treatment is generalizable.
A second form of generalizability focuses on measurements rather than treatments. For a result to be considered generalizable outside of the test group, it must produce the same results with different forms of measurement. In terms of the heuristic example above, the findings will be more generalizable if the same results are obtained when assessed "with questions having a slightly different wording, or when we use a six-point scale instead of a nine-point scale" (Runkel & McGrath, 1972, p.46).
A third type of generalizability concerns the subjects of the test situation. Although the results of an experiment may be internally valid, that is, applicable to the group tested, in many situations the results cannot be generalized beyond that particular group. Researchers who hope to generalize their results to a larger population should ensure that their test group is relatively large and randomly chosen. However, researchers should consider the fact that test populations of over 10,000 subjects do not significantly increase generalizability (Firestone,1993).
Potential Limitations
No matter how carefully these three forms of generalizability are applied, there is no absolute guarantee that the results obtained in a study will occur in every situation outside the study. In order to determine causal relationships in a test environment, precision is of utmost importance. Yet if researchers wish to generalize their findings, scope and variance must be emphasized over precision. Therefore, it becomes difficult to test for precision and generalizability simultaneously, since a focus on one reduces the reliability of the other. One solution to this problem is to perform a greater number of observations, which has a dual effect: first, it increases the sample population, which heightens generalizability; second, precision can be reasonably maintained because the random errors between observations will average out (Runkel and McGrath, 1972).
Transferability
Transferability describes the process of applying the results of research in one situation to other similar situations. In this section, we establish a practical working definition of transferability as it's applied within and outside of academic research. We also outline important considerations researchers must be aware of in order to make their results potentially transferable, as well as the critical role the reader plays in this process. Finally, we discuss possible shortcomings and limitations of transferability that researchers must be aware of when planning and conducting a study that will yield potentially transferable results.
Transferability is a process performed by readers of research. Readers note the specifics of the research situation and compare them to the specifics of an environment or situation with which they are familiar. If there are enough similarities between the two situations, readers may be able to infer that the results of the research would be the same or similar in their own situation. In other words, they "transfer" the results of a study to another context. To do this effectively, readers need to know as much as possible about the original research situation in order to determine whether it is similar to their own. Therefore, researchers must supply a highly detailed description of their research situation and methods.
Results of any type of research method can be applied to other situations, but transferability is most relevant to qualitative research methods such as ethnography and case studies. Reports based on these research methods are detailed and specific. However, because they often consider only one subject or one group, researchers who conduct such studies seldom generalize the results to other populations. The detailed nature of the results, however, makes them ideal for transferability.
Transferability is easy to understand when you consider that we are constantly applying this concept to aspects of our daily lives. If, for example, you are an inexperienced composition instructor and you read a study in which a veteran writing instructor discovered that extensive prewriting exercises helped students in her classes come up with much more narrowly defined paper topics, you could ask yourself how much the instructor's classroom resembled your own. If there were many similarities, you might try to draw conclusions about how increasing the amount of prewriting your students do would impact their ability to arrive at sufficiently narrow paper topics. In doing so, you would be attempting to transfer the composition researcher's techniques to your own classroom.
An example of transferable research in the field of English studies is Berkenkotter, Huckin, and Ackerman's (1988) study of a graduate student in a rhetoric Ph.D. program. In this case study, the researchers describe in detail a graduate student's entrance into the language community of his academic program, and particularly his struggle learning the writing conventions of this community. They make conclusions as to why certain things might have affected the graduate student, "Nate," in certain ways, but they are unable to generalize their findings to all graduate students in rhetoric Ph.D. programs. It is simply one study of one person in one program. However, from the level of detail the researchers provide, readers can take certain aspects of Nate's experience and apply them to other contexts and situations. This is transferability. First-year graduate students who read the Berkenhotter, Huckin, and Ackerman study may recognize similarities in their own situation while professors may recognize difficulties their students are having and understand these difficulties a bit better. The researchers do not claim that their results apply to other situations. Instead, they report their findings and make suggestions about possible causes for Nate's difficulties and eventual success. Readers then look at their own situation and decide if these causes may or may not be relevant.
When designing a study researchers have to consider their goals: Do they want to provide limited information about a broad group in order to indicate trends or patterns? Or do they want to provide detailed information about one person or small group that might suggest reasons for a particular behavior? The method they choose will determine the extent to which their results can be transferred since transferability is more applicable to certain kinds of research methods than others.
Thick Description: When writing up the results of a study, it is important that the researcher provide specific information about and a detailed description of her subject(s), location, methods, role in the study, etc. This is commonly referred to as "thick description" of methods and findings; it is important because it allows readers to make an informed judgment about whether they can transfer the findings to their own situation. For example, if an educator conducts an ethnography of her writing classroom, and finds that her students' writing improved dramatically after a series of student-teacher writing conferences, she must describe in detail the classroom setting, the students she observed, and her own participation. If the researcher does not provide enough detail, it will be difficult for readers to try the same strategy in their own classrooms. If the researcher fails to mention that she conducted this research in a small, upper-class private school, readers may transfer the results to a large, inner-city public school expecting a similar outcome.
The Reader's Role: The role of readers in transferability is to apply the methods or results of a study to their own situation. In doing so, readers must take into account differences between the situation outlined by the researcher and their own. If readers of the Berkenhotter, Huckin, and Ackerman study are aware that the research was conducted in a small, upper-class private school, but decide to test the method in a large inner-city public school, they must make adjustments for the different setting and be prepared for different results.
Likewise, readers may decide that the results of a study are not transferable to their own situation. For example, if a study found that watching more than 30 hours of television a week resulted in a worse GPA for graduate students in physics, graduate students in broadcast journalism may conclude that these results do not apply to them.
Readers may also transfer only certain aspects of the study and not the entire conclusion. For example, in the Berkenhotter, Huckin, and Ackerman study, the researchers suggest a variety of reasons for why the graduate student studied experienced difficulties adjusting to his Ph.D. program. Although composition instructors cannot compare "Nate" to first-year college students in their composition class, they could ask some of the same questions about their own class, offering them insight into some of the writing difficulties the first-year undergraduates are experiencing. It is up to readers to decide what findings are important and which may apply to their own situation; if researchers fulfill their responsibility to provide "thick description," this decision is much easier to make.
Understanding research results can help us understand why and how something happens. However, many researchers believe that such understanding is difficult to achieve in relation to human behaviors which they contend are too difficult to understand and often impossible to predict. "Because of the many and varied ways in which individuals differ from each other and because these differences change over time, comprehensive and definitive experiments in the social sciences are not possible...the most we can ever realistically hope to achieve in educational research is not prediction and control but rather only temporary understanding" (Cziko, 1993, p. 10).
Cziko's point is important because transferability allows for "temporary understanding." Instead of applying research results to every situation that may occur in the future, we can apply a similar method to another, similar situation, observe the new results, apply a modified version to another situation, and so on. Transferability takes into account the fact that there are no absolute answers to given situations; rather, every individual must determine their own best practices. Transferring the results of research performed by others can help us develop and modify these practices. However, it is important for readers of research to be aware that results cannot always be transferred; a result that occurs in one situation will not necessarily occur in a similar situation. Therefore, it is critical to take into account differences between situations and modify the research process accordingly.
Although transferability seems to be an obvious, natural, and important method for applying research results and conclusions, it is not perceived as a valid research approach in some academic circles. Perhaps partly in response to critics, in many modern research articles, researchers refer to their results as generalizable or externally valid. Therefore, it seems as though they are not talking about transferability. However, in many cases those same researchers provide direction about what points readers may want to consider, but hesitate to make any broad conclusions or statements. These are characteristics of transferable results.
Generalizability is actually, as we have seen, quite different from transferability. Unfortunately, confusion surrounding these two terms can lead to misinterpretation of research results. Emphasis on the value of transferable results -- as well as a clear understanding among researchers in the field of English of critical differences between the conditions under which research can be generalized, transferred, or, in some cases, both generalized and transferred -- could help qualitative researchers avoid some of the criticisms launched by skeptics who question the value of qualitative research methods.
Generalizability and Transferability: Synthesis
Generalizability allows us to form coherent interpretations in any situation, and to act purposefully and effectively in daily life. Transferability gives us the opportunity to sort through given methods and conclusions to decide what to apply to our own circumstances. In essence, then, both generalizability and transferability allow us to make comparisons between situations. For example, we can generalize that most people in the United States will drive on the right side of the road, but we cannot transfer this conclusion to England or Australia without finding ourselves in a treacherous situation. It is important, therefore, to always consider context when generalizing or transferring results.
Whether a study emphasizes transferability or generalizability is closely related to the goals of the researcher and the needs of the audience. Studies done for a magazine such as Time or a daily newspaper tend towards generalizability, since the publishers want to provide information relevant to a large portion of the population. A research project pointed toward a small group of specialists studying a similar problem may emphasize transferability, since specialists in the field have the ability to transfer aspects of the study results to their own situations without overt generalizations provided by the researcher. Ultimately, the researcher's subject, audience, and goals will determine the method the researcher uses to perform a study, which will then determine the transferability or generalizability of the results.
A Comparison of Generalizability and Transferability
Although generalizability has been a preferred method of research for quite some time, transferability is relatively a new idea. In theory, however, it has always accompanied research issues. It is important to note that generalizability and transferability are not necessarily mutually exclusive; they can overlap.
From an experimental study to a case study, readers transfer the methods, results, and ideas from the research to their own context. Therefore, a generalizable study can also be transferable. For example, a researcher may generalize the results of a survey of 350 people in a university to the university population as a whole; readers of the results may apply, or transfer, the results to their own situation. They will ask themselves, basically, if they fall into the majority or not. However, a transferable study is not always generalizable. For example, in case studies , transferability allows readers the option of applying results to outside contexts, whereas generalizability is basically impossible because one person or a small group of people is not necessarily representative of the larger population.
Controversy, Worth, and Function
Research in the natural sciences has a long tradition of valuing empirical studies; experimental investigation has been considered "the" way to perform research. As social scientists adapted the methods of natural science research to their own needs, they adopted this preference for empirical research. Therefore, studies that are generalizable have long been thought to be more worthwhile; the value of research was often determined by whether a study was generalizable to a population as a whole. However, more and more social scientists are realizing the value of using a variety of methods of inquiry, and the value of transferability is being recognized.
It is important to recognize that generalizability and transferability do not alone determine a study's worth. They perform different functions in research, depending on the topic and goals of the researcher. Where generalizable studies often indicate phenomena that apply to broad categories such as gender or age, transferability can provide some of the how and why behind these results.
However, there are weaknesses that must be considered. Researchers can study a small group that is representative of a larger group and claim that it is likely that their results are applicable to the larger group, but it is impossible for them to test every single person in the larger group. Their conclusions, therefore, are only valid in relation to their own studies. Another problem is that a non-representative group can lead to a faulty generalization. For example, a study of composition students'; revision capabilities which compared students' progress made during a semester in a computer classroom with progress exhibited by students in a traditional classroom might show that computers do aid students in the overall composing process. However, if it were discovered later that an unusually high number of students in the traditional classrooms suffered from substance abuse problems outside of the classroom, the population studied would not be considered representative of the student population as a whole. Therefore, it would be problematic to generalize the results of the study to a larger student population.
In the case of transferability, readers need to know as much detail as possible about a research situation in order to accurately transfer the results to their own. However, it is impossible to provide an absolutely complete description of a situation, and missing details may lead a reader to transfer results to a situation that is not entirely similar to the original one.
Applications to Research Methods
The degree to which generalizability and transferability are applicable differs from methodology to methodology as well as from study to study. Researchers need to be aware of these degrees so that results are not undermined by over-generalizations, and readers need to ensure that they do not read researched results in such a way that the results are misapplied or misinterpreted.
Applications of Transferability and Generalizability: Case Study
Research Design Case studies examine individuals or small groups within a specific context. Research is typically gathered through qualitative means: interviews, observations, etc. Data is usually analyzed either holistically or by coding methods.
Assumptions In research involving case studies, a researcher typically assumes that the results will be transferable. Generalizing is difficult or impossible because one person or small group cannot represent all similar groups or situations. For example, one group of beginning writing students in a particular classroom cannot represent all beginning student writers. Also, conclusions drawn in case studies are only about the participants being observed. With rare exceptions, case studies are not meant to establish cause/effect relationships between variables. The results of a case study are transferable in that researchers "suggest further questions, hypotheses, and future implications," and present the results as "directions and questions" (Lauer & Asher 32).
Example In order to illustrate the writing skills of beginning college writers, a researcher completing a case study might single out one or more students in a composition classroom and set about talking to them about how they judge their own writing as well as reading actual papers, setting up criteria for judgment, and reviewing paper grades/teacher interpretation.
Results of a Study In presenting the results of the previous example, a researcher should define the criteria that were established in order to determine what the researcher meant by "writing skills," provide noteworthy quotes from student interviews, provide other information depending on the kinds of research methods used (e.g., surveys, classroom observation, collected writing samples), and include possibilities for furthering this type of research. Readers are then able to assess for themselves how the researcher's observations might be transferable to other writing classrooms.
Applications of Transferability and Generalizability: Ethnography
Research Design Ethnographies study groups and/or cultures over a period of time. The goal of this type of research is to comprehend the particular group/culture through observer immersion into the culture or group. Research is completed through various methods, which are similar to those of case studies, but since the researcher is immersed within the group for an extended period of time, more detailed information is usually collected during the research. (Jonathon Kozol's "There Are No Children Here" is a good example of this.)
Assumptions As with case studies, findings of ethnographies are also considered to be transferable. The main goals of an ethnography are to "identify, operationally define, and interrelate variables" within a particular context, which ultimately produce detailed accounts or "thick descriptions" (Lauer & Asher 39). Unlike a case study, the researcher here discovers many more details. Results of ethnographies should "suggest variables for further investigation" and not generalize beyond the participants of a study (Lauer & Asher 43). Also, since analysts completing this type of research tend to rely on multiple methods to collect information (a practice also referred to as triangulation), their results typically help create a detailed description of human behavior within a particular environment.
Example The Iowa Writing Program has a widespread reputation for producing excellent writers. In order to begin to understand their training, an ethnographer might observe students throughout their degree program. During this time, the ethnographer could examine the curriculum, follow the writing processes of individual writers, and become acquainted with the writers and their work. By the end of a two year study, the researcher would have a much deeper understanding of the unique and effective features of the program.
Results of a Study Obviously, the Iowa Writing Program is unique, so generalizing any results to another writing program would be problematic. However, an ethnography would provide readers with insights into the program. Readers could ask questions such as: what qualities make it strong and what is unique about the writers who are trained within the program? At this point, readers could attempt to "transfer" applicable knowledge and observations to other writing environments.
Applications of Transferability and Generalizability: Experimental Research
Research Design A researcher working within this methodology creates an environment in which to observe and interpret the results of a research question. A key element in experimental research is that participants in a study are randomly assigned to groups. In an attempt to create a causal model (i.e., to discover the causal origin of a particular phenomenon), groups are treated differently and measurements are conducted to determine if different treatments appear to lead to different effects.
Assumptions Experimental research is usually thought to be generalizable. This methodology explores cause/effect relationships through comparisons among groups (Lauer & Asher 152). Since participants are randomly assigned to groups, and since most experiments involve enough individuals to reasonably approximate the populations from which individual participants are drawn, generalization is justified because "over a large number of allocations, all the groups of subjects will be expected to be identical on all variables" (155).
Example A simplified example: Six composition classrooms are randomly chosen (as are the students and instructors) in which three instructors incorporate the use of electronic mail as a class activity and three do not. When students in the first three classes begin discussing their papers through e-mail and, as a result, make better revisions to their papers than students in the other three classes, a researcher is likely to conclude that incorporating e-mail within a writing classroom improves the quality of students' writing.
Results of a Study Although experimental research is based on cause/effect relationships, "certainty" can never be obtained, but rather results are "probabilistic" (Lauer and Asher 161). Depending on how the researcher has presented the results, they are generalizable in that the students were selected randomly. Since the quality of writing improved with the use of e-mail within all three classrooms, it is probable that e-mail is the cause of the improvement. Readers of this study would transfer the results when they sorted out the details: Are these students representative of a group of students with which the reader is familiar? What types of previous writing experiences have these students had? What kind of writing was expected from these students? The researcher must have provided these details in order for the results to be transferable.
Applications of Transferability and Generalizability: Survey
Research Design The goal of a survey is to gain specific information about either a specific group or a representative sample of a particular group. Survey respondents are asked to respond to one or more of the following kinds of items: open-ended questions, true-false questions, agree-disagree (or Likert) questions, rankings, ratings, and so on. Results are typically used to understand the attitudes, beliefs, or knowledge of a particular group.
Assumptions Assuming that care has been taken in the development of the survey items and selection of the survey sample and that adequate response rates have been achieved, surveys results are generalizable. Note, however, that results from surveys should be generalized only to the population from which the survey results were drawn.
Example For instance, a survey of Colorado State University English graduate students undertaken to determine how well French philosopher/critic Jacques Derrida is understood before and after students take a course in critical literary theory might inform professors that, overall, Derrida's concepts are understood and that CSU's literary theory class, E615, has helped students grasp Derrida's ideas.
Results of a Study The generalizability of surveys depends on several factors. Whether distributed to a mass of people or a select few, surveys are of a "personal nature and subject to distortion." Survey respondents may or may not understand the questions being asked of them. Depending on whether or not the survey designer is nearby, respondents may or may not have the opportunity to clarify their misunderstandings.
It is also important to keep in mind that errors can occur at the development and processing levels. A researcher may inadequately pose questions (that is, not ask the right questions for the information being sought), disrupt the data collection (surveying certain people and not others), and distort the results during the processing (misreading responses and not being able to question the participant, etc.). One way to avoid these kinds of errors is for researchers to examine other studies of a similar nature and compare their results with results that have been obtained in previous studies. This way, any large discrepancies will be exposed. Depending on how large those discrepancies are and what the context of the survey is, the results may or may not be generalizable. For example, if an improved understanding of Derrida is apparent after students complete E615, it can be theorized that E615 effectively teaches students the concepts of Derrida. Issues of transferability might be visible in the actual survey questions themselves; that is, they could provide critical background information readers might need to know in order to transfer the results to another context.
The Qualitative versus Quantitative Debate
In Miles and Huberman's 1994 book Qualitative Data Analysis , quantitative researcher Fred Kerlinger is quoted as saying, "There's no such thing as qualitative data. Everything is either 1 or 0" (p. 40). To this another researcher, D. T. Campbell, asserts "all research ultimately has a qualitative grounding" (p. 40). This back and forth banter among qualitative and quantitative researchers is "essentially unproductive" according to Miles and Huberman. They and many other researchers agree that these two research methods need each other more often than not. However, because typically qualitative data involves words and quantitative data involves numbers, there are some researchers who feel that one is better (or more scientific) than the other. Another major difference between the two is that qualitative research is inductive and quantitative research is deductive. In qualitative research, a hypothesis is not needed to begin research. However, all quantitative research requires a hypothesis before research can begin.
Another major difference between qualitative and quantitative research is the underlying assumptions about the role of the researcher. In quantitative research, the researcher is ideally an objective observer that neither participates in nor influences what is being studied. In qualitative research, however, it is thought that the researcher can learn the most about a situation by participating and/or being immersed in it. These basic underlying assumptions of both methodologies guide and sequence the types of data collection methods employed.
Although there are clear differences between qualitative and quantitative approaches, some researchers maintain that the choice between using qualitative or quantitative approaches actually has less to do with methodologies than it does with positioning oneself within a particular discipline or research tradition. The difficulty of choosing a method is compounded by the fact that research is often affiliated with universities and other institutions. The findings of research projects often guide important decisions about specific practices and policies. The choice of which approach to use may reflect the interests of those conducting or benefitting from the research and the purposes for which the findings will be applied. Decisions about which kind of research method to use may also be based on the researcher's own experience and preference, the population being researched, the proposed audience for findings, time, money, and other resources available (Hathaway, 1995).
Some researchers believe that qualitative and quantitative methodologies cannot be combined because the assumptions underlying each tradition are so vastly different. Other researchers think they can be used in combination only by alternating between methods: qualitative research is appropriate to answer certain kinds of questions in certain conditions and quantitative is right for others. And some researchers think that both qualitative and quantitative methods can be used simultaneously to answer a research question.
To a certain extent, researchers on all sides of the debate are correct: each approach has its drawbacks. Quantitative research often "forces" responses or people into categories that might not "fit" in order to make meaning. Qualitative research, on the other hand, sometimes focuses too closely on individual results and fails to make connections to larger situations or possible causes of the results. Rather than discounting either approach for its drawbacks, though, researchers should find the most effective ways to incorporate elements of both to ensure that their studies are as accurate and thorough as possible.
It is important for researchers to realize that qualitative and quantitative methods can be used in conjunction with each other. In a study of computer-assisted writing classrooms, Snyder (1995) employed both qualitative and quantitative approaches. The study was constructed according to guidelines for quantitative studies: the computer classroom was the "treatment" group and the traditional pen and paper classroom was the "control" group. Both classes contained subjects with the same characteristics from the population sampled. Both classes followed the same lesson plan and were taught by the same teacher in the same semester. The only variable used was the computers. Although Snyder set this study up as an "experiment," she used many qualitative approaches to supplement her findings. She observed both classrooms on a regular basis as a participant-observer and conducted several interviews with the teacher both during and after the semester. However, there were several problems in using this approach: the strict adherence to the same syllabus and lesson plans for both classes and the restricted access of the control group to the computers may have put some students at a disadvantage. Snyder also notes that in retrospect she should have used case studies of the students to further develop her findings. Although her study had certain flaws, Snyder insists that researchers can simultaneously employ qualitative and quantitative methods if studies are planned carefully and carried out conscientiously.
Annotated Bibliography
Babbie, Earl R. (1979). The practice of social research . Belmont: Wadsworth Publishing Company, Inc.
A comprehensive review of social scientific research, including techniques for research. The logic behind social scientific research is discussed.
Berkenkotter, C., Huckin, T.N., & Ackerman, J. (1988). Conventions, conversations, and the writer: Case study of a student in a rhetoric Ph.D. program. Research in the Teaching of English 22 (1), 9-44.
Describes a case study of a beginning student in a Ph.D. program. Looks at the process of his entry into an academic discourse community.
Black, Susan. (1996). Redefining the teacher's role. Executive Educator,18 (8), 23-26.
Discusses the value of well-trained teacher-researchers performing research in their classrooms. Notes that teacher-research focuses on the particular; it does not look for broad, generalizable principles.
Blank, Steven C. (1984). Practical business research methods . Westport: AVI Publishing Company, Inc.
A comprehensive book of how to set up a research project, collect data, and reach and report conclusions.
Bridges, David. (1993). Transferable Skills: A Philosophical Perspective. Studies in Higher Education 18 (1), 43-51.
Transferability of skills in learning is discussed, focusing on the notions of cross-disciplinary, generic, core, and transferable skills and their role in the college curriculum.
Brookhart, Susan M. & Rusnak, Timothy G. (1993). A pedagogy of enrichment, not poverty: Successful lessons of exemplary urban teachers. Journal of Teacher Education, 44 (1), 17-27.
Reports the results of a study that explored the characteristics of effective urban teachers in Pittsburgh. Suggests that the results may be transferable to urban educators in other contexts.
Bryman, Alan. (1988). Quantity and quality in social research . Boston: Unwin Hyman Ltd.
Butcher, Jude. (1994, July). Cohort and case study components in teacher education research. Paper presented at the annual conference of the Australian Teacher Education Association, Brisbane, Queensland, Australia.
Argues that studies of teacher development will be more generalizable if a broad set of methods are used to collect data, if the data collected is both extensive and intensive, and if the methods used take into account the differences in people and situations being studied.
Carter, Duncan. (1993). Critical thinking for writers: Transferable skills or discipline-specific strategies? Composition Studies/Freshman English News, 21 (1), 86-93.
Questions the context-dependency of critical thinking, and whether critical thinking skills are transferable to writing tasks.
Carter, Kathy. (1993). The place of story in the study of teaching and teacher education. Educational Researcher, 22 (1), 5-12.
Discusses the advantages of story-telling in teaching and teacher education, but cautions instructors, who are currently unfamiliar with story-telling in current pedagogical structures, to be careful in implementing this method in their teaching.
Clonts, Jean G. (1992, January). The concept of reliability as it pertains to data from qualitative studies. Paper presented at the annual meeting of the Southwest Educational Research Association, Houston, TX.
Presents a review of literature on reliability in qualitative studies and defines reliability as the extent to which studies can be replicated by using the same methods and getting the same results. Strategies to enhance reliability through study design, data collection, and data analysis are suggested. Generalizability as an estimate of reliability is also explored.
Connelly, Michael F. & Clandinin D. Jean. (1990). Stories of experience and narrative inquiry. Educational Researcher, 19. (5), 2-14.
Describes narrative as a site of inquiry and a qualitative research methodology in which experiences of observer and observed interact. This form of research necessitates the development of new criteria, which may include apparency, verisimilitude, and transferability (7).
Crocker, Linda & Algina, James. (1986). Introduction to classical & modern test theory. New York: Holt, Rinehart and Winston.
Discusses test theory and its application to psychometrics. Chapters range from general overview of major issues to statistical methods and application.
Cronbach, Lee J. et al. (1967). The dependability of behavioral measurements: multifaceted studies of generalizability. Stanford: Stanford UP.
A technical research report that includes statistical methodology in order to contrast multifaceted generalizability with classical reliability.
Cziko, Gary A. (1992). Purposeful behavior as the control of perception: implications for educational research. Educational Researcher, 21 (9), 10-18. El-Hassan, Karma. (1995). Students' Rating of Instruction: Generalizability of Findings. Studies in Educational Research 21 (4), 411-29.
Issues of dimensionality, validity, reliability, and generalizability of students' ratings of instruction are discussed in relation to a study in which 610 college students who evaluated their instructors on the Teacher Effectiveness Scale.
Feingold, Alan. (1994). Gender differences in variability in intellectual abilities: a cross-cultural perspective. Sex Roles: A Journal of Research 20 (1-2), 81-93.
Feingold conducts a cross-cultural quantitative review of contemporary findings of gender differences in variability in verbal, mathematical, and spatial abilities to assess the generalizability of U.S. findings that males are more variable than females in mathematical and spatial abilities, and the sexes are equally variable in verbal ability.
Firestone,William A. (1993). Alternative arguments for generalizing from data as applied to qualitative research. Educational Researcher, 22 (4), 16-22.
Focuses on generalization in three areas of qualitative research: sample to population extrapolation, analytic generalization, and case-to-case transfer (16). Explains underlying principles, related theories, and criteria for each approach.
Fyans, Leslie J. (Ed.). (1983). Generalizability theory: Inferences and practical applications. In New Directions for Testing and Measurement: Vol. 18. San Francisco: Jossey-Bass.
A collection of articles on generalizability theory. The goal of the book is to present different aspects and applications of generalizability theory in a way that allows the reader to apply the theory.
Hammersley, Martyn. (Ed.). (1993). Social research: Philosophy, politics and practice. Newbury Park, CA: Sage Publications.
A collection of articles that provide an overview of positivism; includes an article on increasing the generalizability of qualitative research by Janet Ward Schofield.
Hathaway, R. (1995). Assumptions underlying quantitative and qualitative research: Implications for institutional research. Research in higher education, 36 (5), 535-562.
Hathaway says that the choice between using qualitative or quantitative approaches is less about methodology and more about aligning oneself with particular theoretical and academic traditions. He concluded that the two approaches address questions in very different ways, each one having its own advantages and drawbacks.
Heck, Ronald H., Marcoulides, George A. (1996). . Research in the Teaching of English 22 (1), 9-44.
Hipps, Jerome A. (1993). Trustworthiness and authenticity: Alternate ways to judge authentic assessments. Paper presented at the annual meeting of the American Educational Research Association, Atlanta, GA.
Contrasts the foundational assumptions of the constructivist approach to traditional research and the positivist approach to authentic assessment in relation to generalizability and other research issues.
Howe, Kenneth & Eisenhart, Margaret. (1990). Standards for qualitative (and quantitative) research: A prolegomenon. Educational Researcher, 19 (4), 2-9.
Huang, Chi-yu, et al. (1995, April). A generalizability theory approach to examining teaching evaluation instruments completed by students. Paper presented at the annual meeting of the American Educational Research Association, San Francisco, CA.
Presents the results of a study that used generalizability theory to investigate the reasons for variability in a teacher and course evaluation mechanism.
Hungerford, Harold R. et al. (1992). Investigating and Evaluating Environmental Issues and Actions: Skill Development Modules .
A guide designed to teach students how to investigate and evaluate environmental issues and actions. The guide is presented in six modules including information collecting and surveys, questionnaires, and opinionnaires.
Jackson, Philip W. (1990). The functions of educational research. Educational Researcher 19 (7), 3-9. Johnson, Randell G. (1993, April). A validity generalization study of the multiple assessment and program services test. Paper presented at the annual meeting of the American Educational Research Association, Atlanta, GA.
Presents results of study of validity reports of the Multiple Assessment and Program Services Test using quantitative analysis to determine the generalizability of the results.
Jones, Elizabeth A & Ratcliff, Gary. (1993). Critical thinking skills for college students. (National Center on Postsecondary Teaching, Learning, and Asessment). University Park, PA.
Reviews research literature exploring the nature of critical thinking; discusses the extent to which critical thinking is generalizable across disciplines.
Karpinski, Jakub. (1990). Causality in Sociological Research . Boston: Kluwer Academic Publishers.
Discusses causality and causal analysis in terms of sociological research. Provides equations and explanations.
Kirsch, Irwin S. & Jungeblut, Ann. (1995). Using large-scale assessment results to identify and evaluate generalizable indicators of literacy. (National Center on Adult Literacy, Publication No. TR94-19). Philadelphia, PA.
Reports analysis of data collected during an extensive literacy survey in order to help understand the different variables involved in literacy proficiency. Finds that literacy skills can be predicted across large, heterogeneous populations, but not as effectively across homogeneous populations.
Lauer, Janice M. & Asher, J. William. (1988). Composition research: empirical designs. New York: Oxford Press.
Explains the selection of subjects, formulation of hypotheses or questions, data collection, data analysis, and variable identification through discussion of each design.
LeCompte, Margaret & Goetz, Judith Preissle. (1982). Problems of reliability and validity in ethnographic research. Review of Educational Research, 52 (1), 31-60.
Concentrates on educational research and ethnography and shows how to better take reliability and validity into account when doing ethnographic research.
Marcoulides, George; Simkin, Mark G. (1991). Evaluating student papers: the case for peer review. Journal of Education for Business 67 (2), 80-83.
A preprinted evaluation form and generalizability theory are used to judge the reliability of student grading of their papers.
Maxwell, Joseph A. (1992). Understanding and validity in qualitative research. Harvard Educational Review, 62 (3), 279-300.
Explores the five types of validity used in qualitative research, including generalizable validity, and examines possible threats to research validity.
McCarthy, Christine L. (1996, Spring). What is "critical thinking"? Is it generalizable? Educational Theory, 46 217-239.
Reviews, compares and contrasts a selection of essays from Stephen P. Norris' book The Generalizability of Critical Thinking: Multiple Perspectives on an Education Ideal in order to explore the diversity of the topic of critical thinking.
Miles, Matthew B. & Huberman, A. Michael. (1994). Qualitative data analysis. Thousand Oaks: Sage Publications.
A comprehensive review of data analysis. Subjects range from collecting data to producing an actual report.
Minium, Edward W. & King, M. Bruce, & Bear, Gordon. (1993). Statistical reasoning in psychology and education . New York: John Wiley & Sons, Inc.
A textbook designed to teach students about statistical data and theory.
Moss, Pamela A. (1992). Shifting conceptions of validity in educational measurement: Implications for performance assessment. Review of Educational Research, 62 (3), 229-258. Nachmias, David & Nachmias, Chava . (1981). Research methods in the social sciences. New York: St. Martin's Press.
Discusses the foundations of empirical research, data collection, data processing and analysis, inferential methods, and the ethics of social science research.
Nagy, Philip; Jarchow, Elaine McNally. (1981). Estimating variance components of essay ratings in a complex design. Speech/Conference Paper .
This paper discusses variables influencing written composition quality and how they can be best controlled to improve the reliability assessment of writing ability.
Nagy, William E., Herman, Patricia A., & Anderson, Richard C. (1985). Learning word meanings from context: How broadly generalizable? (University of Illinois at Urbana-Champaign. Center for the Study of Reading, Technical Report No. 347). Cambridge, MA: Bolt, Beranek and Newman.
Reports the results of a study that investigated how students learn word meanings while reading from context. Claims that the study was designed to be generalized.
Naizer, Gilbert. (1992, January). Basic concepts in generalizability theory: A more powerful approach to evaluating reliability. Presented at the annual meeting of the Southwest Educational Research Association, Houston, TX.
Discusses how a measurement approach called generalizability theory (G-theory) is an important alternative to the more classical measurement theory that yields less useful coefficients. G-theory is about the dependability of behavioral measurements that allows the simultaneous estimation of multiple sources of error variance.
Newman, Isadore & Macdonald, Suzanne. (1993, May). Interpreting qualitative data: A methodological inquiry. Paper presented at the annual meeting of the Ohio Academy of Science, Youngstown, OH.
Issues of consistency, triangulation, and generalizability are discussed in relation to a qualitative study involving graduate student participants. The authors refute Polkinghorne's views of the generalizability of qualitative research, arguing that quantitative research is more suitable for generalizability.
Norris, Stephen P. (Ed.). (1992). The generalizability of critical thinking: multiple perspectives on an education ideal. New York: Teachers College Press. A set of essays from a variety of disciplines presenting different perspectives on the topic of the generalizability of critical thinking. The authors refer and respond to each other. Peshkin, Alan. (1993). The goodness of qualitative research. Educational Researcher, 22 (2), 23-29.
Discusses how effective qualitative research can be in obtaining desired results and concludes that it is an important tool scholars can use in their explorations. The four categories of qualitative research--description, interpretation, verification, and evaluation--are examined.
Rafilson, Fred. (1991, July). The case for validity generalization.
Describes generalization as a quantitative process. Briefly discusses theory, method, examples, and applications of validity generalization, emphasizing unseen local methodological problems.
Rhodebeck, Laurie A. The structure of men's and women's feminist orientations: feminist identity and feminist opinion. Gender & Society 10 (4), 386-404.
This study considers two problems: the extent to which feminist opinions are distinct from feminist identity and the generalizability of these separate constructs across gender and time.
Runkel, Philip J. & McGrath, E. Joseph. (1972). Research on human behavior: A systematic guide to method. New York: Holt, Rinehart and Winston, Inc.
Discusses how researchers can utilize their experiences of human behavior and apply them to research in a systematic and explicit fashion.
Salomon, Gavriel. (1991). Transcending the qualitative-quantitative debate: The analytic and systemic approaches to educational research. Educational Researcher, 20 (6), 10-18.
Examines the complex issues/variables involved in studies. Two types of approaches are explored: an Analytic Approach, which assumes internal and external issues, and a Systematic Approach, in which each component affects the whole. Also discusses how a study can never fully measure how much x affects y because there are so many inter-relations. Knowledge is applied differently within each approach.
Schrag, Francis. (1992). In defense of positivist research paradigms. Educational Researcher, 21 (5), 5-8.
Positivist critics Elliot Eisner, Fredrick Erikson, Henry Giroux, and Thomas Popkewitz are logically committed to propositions that can be tested only by means of positivist research paradigms. A definition of positivism is gathered through example. Overall, it is concluded that educational research need not aspire to be practical.
Sekaran, Uma. (1984). Research methods for managers: A skill-building approach. New York: John Wiley and Sons.
Discusses managerial approaches to conducting research in organizations. Provides understandable definitions and explanations of such methods as sampling and data analysis and interpretation.
Shadish, William R. (1995). The logic of generalization: five principles common to experiments and ethnographies. American Journal of Community Psychology 23 (3), 419-29.
Both experiments and ethnographies are highly localized, so they are often criticized for lack of generalizability. This article describes a logic of generalization that may help solve such problems.
Shavelson, Richard J. & Webb, Noreen M. (1991). Generalizability theory: A primer. Newbury Park, CA: Sage Publications.
Snyder, I. (1995). Multiple perspectives in literacy research: Integrating the quantitative and qualitative. Language and Education, 9 (1), 45-59.
This article explains a study in which the author employed quantitative and qualitative methods simultaneously to compare computer composition classrooms and traditional classrooms. Although there were some problems with integrating both approaches, Snyder says they can be used together if researchers plan carefully and use their methods thoughtfully.
Stallings, William M. (1995). Confessions of a quantitative educational researcher trying to teach qualitative research. Educational Researcher, 24 (3), 31-32.
Discusses the trials and tribulations of teaching a qualitative research course to graduate students. The author describes the successes and failings he encounters and asks colleagues for suggestions of readings for his syllabus.
Wagner, Ellen D. (1993, January). Evaluating distance learning projects: An approach for cross-project comparisons. Paper presented at the annual meeting of the Association for educational Communication and Technology, New Orleans, LA.
Describes a methodology developed to evaluate distance learning projects in a way that takes into account specific institutional issues while producing generalizable, valid and reliable results that allow for discussion among different institutions.
Yin, Robert K. (1989). Case Study Research: Design and Methods. London: Sage Publications.
A small section on the application of generalizability in regards to case studies.
Citation Information
Jeffrey Barnes, Kerri Conrad, Christof Demont-Heinrich, Mary Graziano, Dawn Kowalski, Jamie Neufeld, Jen Zamora, and Mike Palmquist. (1994-2024). Generalizability and Transferability. The WAC Clearinghouse. Colorado State University. Available at https://wac.colostate.edu/repository/writing/guides/.
Copyright Information
Copyright © 1994-2024 Colorado State University and/or this site's authors, developers, and contributors . Some material displayed on this site is used with permission.
- Open access
- Published: 14 May 2024
Research outcomes informing the selection of public health interventions and strategies to implement them: A cross-sectional survey of Australian policy-maker and practitioner preferences
- Luke Wolfenden 1 , 2 , 3 ,
- Alix Hall 1 , 2 , 3 ,
- Adrian Bauman 1 , 4 , 5 ,
- Andrew Milat 6 , 7 ,
- Rebecca Hodder 1 , 2 , 3 ,
- Emily Webb 1 ,
- Kaitlin Mooney 1 ,
- Serene Yoong 1 , 2 , 3 , 8 , 9 ,
- Rachel Sutherland 1 , 2 , 3 &
- Sam McCrabb 1 , 2 , 3
Health Research Policy and Systems volume 22 , Article number: 58 ( 2024 ) Cite this article
1 Altmetric
Metrics details
A key role of public health policy-makers and practitioners is to ensure beneficial interventions are implemented effectively enough to yield improvements in public health. The use of evidence to guide public health decision-making to achieve this is recommended. However, few studies have examined the relative value, as reported by policy-makers and practitioners, of different broad research outcomes (that is, measures of cost, acceptability, and effectiveness). To guide the conduct of research and better inform public health policy and practice, this study aimed at describing the research outcomes that Australian policy-makers and practitioners consider important for their decision-making when selecting: (a) public health interventions; (b) strategies to support their implementation; and (c) to assess the differences in research outcome preferences between policy-makers and practitioners.
An online value-weighting survey was conducted with Australian public health policy-makers and practitioners working in the field of non-communicable disease prevention. Participants were presented with a list of research outcomes and were asked to select up to five they considered most critical to their decision-making. They then allocated 100 points across these – allocating more points to outcomes perceived as more important. Outcome lists were derived from a review and consolidation of evaluation and outcome frameworks in the fields of public health knowledge translation and implementation. We used descriptive statistics to report relative preferences overall and for policy-makers and practitioners separately.
Of the 186 participants; 90 primarily identified as policy-makers and 96 as public health prevention practitioners. Overall, research outcomes of effectiveness, equity, feasibility, and sustainability were identified as the four most important outcomes when considering either interventions or strategies to implement them. Scores were similar for most outcomes between policy-makers and practitioners.
For Australian policy-makers and practitioners working in the field of non-communicable disease prevention, outcomes related to effectiveness, equity, feasibility, and sustainability appear particularly important to their decisions about the interventions they select and the strategies they employ to implement them. The findings suggest researchers should seek to meet these information needs and prioritize the inclusion of such outcomes in their research and dissemination activities. The extent to which these outcomes are critical to informing the decision of policy-makers and practitioners working in other jurisdictions or contexts warrants further investigation.
Peer Review reports
Research evidence has a key role in public health policy-making [ 1 ]. Consideration of research is important to maximize the potential impact of investments in health policies and services. Public health policy-makers and practitioners frequently seek out research to inform their professional decision-making [ 2 ]. However, they report that published research is not well aligned with their evidence needs [ 3 , 4 ]. Public health decision-making is a complex and dynamic process where evidence is used in a variety of ways, and for different purposes [ 3 , 5 , 6 ]. Ensuring research meets the evidence needs of public health policy-makers and practitioners is, therefore, an important strategy to improve its use in decision-making [ 7 , 8 , 9 , 10 ].
“Research outcomes” are broad domains or constructs measured to evaluate the impacts of health policies, practices or interventions, such as their effectiveness or acceptability. They are distinct from “outcome measures”, which are the measures selected to assess an outcome. Outcome measures require detailed specification of measurement parameters, including the measurement techniques and instrument, and consideration of the suitability of its properties (for example, validity) given the research question. The inclusion of research outcomes considered most relevant to public health policy-makers and practitioners is one way in which researchers can support evidence-informed decision-making.
Policy-makers are primarily responsible for developing public health policy and selecting and resourcing health programs. Practitioners are primarily responsible for supporting their implementation. As such, public health policy-makers and practitioners require research to: (i) help identify “what works” to guide the selection of interventions that will be beneficial for their community, for example, those that are effective in improving health, and acceptable to the target population and/or (ii) to help identify “how to implement” effective intervention, for example, strategies that are capable of achieving implementation at a level sufficient to accrue benefit, are affordable and reach the targeted population [ 6 , 11 ]. Research that includes outcomes relevant to these responsibilities facilitates evidence-informed decision-making by public health policy-makers and practitioners.
Initiatives such as the World Health Organization INTEGRATe Evidence (WHO INTEGRATE) framework [ 12 ], and the Grading of Recommendations Assessment, Development and Evaluation (GRADE) Evidence to Decision framework [ 13 ] have been designed to support the selection of public health interventions. Application of these frameworks required the collation and synthesis of a range of scientific evidence including studies employing qualitative and quantitative research designs. Collectively, the frameworks suggest public health policy-makers and practitioners should consider, alongside research outcomes reporting the effectiveness of a public health intervention, other research outcomes such as cost–effectiveness, potential harms and acceptability of an intervention to patients or community.
Several authors have also sought to guide outcomes researchers should include in implementation studies [ 11 ]. Proctor and colleagues defined a range of implementation research outcomes [distinct from service or clinical (intervention) effectiveness outcomes] – including intervention adoption, appropriateness, feasibility, fidelity, cost, penetration and sustainability [ 14 ]. This work helped standardize how the field of implementation science defined, measured and reported implementation outcomes. More recently McKay and colleagues put forward measures of implementation “determinants” and “outcomes” and proposed a “minimum set” of such outcomes to include in implementation and scale-up studies. The implementation research outcomes proposed by both Proctor and McKay and colleagues were developed primarily from the input of researchers to improving the quality and consistency of reporting in implementation science. However, the relative value of these outcomes to the decision-making of public health policy-makers, and in particular practitioners, has largely been unexplored.
While several studies have explored policy-maker and practitioner research evidence preferences, these have focused on a small number of potential outcomes [ 15 , 16 , 17 ]. An appraisal of the potential value, and importance of a comprehensive range of research outcomes to public health policy-maker and practitioner decision-making, therefore, is warranted. In this study, we sought to quantify the relative importance of research outcomes from the perspective of Australian public health policy-makers and practitioners working in the field of non-communicable disease prevention (hereafter referred to as “prevention” policy-makers or practitioners). Specifically, using a value-weighting methodology to elicit relative preferences, the study aimed to describe: (a) research the outcomes prevention policy-makers and practitioners regard as important to their decision-making when selecting a public health intervention to address an identified health issue, (b), research the outcomes prevention policy-makers and practitioners regard as important to their decision-making when selecting a strategy to support the implementation of a public health intervention in the community and (c) assess the differences between prevention policy-makers and practitioners regarding their research outcome preferences.
Design and setting
An online cross-sectional value-weighting survey was conducted with Australian public health prevention policy-makers and practitioners. This study was undertaken as one step of a broader program of work to establish a core outcome set that has been prospectively registered on the Core Outcome Measures in Effectiveness Trials (COMET database; https://www.comet-initiative.org/Studies/Details/1791 ).
Participant eligibility
To be eligible, participants had to self-identify as having worked as a public health prevention policy-maker or practitioner at a government or non-government health organization within the past 5 years. While the term “policy-maker” has been used to describe legislators in US studies, in Australian research it has broadly been used to describe employees of government departments (or non-government agencies) involved in the development of public health policy [ 18 , 19 , 20 , 21 , 22 ]. Policy-makers are not typically involved in the direct implementation of policy or the delivery of health services. We defined a “policy-maker” as a professional who makes decisions, plans and actions that are undertaken to achieve specific public health prevention goals on behalf of a government or non-government organization [ 23 ]. Practitioners are typically employed by government or non-government organizations responsible for prevention service provision, and are directly involved in the implementation or supporting the implementation of public health policies or programs. Specifically, we defined a “practitioner” as a professional engaged in the delivery of public health prevention programs, implementing services or models of care in health and community settings (definition developed by research team). Research and evaluation are a core competency of the public health prevention workforce in Australia [ 24 ], as it is in other countries [ 25 ]. As such, participants may be engaged in research and have published research studies. Researchers, such as those employed by academic institutions only and without an explicit public health policy or practice role in a policy or practice organization, were excluded.
Recruitment
Comprehensive methods were used to recruit individuals through several agencies. First, email invitations were distributed to Australian government health agencies at local (for example, New South Wales Local Health District Population Health units), state (for example, departments or ministries of health) and national levels, as well as to non-government organizations (for example, Cancer Council) and professional societies (for example, Public Health Association Australia). Registered practitioners with the International Union for Health Promotion and Education (IUHPE) from Australia were contacted by public domain emails or on LinkedIn (where identified) with the study invitation. Authors who had published articles of relevant topics from 2018 to 2021 within three Australian public health journals [ Australian and New Zealand Journal of Public Health ( ANZJPH ), Health Promotion Journal of Australia ( HPJA ) and Public Health Research and Practice ( PHRP )] were invited to participate in the study. Invitation emails included links to the information statement for participants and the online survey. The online survey was also promoted on the social media account of a partnering organization [National Centre of Implementation Science (NCOIS)] as well as on Twitter and LinkedIn. From these social media accounts individuals could self-select to participate in the online survey. Reminder emails were sent to non-responders at approximately 2 and 4 weeks following the initial email invitation.
Data collection and measures
The online survey was kept on servers at the Hunter Medical Research Institute, New South Wales, Australia, and deployed using the REDCap software [ 26 ], a secured web-based application for building and managing online surveys and databases. The length of the survey was approximately 20–30 min in duration.
Professional characteristics
Participants completed brief items assessing their professional role (that is, practitioner or policy-maker), the number of years’ experience as policy-makers or practitioners, their professional qualifications and the prevention risk factors (for example, smoking, nutrition, physical activity, injury, sexual health, etc.) for which they had expertise.
Valued intervention and implementation outcomes
We sought to identify outcomes that may be valued by public health policy-makers and practitioners when making decisions about what policies and/or programs of interventions to implement and how implementation could best occur. We separated outcomes on this basis, consistent with recommendations of the evidence policy and practice [ 27 ], the effectiveness–implementation research typology [ 28 , 29 ] and trial conduct and reporting guidelines [ 30 ]. This is illustrated in a broad study logic model (Fig. 1 ).
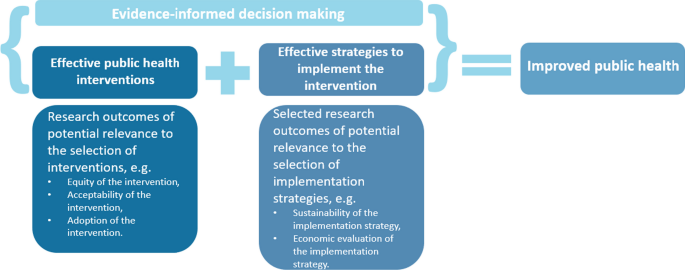
Both effective interventions and effective implementation are required to improve health outcomes
The authors undertook a review of intervention- and implementation-relevant outcome frameworks to determine program and intervention outcomes that may be of interest to policy-makers and practitioners, including the Reach, Effectiveness, Adoption, Implementation, and Maintenance (RE-AIM) Framework [ 31 , 32 ], the Intervention Scalability Assessment Tool (ISAT) [ 18 ] and Proctor and colleagues’ implementation outcome definitions [ 14 ] as well as a series of publications on the topic [ 31 , 33 , 34 , 35 , 36 , 37 , 38 , 39 , 40 , 41 , 42 , 43 ]. This was used to generate a comprehensive inventory of all possible outcomes (and outcome definitions) that may be of interest to public health policy-makers and practitioners. The outcome list was then reduced following grouping of outcomes addressing similar constructs or concepts. A panel of 16 public health policy-makers provided feedback on their perceived importance of each outcome for evidence-informed policy and practice decision-making, as well as the proposed outcome definition. This process occurred over two rounds until no further suggested improvements or clarifications were provided or requested, yielding a final list of 17 outcomes to inform the selection of public health intervention and 16 outcomes for the selection of implementation strategies (Additional file 1 : Table S1). Panel participants also pre-tested the survey instrument; however, they were not invited to participate in the value-weighting study.
Participating public health policy-makers and practitioners completed the value-weighting survey. Value-weighting surveys offer advantages over other methods to identify preferences (such as ranks or mean scores on a rating scale), as they provide an opportunity to quantify the relative preference or value of different dissemination strategies from the perspective of public health policy-makers or practitioners. Specifically, they were only presented with the list of outcomes and their definition, and were asked to select up to 5 of the 17 interventions “that they considered are critical to their decision-making when selecting a public health intervention to address an identified health issue” and 16 implementation outcomes “that they consider to be critical to their decision-making when selecting a strategy to support the implementation of a public health intervention in the community” in a decision-making context. Participants were then asked to value weight, allocating 100 points across their five (or less) intervention and implementation outcomes. A higher allocation of points represented a greater level of perceived importance. In this way, participants weight the allocation of points to outcomes based on preference. No statistical weights are applied in the analysis. Participants were asked to select up to five outcomes as this restriction forced a prioritization of the outcomes among participants. The identification of a small number of critical outcomes, rather than all relevant outcomes, is also recommended to facilitate research outcome harmonization [ 44 , 45 ].
Statistical analysis
All statistical analyses and data management were undertaken in SAS version 9.3. Descriptive statistics were used to describe the study sample. Similar to other value-weighting studies, we used descriptive analyses to identify the intervention and implementation outcomes ranked from highest to lowest importance [ 46 , 47 ]. Items not selected or allocated any points were assumed a score of 0, to reflect that they were not perceived as a high-priority outcome by the participant. Specifically, the mean points allocated to each of the individual outcomes were calculated and ranked in descending order. This was calculated overall for the entire participant sample, as well as separately by policy-makers and practitioners. As points were assigned in free-text fields, in instances where participants allocated more or less than 100 points across the individual items, the points they allocated were standardized to 100. Differences in the points allocated to each individual outcome by policy-maker/practitioner role were explored using Mann–Whitney U test. To examine any differences in the outcome preferences by participant risk factor expertise, we also examined and described outcome preferences among risk factor subgroups (with a combined sample of > 30 participants). These findings are discussed.
A total of 186 eligible participants completed the survey in part or in full.
Of the 186 participants, 90 primarily identified as policy-makers and 96 as public health prevention practitioners (Table 1 ). In all, 37% of participants (47% policy-makers, 27% practitioners) had over 15 years’ experience, and approximately one third (32% policy-makers, 36% practitioners) had a PhD. The most common areas of experience were nutrition and dietetics (38% policy-maker, 53% practitioner), physical activity or sedentary behaviour (46% policy-maker, 44% practitioner), obesity (49% policy-maker, 48% practitioner) and tobacco, alcohol or other drugs (51% policy-maker, 34% practitioner).
Valued outcomes
Intervention outcomes.
A total of 169 participants (83 policy-makers and 86 practitioners, with 7 and 10 missing, respectively) responded to the value-weighting questions for the 17 listed intervention outcomes. Table 2 (Fig. 2 ) reports the mean and standard deviation of points allocated by policy-makers and practitioners for each outcome, ranked in descending order to represent the most to least important. For policy-makers and practitioners combined, the effectiveness of an intervention, and its impact on equity, were clearly identified by participants as the leading two outcomes, with a mean allocation of 24.47 [standard deviation (SD) = 17.43] and 13.44(SD = 12.80), respectively. The mean scores for outcomes of feasibility (9.78) and sustainability (9.04) that ranked third and fourth, respectively, were similar; then scores dropped noticeably to 7.24 for acceptability and 5.81 for economic outcomes.
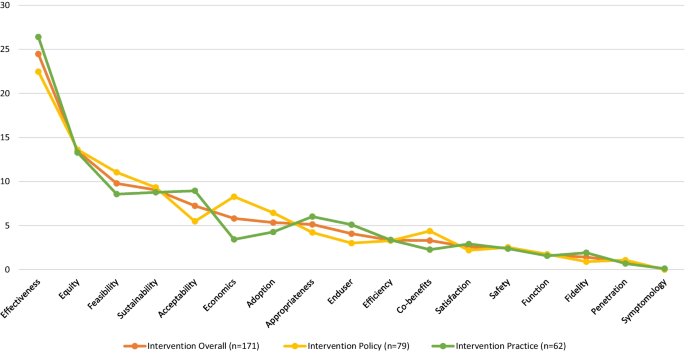
Line graph representing mean points allocated for the 17 intervention outcomes overall and by role
For most outcomes, average scores were similar for policy-makers and practitioners. However, practitioner scores for the outcome of acceptability (mean = 8.95, SD = 9.11), which ranked third most important for practitioners was significantly different than for policy-makers (mean = 5.48, SD = 9.62), where it was ranked seventh ( p = 0.005). Economics/cost outcomes were ranked fifth by policy-makers (mean = 8.28, SD = 10.63), which significantly differed from practitioners (mean = 3.43, SD = 6.56), where it was ranked ninth ( p = 0.002). For co-benefits, ranked eighth by policy-makers (mean = 4.37, SD = 7.78), scores were significantly different than for practitioners (mean = 2.27, SD = 6.49), where it was ranked thirteenth ( p = 0.0215). Rankings for the top five outcomes were identical for those with expertise in nutrition and dietetics, physical activity or sedentary behaviour, obesity and tobacco, alcohol or other drugs (Additional file 1 : Table S2).
Implementation outcomes
A total of 153 participants (75 policy-makers and 78 practitioners, with 15 and 18 missing, respectively) responded to the value-weighting questions for the 16 listed implementation outcomes (Table 3 , Fig. 3 ). The effectiveness of an implementation strategy was clearly identified by participants as the most important intervention outcome, with a mean allocation of 19.82 (SD = 16.85) overall. The mean scores for the next three ranked outcomes namely equity (mean = 10.42, SD = 12.7), feasibility (mean = 10.2, SD = 12.91) and sustainability (mean = 10.08, SD = 10.58) were similar, and thereafter, scores noticeably dropped for measures of adoption (mean = 8.55, SD = 10.90), the fifth-ranked outcome.
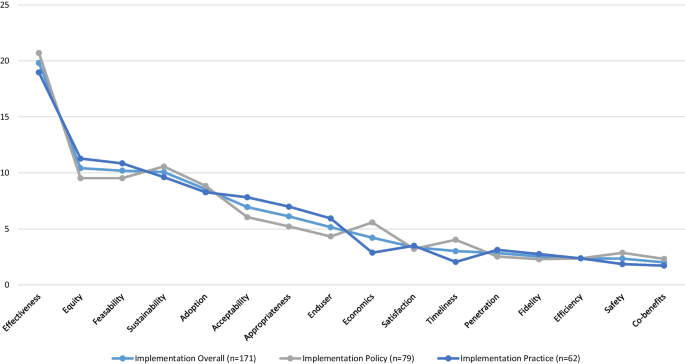
Line graph representing mean points allocated for the 16 implementation outcomes overall and by role
For most implementation outcomes (Fig. 3 ) policy-makers and practitioners scores were similar. However, economics outcomes were ranked seventh for policy-makers with a mean = 5.58 (SD = 9.25), compared with practitioners who had a ranking of eleventh for this outcome (mean = 2.88, SD = 6.67). The difference in the points allocated were statistically significant between the two groups ( p = 0.0439). Timeliness was ranked tenth most important for policy-makers, with a mean allocation of 4.03 (SD = 7.72), compared with practitioners who had a ranking of fourteenth for this outcome and a mean allocation of 2.05 (SD = 5.78). The difference in mean scores between policy-makers and practitioners on this outcome was not significant. Rankings and scores were similar for those with expertise in nutrition and dietetics, physical activity or sedentary behaviour, obesity and tobacco, alcohol or other drugs (Additional file 1 : Table S3).
Broadly, this study sought to better understand the information valued by public health policy-makers and practitioners to support their decisions regarding what and how interventions should be implemented in the community. The most valued research outcomes were the same regardless of whether policy-makers or practitioners were selecting interventions or implementation strategies. Namely outcomes regarding the effectiveness of interventions and implementation strategies. Following this, outcomes about equity, feasibility and sustainability also appeared to represent priorities. The study also found broad convergence among the most valued research outcomes, between policy-makers and practitioners, and across participants with expertise across different non-communicable disease (NCD) risk factors (for example, nutrition, obesity and tobacco). Such findings underscore the importance of research reporting these outcomes to support the translation of public health research into policy and practice.
For outcomes about decisions regarding intervention selection, the findings are broadly consistent with factors recommended by evidence-to-decision frameworks. For example, the top six ranked outcomes (effectiveness, equity, feasibility, sustainability, acceptability and economic), are also represented in both the WHO INTEGRATE framework [ 12 ] and the GRADE Evidence to Decision framework [ 13 ]. However, research outcomes about harms (adverse effects), which are included in both the WHO INTEGRATE and GRADE frameworks were ranked 13th by participants in this study. Such a finding was surprising given that potential benefits and harms of an intervention must be considered to appraise its net impact on patient or public health. Health professionals, however, do not have accurate expectations of the harms and benefits of therapeutic interventions. This appears particularly to be the case for public health professionals who acknowledge the potential for unintended consequences of policies [ 48 ] but consider these risks to be minimal [ 49 ]. The findings, therefore, may reflect the tendency of health professionals to overestimate the benefits of therapeutic interventions, and to a larger extent, underestimate harms [ 50 , 51 ]. In doing so, participants may have elevated their reported value of outcomes regarding the beneficial effectiveness of an intervention and discounted their value of outcomes reporting potential harms. Further research is warranted to substantiate this hypothesis, or explore whether other factors such as participant comprehension or misinterpretation of the outcome description may explain the finding. Nonetheless, the inclusion of measures of adverse effects (or harms) as trial outcomes is prudent to support evidence-informed public health decision-making, as is the use of strategies to facilitate risk communication to ensure the likelihood of such outcomes is understood by policy-makers and practitioners [ 52 , 53 , 54 ].
To our knowledge, this is the first study to examine the research evidence needs of public health policy-makers and practitioners when deciding on what strategies may be used to support policy or program implementation. Most of the eight implementation outcomes recommended by Proctor and colleagues [ 14 ] were ranked within the top eight by participants of this study. However, equity outcomes, ranked second by these participants, were not an outcome included in the list of outcomes defined by Proctor and colleagues. The findings may reflect public health values, which, as a discipline, has equity at its core [ 55 ]. It may also reflect the increasing attention to issues of health equity in implementation science [ 56 ].
Further, one of the eight Proctor outcomes, penetration – defined by Proctor and colleagues as the integration or saturation of an intervention within a service setting and its subsystems – was not ranked highly. Successful penetration implies a level of organization institutionalization of an intervention, which once achieved may continue to provide ongoing benefit to patients or populations. It may also suggest the capacity within the organization to expand implementation or adopt new interventions. Penetration outcomes, therefore, have been suggested to be particularly important to model and understand the potential impact of investment of scarce health resources in the implementation of public health policies and interventions [ 57 ].
At face value, such findings may suggest, at least from the perspective of public health policy-makers and practitioners, that penetration outcomes may not be particularly valued in terms of decision-making. However, it may also reflect a lack of familiarity with this term among public health policy-makers and practitioners, where related outcomes such as “reach” are more commonly used in the literature [ 14 , 58 ]. Alternatively it may be due to the conceptual similarity of this and other outcomes such as adoption, maintenance or sustainability. In other studies, for example, penetration has been operationalized to include the product of “reach”, “adoption” and “organizational maintenance” [ 58 ]. A lack of clear conceptual distinction may have led some participants to allocate points to related outcomes such as “adoption” rather than “penetration”.
The use of concept mapping techniques, consolidation of definitions of existing outcomes, and articulation of specific measures aligned to these outcomes may reduce some of these conceptual challenges. Indeed, best practice processes to develop core outcome sets for clinical trials suggest processes of engagement with end-users [ 45 ], stakeholders and researchers to articulate both broad outcomes and specific measures of these to support a shared understanding of important outcomes (and measures) to be included in such research. For example, there are many measures and economic methods to derive related to a broad outcome of “cost” (for example, absolute costs, cost–effectiveness, cost–benefit, cost–utility, and budget impact analysis) [ 59 ]. However, public health policy-makers’ preference or perceived value of these different measures to their decision-making will likely vary. While work in the field to map or align specific measures to broad outcomes is ongoing [ 57 , 58 , 60 ], extending this to empirically investigate end-user preferences for measures would be an important contribution to the field.
Broadly speaking, there was little variation in the outcomes valued between policy-makers and practitioners. However, economic evaluations were ranked as more important by policy-makers. The findings may reflect differences in the roles of Australian public health policy-makers and practitioners. That is, government policy-makers are often responsible for setting and financing the provision of public health programs, whereas health practitioners are responsible for directly supporting or undertaking their delivery. Economic considerations, therefore, may have greater primacy among policy-makers, who may be more likely to incur program costs [ 19 ]. Further research to explore and better understand these areas of divergence is warranted.
The study intended to provide information about outcomes that were generally of most use in public health policy and practice decision-making. However, such decisions are often highly contextual, and preferences may vary depending on the policy-maker or practitioner, the health issue to be addressed, the target population or broader decision-making circumstances [ 2 , 61 ]. As such, the extent to which the findings reported in this study generalize to other contexts, such as those working in different fields of public health, on different health issues or from countries or jurisdictions outside Australia is unknown. Future research examining the outcome preferences of public health policy-makers and practitioners in different contexts, therefore, is warranted.
The contextual nature of evidence needs of policy-makers and practitioners may explain, in part, the variability in outcome preferences. In many cases, for example, the mean of the outcome preference was less than its standard deviation. The interpretation of the study findings should consider this variability. That is, there is little distinguishing the mean preference ranks of many outcomes. However, the study findings at the extremes are unambiguous, suggesting clear preferences for the highest over the lowest ranking outcomes that did not differ markedly across policy-makers, practitioners or those with expertise in addressing different non-communicable disease risks such as nutrition, physical activity or tobacco or alcohol use.
Several study limitations are worth considering when interpreting the research findings. The initial inventory of outcomes was compiled from outcome frameworks, many of which were generic health or medical research outcomes that are uncommon in public health prevention research. There was considerable overlap in the outcomes included across frameworks, though how these were defined at times varied. Variability in outcome terminology has previously been identified as a problem for the field [ 62 ]. Despite being provided definitions for each, some participants may have responded to survey items based on their pre-existing understanding of these terms. Furthermore, following completion of the study, a programming error was identified whereby the definition of “Acceptability of the implementation strategy” was incorrectly assigned as “A measure of the uptake or reach of an implementation strategy”. The extent to which this may have influenced participant preferences is unclear, so sensitivity analysis was conducted by removing all participants who selected acceptability as a measure of interest. We conducted two analyses, one where the people who chose acceptability were removed but their other rankings remained and another where all their data were deleted. Results indicated that the top five outcomes did not differ after conducting the analysis, with only sustainability moving from fourth to second place in the second sensitivity analysis (Additional file 1 : Tables S4 and S5).
The pathway from research production to research in health policy or practice is complex. While a range of effective public health policies and interventions exist across a range of community settings [ 63 , 64 , 65 , 66 ], their implementation at a level capable of achieving population-level risk reductions remains elusive [ 67 , 68 , 69 , 70 ]. Nonetheless, undertaking research with end-use in mind, including reporting of outcomes valued by decision-makers, will likely facilitate the knowledge translation process [ 7 ]. In this study we found that outcomes related to effectiveness, equity, feasibility and sustainability appear important to decisions policy-makers and practitioners make about the interventions they select and the strategies they employ to implement public health prevention initiatives. Researchers interested in supporting evidence-informed decision-making should seek to provide for these information needs and prioritize such outcomes in dissemination activities to policy-makers and practitioners.
Contribution to the literature
It is essential to the research needs of policy-makers and practitioners to determine core outcomes to facilitate research use and knowledge translation.
Here we quantify the relative values of a variety of research outcomes commonly used in health research.
Findings suggest the primary outcomes of interest to public health prevention policy-makers and practitioners when making decisions about the selection of interventions and strategies to implement them are related to effectiveness, equity, feasibility and sustainability and that these do not differ markedly between public health prevention policy-makers and practitioners.
Availability of data and materials
The datasets used and/or analysed during the current study are available from the corresponding author on reasonable request.
Abbreviations
Australian and New Zealand Journal of Public Health
Health Promotion Journal of Australia
Intervention scalability assessment tool
Non-communicable disease
National Centre of Implementation Science
Public Health Research and Practice
World Health Organization
Campbell D, Moore G, Sax Institute. Increasing the use of research in policymaking. An Evidence Check rapid review brokered by the Sax Institute for the NSW Ministry of Health. 2017. https://www.health.nsw.gov.au/research/Documents/increasing-the-use-of-research.pdf .
Oliver KA, de Vocht F. Defining “evidence” in public health: a survey of policymakers’ uses and preferences. Eur J Public Health. 2017;27(2):112–7. https://doi.org/10.1093/eurpub/ckv082 .
Article PubMed Google Scholar
Newson RS, Rychetnik L, King L, Milat AJ, Bauman AE. Looking for evidence of research impact and use: a qualitative study of an Australian research-policy system. Res Eval. 2021;30(4):458–69. https://doi.org/10.1093/reseval/rvab017 .
Article Google Scholar
van der Graaf P, Cheetham M, McCabe K, Rushmer R. Localising and tailoring research evidence helps public health decision making. Health Info Libr J. 2018;35(3):202–12. https://doi.org/10.1111/hir.12219 .
World Health Organization. Evidence, policy, impact: WHO guide for evidence-informed decision-making. World Health Organization; 2021.
Global Commission on Evidence to Address Societal Challenges. The Evidence Commission Report: a wake-up call and path forward for decisionmakers, evidence intermediaries, and impact-oriented evidence producers. 2022. https://www.mcmasterforum.org/networks/evidence-commission/report/english .
Wolfenden L, Mooney K, Gonzalez S, et al. Increased use of knowledge translation strategies is associated with greater research impact on public health policy and practice: an analysis of trials of nutrition, physical activity, sexual health, tobacco, alcohol and substance use interventions. Health Res Policy Syst. 2022;20(1):15. https://doi.org/10.1186/s12961-022-00817-2 .
Article PubMed PubMed Central Google Scholar
Kathy E, David G, Anne H, et al. Improving knowledge translation for increased engagement and impact in healthcare. BMJ Open Qual. 2020;9(3): e000983. https://doi.org/10.1136/bmjoq-2020-000983 .
Squires JE, Santos WJ, Graham ID, et al. Attributes and features of context relevant to knowledge translation in health settings: a response to recent commentaries. Int J Health Policy Management. 2023;12(1):1–4. https://doi.org/10.34172/ijhpm.2023.7908 .
Thomas A, Bussières A. Leveraging knowledge translation and implementation science in the pursuit of evidence informed health professions education. Adv Health Sci Educ Theory Pract. 2021;26(3):1157–71. https://doi.org/10.1007/s10459-020-10021-y .
Dobbins M, Jack S, Thomas H, Kothari A. Public health decision-makers’ informational needs and preferences for receiving research evidence. Worldviews Evid-Based Nurs. 2007;4(3):156–63. https://doi.org/10.1111/j.1741-6787.2007.00089.x .
Rehfuess EA, Stratil JM, Scheel IB, Portela A, Norris SL, Baltussen R. The WHO-INTEGRATE evidence to decision framework version 10: integrating WHO norms and values and a complexity perspective. BMJ Glob Health. 2019;4(Suppl 1):e000844. https://doi.org/10.1136/bmjgh-2018-000844 .
Moberg J, Oxman AD, Rosenbaum S, et al. The GRADE Evidence to Decision (EtD) framework for health system and public health decisions. Health Res Policy Syst. 2018;16(1):45. https://doi.org/10.1186/s12961-018-0320-2 .
Proctor E, Silmere H, Raghavan R, et al. Outcomes for implementation research: conceptual distinctions, measurement challenges, and research agenda. Adm Policy Ment Health. 2011;38(2):65–76. https://doi.org/10.1007/s10488-010-0319-7 .
Dodson EA, Geary NA, Brownson RC. State legislators’ sources and use of information: bridging the gap between research and policy. Health Educ Res. 2015;30(6):840–8. https://doi.org/10.1093/her/cyv044 .
Morshed AB, Dodson EA, Tabak RG, Brownson RC. Comparison of research framing preferences and information use of state legislators and advocates involved in cancer control, United States, 2012–2013. Prev Chronic Dis. 2017;14:E10. https://doi.org/10.5888/pcd14.160292 .
Turon H, Wolfenden L, Finch M, et al. Dissemination of public health research to prevent non-communicable diseases: a scoping review. BMC Public Health. 2023;23(1):757. https://doi.org/10.1186/s12889-023-15622-x .
Milat A, Lee K, Conte K, et al. Intervention Scalability Assessment Tool: a decision support tool for health policy makers and implementers. Health Res Policy Syst. 2020;18(1):1–17.
Milat AJ, King L, Newson R, et al. Increasing the scale and adoption of population health interventions: experiences and perspectives of policy makers, practitioners, and researchers. Health Res Policy Syst. 2014;12(1):18. https://doi.org/10.1186/1478-4505-12-18 .
Cleland V, McNeilly B, Crawford D, Ball K. Obesity prevention programs and policies: practitioner and policy-maker perceptions of feasibility and effectiveness. Obesity. 2013;21(9):E448–55. https://doi.org/10.1002/oby.20172 .
Wolfenden L, Bolsewicz K, Grady A, et al. Optimisation: defining and exploring a concept to enhance the impact of public health initiatives. Health Res Policy Syst. 2019;17(1):108. https://doi.org/10.1186/s12961-019-0502-6 .
Purtle J, Dodson EA, Nelson K, Meisel ZF, Brownson RC. Legislators’ sources of behavioral health research and preferences for dissemination: variations by political party. Psychiatr Serv. 2018;69(10):1105–8. https://doi.org/10.1176/appi.ps.201800153 .
World Health Organization. WHO Health policy [Internet] 2019;
Australian Health Promotion Association Core competencies for health promotion practitioners. Maroochydore: University of the Sunshine Coast. 2009;
Barry MM, Battel-Kirk B, Dempsey C. The CompHP Core Competencies Framework for Health Promotion in Europe. Health Educ Behav. 2012;39(6):648–62. https://doi.org/10.1177/1090198112465620 .
Harris PA, Taylor R, Thielke R, Payne J, Gonzalez N, Conde JG. Research electronic data capture (REDCap) – a metadata-driven methodology and workflow process for providing translational research informatics support. J Biomed Inform. 2009;42(2):377–81. https://doi.org/10.1016/j.jbi.2008.08.010 .
Wolfenden L, Williams CM, Kingsland M, et al. Improving the impact of public health service delivery and research: a decision tree to aid evidence-based public health practice and research. Aust N Zeal J Public Health. 2020;44(5):331–2. https://doi.org/10.1111/1753-6405.13023 .
Curran GM, Bauer M, Mittman B, Pyne JM, Stetler C. Effectiveness-implementation hybrid designs: combining elements of clinical effectiveness and implementation research to enhance public health impact. Med Care. 2012;50(3):217–26. https://doi.org/10.1097/MLR.0b013e3182408812 .
Wolfenden L, Williams CM, Wiggers J, Nathan N, Yoong SL. Improving the translation of health promotion interventions using effectiveness–implementation hybrid designs in program evaluations. Health Promot J Austr. 2016;27(3):204–7. https://doi.org/10.1071/HE16056 .
Wolfenden L, Foy R, Presseau J, et al. Designing and undertaking randomised implementation trials: guide for researchers. Br Med J. 2021;372:m3721. https://doi.org/10.1136/bmj.m3721 .
Suhonen R, Papastavrou E, Efstathiou G, et al. Patient satisfaction as an outcome of individualised nursing care. Scand J Caring Sci. 2012;26(2):372–80. https://doi.org/10.1111/j.1471-6712.2011.00943.x .
Gaglio B, Shoup JA, Glasgow RE. The RE-AIM framework: a systematic review of use over time. Am J Public Health. 2013;103(6):e38-46. https://doi.org/10.2105/ajph.2013.301299 .
Rye M, Torres EM, Friborg O, Skre I, Aarons GA. The Evidence-based Practice Attitude Scale-36 (EBPAS-36): a brief and pragmatic measure of attitudes to evidence-based practice validated in US and Norwegian samples. Implement Sci. 2017;12(1):44. https://doi.org/10.1186/s13012-017-0573-0 .
Sansoni JE. Health outcomes: an overview from an Australian perspective. 2016;
Sekhon M, Cartwright M, Francis JJ. Acceptability of healthcare interventions: an overview of reviews and development of a theoretical framework. BMC Health Serv Res. 2017;17(1):88. https://doi.org/10.1186/s12913-017-2031-8 .
Weiner BJ, Lewis CC, Stanick C, et al. Psychometric assessment of three newly developed implementation outcome measures. Implement Sci. 2017;12(1):108. https://doi.org/10.1186/s13012-017-0635-3 .
Simoens S. Health economic assessment: a methodological primer. Int J Environ Res Public Health. 2009;6(12):2950–66. https://doi.org/10.3390/ijerph6122950 .
Lorgelly PK, Lawson KD, Fenwick EA, Briggs AH. Outcome measurement in economic evaluations of public health interventions: a role for the capability approach? Int J Environ Res Public Health. 2010;7(5):2274–89. https://doi.org/10.3390/ijerph7052274 .
Williams K, Sansoni J, Morris D, Grootemaat P, Thompson C. Patient-reported outcome measures: literature review. Sydney: Australian Commission on Safety and Quality in Health Care; 2016.
Google Scholar
Zilberberg MD, Shorr AF. Understanding cost-effectiveness. Clin Microbiol Infect. 2010;16(12):1707–12. https://doi.org/10.1111/j.1469-0691.2010.03331.x .
Article CAS PubMed Google Scholar
Feeny DH, Eckstrom E, Whitlock EP, et al. A primer for systematic reviewers on the measurement of functional status and health-related quality of life in older adults [Internet]. Rockville (MD): Agency for Healthcare Research and Quality (US); 2013. Available from: https://www.ncbi.nlm.nih.gov/books/NBK169159/ .
Glasgow RE, Vogt TM, Boles SM. Evaluating the public health impact of health promotion interventions: the RE-AIM framework. Am J Public Health. 1999;89(9):1322–7. https://doi.org/10.2105/ajph.89.9.1322 .
Article CAS PubMed PubMed Central Google Scholar
Institute of Medicine Committee on Quality of Health Care in America. Crossing the quality chasm: a new health system for the 21st century. National Academies Press (US); 2001.
Higgins JP, Thomas J, Chandler J, et al. Cochrane handbook for systematic reviews of interventions. John Wiley & Sons; 2019.
Book Google Scholar
Williamson PR, Altman DG, Bagley H, et al. The COMET Handbook: version 1.0. Trials. 2017;18(3):280. https://doi.org/10.1186/s13063-017-1978-4 .
Paul CL, Sanson-Fisher R, Douglas HE, Clinton-McHarg T, Williamson A, Barker D. Cutting the research pie: a value-weighting approach to explore perceptions about psychosocial research priorities for adults with haematological cancers. Eur J Cancer Care. 2011;20(3):345–53. https://doi.org/10.1111/j.1365-2354.2010.01188.x .
Article CAS Google Scholar
Fradgley EA, Paul CL, Bryant J, Oldmeadow C. Getting right to the point: identifying Australian outpatients’ priorities and preferences for patient-centred quality improvement in chronic disease care. Int J Qual Health Care. 2016;28(4):470–7. https://doi.org/10.1093/intqhc/mzw049 .
Oliver K, Lorenc T, Tinkler J, Bonell C. Understanding the unintended consequences of public health policies: the views of policymakers and evaluators. BMC Public Health. 2019;19(1):1057. https://doi.org/10.1186/s12889-019-7389-6 .
Sally M, Mark P. Good intentions and received wisdom are not enough. J Epidemiol Community Health. 2000;54(11):802. https://doi.org/10.1136/jech.54.11.802 .
Hoffmann TC, Del Mar C. Clinicians’ expectations of the benefits and harms of treatments, screening, and tests: a systematic review. JAMA Intern Med. 2017;177(3):407–19. https://doi.org/10.1001/jamainternmed.2016.8254 .
Hanoch Y, Rolison J, Freund AM. Reaping the benefits and avoiding the risks: unrealistic optimism in the health domain. Risk Anal. 2019;39(4):792–804. https://doi.org/10.1111/risa.13204 .
Oakley GP Jr, Johnston RB Jr. Balancing benefits and harms in public health prevention programmes mandated by governments. Br Med J. 2004;329(7456):41–3. https://doi.org/10.1136/bmj.329.7456.41 .
Pitt AL, Goldhaber-Fiebert JD, Brandeau ML. Public health interventions with harms and benefits: a graphical framework for evaluating tradeoffs. Med Decis Making. 2020;40(8):978–89. https://doi.org/10.1177/0272989x20960458 .
McDowell M, Rebitschek FG, Gigerenzer G, Wegwarth O. A simple tool for communicating the benefits and harms of health interventions: a guide for creating a fact box. MDM Policy Pract. 2016;1(1):2381468316665365. https://doi.org/10.1177/2381468316665365 .
World Health Organization. Social determinants of health. 2022.
Brownson RC, Kumanyika SK, Kreuter MW, Haire-Joshu D. Implementation science should give higher priority to health equity. Implement Sci. 2021;16(1):28. https://doi.org/10.1186/s13012-021-01097-0 .
Brownson RC, Colditz GA, Proctor EK. Dissemination and implementation research in health: translating science to practice. Oxford University Press; 2017.
Reilly KL, Kennedy S, Porter G, Estabrooks P. Comparing, contrasting, and integrating dissemination and implementation outcomes included in the RE-AIM and implementation outcomes frameworks. Front Public Health. 2020;8:430. https://doi.org/10.3389/fpubh.2020.00430 .
Eisman AB, Kilbourne AM, Dopp AR, Saldana L, Eisenberg D. Economic evaluation in implementation science: making the business case for implementation strategies. Psychiatry Res. 2020;283:112433. https://doi.org/10.1016/j.psychres.2019.06.008 .
Allen P, Pilar M, Walsh-Bailey C, et al. Quantitative measures of health policy implementation determinants and outcomes: a systematic review. Implement Sci. 2020;15(1):47. https://doi.org/10.1186/s13012-020-01007-w .
Whitty JA, Lancsar E, Rixon K, Golenko X, Ratcliffe J. A systematic review of stated preference studies reporting public preferences for healthcare priority setting. Patient. 2014;7(4):365–86. https://doi.org/10.1007/s40271-014-0063-2 .
Smith PG MR, Ross DA, editors, Field trials of health interventions: a toolbox. 3rd edition. Chapter 12, Outcome measures and case definition. 2015.
Wolfenden L, Barnes C, Lane C, et al. Consolidating evidence on the effectiveness of interventions promoting fruit and vegetable consumption: an umbrella review. Int J Behav Nutr Phys Act. 2021;18(1):11. https://doi.org/10.1186/s12966-020-01046-y .
Nathan N, Hall A, McCarthy N, et al. Multi-strategy intervention increases school implementation and maintenance of a mandatory physical activity policy: outcomes of a cluster randomised controlled trial. Br J Sports Med. 2022;56(7):385–93. https://doi.org/10.1136/bjsports-2020-103764 .
Sutherland R, Brown A, Nathan N, et al. A multicomponent mHealth-based intervention (SWAP IT) to decrease the consumption of discretionary foods packed in school lunchboxes: type I effectiveness-implementation hybrid cluster randomized controlled trial. J Med Internet Res. 2021;23(6):e25256. https://doi.org/10.2196/25256 .
Breslin G, Shannon S, Cummings M, Leavey G. An updated systematic review of interventions to increase awareness of mental health and well-being in athletes, coaches, officials and parents. Syst Rev. 2022;11(1):99. https://doi.org/10.1186/s13643-022-01932-5 .
McCrabb S, Lane C, Hall A, et al. Scaling-up evidence-based obesity interventions: a systematic review assessing intervention adaptations and effectiveness and quantifying the scale-up penalty. Obesity Rev. 2019;20(7):964–82. https://doi.org/10.1111/obr.12845 .
Wolfenden L, McCrabb S, Barnes C, et al. Strategies for enhancing the implementation of school-based policies or practices targeting diet, physical activity, obesity, tobacco or alcohol use. Cochrane Database Syst Rev. 2022. https://doi.org/10.1002/14651858.CD011677.pub3 .
Wolfenden L, Barnes C, Jones J, et al. Strategies to improve the implementation of healthy eating, physical activity and obesity prevention policies, practices or programmes within childcare services. Cochrane Database Syst Rev. 2020. https://doi.org/10.1002/14651858.CD011779.pub3 .
Sutherland RL, Jackson JK, Lane C, et al. A systematic review of adaptations and effectiveness of scaled-up nutrition interventions. Nutr Rev. 2022;80(4):962–79. https://doi.org/10.1093/nutrit/nuab096 .
Download references
Acknowledgements
Not applicable.
This study was funded in part by a National Health and Medical Research Council (NHMRC) Centre for Research Excellence – National Centre of Implementation Science (NCOIS) Grant (APP1153479) and a New South Wales (NSW) Cancer Council Program Grant (G1500708). LW is supported by an NHMRC Investigator Grant (G1901360).
Author information
Authors and affiliations.
Faculty of Health and Medicine, School of Medicine and Public Health, University of Newcastle, Newcastle, NSW, 2318, Australia
Luke Wolfenden, Alix Hall, Adrian Bauman, Rebecca Hodder, Emily Webb, Kaitlin Mooney, Serene Yoong, Rachel Sutherland & Sam McCrabb
Hunter New England Population Health, Hunter New England Local Health District, Wallsend, NSW, 2287, Australia
Luke Wolfenden, Alix Hall, Rebecca Hodder, Serene Yoong, Rachel Sutherland & Sam McCrabb
Hunter Medical Research Institute, Newcastle, NSW, 2305, Australia
Prevention Research Collaboration, Charles Perkins Centre, School of Public Health, Faculty of Medicine and Health, The University of Sydney, Sydney, NSW, Australia
Adrian Bauman
The Australian Prevention Partnership Centre, Sydney, NSW, Australia
School of Public Health, University of Sydney, Sydney, NSW, Australia
Andrew Milat
Centre for Epidemiology and Evidence, NSW Ministry of Health, Sydney, Australia
School of Health Sciences, Swinburne University of Technology, Melbourne, VIC, 3122, Australia
Serene Yoong
Global Nutrition and Preventive Health, Institute of Health Transformation, School of Health and Social Development, Deakin University, Burwood, VIC, Australia
You can also search for this author in PubMed Google Scholar
Contributions
LW and SMc led the conception and design of the study, were closely involved in data analysis and interpretation and wrote the manuscript. AH, AB, AM and RH comprised the study advisory committee, reviewed the study’s methods and assisted with survey development. AH was responsible for data analysis. KM and EW assisted with survey development, data collection and preliminary analysis. AH, AB, AM, RH, SY and RS were involved in interpretation and revised the manuscript critically for important intellectual content. All authors read and approved the final manuscript.
Corresponding author
Correspondence to Luke Wolfenden .
Ethics declarations
Ethics approval and consent to participate.
Ethics approval was provided by the University of Newcastle Human Research Ethics Committee (H-2014-0070). Implied consent was obtained from participants rather than explicit consent (that is, individuals were not required to expressly provide consent by checking a “I consent box”; rather, undertaking the survey provided implicit consent).
Consent for publication
Competing interests.
The authors declare that they have no competing interests.
Additional information
Publisher’s note.
Springer Nature remains neutral with regard to jurisdictional claims in published maps and institutional affiliations.
Supplementary Information
Additional file 1:.
Table S1. Mean point allocations for each of the 17 intervention outcomes overall and by area of expertise (where field of expertise n ≥ 30). Table S2. Mean point allocations for each of the 16 implementation outcomes overall and by area of expertise (where field of expertise n ≥ 30). Table S3. Mean points for implementation outcomes overall and by area of expertise (field of expertise n ≥ 30). Table S4. Sensitivity analysis, participants who selected ‘acceptability’ removed from the analysis, their other rankings remained. Table S5. Sensitivity analysis, participants who selected ‘acceptability’ whole data set removed from the analysis.
Rights and permissions
Open Access This article is licensed under a Creative Commons Attribution 4.0 International License, which permits use, sharing, adaptation, distribution and reproduction in any medium or format, as long as you give appropriate credit to the original author(s) and the source, provide a link to the Creative Commons licence, and indicate if changes were made. The images or other third party material in this article are included in the article's Creative Commons licence, unless indicated otherwise in a credit line to the material. If material is not included in the article's Creative Commons licence and your intended use is not permitted by statutory regulation or exceeds the permitted use, you will need to obtain permission directly from the copyright holder. To view a copy of this licence, visit http://creativecommons.org/licenses/by/4.0/ . The Creative Commons Public Domain Dedication waiver ( http://creativecommons.org/publicdomain/zero/1.0/ ) applies to the data made available in this article, unless otherwise stated in a credit line to the data.
Reprints and permissions
About this article
Cite this article.
Wolfenden, L., Hall, A., Bauman, A. et al. Research outcomes informing the selection of public health interventions and strategies to implement them: A cross-sectional survey of Australian policy-maker and practitioner preferences. Health Res Policy Sys 22 , 58 (2024). https://doi.org/10.1186/s12961-024-01144-4
Download citation
Received : 12 July 2023
Accepted : 19 April 2024
Published : 14 May 2024
DOI : https://doi.org/10.1186/s12961-024-01144-4
Share this article
Anyone you share the following link with will be able to read this content:
Sorry, a shareable link is not currently available for this article.
Provided by the Springer Nature SharedIt content-sharing initiative
Health Research Policy and Systems
ISSN: 1478-4505
- Submission enquiries: Access here and click Contact Us
- General enquiries: [email protected]
Loading metrics
Open Access
Essays are opinion pieces on a topic of broad interest to a general medical audience.
See all article types »
Why Most Published Research Findings Are False
- John P. A. Ioannidis
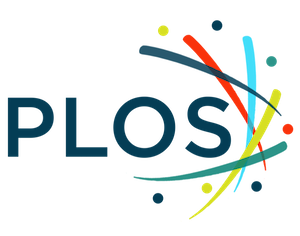
Published: August 30, 2005
- https://doi.org/10.1371/journal.pmed.0020124
- Reader Comments
25 Aug 2022: Ioannidis JPA (2022) Correction: Why Most Published Research Findings Are False. PLOS Medicine 19(8): e1004085. https://doi.org/10.1371/journal.pmed.1004085 View correction
There is increasing concern that most current published research findings are false. The probability that a research claim is true may depend on study power and bias, the number of other studies on the same question, and, importantly, the ratio of true to no relationships among the relationships probed in each scientific field. In this framework, a research finding is less likely to be true when the studies conducted in a field are smaller; when effect sizes are smaller; when there is a greater number and lesser preselection of tested relationships; where there is greater flexibility in designs, definitions, outcomes, and analytical modes; when there is greater financial and other interest and prejudice; and when more teams are involved in a scientific field in chase of statistical significance. Simulations show that for most study designs and settings, it is more likely for a research claim to be false than true. Moreover, for many current scientific fields, claimed research findings may often be simply accurate measures of the prevailing bias. In this essay, I discuss the implications of these problems for the conduct and interpretation of research.
Citation: Ioannidis JPA (2005) Why Most Published Research Findings Are False. PLoS Med 2(8): e124. https://doi.org/10.1371/journal.pmed.0020124
Copyright: © 2005 John P. A. Ioannidis. This is an open-access article distributed under the terms of the Creative Commons Attribution License, which permits unrestricted use, distribution, and reproduction in any medium, provided the original work is properly cited.
Competing interests: The author has declared that no competing interests exist.
Abbreviation: PPV, positive predictive value
Published research findings are sometimes refuted by subsequent evidence, with ensuing confusion and disappointment. Refutation and controversy is seen across the range of research designs, from clinical trials and traditional epidemiological studies [ 1–3 ] to the most modern molecular research [ 4 , 5 ]. There is increasing concern that in modern research, false findings may be the majority or even the vast majority of published research claims [ 6–8 ]. However, this should not be surprising. It can be proven that most claimed research findings are false. Here I will examine the key factors that influence this problem and some corollaries thereof.
Modeling the Framework for False Positive Findings
Several methodologists have pointed out [ 9–11 ] that the high rate of nonreplication (lack of confirmation) of research discoveries is a consequence of the convenient, yet ill-founded strategy of claiming conclusive research findings solely on the basis of a single study assessed by formal statistical significance, typically for a p -value less than 0.05. Research is not most appropriately represented and summarized by p -values, but, unfortunately, there is a widespread notion that medical research articles should be interpreted based only on p -values. Research findings are defined here as any relationship reaching formal statistical significance, e.g., effective interventions, informative predictors, risk factors, or associations. “Negative” research is also very useful. “Negative” is actually a misnomer, and the misinterpretation is widespread. However, here we will target relationships that investigators claim exist, rather than null findings.
It can be proven that most claimed research findings are false
As has been shown previously, the probability that a research finding is indeed true depends on the prior probability of it being true (before doing the study), the statistical power of the study, and the level of statistical significance [ 10 , 11 ]. Consider a 2 × 2 table in which research findings are compared against the gold standard of true relationships in a scientific field. In a research field both true and false hypotheses can be made about the presence of relationships. Let R be the ratio of the number of “true relationships” to “no relationships” among those tested in the field. R is characteristic of the field and can vary a lot depending on whether the field targets highly likely relationships or searches for only one or a few true relationships among thousands and millions of hypotheses that may be postulated. Let us also consider, for computational simplicity, circumscribed fields where either there is only one true relationship (among many that can be hypothesized) or the power is similar to find any of the several existing true relationships. The pre-study probability of a relationship being true is R /( R + 1). The probability of a study finding a true relationship reflects the power 1 - β (one minus the Type II error rate). The probability of claiming a relationship when none truly exists reflects the Type I error rate, α. Assuming that c relationships are being probed in the field, the expected values of the 2 × 2 table are given in Table 1 . After a research finding has been claimed based on achieving formal statistical significance, the post-study probability that it is true is the positive predictive value, PPV. The PPV is also the complementary probability of what Wacholder et al. have called the false positive report probability [ 10 ]. According to the 2 × 2 table, one gets PPV = (1 - β) R /( R - βR + α). A research finding is thus more likely true than false if (1 - β) R > α. Since usually the vast majority of investigators depend on a = 0.05, this means that a research finding is more likely true than false if (1 - β) R > 0.05.
- PPT PowerPoint slide
- PNG larger image
- TIFF original image
https://doi.org/10.1371/journal.pmed.0020124.t001
What is less well appreciated is that bias and the extent of repeated independent testing by different teams of investigators around the globe may further distort this picture and may lead to even smaller probabilities of the research findings being indeed true. We will try to model these two factors in the context of similar 2 × 2 tables.
First, let us define bias as the combination of various design, data, analysis, and presentation factors that tend to produce research findings when they should not be produced. Let u be the proportion of probed analyses that would not have been “research findings,” but nevertheless end up presented and reported as such, because of bias. Bias should not be confused with chance variability that causes some findings to be false by chance even though the study design, data, analysis, and presentation are perfect. Bias can entail manipulation in the analysis or reporting of findings. Selective or distorted reporting is a typical form of such bias. We may assume that u does not depend on whether a true relationship exists or not. This is not an unreasonable assumption, since typically it is impossible to know which relationships are indeed true. In the presence of bias ( Table 2 ), one gets PPV = ([1 - β] R + u β R )/( R + α − β R + u − u α + u β R ), and PPV decreases with increasing u , unless 1 − β ≤ α, i.e., 1 − β ≤ 0.05 for most situations. Thus, with increasing bias, the chances that a research finding is true diminish considerably. This is shown for different levels of power and for different pre-study odds in Figure 1 . Conversely, true research findings may occasionally be annulled because of reverse bias. For example, with large measurement errors relationships are lost in noise [ 12 ], or investigators use data inefficiently or fail to notice statistically significant relationships, or there may be conflicts of interest that tend to “bury” significant findings [ 13 ]. There is no good large-scale empirical evidence on how frequently such reverse bias may occur across diverse research fields. However, it is probably fair to say that reverse bias is not as common. Moreover measurement errors and inefficient use of data are probably becoming less frequent problems, since measurement error has decreased with technological advances in the molecular era and investigators are becoming increasingly sophisticated about their data. Regardless, reverse bias may be modeled in the same way as bias above. Also reverse bias should not be confused with chance variability that may lead to missing a true relationship because of chance.
Panels correspond to power of 0.20, 0.50, and 0.80.
https://doi.org/10.1371/journal.pmed.0020124.g001
https://doi.org/10.1371/journal.pmed.0020124.t002
Testing by Several Independent Teams
Several independent teams may be addressing the same sets of research questions. As research efforts are globalized, it is practically the rule that several research teams, often dozens of them, may probe the same or similar questions. Unfortunately, in some areas, the prevailing mentality until now has been to focus on isolated discoveries by single teams and interpret research experiments in isolation. An increasing number of questions have at least one study claiming a research finding, and this receives unilateral attention. The probability that at least one study, among several done on the same question, claims a statistically significant research finding is easy to estimate. For n independent studies of equal power, the 2 × 2 table is shown in Table 3 : PPV = R (1 − β n )/( R + 1 − [1 − α] n − R β n ) (not considering bias). With increasing number of independent studies, PPV tends to decrease, unless 1 - β < a, i.e., typically 1 − β < 0.05. This is shown for different levels of power and for different pre-study odds in Figure 2 . For n studies of different power, the term β n is replaced by the product of the terms β i for i = 1 to n , but inferences are similar.
https://doi.org/10.1371/journal.pmed.0020124.g002
https://doi.org/10.1371/journal.pmed.0020124.t003
Corollaries
A practical example is shown in Box 1 . Based on the above considerations, one may deduce several interesting corollaries about the probability that a research finding is indeed true.
Box 1. An Example: Science at Low Pre-Study Odds
Let us assume that a team of investigators performs a whole genome association study to test whether any of 100,000 gene polymorphisms are associated with susceptibility to schizophrenia. Based on what we know about the extent of heritability of the disease, it is reasonable to expect that probably around ten gene polymorphisms among those tested would be truly associated with schizophrenia, with relatively similar odds ratios around 1.3 for the ten or so polymorphisms and with a fairly similar power to identify any of them. Then R = 10/100,000 = 10 −4 , and the pre-study probability for any polymorphism to be associated with schizophrenia is also R /( R + 1) = 10 −4 . Let us also suppose that the study has 60% power to find an association with an odds ratio of 1.3 at α = 0.05. Then it can be estimated that if a statistically significant association is found with the p -value barely crossing the 0.05 threshold, the post-study probability that this is true increases about 12-fold compared with the pre-study probability, but it is still only 12 × 10 −4 .
Now let us suppose that the investigators manipulate their design, analyses, and reporting so as to make more relationships cross the p = 0.05 threshold even though this would not have been crossed with a perfectly adhered to design and analysis and with perfect comprehensive reporting of the results, strictly according to the original study plan. Such manipulation could be done, for example, with serendipitous inclusion or exclusion of certain patients or controls, post hoc subgroup analyses, investigation of genetic contrasts that were not originally specified, changes in the disease or control definitions, and various combinations of selective or distorted reporting of the results. Commercially available “data mining” packages actually are proud of their ability to yield statistically significant results through data dredging. In the presence of bias with u = 0.10, the post-study probability that a research finding is true is only 4.4 × 10 −4 . Furthermore, even in the absence of any bias, when ten independent research teams perform similar experiments around the world, if one of them finds a formally statistically significant association, the probability that the research finding is true is only 1.5 × 10 −4 , hardly any higher than the probability we had before any of this extensive research was undertaken!
Corollary 1: The smaller the studies conducted in a scientific field, the less likely the research findings are to be true. Small sample size means smaller power and, for all functions above, the PPV for a true research finding decreases as power decreases towards 1 − β = 0.05. Thus, other factors being equal, research findings are more likely true in scientific fields that undertake large studies, such as randomized controlled trials in cardiology (several thousand subjects randomized) [ 14 ] than in scientific fields with small studies, such as most research of molecular predictors (sample sizes 100-fold smaller) [ 15 ].
Corollary 2: The smaller the effect sizes in a scientific field, the less likely the research findings are to be true. Power is also related to the effect size. Thus research findings are more likely true in scientific fields with large effects, such as the impact of smoking on cancer or cardiovascular disease (relative risks 3–20), than in scientific fields where postulated effects are small, such as genetic risk factors for multigenetic diseases (relative risks 1.1–1.5) [ 7 ]. Modern epidemiology is increasingly obliged to target smaller effect sizes [ 16 ]. Consequently, the proportion of true research findings is expected to decrease. In the same line of thinking, if the true effect sizes are very small in a scientific field, this field is likely to be plagued by almost ubiquitous false positive claims. For example, if the majority of true genetic or nutritional determinants of complex diseases confer relative risks less than 1.05, genetic or nutritional epidemiology would be largely utopian endeavors.
Corollary 3: The greater the number and the lesser the selection of tested relationships in a scientific field, the less likely the research findings are to be true. As shown above, the post-study probability that a finding is true (PPV) depends a lot on the pre-study odds (R) . Thus, research findings are more likely true in confirmatory designs, such as large phase III randomized controlled trials, or meta-analyses thereof, than in hypothesis-generating experiments. Fields considered highly informative and creative given the wealth of the assembled and tested information, such as microarrays and other high-throughput discovery-oriented research [ 4 , 8 , 17 ], should have extremely low PPV.
Corollary 4: The greater the flexibility in designs, definitions, outcomes, and analytical modes in a scientific field, the less likely the research findings are to be true. Flexibility increases the potential for transforming what would be “negative” results into “positive” results, i.e., bias, u . For several research designs, e.g., randomized controlled trials [ 18–20 ] or meta-analyses [ 21 , 22 ], there have been efforts to standardize their conduct and reporting. Adherence to common standards is likely to increase the proportion of true findings. The same applies to outcomes. True findings may be more common when outcomes are unequivocal and universally agreed (e.g., death) rather than when multifarious outcomes are devised (e.g., scales for schizophrenia outcomes) [ 23 ]. Similarly, fields that use commonly agreed, stereotyped analytical methods (e.g., Kaplan-Meier plots and the log-rank test) [ 24 ] may yield a larger proportion of true findings than fields where analytical methods are still under experimentation (e.g., artificial intelligence methods) and only “best” results are reported. Regardless, even in the most stringent research designs, bias seems to be a major problem. For example, there is strong evidence that selective outcome reporting, with manipulation of the outcomes and analyses reported, is a common problem even for randomized trails [ 25 ]. Simply abolishing selective publication would not make this problem go away.
Corollary 5: The greater the financial and other interests and prejudices in a scientific field, the less likely the research findings are to be true. Conflicts of interest and prejudice may increase bias, u . Conflicts of interest are very common in biomedical research [ 26 ], and typically they are inadequately and sparsely reported [ 26 , 27 ]. Prejudice may not necessarily have financial roots. Scientists in a given field may be prejudiced purely because of their belief in a scientific theory or commitment to their own findings. Many otherwise seemingly independent, university-based studies may be conducted for no other reason than to give physicians and researchers qualifications for promotion or tenure. Such nonfinancial conflicts may also lead to distorted reported results and interpretations. Prestigious investigators may suppress via the peer review process the appearance and dissemination of findings that refute their findings, thus condemning their field to perpetuate false dogma. Empirical evidence on expert opinion shows that it is extremely unreliable [ 28 ].
Corollary 6: The hotter a scientific field (with more scientific teams involved), the less likely the research findings are to be true. This seemingly paradoxical corollary follows because, as stated above, the PPV of isolated findings decreases when many teams of investigators are involved in the same field. This may explain why we occasionally see major excitement followed rapidly by severe disappointments in fields that draw wide attention. With many teams working on the same field and with massive experimental data being produced, timing is of the essence in beating competition. Thus, each team may prioritize on pursuing and disseminating its most impressive “positive” results. “Negative” results may become attractive for dissemination only if some other team has found a “positive” association on the same question. In that case, it may be attractive to refute a claim made in some prestigious journal. The term Proteus phenomenon has been coined to describe this phenomenon of rapidly alternating extreme research claims and extremely opposite refutations [ 29 ]. Empirical evidence suggests that this sequence of extreme opposites is very common in molecular genetics [ 29 ].
These corollaries consider each factor separately, but these factors often influence each other. For example, investigators working in fields where true effect sizes are perceived to be small may be more likely to perform large studies than investigators working in fields where true effect sizes are perceived to be large. Or prejudice may prevail in a hot scientific field, further undermining the predictive value of its research findings. Highly prejudiced stakeholders may even create a barrier that aborts efforts at obtaining and disseminating opposing results. Conversely, the fact that a field is hot or has strong invested interests may sometimes promote larger studies and improved standards of research, enhancing the predictive value of its research findings. Or massive discovery-oriented testing may result in such a large yield of significant relationships that investigators have enough to report and search further and thus refrain from data dredging and manipulation.
Most Research Findings Are False for Most Research Designs and for Most Fields
In the described framework, a PPV exceeding 50% is quite difficult to get. Table 4 provides the results of simulations using the formulas developed for the influence of power, ratio of true to non-true relationships, and bias, for various types of situations that may be characteristic of specific study designs and settings. A finding from a well-conducted, adequately powered randomized controlled trial starting with a 50% pre-study chance that the intervention is effective is eventually true about 85% of the time. A fairly similar performance is expected of a confirmatory meta-analysis of good-quality randomized trials: potential bias probably increases, but power and pre-test chances are higher compared to a single randomized trial. Conversely, a meta-analytic finding from inconclusive studies where pooling is used to “correct” the low power of single studies, is probably false if R ≤ 1:3. Research findings from underpowered, early-phase clinical trials would be true about one in four times, or even less frequently if bias is present. Epidemiological studies of an exploratory nature perform even worse, especially when underpowered, but even well-powered epidemiological studies may have only a one in five chance being true, if R = 1:10. Finally, in discovery-oriented research with massive testing, where tested relationships exceed true ones 1,000-fold (e.g., 30,000 genes tested, of which 30 may be the true culprits) [ 30 , 31 ], PPV for each claimed relationship is extremely low, even with considerable standardization of laboratory and statistical methods, outcomes, and reporting thereof to minimize bias.
https://doi.org/10.1371/journal.pmed.0020124.t004
Claimed Research Findings May Often Be Simply Accurate Measures of the Prevailing Bias
As shown, the majority of modern biomedical research is operating in areas with very low pre- and post-study probability for true findings. Let us suppose that in a research field there are no true findings at all to be discovered. History of science teaches us that scientific endeavor has often in the past wasted effort in fields with absolutely no yield of true scientific information, at least based on our current understanding. In such a “null field,” one would ideally expect all observed effect sizes to vary by chance around the null in the absence of bias. The extent that observed findings deviate from what is expected by chance alone would be simply a pure measure of the prevailing bias.
For example, let us suppose that no nutrients or dietary patterns are actually important determinants for the risk of developing a specific tumor. Let us also suppose that the scientific literature has examined 60 nutrients and claims all of them to be related to the risk of developing this tumor with relative risks in the range of 1.2 to 1.4 for the comparison of the upper to lower intake tertiles. Then the claimed effect sizes are simply measuring nothing else but the net bias that has been involved in the generation of this scientific literature. Claimed effect sizes are in fact the most accurate estimates of the net bias. It even follows that between “null fields,” the fields that claim stronger effects (often with accompanying claims of medical or public health importance) are simply those that have sustained the worst biases.
For fields with very low PPV, the few true relationships would not distort this overall picture much. Even if a few relationships are true, the shape of the distribution of the observed effects would still yield a clear measure of the biases involved in the field. This concept totally reverses the way we view scientific results. Traditionally, investigators have viewed large and highly significant effects with excitement, as signs of important discoveries. Too large and too highly significant effects may actually be more likely to be signs of large bias in most fields of modern research. They should lead investigators to careful critical thinking about what might have gone wrong with their data, analyses, and results.
Of course, investigators working in any field are likely to resist accepting that the whole field in which they have spent their careers is a “null field.” However, other lines of evidence, or advances in technology and experimentation, may lead eventually to the dismantling of a scientific field. Obtaining measures of the net bias in one field may also be useful for obtaining insight into what might be the range of bias operating in other fields where similar analytical methods, technologies, and conflicts may be operating.
How Can We Improve the Situation?
Is it unavoidable that most research findings are false, or can we improve the situation? A major problem is that it is impossible to know with 100% certainty what the truth is in any research question. In this regard, the pure “gold” standard is unattainable. However, there are several approaches to improve the post-study probability.
Better powered evidence, e.g., large studies or low-bias meta-analyses, may help, as it comes closer to the unknown “gold” standard. However, large studies may still have biases and these should be acknowledged and avoided. Moreover, large-scale evidence is impossible to obtain for all of the millions and trillions of research questions posed in current research. Large-scale evidence should be targeted for research questions where the pre-study probability is already considerably high, so that a significant research finding will lead to a post-test probability that would be considered quite definitive. Large-scale evidence is also particularly indicated when it can test major concepts rather than narrow, specific questions. A negative finding can then refute not only a specific proposed claim, but a whole field or considerable portion thereof. Selecting the performance of large-scale studies based on narrow-minded criteria, such as the marketing promotion of a specific drug, is largely wasted research. Moreover, one should be cautious that extremely large studies may be more likely to find a formally statistical significant difference for a trivial effect that is not really meaningfully different from the null [ 32–34 ].
Second, most research questions are addressed by many teams, and it is misleading to emphasize the statistically significant findings of any single team. What matters is the totality of the evidence. Diminishing bias through enhanced research standards and curtailing of prejudices may also help. However, this may require a change in scientific mentality that might be difficult to achieve. In some research designs, efforts may also be more successful with upfront registration of studies, e.g., randomized trials [ 35 ]. Registration would pose a challenge for hypothesis-generating research. Some kind of registration or networking of data collections or investigators within fields may be more feasible than registration of each and every hypothesis-generating experiment. Regardless, even if we do not see a great deal of progress with registration of studies in other fields, the principles of developing and adhering to a protocol could be more widely borrowed from randomized controlled trials.
Finally, instead of chasing statistical significance, we should improve our understanding of the range of R values—the pre-study odds—where research efforts operate [ 10 ]. Before running an experiment, investigators should consider what they believe the chances are that they are testing a true rather than a non-true relationship. Speculated high R values may sometimes then be ascertained. As described above, whenever ethically acceptable, large studies with minimal bias should be performed on research findings that are considered relatively established, to see how often they are indeed confirmed. I suspect several established “classics” will fail the test [ 36 ].
Nevertheless, most new discoveries will continue to stem from hypothesis-generating research with low or very low pre-study odds. We should then acknowledge that statistical significance testing in the report of a single study gives only a partial picture, without knowing how much testing has been done outside the report and in the relevant field at large. Despite a large statistical literature for multiple testing corrections [ 37 ], usually it is impossible to decipher how much data dredging by the reporting authors or other research teams has preceded a reported research finding. Even if determining this were feasible, this would not inform us about the pre-study odds. Thus, it is unavoidable that one should make approximate assumptions on how many relationships are expected to be true among those probed across the relevant research fields and research designs. The wider field may yield some guidance for estimating this probability for the isolated research project. Experiences from biases detected in other neighboring fields would also be useful to draw upon. Even though these assumptions would be considerably subjective, they would still be very useful in interpreting research claims and putting them in context.
- View Article
- Google Scholar
- 12. Kelsey JL, Whittemore AS, Evans AS, Thompson WD (1996) Methods in observational epidemiology, 2nd ed. New York: Oxford U Press. 432 p.
Thank you for visiting nature.com. You are using a browser version with limited support for CSS. To obtain the best experience, we recommend you use a more up to date browser (or turn off compatibility mode in Internet Explorer). In the meantime, to ensure continued support, we are displaying the site without styles and JavaScript.
- View all journals
- ADVERTISEMENT FEATURE Advertiser retains sole responsibility for the content of this article
Six factors affecting reproducibility in life science research and how to handle them
Produced by
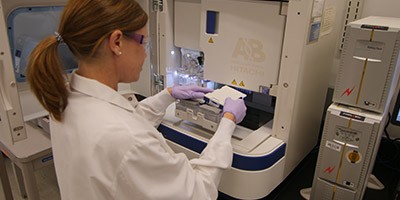
There are several reasons why an experiment cannot be replicated.
Independent verification of data is a fundamental principle of scientific research across the disciplines. The self-correcting mechanisms of the scientific method depend on the ability of researchers to reproduce the findings of published studies in order to strengthen evidence and build upon existing work. Stanford University medical researcher, John Ioannidis, a prominent scholar on reproducibility in science, has pointed out that the importance of reproducibility does not have to do with ensuring the ‘correctness’ of results, but rather with ensuring the transparency of exactly what was done in a given line of research 1 .
In theory, researchers should be able to re-create experiments, generate the same results, and arrive at the same conclusions, thus helping to validate and strengthen the original work. However, reality does not always meet these expectations. Too often, scientific findings in biomedical research cannot be reproduced 2 ; consequently, resources and time are wasted, and the credibility of scientific findings are put at risk. Furthermore, despite recent heightened awareness, there remains a significant need to better educate students and research trainees about the lack of reproducibility in life science research and actions that can be taken to improve it. Here, we review predominant factors affecting reproducibility and outline efforts to improve the situation.
What is reproducibility?
The phrase ‘lack of reproducibility’ is understood in the scientific community, but it is a rather broad expression that incorporates several aspects. Though a standardized definition has not been fully established, the American Society for Cell Biology ® (ASCB ® ) has attempted a multi-tiered approach to defining the term reproducibility by identifying the subtle differences in how the term is perceived throughout the scientific community.
ACSB 4 has discussed these differences with the following terms: direct replication , which are efforts to reproduce a previously observed result by using the same experimental design and conditions as the original study; analytic replication , which aims to reproduce a series of scientific findings through a reanalysis of the original data set; systemic replication , which is an attempt to reproduce a published finding under different experimental conditions (e.g., in a different culture system or animal model); and conceptual replication , where the validity of a phenomenon is evaluated using a different set of experimental conditions or methods.
It is generally thought that the improvement of direct replication and analytic replication is most readily addressed through training, policy modifications, and other interventions, while failures in systematic and conceptual replication are more difficult to connect to problems with how research was performed as there is more natural variability at play.
The reproducibility problem
Many studies claim a significant result, but their findings cannot be reproduced. This problem has attracted increased attention in recent years, with several studies providing evidence that research is often not reproducible. A 2016 Nature survey 3 , for example, revealed that in the field of biology alone, over 70% of researchers were unable to reproduce the findings of other scientists and approximately 60% of researchers could not reproduce their own findings.
The lack of reproducibility in scientific research has negative impacts on health, lower scientific output efficiency, slower 6 , 7 scientific progress, wasted time and money, and erodes the public’s trust in scientific research. Though many of these problems are difficult to quantify, there have been attempts to calculate financial losses. A 2015 meta-analysis 5 of past studies regarding the cost of non-reproducible research estimated that $28 billion per year is spent on preclinical research that is not reproducible. Looking at avoidable waste in biomedical research on the whole, it is estimated that as much as 85% of expenditure may be wasted due to factors that similarly contribute to non-reproducible research such as inappropriate study design, failure to adequately address biases, non-publication of studies with disappointing results, and insufficient descriptions of interventions and methods.
Factors contributing to the lack of reproducibility
Failures of reproducibility cannot be traced to a single cause, but there are several categories of shortcomings that can explain many of the cases where research cannot be reproduced. Here are some of the most significant categories.
A lack of access to methodological details, raw data, and research materials.
For scientists to be able to reproduce published work, they must be able to access the original data, protocols, and key research materials. Without these, reproduction is greatly hindered and researchers are forced to reinvent the wheel as they attempt to repeat previous work. The mechanisms and systems for sharing raw unpublished data and research materials, such as data repositories and biorepositories, need to be made robust so that sharing is not an impediment to reproducibility.
Use of misidentified, cross-contaminated, or over-passaged cell lines and microorganisms.
Reproducibility can be complicated and/or invalidated by biological materials that cannot be traced back to their original source, are not thoroughly authenticated, or are not properly maintained. For example, if a cell line is not identified correctly, or is contaminated with mycoplasma or another cell type, results can be affected significantly and their likelihood of replication diminished. There are many cases of studies conducted with misidentified or cross-contaminated cell lines, so results rendered questionable, and conclusions drawn from them are potentially invalid 8 . Improper maintenance of biological materials via long-term serial passaging can also seriously affect genotype and phenotype, which can make reproducing data difficult. Several studies have demonstrated that serial passaging can lead to variations in gene expression, growth rate, spreading, and migration in cell lines 9 , 10 ; and changes in physiology, virulence factor production, and antibiotic resistance in microorganisms 11 , 12 , 13 .
Inability to manage complex datasets
Advancements in technology have enabled the generation of extensive, complex data sets; however, many researchers do not have the knowledge or tools needed for analyzing, interpreting and storing the data correctly. Further, new technologies or methodologies may not yet have established or standardized protocols, so variations and biases can be easily introduced, which in turn can affect the ability to analytically replicate the data.
Poor research practices and experimental design
Among the findings from scholarly efforts examining non-reproducibility is that, in a significant portion of cases, the cause could be traced to poor practices in reporting research results, and poor experimental design 14 , 15 . Poorly designed studies without a core set of experimental parameters, whose methodology is not reported clearly, are less likely to be reproducible. If a study is designed without a thorough review of existing evidence, or if the efforts to minimize biases are insufficient, reproducibility becomes more problematic.
Cognitive bias
These refer to the ways that judgement and decision-making are affected by the individual subjective social context that each person builds around them. They are errors made in cognitive processes that are due to personal beliefs or perceptions. Researchers strive for impartiality and try to avoid cognitive bias, but it is often difficult to completely shut out the subtle, subconscious ways that cognitive bias can affect the conduct of research 16 , 17 . Scientists have identified dozens of different types of cognitive biases, including confirmation bias, selection bias, the bandwagon effect, cluster illusion, and reporting bias 17 . Confirmation bias is the unconscious act of interpreting new evidence in ways that confirm one’s existing belief system or theories; this type of bias impacts how information is gathered, interpreted, and recalled. Selection bias sees researchers choose subjects or data for analysis that is not properly randomized; here, the sample obtained is not truly representative of the whole population. The bandwagon effect is the tendency to agree with a position too easily, without sufficient evaluation in order to maintain group harmony; this form of bias may lead to the acceptance of unproven ideas that have gained popularity. Cluster illusion is when patterns are perceived in a pool of random data in which no actual pattern exists; a bias based on the tendency of the brain to seek out patterns. Reporting bias is when study participants selectively reveal or suppress information in a study according to their own subconscious drivers; this form of bias may lead to underreporting of negative or undesirable experimental results.
A competitive culture that rewards novel findings and undervalues negative results
The academic research system encourages the rapid publication of novel results. Researchers are rewarded more for publishing novel findings, and not for publishing negative results (e.g., where a correlation was not found) 15 . Indeed, there are limited arenas for publishing negative results, which could hone researchers’ efforts and avoid repeating work that may be difficult to replicate. Overall, reproducibility in research is hindered by under-reporting of studies that yield results deemed disappointing or insignificant. University hiring and promotion criteria often emphasize publishing in high-impact journals and do not generally reward negative results. Also, a competitive environment for research grants may incentivize researchers to limit reporting of details learned through experience that make experiments work better.
Recommended best practices
A number of significant efforts have been aimed at addressing the lack of reproducibility in scientific research. Individual researchers, journal publishers, funding agencies, and universities have all made substantial efforts toward identifying potential policy changes aimed at improving reproducibility 16 , 18 , 19 , 20 , 21 . What has emerged from these efforts is a set of recommended practices and policy prescriptions that are expected to have a large impact.
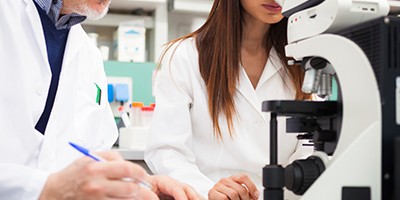
Training on statistical methods and study design is essential for reproducible research.
Robust sharing of data, materials, software, and other tools.
All of the raw data that underlies any published conclusions should be readily available to fellow researchers and reviewers of the published article. Depositing the raw data in a publicly available database would reduce the likelihood that researchers would select only those results that support a prevailing attitude or confirms previous work. Such sharing would accelerate scientific discoveries, and enable scientists to interact and collaborate at a meaningful level.
Use of authenticated biomaterials
Data integrity and assay reproducibility can be greatly improved by using authenticated, low-passage reference materials. Cell lines and microorganisms verified by a multifaceted approach that confirms phenotypic and genotypic traits, and a lack of contaminants, are essential tools for research. By starting a set of experiments with traceable and authenticated reference materials, and routinely evaluating biomaterials throughout the research workflow, the resulting data will be more reliable, and more likely to be reproducible.
Training on statistical methods and study design
Experimental reproducibility could be considerably improved if researchers were trained how to properly structure experiments and perform statistical analyses of results. By strictly adhering to a set of best practices in statistical methodology and experimental design, researchers could boost the validity and reproducibility of their work.
Pre-registration of scientific studies
If scientists pre-register proposed scientific studies (including the approach) prior to initiation of the study, it would allow careful scrutiny of all parts of the research process and would discourage the suppression of negative results.
Publish negative data
Many times, ‘negative’ data that do not support a hypothesis typically go unpublished as they are not considered high impact or innovative. By publishing negative data, it helps to interpret positive results from related studies and can help researchers adjust their experimental design so that further resources and funding are not wasted 22 .
Thorough description of methods
It is important that research methodology is thoroughly described to help improve reproducibility. Researchers should clearly report key experimental parameters, such as whether experiments were blinded, which standards and instruments were used, how many replicates were made, how the results were interpreted, how the statistical analysis was performed, how the randomization was done, and what criteria were used to include or exclude any data.
Ongoing efforts to improve reproducibility
There is a varied and influential group of organizations that are already working to improve the reproducibility of scientific research. The following is a list of initiatives aimed at supporting one or more aspects of the research reproducibility issue.
American Society for Cell Biology (ASCB) - The ASCB Report on Reproducibility
ASCB continues to identify methods and best practices that would enhance reproducibility in basic research. From its original analysis, the ASCB task force identified and published several recommendations focused on supporting existing efforts and initiating new activities on better training, reducing competition, sharing data, improving peer reviews, and providing cell authentication guidelines.
American Type Culture Collection (ATCC) - Cell and Microbial Authentication Services and Programs
Biological resource centers, such as ATCC, provide the research community with standardized, traceable, fully authenticated cell lines and microorganisms to aid in assay reproducibility. At ATCC, microbial strains are authenticated and characterized through genotypic, phenotypic, and functional analyses to confirm identity, purity, virulence, and antibiotic resistance. ATCC has also taken a lead in cell line authentication by publishing the voluntary consensus standard, ANSI/ATCC ASN-0002: Authentication of Human Cell Lines: Standardization of STR Profiling , and by performing STR profiling on all human cell lines managed among its holdings.
Furthermore, ATCC offers online cell line authentication training in partnership with Global Biological Standards Institute, NIH (R25GM116155-03), and Susan G. Komen (SPP160007), which focuses on the best practices for receiving, managing, authenticating, culturing, and preserving cell cultures. To further support cell authentication and reproducibility in the life sciences, ATCC also provides STR profiling and mycoplasma detection testing as services to researchers.
National Institutes of Health (NIH) - Rigor and Reproducibility
To help improve rigor, reproducibility, and transparency in scientific research, the NIH issued a notice in 2015 that informed scientists of revised grant application instructions that focused on improving experimental design, authenticating biological and chemical resources, analyzing and interpreting results, and accurately reporting research findings. These efforts have led to the adoption of similar guidelines by journals across numerous scientific disciplines and has resulted in cell line authentication becoming a prerequisite for publication.
Science Exchange & the Center for Open Science - The Reproducibility Project: Cancer Biology
This initiative was designed to provide evidence of reproducibility in cancer research and to identify possible factors that may affect reproducibility. Here, selected results from high-profile articles are independently replicated by unbiased third parties to evaluate if data could be consistently reproduced. For each evaluated study, a registered report delineating the experimental workflow is reviewed and published before experimentation is initiated; after data collection and analysis, the results are published as a replication study.
Author Policies for Publication
Many peer-reviewed journals have updated their reporting requirements to help improve the reproducibility of published results. The Nature Research journals, for example, have implemented new editorial policies that help ensure the availability of data, key research materials, computer codes and algorithms, and experimental protocols to other scientists. Researchers must now complete an editorial policy checklist to ensure compliance with these policies before their manuscript can be considered for review and publication.
Most people familiar with the issue of reproducibility agree that these efforts are gaining traction. However, progress will require sustained attention on the issue, as well as cooperation and involvement from stakeholders across various fields.
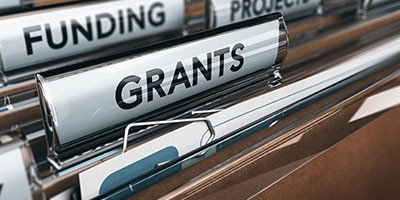
The academic research system encourages the rapid publication of novel results.
Moving forward
Accuracy and reproducibility are essential for fostering robust and credible research and for promoting scientific advancement. There are predominant factors that have contributed to the lack of reproducibility in life science research. This issue has come to light in recent years and a number of guidelines and recommendations on achieving reproducibility in the life sciences have emerged, but the practical implementation of these practices may be challenging. It is essential that the scientific community are objective when designing experiments, take responsibility for depicting their results accurately, and thoroughly and precisely describe all methodologies used. Further, funders, publishers, and policy-makers should continue to raise awareness about the lack of reproducibility and use their position to promote better research practices throughout the life sciences. By taking action and seeking opportunities for improvement, researchers and key stakeholders can help improve research practices and the credibility of scientific data.
For more information on how you can improve the reproducibility of your research, visit ATCC online.
Ioannidis JP. PLoS Medicine 11 : e1001747, 2014.
Article PubMed Google Scholar
Feilden T. Science & Environment, BBC News, February 22, 2017.
Baker M. Nature News Feature , May 25 , 2016.
ASCB. ASCB, 2014.
Freedman LP, Cockburn IM, Simcoe TS. PLoS Biology 13 : e1002165, 2015.
Chalmers I, Glasziou P. Lancet 374 : 86-89, 2009.
Macleod MR, et al. Lancet 383 : 101-104, 2014.
Horbach S, Halffman W. PLoS One 12 : e0186281, 2017.
Mouriaux F, et al. Invest Ophthalmol Vis Sci 57 (13): 5288-5301, 2016.
Liao H, et al. Cytotechnology 66 : 229-238, 2014.
Somerville GA, et al. J Bacteriol 184 : 1430-1437, 2002.
Grimm D, et al. Infect Immun 71 (16): 3138-3145, 2003.
Lee JY, et al. Scientific Reports 6: 25543, 2016.
Resnik DB, Shamoo AE. Account Res 24 (2): 116-123, 2017.
The Academy of Medical Sciences, BBSRC, Medial Research Council, Wellcome Trust. Symposium report, October 2015.
Munafò MR, et al. Nature Human Behaviour 1: 0021, 2017.
Article Google Scholar
Cherry K. Cognitive Psychology, Very Well Mind. October 8, 2018.
Google Scholar
Stodden V, Leisch F, Peng RD. 1st Edition, Chapman and Hall/CRC, 2014.
Landis SC, et al. Nature 490 : 187-191, 2012.
NIH https://www.nih.gov/research-training/rigor-reproducibility 2015.
Davies, EW, Edwards, DD. A Report from the American Academy of Microbiology: Promoting Responsible Scientific Research, 2016.
Weintraub PG. J Insect Sci 16(1): 109, 2016.
Download references
Related Articles
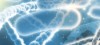
Quick links
- Explore articles by subject
- Guide to authors
- Editorial policies
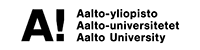
Writing about Design
Principles and tips for design-oriented research.
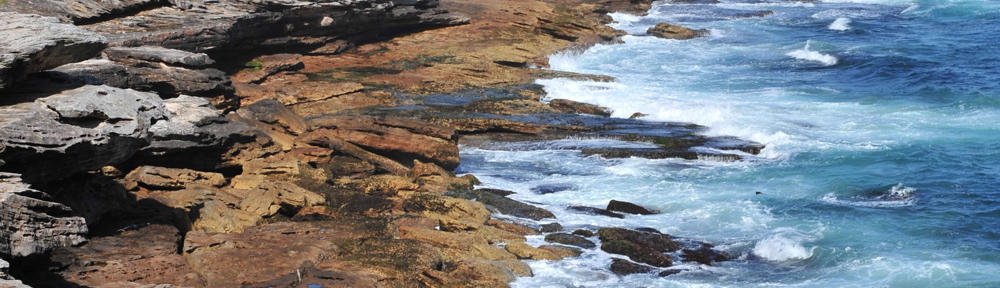
Typology of possible research findings (i.e., “contributions”)
Introduction.
A good academic text delivers a clear and interesting message. That is often described as “contribution”. Good contributions teach something to the text’s readers: they change the reader’s way of thinking or acting, and increase their understanding and knowledge about an interesting subject. Thus, “a contribution is made when a manuscript clearly adds, embellishes, or creates something beyond what is already known” (Ladik & Stewart, 2008, p. 157). Such findings therefore present something that the researchers did not know so far; that is what makes the research article interesting.
Also a BA or a MA thesis deliver contributions. But their requirements for significant contribution are lower, as it is not crucial that a finding of a thesis should be a considered as a research contribution that generates novel understanding in the scientific community.
So, what are the possible findings and contributions that academic texts can make? Understanding what a contribution can be becomes easier over time, as one reads more literature and sees more examples of academic publications. But to get started more quickly, this article presents some classifications that I have found from other researchers’ writings, and finally presents a longer list that I have tailored for the fields of design and HCI.
The presentation of contributions in this article is in two parts: first I will discuss academic articles, and then I will add notes about BA/MA theses in HCI and design.
Types of findings and contributions in academic articles
Contributions can be classified along several dimensions. Some of the existing classifications are oriented to theoretical contributions. For example, Ladik and Stewart’s (2008) “contribution continuum”, written for a marketing research audience, divides the possible contributions to 8 classes, organized from minor to fundamental scientific impacts:
- Straight replications: studies that verify whether a finding that has been already published can be repeated.
- Replication and extension: similar to the one above, but with an adjustment.
- Extension of a new theory/method in a new area.
- Integrative review (e.g., meta-analysis).
- New theories to explain an old phenomenon, possibly also including a comparison between an existing and the new theory against each other to find out which one works better.
- Identifications of new phenomena worth of attention.
- Grand syntheses that integrate earlier theories together.
- New theories that predict new phenomena.
In addition to presenting the continuum, Ladik and Stewart’s (2008) text is great also in emphasizing many other characteristics of good academic texts too, such as a need to think about the target reader audience, need to emphasise surprise, and demonstrate passion and relevance of the topic that has been studied.
In human–computer interaction (HCI), which is more oriented to human-created objects, other kinds of contributions can be recognized. Wobbrock (2012) and Wobbrock and Kientz (2016) do not define the contributions based on their magnitude, but in terms of types of outcomes. As we can see, the theoretical contributions that were listed above are only one possibility in applied fields such as HCI and design:
- empirical research findings (e.g., what factors and phenomena play an important role in different situations where people use technologies)
- artefacts (i.e., designs and technologies)
- surveys and reviews of existing research
In this classification, Wobbrock and Kientz’s papers themselves could be best classified as survey-like contributions, since their focus is on reviewing the kinds of research contributions in a research field as a whole. In this sence they synthesize together and explicate the practices in the field. In addition to being more directly useful also for HCI/design, Wobbrock’s suggestions are also great because both texts list papers from HCI research that exemplify these contribution types.
What is particular in the list above is the role of artifacts as research contributions. This is particular since it highlight’s HCI’s (and also design’s) nature as a “problem-solving” science (Oulasvirta and Hornbæk, 2016): in addition to producing the traditionally well-acknowledged empirical and conceptual (theoretical) contributions, HCI researchers also make constructive contributions by developing new technologies and designs.
Final distinction between contributions is their level of critical stance towards earlier research and practice. Most contributions are knowledge-increasing : they present new findings, expand the research to new areas, make existing theories and methods more detailed, accurate or more appropriate for some context, for example. These contributions are really common: with my colleagues we found, for example, that 94% of research papers in information systems research are knowledge-increasing (Salovaara et al., 2020). Many of the contribution classes presented in the lists above are like these too.
Other contributions are knowledge-contesting : they identify problems in the existing theories and methods, or in the practices by which they are used (Salovaara et al., 2020). They may also identify limits (“boundary conditions”) to the extent to which earlier contributions can be applied. In the spirit of science and research being a self-correcting process, the purpose of these knowledge-contesting contributions is to correct earlier mistakes in research and keep the research on the right track.
To summarise the considerations above, the following table presents a synthesis of possible contributions in HCI and design. A vast majority of the papers represent one (or sometimes several) of these contributions:
This list is not comprehensive, and some areas have been covered in more detail than others. What is however notable in this list’s items is that papers about these contributions can be written using the same narrative format. That is because most of these contributions require a study: some method by which some material is analysed so that findings can be presented. Such papers can be readily written following the IMRaD-style narrative . Only the last two contributions – recommendations/guidelines and research agendas/manifestos – may need a different kind of a narrative and can therefore be harder to write well.
Examples of non-contributions in academic research
Notably, there are also certain types of papers that are often submitted for publication but which are often rejected and will therefore be rarely found in academic literature. When one is writing an article, it is a good idea to make sure that one is not writing one of those types of papers. Four common non-contributions are following:
- Presentation of a well-designed system and its design process. These papers present well-designed systems and include evaluations that demonstrate the high quality of the outcome. The problem with these kinds of papers is that for a researcher looking for novel information, such papers offer very little to learn: they “only” describe well-conducted design process that already uses well-known methods. Only if these design processes solve hard problems in some contexts, and that these problems and their solutions generalise to other contexts too, the papers start to have value in terms of an academic contribution. That is because then the academic reader may conclude that the authors have found a way to address a problem that previously has been considered difficult to tackle. This kind of a study can be turned into an academic contribution by identifying a “design problem” that was solved in the process, and explaining why this problem is difficult and in what design situations similar problems can be encountered (i.e., where does the design problem and solution generalise to).
- Case study report. Papers of this kind present observations or interview-based findings from field studies, and describe carefully methods that were used in these studies. A lot of effort may have been put into gathering all the data and to analyse it. Unfortunately, despite all the effort spent, also in this case, the conclusion by a reader may be that the story is interesting but lacks novelty: papers of this kind may be a well-conducted research projects but which only have applied rigorous methods without yielding novel findings. This kind of a study can be turned into an academic contribution by identifying an interesting and novel finding, and deepening the literature research so that it convinces the reader about the novelty and the need for this finding in the research field (e.g., a “research gap”).
- Mappings of findings to a framework. Some papers present analyses from a complex settings and map these findings to a well-known theoretical framework (e.g. activity theory). The problem with such a finding is that it counts mostly as a demonstration that the framework can be used to make sense of observational data. This may not be surprising, if the same has been shown in numerous earlier studies too. This can be turned into an academic contribution, for example, by finding out that the framework cannot be used to make sense of some parts of the data, or that the framework needs adaptation because of the novel findings.
- Landscaping and clustering studies without conclusions . Some automatic data analysis methods nowadays allow researchers to generate elaborate descriptive visualizations and groupings that can summarise complex phenomena in a neat manner. Examples of these methods include social network analysis, clustering methods of multidimensional data (e.g., factor analysis, k-means clustering and topic modeling), and sentiment analysis about natural language. If a paper only presents the outputs of such analyses, without identifying non-obvious patterns or conclusions, the paper easily lacks a clear contribution. An academic contribution would include an actionable message to the research field: a call for changing the research focus, or think about a common phenomenon in a new way. Typically this requires that the researchers interpret their clusters and identify something unexpected from them.
Contributions and findings in BA and MA theses in HCI and design
In BA and MA theses, the requirements are slightly different than in academic articles. The difference lies in the need for presenting a contribution vs another, more modest kind of a finding. A thesis does not need to demonstrate novelty to an entire research field; it only needs to demonstrate the ability to apply the relevant methods, theories and analytical thinking with respect to a meaningful problem of practical importance. Therefore the three last above-presented examples of non-contributions are, in fact, good candidates for excellent BA or MA theses even if they lack an academic contribution.
One may therefore conclude that in BA and MA theses, the goals can be more practically determined: They may orient to finding good designs or solutions for specific design problems. They may be reflections about the nature of a design process, such as explorations whether a certain design approach yields findings that satisfy the designer. They may also be oriented towards a designer or practitioner community than the researchers. Therefore they may deliver a call or message to those communities to start addressing issues or become aware of matters that are being neglected. Such issues do not need to relate to academic activities, but to societal issues, for example.
One or many contributions?
There can be one or many contributions in a paper. Some contribution types also go naturally together. For example, sometimes the most interesting contributions appear in the Discussion, after the answers to the research question(s) have already been presented. Thus a paper about an exploratory study may be sense-making in its Findings (e.g., by identifying an interesting underlying pattern or concept in the findings and by giving a name for it), but a manifesto-like contribution in its Discussion if it then shows how that concept may be crucial to remember in other situations too. Many readers may find that this manifesto-like contribution is actually more important than the text’s original finding.
However, many instructions on academic writing recommend that every text focuses on delivering only one “contribution”. For example, instructions published in Nature’s web page recommend to “Keep your message clear” (Gewin, 2018). There is a good reason for this: To offer a clear and interesting message, different contributions usually require different investigations. If one tries to combine several contributions together, they may require different methods, and these methods may conflict with each other, leading to biased and compromised results. Another problem is the need to reach a high clarity with the paper: if there are several intended contributions, explaining them clearly can be difficult. Jumping from talking about one contribution to another may be necessary, but this may confuse the reader. It is important to remember that it is the author’s responsibility to demonstrate that the findings are significant and interesting (e.g., Ladik and Stewart, 2008). Confusions should be avoided at all cost.
To conclude, to offer a clear contribution or a finding, it is a good idea to identify early on what kind of a story one wants to tell with their text. Following the recommendations of the IMRaD structure , for instance, all the attention of the paper’s argumentation can then be directed to delivering that message as clearly and convincingly as possible. This helps the readers – evaluators, reviewers, and others – appreciate the work that the author has done.
Acknowledgments
Thanks for Oscar Person for tipping me about Ladik & Stewart’s paper on research continuum.
Bardzell, J. & Bardzell, S. (2011). Pleasure is your birthright: Digitally enabled designer sex toys as a case of third-wave HCI. In Proceedings of the SIGCHI Conference on Human Factors in Computing Systems (CHI 2011) (pp. 257–266). New York, NY: ACM Press. https://doi.org/10.1145/1978942.1978979
Braun, V. & Clarke, V. (2006). Using thematic analysis in psychology. Qualitative Research in Psychology , 3 , 77–101. https://doi.org/10.1191/1478088706qp063oa
Gewin, V. (2018). The write stuff: How to produce a first-class paper that will get published, stand out from the crowd and pull in plenty of readers. Nature, Vol. 555, pp. 129-130. Available at: https://media.nature.com/original/magazine-assets/d41586-018-02404-4/d41586-018-02404-4.pdf. Also available, with a different title, at https://www.nature.com/articles/d41586-018-02404-4 (retrieved 11 November 2020).
Cross, N. (2004). Expertise in design: An overview. Design Studies , 25 (5), 427–441. https://doi.org/10.1016/j.destud.2004.06.002
Gould, J. D., Conti, J., & Hovanyecz, T. (1983). Composing letters with a simulated listening typewriter. Communications of the ACM , 26 (4), 295–308. https://doi.org/10.1145/2163.358100
Gustafson, S., Baudisch, P., Gutwin, C., & Irani, P. (2008). Wedge: Clutter-free visualization of off-screen locations. In Proceedings of the SIGCHI Conference on Human Factors in Computing Systems (CHI 2008) (pp. 787–796). New York, NY: ACM Press.. https://doi.org/10.1145/1357054.1357179
Hardaker, C. (2013). “Uh….not to be nitpicky,,,, but…the past tense of drag is dragged, not drug.” – An overview of trolling strategies. Journal of Language Aggression and Conflict , 1 (1), 58–86. https://doi.org/10.1075/jlac.1.1.04har
Ladik, D. M. & Stewart, D. W. (2008). The contribution continuum. Journal of the Academy of Marketing Science , 36 , 157–165. https://doi.org/10.1007/s11747-008-0087-z
Nardi, B. A., Whittaker, S., & Bradner, E. (2000). Interaction and outeraction: Instant messaging in action. In Proceedings of the 2000 ACM Conference on Computer Supported Cooperative Work (CSCW 2000) (pp. 79–88). New York, NY: ACM Press. https://doi.org/10.1145/358916.358975
Oulasvirta, A., Tamminen, S., Roto, V., & Kuorelahti, J. (2005). Interaction in 4-second bursts: The fragmented nature of attentional resources in mobile HCI. In Proceedings of the SIGCHI Conference on Human Factors in Computing Systems (CHI 2005) (pp. 919–928). New York, NY: ACM Press. https://doi.org/10.1145/1054972.1055101
Oulasvirta, A. & Hornbæk, K. (2016). HCI research as problem-solving. In J. Kaye, A. Druin, C. Lampe, D. Morris, & J. P. Hourcade (Eds.), Proceedings of the SIGCHI Conference on Human Factors in Computing (CHI 2016) (pp. 4956–4967). New York, NY: ACM Press. https://doi.org/10.1145/2858036.2858283
Pirolli, P. & Card, S. (1995). Information foraging in information access environments. In Proceedings of the SIGCHI Conference on Human Factors in Computing Systems (CHI 1995) (pp. 51–58). New York, NY: ACM Press/Addison-Wesley. https://doi.org/10.1145/223904.223911
Salovaara, A., Upreti, B. R., Nykänen, J. I., & Merikivi, J. (2020). Building on shaky foundations? Lack of falsification and knowledge contestation in IS theories, methods, and practices. European Journal of Information Systems , 29 (1), 65–83. https://doi.org/10.1080/0960085X.2019.1685737
Suchman, L. A. (1987). Plans and Situated Actions: The Problem of Human–Machine Communication . Cambridge, UK: Cambridge University Press.
Todi, K., Weir, D., & Oulasvirta, A. (2016). Sketchplore: Sketch and explore with a layout optimiser. In Proceedings of the 2016 ACM Conference on Designing Interactive Systems (CHI 2016) (pp. 543–555). New York, NY: ACM Press. https://doi.org/10.1145/2901790.2901817
Torenvliet, G. (2003). We can’t afford it! The devaluation of a usability term. Interactions , 10 (4), 12–17. https://doi.org/10.1145/838830.838857
Wobbrock, J. O. (2012). Seven Research Contributions in HCI . The Information School, DUB Group, University of Washington. http://faculty.washington.edu/wobbrock/pubs/Wobbrock-2012.pdf (retrieved 12 November 2020).
Wobbrock, J. O. & Kientz, J. A. (2016). Research contributions in human–computer interaction. Interactions (May–June), 38–44. https://doi.org/10.1145/2907069
One thought on “ Typology of possible research findings (i.e., “contributions”) ”
Pingback: From table of contents to a finished text | Writing about Design
Comments are closed.
Research Findings
Pivot Bio is the world’s leading nitrogen innovator. In "Our Take" we share insights from Pivot Bio scientists and experts across academia and agriculture to educate how our biologicals deliver critical nutrition to the world’s most important crops and offer a sustainable replacement for synthetic fertilizer.
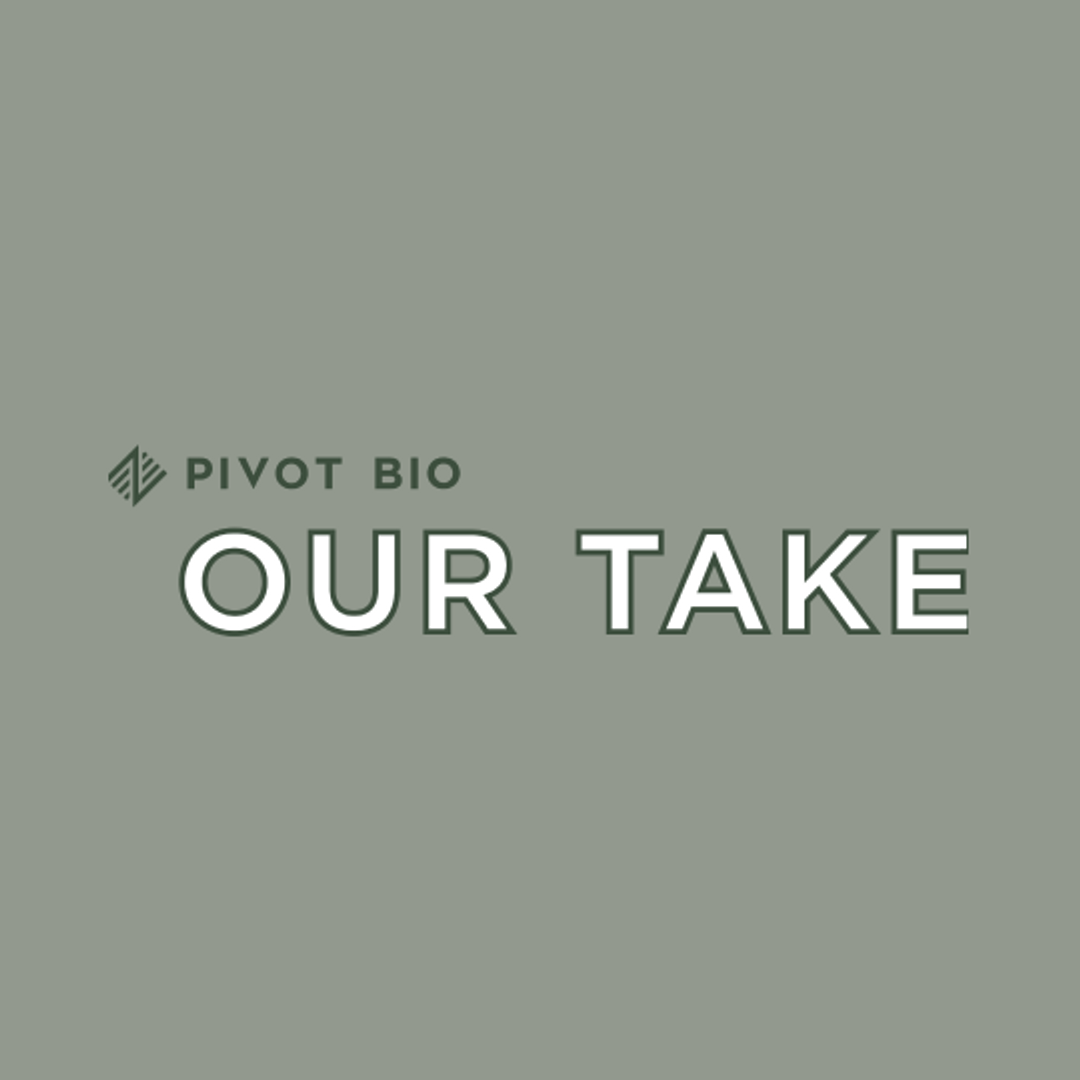
- Independent Research
- Performance Reports
- Peer-Reviewed Science
- University Field Trials
- On-Farm Studies
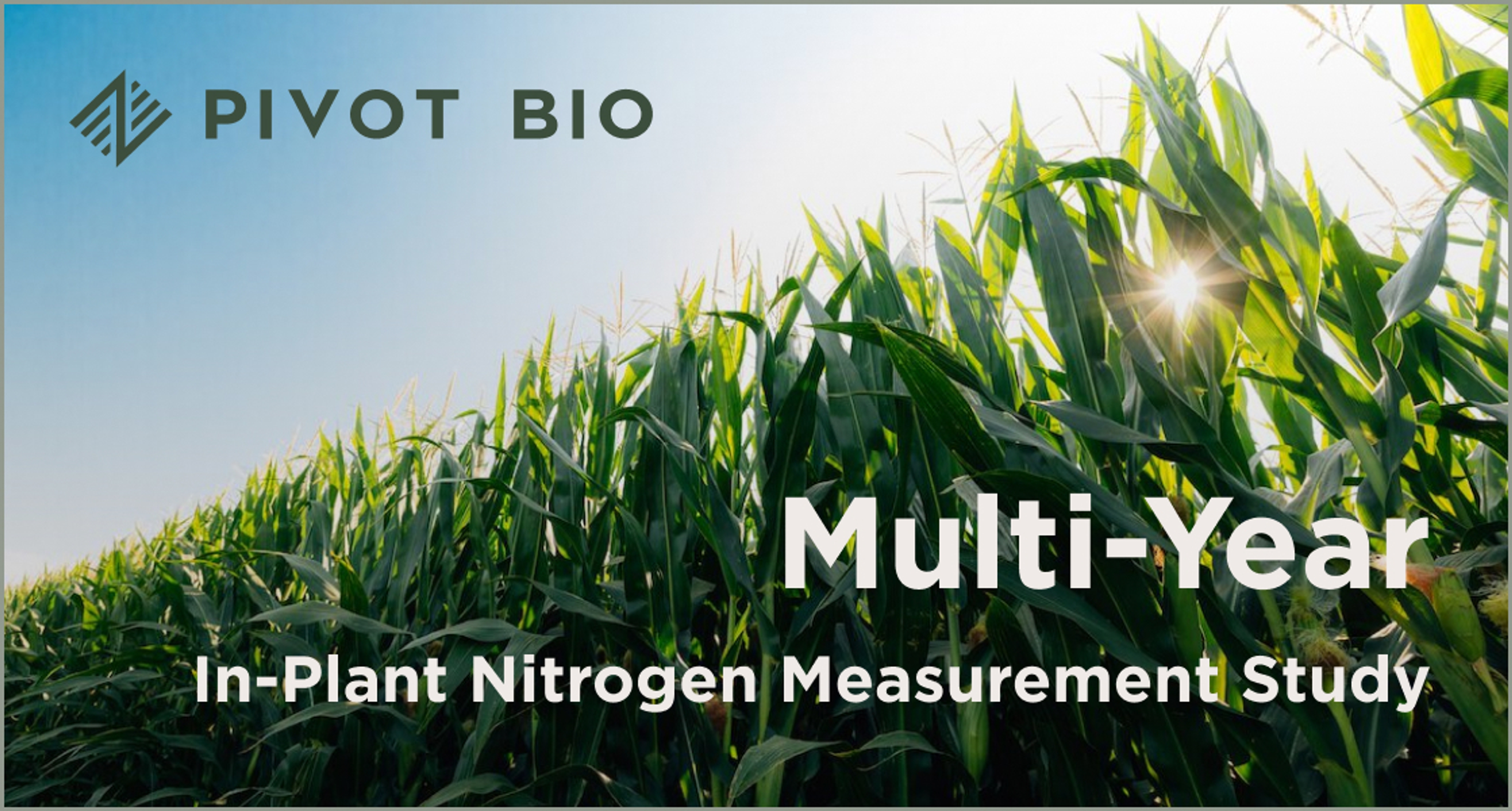
Agronomy First
Cutting-edge science in agriculture only succeeds when it works for farmers. Together, our scientists and agronomists focus on creating products that perform in the field, are compatible with current practices, and increase ROI – taking the guesswork out of nitrogen management and giving control back to farmers.
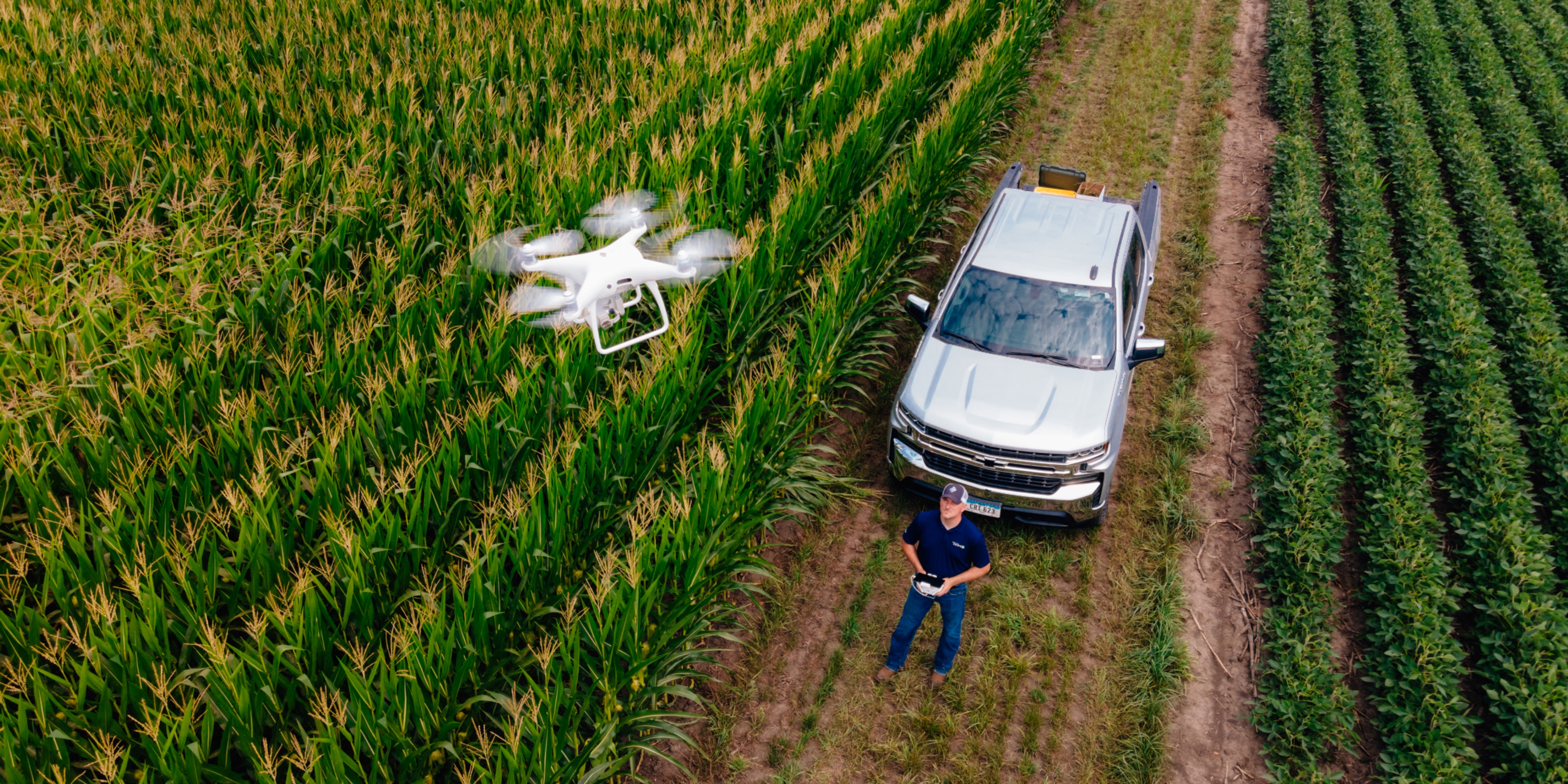
Innovation requires collaboration. Pivot Bio partners closely with leading university agriculture programs to conduct structured trials to demonstrate how our breakthrough technology maintains or improves yield. The goal? To prove that Pivot Bio's products help growers achieve greater profitability, predictability and sustainability.
Product performance varies and depends on many factors, including, but not limited to, weather, soil, and other farming conditions.
Individual results may vary.
Learn About Our Products
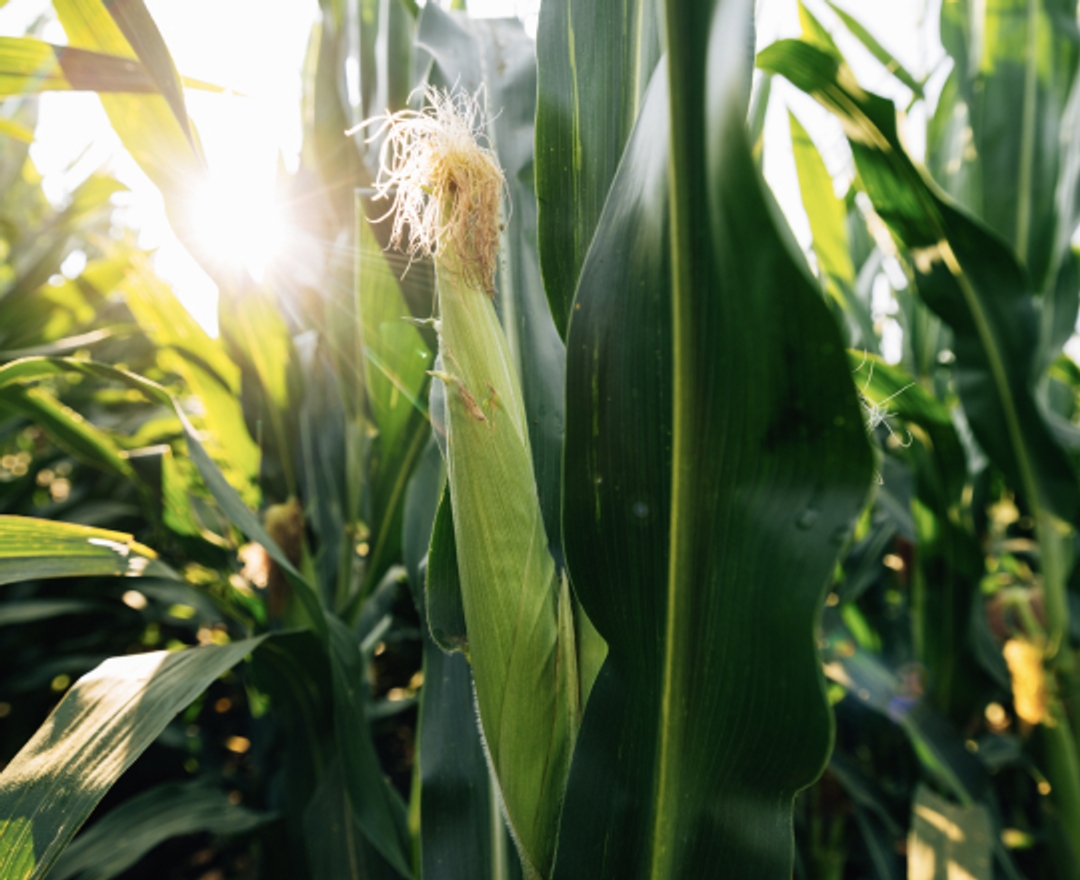
Reduce synthetic nitrogen and boost corn biomass and yield with PROVEN® 40 and PROVEN® 40 On-Seed.
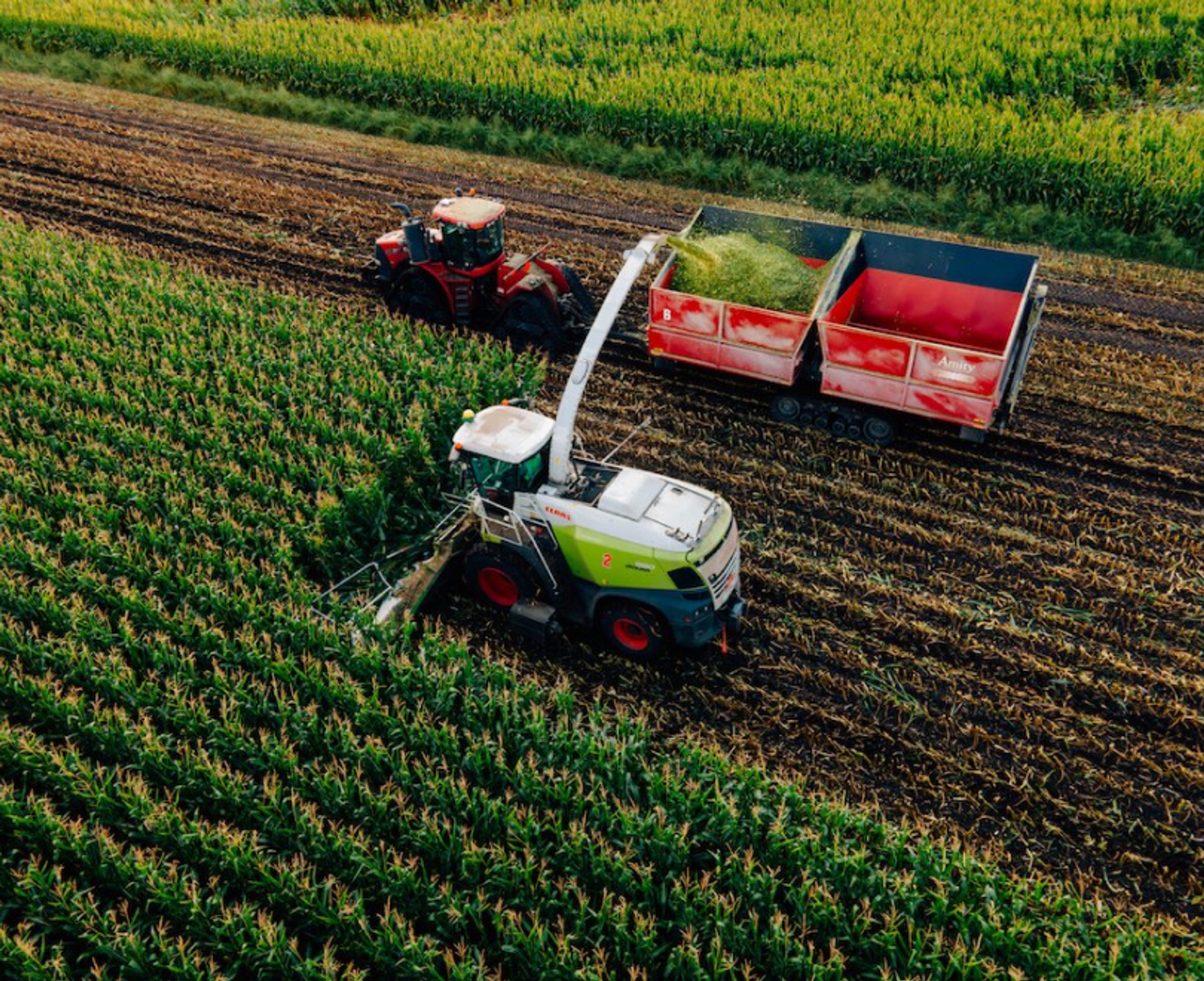
For Corn Silage
PROVEN® 40 ensures more tonnage per acre while providing predictable digestible nutrients, starch and protein levels.
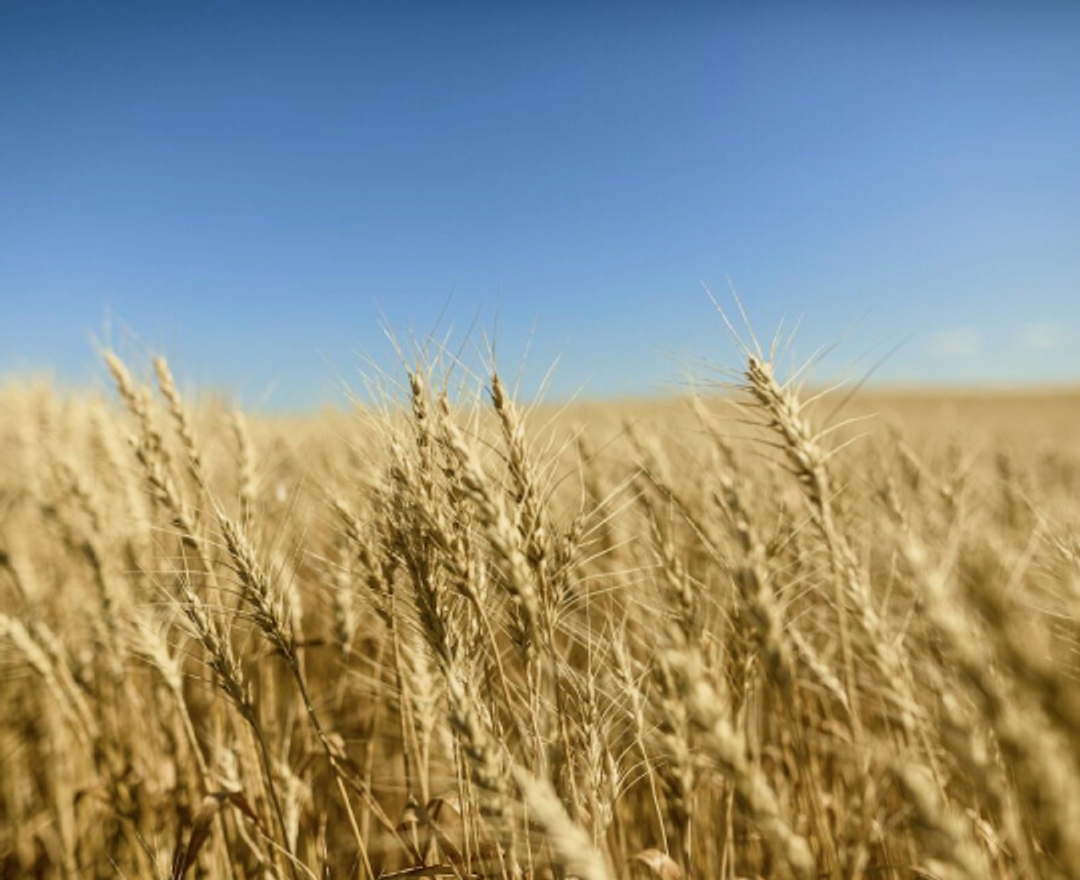
Boost spring and winter wheat biomass and grain yield while reducing synthetic nitrogen with Pivot Bio RETURN® and RETURN® On-Seed.
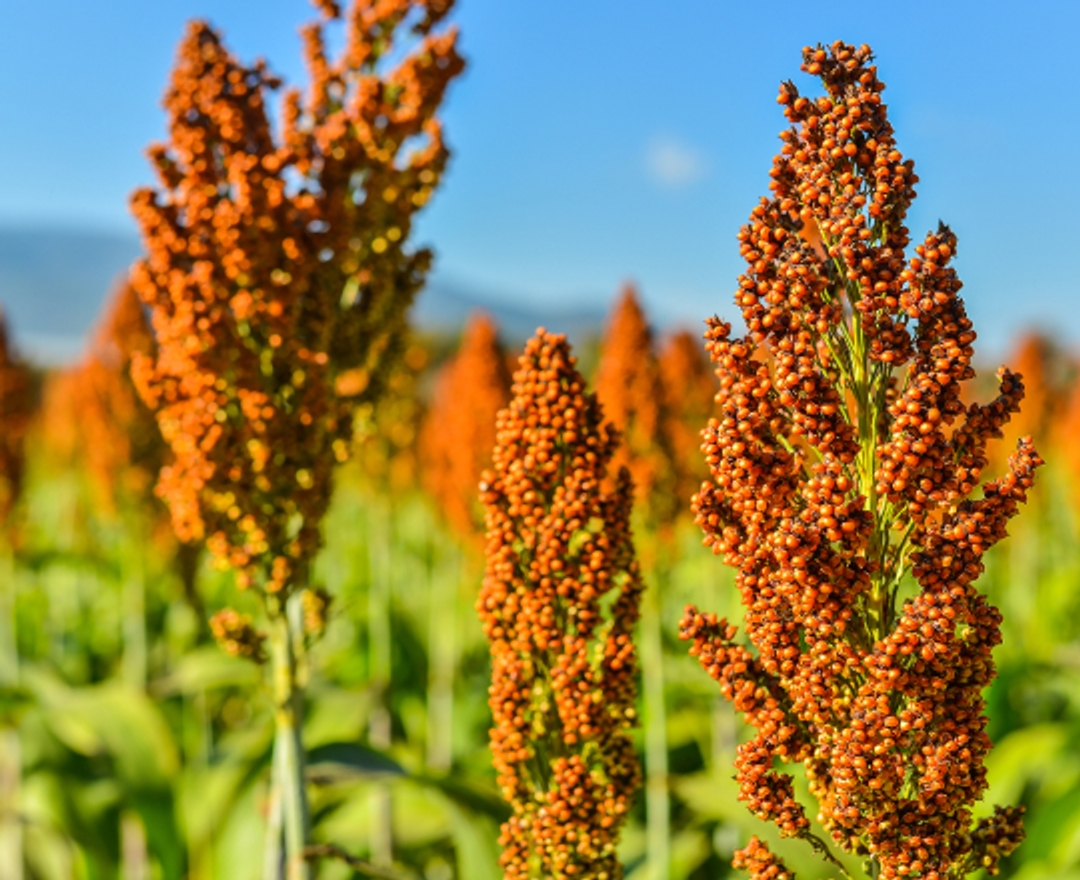
For Small Grains
Deliver steady nutrition to sorghum, barley, millet, oats and sunflower, regardless of weather, with RETURN® and RETURN® On-Seed.
The Macroeconomic Impact of Climate Change: Global vs. Local Temperature
This paper estimates that the macroeconomic damages from climate change are six times larger than previously thought. We exploit natural variability in global temperature and rely on time-series variation. A 1°C increase in global temperature leads to a 12% decline in world GDP. Global temperature shocks correlate much more strongly with extreme climatic events than the country-level temperature shocks commonly used in the panel literature, explaining why our estimate is substantially larger. We use our reduced-form evidence to estimate structural damage functions in a standard neoclassical growth model. Our results imply a Social Cost of Carbon of $1,056 per ton of carbon dioxide. A business-as-usual warming scenario leads to a present value welfare loss of 31%. Both are multiple orders of magnitude above previous estimates and imply that unilateral decarbonization policy is cost-effective for large countries such as the United States.
Adrien Bilal gratefully acknowledges support from the Chae Family Economics Research Fund at Harvard University. The views expressed herein are those of the authors and do not necessarily reflect the views of the National Bureau of Economic Research.
MARC RIS BibTeΧ
Download Citation Data
More from NBER
In addition to working papers , the NBER disseminates affiliates’ latest findings through a range of free periodicals — the NBER Reporter , the NBER Digest , the Bulletin on Retirement and Disability , the Bulletin on Health , and the Bulletin on Entrepreneurship — as well as online conference reports , video lectures , and interviews .
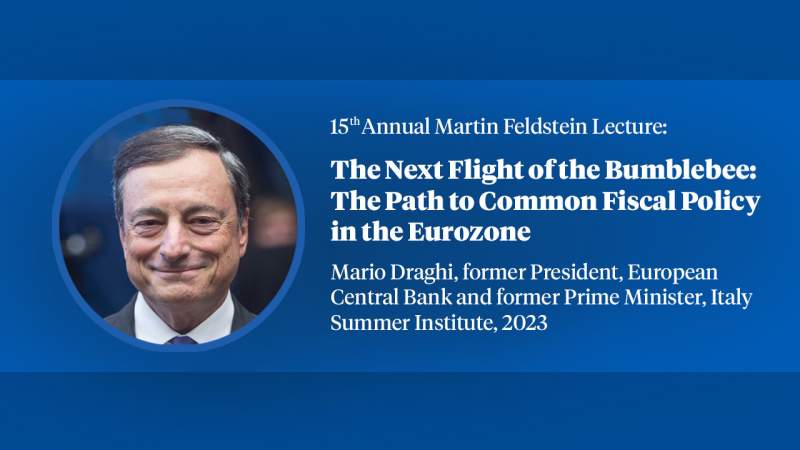
- Discount Window Direct
- FedLine Web
- Reporting Central
- Reserves Central
- Survey Central
- Term Deposit Facility
- Service Status
- Financial Services Home
- Accounting Services Home
- Account Management Information
- Daily Statement of Account
- Daylight Overdraft Reports
- Premium Accounting Information Services
- Statement of Service Charges
- Business Continuity
- Service Setup
- FedACH Products & Services Home
- FedACH Exception Resolution Service
- FedACH Information File Service
- FedACH Origination & Receipt
- FedACH Risk Management Services
- FedACH SameDay Service
- FedGlobal ACH Payments
- FedPayments Insights Service
- FedPayments Reporter Service for FedACH Services
- Testing Opportunities
- Bundled Solutions Home
- FedComplete
- FedTransaction Analyzer
- FedCash Services Home
- Cash Visibility
- Coin Allocation
- Coin Terminal Services
- FedCash Services via the FedLine Web Solution
- Check Products & Services Home
- Check 21-Enabled Services
- Check Adjustments Services
- FedDetect Duplicate Treasury Check Notifier Service
- FedForward Image Deposit Services
- FedImage and Electronic Check Services
- FedPayments Reporter Service for Check Services
- FedReceipt Services
- FedReturn Services
- Foreign and Canadian Check Services
- Image-Enabled Savings Bond Processing
- Paper Check Clearing Services
- FedNow Service Home
- About FedNow
- Blog Articles (Off-site)
- FedNow Explorer (Off-site)
- FedNow User Group
- Instant Payments Education (Off-site)
- Participants and Service Providers
- Sign Up for FedNow Emails
- National Settlement Service Home
- Fedwire Securities Service Home
- Joint Custody Service
- Fedwire Funds Service Home
- Central Bank Home
- Lending Central (Discount Window) Home
- Discount Window Direct Feature Guide
- Reporting Central Home
- Reporting Central User Guides
- Reserves Central Home
- Excess Balance Account
- Treasury Services Home
- Collateral Services
- Savings Bonds For Financial Institutions
- Savings Bonds News You Can Use
- Treasury ACH Reclamation
- Treasury Auctions
- Treasury Check Reclamation
- FedLine Solutions Home
- FedLine Advantage
- FedLine Command
- FedLine Direct
- Application and Connectivity Testing
- Central Bank
- District Information
- FedLine Solutions
- FedNow Service
- National Settlement Service
- Treasury Services
- Central Bank Resources Home
- Frequently Asked Questions
- Reporting Central Resources
- Survey Central Resources
- E-Payments Routing Directory
- FedLine Solutions Resources Home
- End User Authorization Contact (EUAC) Support
- FedLine Command Environment and Configuration Change Matrix
- FedLine Direct File Environment and Configuration Change Matrix
- FedLine Direct Message Environment and Configuration Change Matrix
- Bundled Solutions
- Foreign Exchange Rates
- Industry Links
- Resource Centers Home
- Business Banking Toolbox
- International Payments
- Risk Management Toolbox
- Same Day ACH
- Security and Resiliency Assurance Program
- Rules and Regulations Resources Home
- Operating Circulars
- Regulations
- Service and Access Setup Home
- Current Financial Services Customer
- Financial Institution Merger
- New Financial Services Customer
- Payment Processors and Third-Party Service Providers
- Treasury Services Resources Home
- Savings Bonds for Financial Institutions
- Where to Send Security Deposits
- Federal Reserve Bank Webinars
- Industry Events
- Products & Services Education
- Communications
- Email Notifications
- Press Releases
- Research Studies
- About Federal Reserve Bank Services
- Financial Services Leadership Team
- Bank Offices
- Holiday Schedules
2024 Diary of Consumer Payment Choice

View Full Report of the 2024 Diary of Consumer Payment Choice (PDF)
In May, Federal Reserve Financial Services’ FedCash ® Services released the annual Diary of Consumer Payment Choice (PDF) report from its ongoing research into the payment habits of the U.S. population. The 2024 findings revealed consumers made more payments in 2023 than in previous years, continuing the trend of rising payment transactions since 2020.
Amid increased payments, cash’s share decreased in favor of credit and debit cards, but overall cash use has remained stable as consumers continued to hold more cash than they did before 2020 as both a store-of-value (up 53%) and in their pockets, purses or wallets as a backup payment instrument (up 23%).
The findings also show a growing generational divide among those using cash versus electronic payments. Consumers younger than age 55 used cash for just 12% of payments in 2023, compared to 22% for those age 55 and older. Notably, for the first time in Diary history, cash was not the most-used instrument for smaller-value payments of $25 or less.
Other key findings from this nationally representative survey include:
- Consumers made an average of 46 monthly payments in 2023, an increase of seven payments compared to 2022.
- Increased credit and debit card use between 2022 and 2023 resulted in more than 60% of payments per month being made with credit (32%) and debit cards (30%).

- The share of payments made with cash decreased to 16%, though it remained the third most-used payment instrument behind credit and debit cards.
- Cash use was driven by in-person shopping, as well as by the payment behavior of consumers in low-income households and individuals age 55 and older.
- Individuals age 55 and older relied on cash for 22% of their payments, a rate approximately 1.5 times higher than that of their younger counterparts under 55.

- Consumers used mobile apps for 50% of person-to-person payments, continuing a widespread consumer transition away from paper-based payments.
- More than 90% of consumers intend to use cash as either a means of payment or store of value in the future.
Since 2016, the Federal Reserve has conducted this annual consumer survey to better understand the payment habits of U.S. consumers. Participants report all payments over a three-day period, the value of their cash holdings, payment instruments used and their preferences for various types of payments.
- Download the full report (PDF)
- Download the 2024 Diary of Consumer Payment Choice chart data (XLSX)

About the Diary of Consumer Payment Choice
The Federal Reserve conducts the Diary of Consumer Payment Choice survey every year to understand U.S. consumers’ payment behavior, preferences and how consumer payments change from one year to the next. The latest survey was conducted in October 2023. Understanding the evolving role of cash in the U.S. economy through the Diary studies helps ensure FedCash Services is fulfilling its mission of meeting cash demand in times of both normalcy and stress, maintaining the public’s confidence in U.S. currency, and providing ready access to cash.
Federal Reserve Financial Services uses data from the Diary to understand consumer cash use and anticipate its ongoing role in the payments landscape. By tracking consumer payment transactions and preferences annually during the month of October, Federal Reserve Financial Services compares cash with other payment instruments, such as credit and debit cards, checks and electronic payment options. Diary participants also report the amount of cash on hand after each survey day, cash stored elsewhere and cash deposits or withdrawals. Analysis of the Diary data includes the impact of age and income on an individual’s payment behavior and preferences, as well as cash stocks and flows at an individual level.
Top of Page
- 2024 Research
- 2023 Research
- 2022 Research
- 2021 Research
- 2020 Research
- 2019 Research
- 2018 Research
- 2017 Research
- 2016 Research
- 2013 Research
- 2010 Research
- 2007 Research
- 2006 Research
- 2001–2004 Research
Potato yield and quality are linked to cover crop and soil microbiome, respectively
- ORIGINAL PAPER
- Open access
- Published: 04 April 2024
- Volume 60 , pages 525–545, ( 2024 )
Cite this article
You have full access to this open access article
- Michael Hemkemeyer ORCID: orcid.org/0000-0002-2554-2231 1 na1 ,
- Sanja A. Schwalb ORCID: orcid.org/0000-0002-7112-3505 1 na1 ,
- Clara Berendonk 2 ,
- Stefan Geisen ORCID: orcid.org/0000-0003-0734-727X 3 ,
- Stefanie Heinze ORCID: orcid.org/0000-0002-2524-7355 4 ,
- Rainer Georg Joergensen ORCID: orcid.org/0000-0002-3142-221X 5 ,
- Rong Li 6 ,
- Peter Lövenich 7 ,
- Wu Xiong ORCID: orcid.org/0000-0001-5766-7998 6 &
- Florian Wichern ORCID: orcid.org/0000-0002-4962-4266 1
924 Accesses
2 Altmetric
Explore all metrics
Crop-specific cultivation practices including crop rotation, cover cropping, and fertilisation are key measures for sustainable farming, for which soil microorganisms are important components. This study aims at identifying links between agronomic practices, potato yield and quality as well as soil microorganisms. We analysed the roles of cover crops and of the soil prokaryotic, fungal, and protistan communities in a long-term trial, differing in crop rotation, i.e. winter wheat or silage maize as pre-crop, presence and positioning of oil radish within the rotation, and fertilisation, i.e. mineral fertiliser, straw, manure, or slurry. Up to 16% higher yields were observed when oil radish grew directly before potatoes. Losses of potato quality due to infection with Rhizoctonia solani -induced diseases and common scab was 43–63% lower when wheat + oil radish was pre-crop under manure or straw + slurry fertilisation than for maize as pre-crop. This contrast was also reflected by 42% higher fungal abundance and differences in β-diversity of prokaryotes, fungi, and protists. Those amplicon sequence variants, which were found in the treatments with highest potato qualities and differed in their abundances from other treatments, belonged to Firmicutes (2.4% of the sequences) and Mortierellaceae (28%), which both comprise potential antagonists of phytopathogens. Among protists, Lobosa, especially Copromyxa , was 62% more abundant in the high potato quality plots compared to all others, suggesting that specific higher trophic organisms can improve crop performance. Our findings suggest that successful potato cultivation is related (1) to planting of oil radish before potatoes for increasing yield and (2) to fertilisation with manure or straw + slurry for enriching the microbiome with crop-beneficial taxa.
Similar content being viewed by others
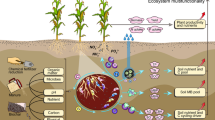
Biochar and organic fertilizer applications enhance soil functional microbial abundance and agroecosystem multifunctionality
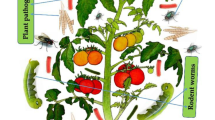
The use of microbial inoculants for biological control, plant growth promotion, and sustainable agriculture: A review
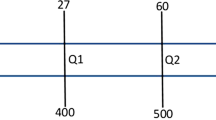
Biochar applications influence soil physical and chemical properties, microbial diversity, and crop productivity: a meta-analysis
Avoid common mistakes on your manuscript.
Introduction
Potatoes ( Solanum tuberosum L.) play an important role in a healthy human diet (Camire et al. 2009 ) and were the fourth most produced food crop in 2021 ( www.fao.org/faostat/ ). However, potatoes have a high demand for fertilisers and are susceptible to a wide range of pathogens and pests, often necessitating pesticide treatments (Wu et al. 2013 ). Among the soil-borne pathogens and pests acting on potatoes, Fiers et al. ( 2012 ) listed 30 genera of bacteria, fungi, protists, and nematodes. Furthermore, there are viruses and arthropod larvae like wireworms (Elateridae), which reduce potato yield and quality (Fiers et al. 2012 ). Reported potato yield losses due to pathogens were 100% by the common scab-inducing actinobacteria Streptomyces spp. (Charkowski et al. 2020 ), up to 47% by the ascomycete Colletotrichum coccodes (Wallr.) S. Hughes (Daami-Remadi et al. 2010 ), and 30% by the dry core-inducing basidiomycete Rhizoctonia solani J.G. Kühn (Tsror 2010 ). Blemishes like common scab, black dots ( C. coccodes ), black scurf ( R. solani ), and silver scurf (ascomycete Helminthosporium solani Durieu & Mont. ) only damage the skin. Consequently, they do not induce yield reduction like for H. solani (Errampalli et al. 2001 ), but severely reduce the economical value of potatoes (Fiers et al. 2012 ).
Crop rotation counteracts decreases in yield and quality associated with monocultures over time. A diverse crop rotation not only increases yields (Blecharczyk et al. 2023 ; Larkin et al. 2021 ; Scholte 1990 ; Wright et al. 2017 ), but also decreases negative effects on quality (Larkin and Honeycutt 2006 ) as well as disease incidents, i.e. abundance of affected plants or their marketable parts, and severity, i.e. the extent of damage (Larkin and Honeycutt 2006 ; Wright et al. 2017 ). For the crop at the end of the rotation, its performance depends on the choice of the preceding one (Honeycutt et al. 1996 ; Mohr et al. 2011 ; Specht and Leach 1987 ).
Cover cropping is an important means to support cash crops like potatoes. Cover crops, also referred to as catch crops, take up soil nitrogen (N) with Brassicaceae like oil radish, trapping up to 200 and more kg N ha −1 (Justes 2017 ). As 40–60% of plant biomass N derives from soil organic matter (Tonitto et al. 2006 ), decayed cover crops play an important role in nutrient management of subsequent cash crops (Wilson et al. 2019 ). They also increase stocks of soil organic carbon (Poeplau and Don 2015 ), thus, improving soil physical conditions for cash crop growth (Kaspar and Singer 2011 ). Overall, cover crops can lead to increased cash crop yields (Marcillo and Miguez 2017 ; Osipitan et al. 2018 ), though the effectiveness depends on agronomic practices like fertilisation (Justes 2017 ). Control of pests and phytopathogens is a further function (Kaspar and Singer 2011 ; Tiwari et al. 2022 ), as inclusion of cover crops can disrupt epidemic cycles by reducing receptiveness of the soil and by allelopathic bio-fumigation (Justes 2017 ). For instance, Brassicaceae cover crops are known to reduce severity of common scab in potatoes (Charkowski et al. 2020 ; Tiwari et al. 2022 ).
The soil microbial community comprising, e.g., prokaryotes, fungi, and protists, provides ecosystem services supporting cash crops. Growth of microorganisms improves soil conditions by aggregation (Oades and Waters 1991 ; Tisdall and Oades 1982 ). Microorganisms release plant available nutrients by weathering of minerals (Gadd 2007 ; Uroz et al. 2009 ) and by degrading soil organic matter (Krishna and Mohan 2017 ) or provide nutrients to plants such as by N 2 fixation, phosphorus (P) solubilisation or production of iron chelating siderophores (Bhattacharyya and Jha 2012 ; Vukicevich et al. 2016 ). Plant growth-promoting rhizobacteria (PGPR), fungi, and oomycetes (both PGPF) as well as mycorrhizal fungi can enhance plant growth by production of phytohormones, relieve of stress or induction of stress tolerance, and induction of immune responses, which strengthens the plants’ resistance and defence against pathogens (Akhtar and Siddiqui 2010 ; Bhattacharyya and Jha 2012 ; Hossain et al. 2017 ; Vukicevich et al. 2016 ). Microorganisms further suppress phytopathogens by competition for nutrients and colonisation sites (Jayaraman et al. 2021 ), by production of antibiotics (Akhtar and Siddiqui 2010 ; Deveau et al. 2018 ; Rodrigo et al. 2021 ), and by parasitising or preying (Geisen et al. 2018 ; Olanya and Lakshman 2015 ; Velicer and Mendes-Soares 2009 ; Vukicevich et al. 2016 ). Higher trophic-level protists control these processes by predation and they also directly benefit plants by liberating nutrients via the microbial loop (Geisen et al. 2018 ). Depending on the microbial community, soils can become disease suppressive or conducive. Suppressive soils harbour higher microbial biomass, biodiversity, and activity than non-suppressive soils (Chandrashekara et al. 2012 ; Jayaraman et al. 2021 ), which can be strongly altered by management, fertilisation (Hemkemeyer et al. 2015 ; Schwalb et al. 2023b ; Zhao et al. 2019 ), and cover cropping (Finney et al. 2017 ; Kim et al. 2020 ).
In the current study, we compared different potato cultivation methods, which aim at increasing yield and quality. For this reason, we analysed soil abiotic and microbial factors in order to explain the link between agronomic approaches, soil microorganisms, and potato production. The Pfalzdorf long-term potato trial, which ran from 2001 to 2019 in the Lower Rhine region, Germany, employed different crop rotations, i.e. winter wheat or silage maize as pre-crop as well as presence and positioning of oil radish within the rotation, and fertilisation types, i.e. mineral fertiliser, straw, manure, or slurry. We hypothesised that those combinations of crop rotation and fertilisation, which led to highest potato yields and qualities in terms of lowest disease incidences, (1) had positioned the cover crop directly before potatoes. These combinations further led to soils containing (2) higher amounts of nutrients and higher microbial (3) abundances, (4) activities, and (5) diversity. Furthermore, they led to (6) microbial communities harbouring more potentially beneficial taxa in terms of plant growth promotion and disease suppression.
Materials and methods
Study site and experimental design.
The study site was located in the Lower Rhine region in Germany (51°43′7.6"N, 6°09′18.1"E, 15 m above sea level) on a loess-derived Stagnic Luvisol with silty loam texture (18.5% 63–2000 µm, 72.3% 2–63 µm, 9.2% < 2 µm). During the trial, mean annual precipitation and temperature was 770 mm and 10.9 °C, respectively (Berendonk 2020 ). The trial ran from 2001 until 2019 starting with potato (cultivar Marabel) as initial crop followed by six full three-year crop rotation cycles (Table 1 ). The treatments differed in the positioning of the cash crops winter wheat ( Triticum aestivum L., different cultivars with Ornica being last) and silage maize ( Zea mays L., different cultivars with Oldham being last) and the cover crop oil radish ( Raphanus sativus var. oleiformis Pers., cultivar Adios) within the crop rotation. Furthermore, there were differences in tillage (absence or timing of ploughing) and fertilisation (mineral fertiliser, shredded straw, cattle manure, pig slurry).
The amounts of N applied depended on the content of inorganic N in the soil and potential delivery from litter decay to reach target values of N content in the soil of 140 kg ha −1 for potatoes, 210 kg ha −1 for winter wheat, 190 kg ha −1 for maize, and 40 kg ha −1 (mineral fertiliser) or 80 kg ha −1 (manure/slurry) for oil radish. Similarly, basic mineral fertilisation of all treatments with P, potassium (K), magnesium (Mg), and calcium (Ca) depended on respective contents and pH in 0–30 cm of soil. For details of fertiliser application see Berendonk ( 2020 ). Seven treatments (T1–T8 with T3 not being sampled) were arranged in a randomised block-design with four replicates at a plot size of 9 × 9 m 2 . The composition of the factors crop rotation, fertilisation, and tillage in the treatments was not factorial, but the treatments followed common farming practices of the region (Table 1 ). As plant protection differed between maize and wheat and, in accordance to needs, between years, treatments T1–T6 (wheat as pre-crop) and T7–T8 (maize as pre-crop) received different types and amounts of pesticides, while within each group of treatments plant protection was the same; it was also similar for potatoes, the most intensively managed crop in the crop rotations (see Supplementary Table S1 ).
Potato harvest and quality assessment, long-term soil sampling
Potatoes were harvested in autumn and yield was determined after each crop rotation cycle, i.e. not for the initial year 2001. At time of the establishment of the field trial, it was common practice to determine potato yield as fresh weight and it is still an important determinant for the market prize. Due to this legacy and the practice-orientation of the trial, only fresh weights were determined, even though dry weights should be reported in future trials (Bashan et al. 2017 ). Potato quality was determined by assessing 100 randomly picked potatoes per replicate visually for tuber deformations, and traits induced by different fungal, bacterial, and faunal pests. In 2016 and 2019 only 25 potatoes per replicate were picked in accordance with the Federal Plant Variety Office but scaled up to 100 to enable comparison. Assessments of the different quality traits started in different years depending on their first appearance.
Accompanying destructive soil sampling took place in autumn of each year from the first 30 cm in order to determine long-term soil nutrient concentrations. The field replicates of each year of these long-term soil samples were combined prior to analyses and sent cooled at + 5 °C to the LUFA Münster, Germany, for analysis. As this combining had led to a loss of field variability, only variability in time could be considered and, thus, the long-term nutrient results are of limited explanatory power.
Soil sampling in 2019 and 2015 and basic soil parameters
For microbial properties and contemporary soil nutrient concentrations, soil sampling took place in October 2019, two days after harvest of the potatoes. Earlier samplings were conducted in February 2015 under young winter wheat (T1–T6) or oil radish (T7–T8) as preceding vegetation and, for molecular genetic analyses only, in May 2019 shortly after seeding of the potatoes with fallow after winter wheat (T1) and maize (T7–T8), respectively, or oil radish (T2–T6) as preceding vegetation. In May 2019 the ridges were sampled from the top down to the level of the furrows without harming the seeding potatoes, while in 2015 and in October 2019 the first 10–15 cm were sampled to account for the levelling of the former ridges. Per replicate, 30 subsamples were taken across a plot, pooled, and homogenised during sieving to < 2 mm. The samples were either stored at + 4 °C until further analysis or, for 2015 and May 2019 samples, frozen at -20 °C for molecular genetic analysis.
Water holding capacity was determined according to Wilke ( 2005 ). For pH measurement the soil was manually stirred at 5 min intervals in a 0.01 M CaCl 2 solution at a ratio of 1:2 (w/v) and measured after 30 min (2015 samples: 1:5 (w/v) in distilled water). For total carbon (C), N, and sulphur (S) determination soil was milled and dry combusted at 900 °C in a Vario Max Cube CHNS (Elementar, Langenselbold, Germany). For extractable soil elements see below.
Incubation experiment, activity measurements, and water-stable aggregates
After moistening the soil up to 50% of its water holding capacity, a 100 g fresh weight sample in a 1-L-bottle and further 10 g in a 100-mL-bottle were pre-incubated at 22 °C in the dark for 7 d. Afterwards the 10 g sample was stored frozen (-20 °C) until determination of inorganic N (t 0 ). The 1-L-bottle was supplied with a vial containing 10 mL 0.5 M NaOH and incubated for a further 7 d to determine basal respiration. Soil from this incubation was used to measure soil extractable and microbial elements (see below) and, after storage at -20 °C, inorganic N (t 1 ), water-stable aggregates, and molecular parameters were measured. For the 2015 samples the incubation conditions were as following: 3 d pre-incubation, 35 d main incubation, and exchange of alkali traps every 7 d with reduction of NaOH concentration to 0.25 M after 14 d; measurements were constrained to basal respiration and soil extractable and microbial biomass C and N.
Inorganic N, i.e. the sum of NH 4 + -N, NO 2 − -N, and NO 3 − -N, was measured using a continuous segmented flow analyser (AA3, SEAL Analytical, Norderstedt, Germany) and net N mineralisation within 7 d was calculated as:
For basal respiration, the alkali traps were back-titrated with 0.1 M HCl according to Pell et al. ( 2006 ) using TitroLine 6000 (SI Analytics, Mainz, Germany). Basal respiration divided by soil microbial biomass C resulted in the metabolic quotient ( q CO 2 ).
Water-stable aggregates of replicates B–D were determined after air-drying and sieving to > 1 mm in a wet-sieving apparatus (Eijkelkamp, Giesbeek, The Netherlands) according to the manufacturer. In brief, a mass of 4 g soil was wetted with distilled water using a sprayer and left to soak for 5 min. Subsequently, the sample was wet-sieved with mesh-size 250 µm at 34 strokes per minute, first in distilled water for 3 min (W H2O ) and subsequently in 0.05 M NaOH for 5 min (W NaOH ). After drying at 105 °C, water-stable aggregates (WSA) were calculated as:
with W NaOH, empty and W H2O, empty being the respective empty weights of the vessels and m NaOH being the mass of NaOH used in the vessel.
Soil microbial and extractable elements
Microbial biomass C, N, and P were determined by fumigation-extraction according to Vance et al. ( 1987 ), Brookes et al. ( 1985 ), and Brookes et al. ( 1982 ), respectively, with conversion values 0.45 (Joergensen 1996 ), 0.54 (Joergensen and Mueller 1996 ), and 0.40 (Brookes et al. 1982 ), respectively. Further chloroform-labile elements, i.e. microbial derived elements for which conversion values are not yet available, were extracted with 0.01 M CaCl 2 at 1:20 (w/v) ratio and shaking at 200 rpm for 1 h (Schwalb et al. 2023a ). While C and N were analysed in the Multi C/N 2100 S analyser (Analytic Jena, Jena, Germany), P and other elements were measured in an inductively coupled argon plasma optical emission spectrometer (ICP-OES, Optima 8000, Perkin Elmer, Waltham, USA). Due to the low microbial biomass, only for manganese (Mn) reliable data were obtained among the chloroform-labile elements. However, all extracts of the non-fumigated samples were considered as extractable, i.e. easily bioavailable, elements including C, N, and P. Elemental ratios were calculated on a molar and not on a mass base (Schwalb et al. 2023b ).
DNA extraction and quantitative real-time PCR
Genomic DNA was extracted using the FastDNA™ SPIN Kit for Soil and FastPrep®-24 bead-based homogeniser (both MP Bio, Santa Ana, USA). The extraction protocol was slightly modified according to Hemkemeyer et al. ( 2014 ) by adjusting volumes of sodium phosphate buffer and supplied “MT” to 950 µL and 120 µL, respectively. The bead-beater was run twice at 6.5 m s −1 for 45 s. Furthermore, DNA bound to the glass milk was additionally washed two times, using 1 mL 5.5 M guanidine thiocyanate to reduce soil contaminants. Finally, eluate obtained with 100 µL distilled water was added back to the column to elute a second time to increase elution efficiency. DNA lost during the extraction process, i.e. remaining in pellets and non-transferred supernatants, was accounted for by dividing gene copy numbers (see below) by k DNA :
with a = mass of empty reaction tube; b = mass of tube and added supplied “PPS”; c = mass of tube, “PPS”, and added crude DNA extract; d = mass of tube after centrifugation and removing supernatant; m s = soil sample fresh weight; u = gravitational soil water content in %; m spb = mass of sodium phosphate buffer; m MT = mass of “MT” buffer.
Quantification of microbial abundances was done in the Light Cycler 480® II (Roche Diagnostics, Mannheim, Germany), using the Light Cycler 480® Probes Master for bacterial 16S rRNA genes (primers BAC338F and BAC805R and probe BAC516F) and archaeal 16S rRNA genes (primers ARC787F and ARC1059R and probe ARC915F) (Yu et al. 2005 ). Fungal ITS1 sequences were quantified using LightCycler® 480 SYBR Green I Master with the primers NSI1 and 58A2R (Martin and Rygiewicz 2005 ). Reaction mixtures, cycling conditions, and, in case of fungi, conditions of melting curve analysis have been published with open access elsewhere (Wichern et al. 2020 ). For 2019 samples the standard curve was prepared from amplicons derived from Bacillus subtilis (bacteria), Methanobacterium oryzae (archaea), and Fusarium graminearum (fungi) and inserted into a plasmid using the pGEM®-T Vector System II Kit (Promega, Madison, USA). In the case of 2015 samples, amplicons derived from environmental samples without insertion into a plasmid. Efficiencies (Eff) of the qPCR calculated as:
with m = slope of the standard curve are given in the Supplementary Table S2 .
Illumina MiSeq sequencing and bioinformatic analyses
Aliquots of the 2019 samples’ DNA extracts were lyophilised prior to library preparation. The V4 region of the 16S rRNA gene was PCR-amplified to investigate prokaryotic communities using the primer set 515F (GTGYCAGCMGCCGCGGTAA) and 806R (GGACTACNVGGGTWTCTAAT) (Caporaso et al. 2011 ). Meanwhile, the V4 region of the 18S rRNA gene was broadly targeted to investigate eukaryotic communities using the primer set V4_1f (CCAGCASCYGCGGTAATWCC) and TAReukREV3 (ACTTTCGTTCTTGATYRA) (Bass et al. 2016 ). PCR was performed in a 20 µl volume consisting of 4 µl of 5× reaction buffer, 2 µL dNTPs (2.5 mM), 0.8 µL of each primer (10 µM), 0.4 µL FastPfu Polymerase, 10 ng of DNA template, and the rest being ddH 2 O. Amplification was performed with the following temperature regime: 5 min of initial denaturation at 95 °C, followed by 30 cycles of denaturation (95 °C for 30 s), annealing (55 °C for 30 s), extension (72 °C for 45 s), and a final extension at 72 °C for 10 min. PCR products were pooled in equimolar concentrations of 10 ng µL −1 . Paired-end sequencing was performed on an Illumina MiSeq sequencer at Personal Biotechnology (Shanghai, China). Sequencing of the 18S rRNA gene of one May 2019 sample (T1, replicate D) failed.
We analysed raw sequencing data of 16S rRNA gene and 18S rRNA gene using previously established protocols (Xiong et al. 2021 ) with some modifications. For 16S rRNA gene analyses, paired-end reads were merged with USEARCH v11 (Edgar 2010 ) and merged sequences with expected errors > 1.0 or a length < 220 bp were removed. We further identified amplicon sequence variant (ASV) with the UNOISE3 algorithm (Edgar 2016 ), which simultaneously removed chimeras. We removed the 16S ASVs that contained fewer than 10 reads across all the samples. Finally, the 16S ASV representative sequences were matched against the RDP database (Cole et al. 2014 ; Wang et al. 2007 ). To focus on prokaryotic communities, we removed the reads assigned as chloroplast, mitochondria, and eukaryotes. For 18S rRNA gene analyses, merged sequences with a length shorter than 300 bp were removed. Representative eukaryotic ASVs were taxonomically classified against the PR 2 database, which though focussing on protists also covers fungi (Guillou et al. 2013 ). To focus on fungal and protistan communities, we removed sequencing reads of Rhodophyta, Streptophyta, and Metazoa. Finally the eukaryotic data set was split into a fungal and a protistan one. In certain cases, consensus sequences were further checked, using the Standard Nucleotide BLAST at https://blast.ncbi.nlm.nih.gov/Blast.cgi (Altschul et al. 1990 ).
Statistical analyses
Statistical analyses were performed in R (R Core Team 2023 ). Microbial and elemental ratios were natural logarithm-transformed prior to statistical analyses (Isles 2020 ) and, if parametric, are given as geometric means ± mean 95% confidence intervals as obtained by R package DescTools (Signorell et al. 2022 ). Other data with continuous response are either given as arithmetic mean ± standard deviation or as median ± median absolute deviation in dependence of the statistical test used. Residuals were checked for normal distribution using Q-Q-plots supplemented by normal curve analysis and Shapiro–Wilk test as provided by stat.desc command from pastecs (Grosjean and Ibanez 2018 ). Similarly, evaluation of residual-versus-fitted plots for checking for homoscedasticity was supplemented by Brown-Forsythe test using leveneTest command from car (Fox and Weisberg 2019 ). In cases requirements for Analysis of Variance (ANOVA) were not met, data were Box-Cox transformed using MASS (Venables and Ripley 2002 ). One-way ANOVA was performed using car and, if requirements after transformation were still not met, Scheirer-Ray-Hare test was employed using rcompanion (Mangiafico 2023 ). For considering block effects, this was included as main effect.
In order to account for repeated measures, for potato yield over time, a linear mixed effects model with treatment, year, and block as fixed effects and plot as random effect was employed using nlme (Pinheiro et al. 2023 ), but the according Box-Cox transformation value λ was obtained from a linear model excluding the random effect. In the case of maize and wheat yields the rotation cycle instead of the year had to be included as fixed effect. In contrast, in the case of long-term soil data, which were obtained after combining the field replicates, sampling time was used for replication and the rotation cycle served as random effect.
Potato quality is expressed as percentage, though data was obtained by counting. Potato quality data over the whole course of the field trial was analysed with generalised linear mixed models and potato quality data of 2019 alone was analysed in addition with generalised linear models, both based on negative binomial distribution using glmer.nb from lme4 (Bates et al. 2015 ) and glm.nb from MASS, respectively, together with Anova command from car . Dispersion was checked using package DHARMa (Hartig 2022 ). Depending on the nature of residuals, as post hoc tests either Estimated Marginal Means using emmeans (Lenth 2023 ) together with the cld command from multcomp (Hothorn et al. 2008 ) or Dunn test using FSA (Ogle et al. 2023 ) together with the cldList command from rcompanion were conducted. All graphics were prepared using ggplot2 (Wickham 2016 ) with support of scales (Wickham et al. 2023 ), patchwork (Pedersen 2023 ) and cowplot (Wilke 2020 ).
Sampling efficiency of high-throughput sequencing data was estimated using rarefaction curves calculated with iNEXT (Chao et al. 2014 ; Hsieh et al. 2020 ) indicating sufficient sampling of prokaryotes, fungi, and protists (Supplementary Fig. S1 ). Calculations for α- and β-diversity were conducted using the R package vegan (Oksanen et al. 2022 ). In order to consider different library sizes with smallest ones being 132,649, 5,895, and 36,969 for prokaryotes, fungi, and protists, respectively (for averages see Supplementary Table S6 ), each ASV table was rarefied randomly 1,000 times and α- and β-diversity were calculated iteratively with finally determining the medians (Hemkemeyer et al. 2019 ). Observed richness, abundance-based coverage estimator (ACE), exponential Shannon–Wiener index (e H’ ), and Pielou’s index (J’) were determined using the estimateR command. The exponential form of the Shannon-Wiener index was chosen, because it uses numbers of species as unit and is thus easier to interpret (Krebs 1999 ). Prior to Bray–Curtis dissimilarity determination ( vegdist command), rarefied counts were square-root transformed to reduce the weight of the most abundant taxa. Differences between treatments were compared by permutational multivariate Analysis of Variance (PERMANOVA, adonis2 command). Despite highly significant differences, subsequent pairwise comparison using pairwiseAdonis (Arbizu 2017 ) could not discern the differing treatments. Homogeneity of variance was checked via permutational analysis of multivariate dispersions (PERMDISP, betadisper command) and for visualisation non-metric multidimensional scaling (NMDS, metaNMDS command) was employed.
The taxa differing between treatments were identified using edgeR (Robinson et al. 2010 ). Prokaryotes, fungi, and protists were analysed separately by starting with selecting data using cut-offs of 50, 200, and 100 counts per million in at least four samples, respectively, in accordance with the different orders of magnitude between libraries of the three taxonomic groups (Chen et al. 2015 ). The different library sizes within each taxonomic group were accounted for by normalisation based on the weighted trimmed mean of log expression ratios “TMM”-method (Robinson and Oshlack 2010 ). This analysis used the generalised linear model approach (McCarthy et al. 2012 ) with control of the false discovery rate using the algorithm by Benjamini and Hochberg ( 1995 ). Results are shown as heatmaps with displayed taxa being restricted to the most abundant ones.
Long-term crop yields
When winter wheat was the preceding cash crop, planting of oil radish as cover crop in between increased potato yields by 11–16% (F = 49.7, p < 0.001, Fig. 1 a). The different fertilisers employed on oil radish had no further effects. When silage maize preceded potatoes and, thus, oil radish within the crop rotation was positioned prior to maize, potato yields were 8% lower than or similar to the treatment completely omitting the cover crop. In contrast, in both maize-as-pre-crop treatments, maize yields were 4% higher compared to all other treatments (F = 4.37, p = 0.007, Fig. 1 b), while wheat yields did not differ between any cultivation method (F = 1.51, p = 0.230, Supplementary Fig. S2 a). During six crop rotations and ignoring the extreme year 2010, potato yields decreased by 36–44% compared to the first harvest in 2004 depending on the treatment (F = 533.9, p < 0.001, Fig. 1 a).
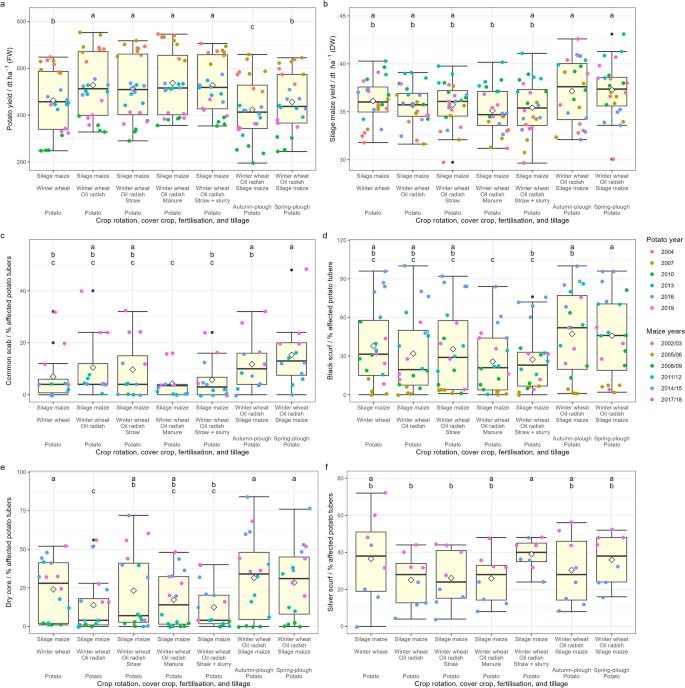
Yield of potatoes ( a ) and silage maize ( b ) and potato quality indicated by infection with pathogenic Streptomyces spp.-induced common scab ( c ), Rhizoctonia solani -induced black scurf ( d ) and dry core ( e ), and Helminthosporium solani -induced silver scurf ( f ) over several years. Diamonds represent means of four replicates; letters indicate significant differences between treatments (p < 0.05)
Long-term potato quality
The reduction of potato quality was highest with maize as pre-crop, contrasting wheat + oil radish as pre-crop with either manure or straw + slurry application, which lowered infections by 63% and 43% with common scab (Χ 2 = 26.5, p < 0.001, Fig. 1 c) and black scurf (Χ 2 = 26.1, p < 0.001, Fig. 1 d), respectively. A similar result was observed for dry core with straw + slurry application, leading to 58% lower infections (Χ 2 = 29.5, p < 0.001, Fig. 1 e). For silver scurf an adverse effect was found in the latter treatment, in which 52% more potatoes were infected than under wheat + oil radish with mineral or straw alone fertilisation (Χ 2 = 17.9, p = 0.006, Fig. 1 f). The different cultivation methods had no long-term effects on deformations (Χ 2 = 9.41, p = 0.152), black dot disease (Χ 2 = 6.56, p = 0.363), and wireworm attacks (Χ 2 = 4.14, p = 0.658), despite of noticeable occasions in specific years (Supplementary Fig. S2 b–d). Like yield, potato quality decreased over time in all rotation systems, i.e. disease incidences increased 3–9-fold for common scab, 16–49-fold for black scurf (except for 2019), 14–65-fold for dry core, and 1.2–3.1-fold for silver scurf (Figs. 1 c–f, Supplementary Table S3 ) and in 2019 wireworms became noticeable.
Long-term soil abiotic characteristics
During the whole trial, wheat + oil radish-pre-crop treatments receiving manure or straw + slurry contained 9–12% and 13–15% higher concentrations of soil organic matter (SOM) and Mg, respectively, than under maize as pre-crop ploughed in spring. Both treatments showed 13–21% more P and 21–27% more K than most other treatments (Supplementary Fig. S3 a–d, Table S4 ). Where oil radish grew in autumn, the content of inorganic N was three-fold lower than where the cover crop was omitted (F = 9.0, p < 0.001, Fig. S3 e). Soil pH did not differ (Fig. S3 f, Table S4 ).
Plant and soil abiotic characteristics in 2019 and 2015
In contrast to the long-term results given above, at potato harvest in 2019, plant quality characteristics hardly differed between treatments (Table S3 ). Similarly, no treatment effects were observed on soil abiotic factors (Table 2 ). Soil had 62% water-stable aggregates and a pH of 6.4. Total contents of C and N were 11.7 and 0.95 mg g −1 , respectively, and for extractable nutrients like P (65.7 µg g −1 ) and K (181 µg g −1 ), see Table 2 . Already in February 2015, when sampling took place at another stage of the crop rotation, hardly any differences in abiotic characteristics were observed (Supplementary Table S5 , Fig. S4 a).
Microbial abundances, activities, and α-diversities in 2019 and 2015
At harvest in 2019, microbial biomass C was unaffected by cultivation treatments, which was also true for microbial derived N, P, and Mn, microbial elemental molar ratios (C:N:P 13:2:1) and the metabolic quotient q CO 2 (Table 3 ). However, fungal abundances, indicated by ITS1 copies, were 42% more abundant where wheat + oil radish grew before potatoes with manure or straw + slurry application than in treatments with maize as pre-crop (F = 7.05, p < 0.001, Fig. 2 a). In contrast, bacterial and archaeal 16S rRNA gene copies g −1 soil as well as microbial ratios did not respond to treatments (Table 3 , molecular bacteria:archaea:fungi ratio 70:2:1). Observed richness of prokaryotic, fungal, and protistan amplicon sequence variants (ASVs) as well as estimated richness, diversity index, and, except for protists, evenness were also unaffected by cultivation treatments (Supplementary Table S6 , Fig. S4 b). With few exceptions, these patterns were in line with findings from sampling in 2015 (Table S5 , Fig. S4 c) and at seeding time (Table 3 , Figs. 2 b–d, Supplementary Table S6 , Fig. S4 d).
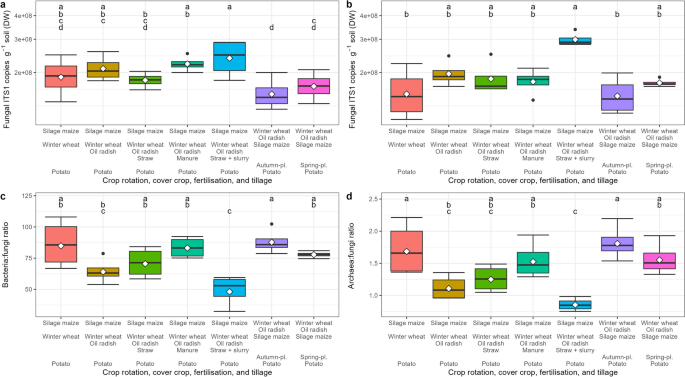
Fungal abundance in samples of October 2019 ( a ) and May 2019 ( b ) and fungal ratios in May 2019 with bacteria ( c ) and archaea ( d ). Diamonds represent means of four replicates; letters indicate significant differences between treatments (p < 0.05); statistical results of ANOVA (F) and Scheirer-Ray-Hare (H) tests were a: F = 7.05, p < 0.001; b: H = 12.94, p = 0.044; c: F = 9.02, p < 0.001; d: F = 12.29, p < 0.001
Microbial β-diversity and taxa differing between cultivation treatments in 2019
Among prokaryotic communities, two groups of treatments clustered most strongly away from each other at harvest: one group consisted of those treatments with wheat + oil radish as pre-crop and manure or straw + slurry application, while the other group contained the treatments with maize as pre-crop (F = 1.16, p = 0.009, Fig. 3 a). Clustering between different pre-crops was even more pronounced for fungi (F = 1.63, p = 0.001, Fig. 3 b) and protists (F = 1.46, p = 0.001, Fig. 3 c). Where wheat was the pre-crop, fungi were further separated by straw + slurry application. At seeding, mentioned clusters in all three microbial groups were even stronger separated from each other (Fig. 4 ). An overview of the microbial community compositions is given in Supplementary Results S1 and Figs. S5 – S7 .
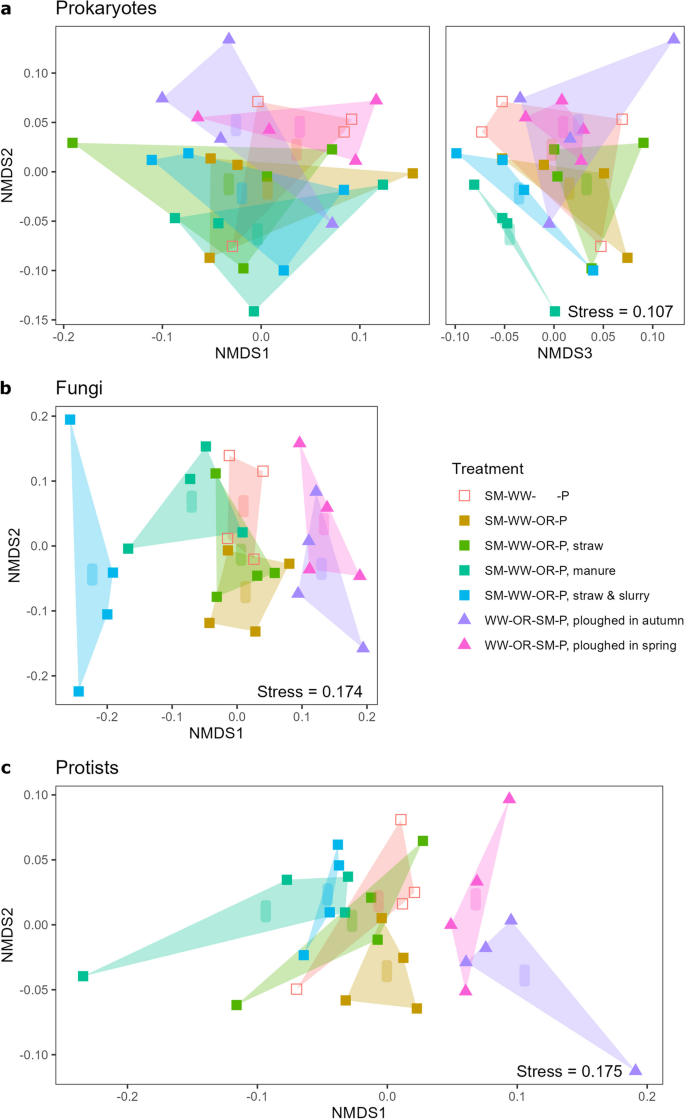
Non-metric multidimensional scaling plots of samples taken at harvest in October 2019 for treatments differing in the crop rotations of silage maize (SM), winter wheat (WW), oil radish (OR), and potatoes (P). Upright rounded rectangles indicate the position of the centroids
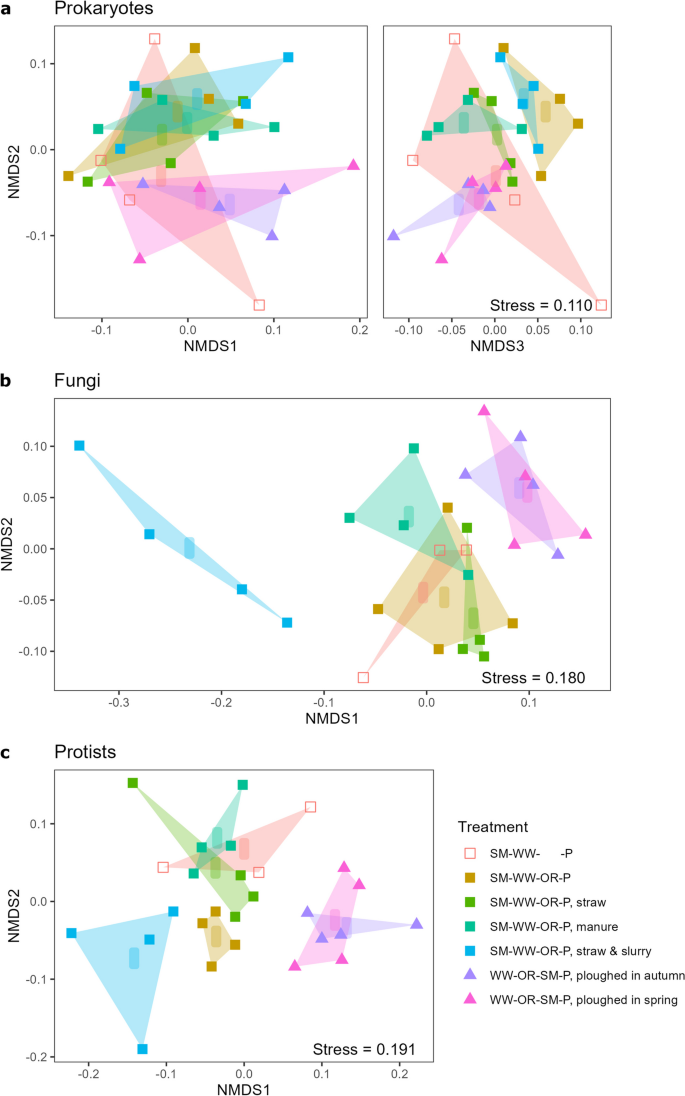
Non-metric multidimensional scaling plots of samples taken at seeding in May 2019 for treatments differing in the crop rotations of silage maize (SM), winter wheat (WW), oil radish (OR), and potatoes (P); upright rounded rectangles indicate the position of the centroids. Results of PERMANOVA were a: F = 1.232, p = 0.001; b: F = 2.006, p = 0.001; c: F = 1.574, p = 0.001
Analysis of ASVs differing between cultivation methods at harvest showed that 0.5% of the prokaryotic ones making up to about 3% of the sequences in the libraries derived from treatments with wheat + oil radish as pre-crops under manure or straw + slurry application, while they accounted for 1.3–1.6% of the sequences in the other treatments. Strongest drivers of this pattern were Firmicutes (2.0–2.4%) with the classes Bacilli, Clostridia, and Erysipelotrichia (Fig. 5 , Supplementary Table S7 ). Both maize-as-pre-crop treatments shared a Streptomyces ASV (Zotu569, Actinobacteria, 0.06%), which according to BLAST was not related to common scab-inducing species.
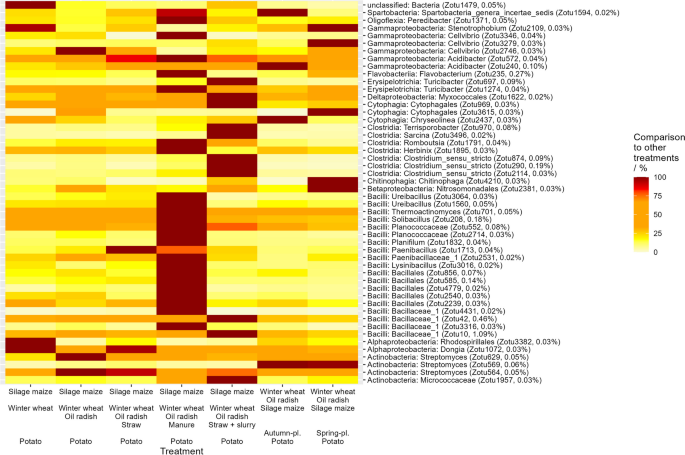
Top 50 abundant prokaryotic ASVs significantly differing between potato cultivation treatments at harvest in October 2019. ASVs are given as class for purpose of ordering, the lowest taxonomic rank to which an ASV was identified, ASV number (Zotu), and the mean percentage of ASV sequences to all sequences in the treatment containing the highest abundance of the given ASV. For statistical results see Supplementary Table S7
Among fungi, 8% of ASVs differed between treatments, of which most of them could only be classified to subphylum or class level (Fig. 6 , Supplementary Table S8 ). While in most treatments they accounted for 3–10% of the sequences, under straw + slurry application they reached 38%. This treatment fostered several ASVs comprising Mortierella /Mucoromycotina (28%) with next relatives all belonging to Mortierellaceae according to BLAST (Mucoromycota) and Pezizomycotina/ Pezizomycetes (Ascomycota, 9%) with the majority being related to Ascodesmis . Both maize-as-pre-crop treatments shared Acremonium persicinum (Ascomycota, 0.3%).
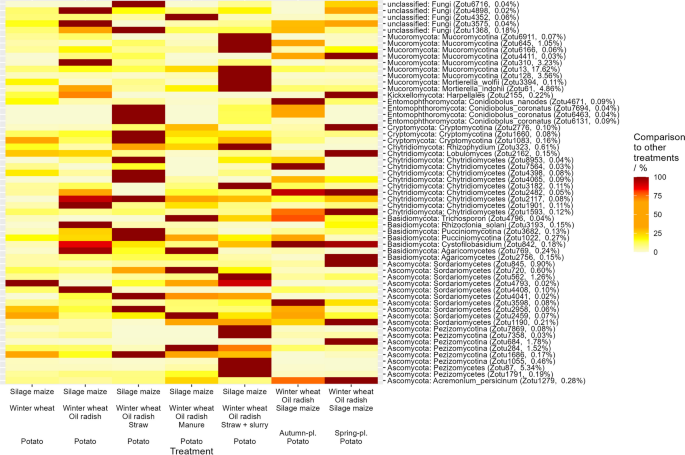
Fungal ASVs significantly differing between potato cultivation treatments at harvest in October 2019. ASVs are given as phylum for purpose of ordering, the lowest taxonomic rank to which an ASV was identified, ASV number (Zotu), and the mean percentage of ASV sequences to all sequences in the treatment containing the highest abundance of the given ASV. For statistical results see Supplementary Table S8
Also, the 3% protistan ASVs differing were most abundant in the treatment with straw + slurry application with 18% of the sequences, while in the other treatments they ranged 11–14%. Here a Neoheteromita -ASV (Cercozoa, 3.2%) was 2.3-fold more abundant (Fig. 7 , Supplementary Table S9 ). However, in both the straw + slurry and the manured treatment ASVs of Lobosa, especially Copromyxa , and Ochrophyta were 62% and two-fold, respectively, more abundant than in other treatments. Where maize was the pre-crop, Chlorophyta-ASVs were 64% more abundant. For more differing ASVs, see Supplementary Results S2.
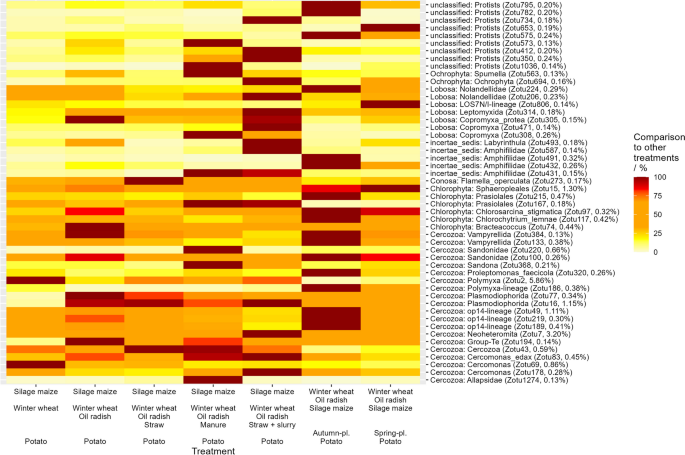
Top 50 abundant protistan ASVs significantly differing between potato cultivation treatments at harvest in October 2019. ASVs are given on a high rank, e.g. phylum, for purpose of ordering, the lowest taxonomic rank to which an ASV was identified, ASV number (Zotu), and the mean percentage of ASV sequences to all sequences in the treatment containing the highest abundance of the given ASV. For statistical results see Supplementary Table S9
When calculating β-diversity across seeding and harvest data, NMDS (data not shown) and PERMANOVA indicated a strong effect of sampling time for composition of prokaryotic (F = 3.50, p = 0.001), fungal (F = 4.66, p = 0.001), and protistan communities (F = 8.22, p = 0.001) without significant interaction of treatment and sampling time (p ≥ 0.478). Percentages of differing prokaryotic, fungal, and protistan ASVs and their contribution to respective libraries were always much larger at seeding with 0.8% (library contribution 3.0–6.9%), 14% (9.2–45.7%), and 4.7% (15.9–25.4%), respectively, than at harvest. Also, the 50 most abundant differing ASVs differed between both sampling times ( cf. Figure 5 – 7 and Supplementary Figs. S8 – S10 , Tables S10 – 12 ). However, several ASVs showing differences at harvest already displayed these differences at seeding time like several ASVs of the Firmicutes (e.g. Zotu10, 290, 697), Streptomyces (Zotu629, 569), Mucoromycotina (Zotu310, 12, 128), Pezizomycetes (Zotu87, 1791), A. persicinum (Zotu1279), Plasmodiophorida (Zotu16), Neoheteromita (Zotu7), Prasiolales (Zotu167), Cercozoa (Zotu43), and Sandonidae (Zotu100).
Potato yield and the role of oil radish as cover crop
Combinations of wheat as pre-cash crop and subsequent oil radish led to highest potato yields in the current study. The different cultivation methods led to differences in the long-term soil characteristics (SOM, P, K, Mg), but their patterns did not match with yield, thus, there is no correlation between yield and concentration of these nutrients. For samplings in 2015 and 2019, there were hardly any differences in the nutritional status of soils, i.e. hypothesis 2 partly rejected for yield. Also, the differences in microbial abundances, activities, and α-diversities, i.e. hypotheses 3–5 must be rejected for yield. The same is true for microbial β-diversity patterns. Thus, yield effects can be related to the presence of the cover crop or its position within the rotation.
The lower yield in the treatment omitting the cover crop could be connected to the number of fertilisation occasions, which was one less per cycle. Furthermore, soil inorganic N content was lower after oil radish cropping (T2–T8) than under fallow after wheat (T1), despite omitted fertilisation, indicating N uptake by the cover crop. Nutrients taken up remain on the field and get released upon the decay of cover crops (Kaspar and Singer 2011 ; Maltais-Landry and Frossard 2015 ). On the one hand, the cover crop with its accompanying fertilisation represents a direct input of additional nutrients and, on the other hand, it prevents the loss of already available nutrients by leaching, e.g. nitrate, or gaseous emission, e.g. nitrous oxide. Accordingly, potatoes of the treatment omitting oil radish missed out on further nutrition. Thus, hypothesis 2 is rejected for yield, as the according nutrients were not stored freely in the soil but in the cover crop.
When maize was the pre-crop of potatoes, oil radish was grown one year earlier and, thus, directly before maize. Therefore, it was maize, which benefitted from the cover crop as shown by higher maize yields in these treatments as also observed by Kaye and Quemada ( 2017 ). In contrast, wheat always grew before the cover crop and did not show direct yield benefits. Consequently, the cover crop directly grown before potatoes causes the difference in yield, rather than direct impacts by wheat or maize as pre-crops. Benefits in potato yield by oil radish have also been reported by Hamzaev et al. ( 2007 ). As silage maize gets harvested later than winter wheat, there is not enough time for subsequent growth of cover crops for sufficient N uptake (Kivelitz 2017 ; Komainda et al. 2016 ). Thus, maize as pre-crop has an indirect effect on potato yield by precluding cover crops with function as catch crops, i.e. hypothesis 1 confirmed for yield.
Potato quality and microbial communities
Highest long-term potato qualities regarding lowest infection with common scab, black scurf, and dry core were found where wheat + oil radish was the pre-crop under application of manure or straw + slurry, contrasting both treatments with maize as pre-crop. The high qualities were accompanied by highest long-term concentrations of extractable P and K, whereas both contrasting treatments only partially matched the long-term patterns of SOM and extractable Mg, i.e. hypothesis 2 partly confirmed, partly rejected for quality depending on nutrient. Higher nutritional supply can strengthen the resistance of plants against pathogens (Chandrashekara et al. 2012 ). In 2019, when there were hardly any differences in potato qualities, there were no differences in soil abiotic characteristics detectable. Similar to abiotic factors in 2015, this came along with missing differences in microbial abundances, activity, and α-diversity, i.e. hypotheses 4–5 rejected for quality. Suppressive soils generally contain higher values in these microbial properties than non-suppressive soils (Chandrashekara et al. 2012 ; Jayaraman et al. 2021 ).
However, fungal abundance in 2019 was an exception, indeed matching the long-term quality patterns with positive correlation, i.e. hypothesis 3 mainly rejected for quality except in case of fungi. A similar result for fungi was not observed for the 2015 samples, when the field was in another stage of the crop rotation and two characteristic treatments (T6 and T8) were not included in qPCR analysis. However, the 2019 patterns of β-diversity of prokaryotes, fungi, and protists matched the long-term quality patterns. The different cultivation methods, i.e. pre-crop (wheat vs. maize including differences in tillage intensity and pesticide application) and different fertilisation types led to changes in the microbial community compositions. For instance, regarding the different types of fertilisation associated with the cover crop, a nutrient that is supplied in mineral form can address other microbial species than one supplied in organic form (Lilleskov et al. 2002 ). The match of disease suppression in treatments between 2004 and 2016 with fungal abundance and microbial β-diversity in 2019 could be related to a microbial legacy as discussed below. In contrast, silver scurf showed a different pattern of treatments in regard to lowest or highest percentages of infected tubers, whereas black dot disease was unaffected by the different cultivation methods. Accordingly, confirmation or rejection of hypotheses in regard to quality depends on kind of disease.
The omission of the cover crop leading to a fallow period had no obvious effect, as this treatment had medium disease incidence. Microbial communities clustered between both contrasting groups, together with the other wheat-as-pre-crop treatments without animal-derived fertiliser. In contrast to meta-analytical findings (Kim et al. 2020 ), cover crop inclusion compared to omission had no significant effects on microbial abundance, activity, or diversity in our study, i.e. hypothesis 1 cannot be related to hypotheses 3–5 for quality. For fungal communities in intensive potato production, it has been shown that, despite a three-year crop rotation, they will shift to a potato adopted community (Manici and Caputo 2009 ).
Comparison of differing ASVs between seeding and harvest
At harvest, in all treatments potatoes were grown, while at seeding time the recent rhizospheric legacy derived from three different preceding crops, i.e. oil radish cover crop, maize, or wheat still prevailed to an unknown extent. During potato growth the numbers and sums of contributions of differing ASVs were reduced along with the reduced plant diversity (from three pre-crops to one main crop), revealing the importance of considering the pre-crop legacy for soil microbial investigations. Nevertheless, several ASVs showing a specific association with a certain treatment were found at both time points. Remaining relic DNA cannot be ruled out (Levy-Booth et al. 2007 ) and, as many of the ASVs were classified as spore formers or showing other kinds of persistence (Bulman and Neuhauser 2017 ; Howe et al. 2009 ; Ottow 2011 ) like dormancy (Joergensen and Wichern 2018 ), inactive cells might have contributed to the observed patterns. However, several of these ASVs include potential antagonists and plant growth promoting rhizobacteria, which could have played a role in influencing the quality of potatoes. As no microbiological investigations were done at the beginning of the trial or throughout the 19 year trial, it can only be speculated whether the same or similar taxa were already involved in potato quality in the past.
Microbial members potentially involved in potato quality
Firmicutes, which drove the difference under manure and (straw +)slurry application, process fresh and simple substrates with some taxa even being specialised on urinary sources and, after food sources decline, their cells can survive in the form of spores (Ottow 2011 ). The effect on the bacterial community composition in the manure and slurry treatments mainly derives from fostering members of the autochthonous community rather than from establishment of allochthonous livestock gut-derived bacteria (Chu et al. 2007 ; Sun et al. 2015 ). Bacillus species/isolates are commonly found among genera suppressing diseases (Agrios 2005 ), including common scab (Braun et al. 2017 ), R. solani -induced diseases (Kiptoo et al. 2021 ), and silver scurf (Avis et al. 2010 ). Several Bacillus species/isolates are often considered as plant growth promoting rhizobacteria for potatoes (Calvo et al. 2010 ; Ekin 2019 ; Ghyselinck et al. 2013 ; Hanif et al. 2015 ). Paenibacillus is a known antagonist of R. solani (Brewer and Larkin 2005 ), while Lysinibacillus , Clostridium sensu stricto, and Turicibacter were enriched in the geocaulosphere soil, i.e. the soil surrounding the tuber, associated with reduced common scab occurrence (Shi et al. 2019 ).
The finding of Ascodesmis among the Pezizomycotina/Pezizomycetes in straw + slurry is not surprising, as this genus, though also found in soil, is strictly coprophilous (Kristiansen 2011 ; van Brummelen 1981 ) and also some members of Mortierellaceae have been isolated from dung (Domsch et al. 2007 ). The latter family is often reported to degrade chitin (Domsch et al. 2007 ) and one member of Mortierella was found being antagonistic against common scab-inducing Streptomyces spp. (Tagawa et al. 2010 ). Under potato monocultures, which become prone to pathogens over time, abundances of Mortierellales were decreased (Liu et al. 2014 ).
Among protistan Lobosa, several mycophagous species also feed on spores (Geisen et al. 2016 ) and could thus be potential predators of phytopathogenic fungi. Especially the appearance of Copromyxa spp. in the manure/straw + slurry treatments is not surprising as these are coprophilic organisms which employ partly a “slime mould” life style (Brown et al. 2011 ). Thus, the treatments leading to highest potato quality harboured more beneficial prokaryotic, fungal, and protistan taxa in terms of plant growth promotion and disease suppression, i.e. confirming hypothesis 6 in regard to potato quality.
In the treatments with maize as pre-crop, the time point of ploughing led to different driving ASVs. However, some ASVs were common amongst both. The genus Acremonium was increased in potato monocultures, which became prone to pathogens over time (Liu et al. 2014 ). As the Streptomyces ASVs could not be identified further, their role remains unclear. Many non-pathogenic Streptomyces species are also common PGPR (Bhat et al. 2022 ) and suppressors of diseases (Agrios 2005 ), including common scab (Braun et al. 2017 ) and silver scurf (Avis et al. 2010 ). Despite incidences of infections increased over time, ASVs of phytopathogens were hardly detected in 2019 samples as discussed in Supplementary Discussion S1.
Limitations of the study design
The design of the field trial was based on regional potato cultivation and crop rotation practices and, thus, had not a factorial design. Accordingly, some factors cannot be discerned. Tillage intensity and pest management differed not within, but between the treatments having wheat (T1–T6) and maize (T7–T8) as pre-crop and might had influenced the outcomes, which in the following are ascribed to the both pre-crop treatment groups. Furthermore, when in 2019 the samplings for microbial community analyses were completed, potato qualities showed no significant differences for the first time. Therefore, this study discusses microbial data in the context of long-term agronomic results rather than just focussing on the concerted sampling year 2019. However, microbial community abundances, activities, and diversities were only analysed in 2019 when potatoes were present and in the previous crop rotation cycle in 2015 with no potatoes being present. These communities represent the legacy of five to six crop rotation cycles under different treatments and the contemporary conditions at the sampling times, which we cannot disentangle, as accompanying samplings had not been done during the earlier cycles. Nevertheless, we still believe that the major impact arises from the direct predecessor and results should mostly represent the legacy left by the crop grown directly before.
The results discussed above are in particular representative for the last crop rotation cycles, however, they likely show the imprint of the differences in crop rotation and management. It would be of high value if future long-term trials combine agronomic and microbial measurements constantly to also document potential temporal changes in the microbiome with potential impact on potato yield and quality effects. However, this is hardly the case in the current literature, as either microbiologists or plant scientists with a focus on agronomy initiate investigations. We therefore have a strong plea for more truly interdisciplinary research with relevance for agricultural practise.
Conclusions
Six three-year crop rotation cycles of cultivation methods for potatoes, differing in pre-crop/tillage intensity, cover crop inclusion, and type of fertilisation led to clear impacts on yield and quality. On the one hand, the long-term differences in potato quality regarding common scab and R. solani -induced diseases were reflected by the community compositions of prokaryotes, fungi, and protists in 2019. This contrasted between wheat + oil radish under manure or straw + slurry application and the treatments with preceding maize. Several ASVs found in the first group of treatments correlated with higher potato qualities potentially caused by plant growth promoting rhizobacteria or antagonists of phytopathogens. Whether these taxa were already earlier involved in improved potato quality remains speculative in the current long-term trial. This first group of treatments also contained highest fungal abundances and long-term soil P and K. On the other hand, potato yield was reflected by the presence or positioning of the cover crop within the rotation, as the crop directly succeeding oil radish received its benefits and produced higher yields. This study demonstrates that a cover crop preceding potatoes and fertilisation with manure or straw + slurry can be recommended for obtaining high yields and qualities. Future agronomic long-term trials should consider microbiological analyses right from the beginning. Such a combined monitoring could also take dynamics due to temporal variability into account enabling the disentanglement of short- and long-term effects of the interplay between agronomic management, crop species and soil microbial communities.
Data availability
The long-term agronomic and soil data are available at request ([email protected]). The single time point soil and microbial data obtained in 2019 and 2015 are provided as Supplementary Data S1 and S2 , respectively. The raw sequences are available from the Genome Sequence Archive ( https://ngdc.cncb.ac.cn/gsa ) under accession number CRA010961 with the sample description consisting of treatment number, block, and indicator for sampling time, i.e. S for seeding and H for harvest, to be found under BioProject number PRJCA016846.
Agrios GN (2005) Plant Pathology, 5th edn. Elsevier Academic Press, Burlington / San Diego / London
Google Scholar
Akhtar MS, Siddiqui ZA (2010) Role of plant growth promoting rhizobacteria in biocontrol of plant diseases and sustainable agriculture. In: Maheshwari DK (ed) Plant Growth and Health Promoting Bacteria. Springer, Berlin / Heidelberg, pp 157–195
Chapter Google Scholar
Altschul SF, Gish W, Miller W, Myers EW, Lipman DJ (1990) Basic local alignment search tool. J Mol Biol 215:403–410. https://doi.org/10.1016/s0022-2836(05)80360-2
Article CAS PubMed Google Scholar
Arbizu PM (2017) pairwiseAdonis: Pairwise Multilevel Comparison using Adonis. R package version 0.4.1. https://github.com/pmartinezarbizu/pairwiseAdonis
Avis TJ, Martinez C, Tweddell RJ (2010) Integrated management of potato silver scurf ( Helminthosporium solani ). Can J Plant Pathol 32:287–297. https://doi.org/10.1080/07060661.2010.508627
Article CAS Google Scholar
Bashan Y, Huang P, Kloepper JW, de-Bashan L, (2017) A proposal for avoiding fresh-weight measurements when reporting the effect of plant growth-promoting (rhizo)bacteria on growth promotion of plants. Biol Fertil Soils 53:1–2. https://doi.org/10.1007/s00374-016-1153-1
Article Google Scholar
Bass D, Silberman JD, Brown MW, Pearce RA, Tice AK, Jousset A, Geisen S, Hartikainen H (2016) Coprophilic amoebae and flagellates, including Guttulinopsis , Rosculus and Helkesimastix , characterise a divergent and diverse rhizarian radiation and contribute to a large diversity of faecal-associated protists. Environ Microbiol 18:1604–1619. https://doi.org/10.1111/1462-2920.13235
Bates D, Mächler M, Bolker B, Walker S (2015) Fitting linear mixed-effects models using lme4. J Stat Softw 67:1–48. https://doi.org/10.18637/jss.v067.i01
Benjamini Y, Hochberg Y (1995) Controlling the False Discovery Rate: a practical and powerful approach to multiple testing. J Roy Stat Soc B Met 57:289–300. https://doi.org/10.1111/j.2517-6161.1995.tb02031.x
Berendonk C (2020) Kartoffelfruchtfolgeversuch Goch-Pfalzdorf 2002–2019. Untersuchungen zur Verbesserung von Ertrag, Qualität, Pflanzengesundheit, Humusbilanz, Bodenstruktur und Stickstoffverwertung in einer intensiven Hackfruchtfruchtfolge mit Kartoffeln. Landwirtschaftskammer Nordrhein-Westfalen, https://www.landwirtschaftskammer.de/riswick/versuche/pflanzenbau/zwischenfruechte/veroeffentlichungen/endbericht_kartoffelfruchtfolgeversuch.pdf
Bhat BA, Tariq L, Nissar S, Islam ST, Islam SU, Mangral Z, Ilyas N, Sayyed RZ, Muthusamy G, Kim W, Dar TUH (2022) The role of plant-associated rhizobacteria in plant growth, biocontrol and abiotic stress management. J Appl Microbiol 133:2717–2741. https://doi.org/10.1111/jam.15796
Bhattacharyya PN, Jha DK (2012) Plant growth-promoting rhizobacteria (PGPR): emergence in agriculture. World J Microbiol Biotechnol 28:1327–1350. https://doi.org/10.1007/s11274-011-0979-9
Blecharczyk A, Kowalczewski PŁ, Sawinska Z, Rybacki P, Radzikowska-Kujawska D (2023) Impact of crop sequence and fertilization on potato yield in a long-term study. Plants 12:495. https://doi.org/10.3390/plants12030495
Article CAS PubMed PubMed Central Google Scholar
Braun S, Gevens A, Charkowski A, Allen C, Jansky S (2017) Potato common scab: a review of the causal pathogens, management practices, varietal resistance screening methods, and host resistance. Am J Potato Res 94:283–296. https://doi.org/10.1007/s12230-017-9575-3
Brewer MT, Larkin RP (2005) Efficacy of several potential biocontrol organisms against Rhizoctonia solani on potato. Crop Protect 24:939–950. https://doi.org/10.1016/j.cropro.2005.01.012
Brookes PC, Powlson DS, Jenkinson DS (1982) Measurement of microbial biomass phosphorus in soil. Soil Biol Biochem 14:319–329. https://doi.org/10.1016/0038-0717(82)90001-3
Brookes PC, Landman A, Pruden G, Jenkinson DS (1985) Chloroform fumigation and the release of soil nitrogen: a rapid direct extraction method to measure microbial biomass nitrogen in soil. Soil Biol Biochem 17:837–842. https://doi.org/10.1016/0038-0717(85)90144-0
Brown MW, Silberman JD, Spiegel FW (2011) “Slime molds” among the Tubulinea (Amoebozoa): Molecular systematics and taxonomy of Copromyxa . Protist 162:277–287. https://doi.org/10.1016/j.protis.2010.09.003
Article PubMed Google Scholar
Bulman S, Neuhauser S (2017) Phytomyxea. In: Archibald JM, Simpson AGB, Slamovits CH (eds) Handbook of the Protists, 2nd edn. Springer, London, pp 783–803
Calvo P, Ormeño-Orrillo E, Martínez-Romero E, Zúñiga D (2010) Characterization of Bacillus isolates of potato rhizosphere from andean soils of Peru and their potential PGPR characteristics. Braz J Microbiol 41:899–906. https://doi.org/10.1590/S1517-83822010000400008
Article PubMed PubMed Central Google Scholar
Camire ME, Kubow S, Donnelly DJ (2009) Potatoes and human health. Crit Rev Food Sci Nutr 49:823–840. https://doi.org/10.1080/10408390903041996
Caporaso JG, Lauber CL, Walters WA, Berg-Lyons D, Lozupone CA, Turnbaugh PJ, Fierer N, Knight R (2011) Global patterns of 16S rRNA diversity at a depth of millions of sequences per sample. Proc Natl Acad Sci 108:4516–4522. https://doi.org/10.1073/pnas.1000080107
Chandrashekara C, Bhatt JC, Kumar R, Chandrashekara KN (2012) Suppressive soils in plant disease management. In: Singh VK, Singh Y, Singh A (eds) Eco-friendly innovative approaches in plant disease management. International Book Publishers and Distributors, Dehradun, India, pp 241–256
Chao A, Gotelli NJ, Hsieh TC, Sander EL, Ma KH, Colwell RK, Ellison AM (2014) Rarefaction and extrapolation with Hill numbers: a framework for sampling and estimation in species diversity studies. Ecol Monogr 84:45–67. https://doi.org/10.1890/13-0133.1
Charkowski A, Sharma K, Parker ML, Secor GA, Elphinstone J (2020) Bacterial diseases of potato. In: Campos H, Ortiz O (eds) The Potato Crop. Its Agricultural, Nutritional and Social Contribution to Humankind. Springer, Cham, Switzerland, pp 351–388
Chen Y, McCarthy D, Robinson M, Smyth GK (2015) edgeR: differential expression analysis of digital gene expression data - User's Guide. Version 2015–04–08. http://www.bioconductor.org/packages/release/bioc/vignettes/edgeR/inst/doc/edgeRUsersGuide.pdf
Chu H, Lin X, Fujii T, Morimoto S, Yagi K, Hu J, Zhang J (2007) Soil microbial biomass, dehydrogenase activity, bacterial community structure in response to long-term fertilizer management. Soil Biol Biochem 39:2971–2976. https://doi.org/10.1016/j.soilbio.2007.05.031
Cole JR, Wang Q, Fish JA, Chai B, McGarrell DM, Sun Y, Brown CT, Porras-Alfaro A, Kuske CR, Tiedje JM (2014) Ribosomal Database Project: data and tools for high throughput rRNA analysis. Nucleic Acids Res 42:D633–D642. https://doi.org/10.1093/nar/gkt1244
Daami-Remadi M, Bouallègue R, Jabnoun-Khiareddine H, El Mahjoub M (2010) Comparative aggressiveness of Tunisian Colletotrichum coccodes isolates on potato assessed via black dot severity, plant growth and yield loss. Pest Technol 4:45–53
Deveau A, Bonito G, Uehling J, Paoletti M, Becker M, Bindschedler S, Hacquard S, Hervé V, Labbé J, Lastovetsky OA, Mieszkin S, Millet LJ, Vajna B, Junier P, Bonfante P, Krom BP, Olsson S, van Elsas JD, Wick LY (2018) Bacterial–fungal interactions: ecology, mechanisms and challenges. FEMS Microbiol Rev 42:335–352. https://doi.org/10.1093/femsre/fuy008
Domsch KH, Gams W, Anderson T-H (2007) Compendium of soil fungi, 2nd edn. IHW-Verlag, Eching
Edgar RC (2010) Search and clustering orders of magnitude faster than BLAST. Bioinformatics 26:2460–2461. https://doi.org/10.1093/bioinformatics/btq461
Edgar RC (2016) UNOISE2: improved error-correction for Illumina 16S and ITS amplicon sequencing. bioRxiv 13(1):081257. https://doi.org/10.1101/081257
Ekin Z (2019) Integrated use of humic acid and plant growth promoting rhizobacteria to ensure higher potato productivity in sustainable agriculture. Sustainability 11:3417. https://doi.org/10.3390/su11123417
Errampalli D, Saunders JM, Holley JD (2001) Emergence of silver scurf ( Helminthosporium solani ) as an economically important disease of potato. Plant Pathol 50:141–153. https://doi.org/10.1046/j.1365-3059.2001.00555.x
Fiers M, Edel-Hermann V, Chatot C, Le Hingrat Y, Alabouvette C, Steinberg C (2012) Potato soil-borne diseases. A Review Agron Sustain Dev 32:93–132. https://doi.org/10.1007/s13593-011-0035-z
Finney DM, Buyer JS, Kaye JP (2017) Living cover crops have immediate impacts on soil microbial community structure and function. J Soil Water Conserv 72:361–373. https://doi.org/10.2489/jswc.72.4.361
Fox J, Weisberg S (2019) An R Companion to Applied Regression, 3rd edn. Sage, Thousand Oaks, CA
Gadd GM (2007) Geomycology: biogeochemical transformations of rocks, minerals, metals and radionuclides by fungi, bioweathering and bioremediation. Mycol Res 111:3–49. https://doi.org/10.1016/j.mycres.2006.12.001
Geisen S, Koller R, Hünninghaus M, Dumack K, Urich T, Bonkowski M (2016) The soil food web revisited: Diverse and widespread mycophagous soil protists. Soil Biol Biochem 94:10–18. https://doi.org/10.1016/j.soilbio.2015.11.010
Geisen S, Mitchell EAD, Adl S, Bonkowski M, Dunthorn M, Ekelund F, Fernández LD, Jousset A, Krashevska V, Singer D, Spiegel FW, Walochnik J, Lara E (2018) Soil protists: a fertile frontier in soil biology research. FEMS Microbiol Rev 42:293–323. https://doi.org/10.1093/femsre/fuy006
Ghyselinck J, Velivelli SLS, Heylen K, O’Herlihy E, Franco J, Rojas M, De Vos P, Prestwich BD (2013) Bioprospecting in potato fields in the Central Andean Highlands: Screening of rhizobacteria for plant growth-promoting properties. Syst Appl Microbiol 36:116–127. https://doi.org/10.1016/j.syapm.2012.11.007
Grosjean P, Ibanez F (2018) pastecs: Package for analysis of space-time ecological series. R package version 1.3.21. https://www.CRANR-projectorg/package=pastecs
Guillou L, Bachar D, Audic S, Bass D, Berney C, Bittner L, Boutte C, Burgaud G, de Vargas C, Decelle J, del Campo J, Dolan JR, Dunthorn M, Edvardsen B, Holzmann M, Kooistra WHCF, Lara E, Le Bescot N, Logares R, Mahé F, Massana R, Montresor M, Morard R, Not F, Pawlowski J, Probert I, Sauvadet A-L, Siano R, Stoeck T, Vaulot D, Zimmermann P, Christen R (2013) The Protist Ribosomal Reference database (PR 2 ): a catalog of unicellular eukaryote Small Sub-Unit rRNA sequences with curated taxonomy. Nucleic Acids Res 41:D597–D604. https://doi.org/10.1093/nar/gks1160
Hamzaev AX, Astanakulov TE, Ganiev IM, Ibragimov GA, Oripov MA, Islam KR (2007) Cover crops impacts on irrigated soil quality and potato production in Uzbekistan. In: Change C, Carbon T (eds) R Lal, M Suleimenov, BA Stewart, DO Hansen, P Doraiswamy. Sequestration in Central Asia Taylor & Francis, London, pp 349–359
Hanif K, Hameed S, Imran A, Naqqash T, Shahid M, van Elsas JD (2015) Isolation and characterization of a β-propeller gene containing phosphobacterium Bacillus subtilis strain KPS-11 for growth promotion of potato ( Solanum tuberosum L.). Front Microbiol 6. https://doi.org/10.3389/fmicb.2015.00583
Hartig F (2022) DHARMa: residual diagnostics for hierarchical (multi-level / mixed) regression models. R package version 0.4.5. https://www.CRANR-projectorg/package=DHARMa
Hemkemeyer M, Pronk GJ, Heister K, Kögel-Knabner I, Martens R, Tebbe CC (2014) Artificial soil studies reveal domain-specific preferences of microorganisms for the colonisation of different soil minerals and particle size fractions. FEMS Microbiol Ecol 90:770–782. https://doi.org/10.1111/1574-6941.12436
Hemkemeyer M, Christensen BT, Martens R, Tebbe CC (2015) Soil particle size fractions harbour distinct microbial communities and differ in potential for microbial mineralisation of organic pollutants. Soil Biol Biochem 90:255–265. https://doi.org/10.1016/j.soilbio.2015.08.018
Hemkemeyer M, Christensen BT, Tebbe CC, Hartmann M (2019) Taxon-specific fungal preference for distinct soil particle size fractions. Eur J Soil Biol 94:103103. https://doi.org/10.1016/j.ejsobi.2019.103103
Honeycutt CW, Clapham WM, Leach SS (1996) Crop rotation and N fertilization effects on growth, yield, and disease incidence in potato. Am Potato J 73:45–61. https://doi.org/10.1007/BF02854760
Hossain MM, Sultana F, Islam S (2017) Plant growth-promoting fungi (PGPF): Phytostimulation and induced systemic resistance. In: Singh DP, Singh HB, Prabha R (eds) Plant-Microbe Interactions in Agro-Ecological Perspectives, vol 2. Microbial Interactions and Agro-Ecological Impacts. Springer, Singapore, pp 135–191
Hothorn T, Bretz F, Westfall P (2008) Simultaneous inference in general parametric models. Biometrical J 50:346–363. https://doi.org/10.1002/bimj.200810425
Howe AT, Bass D, Vickerman K, Chao EE, Cavalier-Smith T (2009) Phylogeny, taxonomy, and astounding genetic diversity of Glissomonadida ord. nov., the dominant gliding zooflagellates in soil (Protozoa: Cercozoa). Protist 160:159–189. https://doi.org/10.1016/j.protis.2008.11.007
Hsieh TC, Ma KH, Chao A (2020) iNEXT: iNterpolation and EXTrapolation for species diversity. R package version 2.0.20. https://www.CRANR-projectorg/package=iNEXT
Isles PDF (2020) The misuse of ratios in ecological stoichiometry. Ecology 101:e03153. https://doi.org/10.1002/ecy.3153
Jayaraman S, Naorem AK, Lal R, Dalal RC, Sinha NK, Patra AK, Chaudhari SK (2021) Disease-suppressive soils—beyond food production: a critical review. J Soil Sci Plant Nut 21:1437–1465. https://doi.org/10.1007/s42729-021-00451-x
Joergensen RG (1996) The fumigation-extraction method to estimate soil microbial biomass: calibration of the k EC value. Soil Biol Biochem 28:25–31. https://doi.org/10.1016/0038-0717(95)00102-6
Joergensen RG, Mueller T (1996) The fumigation-extraction method to estimate soil microbial biomass: calibration of the k EN value. Soil Biol Biochem 28:33–37. https://doi.org/10.1016/0038-0717(95)00101-8
Joergensen RG, Wichern F (2018) Alive and kicking: Why dormant soil microorganisms matter. Soil Biol Biochem 116:419–430. https://doi.org/10.1016/j.soilbio.2017.10.022
Justes E (2017) Cover Crops for Sustainable Farming. Springer, Dordrecht
Book Google Scholar
Kaspar TC, Singer JW (2011) The use of cover crops to manage soil. In: Hatfield JL, Sauer TJ (eds) Soil Management: Building a Stable Base for Agriculture. American Society of Agronomy and Soil Science Society of America, Madison, WI, pp 321–337
Kaye JP, Quemada M (2017) Using cover crops to mitigate and adapt to climate change. A Review Agron Sustain Dev 37:4. https://doi.org/10.1007/s13593-016-0410-x
Kim N, Zabaloy MC, Guan K, Villamil MB (2020) Do cover crops benefit soil microbiome? A meta-analysis of current research. Soil Biol Biochem 142:107701. https://doi.org/10.1016/j.soilbio.2019.107701
Kiptoo J, Abbas A, Bhatti AM, Usman HM, Shad MA, Umer M, Atiq MN, Alam SM, Ateeq M, Khan M, Peris NW, Razaq Z, Anwar N, Iqbal S (2021) Rhizoctonia solani of potato and its management: A review. Plant Prot 5:157–169. https://doi.org/10.33804/pp.005.03.3925
Kivelitz H (2017) Saatzeiten von Zwischenfrüchten optimieren. Landwirtschaftskammer Nordrhein-Westfalen. https://www.landwirtschaftskammer.de/riswick/versuche/pflanzenbau/zwischenfruechte/veroeffentlichungen/Saatzeiten_Zwischenfruechte_2017.pdf
Komainda M, Taube F, Kluß C, Herrmann A (2016) Above- and belowground nitrogen uptake of winter catch crops sown after silage maize as affected by sowing date. Eur J Agron 79:31–42. https://doi.org/10.1016/j.eja.2016.05.007
Krebs CJ (1999) Ecological Methodology. Benjamin/Cummings, Menlo Park, CA
Krishna MP, Mohan M (2017) Litter decomposition in forest ecosystems: a review. Energ Ecol Environ 2:236–249. https://doi.org/10.1007/s40974-017-0064-9
Kristiansen R (2011) The genus Ascodesmis (Pezizales) in Norway. Ascomyceteorg 2:65–69. https://doi.org/10.25664/art-0040
Larkin RP, Honeycutt CW (2006) Effects of different 3-year cropping systems on soil microbial communities and rhizoctonia diseases of potato. Phytopathology 96:68–79. https://doi.org/10.1094/phyto-96-0068
Larkin RP, Honeycutt CW, Griffin TS, Olanya OM, He Z (2021) Potato growth and yield characteristics under different cropping system management strategies in Northeastern U.S. Agronomy 11:165
Lenth R (2023) emmeans: Estimated Marginal Means, aka Least-Squares Means. R package version 1.8.9. https://www.CRANR-projectorg/package=emmeans
Levy-Booth DJ, Campbell RG, Gulden RH, Hart MM, Powell JR, Klironomos JN, Pauls KP, Swanton CJ, Trevors JT, Dunfield KE (2007) Cycling of extracellular DNA in the soil environment. Soil Biol Biochem 39:2977–2991. https://doi.org/10.1016/j.soilbio.2007.06.020
Lilleskov EA, Hobbie EA, Fahey TJ (2002) Ectomycorrhizal fungal taxa differing in response to nitrogen deposition also differ in pure culture organic nitrogen use and natural abundance of nitrogen isotopes. New Phytol 154:219–231. https://doi.org/10.1046/j.1469-8137.2002.00367.x
Liu X, Zhang J, Gu T, Zhang W, Shen Q, Yin S, Qiu H (2014) Microbial community diversities and taxa abundances in soils along a seven-year gradient of potato monoculture using high throughput pyrosequencing approach. PLoS ONE 9:e86610. https://doi.org/10.1371/journal.pone.0086610
Maltais-Landry G, Frossard E (2015) Similar phosphorus transfer from cover crop residues and water-soluble mineral fertilizer to soils and a subsequent crop. Plant Soil 393:193–205. https://doi.org/10.1007/s11104-015-2477-6
Mangiafico S (2023) rcompanion: Functions to Support Extension Education Program Evaluation. R package version 2.4.34. Rutgers Cooperative Extension, New Brunswick, New Jersey. https://CRAN.R-project.org/package=rcompanion
Manici LM, Caputo F (2009) Fungal community diversity and soil health in intensive potato cropping systems of the east Po valley, northern Italy. Ann Appl Biol 155:245–258. https://doi.org/10.1111/j.1744-7348.2009.00335.x
Marcillo GS, Miguez FE (2017) Corn yield response to winter cover crops: An updated meta-analysis. J Soil Water Conserv 72:226–239. https://doi.org/10.2489/jswc.72.3.226
Martin KJ, Rygiewicz PT (2005) Fungal-specific PCR primers developed for analysis of the ITS region of environmental DNA extracts. BMC Microbiol 5:28. https://doi.org/10.1186/1471-2180-5-28
McCarthy DJ, Chen YS, Smyth GK (2012) Differential expression analysis of multifactor RNA-Seq experiments with respect to biological variation. Nucleic Acids Res 40:4288–4297. https://doi.org/10.1093/nar/gks042
Mohr RM, Volkmar K, Derksen DA, Irvine RB, Khakbazan M, McLaren DL, Monreal MA, Moulin AP, Tomasiewicz DJ (2011) Effect of rotation on crop yield and quality in an irrigated potato system. Am J Potato Res 88:346–359. https://doi.org/10.1007/s12230-011-9200-9
Oades JM, Waters AG (1991) Aggregate hierarchy in soils. Aust J Soil Res 29:815–828. https://doi.org/10.1071/Sr9910815
Ogle DH, Doll JC, Wheeler P, Dinno A (2023) FSA: Simple Fisheries Stock Assessment Methods. R package version 0.9.5. https://www.CRANR-projectorg/package=FSA
Oksanen J, Simpson GL, Blanchet FG, Kindt R, Legendre P, Minchin PR, O'Hara RB, Solymos P, Stevens MHH, Szoecs E, Wagner H, Barbour M, Bedward M, Bolker B, Borcard D, Carvalho G, Chirico M, De Caceres M, Durand S, Evangelista HBA, FitzJohn R, Friendly M, Furneaux B, Hannigan G, Hill MO, Lahti L, McGlinn D, Ouellette M-H, Cunha ER, Smith T, Stier A, Ter Braak CJF, Weedon J (2022) vegan: Community Ecology Package. R package version 2.6–2. https://www.CRANR-projectorg/package=vegan
Olanya OM, Lakshman DK (2015) Potential of predatory bacteria as biocontrol agents for foodborne and plant pathogens. J Plant Pathol 97:405–417
Osipitan OA, Dille JA, Assefa Y, Knezevic SZ (2018) Cover crop for early season weed suppression in crops: Systematic review and meta-analysis. Agron J 110:2211–2221. https://doi.org/10.2134/agronj2017.12.0752
Ottow JCG (2011) Mikrobiologie von Böden. Springer, Berlin, Biodiversität, Ökophysiologie und Metagenomik
Pedersen TL (2023) patchwork: The composer of plots. R package version 1.1.3. https://www.CRANR-projectorg/package=patchwork
Pell M, Stenström J, Granhall U (2006) Soil respiration. In: Bloem J, Hopkins DW, Benedetti A (eds) Microbiological Methods for Assessing Soil Quality. CAB International, Wallingford, UK, pp 117–126
Pinheiro J, Bates D, Team RC (2023) nlme: Linear and nonlinear mixed effects models. R package version 3.1–164. https://www.CRANR-projectorg/package=nlme
Poeplau C, Don A (2015) Carbon sequestration in agricultural soils via cultivation of cover crops – A meta-analysis. Agric, Ecosyst Environ 200:33–41. https://doi.org/10.1016/j.agee.2014.10.024
R Core Team (2023) R: A language and environment for statistical computing. 4.3.2 edn. R Foundation for Statistical Computing, Vienna. https://www.R-project.org
Robinson MD, Oshlack A (2010) A scaling normalization method for differential expression analysis of RNA-seq data. Genome Biol 11:R25. https://doi.org/10.1186/Gb-2010-11-3-R25
Robinson MD, McCarthy DJ, Smyth GK (2010) edgeR: a Bioconductor package for differential expression analysis of digital gene expression data. Bioinformatics 26:139–140. https://doi.org/10.1093/bioinformatics/btp616
Rodrigo S, García-Latorre C, Santamaria O (2021) Metabolites produced by fungi against fungal phytopathogens: Review, implementation and perspectives. Plants 11:81. https://doi.org/10.3390/plants11010081
Scholte K (1990) Causes of differences in growth pattern, yield and quality of potatoes ( Solanum tuberosum L.) in short rotations on sandy soil as affected by crop rotation, cultivar and application of granular nematicides. Potato Res 33:181–190. https://doi.org/10.1007/BF02358445
Schwalb SA, Khan KS, Hemkemeyer M, Heinze S, Oskonbaeva Z, Joergensen RG, Wichern F (2023a) Chloroform-labile trace elements in soil via fumigation-extraction: steps towards the soil microbial ionome beyond C:N:P. Eur J Soil Sci 74:e13356. https://doi.org/10.1111/ejss.13356
Schwalb SA, Li S, Hemkemeyer M, Heinze S, Joergensen RG, Mayer J, Mäder P, Wichern F (2023b) Long-term differences in fertilisation type change the bacteria:archaea:fungi ratios and reveal a heterogeneous response of the soil microbial ionome in a Haplic Luvisol. Soil Biol Biochem 177:108892. https://doi.org/10.1016/j.soilbio.2022.108892
Shi W, Li M, Wei G, Tian R, Li C, Wang B, Lin R, Shi C, Chi X, Zhou B, Gao Z (2019) The occurrence of potato common scab correlates with the community composition and function of the geocaulosphere soil microbiome. Microbiome 7:14. https://doi.org/10.1186/s40168-019-0629-2
Signorell A, Aho K, Alfons A, Anderegg N, Aragon T, Arachchige C, Arppe A, Baddeley A, Barton K, Bolker B, Borchers HW, Caeiro F, Champely S, Chessel D, Chhay L, Cooper N, Cummins C, Dewey M, Doran HC, Stephane D, Dupont C, Eddelbuettel D, Ekstrom C, Elff M, Enos J, Farebrother RW, Fox J, Francois R, Friendly M, Galili T, Gamer M, Gastwirth JL, Gegzna V, Gel YR, Graber S, Gross J, Grothendieck G, Harrell FE, Jr, Heiberger R, Hoehle M, Hoffmann CW, Hojsgaard S, Hothorn T, Huerzeler M, Hui WW, Hurd P, Hyndman RJ, Jackson C, Kohl M, Korpela M, Kuhn M, Labes D, Leisch F, Lemon J, Li D, Maechler M, Magnusson A, Mainwaring B, Malter D, Marsaglia G, Marsaglia J, Matei A, Meyer D, Miao W, Millo G, Min Y, Mitchell D, Mueller F, Naepflin M, Navarro D, Nilsson H, Nordhausen K, Ogle D, Ooi H, Parsons N, Pavoine S, Plate T, Prendergast L, Rapold R, Revelle W, Rinker T, Ripley BD, Rodriguez C, Russell N, Sabbe N, Scherer R, Seshan VE, Smithson M, Snow G, Soetaert K, Stahel WA, Stephenson A, Stevenson M, Stubner R, Templ M, Temple Lang D, Therneau T, Tille Y, Torgo L, Trapletti A, Ulrich J, Ushey K, VanDerWal J, Venables B, Verzani J, Villacorta Iglesias PJ, Warnes GR, Wellek S, Wickham H, Wilcox RR, Wolf P, Wollschlaeger D, Wood J, Wu Y, Yee T, Zeileis A (2022) DescTools: Tools for descriptive statistics. R package version 0.99.45. https://www.cranr-projectorg/package=DescTools
Specht LP, Leach SS (1987) Effects of crop rotation on Rhizoctonia disease of white potato. Plant Dis 71:433–437. https://doi.org/10.1094/PD-71-0433
Sun R, Zhang X-X, Guo X, Wang D, Chu H (2015) Bacterial diversity in soils subjected to long-term chemical fertilization can be more stably maintained with the addition of livestock manure than wheat straw. Soil Biol Biochem 88:9–18. https://doi.org/10.1016/j.soilbio.2015.05.007
Tagawa M, Tamaki H, Manome A, Koyama O, Kamagata Y (2010) Isolation and characterization of antagonistic fungi against potato scab pathogens from potato field soils. FEMS Microbiol Lett 305:136–142. https://doi.org/10.1111/j.1574-6968.2010.01928.x
Tisdall JM, Oades JM (1982) Organic matter and water-stable aggregates in soils. J Soil Sci 33:141–163. https://doi.org/10.1111/j.1365-2389.1982.tb01755.x
Tiwari RK, Kumar R, Sharma S, Naga KC, Subhash S, Sagar V (2022) Continuous and emerging challenges of silver scurf disease in potato. Int J Pest Manage 68:89–101. https://doi.org/10.1080/09670874.2020.1795302
Tonitto C, David MB, Drinkwater LE (2006) Replacing bare fallows with cover crops in fertilizer-intensive cropping systems: A meta-analysis of crop yield and N dynamics. Agric, Ecosyst Environ 112:58–72. https://doi.org/10.1016/j.agee.2005.07.003
Tsror L (2010) Biology, epidemiology and management of Rhizoctonia solani on potato. J Phytopathol 158:649–658. https://doi.org/10.1111/j.1439-0434.2010.01671.x
Uroz S, Calvaruso C, Turpault MP, Frey-Klett P (2009) Mineral weathering by bacteria: ecology, actors and mechanisms. Trends Microbiol 17:378–387. https://doi.org/10.1016/j.tim.2009.05.004
van Brummelen J (1981) The genus Ascodesmis (Pezizales, Ascomycetes). Persoonia 11:333–358
Vance ED, Brookes PC, Jenkinson DS (1987) An extraction method for measuring soil microbial biomass C. Soil Biol Biochem 19:703–707. https://doi.org/10.1016/0038-0717(87)90052-6
Velicer GJ, Mendes-Soares H (2009) Bacterial predators. Curr Biol 19:R55–R56. https://doi.org/10.1016/j.cub.2008.10.043
Venables WN, Ripley BD (2002) Modern Applied Statistics with S, 4th edn. Springer, New York
Vukicevich E, Lowery T, Bowen P, Úrbez-Torres JR, Hart M (2016) Cover crops to increase soil microbial diversity and mitigate decline in perennial agriculture. A Review Agron Sustain Dev 36:48. https://doi.org/10.1007/s13593-016-0385-7
Wang Q, Garrity GM, Tiedje JM, Cole JR (2007) Naïve Bayesian classifier for rapid assignment of rRNA sequences into the new bacterial taxonomy. Appl Environ Microbiol 73:5261–5267. https://doi.org/10.1128/Aem.00062-07
Wichern F, Islam MR, Hemkemeyer M, Watson C, Joergensen RG (2020) Organic amendments alleviate salinity effects on soil microorganisms and mineralisation processes in aerobic and anaerobic paddy rice soils. Front Sustain Food Syst 4:30. https://doi.org/10.3389/fsufs.2020.00030
Wickham H (2016) ggplot2: Elegant Graphics for Data Analysis. Springer, New York
Wickham H, Pedersen TL, Seidel D (2023) scales: Scale functions for visualization. R package version 1.3.0. https://www.CRANR-projectorg/package=scales
Wilke B-M (2005) Determination of chemical and physical soil properties. In: Margesin R, Schinner F (eds) Manual for Soil Analysis – Monitoring and Assessing Soil Bioremediation. Springer, Berlin, pp 47–95
Wilke CO (2020) cowplot: Streamlined Plot Theme and Plot Annotations for 'ggplot2'. R package version 1.1.1. https://www.CRANR-projectorg/package=cowplot
Wilson R, Culp DA, Peterson S, Nicholson K, Geisseler DJ (2019) Cover crops prove effective at increasing soil nitrogen for organic potato production. Calif Agric 73:79–89. https://doi.org/10.3733/ca.2019a0005
Wright PJ, Falloon RE, Hedderley D (2017) A long-term vegetable crop rotation study to determine effects on soil microbial communities and soilborne diseases of potato and onion. N Z J Crop Hortic Sci 45:29–54. https://doi.org/10.1080/01140671.2016.1229345
Wu F, Wang W, Ma Y, Liu Y, Ma X, An L, Feng H (2013) Prospect of beneficial microorganisms applied in potato cultivation for sustainbale agriculture. Afr J Microbiol Res 7:2150–2158. https://doi.org/10.5897/AJMR12x.005
Xiong W, Jousset A, Li R, Delgado-Baquerizo M, Bahram M, Logares R, Wilden B, de Groot GA, Amacker N, Kowalchuk GA, Shen Q, Geisen S (2021) A global overview of the trophic structure within microbiomes across ecosystems. Environ Int 151:106438. https://doi.org/10.1016/j.envint.2021.106438
Yu Y, Lee C, Kim J, Hwang S (2005) Group-specific primer and probe sets to detect methanogenic communities using quantitative real-time polymerase chain reaction. Biotechnol Bioeng 89:670–679. https://doi.org/10.1002/bit.20347
Zhao Z-B, He J-Z, Geisen S, Han L-L, Wang J-T, Shen J-P, Wei W-X, Fang Y-T, Li P-P, Zhang L-M (2019) Protist communities are more sensitive to nitrogen fertilization than other microorganisms in diverse agricultural soils. Microbiome 7:33. https://doi.org/10.1186/s40168-019-0647-0
Download references
Acknowledgements
We like to thank all the technicians of the Agricultural Chamber involved in the field trial as well as the academic technician and the student assistants for their excellent work and support in the field and the labs. Liu Chen kindly uploaded the sequences. We also acknowledge the constructive feedback of the editor and the anonymous reviewers.
Open Access funding enabled and organized by Projekt DEAL. The field trial and the long-term analyses were funded by the Agricultural Chamber of North Rhine-Westphalia. The academic analyses were funded by the Ministry of [Climate Action,] Environment, Agriculture, Nature Conservation and Consumer Protection of the State North Rhine-Westphalia (project EffiZwisch) and the Ministry of Culture and Science of the State North Rhine-Westphalia in association with Project Management Jülich (grant number 005–1703-0025, project Soil ionoMICS). Open access publication was enabled by project DEAL.
Author information
Michael Hemkemeyer and Sanja A. Schwalb contributed equally.
Authors and Affiliations
Soil Science and Plant Nutrition, Sustainable Food Systems Research Centre, Rhine-Waal University of Applied Sciences, Marie-Curie-Str. 1, 47533, Kleve, Germany
Michael Hemkemeyer, Sanja A. Schwalb & Florian Wichern
Agricultural Centre Riswick, Agricultural Chamber of North Rhine-Westphalia, Elsenpaß 5, 47533, Kleve, Germany
Clara Berendonk
Laboratory for Nematology, Department of Plant Sciences, Wageningen University and Research, Droevendaalsesteeg 1, 6708PB, Wageningen, The Netherlands
Stefan Geisen
Department of Soil Science & Soil Ecology, Ruhr-University Bochum, Universitätsstr. 150, 44801, Bochum, Germany
Stefanie Heinze
Department of Soil Biology and Plant Nutrition, University of Kassel, Nordbahnhofstr. 1a, 37213, Witzenhausen, Germany
Rainer Georg Joergensen
College of Resources and Environmental Sciences, Nanjing Agricultural University, Nanjing, 210095, Jiangsu, People’s Republic of China
Rong Li & Wu Xiong
Agricultural Centre Köln-Auweiler, Agricultural Chamber of North Rhine-Westphalia, Gartenstr. 11, 50765, Köln-Auweiler, Germany
Peter Lövenich
You can also search for this author in PubMed Google Scholar
Contributions
Conceptualisation: Clara Berendonk (field trial), Stefan Geisen, Stefanie Heinze, Florian Wichern; Data curation: Clara Berendonk, Wu Xiong; Formal analysis: Michael Hemkemeyer, Sanja A. Schwalb; Funding acquisition: Clara Berendonk (field trial), Florian Wichern; Investigation: Michael Hemkemeyer, Sanja A. Schwalb, Clara Berendonk, Peter Lövenich, Wu Xiong; Methodology: Sanja A. Schwalb; Project administration: Clara Berendonk (field trial), Peter Lövenich (field trial), Florian Wichern; Resources: Rong Li; Supervision: Rainer Georg Joergensen, Florian Wichern; Validation: Stefan Geisen; Visualisation: Michael Hemkemeyer, Sanja A. Schwalb; Writing – Original Draft: Michael Hemkemeyer; Writing – Review & Editing: all authors.
Corresponding author
Correspondence to Michael Hemkemeyer .
Ethics declarations
Competing interests.
The authors declare that they have no known competing financial interests or personal relationships that could have appeared to influence the work reported in this paper.
Additional information
Publisher's note.
Springer Nature remains neutral with regard to jurisdictional claims in published maps and institutional affiliations.
Supplementary Information
Below is the link to the electronic supplementary material.
Supplementary file1 (PDF 4711 KB)
Supplementary file2 (xlsx 5190 kb), supplementary file3 (txt 6 kb), supplementary file4 (txt 4 kb), rights and permissions.
Open Access This article is licensed under a Creative Commons Attribution 4.0 International License, which permits use, sharing, adaptation, distribution and reproduction in any medium or format, as long as you give appropriate credit to the original author(s) and the source, provide a link to the Creative Commons licence, and indicate if changes were made. The images or other third party material in this article are included in the article's Creative Commons licence, unless indicated otherwise in a credit line to the material. If material is not included in the article's Creative Commons licence and your intended use is not permitted by statutory regulation or exceeds the permitted use, you will need to obtain permission directly from the copyright holder. To view a copy of this licence, visit http://creativecommons.org/licenses/by/4.0/ .
Reprints and permissions
About this article
Hemkemeyer, M., Schwalb, S.A., Berendonk, C. et al. Potato yield and quality are linked to cover crop and soil microbiome, respectively. Biol Fertil Soils 60 , 525–545 (2024). https://doi.org/10.1007/s00374-024-01813-0
Download citation
Received : 04 July 2023
Revised : 09 March 2024
Accepted : 11 March 2024
Published : 04 April 2024
Issue Date : May 2024
DOI : https://doi.org/10.1007/s00374-024-01813-0
Share this article
Anyone you share the following link with will be able to read this content:
Sorry, a shareable link is not currently available for this article.
Provided by the Springer Nature SharedIt content-sharing initiative
- Common scab
- Crop rotation
- Helminthosporium solani
- Rhizoctonia solani
- Silver scurf
- Find a journal
- Publish with us
- Track your research
share this!
May 13, 2024
This article has been reviewed according to Science X's editorial process and policies . Editors have highlighted the following attributes while ensuring the content's credibility:
fact-checked
trusted source
Advancing fruit crop resilience: Unveiling the molecular dynamics of abscission in woody fruit crops
by Chinese Academy of Sciences
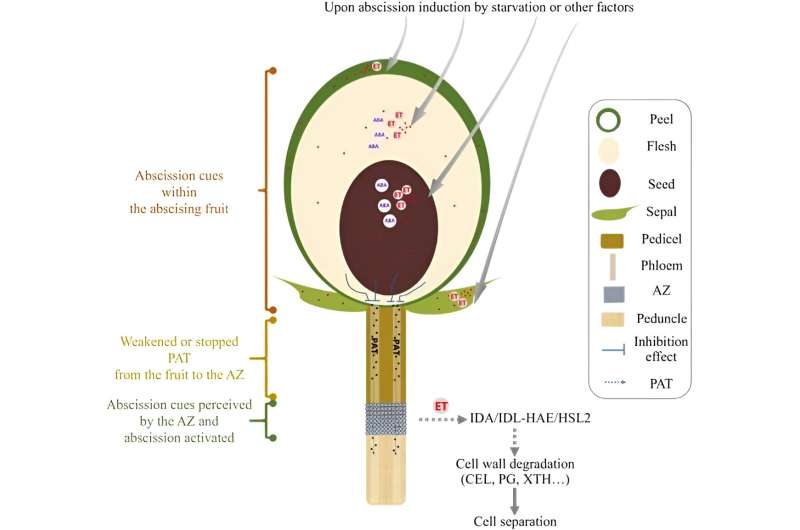
A research team has made significant strides in understanding the mechanisms of fruit abscission in woody fruit crops, an essential process affecting fruit yield and economic value. This review highlights key findings, such as the role of the IDA-HAE/HSL2 signaling pathway in reacting to abscission cues and the influence of reactive oxygen species in controlling abscission dynamics.
The value of this research lies in its potential applications in enhancing fruit crop breeding and refining harvesting techniques by manipulating these molecular processes. Future studies will further clarify the interplay between ethylene signaling and abscission cues, aiming to develop more precise genetic interventions to improve crop resilience and productivity.
Fruit abscission is pivotal for the vegetative and reproductive growth of fruit crop species and has been a focus of plant biology research from historical to modern times. This natural process, crucial for thinning excess flowers and managing fruit loads, involves complex genetic and hormonal interactions, particularly in model plants like Arabidopsis and tomato.
However, challenges persist in woody fruit crops such as litchi and citrus, where uncontrolled abscission leads to significant fruit drop, necessitating physical and chemical interventions to maintain yield.
A review article published in Fruit Research on 2 April 2024 aims to explore the specific mechanisms of fruit abscission in woody species, focusing on signal generation, transmission, and perception within the abscission zone, areas that remain underexplored and crucial for improving commercial cultivation practices.
This review comprehensively examines the mechanisms of fruit abscission in woody fruit crops, emphasizing the critical roles of abscission zones (AZs) and the molecular pathways that regulate this process. The AZs, forming early during organ development, are specialized tissues that differentiate to facilitate cell separation upon receiving abscission cues.
Significant advances in genetic and molecular studies , particularly in model plants like Arabidopsis and tomato, have highlighted the role of key transcription factors and signaling pathways, such as the IDA-HAE/HSL2 module, in regulating abscission. However, research on woody fruit crops lags, with many regulatory genes and mechanisms still unidentified or poorly understood. Furthermore, the review points out that ethylene and auxin play central roles in the abscission process, with ethylene promoting and auxin inhibiting abscission.
This review focuses on the signals leading to abscission, such as the depletion of polar auxin transport (PAT) triggered by carbohydrate shortages, which then activates activated ethylene signaling pathways. This cascade is hypothesized to be sensed by the IDA-HAE/HSL2 pathway, leading to the initiation of the abscission process.
The study's lead researcher, Jianguo Li, says, "In this review, we focus on fruit abscission, particularly discussing the nature of abscission cues within the abscising fruit, how these signals are generated and transmitted, and how the abscission zone cells perceive and respond to these signals in woody fruit crops."
This review identifies significant gaps in understanding the specific tissue roles in hormone synthesis and the interactions between ethylene and auxin in regulating fruit abscission, suggesting critical areas for future research in the physiology and molecular biology of fruit abscission in woody crops.
Provided by Chinese Academy of Sciences
Explore further
Feedback to editors

From roots to resilience: Investigating the vital role of microbes in coastal plant health
4 hours ago

Temperature, time and blueberry wine: Researchers examine fermentation's effects on health-promoting compounds

Heating proteins to body temperature reveals new drug targets

What fire ants can teach us about making better self-healing materials
5 hours ago

Robotic 'superlimbs' could help moonwalkers recover from falls

A novel multifunctional catalyst turns methane into valuable hydrocarbons
6 hours ago

NASA's Juno provides high-definition views of Europa's icy shell

New research addresses alleged benefits of a vegan diet for dogs

Trees on a university campus endure droughts with help from leaky pipes

First direct imaging of radioactive cesium atoms in environmental samples
7 hours ago
Relevant PhysicsForums posts
Is it usual for vaccine injection site to hurt again during infection, a brief biography of dr virgina apgar, creator of the baby apgar test.
May 12, 2024
Who chooses official designations for individual dolphins, such as FB15, F153, F286?
May 9, 2024
The Cass Report (UK)
May 1, 2024
Is 5 milliamps at 240 volts dangerous?
Apr 29, 2024
Major Evolution in Action
Apr 22, 2024
More from Biology and Medical
Related Stories

Understanding jasmonic acid: A switch that activates autophagy in Arabidopsis petals
Feb 7, 2024

Xylem functionality is not a direct indicator of apple preharvest fruit drop: Study
Jan 15, 2024

Revealing the interactions between ABA and ethylene signaling during tomato fruit ripening
Aug 15, 2022

Study integrates key genetic factors to enhance melon quality and aroma
Dec 21, 2023

Regulation of fleshy fruit ripening: From transcription factors to epigenetic modifications
Aug 8, 2022

Deciphering the ethylene biosynthesis puzzle in banana fruit ripening
Feb 5, 2024
Recommended for you

New strategy suppresses unwanted deletion events to make genome editing safer and more precise

Research examines how embryonic development decisions are controlled by multiple pathways simultaneously
9 hours ago

How neighboring whales learn each other's language
10 hours ago

Scientists discover some mice are monogamous due to previously unknown hormone-generating cells

Genetics provide key to fight crown-of-thorns starfish
11 hours ago
Let us know if there is a problem with our content
Use this form if you have come across a typo, inaccuracy or would like to send an edit request for the content on this page. For general inquiries, please use our contact form . For general feedback, use the public comments section below (please adhere to guidelines ).
Please select the most appropriate category to facilitate processing of your request
Thank you for taking time to provide your feedback to the editors.
Your feedback is important to us. However, we do not guarantee individual replies due to the high volume of messages.
E-mail the story
Your email address is used only to let the recipient know who sent the email. Neither your address nor the recipient's address will be used for any other purpose. The information you enter will appear in your e-mail message and is not retained by Phys.org in any form.
Newsletter sign up
Get weekly and/or daily updates delivered to your inbox. You can unsubscribe at any time and we'll never share your details to third parties.
More information Privacy policy
Donate and enjoy an ad-free experience
We keep our content available to everyone. Consider supporting Science X's mission by getting a premium account.
E-mail newsletter
ORIGINAL RESEARCH article
Long term organic farming impact on soil nutrient status and grain yield at foothill of himalayas provisionally accepted.
- 1 Indian Institute of Technology Madras, India
- 2 Vidyadayini Institute of Science, Management and Technology, India
- 3 ICAR-Research Complex for Eastern Region, India
- 4 G. B. Pant University of Agriculture and Technology, India
- 5 G. L. A. University, India
The final, formatted version of the article will be published soon.
This study aimed to document the effects of long-term organic farming (OF) impact on the soil quality, agronomical parameters, crop productivity and food grain yield compared to conventional farming system (CF). The crop in this study was chickpea (Cicer arietinum) and the field was located at Pantnagar, India in the foothills of Himalayas. Organic farming approach involved utilizing a blend of farmyard manure and vermicompost, combined with a biopesticide comprising neem oil and cow urine. Chickpea grain micronutrient analysis was done using Atomic Absorption Spectrophotometer. It was found that the soil physico-chemical properties of the organic plot were improved over the conventional partner. At the postharvesting stage, organically managed field had higher soil organic carbon than conventional field (OF-0.93± 0.05%, CF-0.75± 0.12%), higher available nitrogen (OF-317± 11 kg/ha, CF-240± 22 kg/ha) as well as more available phosphorus (OF-37.4± 1.3kg/ha, CF-25.2± 2.5 kg/ha).The agronomical parameters of chickpea crop were better under organic cultivation with significantly high nodule number, nodule dry weight and grains per pod. Hence the grain yield of the crop was better under organic cultivation with the yield of 1048 kg ha -1 whereas it was 896.5kg ha -1 for conventional plot. The Fe and Zn content of organically produced chickpea grains were almost double of their conventional counterpart. Therefore, organic cultivation led to better soil fertility, chickpea grains yield, and nutrient status of the crop. It will be beneficial for the nutritious and sustainable production of chickpea in Himalayan regions.
Keywords: Organic farming, Soil derived nutrient, chickpea, micronutrient, Available nitrogen
Received: 30 Jan 2024; Accepted: 15 May 2024.
Copyright: © 2024 Singh, SUYAL, Kumar, Singh and Goel. This is an open-access article distributed under the terms of the Creative Commons Attribution License (CC BY) . The use, distribution or reproduction in other forums is permitted, provided the original author(s) or licensor are credited and that the original publication in this journal is cited, in accordance with accepted academic practice. No use, distribution or reproduction is permitted which does not comply with these terms.
* Correspondence: Dr. DEEP C. SUYAL, Vidyadayini Institute of Science, Management and Technology, Bhopal, India Dr. Reeta Goel, G. L. A. University, Mathura, India
People also looked at

An official website of the United States government
The .gov means it’s official. Federal government websites often end in .gov or .mil. Before sharing sensitive information, make sure you’re on a federal government site.
The site is secure. The https:// ensures that you are connecting to the official website and that any information you provide is encrypted and transmitted securely.
- Publications
- Account settings
Preview improvements coming to the PMC website in October 2024. Learn More or Try it out now .
- Advanced Search
- Journal List
- J Family Med Prim Care
- v.4(3); Jul-Sep 2015
Validity, reliability, and generalizability in qualitative research
Lawrence leung.
1 Department of Family Medicine, Queen's University, Kingston, Ontario, Canada
2 Centre of Studies in Primary Care, Queen's University, Kingston, Ontario, Canada
In general practice, qualitative research contributes as significantly as quantitative research, in particular regarding psycho-social aspects of patient-care, health services provision, policy setting, and health administrations. In contrast to quantitative research, qualitative research as a whole has been constantly critiqued, if not disparaged, by the lack of consensus for assessing its quality and robustness. This article illustrates with five published studies how qualitative research can impact and reshape the discipline of primary care, spiraling out from clinic-based health screening to community-based disease monitoring, evaluation of out-of-hours triage services to provincial psychiatric care pathways model and finally, national legislation of core measures for children's healthcare insurance. Fundamental concepts of validity, reliability, and generalizability as applicable to qualitative research are then addressed with an update on the current views and controversies.
Nature of Qualitative Research versus Quantitative Research
The essence of qualitative research is to make sense of and recognize patterns among words in order to build up a meaningful picture without compromising its richness and dimensionality. Like quantitative research, the qualitative research aims to seek answers for questions of “how, where, when who and why” with a perspective to build a theory or refute an existing theory. Unlike quantitative research which deals primarily with numerical data and their statistical interpretations under a reductionist, logical and strictly objective paradigm, qualitative research handles nonnumerical information and their phenomenological interpretation, which inextricably tie in with human senses and subjectivity. While human emotions and perspectives from both subjects and researchers are considered undesirable biases confounding results in quantitative research, the same elements are considered essential and inevitable, if not treasurable, in qualitative research as they invariable add extra dimensions and colors to enrich the corpus of findings. However, the issue of subjectivity and contextual ramifications has fueled incessant controversies regarding yardsticks for quality and trustworthiness of qualitative research results for healthcare.
Impact of Qualitative Research upon Primary Care
In many ways, qualitative research contributes significantly, if not more so than quantitative research, to the field of primary care at various levels. Five qualitative studies are chosen to illustrate how various methodologies of qualitative research helped in advancing primary healthcare, from novel monitoring of chronic obstructive pulmonary disease (COPD) via mobile-health technology,[ 1 ] informed decision for colorectal cancer screening,[ 2 ] triaging out-of-hours GP services,[ 3 ] evaluating care pathways for community psychiatry[ 4 ] and finally prioritization of healthcare initiatives for legislation purposes at national levels.[ 5 ] With the recent advances of information technology and mobile connecting device, self-monitoring and management of chronic diseases via tele-health technology may seem beneficial to both the patient and healthcare provider. Recruiting COPD patients who were given tele-health devices that monitored lung functions, Williams et al. [ 1 ] conducted phone interviews and analyzed their transcripts via a grounded theory approach, identified themes which enabled them to conclude that such mobile-health setup and application helped to engage patients with better adherence to treatment and overall improvement in mood. Such positive findings were in contrast to previous studies, which opined that elderly patients were often challenged by operating computer tablets,[ 6 ] or, conversing with the tele-health software.[ 7 ] To explore the content of recommendations for colorectal cancer screening given out by family physicians, Wackerbarth, et al. [ 2 ] conducted semi-structure interviews with subsequent content analysis and found that most physicians delivered information to enrich patient knowledge with little regard to patients’ true understanding, ideas, and preferences in the matter. These findings suggested room for improvement for family physicians to better engage their patients in recommending preventative care. Faced with various models of out-of-hours triage services for GP consultations, Egbunike et al. [ 3 ] conducted thematic analysis on semi-structured telephone interviews with patients and doctors in various urban, rural and mixed settings. They found that the efficiency of triage services remained a prime concern from both users and providers, among issues of access to doctors and unfulfilled/mismatched expectations from users, which could arouse dissatisfaction and legal implications. In UK, a care pathways model for community psychiatry had been introduced but its benefits were unclear. Khandaker et al. [ 4 ] hence conducted a qualitative study using semi-structure interviews with medical staff and other stakeholders; adopting a grounded-theory approach, major themes emerged which included improved equality of access, more focused logistics, increased work throughput and better accountability for community psychiatry provided under the care pathway model. Finally, at the US national level, Mangione-Smith et al. [ 5 ] employed a modified Delphi method to gather consensus from a panel of nominators which were recognized experts and stakeholders in their disciplines, and identified a core set of quality measures for children's healthcare under the Medicaid and Children's Health Insurance Program. These core measures were made transparent for public opinion and later passed on for full legislation, hence illustrating the impact of qualitative research upon social welfare and policy improvement.
Overall Criteria for Quality in Qualitative Research
Given the diverse genera and forms of qualitative research, there is no consensus for assessing any piece of qualitative research work. Various approaches have been suggested, the two leading schools of thoughts being the school of Dixon-Woods et al. [ 8 ] which emphasizes on methodology, and that of Lincoln et al. [ 9 ] which stresses the rigor of interpretation of results. By identifying commonalities of qualitative research, Dixon-Woods produced a checklist of questions for assessing clarity and appropriateness of the research question; the description and appropriateness for sampling, data collection and data analysis; levels of support and evidence for claims; coherence between data, interpretation and conclusions, and finally level of contribution of the paper. These criteria foster the 10 questions for the Critical Appraisal Skills Program checklist for qualitative studies.[ 10 ] However, these methodology-weighted criteria may not do justice to qualitative studies that differ in epistemological and philosophical paradigms,[ 11 , 12 ] one classic example will be positivistic versus interpretivistic.[ 13 ] Equally, without a robust methodological layout, rigorous interpretation of results advocated by Lincoln et al. [ 9 ] will not be good either. Meyrick[ 14 ] argued from a different angle and proposed fulfillment of the dual core criteria of “transparency” and “systematicity” for good quality qualitative research. In brief, every step of the research logistics (from theory formation, design of study, sampling, data acquisition and analysis to results and conclusions) has to be validated if it is transparent or systematic enough. In this manner, both the research process and results can be assured of high rigor and robustness.[ 14 ] Finally, Kitto et al. [ 15 ] epitomized six criteria for assessing overall quality of qualitative research: (i) Clarification and justification, (ii) procedural rigor, (iii) sample representativeness, (iv) interpretative rigor, (v) reflexive and evaluative rigor and (vi) transferability/generalizability, which also double as evaluative landmarks for manuscript review to the Medical Journal of Australia. Same for quantitative research, quality for qualitative research can be assessed in terms of validity, reliability, and generalizability.
Validity in qualitative research means “appropriateness” of the tools, processes, and data. Whether the research question is valid for the desired outcome, the choice of methodology is appropriate for answering the research question, the design is valid for the methodology, the sampling and data analysis is appropriate, and finally the results and conclusions are valid for the sample and context. In assessing validity of qualitative research, the challenge can start from the ontology and epistemology of the issue being studied, e.g. the concept of “individual” is seen differently between humanistic and positive psychologists due to differing philosophical perspectives:[ 16 ] Where humanistic psychologists believe “individual” is a product of existential awareness and social interaction, positive psychologists think the “individual” exists side-by-side with formation of any human being. Set off in different pathways, qualitative research regarding the individual's wellbeing will be concluded with varying validity. Choice of methodology must enable detection of findings/phenomena in the appropriate context for it to be valid, with due regard to culturally and contextually variable. For sampling, procedures and methods must be appropriate for the research paradigm and be distinctive between systematic,[ 17 ] purposeful[ 18 ] or theoretical (adaptive) sampling[ 19 , 20 ] where the systematic sampling has no a priori theory, purposeful sampling often has a certain aim or framework and theoretical sampling is molded by the ongoing process of data collection and theory in evolution. For data extraction and analysis, several methods were adopted to enhance validity, including 1 st tier triangulation (of researchers) and 2 nd tier triangulation (of resources and theories),[ 17 , 21 ] well-documented audit trail of materials and processes,[ 22 , 23 , 24 ] multidimensional analysis as concept- or case-orientated[ 25 , 26 ] and respondent verification.[ 21 , 27 ]
Reliability
In quantitative research, reliability refers to exact replicability of the processes and the results. In qualitative research with diverse paradigms, such definition of reliability is challenging and epistemologically counter-intuitive. Hence, the essence of reliability for qualitative research lies with consistency.[ 24 , 28 ] A margin of variability for results is tolerated in qualitative research provided the methodology and epistemological logistics consistently yield data that are ontologically similar but may differ in richness and ambience within similar dimensions. Silverman[ 29 ] proposed five approaches in enhancing the reliability of process and results: Refutational analysis, constant data comparison, comprehensive data use, inclusive of the deviant case and use of tables. As data were extracted from the original sources, researchers must verify their accuracy in terms of form and context with constant comparison,[ 27 ] either alone or with peers (a form of triangulation).[ 30 ] The scope and analysis of data included should be as comprehensive and inclusive with reference to quantitative aspects if possible.[ 30 ] Adopting the Popperian dictum of falsifiability as essence of truth and science, attempted to refute the qualitative data and analytes should be performed to assess reliability.[ 31 ]
Generalizability
Most qualitative research studies, if not all, are meant to study a specific issue or phenomenon in a certain population or ethnic group, of a focused locality in a particular context, hence generalizability of qualitative research findings is usually not an expected attribute. However, with rising trend of knowledge synthesis from qualitative research via meta-synthesis, meta-narrative or meta-ethnography, evaluation of generalizability becomes pertinent. A pragmatic approach to assessing generalizability for qualitative studies is to adopt same criteria for validity: That is, use of systematic sampling, triangulation and constant comparison, proper audit and documentation, and multi-dimensional theory.[ 17 ] However, some researchers espouse the approach of analytical generalization[ 32 ] where one judges the extent to which the findings in one study can be generalized to another under similar theoretical, and the proximal similarity model, where generalizability of one study to another is judged by similarities between the time, place, people and other social contexts.[ 33 ] Thus said, Zimmer[ 34 ] questioned the suitability of meta-synthesis in view of the basic tenets of grounded theory,[ 35 ] phenomenology[ 36 ] and ethnography.[ 37 ] He concluded that any valid meta-synthesis must retain the other two goals of theory development and higher-level abstraction while in search of generalizability, and must be executed as a third level interpretation using Gadamer's concepts of the hermeneutic circle,[ 38 , 39 ] dialogic process[ 38 ] and fusion of horizons.[ 39 ] Finally, Toye et al. [ 40 ] reported the practicality of using “conceptual clarity” and “interpretative rigor” as intuitive criteria for assessing quality in meta-ethnography, which somehow echoed Rolfe's controversial aesthetic theory of research reports.[ 41 ]
Food for Thought
Despite various measures to enhance or ensure quality of qualitative studies, some researchers opined from a purist ontological and epistemological angle that qualitative research is not a unified, but ipso facto diverse field,[ 8 ] hence any attempt to synthesize or appraise different studies under one system is impossible and conceptually wrong. Barbour argued from a philosophical angle that these special measures or “technical fixes” (like purposive sampling, multiple-coding, triangulation, and respondent validation) can never confer the rigor as conceived.[ 11 ] In extremis, Rolfe et al. opined from the field of nursing research, that any set of formal criteria used to judge the quality of qualitative research are futile and without validity, and suggested that any qualitative report should be judged by the form it is written (aesthetic) and not by the contents (epistemic).[ 41 ] Rolfe's novel view is rebutted by Porter,[ 42 ] who argued via logical premises that two of Rolfe's fundamental statements were flawed: (i) “The content of research report is determined by their forms” may not be a fact, and (ii) that research appraisal being “subject to individual judgment based on insight and experience” will mean those without sufficient experience of performing research will be unable to judge adequately – hence an elitist's principle. From a realism standpoint, Porter then proposes multiple and open approaches for validity in qualitative research that incorporate parallel perspectives[ 43 , 44 ] and diversification of meanings.[ 44 ] Any work of qualitative research, when read by the readers, is always a two-way interactive process, such that validity and quality has to be judged by the receiving end too and not by the researcher end alone.
In summary, the three gold criteria of validity, reliability and generalizability apply in principle to assess quality for both quantitative and qualitative research, what differs will be the nature and type of processes that ontologically and epistemologically distinguish between the two.
Source of Support: Nil.
Conflict of Interest: None declared.
- Open access
- Published: 14 May 2024
Overexpression of wheat spermidine synthase gene enhances wheat resistance to Fusarium head blight
- Jingyi Ren 1 ,
- Chengliang Li 1 ,
- Ming Xu 1 &
- Huiquan Liu ORCID: orcid.org/0000-0002-4723-845X 1
Phytopathology Research volume 6 , Article number: 24 ( 2024 ) Cite this article
2 Altmetric
Metrics details
Polyamines, such as putrescine, spermidine, and spermine, are crucial for plant defense against both abiotic and biotic stresses. Putrescine is also known as a significant inducer of deoxynivalenol (DON) production in Fusarium graminearum , the primary causal agent of Fusarium head blight (FHB). However, the impact of other polyamines on DON production and whether modifying polyamine biosynthesis could improve wheat resistance to FHB are currently unknown. In this study, we demonstrate that key precursor components of putrescine synthesis, including arginine, ornithine, and agmatine, can induce DON production, albeit to a lesser extent than putrescine in trichothecene biosynthesis-inducing (TBI) culture under the same total nitrogen conditions. Intriguingly, spermidine and spermine, downstream products of putrescine in the polyamine biosynthesis pathway, do not induce DON production under the same conditions. Additionally, externally applying either spermidine or spermine to wheat heads significantly reduces the diseased spikelet number caused by F. graminearum . Furthermore, our results show that overexpression of the wheat spermidine synthase (SPDS) gene TaSPDS -7D1 significantly enhances the spermidine content and wheat resistance to FHB. In addition, the TaSPDS -7D1-overexpressing line OE3 exhibited a 1000-grain weight and plant height increase compared to the wild type. Our findings reveal that overexpression of the spermidine synthase gene can enhance wheat resistance to FHB without compromising wheat yield.
Polyamines are small organic amine molecules found in almost all living organisms. The primary polyamine species consist of putrescine, spermidine, and spermine, with the latter two also referred to as higher polyamines (Pal and Janda 2017 ; Gerlin et al. 2021 ). The biosynthesis pathway of polyamines has been well documented, involving the participation of several key enzymes. Briefly, putrescine is synthesized through the decarboxylation of ornithine, catalyzed by the enzyme ornithine decarboxylase (ODC), or indirectly synthesized by the decarboxylation of arginine via agmatine, catalyzed by the enzyme arginine decarboxylase (ADC). Spermidine and spermine are synthesized by sequentially adding aminopropyl moieties to the putrescine skeleton through enzymatic reactions, which are catalyzed by spermidine synthase (SPDS) and spermine synthase (SPMS), respectively. Decarboxylated S-adenosylmethionine (dcSAM), which is used for the addition of the aminopropyl moiety, is synthesized by S-adenosylmethionine decarboxylase (SAMDC) (Michael 2016 ; Pal and Janda 2017 ).
Due to their amine functions, polyamines possess a polycationic nature at physiological pH. Thus, they can stabilize or destabilize anionic macromolecules or negatively charged ions. The chemical property of polyamines as pleiotropic molecules allows them to contribute to a wide range of molecular and biochemical processes. In plants, polyamines contribute to diverse pathways involved in cell proliferation, plant development, abiotic and biotic stress responses (Moschou et al. 2012 ; Gerlin et al. 2021 ). In Arabidopsis ( Arabidopsis thaliana ), SPDS genes are required for spermidine biosynthesis and essential for plant survival as the spds1-1 spds2-1 double mutant are embryo lethal (Imai et al. 2004 ). In tobacco, silencing of spermidine synthase gene SPDS resulted in decreased spermidine levels and a slightly increased putrescine, leading to smaller size of flowers, decreased pollen viability, and a reduced and delayed seed germination, but the RNAi transgenic plant showed increased tolerance to salinity and drought conditions (Choubey and Rajam 2018 ). Several studies have highlighted the role of polyamines in plant immune responses to pathogens. In Arabidopsis, the expression of ADC2 , a key enzyme in putrescine biosynthesis, was induced by Pseudomonas syringae pv. tomato DC3000 ( Pst DC3000) infection. The adc2 knock-out mutant was defective in putrescine biosynthesis and was more susceptible to pathogen infection (Kim et al. 2013 ). Overexpression of the Arabidopsis spermine synthase gene AtSPMS led to enhanced spermine levels and exhibited increased resistance to Pseudomonas viridiflava as compared with the wild-type plant (Gonzalez et al. 2011 ). Recent transcriptomics and metabolomics analyses of the interaction between Aspergillus flavus and both resistant/susceptible maize lines revealed resistant lines accumulated higher amounts of spermidine and spermine compared to the susceptible line at the earliest time after inoculation, indicating that higher polyamine content in maize genotypes may confer higher resistance to A. flavus and reduce aflatoxin production (Majumdar et al. 2019 ).
Fusarium head blight (FHB), caused by the Fusarium graminearum species complex, is a destructive fungal disease that affects wheat and barley crops globally. FHB not only causes substantial yield losses in crops but also leads to grain contamination with various mycotoxins, particularly deoxynivalenol (DON) (Xia et al. 2020 ). In mammals, DON exerts its effects by binding to the ribosome, leading to the inhibition of protein synthesis, then causing emetic effects, anorexia, immune dysregulation, as well as growth and reproductive inhibition, and teratogenic effects (Pestka 2010 ). To minimize human and animal exposure to DON, maximum permissible levels for DON in cereals and their products have been established in many countries (Ji et al. 2014 ; Bianchini et al. 2015 ). Besides, DON is also an important virulence factor for F. graminearum . The TRI5 gene, which is essential for DON biosynthesis, is expressed in infection cushions during the early stages of infection (Boenisch and Schäfer 2011 ). Deletion of the TRI5 gene abolishes DON production. Although the tri5 mutant can cause the initial infection, it is trapped in the infected floret and fails to spread via the rachis node (Jansen et al. 2005 ).
Several factors that may induce the production of DON by F. graminearum have been proposed, including hydrogen peroxide, carbon and nitrogen sources, and acidic pH (Chen et al. 2019 ). In particular, in a screen of large-scale nutrient profiling, the most potent inducers of DON production in vitro by F. graminearum are revealed as metabolites of the plant polyamine biosynthetic pathway, such as arginine, ornithine, agmatine, citrulline, and putrescine (Gardiner et al. 2009 ). The core polyamine biosynthetic pathway is activated at the early stage during F. graminearum infection on wheat and putrescine accumulation occurs before toxin production by the pathogen (Gardiner et al. 2010 ). It is hypothesized that the pathogen may absorb putrescine and related amino acids as signals for DON production during infection (Gardiner et al. 2009 , 2010 ). In the presence of putrescine, the transcription factor FgAreA facilitates the enrichment of histone H2B monoubiquitination (H2B ub1) and histone 3 lysine 4 di- and trimethylations (H3K4 me2/3) on trichothecene biosynthesis genes to induce their expression (Ma et al. 2021 ). However, whether manipulation of wheat polyamine biosynthetic pathways to increase the conversion of putrescine to higher polyamines could reduce DON induction and enhance wheat resistance against FHB is largely unknown.
In this study, we showed that, in addition to putrescine, arginine, ornithine, and agmatine, the primary precursor components of putrescine synthesis, can induce DON production. Conversely, spermidine and spermine, which are downstream products of putrescine in the polyamine biosynthesis pathway, do not induce DON production. Notably, the external application of both spermidine and spermine to wheat heads significantly reduces the diseased spikelet number caused by F. graminearum . We performed overexpression of the spermidine synthase (SPDS) gene TaSPDS -7D1 in wheat to reduce DON production and enhance wheat resistance to FHB by decreasing the content of putrescine and increasing the content of spermidine and spermine. The TaSPDS -7D1-overexpressing wheat lines exhibited significantly enhanced resistance to FHB without compromising wheat yield. However, the same level of DON was accumulated in the inoculated spikelet of TaSPDS -7D1-overexpressing wheat lines as in the wild type. Analysis of polyamine contents revealed a simultaneous enhancement of putrescine and spermidine in the wheat heads of TaSPDS -7D1-overexpressing lines infected by F. graminearum . Our findings demonstrate that overexpression of the spermidine synthase gene can indeed enhance wheat resistance to FHB.
Putrescine and its precursors stimulate DON production, while spermidine and spermine inhibit F. graminearum infection
We investigated the impact of polyamine synthesis pathway components (Fig. 1 a) – including arginine, ornithine, agmatine, putrescine, spermidine, and spermine – on DON production using trichothecene biosynthesis-inducing (TBI) medium, with each serving as the sole nitrogen source while maintaining a consistent total nitrogen level (Fig. 1 b). As depicted in Fig. 1 b, putrescine's synthesis precursors (arginine, ornithine, and agmatine) significantly stimulated DON production. Conversely, putrescine's downstream products (spermidine and spermine) did not induce DON production. The precursor components induced a relatively lower level of DON production compared to putrescine. Notably, the concentration of DON induced by putrescine in the TBI medium exceeded 10,000 μg/g dry-weight tissue (Fig. 1 b). These findings indicate that putrescine is the most effective polyamine for inducing DON production.
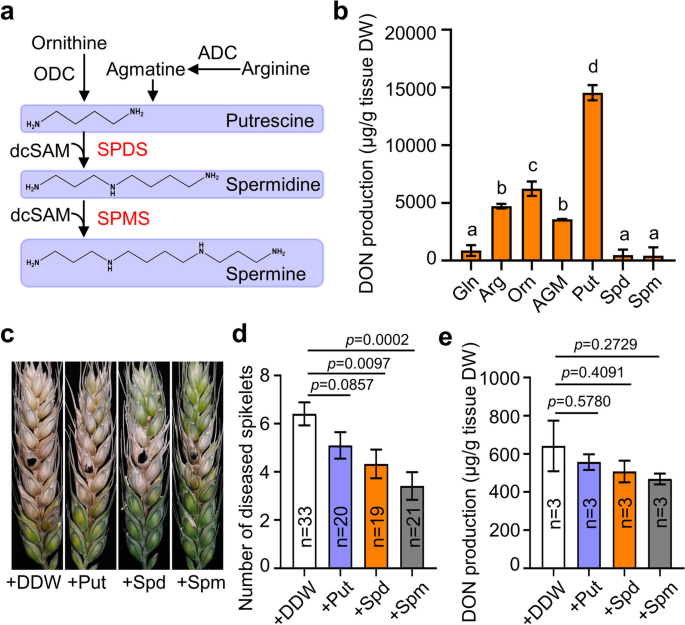
Functional analysis of polyamines in F. graminearum infection and Deoxynivalenol (DON) production on wheat. a Schematic diagram of putrescine, spermidine, and spermine biosynthesis in plants. SPDS and SPMS are responsible for the transfer of aminopropyl groups to spermidine and spermine, respectively. ADC, arginine decarboxylase; SPDS, spermidine synthase; SPMS, spermine synthase; dc-SAM, decarboxylated S-adenosylmethionine; ADC, ornithine decarboxylase. b DON production in trichothecene biosynthesis-inducing (TBI) medium with glutamine (Gln), arginine (Arg), ornithine (Orn), agmatine (AGM), putrescine (Put), spermidine (Spd), and spermine (Spm) as the sole nitrogen source. DW, Dry weight. The error bar represents standard errors (SEs) from three independent replicates ( n = 3). Different letters indicate significant differences based on ANOVA analysis followed by Duncan’s multiple range test ( P = 0.05). c Representative images of polyamine-treated wheat head with F. graminearum inoculation. d Diseased spikelet number of polyamine-treated and F . graminearum infected wheat. e DON levels of polyamine-treated and F . graminearum infected wheat. The error bar indicates standard errors of the means (n varies for each column and is shown in each case directly on the graphs). P -values are from the Student’s t -tests
We further assayed the role of putrescine, spermidine, and spermine in F. graminearum infection by spraying them on wheat heads along with F. graminearum inoculation. As shown in Fig. 1 c, d, the exogenous spray of either spermidine or spermine notably decreased the diseased spikelet number, while putrescine did not. The result indicates that spermidine and spermine, but not putrescine, enhance FHB resistance in wheat. However, exogenous addition of putrescine, spermidine, and spermine had no effect on DON production (Fig. 1 e).
Identification and expression analysis of wheat SPDS genes
Given that SPDS and SPMS facilitate the conversion of putrescine to spermidine and spermine (Fig. 1 a), it may be feasible to overexpress the wheat SPDS/SPMS genes to reduce putrescine levels while increasing spermidine and spermine levels. The SPDS/SPMS enzyme possesses a Spermine_synth domain. Genes encoding SPDS/SPMS in Arabidopsis ( A. thaliana ) (Hanzawa et al. 2002 ), rice ( Oryza sativa ) (Tao et al. 2018 ), tomato ( Solanum lycopersicum ) (Upadhyay et al. 2021 ), maize ( Zea mays ) ( https://plants.ensembl.org/ ), tobacco ( Nicotiana benthamiana ) (Choubey and Rajam 2018 ), figleaf gourd ( Cucurbita ficifolia ) (Kasukabe et al. 2004 ), pepper ( Capsicum annuum ) (Zhang et al. 2023 ), and datura ( Datura stramonium ) (Franceschetti et al. 2004 ) were used as a query against wheat ( Triticum. aestivum ) genome (Additional file 1 : Table S1). In the wheat genome, we obtained six sequences related to TaSPDS and six related to TaSPMS. Phylogenetic analysis revealed that a gene duplication event in the wheat ancestor resulted in the emergence of two SPDS paralogs (each occurring in the three subgenomes), while the six TaSPMS sequences originated from an ancestral duplication event, at least in the last common ancestor of the grass family (Fig. 2 a). Given that overexpression of OsSPMS1 in rice resulted in no significant difference in spermine content between the wild-type and overexpressing plants (Tao et al. 2018 ), we focused on investigating the roles of TaSPDS in wheat. We named TraesCS7A02G265200, TraesCS7B02G163300, and TraesCS7D02G265900 as TaSPDS -7A1, TaSPDS -7B1, and TaSPDS -7D1, respectively. Additionally, TraesCS7A02G265300, TraesCS7B02G163500, and TraesCS7D02G266000 were named TaSPDS -7A2, TaSPDS -7B2, and TaSPDS -7D2, respectively (Additional file 2 : Fig. S1).
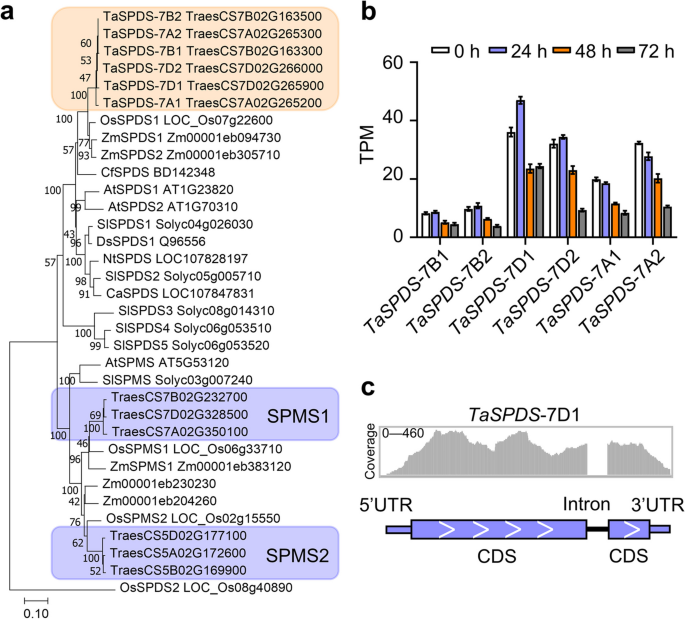
Phylogenetic analysis of SPDS/SPMS members in wheat and gene expression pattern of TaSPDS during F. graminearum infection. a Phylogenetic tree of SPDS/SPMS proteins from wheat ( Triticum aestivum ), Arabidopsis ( Arabidopsis thaliana ), rice ( Oryza sativa ) , tomato ( Solanum lycopersicum ), maize ( Zea mays ), tobacco ( Nicotiana benthamiana ), figleaf gourd ( Cucurbita ficifolia ), pepper ( Capsicum annuum ), and datura ( Datura stramonium ). The phylogenetic tree was constructed using the neighbor-joining method with 1000 bootstrap repetitions with the MEGA 11.0.9 software. b The TPM (transcripts per million) values of 6 TaSPDS genes from RNA-seq data of F. graminearum inoculated wheat. c Gene structure, RNA-seq read coverage of TaSPDS- 7D1
Based on our previous RNA-seq data of the wheat- F. graminearum interaction (Jiang et al. 2019 ), the transcriptional level of TaSPDS -7D1 was slightly elevated at 24 h post inoculation (hpi), followed by a reduction at 48 hpi and 72 hpi (Fig. 2 b). Given that TaSPDS -7D1 exhibited the highest expression level in wheat both before and after F. graminearum infection, it was chosen for further functional analysis in this study. TaSPDS -7D1 consists of two exons and encodes 324 amino acids. The genomic and cDNA sequences are 1067 and 975 bp in length, respectively (Fig. 2 c).
Overexpression of TaSPDS- 7D1 in wheat confers resistance to FHB
The overexpression (OE) construct of TaSPDS- 7D1 was generated under the control of the maize ubiquitin promoter, which was then transformed into the spring wheat cultivar Fielder. After conducting a PCR test with primers 6E-check-F/6E-check-R for the presence of the transgene, 20 positive transgene lines of the first generation (T 0 ) were identified. T 1 generation of four transgenic lines (OE1, OE3, OE15, and OE21) were chosen for further analysis. According to the segregation analysis based on PCR with primers 6E-check-F/6E-check-R in all the plants investigated, lines OE3 and OE21 were identified as likely homozygous, while lines OE1 and OE15 were verified to be heterozygous (Table 1 ). We selected the T 3 generation of OE3 and OE21 for further analysis. PCR analysis revealed the presence of a band of the expected size in both transgenic lines (Fig. 3 a), indicating the successful insertion of the TaSPDS- 7D1-overexpressing construct into the wheat genome. The relative expression level of TaSPDS- 7D1 was significantly increased in OE3 and OE21 lines as compared to the wild-type plant, indicating a stable overexpression of TaSPDS- 7D1 in the transgenic lines. The means of TaSPDS- 7D1 relative expression levels were 17.84 in OE3 and 9.81 in OE21 as compared with the wild type (Fig. 3 b), respectively. Western blotting analysis showed that the TaSPDS-7D1-HA fusion proteins were accumulated in the OE3 and OE21 lines (Fig. 3 c).
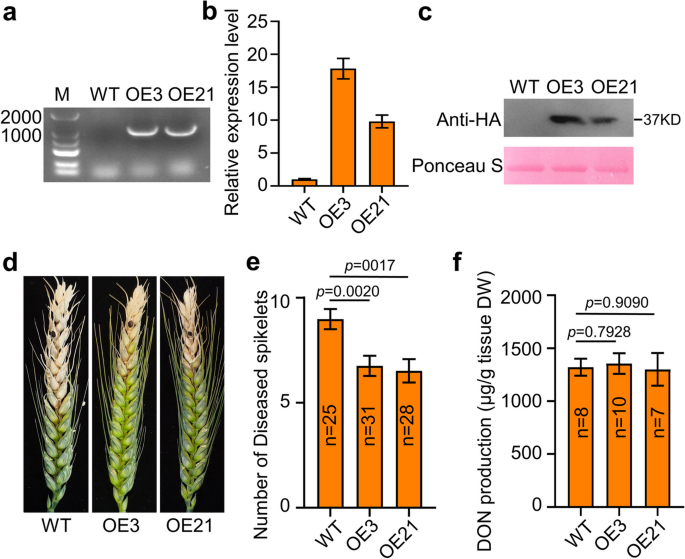
Overexpression of TaSPDS- 7D1 in wheat confers resistance to FHB. a PCR detection of the wild type (WT) and TaSPDS -7D1-overexpressing lines (OE3 and OE21). M, DL2000 DNA marker. b Relative expression levels of TaSPDS- 7D1 in WT and TaSPDS -7D1-overexpressing lines. The error bar indicates standard deviation (SD) from three independent replicates ( n = 3). c Western blot of the WT and TaSPDS -7D1-overexpressing lines using an anti-HA antibody. d Representative images of the WT and TaSPDS- 7D1-overexpressing lines with F. graminearum inoculation. e Diseased spikelet number of the WT and TaSPDS- 7D1-overexpressing lines with F. graminearum inoculation. f DON levels of WT and TaSPDS- 7D1-overexpressing lines. The error bar indicates standard errors of the means (n varies for each column and is shown in each case directly on the graphs). P -values are from the Student’s t -tests
To investigate the potential functions of TaSPDS- 7D1 in wheat defense against F. graminearum , OE3, OE21, and wild-type plants were inoculated with the F. graminearum wild-type strain PH-1. The two TaSPDS- 7D1-overexpressing wheat lines, OE3 and OE21, displayed significantly enhanced resistance to FHB (Fig. 3 d). The average diseased spikelet number of the wild-type was 9.0, while those of the two transgenic lines were 6.8 and 6.5 in OE3 and OE21, respectively (Fig. 3 e). But compared with the wild-type wheat, the two TaSPDS- 7D1-overexpressing lines produced the same level of DON in the inoculated spikelet (Fig. 3 f). These results indicate that overexpression of TaSPDS- 7D1 improved wheat resistance to FHB, but had limited effect on reducing DON accumulation.
Overexpression of TaSPDS -7D1 do not compromise wheat yield
Several agronomic traits were compared between the wild-type plants and OE lines. At the maturity stage, the two OE lines were significantly taller than the wild type (Fig. 4 a, d). Compared with the wild type, the plant height of the OE lines increased by 7.7% and 10.8% in OE3 and OE21, respectively. Whereas there was no significant difference in tiller number (Fig. 4 c) and spike length (Fig. 4 e), the 1000-grain weight increased by 10.2% in the OE3 line compared with the wild type (Fig. 4 b, f). The difference between the two TaSPDS- 7D1-overexpressing lines may be attributed to variations in the expression levels since OE3 exhibited a 1.8-fold higher than that of OE21.
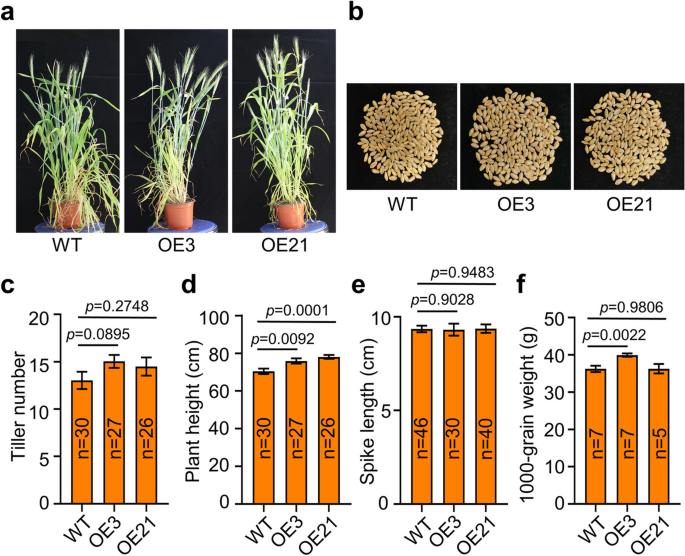
Overexpression of TaSPDS- 7D1 affects plant architecture and 1000-grain weight. a Plant architecture of WT and TaSPDS- 7D1-overexpressing lines at the mature stage. b Grain shape of WT and TaSPDS- 7D1-overexpressing lines. c Tiller number, d Plant height, e Spike length, f 1000-grain weight of the WT and TaSPDS- 7D1-overexpressing lines. The error bar indicates standard errors of the means (n varies for each column and is shown in each case directly on the graphs). P -values are from the Student’s t -tests
Overexpression of TaSPDS -7D1 leads to increased synthesis of both spermidine and putrescine
To investigate the resistance mechanism, we assayed the level of spermidine, spermine, and putrescine in the OE3 transgenic line in the spikelet and rachis tissues inoculated with F. graminearum . As expected, overexpression of TaSPDS- 7D1 significantly enhanced the spermidine content in both spikelets and rachises as compared to the wild-type plants (Fig. 5 a). The increased level was even higher after F. graminearum infection. Spermidine content in the rachis of OE line was increased more than twofold as compared with that of the wild-type plant (Fig. 5 a). These results suggest that TaSPDS-7D1 functions as a SPDS and promotes the accumulation of spermidine in wheat heads that inhibit F. graminearum infection.
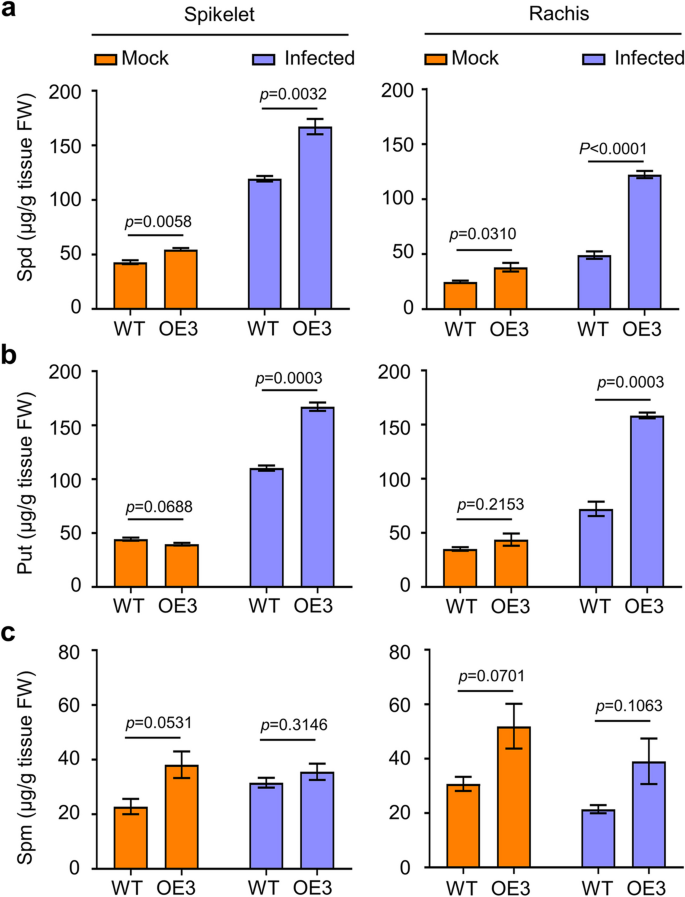
Overexpression of TaSPDS- 7D1 affects polyamine synthesis. Contents of a spermidine, b putrescine, and c spermine in the WT and TaSPDS- 7D1-overexpressing lines with (Infect) or without (Mock) F. graminearum inoculation. FW, Fresh weight. The error bar indicates standard errors from three independent experiments ( n = 3). P -values are from the Student’s t -tests
While the putrescine content showed a significant increase in both the spikelets and rachises of the OE3 line after F. graminearum infection compared to the wild type (Fig. 5 b), there were no significant changes in the spermine content (Fig. 5 c). These findings suggest that the polyamine biosynthesis pathway underwent positive feedback, and the increased levels of spermidine led to an increase in the levels of its synthetic precursor, putrescine. These observations help explain why the overexpression of TaSPDS -7D1 enhances resistance to FHB but has a limited effect on reducing DON production.
The host stress metabolite putrescine is one of the most potent DON-inducing factors in culture. Compared to other metabolites in the polyamine biosynthesis pathway, putrescine induces the highest DON level in TBI culture while maintaining a consistent total nitrogen level. Exogenously sprayed both spermidine and spermine on wheat head along with F. graminearum inoculation significantly reduced the diseased spikelet number, indicating that spermidine and spermine positively contribute to FHB resistance of wheat. According to phylogenetic analyses, wheat TaSPDS genes had been identified and named. Expression pattern analysis of TaSPDS genes using RNA-seq data showed that the transcript level of TaSPDS- 7D1 was slightly elevated at 24 hpi, and then reduced at 48 hpi and 72 hpi. We investigated the impact of overexpression of TaSPDS- 7D1 on FHB resistance in wheat and regulation of the abundance of polyamines. Our results showed that overexpression of TaSPDS- 7D1 significantly enhances wheat resistance to FHB, although TaSPDS -7D1-overexpressing wheat lines accumulate the same level of DON as the wild type. It may be because of a simultaneous increase of spermidine and putrescine in the wheat head after F. graminearum inoculation. Moreover, The TaSPDS -7D1-overexpressing line OE3 exhibited a 1000-grain weight and plant height increase compared to the wild type. These findings declare a positive effect of the spermidine synthase gene on enhance wheat resistance to FHB without compromising wheat yield.
During FHB development, the level of putrescine increases at the same time as DON production and with a significant increase in the transcription levels of wheat putrescine biosynthesis genes, suggesting that putrescine may play a role in promoting DON production (Gardiner et al. 2010 ; Ma et al. 2021 ). However, F. graminearum putrescine synthesis gene FgODC is not upregulated during infection on wheat, suggesting unessential role of endogenous putrescine synthesis on DON production during F. graminearum infection (Ma et al. 2021 ). In this study, while maintaining a consistent total nitrogen level, putrescine induced the higher DON level than its precursors in TBI culture. Exogenously application of putrescine had no effect on the diseased spikelet number. On the contrary, spermidine and spermine did not induce DON production, and exogenously application of these two higher polyamines on wheat head significantly reduced the diseased spikelet number, which gives support to the consequence that higher polyamines in the host contribute to FHB resistance.
It is well acknowledged that polyamines contribute to plant resistance against fungal pathogens by multiple functions (KoÇ 2015; Takahashi 2016 ; Gonzalez et al. 2021 ). Polyamines have a crucial impact on regulating the production of hydrogen peroxide (H 2 O 2 ), which is recognized as the primary reactive oxygen species (ROS) in plants. Polyamines participate in enhancing H 2 O 2 levels through their catabolism by amine oxidases (Gerlin et al. 2021 ). Generally, putrescine can be oxidized by diamine oxidases (DAO), spermidine and spermine can be oxidized by polyamine oxidases (PAO), these reactions release H 2 O 2 (Moschou et al. 2012 ; Gerlin et al. 2021 ). In A. thaliana , exogenously applied spermine induces the accumulation of H 2 O 2 , primming resistance against necrotrophic fungus Botrytis cinerea (Janse van Rensburg et al. 2021 ). The increase in H 2 O 2 may also be involved in signaling for phytoalexin production (Handa et al. 2018 ; Gonzalez et al. 2021 ), and in the initiation of the well-documented hypersensitive response (HR) (Zeiss et al. 2021 ). In rice, exogenously application of spermidine induces resistance marker genes OsPR1b and PBZ1 expression, as well as phytoalexin biosynthesis genes CSP4 and NOMT , leading to increased resistance against rice blast fungus Magnaporthe oryzae (Moselhy et al. 2016 ). In addition to their free forms, plant polyamines can also occur as conjugated forms. Conjugation of amines to hydroxycinnamic acids (HCAs) generates HCA amides (HCAAs), which are considered as part of the polyamine storage pool. HCAAs have been classified as phytoalexins and their accumulation in infected tissues served as biomarkers of pathogen infection (Zeiss et al. 2021 ).
Interestingly, the TaSPDS- 7D1 OE line OE3 exhibited an increase of 1000-grain weight and plant height compared to the wild-type, indicating a positive role of TaSPDS in the regulation of wheat growth. It was reported that exogenous spermidine can effectively regulate carbohydrate metabolism in cucumber leaves (Chen et al. 2011 ). Foliar spray treatment with putrescine and spermine on wheat increases the concentration of soluble, insoluble, and total sugars concentrations of leaves (Ebeed et al. 2017 ). Such an increase triggered a surge in the flow of sugars into the grains, leading to a significant increase in the grain weight (Kaur-Sawhney et al. 1982 ). The previous study has suggested that polyamines are notably involved in the grain filling of wheat (Liu et al. 2013 ). It is reported that a high spermidine level in grain is one of the reasons why superior grain has a higher grain filling rate than inferior grain (Yang et al. 2008 ). Luo and colleagues reported that exogenous spermidine significantly increased grain filling (Luo et al. 2019 ). Therefore, the increase in spermidine content may contribute to the enhanced 1000-grain weight of TaSPDS -7D1-overexpressing line OE3.
In this study, overexpression of TaSPDS- 7D1 significantly enhanced the spermidine content but did not affect putrescine and spermine content in the uninfected stage. After F. graminearum infection, TaSPDS- 7D1-overexpressing line showed a significant increase of spermidine and putrescine in the spikelets and rachises compared to the wild type. These findings suggest that the polyamine biosynthesis pathway undergoes positive feedback, and the increased levels of spermidine led to an increase in the levels of its synthetic precursor, putrescine. These observations help explain why the overexpression of TaSPDS -7D1 enhances resistance to FHB but has a limited effect on reducing DON production. Overexpression of SPDS genes from figleaf gourd ( Cucurbita ficifolia ), pepper ( Capsicum annuum ), and datura ( Datura stramonium ) in Arabidopsis or tobacco has also been conducted. Overexpression of figleaf gourd SPDS gene increased the spermidine content (1.3- to twofold), and slightly increased putrescine content (Kasukabe et al. 2004 ). The transgenic Arabidopsis showed enhanced tolerance to multiple stresses such as freezing, drought, and paraquat toxicity (Kasukabe et al. 2004 ). Overexpression of pepper ( C. annuum L.) SPDS gene in Arabidopsis resulted in higher spermidine content, and cold tolerance (Zhang et al. 2023 ). Overexpression of the datura spermidine synthase gene in tobacco results in an increasing activity of SPDS, which elevated the spermidine content, but made no difference to spermine and total polyamine level. The resulting transgenic tobacco plants displayed no observable phenotypic alterations (Franceschetti et al. 2004 ). In rice, overexpression of OsSPMS1 led to a decreased level of spermidine and accumulation of putrescine, but there was no significant difference in spermine content between the wild-type and OsSPMS1- overexpressing plants (Tao et al. 2018 ). Therefore, polyamine levels are not only determined by the expression of SPDS / SPMS or activities of SPDS/SPMS but are also affected by global regulation mechanisms.
Although overexpression of TaSPDS- 7D1 increases wheat resistance to FHB, it has limited function in reducing DON production on wheat. Since the external application of putrescine did not lead to an increase in DON production (Fig. 1 e), a possible explanation for the lack of impact of TaSPDS -7D1 overexpression on DON production is that the putrescine levels in wild-type wheat during infection might already be adequate to induce high levels of DON. The heightened putrescine content in TaSPDS -7D1 overexpression lines may not further stimulate DON production. Therefore, other approaches need to be explored to generate FHB resistance wheat or reduce FHB severity based on reducing DON accumulation. Transgenic wheat plants silenced for or mutation in ODC and/or ADC may result in significantly reduced putrescine levels in wheat heads, as it may reduce DON accumulation. However, since polyamines play important roles in many biological processes, it may be difficult to generate such kind of low-polyamine content transgenic wheat without affecting main agronomic traits. Therefore, suppressing the polyamine ingestion pathway by F. graminearum would be an effective way. Crespo-Sempere and colleagues studied the effect of polyamine biosynthesis inhibitor DFMO (D, L-α-difluoromethylornithine) and polyamine transport inhibitors on growth, DON production, and F . graminearum infection. They found that DFMO can reduce growth and DON production but exogenous putrescine recovers its effects in culture, suggesting an ingestion event of pathogens from outside (Crespo-Sempere et al. 2015 ). Meanwhile, polyamine transport inhibitors suppress F. graminearum growth and DON contamination in planta . Therefore, high-affinity and specific polyamine transport inhibitors could be developed into target-specific fungicides for controlling FHB. In addition, reducing host polyamine export by silencing or editing putrescine transporter genes may reduce apoplastic putrescine levels and DON accumulation.
In summary, the present study showed that while maintaining the same amount of total nitrogen in TBI culture, putrescine is the most potent inducer of DON compared to other metabolites in the polyamine biosynthesis pathway. Exogenously application of higher polyamines on wheat head significantly reduced the diseased spikelet number. TaSPDS- 7D1-overexpressing line confers a higher spermidine level and elevated resistance to F. graminearum infection than the wild-type wheat. However, there was no significant difference in DON accumulation. The TaSPDS- 7D1-overexpressing line OE3 exhibited higher 1000-grain weight and plant height compared to the wild type. Our results showed that overexpression of the spermidine synthase gene enhances wheat resistance to FHB without compromising wheat yield.
Plant and fungal materials and treatments
Wheat ( T. aestivum L.) cultivar Fielder was obtained from the State Key Laboratory for Crop Stress Resistance and High-Efficiency Production (Yangling, Shaanxi). All wheat plants were grown in the greenhouse at a relative humidity of 65%, 40,000 Lux with a 16 h light/8 h dark photoperiod, and a temperature of 22°C during the day and then 16°C during the night. Their agronomic traits were recorded and plants were harvested subsequently. The F. graminearum wild-type strain PH-1 was cultured on potato dextrose agar (PDA) plates at 25°C.
Sequence identification and generation of transgenic wheat
The 972-bp full-length coding sequence (CDS) of TaSPDS- 7D1 was cloned into the pANIC-6E plasmid under the control of the maize ubiquitin promoter using the Gateway system (Invitrogen, USA). Then, the construct was transformed into Agrobacterium strain EHA105. The TaSPDS- 7D1-overexpressing wheat lines were generated in the State Key Laboratory for Crop Stress Resistance and High-Efficiency Production (Yangling, Shaanxi) using Agrobacterium -mediated transformation (Ishida et al. 2015 ) and screened using 10 mg/L glufosinate-ammonium. The 6E-Check-F/6E-Check-R were used for certifying the presence of the pANIC-6E in the transgenic plants. Real-time quantitative PCR (qRT-PCR) was employed to detect the expression levels, TaSPDS- 7D1 gene expression levels are normalized to that of TaEF-1α (Schoonbeek et al. 2015 ). All primers used in this study are listed in Additional file 1 : Table S2. The presence of TaSPDS-7D1-HA fusion protein was detected by western blot analysis with the anti-HA (1:3000 dilution, AE008, ABclonal, USA) antibodies.
F. graminearum inoculation and deoxynivalenol production assay
The wheat heads at the anthesis stage were inoculated with 10 μL of 2 × 10 5 spores/mL conidium suspensions at the fifth spikelet from the top. Inoculated wheat heads were moisturized for 36 h with plastic bags (Ren et al. 2022 ). For spraying application of exogenous polyamines, 5 mM of putrescine, spermidine, and spermine were sprayed on the wheat head three times separately along with and every two days after F. graminearum inoculation. The number of diseased spikelets per head was examined 14 days post inoculation (dpi). DON production in the inoculated spikelets sampled at 14 dpi was assayed by gas chromatography-mass spectrometry (GCMS-QP2010) system with AOC-20i autoinjector (Shimadzu Co. Japan). TBI medium was used for in vitro induction of DON. 5 mM Glutamine, 2.5 mM arginine, 5 mM ornithine, 2.5 mM agmatine, 5 mM putrescine, 3.33 mM spermidine, and 2.5 mM spermine were used as the sole nitrogen source. A 20 μL aliquot of F. graminearum conidial suspension (1 × 10 6 /mL) was mixed with 1.98 mL TBI in 24-well polypropylene plates and incubated in the dark without shaking at 28°C for 7 days.
Polyamines measurement
For polyamines measurement, the inoculation method was the same as introduced before (Ren et al. 2022 ), the only difference is conidium suspensions of F. graminearum were injected into all of the florets on the wheat head during anthesis, while sterile distilled water was inoculated in the same manner as the control. The spikelets and rachises were collected 5 dpi. Polyamines were estimated using high-performance liquid chromatography (HPLC) as described by Flores and Galston (Flores and Galston 1982 ) with minor modifications. HPLC analysis was performed using a Shimadzu LC-20AD HPLC instrument (Shimadzu Co. Japan). The sample (5 μL) was injected and chromatographed on a WondaSil C18 column (150 mm × 3.0 mm, 5 μm) using an auto-injector with 60% methanol at a flow rate of 0.7 mL/min. Fluorescence was detected at 230 nm. Chromatographic data were analyzed using the Chromatopac system.
Availability of data and materials
Not applicable.
Abbreviations
Arginine decarboxylase
Complementary DNA
Coding sequence
Carboxymethyl cellulose
Decarboxylated S-adenosylmethionine
D, L-α-difluoromethylornithine
Deoxynivalenol
Days post inoculation
Fusarium graminearum
- Fusarium head blight
Hydroxycinnamic acids amides
Hydroxycinnamic acids
Hours post inoculation
High-performance liquid chromatography
Hypersensitive response
Ornithine decarboxylase
Polyamine oxidases
Polymerase Chain Reaction
Potato dextrose agar
Quantitative real-time PCR
RNA sequencing
Reactive oxygen species
S-adenosylmethionine decarboxylase
Sodium dodecyl sulfate
Spermidine synthase
Spermine synthase
Trichothecene biosynthesis-inducing
Transcripts per million
Bianchini A, Horsley R, Jack MM, Kobielush B, Ryu D, Tittlemier S, et al. DON occurrence in grains: a north American perspective. Cereal Foods World. 2015;60:32–56. https://doi.org/10.1094/CFW-60-1-0032 .
Article Google Scholar
Boenisch MJ, Schäfer W. Fusarium graminearum forms mycotoxin producing infection structures on wheat. BMC Plant Biol. 2011;11:1–14. https://doi.org/10.1186/1471-2229-11-110 .
Article CAS Google Scholar
Chen LF, Lu W, Sun J, Guo SR, Zhang ZX, Yang YJ. Effects of exogenous spermidine on photosynthesis and carbohydrate accumulation in roots and leaves of cucumber ( Cucumis sativus L.) seedlings under salt stress. J Nanjing Agric Uni. 2011;34:31–6. (in Chinese).
Google Scholar
Chen Y, Kistler HC, Ma ZH. Fusarium graminearum trichothecene mycotoxins: biosynthesis, regulation, and management. Annu Rev Phytopathol. 2019;57:15–39. https://doi.org/10.1146/annurev-phyto-082718-100318 .
Article CAS PubMed Google Scholar
Choubey A, Rajam MV. RNAi-mediated silencing of spermidine synthase gene results in reduced reproductive potential in tobacco. Physiol Mol Biol Plants. 2018;24:1069–81. https://doi.org/10.1007/s12298-018-0572-x .
Article CAS PubMed PubMed Central Google Scholar
Crespo-Sempere A, Estiarte N, Marin S, Sanchis V, Ramos AJ. Targeting Fusarium graminearum control via polyamine enzyme inhibitors and polyamine analogs. Food Microbiol. 2015;49:95–103. https://doi.org/10.1016/j.fm.2015.01.020 .
Ebeed HT, Hassan NM, Aljarani AM. Exogenous applications of polyamines modulate drought responses in wheat through osmolytes accumulation, increasing free polyamine levels and regulation of polyamine biosynthetic genes. Plant Physiol Biochem. 2017;118:438–48. https://doi.org/10.1016/j.plaphy.2017.07.014 .
Flores HE, Galston AW. Analysis of polyamines in higher plants by high performance liquid chromatography. Plant Physiol. 1982;69:701–6. https://doi.org/10.1104/pp.69.3.701 .
Franceschetti M, Fornalé S, Tassonia A, Zuccherelli K, Mayer MJ, Bagni N. Effects of spermidine synthase overexpression on polyamine biosynthetic pathway in tobacco plants. J Plant Physiol. 2004;161:989–1001. https://doi.org/10.1016/j.jplph.2004.02.004 .
Gardiner DM, Kazan K, Manners JM. Nutrient profiling reveals potent inducers of trichothecene biosynthesis in Fusarium Graminearum . Fungal Genet Biol. 2009. https://doi.org/10.1016/j.fgb.2009.04.004 . 46:604 – 13.
Article PubMed Google Scholar
Gardiner DM, Kazan K, Praud S, Torney FJ, Rusu A, Manners JM. Early activation of wheat polyamine biosynthesis during Fusarium head blight implicates putrescine as an inducer of trichothecene mycotoxin production. BMC Plant Biol. 2010;10:1–13. https://doi.org/10.1186/1471-2229-10-289 .
Gerlin L, Baroukh C, Genin S. Polyamines: double agents in disease and plant immunity. Trends Plant Sci. 2021;26:1061–71. https://doi.org/10.1016/j.tplants.2021.05.007 .
Gonzalez ME, Marco F, Minguet EG, Carrasco-Sorli P, Blázquez MA, Carbonell J, et al. Perturbation of spermine synthase gene expression and transcript profiling provide new insights on the role of the tetraamine spermine in Arabidopsis defense against Pseudomonas viridiflava . Plant Physiol. 2011;156:2266–77. https://doi.org/10.1104/pp.110.171413 .
Gonzalez ME, Jasso-Robles FI, Flores‐Hernández E, Rodríguez‐Kessler M, Pieckenstain FL. Current status and perspectives on the role of polyamines in plant immunity. Ann Appl Biol. 2021;178:244–55. https://doi.org/10.1111/aab.12670 .
Handa AK, Fatima T, Mattoo AK, Polyamines. Bio-molecules with diverse functions in plant and human health and disease. Front Chem. 2018;6:10. https://doi.org/10.3389/fchem.2018.00010 .
Hanzawa Y, Imai A, Michael AJ, Komeda Y, Takahashi T. Characterization of the spermidine synthase-related gene family in Arabidopsis thaliana . FEBS Letters. 2002;527:176 – 80. https://doi.org/10.1016/s0014-5793(02)03217-9 .
Imai A, Matsuyama T, Hanzawa Y, Akiyama T, Tamaoki M, Saji H, et al. Spermidine synthase genes are essential for survival of Arabidopsis. Plant Physiol. 2004;135:1565 73. https://doi.org/10.1104/pp.104.041699 .
Ishida Y, Tsunashima M, Hiei Y, Komari T. Wheat ( Triticum aestivum L.) transformation using immature embryos. In: Wang K, editor. Agrobacterium protocols: volume 1. New York, NY: Springer New York; 2015. pp. 189–98. https://doi.org/10.1007/978-1-4939-1695-5_15 .
Chapter Google Scholar
Jansen C, von Wettstein D, Schäfer W, Kogel KH, Felk A, Maier FJ. Infection patterns in barley and wheat spikes inoculated with wild-type and trichodiene synthase gene disrupted Fusarium graminearum . Proc Natl Acad Sci U S A. 2005;102:16892–7. https://doi.org/10.1073/pnas.0508467102 .
Ji F, Xu JH, Liu X, Yin XC, Shi JR. Natural occurrence of deoxynivalenol and zearalenone in wheat from Jiangsu province, China. Food Chem. 2014;157:393–7. https://doi.org/10.1016/j.foodchem.2014.02.058 .
Jiang C, Cao SL, Wang ZY, Xu HJ, Liang J, Liu HQ, et al. An expanded subfamily of G-protein-coupled receptor genes in Fusarium graminearum required for wheat infection. Nat Microbiol. 2019;4:1582–91. https://doi.org/10.1038/s41564-019-0468-8 .
Kasukabe Y, He L, Nada K, Misawa S, Ihara I, Tachibana S. Overexpression of spermidine synthase enhances tolerance to multiple environmental stresses and up-regulates the expression of various stress-regulated genes in transgenic Arabidopsis thaliana . Plant Cell Physiol. 2004;45:712–22. https://doi.org/10.1093/pcp/pch083 .
Kaur-Sawhney R, Shih L-M, Flores HE, Galston AW. Relation of polyamine synthesis and titer to aging and senescence in oat leaves. Plant Physiol. 1982;69:405–10. https://doi.org/10.1104/pp.69.2.405 .
Kim S-H, Kim S-H, Yoo S-J, Min K-H, Nam S-H, Cho BH, et al. Putrescine regulating by stress-responsive MAPK cascade contributes to bacterial pathogen defense in Arabidopsis. Biochem Biophys Res Commun. 2013;437:502–8. https://doi.org/10.1016/j.bbrc.2013.06.080 .
KoÇ E. Exogenous application of spermidine enhanced tolerance of pepper against Phytophthora capsici stress. Plant Prot Sci. 2015;51:127–35. https://doi.org/10.17221/86/2014-pps .
Liu Y, Gu DD, Wu W, Wen XX, Liao YC. The relationship between polyamines and hormones in the regulation of wheat grain filling. PLoS ONE. 2013;8:e78196. https://doi.org/10.1371/journal.pone.0078196 .
Luo J, Wei B, Han J, Liao YC, Liu Y. Spermidine increases the sucrose content in inferior grain of eheat and thereby promotes its grain filling. Front Plant Sci. 2019;10:1309. https://doi.org/10.3389/fpls.2019.01309 .
Article PubMed PubMed Central Google Scholar
Ma TL, Zhang LX, Wang MH, Li YQ, Jian YQ, Wu L, et al. Plant defense compound triggers mycotoxin synthesis by regulating H2B ub1 and H3K4 me2/3 deposition. New Phytol. 2021;232:2106–23. https://doi.org/10.1111/nph.17718 .
Majumdar R, Minocha R, Lebar MD, Rajasekaran K, Long S, Carter-Wientjes C, et al. Contribution of maize polyamine and amino acid metabolism toward resistance against Aspergillus flavus infection and aflatoxin production. Front Plant Sci. 2019;10:692. https://doi.org/10.3389/fpls.2019.00692 .
Michael AJ. Biosynthesis of polyamines and polyamine-containing molecules. Biochem J. 2016;473:2315–29. https://doi.org/10.1042/BCJ20160185 .
Moschou PN, Wu J, Cona A, Tavladoraki P, Angelini R, Roubelakis-Angelakis KA. The polyamines and their catabolic products are significant players in the turnover of nitrogenous molecules in plants. J Exp Bot. 2012;63:5003–15. https://doi.org/10.1093/jxb/ers202 .
Moselhy SS, Asami T, Abualnaja KO, Al-Malki AL, Yamano H, Akiyama T, et al. Spermidine, a polyamine, confers resistance to rice blast. J Pestic Sci. 2016;41:79–82. https://doi.org/10.1584/jpestics.D16-008 .
Pal M, Janda T. Role of polyamine metabolism in plant pathogen interactions. J Plant Sci Phytopathol. 2017;1:095–100. https://doi.org/10.29328/journal.jpsp.1001012 .
Pestka JJ. Deoxynivalenol: mechanisms of action, human exposure, and toxicological relevance. Arch Toxicol. 2010. https://doi.org/10.1007/s00204-010-0579-8 . 84:663 – 79.
Ren J, Zhang Y, Wang Y, Li C, Bian Z, Zhang X, et al. Deletion of all three MAP kinase genes results in severe defects in stress responses and pathogenesis in Fusarium graminearum . Stress Biol. 2022;2. https://doi.org/10.1007/s44154-021-00025-y .
Schoonbeek Hj, Wang HH, Stefanato FL, Craze M, Bowden S, Wallington E, et al. Arabidopsis EF-Tu receptor enhances bacterial disease resistance in transgenic wheat. New Phytol. 2015;206:606–13. https://doi.org/10.1111/nph.13356 .
Takahashi Y. The role of polyamines in plant disease resistance. Environ Control Biol. 2016;54:17–21. https://doi.org/10.2525/ecb.54.17 .
Tao YJ, Wang J, Miao J, Chen J, Wu SJ, Zhu JY, et al. The spermine synthase OsSPMS1 regulates seed germination, grain size, and yield. Plant Physiol. 2018;178:1522–36. https://doi.org/10.1104/pp.18.00877 .
Upadhyay RK, Shao J, Mattoo AK. Genomic analysis of the polyamine biosynthesis pathway in duckweed Spirodela polyrhiza L.: presence of the arginine decarboxylase pathway, absence of the ornithine decarboxylase pathway, and response to abiotic stresses. Planta. 2021;254:108. https://doi.org/10.1007/s00425-021-03755-5 .
van Janse HC, Limami AM, Van den Ende W. Spermine and spermidine priming against Botrytis cinerea modulates ROS dynamics and metabolism in Arabidopsis. Biomolecules. 2021;11:223. https://doi.org/10.3390/biom11020223 .
Xia R, Schaafsma A, Wu F, Hooker D. Impact of the improvements in Fusarium head blight and agronomic management on economics of winter wheat. World Mycotoxin J. 2020;13:423–39.
Yang JC, Cao Y, Zhang H, Liu LJ, Zhang JH. Involvement of polyamines in the post-anthesis development of inferior and superior spikelets in rice. Planta. 2008;228:137–49. https://doi.org/10.1007/s00425-008-0725-1 .
Zeiss DR, Piater LA, Dubery IA. Hydroxycinnamate amides: intriguing conjugates of plant protective metabolites. Trends Plant Sci. 2021;26:184–95. https://doi.org/10.1016/j.tplants.2020.09.011 .
Zhang J, Xie M, Yu G, Wang D, Xu Z, Liang L, et al. CaSPDS , a spermidine synthase gene from pepper ( Capsicum annuum L.), plays an important role in response to cold stress. Int J Mol Sci. 2023;24:5013. https://doi.org/10.3390/ijms24055013 .
Download references
Acknowledgements
We thank Dr. Qinhu Wang at Northwest A&F University for help with the phylogenetic analysis, and Ms. Ping Xiang at Northwest A&F University for help with the measurement of polyamines, and Dr. Cong Jiang and Dr. Guanghui Wang at Northwest A&F University for fruitful discussions.
This work was supported by grants from the National Key R&D Program of China (2022YFD1400100) and the Chinese Universities Scientific Fund (2452023045).
Author information
Authors and affiliations.
State Key Laboratory for Crop Stress Resistance and High-Efficiency Production and College of Plant Protection, Northwest A&F University, Yangling, 712100, Shaanxi, China
Jingyi Ren, Chengliang Li, Qi Xiu, Ming Xu & Huiquan Liu
You can also search for this author in PubMed Google Scholar
Contributions
HL, MX, and JR designed experiments; JR, CL, and QX performed the experiments; JR, CL, and QX analyzed the data; JR, MX, and HL wrote the manuscript. All authors read and approved the final manuscript.
Corresponding authors
Correspondence to Ming Xu or Huiquan Liu .
Ethics declarations
Ethics approval and consent to participate, consent for publication, competing interests.
The authors declare that they have no competing interests.
Supplementary Information
Additional file 1: table s1..
SPDS/SPMS protein sequences from different plant species used for phylogenetic tree. Table S2. Primers used in this study.
Additional file 2: Figure S1.
Alignment of TaSPDS protein sequence. The sequence of the Spermine_synth domain is underlined with a red solid line.
Rights and permissions
Open Access This article is licensed under a Creative Commons Attribution 4.0 International License, which permits use, sharing, adaptation, distribution and reproduction in any medium or format, as long as you give appropriate credit to the original author(s) and the source, provide a link to the Creative Commons licence, and indicate if changes were made. The images or other third party material in this article are included in the article's Creative Commons licence, unless indicated otherwise in a credit line to the material. If material is not included in the article's Creative Commons licence and your intended use is not permitted by statutory regulation or exceeds the permitted use, you will need to obtain permission directly from the copyright holder. To view a copy of this licence, visit http://creativecommons.org/licenses/by/4.0/ .
Reprints and permissions
About this article
Cite this article.
Ren, J., Li, C., Xiu, Q. et al. Overexpression of wheat spermidine synthase gene enhances wheat resistance to Fusarium head blight. Phytopathol Res 6 , 24 (2024). https://doi.org/10.1186/s42483-024-00243-y
Download citation
Received : 28 January 2024
Accepted : 10 April 2024
Published : 14 May 2024
DOI : https://doi.org/10.1186/s42483-024-00243-y
Share this article
Anyone you share the following link with will be able to read this content:
Sorry, a shareable link is not currently available for this article.
Provided by the Springer Nature SharedIt content-sharing initiative
- Deoxynivalenol (DON)
Phytopathology Research
ISSN: 2524-4167

IMAGES
VIDEO
COMMENTS
A formal comparison of the two methods and a sufficient condition under which they will yield the same results when nonparametric estimators are ... Doubly-robust estimation of the population average treatment effect is an area for future research. ... Pearl J. Generalizing experimental findings. Journal of Causal Inference. 2015; 3 (2):259 ...
'Summary of findings' tables are usually supported by full evidence profiles which include the detailed ratings of the evidence (Guyatt et al 2011a, Guyatt et al 2013a, Guyatt et al 2013b, Santesso et al 2016). ... in which case even a modest difference from the intervention group will yield a large and therefore misleading ratio of means ...
1. Determine a clear and focused question. A systematic search can best be applied to a well-defined and precise research or clinical question. Questions that are too broad or too vague cannot be answered easily in a systematic way and will generally result in an overwhelming number of search results.
Abstract. This paper aims to provide an overview of the use and assessment of qualitative research methods in the health sciences. Qualitative research can be defined as the study of the nature of phenomena and is especially appropriate for answering questions of why something is (not) observed, assessing complex multi-component interventions ...
It can be defined as the extension of research findings and conclusions from a study conducted on a sample population to the population at large. ... and others -- would have to be addressed in order for the study to yield potentially valid results. However, even if virtually all variables were isolated, results of the study would not be 100% ...
A key role of public health policy-makers and practitioners is to ensure beneficial interventions are implemented effectively enough to yield improvements in public health. The use of evidence to guide public health decision-making to achieve this is recommended. However, few studies have examined the relative value, as reported by policy-makers and practitioners, of different broad research ...
Underlying crop yield prediction is a fundamental research question in ... and that late planting time is associated with lower yield performance. These findings are consistent with results from ...
An essential element of scientific realism is the frequent and long-term corroboration of statements based on empirical tests. From an empirical perspective, it is about the question of generalizability, and to what extent empirical findings on the same statement found in various other studies are confirmed.The current chapter deals with approaches in which different results are summarized for ...
Published research findings are sometimes refuted by subsequent evidence, with ensuing confusion and disappointment. Refutation and controversy is seen across the range of research designs, from clinical trials and traditional epidemiological studies [] to the most modern molecular research [4,5].There is increasing concern that in modern research, false findings may be the majority or even ...
Climate change challenges efforts to maintain and improve crop production in many regions. In this Review, we examine yield responses to warmer temperatures, elevated carbon dioxide and changes in ...
Among the findings from scholarly efforts examining non-reproducibility is that, in a significant portion of cases, the cause could be traced to poor practices in reporting research results, and ...
Revised on March 3, 2023. Generalizability is the degree to which you can apply the results of your study to a broader context. Research results are considered generalizable when the findings can be applied to most contexts, most people, most of the time. Example: Generalizability. Suppose you want to investigate the shopping habits of people ...
This kind of a study can be turned into an academic contribution by identifying an interesting and novel finding, and deepening the literature research so that it convinces the reader about the novelty and the need for this finding in the research field (e.g., a "research gap"). Mappings of findings to a framework. Some papers present ...
Section 5 explains the deep learning-based crop yield prediction research. Section 5 presents the discussion, and Section 7 concludes this paper. 2. Related work. Crop yield prediction is an essential task for the decision-makers at national and regional levels (e.g., the EU level) for rapid decision-making.
physical design methods to improve yield. Yield is defined as the ratio of the number of. products that can be sold to the number of products that can b e manufactured. Estimated. typical cost of ...
Measured yield performance of any product is the result of the genetics (G) of the product and the environment (E) in which it is tested, with the combined effects known as the G x E interaction. One must always keep in mind that yield trials deal with many variables that can contribute to yield performance. Average yields can also change as more data is accumulated across locations.
This research presents a bibliometric analysis of articles predicting crop yield using machine learning methods. While several systematic review articles exist, a comprehensive bibliometric analysis illustrating the knowledge structure and research trends, along with collaboration networks among authors, institutions, and countries in this field, has not been conducted. The study focused on ...
ISU Research Findings. Read Our Take. PROVEN® 40 Impact on Nutrient Uptake & Yield . NC State Research Findings. Read Our Take. Decoding the Data. 2023 Beck's PFR. ... researchers at the University of Kentucky observed notable grain yield and plant health benefits. PROVEN® 40 Silage Report 2022-2023.
"Diagnostic yield" is a parameter that is positioned between diagnostic accuracy and diagnosis-related patient outcomes in studies of diagnostic tests [3,8]. Diagnostic yield studies have focused on the effects of test results on clinical decisions [2,3,9]. These studies target diagnostic cohorts with a particular indication for a test and ...
Working Paper 32450. DOI 10.3386/w32450. Issue Date May 2024. This paper estimates that the macroeconomic damages from climate change are six times larger than previously thought. We exploit natural variability in global temperature and rely on time-series variation. A 1°C increase in global temperature leads to a 12% decline in world GDP.
The frequency distribution histogram of yield for all the trials is given in Fig. 2 and some statistical data about the yield in these trials are given in Table 2. From 2017 to 2021, the number of trials conducted was 6152, 8262, 9786, 8927 and 8846 with mean yields of 693.91, 698.24, 720.62, 711.86 and 696.7 kg/mu respectively.
Water scarcity and the overuse of chemical fertilizers present significant challenges to modern agriculture, critically affecting crop photosynthesis, yield, quality, and productivity sustainability. This research assesses the impact of organic fertilizer on the photosynthetic attributes, yield, and quality of pakchoi under varying irrigation water conditions, including fresh water and ...
Our findings indicate that tonic alertness continues to develop from ages 6 to 13 in both deaf and typical hearing children (Dye and Hauser, 2014; Dye and Terhune-Cotter, 2023). This aligns with previous research on typical hearing children using the same task, which showed a specific development ceiling at 10 years old (Betts et al., 2006).
In May, Federal Reserve Financial Services' FedCash ® Services released the annual Diary of Consumer Payment Choice (PDF) report from its ongoing research into the payment habits of the U.S. population. The 2024 findings revealed consumers made more payments in 2023 than in previous years, continuing the trend of rising payment transactions since 2020.
Our findings suggest that successful potato cultivation is related (1) to planting of oil radish before potatoes for increasing yield and (2) to fertilisation with manure or straw + slurry for enriching the microbiome with crop-beneficial taxa. ... Sustainable Food Systems Research Centre, Rhine-Waal University of Applied Sciences, Marie-Curie ...
A research team has made significant strides in understanding the mechanisms of fruit abscission in woody fruit crops, an essential process affecting fruit yield and economic value. This review ...
This study aimed to document the effects of long-term organic farming (OF) impact on the soil quality, agronomical parameters, crop productivity and food grain yield compared to conventional farming system (CF). The crop in this study was chickpea (Cicer arietinum) and the field was located at Pantnagar, India in the foothills of Himalayas. Organic farming approach involved utilizing a blend ...
Hence, the essence of reliability for qualitative research lies with consistency.[24,28] A margin of variability for results is tolerated in qualitative research provided the methodology and epistemological logistics consistently yield data that are ontologically similar but may differ in richness and ambience within similar dimensions.
In addition, the TaSPDS-7D1-overexpressing line OE3 exhibited a 1000-grain weight and plant height increase compared to the wild type. Our findings reveal that overexpression of the spermidine synthase gene can enhance wheat resistance to FHB without compromising wheat yield.