Get science-backed answers as you write with Paperpal's Research feature

How to Write a Conclusion for Research Papers (with Examples)
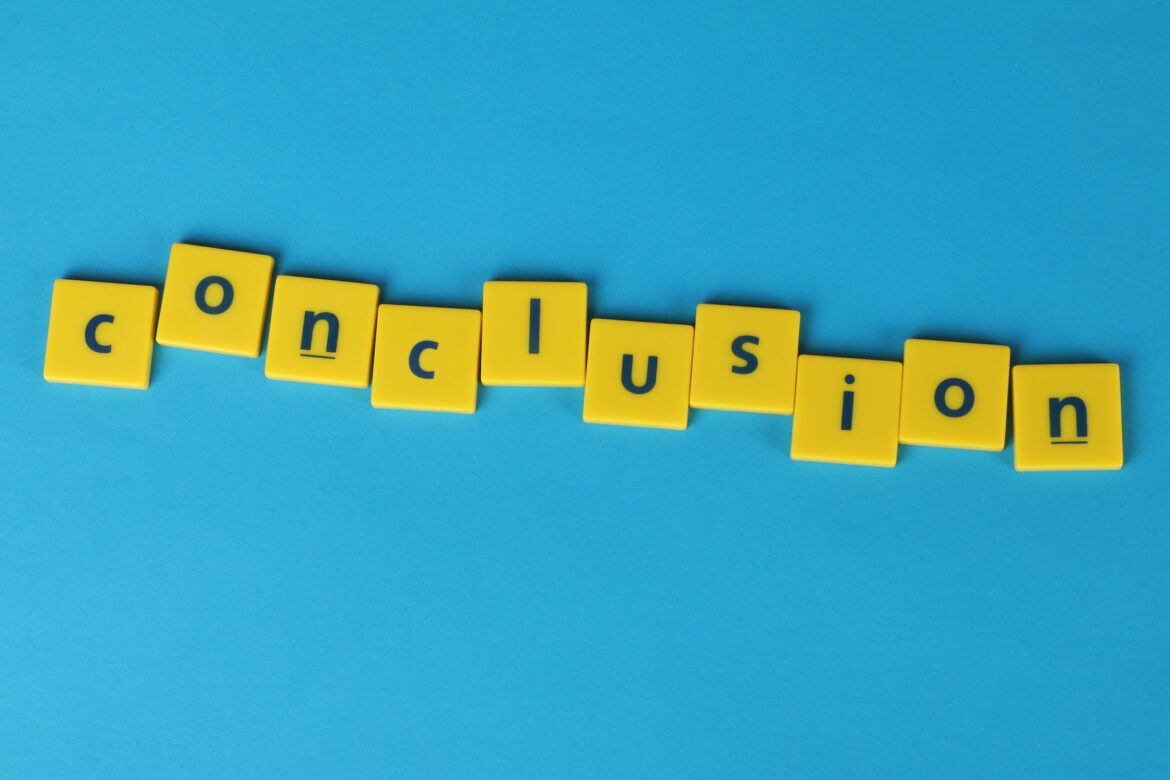
The conclusion of a research paper is a crucial section that plays a significant role in the overall impact and effectiveness of your research paper. However, this is also the section that typically receives less attention compared to the introduction and the body of the paper. The conclusion serves to provide a concise summary of the key findings, their significance, their implications, and a sense of closure to the study. Discussing how can the findings be applied in real-world scenarios or inform policy, practice, or decision-making is especially valuable to practitioners and policymakers. The research paper conclusion also provides researchers with clear insights and valuable information for their own work, which they can then build on and contribute to the advancement of knowledge in the field.
The research paper conclusion should explain the significance of your findings within the broader context of your field. It restates how your results contribute to the existing body of knowledge and whether they confirm or challenge existing theories or hypotheses. Also, by identifying unanswered questions or areas requiring further investigation, your awareness of the broader research landscape can be demonstrated.
Remember to tailor the research paper conclusion to the specific needs and interests of your intended audience, which may include researchers, practitioners, policymakers, or a combination of these.
Table of Contents
What is a conclusion in a research paper, summarizing conclusion, editorial conclusion, externalizing conclusion, importance of a good research paper conclusion, how to write a conclusion for your research paper, research paper conclusion examples.
- How to write a research paper conclusion with Paperpal?
Frequently Asked Questions
A conclusion in a research paper is the final section where you summarize and wrap up your research, presenting the key findings and insights derived from your study. The research paper conclusion is not the place to introduce new information or data that was not discussed in the main body of the paper. When working on how to conclude a research paper, remember to stick to summarizing and interpreting existing content. The research paper conclusion serves the following purposes: 1
- Warn readers of the possible consequences of not attending to the problem.
- Recommend specific course(s) of action.
- Restate key ideas to drive home the ultimate point of your research paper.
- Provide a “take-home” message that you want the readers to remember about your study.
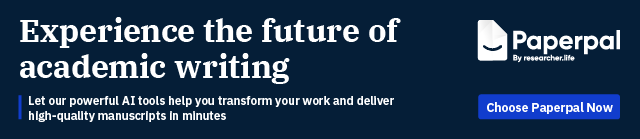
Types of conclusions for research papers
In research papers, the conclusion provides closure to the reader. The type of research paper conclusion you choose depends on the nature of your study, your goals, and your target audience. I provide you with three common types of conclusions:
A summarizing conclusion is the most common type of conclusion in research papers. It involves summarizing the main points, reiterating the research question, and restating the significance of the findings. This common type of research paper conclusion is used across different disciplines.
An editorial conclusion is less common but can be used in research papers that are focused on proposing or advocating for a particular viewpoint or policy. It involves presenting a strong editorial or opinion based on the research findings and offering recommendations or calls to action.
An externalizing conclusion is a type of conclusion that extends the research beyond the scope of the paper by suggesting potential future research directions or discussing the broader implications of the findings. This type of conclusion is often used in more theoretical or exploratory research papers.
Align your conclusion’s tone with the rest of your research paper. Start Writing with Paperpal Now!
The conclusion in a research paper serves several important purposes:
- Offers Implications and Recommendations : Your research paper conclusion is an excellent place to discuss the broader implications of your research and suggest potential areas for further study. It’s also an opportunity to offer practical recommendations based on your findings.
- Provides Closure : A good research paper conclusion provides a sense of closure to your paper. It should leave the reader with a feeling that they have reached the end of a well-structured and thought-provoking research project.
- Leaves a Lasting Impression : Writing a well-crafted research paper conclusion leaves a lasting impression on your readers. It’s your final opportunity to leave them with a new idea, a call to action, or a memorable quote.
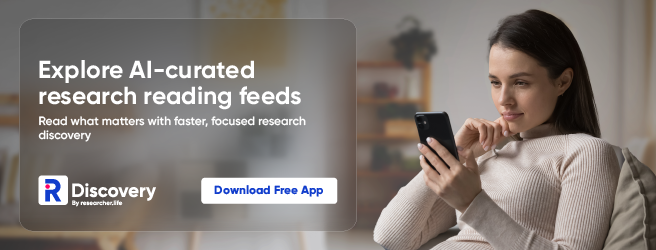
Writing a strong conclusion for your research paper is essential to leave a lasting impression on your readers. Here’s a step-by-step process to help you create and know what to put in the conclusion of a research paper: 2
- Research Statement : Begin your research paper conclusion by restating your research statement. This reminds the reader of the main point you’ve been trying to prove throughout your paper. Keep it concise and clear.
- Key Points : Summarize the main arguments and key points you’ve made in your paper. Avoid introducing new information in the research paper conclusion. Instead, provide a concise overview of what you’ve discussed in the body of your paper.
- Address the Research Questions : If your research paper is based on specific research questions or hypotheses, briefly address whether you’ve answered them or achieved your research goals. Discuss the significance of your findings in this context.
- Significance : Highlight the importance of your research and its relevance in the broader context. Explain why your findings matter and how they contribute to the existing knowledge in your field.
- Implications : Explore the practical or theoretical implications of your research. How might your findings impact future research, policy, or real-world applications? Consider the “so what?” question.
- Future Research : Offer suggestions for future research in your area. What questions or aspects remain unanswered or warrant further investigation? This shows that your work opens the door for future exploration.
- Closing Thought : Conclude your research paper conclusion with a thought-provoking or memorable statement. This can leave a lasting impression on your readers and wrap up your paper effectively. Avoid introducing new information or arguments here.
- Proofread and Revise : Carefully proofread your conclusion for grammar, spelling, and clarity. Ensure that your ideas flow smoothly and that your conclusion is coherent and well-structured.
Write your research paper conclusion 2x faster with Paperpal. Try it now!
Remember that a well-crafted research paper conclusion is a reflection of the strength of your research and your ability to communicate its significance effectively. It should leave a lasting impression on your readers and tie together all the threads of your paper. Now you know how to start the conclusion of a research paper and what elements to include to make it impactful, let’s look at a research paper conclusion sample.
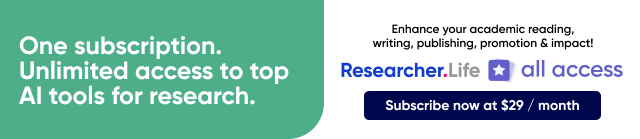
How to write a research paper conclusion with Paperpal?
A research paper conclusion is not just a summary of your study, but a synthesis of the key findings that ties the research together and places it in a broader context. A research paper conclusion should be concise, typically around one paragraph in length. However, some complex topics may require a longer conclusion to ensure the reader is left with a clear understanding of the study’s significance. Paperpal, an AI writing assistant trusted by over 800,000 academics globally, can help you write a well-structured conclusion for your research paper.
- Sign Up or Log In: Create a new Paperpal account or login with your details.
- Navigate to Features : Once logged in, head over to the features’ side navigation pane. Click on Templates and you’ll find a suite of generative AI features to help you write better, faster.
- Generate an outline: Under Templates, select ‘Outlines’. Choose ‘Research article’ as your document type.
- Select your section: Since you’re focusing on the conclusion, select this section when prompted.
- Choose your field of study: Identifying your field of study allows Paperpal to provide more targeted suggestions, ensuring the relevance of your conclusion to your specific area of research.
- Provide a brief description of your study: Enter details about your research topic and findings. This information helps Paperpal generate a tailored outline that aligns with your paper’s content.
- Generate the conclusion outline: After entering all necessary details, click on ‘generate’. Paperpal will then create a structured outline for your conclusion, to help you start writing and build upon the outline.
- Write your conclusion: Use the generated outline to build your conclusion. The outline serves as a guide, ensuring you cover all critical aspects of a strong conclusion, from summarizing key findings to highlighting the research’s implications.
- Refine and enhance: Paperpal’s ‘Make Academic’ feature can be particularly useful in the final stages. Select any paragraph of your conclusion and use this feature to elevate the academic tone, ensuring your writing is aligned to the academic journal standards.
By following these steps, Paperpal not only simplifies the process of writing a research paper conclusion but also ensures it is impactful, concise, and aligned with academic standards. Sign up with Paperpal today and write your research paper conclusion 2x faster .
The research paper conclusion is a crucial part of your paper as it provides the final opportunity to leave a strong impression on your readers. In the research paper conclusion, summarize the main points of your research paper by restating your research statement, highlighting the most important findings, addressing the research questions or objectives, explaining the broader context of the study, discussing the significance of your findings, providing recommendations if applicable, and emphasizing the takeaway message. The main purpose of the conclusion is to remind the reader of the main point or argument of your paper and to provide a clear and concise summary of the key findings and their implications. All these elements should feature on your list of what to put in the conclusion of a research paper to create a strong final statement for your work.
A strong conclusion is a critical component of a research paper, as it provides an opportunity to wrap up your arguments, reiterate your main points, and leave a lasting impression on your readers. Here are the key elements of a strong research paper conclusion: 1. Conciseness : A research paper conclusion should be concise and to the point. It should not introduce new information or ideas that were not discussed in the body of the paper. 2. Summarization : The research paper conclusion should be comprehensive enough to give the reader a clear understanding of the research’s main contributions. 3 . Relevance : Ensure that the information included in the research paper conclusion is directly relevant to the research paper’s main topic and objectives; avoid unnecessary details. 4 . Connection to the Introduction : A well-structured research paper conclusion often revisits the key points made in the introduction and shows how the research has addressed the initial questions or objectives. 5. Emphasis : Highlight the significance and implications of your research. Why is your study important? What are the broader implications or applications of your findings? 6 . Call to Action : Include a call to action or a recommendation for future research or action based on your findings.
The length of a research paper conclusion can vary depending on several factors, including the overall length of the paper, the complexity of the research, and the specific journal requirements. While there is no strict rule for the length of a conclusion, but it’s generally advisable to keep it relatively short. A typical research paper conclusion might be around 5-10% of the paper’s total length. For example, if your paper is 10 pages long, the conclusion might be roughly half a page to one page in length.
In general, you do not need to include citations in the research paper conclusion. Citations are typically reserved for the body of the paper to support your arguments and provide evidence for your claims. However, there may be some exceptions to this rule: 1. If you are drawing a direct quote or paraphrasing a specific source in your research paper conclusion, you should include a citation to give proper credit to the original author. 2. If your conclusion refers to or discusses specific research, data, or sources that are crucial to the overall argument, citations can be included to reinforce your conclusion’s validity.
The conclusion of a research paper serves several important purposes: 1. Summarize the Key Points 2. Reinforce the Main Argument 3. Provide Closure 4. Offer Insights or Implications 5. Engage the Reader. 6. Reflect on Limitations
Remember that the primary purpose of the research paper conclusion is to leave a lasting impression on the reader, reinforcing the key points and providing closure to your research. It’s often the last part of the paper that the reader will see, so it should be strong and well-crafted.
- Makar, G., Foltz, C., Lendner, M., & Vaccaro, A. R. (2018). How to write effective discussion and conclusion sections. Clinical spine surgery, 31(8), 345-346.
- Bunton, D. (2005). The structure of PhD conclusion chapters. Journal of English for academic purposes , 4 (3), 207-224.
Paperpal is a comprehensive AI writing toolkit that helps students and researchers achieve 2x the writing in half the time. It leverages 21+ years of STM experience and insights from millions of research articles to provide in-depth academic writing, language editing, and submission readiness support to help you write better, faster.
Get accurate academic translations, rewriting support, grammar checks, vocabulary suggestions, and generative AI assistance that delivers human precision at machine speed. Try for free or upgrade to Paperpal Prime starting at US$19 a month to access premium features, including consistency, plagiarism, and 30+ submission readiness checks to help you succeed.
Experience the future of academic writing – Sign up to Paperpal and start writing for free!
Related Reads:
- 5 Reasons for Rejection After Peer Review
- Ethical Research Practices For Research with Human Subjects
7 Ways to Improve Your Academic Writing Process
- Paraphrasing in Academic Writing: Answering Top Author Queries
Preflight For Editorial Desk: The Perfect Hybrid (AI + Human) Assistance Against Compromised Manuscripts
You may also like, how to write a high-quality conference paper, academic editing: how to self-edit academic text with..., measuring academic success: definition & strategies for excellence, phd qualifying exam: tips for success , ai in education: it’s time to change the..., is it ethical to use ai-generated abstracts without..., what are journal guidelines on using generative ai..., quillbot review: features, pricing, and free alternatives, what is an academic paper types and elements , should you use ai tools like chatgpt for....
- USC Libraries
- Research Guides
Organizing Your Social Sciences Research Paper
- Independent and Dependent Variables
- Purpose of Guide
- Design Flaws to Avoid
- Glossary of Research Terms
- Reading Research Effectively
- Narrowing a Topic Idea
- Broadening a Topic Idea
- Extending the Timeliness of a Topic Idea
- Academic Writing Style
- Applying Critical Thinking
- Choosing a Title
- Making an Outline
- Paragraph Development
- Research Process Video Series
- Executive Summary
- The C.A.R.S. Model
- Background Information
- The Research Problem/Question
- Theoretical Framework
- Citation Tracking
- Content Alert Services
- Evaluating Sources
- Primary Sources
- Secondary Sources
- Tiertiary Sources
- Scholarly vs. Popular Publications
- Qualitative Methods
- Quantitative Methods
- Insiderness
- Using Non-Textual Elements
- Limitations of the Study
- Common Grammar Mistakes
- Writing Concisely
- Avoiding Plagiarism
- Footnotes or Endnotes?
- Further Readings
- Generative AI and Writing
- USC Libraries Tutorials and Other Guides
- Bibliography
Definitions
Dependent Variable The variable that depends on other factors that are measured. These variables are expected to change as a result of an experimental manipulation of the independent variable or variables. It is the presumed effect.
Independent Variable The variable that is stable and unaffected by the other variables you are trying to measure. It refers to the condition of an experiment that is systematically manipulated by the investigator. It is the presumed cause.
Cramer, Duncan and Dennis Howitt. The SAGE Dictionary of Statistics . London: SAGE, 2004; Penslar, Robin Levin and Joan P. Porter. Institutional Review Board Guidebook: Introduction . Washington, DC: United States Department of Health and Human Services, 2010; "What are Dependent and Independent Variables?" Graphic Tutorial.
Identifying Dependent and Independent Variables
Don't feel bad if you are confused about what is the dependent variable and what is the independent variable in social and behavioral sciences research . However, it's important that you learn the difference because framing a study using these variables is a common approach to organizing the elements of a social sciences research study in order to discover relevant and meaningful results. Specifically, it is important for these two reasons:
- You need to understand and be able to evaluate their application in other people's research.
- You need to apply them correctly in your own research.
A variable in research simply refers to a person, place, thing, or phenomenon that you are trying to measure in some way. The best way to understand the difference between a dependent and independent variable is that the meaning of each is implied by what the words tell us about the variable you are using. You can do this with a simple exercise from the website, Graphic Tutorial. Take the sentence, "The [independent variable] causes a change in [dependent variable] and it is not possible that [dependent variable] could cause a change in [independent variable]." Insert the names of variables you are using in the sentence in the way that makes the most sense. This will help you identify each type of variable. If you're still not sure, consult with your professor before you begin to write.
Fan, Shihe. "Independent Variable." In Encyclopedia of Research Design. Neil J. Salkind, editor. (Thousand Oaks, CA: SAGE, 2010), pp. 592-594; "What are Dependent and Independent Variables?" Graphic Tutorial; Salkind, Neil J. "Dependent Variable." In Encyclopedia of Research Design , Neil J. Salkind, editor. (Thousand Oaks, CA: SAGE, 2010), pp. 348-349;
Structure and Writing Style
The process of examining a research problem in the social and behavioral sciences is often framed around methods of analysis that compare, contrast, correlate, average, or integrate relationships between or among variables . Techniques include associations, sampling, random selection, and blind selection. Designation of the dependent and independent variable involves unpacking the research problem in a way that identifies a general cause and effect and classifying these variables as either independent or dependent.
The variables should be outlined in the introduction of your paper and explained in more detail in the methods section . There are no rules about the structure and style for writing about independent or dependent variables but, as with any academic writing, clarity and being succinct is most important.
After you have described the research problem and its significance in relation to prior research, explain why you have chosen to examine the problem using a method of analysis that investigates the relationships between or among independent and dependent variables . State what it is about the research problem that lends itself to this type of analysis. For example, if you are investigating the relationship between corporate environmental sustainability efforts [the independent variable] and dependent variables associated with measuring employee satisfaction at work using a survey instrument, you would first identify each variable and then provide background information about the variables. What is meant by "environmental sustainability"? Are you looking at a particular company [e.g., General Motors] or are you investigating an industry [e.g., the meat packing industry]? Why is employee satisfaction in the workplace important? How does a company make their employees aware of sustainability efforts and why would a company even care that its employees know about these efforts?
Identify each variable for the reader and define each . In the introduction, this information can be presented in a paragraph or two when you describe how you are going to study the research problem. In the methods section, you build on the literature review of prior studies about the research problem to describe in detail background about each variable, breaking each down for measurement and analysis. For example, what activities do you examine that reflect a company's commitment to environmental sustainability? Levels of employee satisfaction can be measured by a survey that asks about things like volunteerism or a desire to stay at the company for a long time.
The structure and writing style of describing the variables and their application to analyzing the research problem should be stated and unpacked in such a way that the reader obtains a clear understanding of the relationships between the variables and why they are important. This is also important so that the study can be replicated in the future using the same variables but applied in a different way.
Fan, Shihe. "Independent Variable." In Encyclopedia of Research Design. Neil J. Salkind, editor. (Thousand Oaks, CA: SAGE, 2010), pp. 592-594; "What are Dependent and Independent Variables?" Graphic Tutorial; “Case Example for Independent and Dependent Variables.” ORI Curriculum Examples. U.S. Department of Health and Human Services, Office of Research Integrity; Salkind, Neil J. "Dependent Variable." In Encyclopedia of Research Design , Neil J. Salkind, editor. (Thousand Oaks, CA: SAGE, 2010), pp. 348-349; “Independent Variables and Dependent Variables.” Karl L. Wuensch, Department of Psychology, East Carolina University [posted email exchange]; “Variables.” Elements of Research. Dr. Camille Nebeker, San Diego State University.
- << Previous: Design Flaws to Avoid
- Next: Glossary of Research Terms >>
- Last Updated: May 22, 2024 11:13 AM
- URL: https://libguides.usc.edu/writingguide

Want to create or adapt books like this? Learn more about how Pressbooks supports open publishing practices.
Drawing Conclusions and Reporting the Results
Rajiv S. Jhangiani; I-Chant A. Chiang; Carrie Cuttler; and Dana C. Leighton
Learning Objectives
- Identify the conclusions researchers can make based on the outcome of their studies.
- Describe why scientists avoid the term “scientific proof.”
- Explain the different ways that scientists share their findings.
Drawing Conclusions
Since statistics are probabilistic in nature and findings can reflect type I or type II errors, we cannot use the results of a single study to conclude with certainty that a theory is true. Rather theories are supported, refuted, or modified based on the results of research.
If the results are statistically significant and consistent with the hypothesis and the theory that was used to generate the hypothesis, then researchers can conclude that the theory is supported. Not only did the theory make an accurate prediction, but there is now a new phenomenon that the theory accounts for. If a hypothesis is disconfirmed in a systematic empirical study, then the theory has been weakened. It made an inaccurate prediction, and there is now a new phenomenon that it does not account for.
Although this seems straightforward, there are some complications. First, confirming a hypothesis can strengthen a theory but it can never prove a theory. In fact, scientists tend to avoid the word “prove” when talking and writing about theories. One reason for this avoidance is that the result may reflect a type I error. Another reason for this avoidance is that there may be other plausible theories that imply the same hypothesis, which means that confirming the hypothesis strengthens all those theories equally. A third reason is that it is always possible that another test of the hypothesis or a test of a new hypothesis derived from the theory will be disconfirmed. This difficulty is a version of the famous philosophical “problem of induction.” One cannot definitively prove a general principle (e.g., “All swans are white.”) just by observing confirming cases (e.g., white swans)—no matter how many. It is always possible that a disconfirming case (e.g., a black swan) will eventually come along. For these reasons, scientists tend to think of theories—even highly successful ones—as subject to revision based on new and unexpected observations.
A second complication has to do with what it means when a hypothesis is disconfirmed. According to the strictest version of the hypothetico-deductive method, disconfirming a hypothesis disproves the theory it was derived from. In formal logic, the premises “if A then B ” and “not B ” necessarily lead to the conclusion “not A .” If A is the theory and B is the hypothesis (“if A then B ”), then disconfirming the hypothesis (“not B ”) must mean that the theory is incorrect (“not A ”). In practice, however, scientists do not give up on their theories so easily. One reason is that one disconfirmed hypothesis could be a missed opportunity (the result of a type II error) or it could be the result of a faulty research design. Perhaps the researcher did not successfully manipulate the independent variable or measure the dependent variable.
A disconfirmed hypothesis could also mean that some unstated but relatively minor assumption of the theory was not met. For example, if Zajonc had failed to find social facilitation in cockroaches, he could have concluded that drive theory is still correct but it applies only to animals with sufficiently complex nervous systems. That is, the evidence from a study can be used to modify a theory. This practice does not mean that researchers are free to ignore disconfirmations of their theories. If they cannot improve their research designs or modify their theories to account for repeated disconfirmations, then they eventually must abandon their theories and replace them with ones that are more successful.
The bottom line here is that because statistics are probabilistic in nature and because all research studies have flaws there is no such thing as scientific proof, there is only scientific evidence.
Reporting the Results
The final step in the research process involves reporting the results. As described in the section on Reviewing the Research Literature in this chapter, results are typically reported in peer-reviewed journal articles and at conferences.
The most prestigious way to report one’s findings is by writing a manuscript and having it published in a peer-reviewed scientific journal. Manuscripts published in psychology journals typically must adhere to the writing style of the American Psychological Association (APA style). You will likely be learning the major elements of this writing style in this course.
Another way to report findings is by writing a book chapter that is published in an edited book. Preferably the editor of the book puts the chapter through peer review but this is not always the case and some scientists are invited by editors to write book chapters.
A fun way to disseminate findings is to give a presentation at a conference. This can either be done as an oral presentation or a poster presentation. Oral presentations involve getting up in front of an audience of fellow scientists and giving a talk that might last anywhere from 10 minutes to 1 hour (depending on the conference) and then fielding questions from the audience. Alternatively, poster presentations involve summarizing the study on a large poster that provides a brief overview of the purpose, methods, results, and discussion. The presenter stands by their poster for an hour or two and discusses it with people who pass by. Presenting one’s work at a conference is a great way to get feedback from one’s peers before attempting to undergo the more rigorous peer-review process involved in publishing a journal article.
Drawing Conclusions and Reporting the Results Copyright © by Rajiv S. Jhangiani; I-Chant A. Chiang; Carrie Cuttler; and Dana C. Leighton is licensed under a Creative Commons Attribution-NonCommercial-ShareAlike 4.0 International License , except where otherwise noted.
Share This Book
When you choose to publish with PLOS, your research makes an impact. Make your work accessible to all, without restrictions, and accelerate scientific discovery with options like preprints and published peer review that make your work more Open.
- PLOS Biology
- PLOS Climate
- PLOS Complex Systems
- PLOS Computational Biology
- PLOS Digital Health
- PLOS Genetics
- PLOS Global Public Health
- PLOS Medicine
- PLOS Mental Health
- PLOS Neglected Tropical Diseases
- PLOS Pathogens
- PLOS Sustainability and Transformation
- PLOS Collections
- How to Write Discussions and Conclusions

The discussion section contains the results and outcomes of a study. An effective discussion informs readers what can be learned from your experiment and provides context for the results.
What makes an effective discussion?
When you’re ready to write your discussion, you’ve already introduced the purpose of your study and provided an in-depth description of the methodology. The discussion informs readers about the larger implications of your study based on the results. Highlighting these implications while not overstating the findings can be challenging, especially when you’re submitting to a journal that selects articles based on novelty or potential impact. Regardless of what journal you are submitting to, the discussion section always serves the same purpose: concluding what your study results actually mean.
A successful discussion section puts your findings in context. It should include:
- the results of your research,
- a discussion of related research, and
- a comparison between your results and initial hypothesis.
Tip: Not all journals share the same naming conventions.
You can apply the advice in this article to the conclusion, results or discussion sections of your manuscript.
Our Early Career Researcher community tells us that the conclusion is often considered the most difficult aspect of a manuscript to write. To help, this guide provides questions to ask yourself, a basic structure to model your discussion off of and examples from published manuscripts.

Questions to ask yourself:
- Was my hypothesis correct?
- If my hypothesis is partially correct or entirely different, what can be learned from the results?
- How do the conclusions reshape or add onto the existing knowledge in the field? What does previous research say about the topic?
- Why are the results important or relevant to your audience? Do they add further evidence to a scientific consensus or disprove prior studies?
- How can future research build on these observations? What are the key experiments that must be done?
- What is the “take-home” message you want your reader to leave with?
How to structure a discussion
Trying to fit a complete discussion into a single paragraph can add unnecessary stress to the writing process. If possible, you’ll want to give yourself two or three paragraphs to give the reader a comprehensive understanding of your study as a whole. Here’s one way to structure an effective discussion:
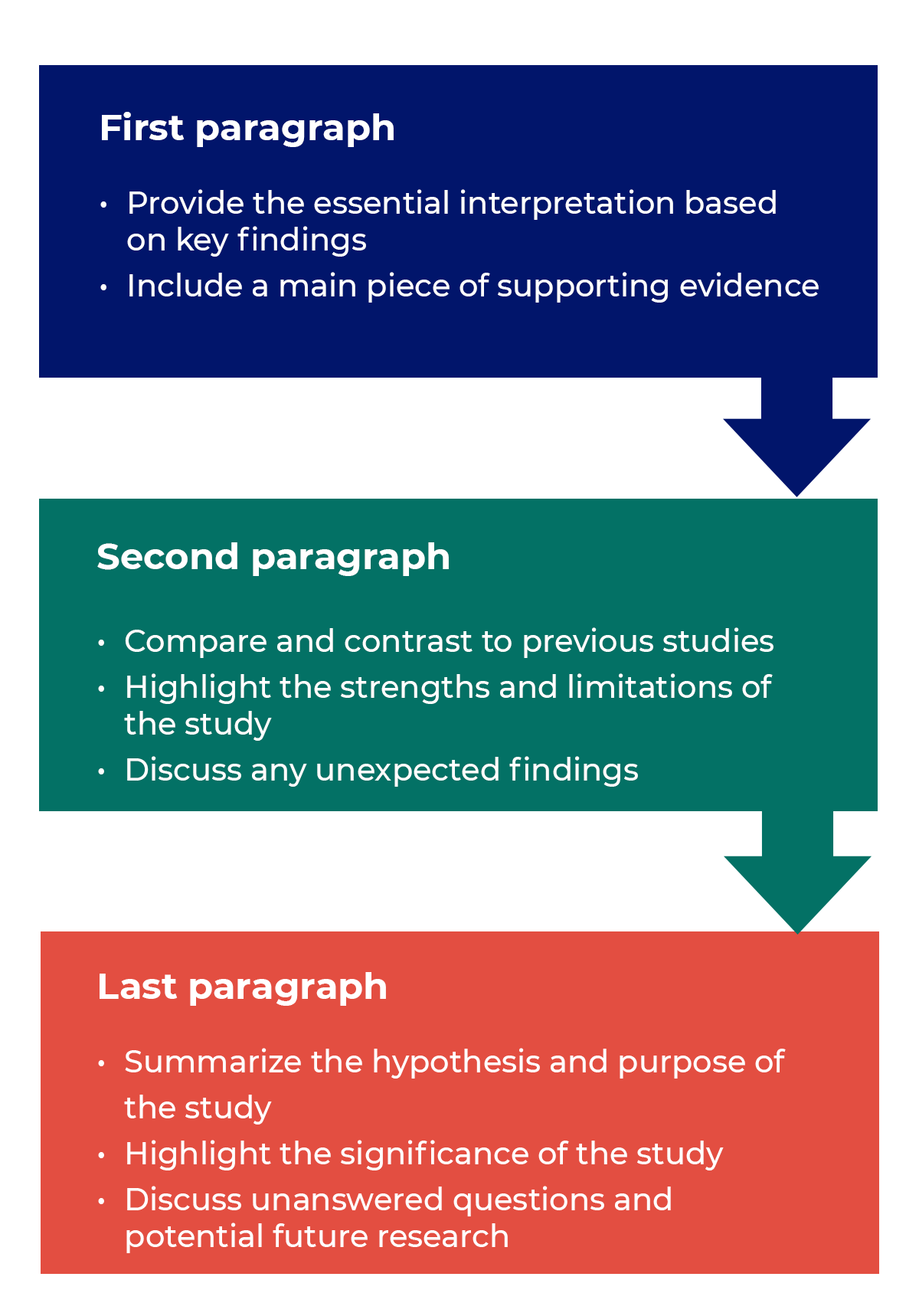
Writing Tips
While the above sections can help you brainstorm and structure your discussion, there are many common mistakes that writers revert to when having difficulties with their paper. Writing a discussion can be a delicate balance between summarizing your results, providing proper context for your research and avoiding introducing new information. Remember that your paper should be both confident and honest about the results!

- Read the journal’s guidelines on the discussion and conclusion sections. If possible, learn about the guidelines before writing the discussion to ensure you’re writing to meet their expectations.
- Begin with a clear statement of the principal findings. This will reinforce the main take-away for the reader and set up the rest of the discussion.
- Explain why the outcomes of your study are important to the reader. Discuss the implications of your findings realistically based on previous literature, highlighting both the strengths and limitations of the research.
- State whether the results prove or disprove your hypothesis. If your hypothesis was disproved, what might be the reasons?
- Introduce new or expanded ways to think about the research question. Indicate what next steps can be taken to further pursue any unresolved questions.
- If dealing with a contemporary or ongoing problem, such as climate change, discuss possible consequences if the problem is avoided.
- Be concise. Adding unnecessary detail can distract from the main findings.
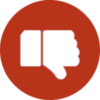
Don’t
- Rewrite your abstract. Statements with “we investigated” or “we studied” generally do not belong in the discussion.
- Include new arguments or evidence not previously discussed. Necessary information and evidence should be introduced in the main body of the paper.
- Apologize. Even if your research contains significant limitations, don’t undermine your authority by including statements that doubt your methodology or execution.
- Shy away from speaking on limitations or negative results. Including limitations and negative results will give readers a complete understanding of the presented research. Potential limitations include sources of potential bias, threats to internal or external validity, barriers to implementing an intervention and other issues inherent to the study design.
- Overstate the importance of your findings. Making grand statements about how a study will fully resolve large questions can lead readers to doubt the success of the research.
Snippets of Effective Discussions:
Consumer-based actions to reduce plastic pollution in rivers: A multi-criteria decision analysis approach
Identifying reliable indicators of fitness in polar bears
- How to Write a Great Title
- How to Write an Abstract
- How to Write Your Methods
- How to Report Statistics
- How to Edit Your Work
The contents of the Peer Review Center are also available as a live, interactive training session, complete with slides, talking points, and activities. …
The contents of the Writing Center are also available as a live, interactive training session, complete with slides, talking points, and activities. …
There’s a lot to consider when deciding where to submit your work. Learn how to choose a journal that will help your study reach its audience, while reflecting your values as a researcher…
The Conclusion: How to End a Scientific Report in Style
- First Online: 26 April 2023
Cite this chapter
- Siew Mei Wu 3 ,
- Kooi Cheng Lee 3 &
- Eric Chun Yong Chan 4
869 Accesses
Sometimes students have the mistaken belief that the conclusion of a scientific report is just a perfunctory ending that repeats what was presented in the main sections of the report. However, impactful conclusions fulfill a rhetorical function. Besides giving a closing summary, the conclusion reflects the significance of what has been uncovered and how this is connected to a broader issue. At the very least, the conclusion of a scientific report should leave the reader with a new perspective of the research area and something to think about.
This is a preview of subscription content, log in via an institution to check access.
Access this chapter
- Available as EPUB and PDF
- Read on any device
- Instant download
- Own it forever
- Durable hardcover edition
- Dispatched in 3 to 5 business days
- Free shipping worldwide - see info
Tax calculation will be finalised at checkout
Purchases are for personal use only
Institutional subscriptions
Similar content being viewed by others
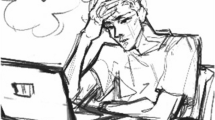
Writing and publishing a scientific paper
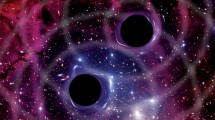
Too good to be true?
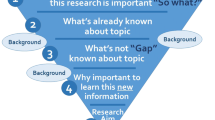
How to Write and Publish a Research Paper for a Peer-Reviewed Journal
Goh, Z.-H., Tee, J. K., & Ho, H. K. (2020). An Evaluation of the in vitro roles and mechanisms of silibinin in reducing pyrazinamide and isoniazid-induced hepatocellular damage. International Journal of Molecular Sciences, 21 , 3714–3734. https://doi.org/10.3390/ijms21103714
Article CAS PubMed PubMed Central Google Scholar
Swales, J. M., & Feak, C. B. (2012). Academic writing for graduate students (3rd ed.). University of Michigan Press.
Book Google Scholar
Download references
Author information
Authors and affiliations.
Centre for English Language Communication, National University of Singapore, Singapore, Singapore
Siew Mei Wu & Kooi Cheng Lee
Pharmacy, National University of Singapore, Singapore, Singapore
Eric Chun Yong Chan
You can also search for this author in PubMed Google Scholar
Corresponding author
Correspondence to Siew Mei Wu .
Editor information
Editors and affiliations.
School of Chemical and Molecular Biosciences, The University of Queensland, Brisbane, QLD, Australia
Susan Rowland
School of Biological Sciences, The University of Queensland, Brisbane, QLD, Australia
Louise Kuchel
Appendix 1: Tutorial Notes for Conclusion Activity
1.1 learning outcomes.
At the end of the tutorial, you should be able to:
Identify and demonstrate understanding of the roles of Conclusion section of research reports
Analyze the rhetorical moves of Conclusion and apply them effectively in research reports
1.2 Introduction
The Conclusion of a paper is a closing summary of what the report is about. The key role of a Conclusion is to provide a reflection on what has been uncovered during the course of the study and to reflect on the significance of what has been learned (Craswell & Poore, 2012). It should show the readers why all the analysis and information matters.
Besides having a final say on the issues in the report, a Conclusion allows the writer to do the following:
Demonstrate the importance of ideas presented through a synthesis of thoughts
Consider broader issues, make new connections, and elaborate on the significance of the findings
Propel the reader to a new view of the subject
Make a good final impression
End the paper on a positive note
(University of North Carolina at Chapel Hill, 2019)
In other words, a Conclusion gives the readers something to take away that will help them see things differently or appreciate the topic in new ways. It can suggest broader implications that will not only interest the readers, but also enrich their knowledge (Craswell & Poore, 2012), and leave them with something interesting to think about (University of North Carolina at Chapel Hill, 2019).
1.3 About the Conclusion Section
In most universities, undergraduate students, especially those in the last year of their programs, are required to document their research work in the form of a research report. The process of taking what you have done in the lab or from systematic review, and writing it for your academic colleagues is a highly structured activity that stretches and challenges the mind. Overall, a research paper should appeal to the academic community for whom you are writing and should cause the reader to want to know more about your research.
As an undergraduate student in your discipline, you have the advantage of being engaged in a niche area of research. As such, your research is current and will most likely be of interest to scholars in your community.
A typical research paper has the following main sections: introduction, methods, results, discussion, and conclusion. The other front and back matters of a research paper are the title, abstract, acknowledgments, and reference list. This structure is commonly adopted and accepted in the scientific fields. The research report starts with a general idea. The report then leads the reader to a discussion on a specific research area. It then ends with applicability to a bigger area. The last section, Conclusion, is the focus of this lesson.
The rhetorical moves of a Conclusion reflect its roles (see Fig. 54.1 ). It starts by reminding the reader of what is presented in the Introduction. For example, if a problem is described in the Introduction, that same problem can be revisited in the Conclusion to provide evidence that the report is helpful in creating a new understanding of the problem. The writer can also refer to the Introduction by using keywords or parallel concepts that were presented there.

Rhetorical moves of Conclusion (the University of North Carolina at Chapel Hill Writing Center,2019)
Next is a synthesis and not a summary of the outcomes of the study. Ideas should not simply be repeated as they were in the earlier parts of the report. The writer must show how the points made, and the support and examples that were given, fit together.
In terms of limitations, if it is not already mentioned in the Discussion section, the writer should acknowledge the weaknesses and shortcomings in the design and/or conduct of the study.
Finally, in connecting to the wider context, the writer should propose a course of action, a solution to an issue, or pose questions for further study. This can redirect readers’ thoughts and help them apply the information and ideas in the study to their own research context or to see the broader implications of the study.
1.4 Linguistic Features of the Conclusion Section
In terms of linguistic features, the use of tense in the Conclusion section is primarily present where the writer’s voice, position, and interpretation are prominent. This is followed by the use of the future tense in sharing what is ahead and some use of past when referring to the study that was done. As summarized by Swales and Feak (2012), Table 54.1 presents the frequency of use of the present tense and past tense in a research report.
1.5 Writing the Conclusion Section
Often, writing a Conclusion is not as easy as it first seems. Using the Question and Answer approach, below is a description of what is usually included in the Conclusion section.
How long should the Conclusion be?
One or two paragraphs comprising 1 sentence summarizing what the paper was about
Two to three sentences summarizing and synthesizing the key findings related to the thesis or objectives of the study
One sentence on limitations (if not in Discussion)
One to two sentences highlighting the significance and implications
One sentence on potential directions for further research
Should the objective be referred to in a Conclusion?
An effective Conclusion reiterates the issue or problem the hypothesis or objective(s) set out to solve. It is important to remind the readers what the hypothesis or objective(s) of the report are and to what extent they are addressed
How far should the Conclusion reflect the Introduction?
Referring to points made in the Introduction in the conclusion ties the paper together and provides readers with a sense of closure.
How much summarizing should there be in a Conclusion?
The conclusion can loosely follow the organization of your paper to parallel, but the focus should be on the paper’s analysis rather than on the organization.
Should newly found information be added to a Conclusion?
Well-written conclusions do not bring in new information or analysis; instead, they sum up what is already contained in the paper.
(Bahamani et al., 2017; Markowsky, 2010)
1.6 Task: Analysing a Conclusion Section
Consider Examples 1 to 4. How do the writers communicate the following information?
Restatement of objective(s)
Refection of outcome(s)
Acknowledgment of limitations, if any
Connection to wider context
“According to this study, the use of educational models, such as a Precaution Adoption Process Model (PAPM) that most people are associated with the process of decision-making in higher education will be beneficial. Moreover, in the preparation, development and implementation of training programs, factors like increased perceived susceptibility, and perceived benefits should be dealt with and some facilities should be provided to facilitate or resolve the barriers of doing the Pap smear test as much as possible.”
(Bahamani et al., 2016)
“Community pharmacists perceived the NMS service as being of benefit to patients by providing advice and reassurance. Implementation of NMS was variable and pharmacists’ perceptions of its feasibility and operationalisation were mixed. Some found the logistics of arranging and conducting the necessary follow-ups challenging, as were service targets. Patient awareness and understanding of NMS was reported to be low and there was a perceived need for publicity about the service. NMS appeared to have strengthened existing good relationships between pharmacists and GPs. Some pharmacists’ concerns about possible overlap of NMS with GP and nurse input may have impacted on their motivation. Overall, our findings indicate that NMS provides an opportunity for patient benefit (patient interaction and medicines management) and the development of contemporary pharmacy practice.”
(Lucas & Blenkinsopp, 2015)
“In this review, we discussed several strategies for the engineering of RiPP pathways to produce artificial pep-tides bearing non-proteinogenic structures characteristic of peptidic natural products. In the RiPP pathways, the structures of the final products are defined by the primary sequences of the precursor genes. Moreover, only a small number of modifying enzymes are involved, and the enzymes function modularly. These features have greatly facilitated both in vivo and in vitro engineering of the pathways, leading to a wide variety of artificial derivatives of naturally occurring RiPPs. In principle, the engineering strategies introduced here can be interchangeably applied for other classes of RiPP enzymes/pathways. Post-biosynthetic chemical modification of RiPPs would be an alternative approach to further increase the structural variation of the products [48–50]. Given that new classes of RiPP enzymes have been frequently reported, and that genetic information of putative RiPP enzymes continues to arise, the array of molecules feasible by RiPP engineering will be further expanded. Some of the artificial RiPP derivatives exhibited elevated bioactivities or different selectivities as compared with their wild type RiPPs. Although these precedents have demonstrated the pharmaceutical relevance of RiPP ana-logs, the next important step in RiPP engineering is the development of novel RiPP derivatives with artificial bioactivities. In more recent reports [51 __,52 __,53 __], the integration of combinatorial lanthipeptide biosynthesis with in vitro selection or bacterial reverse two-hybrid screening methods have successfully obtained artificial ligands specific to certain target proteins. Such approaches, including other strategies under investigation in laboratories in this field, for constructing and screening vast RiPP libraries would lead to the creation of artificial bioactive peptides with non-proteinogenic structures in the near feature.”
(Goto & Suga, 2018)
“Our study is the first to assess and characterise silibinin’s various roles as an adjuvant in protecting against PZA- and INH-induced hepatotoxicity. Most promisingly, we demonstrated silibinin’s safety and efficacy as a rescue adjuvant in vitro , both of which are fundamental considerations in the use of any drug. We also identified silibinin’s potential utility as a rescue hepatoprotectant, shed important mechanistic insights on its hepatoprotective effect, and identified novel antioxidant targets in ameliorating ATT-induced hepatotoxicity. The proof-of-concept demonstrated in this project forms the ethical and scientific foundation to justify and inform subsequent in vivo preclinical studies and clinical trials. Given the lack of alternative treatments in tuberculosis, the need to preserve our remaining antibiotics is paramount. The high stakes involved necessitate future efforts to support our preliminary work in making silibinin clinically relevant to patients and healthcare professionals alike.”
(Goh, 2018)
1.7 In Summary
To recap, in drafting the Conclusion section, you should keep in mind that final remarks can leave the readers with a long-lasting impression of the report especially on the key point(s) that the writer intends to convey. Therefore, you should be careful in crafting this last section of your report.
1.8 References
Bahamani, A. et al. (2017). The Effect of Training Based on Precaution Adoption Process Model (PAPM) on Rural Females’ Participation in Pap smear. BJPR, 16 , 6. Retrieved from http://www.journalrepository.org/media/journals/BJPR_14/2017/May/Bahmani1662017BJPR32965.pdf
Craswell G., & Poore, M. (2012). Writing for Academic Success, 2nd. London: Sage.
Goh, Z-H. (2018). An Evaluation of the Roles and Mechanisms of Silibinin in Reducing Pyrazinamide- and Isoniazid-Induced Hepatotoxicity . Unpublished Final Year Project. National University of Singapore: Department of Pharmacy.
Goto, Y., & Suga, H. (2018). Engineering of RiPP pathways for the production of artificial peptides bearing various non-proteinogenic structures. Current Opinion in Chemical Biology , 46 , 82–90.
Lucas, B., & Blenkinsopp, A. (2015). Community pharmacists’ experience and perceptions of the New Medicines Serves (NMS). IJPP , 23 , 6. Retrieved from http://onlinelibrary.wiley.com/doi/10.1111/ijpp.12180/full
Markowski (2010). WPPD Evaluation form for capstone paper . Retrieved from https://cop-main.sites.medinfo.ufl.edu/files/2010/12/Capstone-Paper-Checklist-and-Reviewer-Evaluation-Form.pdf
Swales, J.M., & Feak, C.B. (2012). Academic writing for graduate students , 3 rd ed. Michigan: University of Michigan Press.
University of North Carolina at Chapel Hill, The Writing Center. (2019). Conclusions . Retrieved from https://writingcenter.unc.edu/tips-and-tools/conclusions/
Appendix 2: Quiz for Conclusion Activity
Instructions
There are 6 questions in this quiz. Choose the most appropriate answer among the options provided.
What does the Conclusion section of a scientific report do?
It provides a recap of report, with reference to the objective(s).
It gives a closure to what has been discussed in relation to the topic.
It shares future direction(s) and in doing so connects to a wider context.
It propels the reader to have an enhanced understanding of the topic.
i, ii, and iii
i, ii and iv
ii, iii and iv
i, ii, iii and iv
The first rhetorical move of the Conclusion section is restatement of objective(s). It …
reminds the reader the objective(s) of the report.
restates reason(s) of each objective of the report.
revisits issue(s) presented requiring investigation.
reiterates the importance of the research project.
The second rhetorical move of the Conclusion section is reflection of outcome(s). It …
summarizes all the findings of the research project.
synthesizes outcomes of the research project.
is a repeat of important ideas mentioned in the report.
shows how key points, evidence, and support fit together.
In connecting to a wider context, the authors …
remind the reader of the importance of the topic.
propose a course of action for the reader.
pose a question to the reader for further research.
direct the reader to certain direction(s).
Following is the Conclusion section of a published article.
“In summary, we have assessed and characterised silibinin’s various roles as an adjuvant in protecting against PZA- and INH-induced hepatotoxicity. Our in vitro experiments suggest that silibinin may be safe and efficacious as a rescue adjuvant, both fundamental considerations in the use of any drug. Further optimisation of our in vitro model may also enhance silibinin’s hepatoprotective effect in rescue, prophylaxis, and recovery. Using this model, we have gleaned important mechanistic insights into its hepatoprotective effect and identified novel antioxidant targets in ameliorating HRZE-induced hepatotoxicity. Future directions will involve exploring the two main mechanisms by which silibinin may ameliorate hepatotoxicity; the proof-of-concept demonstrated in this project will inform subsequent in vitro and in vivo preclinical studies. Given the lack of alternative treatments in tuberculosis, the need to preserve our remaining antibiotics is paramount. These high stakes necessitate future efforts to support our preliminary work, making silibinin more clinically relevant to patients and healthcare professionals alike.” (Goh et al., 2020)
This excerpt of the Conclusion section…
restates objectives of the research.
synthesizes outcomes of the research.
acknowledges limitations of the research
connects the reader to a wider context.
i, ii and iii
What can one observe about the use of tenses in the Conclusion section? The frequency of use of present and future tenses …
demonstrates the importance results being synthesized.
is ungrammatical as the past tense should be used to state the outcomes.
propels the reader to think of future research.
suggests an encouraging tone to end the report.
Rights and permissions
Reprints and permissions
Copyright information
© 2023 The Author(s), under exclusive license to Springer Nature Switzerland AG
About this chapter
Wu, S.M., Lee, K.C., Chan, E.C.Y. (2023). The Conclusion: How to End a Scientific Report in Style. In: Rowland, S., Kuchel, L. (eds) Teaching Science Students to Communicate: A Practical Guide. Springer, Cham. https://doi.org/10.1007/978-3-030-91628-2_54
Download citation
DOI : https://doi.org/10.1007/978-3-030-91628-2_54
Published : 26 April 2023
Publisher Name : Springer, Cham
Print ISBN : 978-3-030-91627-5
Online ISBN : 978-3-030-91628-2
eBook Packages : Biomedical and Life Sciences Biomedical and Life Sciences (R0)
Share this chapter
Anyone you share the following link with will be able to read this content:
Sorry, a shareable link is not currently available for this article.
Provided by the Springer Nature SharedIt content-sharing initiative
- Publish with us
Policies and ethics
- Find a journal
- Track your research
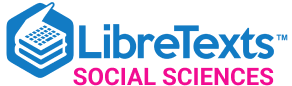
- school Campus Bookshelves
- menu_book Bookshelves
- perm_media Learning Objects
- login Login
- how_to_reg Request Instructor Account
- hub Instructor Commons
Margin Size
- Download Page (PDF)
- Download Full Book (PDF)
- Periodic Table
- Physics Constants
- Scientific Calculator
- Reference & Cite
- Tools expand_more
- Readability
selected template will load here
This action is not available.
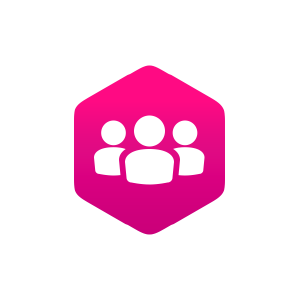
5.1: Key Attributes of a Research Design
- Last updated
- Save as PDF
- Page ID 26232
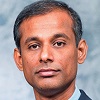
- Anol Bhattacherjee
- University of South Florida via Global Text Project
\( \newcommand{\vecs}[1]{\overset { \scriptstyle \rightharpoonup} {\mathbf{#1}} } \)
\( \newcommand{\vecd}[1]{\overset{-\!-\!\rightharpoonup}{\vphantom{a}\smash {#1}}} \)
\( \newcommand{\id}{\mathrm{id}}\) \( \newcommand{\Span}{\mathrm{span}}\)
( \newcommand{\kernel}{\mathrm{null}\,}\) \( \newcommand{\range}{\mathrm{range}\,}\)
\( \newcommand{\RealPart}{\mathrm{Re}}\) \( \newcommand{\ImaginaryPart}{\mathrm{Im}}\)
\( \newcommand{\Argument}{\mathrm{Arg}}\) \( \newcommand{\norm}[1]{\| #1 \|}\)
\( \newcommand{\inner}[2]{\langle #1, #2 \rangle}\)
\( \newcommand{\Span}{\mathrm{span}}\)
\( \newcommand{\id}{\mathrm{id}}\)
\( \newcommand{\kernel}{\mathrm{null}\,}\)
\( \newcommand{\range}{\mathrm{range}\,}\)
\( \newcommand{\RealPart}{\mathrm{Re}}\)
\( \newcommand{\ImaginaryPart}{\mathrm{Im}}\)
\( \newcommand{\Argument}{\mathrm{Arg}}\)
\( \newcommand{\norm}[1]{\| #1 \|}\)
\( \newcommand{\Span}{\mathrm{span}}\) \( \newcommand{\AA}{\unicode[.8,0]{x212B}}\)
\( \newcommand{\vectorA}[1]{\vec{#1}} % arrow\)
\( \newcommand{\vectorAt}[1]{\vec{\text{#1}}} % arrow\)
\( \newcommand{\vectorB}[1]{\overset { \scriptstyle \rightharpoonup} {\mathbf{#1}} } \)
\( \newcommand{\vectorC}[1]{\textbf{#1}} \)
\( \newcommand{\vectorD}[1]{\overrightarrow{#1}} \)
\( \newcommand{\vectorDt}[1]{\overrightarrow{\text{#1}}} \)
\( \newcommand{\vectE}[1]{\overset{-\!-\!\rightharpoonup}{\vphantom{a}\smash{\mathbf {#1}}}} \)
The quality of research designs can be defined in terms of four key design attributes: internal validity, external validity, construct validity, and statistical conclusion validity.
Internal validity , also called causality, examines whether the observed change in a dependent variable is indeed caused by a corresponding change in hypothesized independent variable, and not by variables extraneous to the research context. Causality requires three conditions: (1) covariation of cause and effect (i.e., if cause happens, then effect also happens; and if cause does not happen, effect does not happen), (2) temporal precedence: cause must precede effect in time, (3) no plausible alternative explanation (or spurious correlation). Certain research designs, such as laboratory experiments, are strong in internal validity by virtue of their ability to manipulate the independent variable (cause) via a treatment and observe the effect (dependent variable) of that treatment after a certain point in time, while controlling for the effects of extraneous variables. Other designs, such as field surveys, are poor in internal validity because of their inability to manipulate the independent variable (cause), and because cause and effect are measured at the same point in time which defeats temporal precedence making it equally likely that the expected effect might have influenced the expected cause rather than the reverse. Although higher in internal validity compared to other methods, laboratory experiments are, by no means, immune to threats of internal validity, and are susceptible to history, testing, instrumentation, regression, and other threats that are discussed later in the chapter on experimental designs. Nonetheless, different research designs vary considerably in their respective level of internal validity.
External validity or generalizability refers to whether the observed associations can be generalized from the sample to the population (population validity), or to other people, organizations, contexts, or time (ecological validity). For instance, can results drawn from a sample of financial firms in the United States be generalized to the population of financial firms (population validity) or to other firms within the United States (ecological validity)? Survey research, where data is sourced from a wide variety of individuals, firms, or other units of analysis, tends to have broader generalizability than laboratory experiments where artificially contrived treatments and strong control over extraneous variables render the findings less generalizable to real-life settings where treatments and extraneous variables cannot be controlled. The variation in internal and external validity for a wide range of research designs are shown in Figure 5.1.
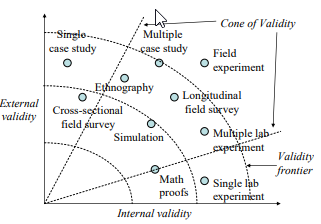
Some researchers claim that there is a tradeoff between internal and external validity: higher external validity can come only at the cost of internal validity and vice-versa. But this is not always the case. Research designs such as field experiments, longitudinal field surveys, and multiple case studies have higher degrees of both internal and external validities. Personally, I prefer research designs that have reasonable degrees of both internal and external validities, i.e., those that fall within the cone of validity shown in Figure 5.1. But this should not suggest that designs outside this cone are any less useful or valuable. Researchers’ choice of designs is ultimately a matter of their personal preference and competence, and the level of internal and external validity they desire.
Construct validity examines how well a given measurement scale is measuring the theoretical construct that it is expected to measure. Many constructs used in social science research such as empathy, resistance to change, and organizational learning are difficult to define, much less measure. For instance, construct validity must assure that a measure of empathy is indeed measuring empathy and not compassion, which may be difficult since these constructs are somewhat similar in meaning. Construct validity is assessed in positivist research based on correlational or factor analysis of pilot test data, as described in the next chapter.
Statistical conclusion validity examines the extent to which conclusions derived using a statistical procedure is valid. For example, it examines whether the right statistical method was used for hypotheses testing, whether the variables used meet the assumptions of that statistical test (such as sample size or distributional requirements), and so forth. Because interpretive research designs do not employ statistical test, statistical conclusion validity is not applicable for such analysis. The different kinds of validity and where they exist at the theoretical/empirical levels are illustrated in Figure 5.2.
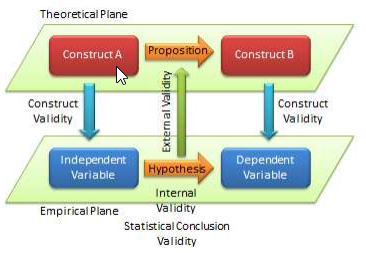
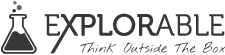
- Foundations
- Write Paper
Search form
- Experiments
- Anthropology
- Self-Esteem
- Social Anxiety
- Statistics >
Relationship Between Variables
It is very important to understand relationship between variables to draw the right conclusion from a statistical analysis. The relationship between variables determines how the right conclusions are reached. Without an understanding of this, you can fall into many pitfalls that accompany statistical analysis and infer wrong results from your data.
This article is a part of the guide:
- Correlation
- Two-Way ANOVA
- Multiple Regression
- One-Way ANOVA
Browse Full Outline
- 1 Statistical Hypothesis Testing
- 2.1 Linear Relationship
- 2.2 Non-Linear Relationship
- 3.1 Pearson Product-Moment Correlation
- 3.2 Spearman rho
- 3.3 Partial Correlation
- 3.4 Correlation and Causation
- 4.1 Linear Regression
- 4.2 Multiple Regression
- 4.3 Correlation and Regression
- 5.1 Independent One-Sample T-Test
- 5.2 Independent Two-Sample T-Test
- 5.3 Dependent T-Test for Paired Samples
- 5.4 Another article on Student’s T-Test
- 6.1 One-Way ANOVA
- 6.2 Two-Way ANOVA
- 6.3 Factorial ANOVA
- 6.4 Repeated Measures ANOVA
- 7.1 Cohen’s Kappa
- 7.2 Mann-Whitney U-Test
- 7.3 Wilcoxon Signed Rank Test
- 8.1 Chi Square Test
- 8.4 Factor Analysis
- 8.5 ROC Curve Analysis
- 8.6 Meta Analysis
There are several different kinds of relationships between variables . Before drawing a conclusion , you should first understand how one variable changes with the other. This means you need to establish how the variables are related - is the relationship linear or quadratic or inverse or logarithmic or something else?
Suppose you measure a volume of a gas in a cylinder and measure its pressure. Now you start compressing the gas by pushing a piston all while maintaining the gas at the room temperature. The volume of gas decreases while the pressure increases. You note down different values on a graph paper.
If you take enough measurements, you can see a shape of a parabola defined by xy=constant. This is because gases follow Boyle's law that says when temperature is constant, PV = constant. Here, by taking data you are relating the pressure of the gas with its volume. Similarly, many relationships are linear in nature.
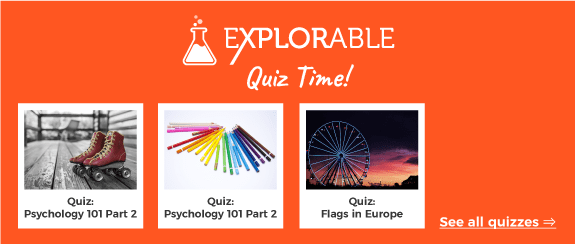
Relationships in Physical and Social Sciences
Relationships between variables need to be studied and analyzed before drawing conclusions based on it. In natural science and engineering, this is usually more straightforward as you can keep all parameters except one constant and study how this one parameter affects the result under study.
However, in social sciences, things get much more complicated because parameters may or may not be directly related. There could be a number of indirect consequences and deducing cause and effect can be challenging.
Only when the change in one variable actually causes the change in another parameter is there a causal relationship. Otherwise, it is simply a correlation . Correlation doesn't imply causation . There are ample examples and various types of fallacies in use.
A famous example to prove the point: Increased ice-cream sales shows a strong correlation to deaths by drowning. It would obviously be wrong to conclude that consuming ice-creams causes drowning. The explanation is that more ice-cream gets sold in the summer, when more people go to the beach and other water bodies and therefore increased deaths by drowning.
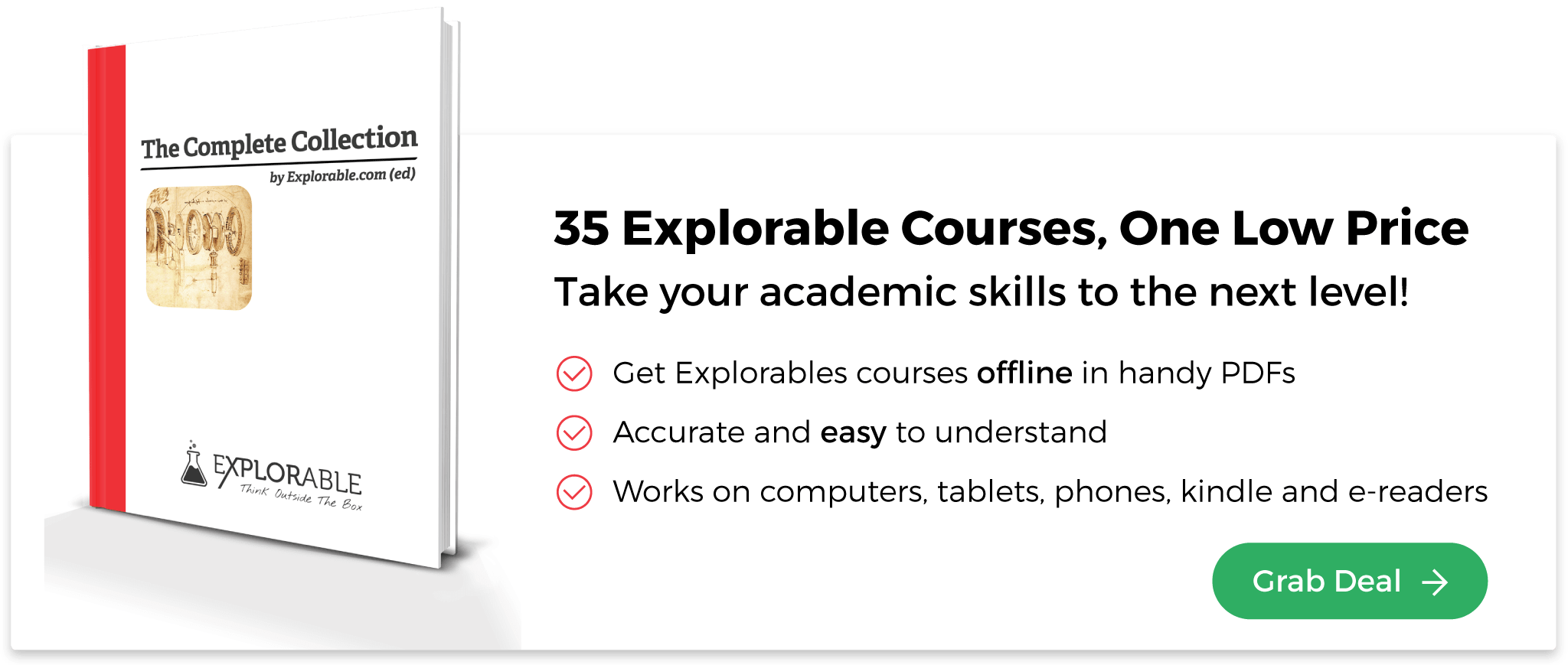
Positive and Negative Correlation
Correlation between variables can be positive or negative. Positive correlation implies an increase of one quantity causes an increase in the other whereas in negative correlation, an increase in one variable will cause a decrease in the other.
It is important to understand the relationship between variables to draw the right conclusions. Even the best scientists can get this wrong and there are several instances of how studies get correlation and causation mixed up.
- Psychology 101
- Flags and Countries
- Capitals and Countries
Siddharth Kalla (Jul 26, 2011). Relationship Between Variables. Retrieved May 22, 2024 from Explorable.com: https://explorable.com/relationship-between-variables
You Are Allowed To Copy The Text
The text in this article is licensed under the Creative Commons-License Attribution 4.0 International (CC BY 4.0) .
This means you're free to copy, share and adapt any parts (or all) of the text in the article, as long as you give appropriate credit and provide a link/reference to this page.
That is it. You don't need our permission to copy the article; just include a link/reference back to this page. You can use it freely (with some kind of link), and we're also okay with people reprinting in publications like books, blogs, newsletters, course-material, papers, wikipedia and presentations (with clear attribution).
Want to stay up to date? Follow us!
Save this course for later.
Don't have time for it all now? No problem, save it as a course and come back to it later.
Footer bottom
- Privacy Policy
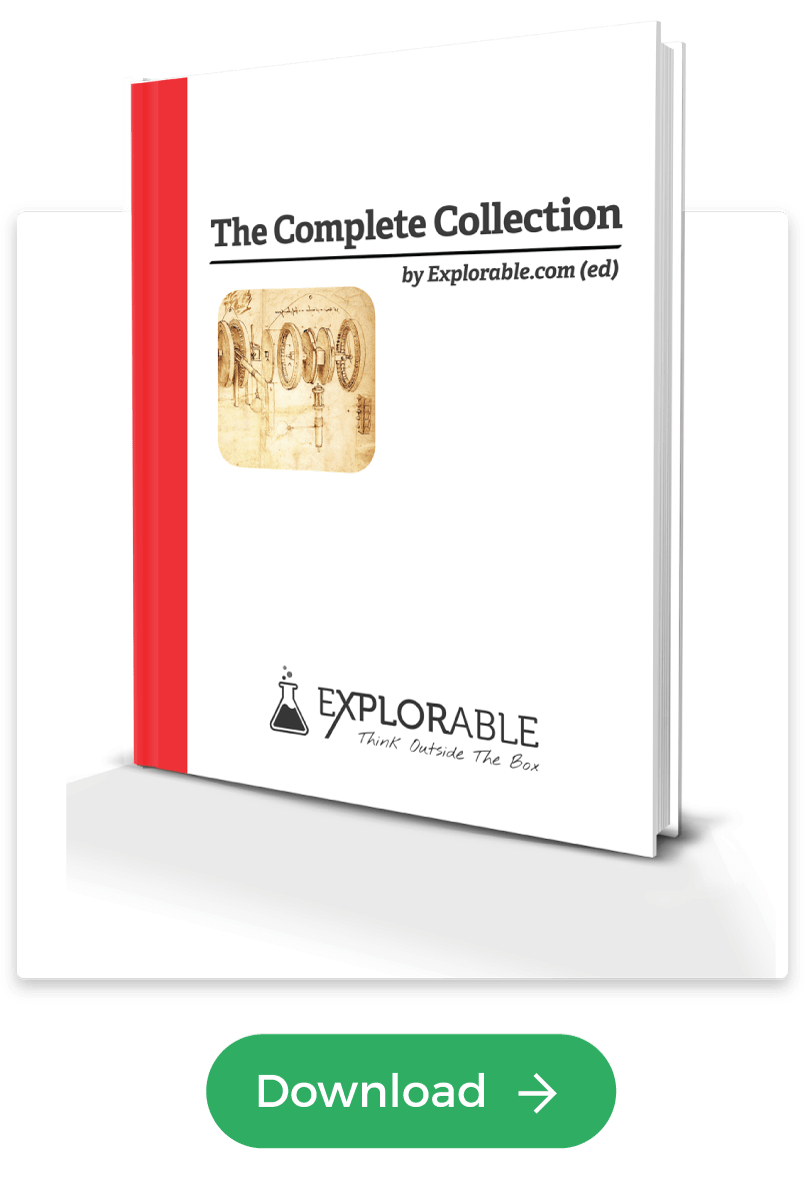
- Subscribe to our RSS Feed
- Like us on Facebook
- Follow us on Twitter
Academia.edu no longer supports Internet Explorer.
To browse Academia.edu and the wider internet faster and more securely, please take a few seconds to upgrade your browser .
Enter the email address you signed up with and we'll email you a reset link.
- We're Hiring!
- Help Center
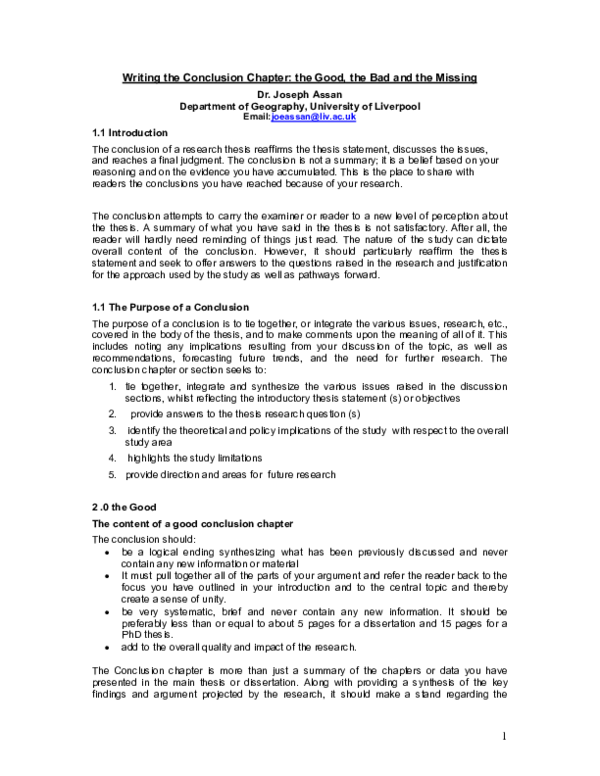
Writing the Conclusion Chapter: the Good, the Bad and the Missing

Related Papers
Dr. Khalil A. Agha
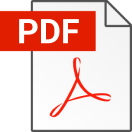
Writing a succesful a cademic thesis
Raphael Akeyo
This article is a brief guidance on effective writing of academic research thesis with a focus on the results/ findings section/ chapters. It provides step by step highlights on how to present data from the field, interpretation of the findings, corroborating the findings with existing studies as well as the use of theoretical tenets to discuss the findings. The conclusions and recommendations sections are also highlighted.
Journal of English for Academic Purposes
Nasrin Nejad
This paper considers the generic structure of Conclusion chapters in PhD theses or dissertations. From a corpus of 45 PhD theses covering a range of disciplines, chapters playing a concluding role were identified and analysed for their functional moves and steps. Most Conclusions were found to restate purpose, consolidate research space with a varied array of steps, recommend future research and cover practical applications, implications or recommendations. However a minority were found to focus more on the field than on the thesis itself. These field-oriented Conclusions tended to adopt a problem–solution text structure, or in one case, an argument structure. Variations in focus and structure between disciplines were also found.
Scientific Research Publishing: Creative Education
Dr. Qais Faryadi
I have already discussed the PhD introduction and literature review in detail. In this paper, I discuss the PhD methodology, results and how to write a stunning conclusion for your thesis. The main objective of this paper is to help PhD candidates to understand what is a PhD methodology and guide them in writing a systematic and meaningful PhD methodology, results and conclusion. The methodology used in this research is a descriptive method as it deliberates and defines the various parts of PhD methodology, results and conclusion writing process and elucidates the "how to do" in a very unpretentious and understanding manner. As thus, this paper summarises the various steps of thesis methodology, results and conclusion writing to pilot the PhD students. This road map is a useful guidance especially for students of social science studies. Additionally, in this paper, methodology writing techniques , procedures and important strategies are enlightened in a simple manner. This paper adopts a "how-to approach" when discussing a variety of relevant topics such as introduction, formulation of the methodology, variables , research design process, types of sampling, data collection process, interviews, questionnaires, data analysis techniques and so on. Results and conclusions are also discussed in detail, so that PhD candidates can follow the guide clearly. This paper has 5 parts such as Introduction, Literature reviews, Methodology, Results and Conclusion. As such, I discuss Methodology, Results and Conclusion as the final assessment of the PhD thesis writing process.
Pamela Olmos
"The conclusions section of a thesis is the last chapter people read and usually the section that leaves the lasting impression. This study presents a framework for the analysis of thesis conclusions at an undergraduate level in the field of humanities, which –as the literature reveals–, lacks an agenda for its analysis at the undergraduate level. A sevenmove generic organization is proposed as a Framework for Undergraduate Thesis Conclusions (FUTC). This framework sheds a light on the complex construction of the thesis conclusions chapter towards its analysis. Moreover, the FUTC shows potentiality for further research, pedagogic implications and applications for genre and writing studies. Moreover, the FUTC shows potentiality for further research, pedagogic implications and applications for genre and writing studies."
Vernon Trafford , Prof Shosh Leshem
This study investigated how candidates claimed to have made an original contribution to knowledge in the conclusion chapters of 100 PhD theses. Documentary analysis was used to discover how this was explained within theses at selected universities in three countries. No other documents were accessed and neither were candidates, supervisors or examiners contacted. The evidence showed that the function of Discussion and Conclusion chapters was interpreted differently between disciplines and national academic traditions. The relative size of conclusion chapters to other chapters was consistently small. Explicit claims for originality and contributing to knowledge appeared in 54 per cent of theses thus meeting their universities' stated criteria for PhD awards but were not adequately explained in 46 per cent of theses. Introduction As doctoral supervisors and examiners we have recognised an absence of research-based literature on the chapter of conclusions in doctoral theses. Thus, ...
Phuc.CX4074 2004074
Kofi Amissah
Chapter by chapter summary of my PhD thesis
Informal Channels for Conflict Resolution in Ibadan, Nigeria
Wuyi Omitoogun
Theo Lieven
This chapter summarizes the results of the preceding chapters, briefly discusses their implications, and concludes the book.
RELATED PAPERS
Call/Wa : 0812 1776 0588 | Tongkat Komando Di Kabupaten Konawe Utara
0812 1776 0588 Produsen Tongkat Komando Indonesia
Ingrid Glorie
Molecular Crystals and Liquid Crystals
Operators and Matrices
Jadranka Hot
Revista Brasileira de Enfermagem
Marta Lenise Prado
refdi mandjurungi
Tiến Đức Triệu
Le Centre pour la Communication Scientifique Directe - HAL - Inria
Clémentine Raffy
Eugenio Miranda Sperandio
Roman Voliansky
International Letters of Chemistry, Physics and Astronomy
PROFESOR MADYA TS DR ZETY SHARIZAT HAMIDI
Larry Smith
Biophysical Journal
nguyen linh
isabelle Ville
Academia Environmental Sciences and Sustainability
Milton Speer
Nurfaikah Ratna sari
Experimental Dermatology
vanyo mitev
hbgjfgf hyetgwerf
Health Sciences and Disease
Kathleen Blackett
Patient Experience Journal
Julia Corcoran
RELATED TOPICS
- We're Hiring!
- Help Center
- Find new research papers in:
- Health Sciences
- Earth Sciences
- Cognitive Science
- Mathematics
- Computer Science
- Academia ©2024
- Privacy Policy

Home » Variables in Research – Definition, Types and Examples
Variables in Research – Definition, Types and Examples
Table of Contents

Variables in Research
Definition:
In Research, Variables refer to characteristics or attributes that can be measured, manipulated, or controlled. They are the factors that researchers observe or manipulate to understand the relationship between them and the outcomes of interest.
Types of Variables in Research
Types of Variables in Research are as follows:
Independent Variable
This is the variable that is manipulated by the researcher. It is also known as the predictor variable, as it is used to predict changes in the dependent variable. Examples of independent variables include age, gender, dosage, and treatment type.
Dependent Variable
This is the variable that is measured or observed to determine the effects of the independent variable. It is also known as the outcome variable, as it is the variable that is affected by the independent variable. Examples of dependent variables include blood pressure, test scores, and reaction time.
Confounding Variable
This is a variable that can affect the relationship between the independent variable and the dependent variable. It is a variable that is not being studied but could impact the results of the study. For example, in a study on the effects of a new drug on a disease, a confounding variable could be the patient’s age, as older patients may have more severe symptoms.
Mediating Variable
This is a variable that explains the relationship between the independent variable and the dependent variable. It is a variable that comes in between the independent and dependent variables and is affected by the independent variable, which then affects the dependent variable. For example, in a study on the relationship between exercise and weight loss, the mediating variable could be metabolism, as exercise can increase metabolism, which can then lead to weight loss.
Moderator Variable
This is a variable that affects the strength or direction of the relationship between the independent variable and the dependent variable. It is a variable that influences the effect of the independent variable on the dependent variable. For example, in a study on the effects of caffeine on cognitive performance, the moderator variable could be age, as older adults may be more sensitive to the effects of caffeine than younger adults.
Control Variable
This is a variable that is held constant or controlled by the researcher to ensure that it does not affect the relationship between the independent variable and the dependent variable. Control variables are important to ensure that any observed effects are due to the independent variable and not to other factors. For example, in a study on the effects of a new teaching method on student performance, the control variables could include class size, teacher experience, and student demographics.
Continuous Variable
This is a variable that can take on any value within a certain range. Continuous variables can be measured on a scale and are often used in statistical analyses. Examples of continuous variables include height, weight, and temperature.
Categorical Variable
This is a variable that can take on a limited number of values or categories. Categorical variables can be nominal or ordinal. Nominal variables have no inherent order, while ordinal variables have a natural order. Examples of categorical variables include gender, race, and educational level.
Discrete Variable
This is a variable that can only take on specific values. Discrete variables are often used in counting or frequency analyses. Examples of discrete variables include the number of siblings a person has, the number of times a person exercises in a week, and the number of students in a classroom.
Dummy Variable
This is a variable that takes on only two values, typically 0 and 1, and is used to represent categorical variables in statistical analyses. Dummy variables are often used when a categorical variable cannot be used directly in an analysis. For example, in a study on the effects of gender on income, a dummy variable could be created, with 0 representing female and 1 representing male.
Extraneous Variable
This is a variable that has no relationship with the independent or dependent variable but can affect the outcome of the study. Extraneous variables can lead to erroneous conclusions and can be controlled through random assignment or statistical techniques.
Latent Variable
This is a variable that cannot be directly observed or measured, but is inferred from other variables. Latent variables are often used in psychological or social research to represent constructs such as personality traits, attitudes, or beliefs.
Moderator-mediator Variable
This is a variable that acts both as a moderator and a mediator. It can moderate the relationship between the independent and dependent variables and also mediate the relationship between the independent and dependent variables. Moderator-mediator variables are often used in complex statistical analyses.
Variables Analysis Methods
There are different methods to analyze variables in research, including:
- Descriptive statistics: This involves analyzing and summarizing data using measures such as mean, median, mode, range, standard deviation, and frequency distribution. Descriptive statistics are useful for understanding the basic characteristics of a data set.
- Inferential statistics : This involves making inferences about a population based on sample data. Inferential statistics use techniques such as hypothesis testing, confidence intervals, and regression analysis to draw conclusions from data.
- Correlation analysis: This involves examining the relationship between two or more variables. Correlation analysis can determine the strength and direction of the relationship between variables, and can be used to make predictions about future outcomes.
- Regression analysis: This involves examining the relationship between an independent variable and a dependent variable. Regression analysis can be used to predict the value of the dependent variable based on the value of the independent variable, and can also determine the significance of the relationship between the two variables.
- Factor analysis: This involves identifying patterns and relationships among a large number of variables. Factor analysis can be used to reduce the complexity of a data set and identify underlying factors or dimensions.
- Cluster analysis: This involves grouping data into clusters based on similarities between variables. Cluster analysis can be used to identify patterns or segments within a data set, and can be useful for market segmentation or customer profiling.
- Multivariate analysis : This involves analyzing multiple variables simultaneously. Multivariate analysis can be used to understand complex relationships between variables, and can be useful in fields such as social science, finance, and marketing.
Examples of Variables
- Age : This is a continuous variable that represents the age of an individual in years.
- Gender : This is a categorical variable that represents the biological sex of an individual and can take on values such as male and female.
- Education level: This is a categorical variable that represents the level of education completed by an individual and can take on values such as high school, college, and graduate school.
- Income : This is a continuous variable that represents the amount of money earned by an individual in a year.
- Weight : This is a continuous variable that represents the weight of an individual in kilograms or pounds.
- Ethnicity : This is a categorical variable that represents the ethnic background of an individual and can take on values such as Hispanic, African American, and Asian.
- Time spent on social media : This is a continuous variable that represents the amount of time an individual spends on social media in minutes or hours per day.
- Marital status: This is a categorical variable that represents the marital status of an individual and can take on values such as married, divorced, and single.
- Blood pressure : This is a continuous variable that represents the force of blood against the walls of arteries in millimeters of mercury.
- Job satisfaction : This is a continuous variable that represents an individual’s level of satisfaction with their job and can be measured using a Likert scale.
Applications of Variables
Variables are used in many different applications across various fields. Here are some examples:
- Scientific research: Variables are used in scientific research to understand the relationships between different factors and to make predictions about future outcomes. For example, scientists may study the effects of different variables on plant growth or the impact of environmental factors on animal behavior.
- Business and marketing: Variables are used in business and marketing to understand customer behavior and to make decisions about product development and marketing strategies. For example, businesses may study variables such as consumer preferences, spending habits, and market trends to identify opportunities for growth.
- Healthcare : Variables are used in healthcare to monitor patient health and to make treatment decisions. For example, doctors may use variables such as blood pressure, heart rate, and cholesterol levels to diagnose and treat cardiovascular disease.
- Education : Variables are used in education to measure student performance and to evaluate the effectiveness of teaching strategies. For example, teachers may use variables such as test scores, attendance, and class participation to assess student learning.
- Social sciences : Variables are used in social sciences to study human behavior and to understand the factors that influence social interactions. For example, sociologists may study variables such as income, education level, and family structure to examine patterns of social inequality.
Purpose of Variables
Variables serve several purposes in research, including:
- To provide a way of measuring and quantifying concepts: Variables help researchers measure and quantify abstract concepts such as attitudes, behaviors, and perceptions. By assigning numerical values to these concepts, researchers can analyze and compare data to draw meaningful conclusions.
- To help explain relationships between different factors: Variables help researchers identify and explain relationships between different factors. By analyzing how changes in one variable affect another variable, researchers can gain insight into the complex interplay between different factors.
- To make predictions about future outcomes : Variables help researchers make predictions about future outcomes based on past observations. By analyzing patterns and relationships between different variables, researchers can make informed predictions about how different factors may affect future outcomes.
- To test hypotheses: Variables help researchers test hypotheses and theories. By collecting and analyzing data on different variables, researchers can test whether their predictions are accurate and whether their hypotheses are supported by the evidence.
Characteristics of Variables
Characteristics of Variables are as follows:
- Measurement : Variables can be measured using different scales, such as nominal, ordinal, interval, or ratio scales. The scale used to measure a variable can affect the type of statistical analysis that can be applied.
- Range : Variables have a range of values that they can take on. The range can be finite, such as the number of students in a class, or infinite, such as the range of possible values for a continuous variable like temperature.
- Variability : Variables can have different levels of variability, which refers to the degree to which the values of the variable differ from each other. Highly variable variables have a wide range of values, while low variability variables have values that are more similar to each other.
- Validity and reliability : Variables should be both valid and reliable to ensure accurate and consistent measurement. Validity refers to the extent to which a variable measures what it is intended to measure, while reliability refers to the consistency of the measurement over time.
- Directionality: Some variables have directionality, meaning that the relationship between the variables is not symmetrical. For example, in a study of the relationship between smoking and lung cancer, smoking is the independent variable and lung cancer is the dependent variable.
Advantages of Variables
Here are some of the advantages of using variables in research:
- Control : Variables allow researchers to control the effects of external factors that could influence the outcome of the study. By manipulating and controlling variables, researchers can isolate the effects of specific factors and measure their impact on the outcome.
- Replicability : Variables make it possible for other researchers to replicate the study and test its findings. By defining and measuring variables consistently, other researchers can conduct similar studies to validate the original findings.
- Accuracy : Variables make it possible to measure phenomena accurately and objectively. By defining and measuring variables precisely, researchers can reduce bias and increase the accuracy of their findings.
- Generalizability : Variables allow researchers to generalize their findings to larger populations. By selecting variables that are representative of the population, researchers can draw conclusions that are applicable to a broader range of individuals.
- Clarity : Variables help researchers to communicate their findings more clearly and effectively. By defining and categorizing variables, researchers can organize and present their findings in a way that is easily understandable to others.
Disadvantages of Variables
Here are some of the main disadvantages of using variables in research:
- Simplification : Variables may oversimplify the complexity of real-world phenomena. By breaking down a phenomenon into variables, researchers may lose important information and context, which can affect the accuracy and generalizability of their findings.
- Measurement error : Variables rely on accurate and precise measurement, and measurement error can affect the reliability and validity of research findings. The use of subjective or poorly defined variables can also introduce measurement error into the study.
- Confounding variables : Confounding variables are factors that are not measured but that affect the relationship between the variables of interest. If confounding variables are not accounted for, they can distort or obscure the relationship between the variables of interest.
- Limited scope: Variables are defined by the researcher, and the scope of the study is therefore limited by the researcher’s choice of variables. This can lead to a narrow focus that overlooks important aspects of the phenomenon being studied.
- Ethical concerns: The selection and measurement of variables may raise ethical concerns, especially in studies involving human subjects. For example, using variables that are related to sensitive topics, such as race or sexuality, may raise concerns about privacy and discrimination.
About the author
Muhammad Hassan
Researcher, Academic Writer, Web developer
You may also like

Control Variable – Definition, Types and Examples

Moderating Variable – Definition, Analysis...

Categorical Variable – Definition, Types and...

Independent Variable – Definition, Types and...

Ratio Variable – Definition, Purpose and Examples

Ordinal Variable – Definition, Purpose and...
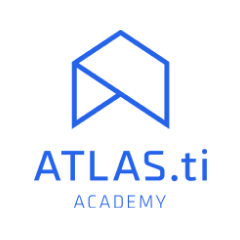
Variables in Research | Types, Definiton & Examples
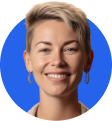
Introduction
What is a variable, what are the 5 types of variables in research, other variables in research.
Variables are fundamental components of research that allow for the measurement and analysis of data. They can be defined as characteristics or properties that can take on different values. In research design , understanding the types of variables and their roles is crucial for developing hypotheses , designing methods , and interpreting results .
This article outlines the the types of variables in research, including their definitions and examples, to provide a clear understanding of their use and significance in research studies. By categorizing variables into distinct groups based on their roles in research, their types of data, and their relationships with other variables, researchers can more effectively structure their studies and achieve more accurate conclusions.
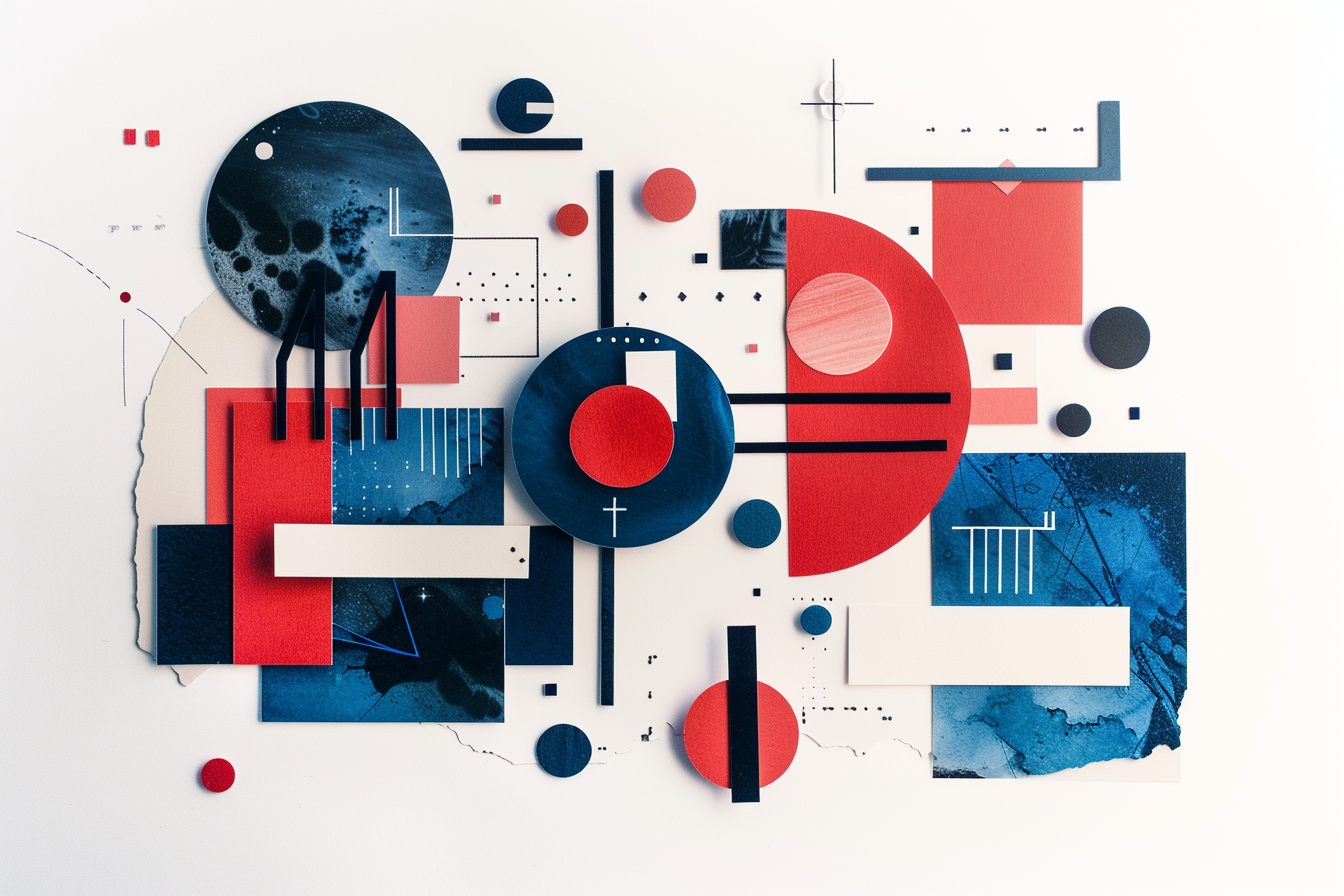
A variable represents any characteristic, number, or quantity that can be measured or quantified. The term encompasses anything that can vary or change, ranging from simple concepts like age and height to more complex ones like satisfaction levels or economic status. Variables are essential in research as they are the foundational elements that researchers manipulate, measure, or control to gain insights into relationships, causes, and effects within their studies. They enable the framing of research questions, the formulation of hypotheses, and the interpretation of results.
Variables can be categorized based on their role in the study (such as independent and dependent variables ), the type of data they represent (quantitative or categorical), and their relationship to other variables (like confounding or control variables). Understanding what constitutes a variable and the various variable types available is a critical step in designing robust and meaningful research.
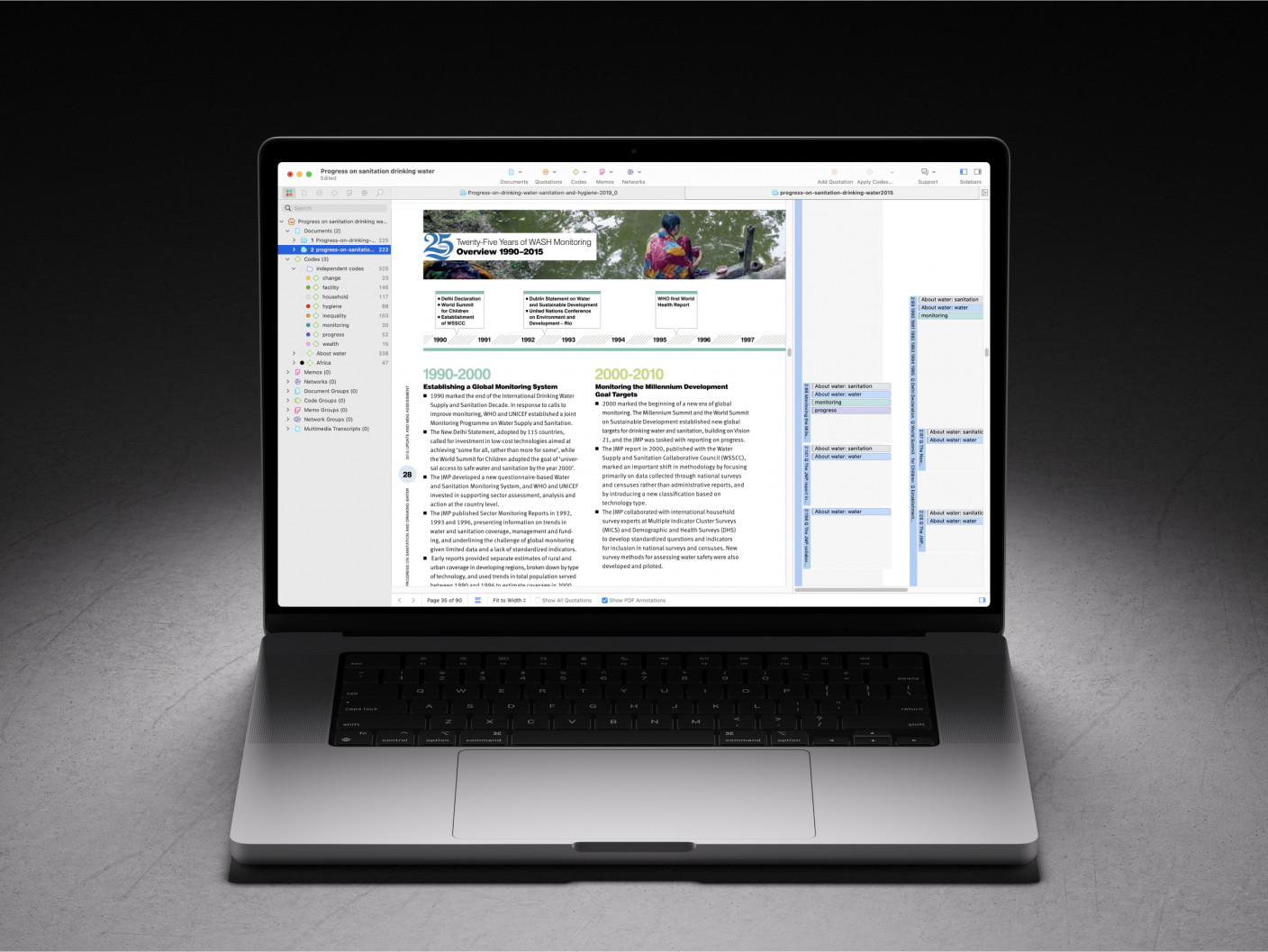
ATLAS.ti makes complex data easy to understand
Turn to our powerful data analysis tools to make the most of your research. Get started with a free trial.
Variables are crucial components in research, serving as the foundation for data collection , analysis , and interpretation . They are attributes or characteristics that can vary among subjects or over time, and understanding their types is essential for any study. Variables can be broadly classified into five main types, each with its distinct characteristics and roles within research.
This classification helps researchers in designing their studies, choosing appropriate measurement techniques, and analyzing their results accurately. The five types of variables include independent variables, dependent variables, categorical variables, continuous variables, and confounding variables. These categories not only facilitate a clearer understanding of the data but also guide the formulation of hypotheses and research methodologies.
Independent variables
Independent variables are foundational to the structure of research, serving as the factors or conditions that researchers manipulate or vary to observe their effects on dependent variables. These variables are considered "independent" because their variation does not depend on other variables within the study. Instead, they are the cause or stimulus that directly influences the outcomes being measured. For example, in an experiment to assess the effectiveness of a new teaching method on student performance, the teaching method applied (traditional vs. innovative) would be the independent variable.
The selection of an independent variable is a critical step in research design, as it directly correlates with the study's objective to determine causality or association. Researchers must clearly define and control these variables to ensure that observed changes in the dependent variable can be attributed to variations in the independent variable, thereby affirming the reliability of the results. In experimental research, the independent variable is what differentiates the control group from the experimental group, thereby setting the stage for meaningful comparison and analysis.
Dependent variables
Dependent variables are the outcomes or effects that researchers aim to explore and understand in their studies. These variables are called "dependent" because their values depend on the changes or variations of the independent variables.
Essentially, they are the responses or results that are measured to assess the impact of the independent variable's manipulation. For instance, in a study investigating the effect of exercise on weight loss, the amount of weight lost would be considered the dependent variable, as it depends on the exercise regimen (the independent variable).
The identification and measurement of the dependent variable are crucial for testing the hypothesis and drawing conclusions from the research. It allows researchers to quantify the effect of the independent variable , providing evidence for causal relationships or associations. In experimental settings, the dependent variable is what is being tested and measured across different groups or conditions, enabling researchers to assess the efficacy or impact of the independent variable's variation.
To ensure accuracy and reliability, the dependent variable must be defined clearly and measured consistently across all participants or observations. This consistency helps in reducing measurement errors and increases the validity of the research findings. By carefully analyzing the dependent variables, researchers can derive meaningful insights from their studies, contributing to the broader knowledge in their field.
Categorical variables
Categorical variables, also known as qualitative variables, represent types or categories that are used to group observations. These variables divide data into distinct groups or categories that lack a numerical value but hold significant meaning in research. Examples of categorical variables include gender (male, female, other), type of vehicle (car, truck, motorcycle), or marital status (single, married, divorced). These categories help researchers organize data into groups for comparison and analysis.
Categorical variables can be further classified into two subtypes: nominal and ordinal. Nominal variables are categories without any inherent order or ranking among them, such as blood type or ethnicity. Ordinal variables, on the other hand, imply a sort of ranking or order among the categories, like levels of satisfaction (high, medium, low) or education level (high school, bachelor's, master's, doctorate).
Understanding and identifying categorical variables is crucial in research as it influences the choice of statistical analysis methods. Since these variables represent categories without numerical significance, researchers employ specific statistical tests designed for a nominal or ordinal variable to draw meaningful conclusions. Properly classifying and analyzing categorical variables allow for the exploration of relationships between different groups within the study, shedding light on patterns and trends that might not be evident with numerical data alone.

Continuous variables
Continuous variables are quantitative variables that can take an infinite number of values within a given range. These variables are measured along a continuum and can represent very precise measurements. Examples of continuous variables include height, weight, temperature, and time. Because they can assume any value within a range, continuous variables allow for detailed analysis and a high degree of accuracy in research findings.
The ability to measure continuous variables at very fine scales makes them invaluable for many types of research, particularly in the natural and social sciences. For instance, in a study examining the effect of temperature on plant growth, temperature would be considered a continuous variable since it can vary across a wide spectrum and be measured to several decimal places.
When dealing with continuous variables, researchers often use methods incorporating a particular statistical test to accommodate a wide range of data points and the potential for infinite divisibility. This includes various forms of regression analysis, correlation, and other techniques suited for modeling and analyzing nuanced relationships between variables. The precision of continuous variables enhances the researcher's ability to detect patterns, trends, and causal relationships within the data, contributing to more robust and detailed conclusions.
Confounding variables
Confounding variables are those that can cause a false association between the independent and dependent variables, potentially leading to incorrect conclusions about the relationship being studied. These are extraneous variables that were not considered in the study design but can influence both the supposed cause and effect, creating a misleading correlation.
Identifying and controlling for a confounding variable is crucial in research to ensure the validity of the findings. This can be achieved through various methods, including randomization, stratification, and statistical control. Randomization helps to evenly distribute confounding variables across study groups, reducing their potential impact. Stratification involves analyzing the data within strata or layers that share common characteristics of the confounder. Statistical control allows researchers to adjust for the effects of confounders in the analysis phase.
Properly addressing confounding variables strengthens the credibility of research outcomes by clarifying the direct relationship between the dependent and independent variables, thus providing more accurate and reliable results.
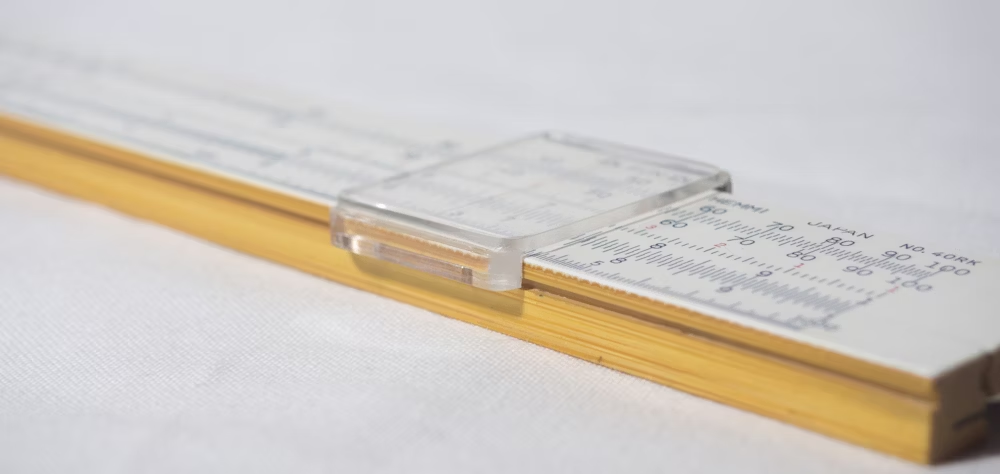
Beyond the primary categories of variables commonly discussed in research methodology , there exists a diverse range of other variables that play significant roles in the design and analysis of studies. Below is an overview of some of these variables, highlighting their definitions and roles within research studies:
- Discrete variables : A discrete variable is a quantitative variable that represents quantitative data , such as the number of children in a family or the number of cars in a parking lot. Discrete variables can only take on specific values.
- Categorical variables : A categorical variable categorizes subjects or items into groups that do not have a natural numerical order. Categorical data includes nominal variables, like country of origin, and ordinal variables, such as education level.
- Predictor variables : Often used in statistical models, a predictor variable is used to forecast or predict the outcomes of other variables, not necessarily with a causal implication.
- Outcome variables : These variables represent the results or outcomes that researchers aim to explain or predict through their studies. An outcome variable is central to understanding the effects of predictor variables.
- Latent variables : Not directly observable, latent variables are inferred from other, directly measured variables. Examples include psychological constructs like intelligence or socioeconomic status.
- Composite variables : Created by combining multiple variables, composite variables can measure a concept more reliably or simplify the analysis. An example would be a composite happiness index derived from several survey questions .
- Preceding variables : These variables come before other variables in time or sequence, potentially influencing subsequent outcomes. A preceding variable is crucial in longitudinal studies to determine causality or sequences of events.
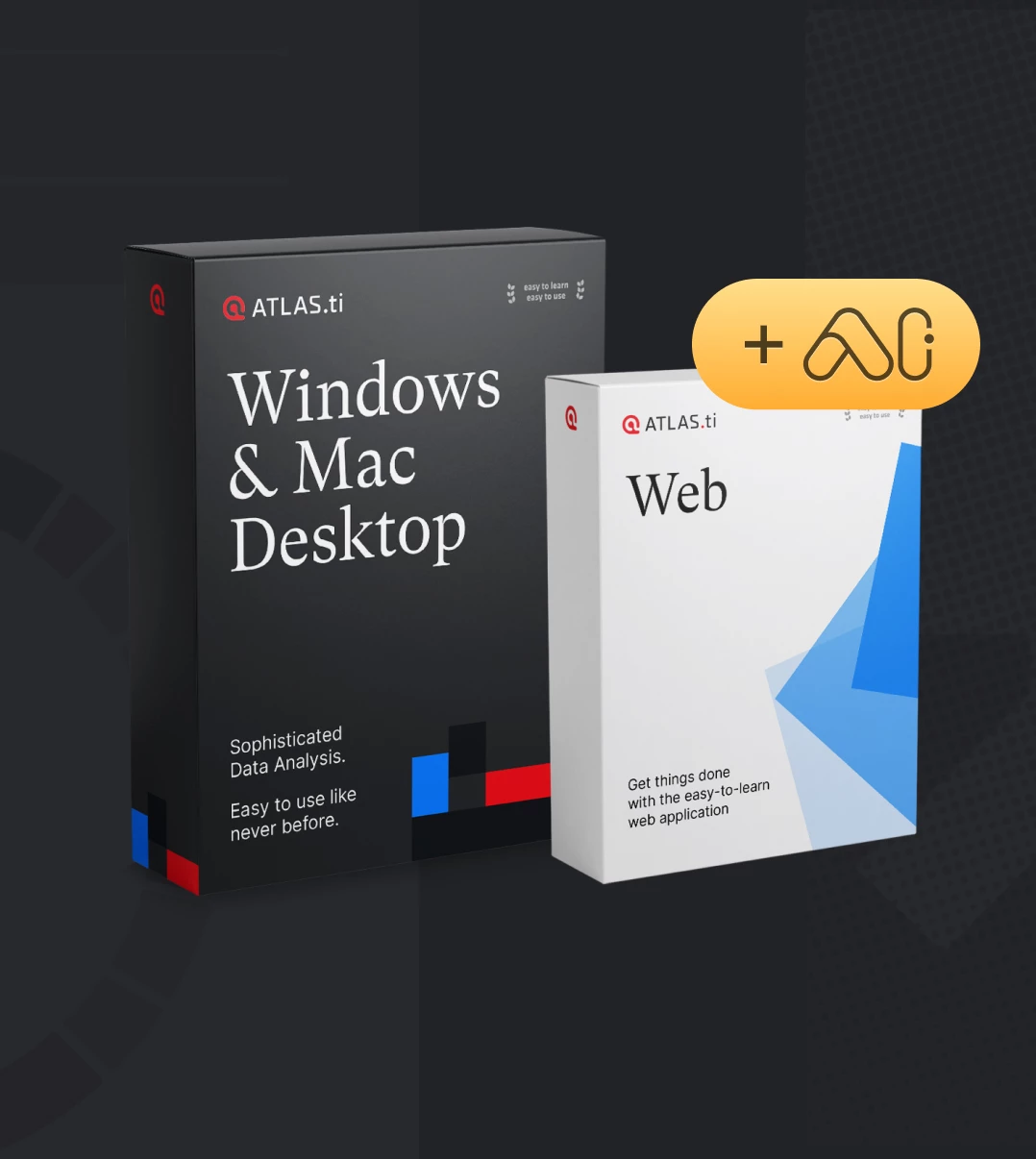
Master qualitative research with ATLAS.ti
Turn data into critical insights with our data analysis platform. Try out a free trial today.
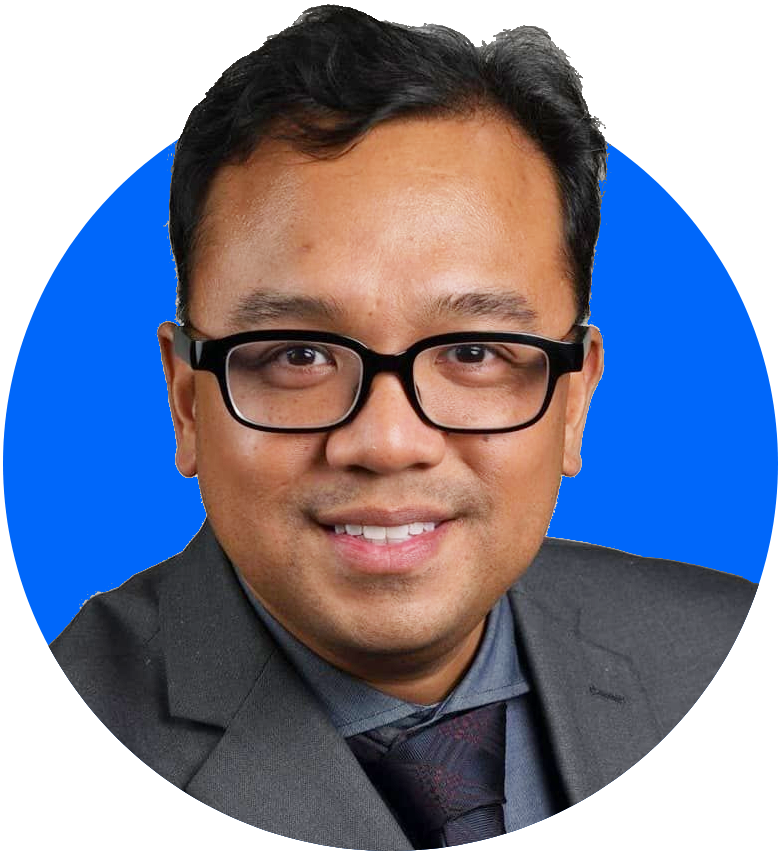
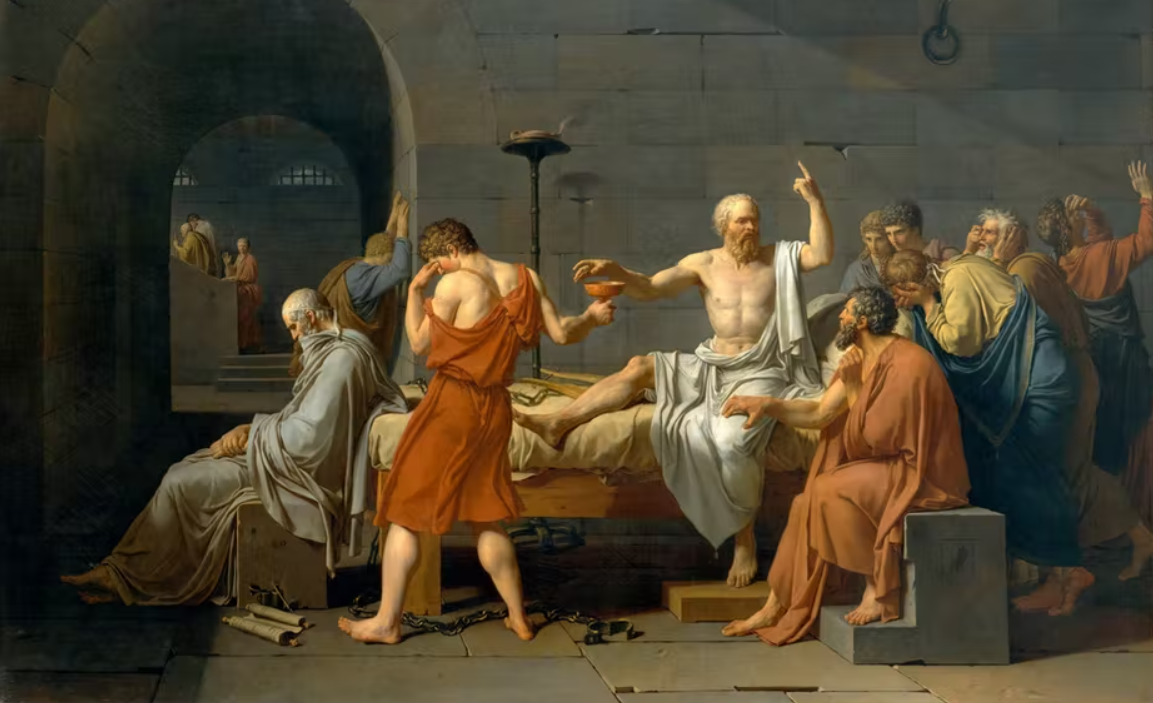
PHILO-notes
Free Online Learning Materials
What are Variables and Why are They Important in Research?
In research, variables are crucial components that help to define and measure the concepts and phenomena under investigation. Variables are defined as any characteristic or attribute that can vary or change in some way. They can be measured, manipulated, or controlled to investigate the relationship between different factors and their impact on the research outcomes. In this essay, I will discuss the importance of variables in research, highlighting their role in defining research questions, designing studies, analyzing data, and drawing conclusions.
Defining Research Questions
Variables play a critical role in defining research questions. Research questions are formulated based on the variables that are under investigation. These questions guide the entire research process, including the selection of research methods, data collection procedures, and data analysis techniques. Variables help researchers to identify the key concepts and phenomena that they wish to investigate, and to formulate research questions that are specific, measurable, and relevant to the research objectives.
For example, in a study on the relationship between exercise and stress, the variables would be exercise and stress. The research question might be: “What is the relationship between the frequency of exercise and the level of perceived stress among young adults?”
Designing Studies
Variables also play a crucial role in the design of research studies. The selection of variables determines the type of research design that will be used, as well as the methods and procedures for collecting and analyzing data. Variables can be independent, dependent, or moderator variables, depending on their role in the research design.
Independent variables are the variables that are manipulated or controlled by the researcher. They are used to determine the effect of a particular factor on the dependent variable. Dependent variables are the variables that are measured or observed to determine the impact of the independent variable. Moderator variables are the variables that influence the relationship between the independent and dependent variables.
For example, in a study on the effect of caffeine on athletic performance, the independent variable would be caffeine, and the dependent variable would be athletic performance. The moderator variables could include factors such as age, gender, and fitness level.
Analyzing Data
Variables are also essential in the analysis of research data. Statistical methods are used to analyze the data and determine the relationships between the variables. The type of statistical analysis that is used depends on the nature of the variables, their level of measurement, and the research design.
For example, if the variables are categorical or nominal, chi-square tests or contingency tables can be used to determine the relationships between them. If the variables are continuous, correlation analysis or regression analysis can be used to determine the strength and direction of the relationship between them.
Drawing Conclusions
Finally, variables are crucial in drawing conclusions from research studies. The results of the study are based on the relationship between the variables and the conclusions drawn depend on the validity and reliability of the research methods and the accuracy of the statistical analysis. Variables help to establish the cause-and-effect relationships between different factors and to make predictions about the outcomes of future events.
For example, in a study on the effect of smoking on lung cancer, the independent variable would be smoking, and the dependent variable would be lung cancer. The conclusion would be that smoking is a risk factor for lung cancer, based on the strength and direction of the relationship between the variables.
In conclusion, variables play a crucial role in research across different fields and disciplines. They help to define research questions, design studies, analyze data, and draw conclusions. By understanding the importance of variables in research, researchers can design studies that are relevant, accurate, and reliable, and can provide valuable insights into the phenomena under investigation. Therefore, it is essential to consider variables carefully when designing, conducting, and interpreting research studies.

CRO Platform
Test your insights. Run experiments. Win. Or learn. And then win.

eCommerce Customer Analytics Platform

Acquisition matters. But retention matters more. Understand, monitor & nurture the best customers.
- Case Studies
- Ebooks, Tools, Templates
- Digital Marketing Glossary
- eCommerce Growth Stories
- eCommerce Growth Show
- Help & Technical Documentation
CRO Guide > Chapter 3.1
Qualitative Research: Definition, Methodology, Limitation & Examples
Qualitative research is a method focused on understanding human behavior and experiences through non-numerical data. Examples of qualitative research include:
- One-on-one interviews,
- Focus groups, Ethnographic research,
- Case studies,
- Record keeping,
- Qualitative observations
In this article, we’ll provide tips and tricks on how to use qualitative research to better understand your audience through real world examples and improve your ROI. We’ll also learn the difference between qualitative and quantitative data.

Table of Contents
Marketers often seek to understand their customers deeply. Qualitative research methods such as face-to-face interviews, focus groups, and qualitative observations can provide valuable insights into your products, your market, and your customers’ opinions and motivations. Understanding these nuances can significantly enhance marketing strategies and overall customer satisfaction.
What is Qualitative Research
Qualitative research is a market research method that focuses on obtaining data through open-ended and conversational communication. This method focuses on the “why” rather than the “what” people think about you. Thus, qualitative research seeks to uncover the underlying motivations, attitudes, and beliefs that drive people’s actions.
Let’s say you have an online shop catering to a general audience. You do a demographic analysis and you find out that most of your customers are male. Naturally, you will want to find out why women are not buying from you. And that’s what qualitative research will help you find out.
In the case of your online shop, qualitative research would involve reaching out to female non-customers through methods such as in-depth interviews or focus groups. These interactions provide a platform for women to express their thoughts, feelings, and concerns regarding your products or brand. Through qualitative analysis, you can uncover valuable insights into factors such as product preferences, user experience, brand perception, and barriers to purchase.
Types of Qualitative Research Methods
Qualitative research methods are designed in a manner that helps reveal the behavior and perception of a target audience regarding a particular topic.
The most frequently used qualitative analysis methods are one-on-one interviews, focus groups, ethnographic research, case study research, record keeping, and qualitative observation.
1. One-on-one interviews
Conducting one-on-one interviews is one of the most common qualitative research methods. One of the advantages of this method is that it provides a great opportunity to gather precise data about what people think and their motivations.
Spending time talking to customers not only helps marketers understand who their clients are, but also helps with customer care: clients love hearing from brands. This strengthens the relationship between a brand and its clients and paves the way for customer testimonials.
- A company might conduct interviews to understand why a product failed to meet sales expectations.
- A researcher might use interviews to gather personal stories about experiences with healthcare.
These interviews can be performed face-to-face or on the phone and usually last between half an hour to over two hours.
When a one-on-one interview is conducted face-to-face, it also gives the marketer the opportunity to read the body language of the respondent and match the responses.
2. Focus groups
Focus groups gather a small number of people to discuss and provide feedback on a particular subject. The ideal size of a focus group is usually between five and eight participants. The size of focus groups should reflect the participants’ familiarity with the topic. For less important topics or when participants have little experience, a group of 10 can be effective. For more critical topics or when participants are more knowledgeable, a smaller group of five to six is preferable for deeper discussions.
The main goal of a focus group is to find answers to the “why”, “what”, and “how” questions. This method is highly effective in exploring people’s feelings and ideas in a social setting, where group dynamics can bring out insights that might not emerge in one-on-one situations.
- A focus group could be used to test reactions to a new product concept.
- Marketers might use focus groups to see how different demographic groups react to an advertising campaign.
One advantage that focus groups have is that the marketer doesn’t necessarily have to interact with the group in person. Nowadays focus groups can be sent as online qualitative surveys on various devices.
Focus groups are an expensive option compared to the other qualitative research methods, which is why they are typically used to explain complex processes.
3. Ethnographic research
Ethnographic research is the most in-depth observational method that studies individuals in their naturally occurring environment.
This method aims at understanding the cultures, challenges, motivations, and settings that occur.
- A study of workplace culture within a tech startup.
- Observational research in a remote village to understand local traditions.
Ethnographic research requires the marketer to adapt to the target audiences’ environments (a different organization, a different city, or even a remote location), which is why geographical constraints can be an issue while collecting data.
This type of research can last from a few days to a few years. It’s challenging and time-consuming and solely depends on the expertise of the marketer to be able to analyze, observe, and infer the data.
4. Case study research
The case study method has grown into a valuable qualitative research method. This type of research method is usually used in education or social sciences. It involves a comprehensive examination of a single instance or event, providing detailed insights into complex issues in real-life contexts.
- Analyzing a single school’s innovative teaching method.
- A detailed study of a patient’s medical treatment over several years.
Case study research may seem difficult to operate, but it’s actually one of the simplest ways of conducting research as it involves a deep dive and thorough understanding of the data collection methods and inferring the data.
5. Record keeping
Record keeping is similar to going to the library: you go over books or any other reference material to collect relevant data. This method uses already existing reliable documents and similar sources of information as a data source.
- Historical research using old newspapers and letters.
- A study on policy changes over the years by examining government records.
This method is useful for constructing a historical context around a research topic or verifying other findings with documented evidence.
6. Qualitative observation
Qualitative observation is a method that uses subjective methodologies to gather systematic information or data. This method deals with the five major sensory organs and their functioning, sight, smell, touch, taste, and hearing.
- Sight : Observing the way customers visually interact with product displays in a store to understand their browsing behaviors and preferences.
- Smell : Noting reactions of consumers to different scents in a fragrance shop to study the impact of olfactory elements on product preference.
- Touch : Watching how individuals interact with different materials in a clothing store to assess the importance of texture in fabric selection.
- Taste : Evaluating reactions of participants in a taste test to identify flavor profiles that appeal to different demographic groups.
- Hearing : Documenting responses to changes in background music within a retail environment to determine its effect on shopping behavior and mood.
Below we are also providing real-life examples of qualitative research that demonstrate practical applications across various contexts:
Qualitative Research Real World Examples
Let’s explore some examples of how qualitative research can be applied in different contexts.
1. Online grocery shop with a predominantly male audience
Method used: one-on-one interviews.
Let’s go back to one of the previous examples. You have an online grocery shop. By nature, it addresses a general audience, but after you do a demographic analysis you find out that most of your customers are male.
One good method to determine why women are not buying from you is to hold one-on-one interviews with potential customers in the category.
Interviewing a sample of potential female customers should reveal why they don’t find your store appealing. The reasons could range from not stocking enough products for women to perhaps the store’s emphasis on heavy-duty tools and automotive products, for example. These insights can guide adjustments in inventory and marketing strategies.
2. Software company launching a new product
Method used: focus groups.
Focus groups are great for establishing product-market fit.
Let’s assume you are a software company that wants to launch a new product and you hold a focus group with 12 people. Although getting their feedback regarding users’ experience with the product is a good thing, this sample is too small to define how the entire market will react to your product.
So what you can do instead is holding multiple focus groups in 20 different geographic regions. Each region should be hosting a group of 12 for each market segment; you can even segment your audience based on age. This would be a better way to establish credibility in the feedback you receive.
3. Alan Pushkin’s “God’s Choice: The Total World of a Fundamentalist Christian School”
Method used: ethnographic research.
Moving from a fictional example to a real-life one, let’s analyze Alan Peshkin’s 1986 book “God’s Choice: The Total World of a Fundamentalist Christian School”.
Peshkin studied the culture of Bethany Baptist Academy by interviewing the students, parents, teachers, and members of the community alike, and spending eighteen months observing them to provide a comprehensive and in-depth analysis of Christian schooling as an alternative to public education.
The study highlights the school’s unified purpose, rigorous academic environment, and strong community support while also pointing out its lack of cultural diversity and openness to differing viewpoints. These insights are crucial for understanding how such educational settings operate and what they offer to students.
Even after discovering all this, Peshkin still presented the school in a positive light and stated that public schools have much to learn from such schools.
Peshkin’s in-depth research represents a qualitative study that uses observations and unstructured interviews, without any assumptions or hypotheses. He utilizes descriptive or non-quantifiable data on Bethany Baptist Academy specifically, without attempting to generalize the findings to other Christian schools.
4. Understanding buyers’ trends
Method used: record keeping.
Another way marketers can use quality research is to understand buyers’ trends. To do this, marketers need to look at historical data for both their company and their industry and identify where buyers are purchasing items in higher volumes.
For example, electronics distributors know that the holiday season is a peak market for sales while life insurance agents find that spring and summer wedding months are good seasons for targeting new clients.
5. Determining products/services missing from the market
Conducting your own research isn’t always necessary. If there are significant breakthroughs in your industry, you can use industry data and adapt it to your marketing needs.
The influx of hacking and hijacking of cloud-based information has made Internet security a topic of many industry reports lately. A software company could use these reports to better understand the problems its clients are facing.
As a result, the company can provide solutions prospects already know they need.
Real-time Customer Lifetime Value (CLV) Benchmark Report
See where your business stands compared to 1,000+ e-stores in different industries.
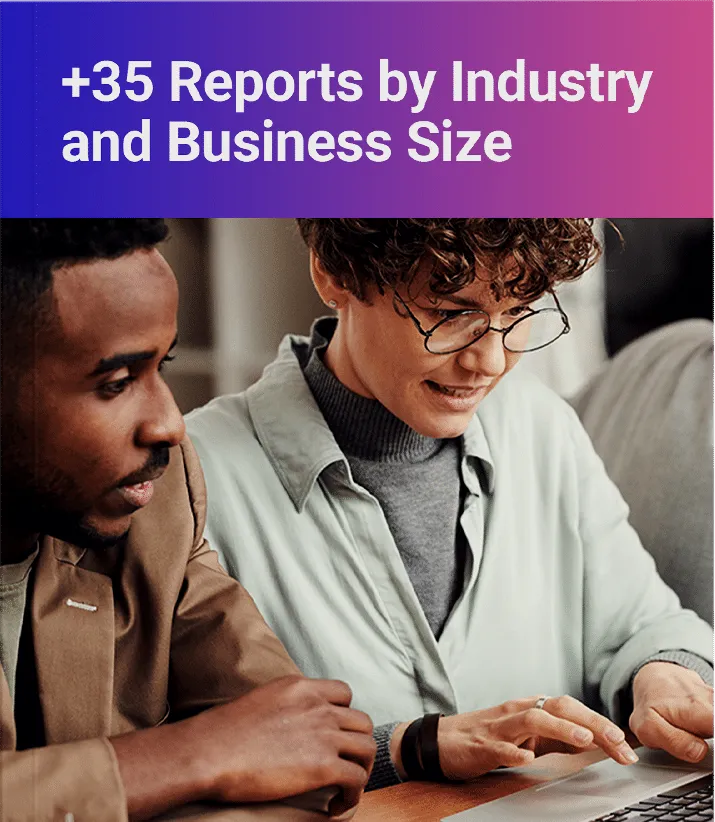
Qualitative Research Approaches
Once the marketer has decided that their research questions will provide data that is qualitative in nature, the next step is to choose the appropriate qualitative approach.
The approach chosen will take into account the purpose of the research, the role of the researcher, the data collected, the method of data analysis , and how the results will be presented. The most common approaches include:
- Narrative : This method focuses on individual life stories to understand personal experiences and journeys. It examines how people structure their stories and the themes within them to explore human existence. For example, a narrative study might look at cancer survivors to understand their resilience and coping strategies.
- Phenomenology : attempts to understand or explain life experiences or phenomena; It aims to reveal the depth of human consciousness and perception, such as by studying the daily lives of those with chronic illnesses.
- Grounded theory : investigates the process, action, or interaction with the goal of developing a theory “grounded” in observations and empirical data.
- Ethnography : describes and interprets an ethnic, cultural, or social group;
- Case study : examines episodic events in a definable framework, develops in-depth analyses of single or multiple cases, and generally explains “how”. An example might be studying a community health program to evaluate its success and impact.
How to Analyze Qualitative Data
Analyzing qualitative data involves interpreting non-numerical data to uncover patterns, themes, and deeper insights. This process is typically more subjective and requires a systematic approach to ensure reliability and validity.
1. Data Collection
Ensure that your data collection methods (e.g., interviews, focus groups, observations) are well-documented and comprehensive. This step is crucial because the quality and depth of the data collected will significantly influence the analysis.
2. Data Preparation
Once collected, the data needs to be organized. Transcribe audio and video recordings, and gather all notes and documents. Ensure that all data is anonymized to protect participant confidentiality where necessary.
3. Familiarization
Immerse yourself in the data by reading through the materials multiple times. This helps you get a general sense of the information and begin identifying patterns or recurring themes.
Develop a coding system to tag data with labels that summarize and account for each piece of information. Codes can be words, phrases, or acronyms that represent how these segments relate to your research questions.
- Descriptive Coding : Summarize the primary topic of the data.
- In Vivo Coding : Use language and terms used by the participants themselves.
- Process Coding : Use gerunds (“-ing” words) to label the processes at play.
- Emotion Coding : Identify and record the emotions conveyed or experienced.
5. Thematic Development
Group codes into themes that represent larger patterns in the data. These themes should relate directly to the research questions and form a coherent narrative about the findings.
6. Interpreting the Data
Interpret the data by constructing a logical narrative. This involves piecing together the themes to explain larger insights about the data. Link the results back to your research objectives and existing literature to bolster your interpretations.
7. Validation
Check the reliability and validity of your findings by reviewing if the interpretations are supported by the data. This may involve revisiting the data multiple times or discussing the findings with colleagues or participants for validation.
8. Reporting
Finally, present the findings in a clear and organized manner. Use direct quotes and detailed descriptions to illustrate the themes and insights. The report should communicate the narrative you’ve built from your data, clearly linking your findings to your research questions.
Limitations of qualitative research
The disadvantages of qualitative research are quite unique. The techniques of the data collector and their own unique observations can alter the information in subtle ways. That being said, these are the qualitative research’s limitations:
1. It’s a time-consuming process
The main drawback of qualitative study is that the process is time-consuming. Another problem is that the interpretations are limited. Personal experience and knowledge influence observations and conclusions.
Thus, qualitative research might take several weeks or months. Also, since this process delves into personal interaction for data collection, discussions often tend to deviate from the main issue to be studied.
2. You can’t verify the results of qualitative research
Because qualitative research is open-ended, participants have more control over the content of the data collected. So the marketer is not able to verify the results objectively against the scenarios stated by the respondents. For example, in a focus group discussing a new product, participants might express their feelings about the design and functionality. However, these opinions are influenced by individual tastes and experiences, making it difficult to ascertain a universally applicable conclusion from these discussions.
3. It’s a labor-intensive approach
Qualitative research requires a labor-intensive analysis process such as categorization, recording, etc. Similarly, qualitative research requires well-experienced marketers to obtain the needed data from a group of respondents.
4. It’s difficult to investigate causality
Qualitative research requires thoughtful planning to ensure the obtained results are accurate. There is no way to analyze qualitative data mathematically. This type of research is based more on opinion and judgment rather than results. Because all qualitative studies are unique they are difficult to replicate.
5. Qualitative research is not statistically representative
Because qualitative research is a perspective-based method of research, the responses given are not measured.
Comparisons can be made and this can lead toward duplication, but for the most part, quantitative data is required for circumstances that need statistical representation and that is not part of the qualitative research process.
While doing a qualitative study, it’s important to cross-reference the data obtained with the quantitative data. By continuously surveying prospects and customers marketers can build a stronger database of useful information.
Quantitative vs. Qualitative Research

Image source
Quantitative and qualitative research are two distinct methodologies used in the field of market research, each offering unique insights and approaches to understanding consumer behavior and preferences.
As we already defined, qualitative analysis seeks to explore the deeper meanings, perceptions, and motivations behind human behavior through non-numerical data. On the other hand, quantitative research focuses on collecting and analyzing numerical data to identify patterns, trends, and statistical relationships.
Let’s explore their key differences:
Nature of Data:
- Quantitative research : Involves numerical data that can be measured and analyzed statistically.
- Qualitative research : Focuses on non-numerical data, such as words, images, and observations, to capture subjective experiences and meanings.
Research Questions:
- Quantitative research : Typically addresses questions related to “how many,” “how much,” or “to what extent,” aiming to quantify relationships and patterns.
- Qualitative research: Explores questions related to “why” and “how,” aiming to understand the underlying motivations, beliefs, and perceptions of individuals.
Data Collection Methods:
- Quantitative research : Relies on structured surveys, experiments, or observations with predefined variables and measures.
- Qualitative research : Utilizes open-ended interviews, focus groups, participant observations, and textual analysis to gather rich, contextually nuanced data.
Analysis Techniques:
- Quantitative research: Involves statistical analysis to identify correlations, associations, or differences between variables.
- Qualitative research: Employs thematic analysis, coding, and interpretation to uncover patterns, themes, and insights within qualitative data.

Do Conversion Rate Optimization the Right way.
Explore helps you make the most out of your CRO efforts through advanced A/B testing, surveys, advanced segmentation and optimised customer journeys.

If you haven’t subscribed yet to our newsletter, now is your chance!

Like what you’re reading?
Join the informed ecommerce crowd.
We will never bug you with irrelevant info.
By clicking the Button, you confirm that you agree with our Terms and Conditions .
Continue your Conversion Rate Optimization Journey
- Last modified: January 3, 2023
- Conversion Rate Optimization , User Research
Valentin Radu

We’re a team of people that want to empower marketers around the world to create marketing campaigns that matter to consumers in a smart way. Meet us at the intersection of creativity, integrity, and development, and let us show you how to optimize your marketing.
Our Software
- > Book a Demo
- > Partner Program
- > Affiliate Program
- Blog Sitemap
- Terms and Conditions
- Privacy & Security
- Cookies Policy
- REVEAL Terms and Conditions
Log in using your username and password
- Search More Search for this keyword Advanced search
- Latest content
- BMJ NPH Collections
- BMJ Journals More You are viewing from: Google Indexer
You are here
- Online First
- Apple cider vinegar for weight management in Lebanese adolescents and young adults with overweight and obesity: a randomised, double-blind, placebo-controlled study
- Article Text
- Article info
- Citation Tools
- Rapid Responses
- Article metrics

- http://orcid.org/0000-0002-0214-242X Rony Abou-Khalil 1 ,
- Jeanne Andary 2 and
- Elissar El-Hayek 1
- 1 Department of Biology , Holy Spirit University of Kaslik , Jounieh , Lebanon
- 2 Nutrition and Food Science Department , American University of Science and Technology , Beirut , Lebanon
- Correspondence to Dr Rony Abou-Khalil, Department of Biology, Holy Spirit University of Kaslik, Jounieh, Lebanon; ronyaboukhalil{at}usek.edu.lb
Background and aims Obesity and overweight have become significant health concerns worldwide, leading to an increased interest in finding natural remedies for weight reduction. One such remedy that has gained popularity is apple cider vinegar (ACV).
Objective To investigate the effects of ACV consumption on weight, blood glucose, triglyceride and cholesterol levels in a sample of the Lebanese population.
Materials and methods 120 overweight and obese individuals were recruited. Participants were randomly assigned to either an intervention group receiving 5, 10 or 15 mL of ACV or a control group receiving a placebo (group 4) over a 12-week period. Measurements of anthropometric parameters, fasting blood glucose, triglyceride and cholesterol levels were taken at weeks 0, 4, 8 and 12.
Results Our findings showed that daily consumption of the three doses of ACV for a duration of between 4 and 12 weeks is associated with significant reductions in anthropometric variables (weight, body mass index, waist/hip circumferences and body fat ratio), blood glucose, triglyceride and cholesterol levels. No significant risk factors were observed during the 12 weeks of ACV intake.
Conclusion Consumption of ACV in people with overweight and obesity led to an improvement in the anthropometric and metabolic parameters. ACV could be a promising antiobesity supplement that does not produce any side effects.
- Weight management
- Lipid lowering
Data availability statement
All data relevant to the study are included in the article or uploaded as supplementary information.
This is an open access article distributed in accordance with the Creative Commons Attribution Non Commercial (CC BY-NC 4.0) license, which permits others to distribute, remix, adapt, build upon this work non-commercially, and license their derivative works on different terms, provided the original work is properly cited, appropriate credit is given, any changes made indicated, and the use is non-commercial. See: http://creativecommons.org/licenses/by-nc/4.0/ .
https://doi.org/10.1136/bmjnph-2023-000823
Statistics from Altmetric.com
Request permissions.
If you wish to reuse any or all of this article please use the link below which will take you to the Copyright Clearance Center’s RightsLink service. You will be able to get a quick price and instant permission to reuse the content in many different ways.
WHAT IS ALREADY KNOWN ON THIS TOPIC
Recently, there has been increasing interest in alternative remedies to support weight management, and one such remedy that has gained popularity is apple cider vinegar (ACV).
A few small-scale studies conducted on humans have shown promising results, with ACV consumption leading to weight loss, reduced body fat and decreased waist circumference.
WHAT THIS STUDY ADDS
No study has been conducted to investigate the potential antiobesity effect of ACV in the Lebanese population. By conducting research in this demographic, the study provides region-specific data and offers a more comprehensive understanding of the impact of ACV on weight loss and metabolic health.
HOW THIS STUDY MIGHT AFFECT RESEARCH, PRACTICE OR POLICY
The results might contribute to evidence-based recommendations for the use of ACV as a dietary intervention in the management of obesity.
The study could stimulate further research in the field, prompting scientists to explore the underlying mechanisms and conduct similar studies in other populations.
Introduction
Obesity is a growing global health concern characterised by excessive body fat accumulation, often resulting from a combination of genetic, environmental and lifestyle factors. 1 It is associated with an increased risk of numerous chronic illnesses such as type 2 diabetes, cardiovascular diseases, several common cancers and osteoarthritis. 1–3
According to the WHO, more than 1.9 billion adults were overweight worldwide in 2016, of whom more than 650 million were obese. 4 Worldwide obesity has nearly tripled since 1975. 4 The World Obesity Federation’s 2023 Atlas predicts that by 2035 more than half of the world’s population will be overweight or obese. 5
According to the 2022 Global Nutrition Report, Lebanon has made limited progress towards meeting its diet-related non-communicable diseases target. A total of 39.9% of adult (aged ≥18 years) women and 30.5% of adult men are living with obesity. Lebanon’s obesity prevalence is higher than the regional average of 10.3% for women and 7.5% for men. 6 In Lebanon, obesity was considered as the most important health problem by 27.6% and ranked fifth after cancer, cardiovascular, smoking and HIV/AIDS. 7
In recent years, there has been increasing interest in alternative remedies to support weight management, and one such remedy that has gained popularity is apple cider vinegar (ACV), which is a type of vinegar made by fermenting apple juice. ACV contains vitamins, minerals, amino acids and polyphenols such as flavonoids, which are believed to contribute to its potential health benefits. 8 9
It has been used for centuries as a traditional remedy for various ailments and has recently gained attention for its potential role in weight management.
In hypercaloric-fed rats, the daily consumption of ACV showed a lower rise in blood sugar and lipid profile. 10 In addition, ACV seems to decrease oxidative stress and reduces the risk of obesity in male rats with high-fat consumption. 11
A few small-scale studies conducted on humans have shown promising results, with ACV consumption leading to weight loss, reduced body fat and decreased waist circumference. 12 13 In fact, It has been suggested that ACV by slowing down gastric emptying, might promote satiety and reduce appetite. 14–16 Furthermore, ACV intake seems to ameliorate the glycaemic and lipid profile in healthy adults 17 and might have a positive impact on insulin sensitivity, potentially reducing the risk of type 2 diabetes. 8 10 18
Unfortunately, the sample sizes and durations of these studies were limited, necessitating larger and longer-term studies for more robust conclusions.
This work aims to study the efficacy and safety of ACV in reducing weight and ameliorating the lipid and glycaemic profiles in a sample of overweight and obese adolescents and young adults of the Lebanese population. To the best of our knowledge, no study has been conducted to investigate the potential antiobesity effect of ACV in the Lebanese population.
Materials and methods
Participants.
A total of 120 overweight and obese adolescents and young adults (46 men and 74 women) were enrolled in the study and assigned to either placebo group or experimental groups (receiving increasing doses of ACV).
The subjects were evaluated for eligibility according to the following inclusion criteria: age between 12 and 25 years, BMIs between 27 and 34 kg/m 2 , no chronic diseases, no intake of medications, no intake of ACV over the past 8 weeks prior to the beginning of the study. The subjects who met the inclusion criteria were selected by convenient sampling technique. Those who experienced heartburn due to vinegar were excluded.
Demographic, clinical data and eating habits were collected from all participants by self-administered questionnaire.
Study design
This study was a double-blind, randomised clinical trial conducted for 12 weeks.
Subjects were divided randomly into four groups: three treatment groups and a placebo group. A simple randomisation method was employed using the randomisation allocation software. Groups 1, 2 and 3 consumed 5, 10 and 15 mL, respectively, of ACV (containing 5% of acetic acid) diluted in 250 mL of water daily, in the morning on an empty stomach, for 12 weeks. The control group received a placebo consisting of water with similar taste and appearance. In order to mimic the taste of vinegar, the placebo group’s beverage (250 mL of water) contained lactic acid (250 mg/100 mL). Identical-looking ACV and placebo bottles were used and participants were instructed to consume their assigned solution without knowing its identity. The subject’s group assignment was withheld from the researchers performing the experiment.
Subjects consumed their normal diets throughout the study. The contents of daily meals and snacks were recorded in a diet diary. The physical activity of the subjects was also recorded. Daily individual phone messages were sent to all participants to remind them to take the ACV or the placebo. A mailing group was also created. Confidentiality was maintained throughout the procedure.
At weeks 0, 4, 8 and 12, anthropometric measurements were taken for all participants, and the level of glucose, triglycerides and total cholesterol was assessed by collecting 5 mL of fasting blood from each subject.
Anthropometric measurements
Body weight was measured in kg, to the nearest 0.01 kg, by standardised and calibrated digital scale. Height was measured in cm, to the nearest 0.1 cm, by a stadiometer. Anthropometric measurements were taken for all participants, by a team of trained field researchers, after 10–12 hours fast and while wearing only undergarments.
Body mass indices (BMIs) were calculated using the following equation:
The waist circumference measurement was taken between the lowest rib margin and the iliac crest while the subject was in a standing position (to the nearest 0.1 cm). Hip circumference was measured at the widest point of the hip (to the nearest 0.1 cm).
The body fat ratio (BFR) was measured by the bioelectrical impedance analysis method (OMRON Fat Loss Monitor, Model No HBF-306C; Japan). Anthropometric variables are shown in table 1 .
- View inline
Baseline demographic, anthropometric and biochemical variables of the three apple cider vinegar groups (group 1, 2 and 3) and the placebo group (group 4)
Blood biochemical analysis
Serum glucose was measured by the glucose oxidase method. 19 Triglyceride levels were determined using a serum triglyceride determination kit (TR0100, Sigma-Aldrich). Cholesterol levels were determined using a cholesterol quantitation kit (MAK043, Sigma-Aldrich). Biochemical variables are shown in table 1 .
Statistical methods and data analysis
Data are presented as mean±SD. Statistical analyses were performed using Statistical Package for the Social Sciences (SPSS) software (version 23.0). Significant differences between groups were determined by using an independent t-test. Statistical significance was set at p<0.05.
Ethical approval
The study protocol was reviewed and approved by the research ethics committee (REC) of the Higher Centre for Research (HCR) at The Holy Spirit University of Kaslik (USEK), Lebanon. The number/ID of the approval is HCR/EC 2023–005. The participants were informed of the study objectives and signed a written informed consent before enrolment. The study was conducted in accordance to the International Conference and Harmonisation E6 Guideline for Good Clinical Practice and the Ethical principles of the Declaration of Helsinki.
Sociodemographic, nutritional and other baseline characteristics of the participants
A total of 120 individuals (46 men and 74 women) with BMIs between 27 and 34 kg/m 2 , were enrolled in the study. The mean age of the subjects was 17.8±5.7 years and 17.6±5.4 years in the placebo and experimental groups respectively.
The majority of participants, approximately 98.3%, were non-vegetarian and 89% of them reported having a high eating frequency, with more than four meals per day. Eighty-seven per cent had no family history of obesity and 98% had no history of childhood obesity. The majority reported not having a regular exercise routine and experiencing negative emotions or anxiety. All participants were non-smokers and non-drinkers. A small percentage (6.7%) were following a therapeutic diet.
Effects of ACV intake on anthropometric variables
The addition of 5 mL, 10 mL or 15 mL of ACV to the diet resulted in significant decreases in body weight and BMI at weeks 4, 8 and 12 of ACV intake, when compared with baseline (week 0) (p<0.05). The decrease in body weight and BMI seemed to be dose-dependent, with the group receiving 15 mL of ACV showing the most important reduction ( table 2 ).
Anthropometric variables of the participants at weeks 0, 4, 8 and 12
The impact of ACV on body weight and BMI seems to be time-dependent as well. Reductions were more pronounced as the study progressed, with the most significant changes occurring at week 12.
The circumferences of the waist and hip, along with the Body Fat Ratio (BFR), decreased significantly in the three treatment groups at weeks 8 and 12 compared with week 0 (p<0.05). No significant effect was observed at week 4, compared with baseline (p>0.05). The effect of ACV on these parameters seems to be time-dependent with the most prominent effect observed at week 12 compared with week 4 and 8. However it does not seem to be dose dependent, as the three doses of ACV showed a similar level of efficacy in reducing the circumferences of the waist/hip circumferences and the BFR at week 8 and 12, compared with baseline ( table 2 ).
The placebo group did not experience any significant changes in the anthropometric variables throughout the study (p>0.05). This highlights that the observed improvements in body weight, BMI, waist and hip circumferences and Body Fat Ratio were likely attributed to the consumption of ACV.
Effects of ACV on blood biochemical parameters
The consumption of ACV also led to a time and dose dependent decrease in serum glucose, serum triglyceride and serum cholesterol levels. ( table 3 ).
Biochemical variables of the participants at weeks 0, 4, 8 and 12
Serum glucose levels decreased significantly by three doses of ACV at week 4, 8 and 12 compared with week 0 (p<0.05) ( table 3 ). Triglycerides and total cholesterol levels decreased significantly at weeks 8 and 12, compared with week 0 (p<0.05). A dose of 15 mL of ACV for a duration of 12 weeks seems to be the most effective dose in reducing these three blood biochemical parameters.
There were no changes in glucose, triglyceride and cholesterol levels in the placebo groups at weeks 4, 8 and 12 compared with week 0 ( table 3 ).
These data suggest that continued intake of 15 mL of ACV for more than 8 weeks is effective in reducing blood fasting sugar, triglyceride and total cholesterol levels in overweight/obese people.
Adverse reactions of ACV
No apparent adverse or harmful effects were reported by the participants during the 12 weeks of ACV intake.
During the past two decades of the last century, childhood and adolescent obesity have dramatically increased healthcare costs. 20 21 Diet and exercise are the basic elements of weight loss. Many complementary therapies have been promoted to treat obesity, but few are truly beneficial.
The present study is the first to investigate the antiobesity effectiveness of ACV, the fermented juice from crushed apples, in the Lebanese population.
A total of 120 overweight and obese adolescents and young adults (46 men and 74 women) with BMIs between 27 and 34 kg/m 2 , were enrolled. Participants were randomised to receive either a daily dose of ACV (5, 10 or 15 mL) or a placebo for a duration of 12 weeks.
Some previous studies have suggested that taking ACV before or with meals might help to reduce postprandial blood sugar levels, 22 23 but in our study, participants took ACV in the morning on an empty stomach. The choice of ACV intake timing was motivated by the aim to study the impact of apple cider vinegar without the confounding variables introduced by simultaneous food intake. In addition, taking ACV before meals could better reduce appetite and increase satiety.
Our findings reveal that the consumption of ACV in people with overweight and obesity led to an improvement in the anthropometric and metabolic parameters.
It is important to note that the diet diary and physical activity did not differ among the three treatment groups and the placebo throughout the whole study, suggesting that the decrease in anthropometric and biochemical parameters was caused by ACV intake.
Studies conducted on animal models often attribute these effects to various mechanisms, including increased energy expenditure, improved insulin sensitivity, appetite and satiety regulation.
While vinegar is composed of various ingredients, its primary component is acetic acid (AcOH). It has been shown that after 15 min of oral ingestion of 100 mL vinegar containing 0.75 g acetic acid, the serum acetate levels increases from 120 µmol/L at baseline to 350 µmol/L 24 ; this fast increase in circulatory acetate is due to its fast absorption in the upper digestive tract. 24 25
Biological action of acetate may be mediated by binding to the G-protein coupled receptors (GPRs), including GPR43 and GPR41. 25 These receptors are expressed in various insulin-sensitive tissues, such as adipose tissue, 26 skeletal muscle, liver, 27 and pancreatic beta cells, 28 but also in the small intestine and colon. 29 30
Yamashita and colleagues have revealed that oral administration of AcOH to type 2 diabetic Otsuka Long-Evans Tokushima Fatty rats, improves glucose tolerance and reduces lipid accumulation in the adipose tissue and liver. This improvement in obesity-linked type 2 diabetes is due to the capacity of AcOH to inhibit the activity of carbohydrate-responsive, element-binding protein, a transcription factor involved in regulating the expression of lipogenic genes such as fatty acid synthase and acetyl-CoA carboxylase. 26 31 Sakakibara and colleagues, have reported that AcOH, besides inhibiting lipogenesis, reduces the expression of genes involved in gluconeogenesis, such as glucose-6-phosphatase. 32 The effect of AcOH on lipogenesis and gluconeogenesis is in part mediated by the activation of 5'-AMP-activated protein kinase in the liver. 32 This enzyme seems to be an important pharmacological target for the treatment of metabolic disorders such as obesity, type 2 diabetes and hyperlipidaemia. 32 33
5'-AMP-activated protein kinase is also known to stimulate fatty acid oxidation, thereby increasing energy expenditure. 32 33 These data suggest that the effect of ACV on weight and fat loss may be partly due to the ability of AcOH to inhibit lipogenesis and gluconeogenesis and activate fat oxidation.
Animal studies suggest that besides reducing energy expenditure, acetate may also reduce energy intake, by regulating appetite and satiety. In mice, an intraperitoneal injection of acetate significantly reduced food intake by activating vagal afferent neurons. 32–34 It is important to note that animal studies done on the effect of acetate on vagal activation are contradictory. This might be due to the site of administration of acetate and the use of different animal models.
In addition, in vitro and in vivo animal model studies suggest that acetate increases the secretion of gut-derived satiety hormones by enter endocrine cells (located in the gut) such as GLP-1 and PYY hormones. 25 32–35
Human studies related to the effect of vinegar on body weight are limited.
In accordance with our study, a randomised clinical trial conducted by Khezri and his colleagues has shown that daily consumption of 30 mL of ACV for 12 weeks significantly reduced body weight, BMI, hip circumference, Visceral Adiposity Index and appetite score in obese subjects subjected to a restricted calorie diet, compared with the control group (restricted calorie diet without ACV). Furthermore, Khezri and his colleagues showed that plasma triglyceride and total cholesterol levels significantly decreased, and high density lipoprotein cholesterol concentration significantly increased, in the ACV group in comparison with the control group. 13 32–34
Similarly, Kondo and his colleagues showed that daily consumption of 15 or 30 mL of ACV for 12 weeks reduced body weight, BMI and serum triglyceride in a sample of the Japanese population. 12 13 32–34
In contrast, Park et al reported that daily consumption of 200 mL of pomegranate vinegar for 8 weeks significantly reduced total fat mass in overweight or obese subjects compared with the control group without significantly affecting body weight and BMI. 36 This contradictory result could be explained by the difference in the percentage of acetate and other potentially bioactive compounds (such as flavonoids and other phenolic compounds) in different vinegar types.
In Lebanon, the percentage of the population with a BMI of 30 kg/m 2 or more is approximately 32%. The results of the present study showed that in obese Lebanese subjects who had BMIs ranging from 27 and 34 kg/m 2 , daily oral intake of ACV for 12 weeks reduced the body weight by 6–8 kg and BMIs by 2.7–3.0 points.
It would be interesting to investigate in future studies the effect of neutralised acetic acid on anthropometric and metabolic parameters, knowing that acidic substances, including acetic acid, could contribute to enamel erosion over time. In addition to promoting oral health, neutralising the acidity of ACV could improve its taste, making it more palatable. Furthermore, studying the effects of ACV on weight loss in young Lebanese individuals provides valuable insights, but further research is needed for a comprehensive understanding of how the effect of ACV might vary across different age groups, particularly in older populations and menopausal women.
The findings of this study indicate that ACV consumption for 12 weeks led to significant reduction in anthropometric variables and improvements in blood glucose, triglyceride and cholesterol levels in overweight/obese adolescents/adults. These results suggest that ACV might have potential benefits in improving metabolic parameters related to obesity and metabolic disorders in obese individuals. The results may contribute to evidence-based recommendations for the use of ACV as a dietary intervention in the management of obesity. The study duration of 12 weeks limits the ability to observe long-term effects. Additionally, a larger sample size would enhance the generalisability of the results.
Ethics statements
Patient consent for publication.
Consent obtained from parent(s)/guardian(s)
Ethics approval
This study involves human participants and was approved by the research ethics committee of the Higher Center for Research (HCR) at The Holy Spirit University of Kaslik (USEK), Lebanon. The number/ID of the approval is HCR/EC 2023-005. Participants gave informed consent to participate in the study before taking part.
- Pandi-Perumal SR , et al
- Poirier P ,
- Bray GA , et al
- World Health Organization
- Global Nutrition Report
- Geagea AG ,
- Jurjus RA , et al
- Liao H-J , et al
- Serafin V ,
- Ousaaid D ,
- Laaroussi H ,
- Bakour M , et al
- Halima BH ,
- Sarra K , et al
- Fushimi T , et al
- Khezri SS ,
- Saidpour A ,
- Hosseinzadeh N , et al
- Montaser R , et al
- Hlebowicz J ,
- Darwiche G ,
- Björgell O , et al
- Santos HO ,
- de Moraes WMAM ,
- da Silva GAR , et al
- Pourmasoumi M ,
- Najafgholizadeh A , et al
- Walker HK ,
- Sanyaolu A ,
- Qi X , et al
- Nosrati HR ,
- Mousavi SE ,
- Sajjadi P , et al
- Johnston CS ,
- Quagliano S ,
- Sugiyama S ,
- Fushimi T ,
- Kishi M , et al
- Hernández MAG ,
- Canfora EE ,
- Jocken JWE , et al
- Le Poul E ,
- Struyf S , et al
- Goldsworthy SM ,
- Barnes AA , et al
- Priyadarshini M ,
- Fuller M , et al
- Karaki S-I ,
- Hayashi H , et al
- Karaki S-I , et al
- Yamashita H ,
- Fujisawa K ,
- Ito E , et al
- Sakakibara S ,
- Yamauchi T ,
- Oshima Y , et al
- Schimmack G ,
- Defronzo RA ,
- Goswami C ,
- Iwasaki Y ,
- Kim J , et al
Supplementary materials
- Press release
Contributors RA-K: conceptualisation, methodology, data curation, supervision, guarantor, project administration, visualisation, writing–original draft. EE-H: conceptualisation, methodology, data curation, visualisation, writing–review and editing. JA: investigation, validation, writing–review and editing.
Funding The authors have not declared a specific grant for this research from any funding agency in the public, commercial or not-for-profit sectors.
Competing interests No, there are no competing interests.
Provenance and peer review Not commissioned; externally peer reviewed.
Read the full text or download the PDF:

Victimization Status Among Persons with Disabilities and its Predictors: Evidence from Bangladesh National Survey on Persons with Disabilities
- Find this author on Google Scholar
- Find this author on PubMed
- Search for this author on this site
- ORCID record for Mizanur Rahman
- ORCID record for Md Shohel Rana
- ORCID record for Md. Nuruzzaman Khan
- For correspondence: [email protected]
- Info/History
- Preview PDF
Background: Persons with disabilities often face various forms of victimization, yet there is limited research exploring this phenomenon in Bangladesh. This study aims to investigate the victimization status among persons with disabilities and identify its predictors. Methods: Data of 4293 persons with disabilities analyzed in this study were extracted from the 2021 National Survey on Persons with Disabilities. Victimization status (yes, no) was considered as the outcome variables. Explanatory variables considered were factors at the individual, household, and community levels. A multilevel mixed-effect logistic regression model was used to explore the association of the outcome variable with explanatory variables by dividing the total sample into age groups of 0-17 years and ≥ 18 years. Results: The study found that 44% of persons with disabilities in Bangladesh experienced victimization, predominantly involving neighbours (90.64%), friends (28.41%), and family members (27.07%). Among persons aged 0-17 years, increasing age was associated with higher likelihood of being victimized, while residing in the richest households or certain divisions like Khulna and Rangpur was associated with lower likelihoods. Conversely, among respondents aged 18-95 years, increasing age was associated with lower likelihood of being victimized. Unmarried respondents had increased likelihood of victimization compared to married individuals. Furthermore, persons residing in the richest wealth quintile compared to the poorest, and residence in certain divisions such as Chattogram, Khulna, Mymensingh, Rangpur, and Sylhet reported higher likelihoods of victimization compared to those in the Barishal division. Conclusion: This study's findings underscore that around 4 in 10 persons with disabilities are being victimized. Tailored programs and awareness-building initiatives covering neighbours, friends, and family members of persons with disabilities are important to ensure dignified lives for this population.
Competing Interest Statement
The authors have declared no competing interest.
Funding Statement
The author(s) received no specific funding for this work.
Author Declarations
I confirm all relevant ethical guidelines have been followed, and any necessary IRB and/or ethics committee approvals have been obtained.
The details of the IRB/oversight body that provided approval or exemption for the research described are given below:
The survey analyzed in this study underwent review and approval by the Ethics Committee of the BBS. De-identified data were obtained from the BBS upon submission of a research proposal. As the data received were de-identified, further ethical approval was not necessary.
I confirm that all necessary patient/participant consent has been obtained and the appropriate institutional forms have been archived, and that any patient/participant/sample identifiers included were not known to anyone (e.g., hospital staff, patients or participants themselves) outside the research group so cannot be used to identify individuals.
I understand that all clinical trials and any other prospective interventional studies must be registered with an ICMJE-approved registry, such as ClinicalTrials.gov. I confirm that any such study reported in the manuscript has been registered and the trial registration ID is provided (note: if posting a prospective study registered retrospectively, please provide a statement in the trial ID field explaining why the study was not registered in advance).
I have followed all appropriate research reporting guidelines, such as any relevant EQUATOR Network research reporting checklist(s) and other pertinent material, if applicable.
Data Availability
The authors have made the data associated with this study accessible to interested researchers. To access the raw data file, interested researchers need to submit a research proposal to Bangladesh Bureau of Statistics through the following link: https://bbs.gov.bd/site/page/b588b454-0f88-4679-bf20-90e06dc1d10b/-.
View the discussion thread.
Thank you for your interest in spreading the word about medRxiv.
NOTE: Your email address is requested solely to identify you as the sender of this article.

Citation Manager Formats
- EndNote (tagged)
- EndNote 8 (xml)
- RefWorks Tagged
- Ref Manager
- Tweet Widget
- Facebook Like
- Google Plus One
- Addiction Medicine (324)
- Allergy and Immunology (629)
- Anesthesia (166)
- Cardiovascular Medicine (2391)
- Dentistry and Oral Medicine (289)
- Dermatology (207)
- Emergency Medicine (380)
- Endocrinology (including Diabetes Mellitus and Metabolic Disease) (843)
- Epidemiology (11786)
- Forensic Medicine (10)
- Gastroenterology (703)
- Genetic and Genomic Medicine (3758)
- Geriatric Medicine (350)
- Health Economics (636)
- Health Informatics (2403)
- Health Policy (935)
- Health Systems and Quality Improvement (902)
- Hematology (341)
- HIV/AIDS (782)
- Infectious Diseases (except HIV/AIDS) (13330)
- Intensive Care and Critical Care Medicine (769)
- Medical Education (366)
- Medical Ethics (105)
- Nephrology (400)
- Neurology (3517)
- Nursing (199)
- Nutrition (528)
- Obstetrics and Gynecology (677)
- Occupational and Environmental Health (665)
- Oncology (1828)
- Ophthalmology (538)
- Orthopedics (219)
- Otolaryngology (287)
- Pain Medicine (234)
- Palliative Medicine (66)
- Pathology (447)
- Pediatrics (1035)
- Pharmacology and Therapeutics (426)
- Primary Care Research (423)
- Psychiatry and Clinical Psychology (3186)
- Public and Global Health (6160)
- Radiology and Imaging (1283)
- Rehabilitation Medicine and Physical Therapy (750)
- Respiratory Medicine (830)
- Rheumatology (379)
- Sexual and Reproductive Health (372)
- Sports Medicine (324)
- Surgery (402)
- Toxicology (50)
- Transplantation (172)
- Urology (146)
A new variable-resolution global chemistry-climate model for research at the nexus of US climate and air quality extremes
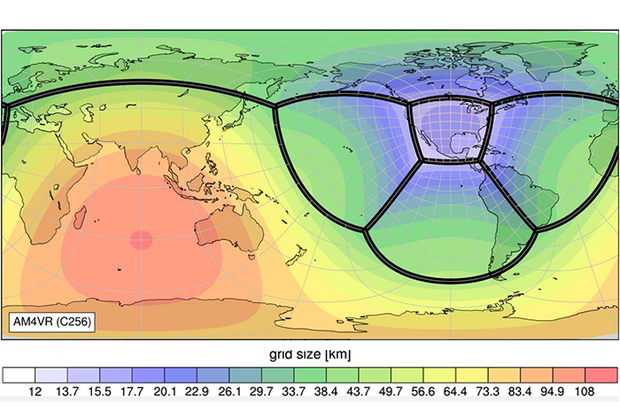
The new model is a seamless system that can provide detailed information over a targeted region, while still integrating the global Earth system components. Credit: doi:10.1029/2023MS003984
In the U.S., air pollution includes contributions from multiple local human and natural sources, as well as transported sources like wildfire smoke from Canada, dust plumes from Africa, and intercontinental pollution from Asia. Accurate projection of future climate and air quality at scales relevant to local and regional stakeholders requires a seamless modeling system that can provide detailed information over a targeted region, while still integrating the global Earth system components in a computationally efficient manner. Scientists at GFDL have developed a novel variable-resolution global chemistry-climate model, known as AM4VR, for research at the nexus of U.S. climate and air quality extremes.
In contrast with the global models contributing to the latest Intergovernmental Panel on Climate Change Report, this model features more than 10 times finer spatial resolution over the contiguous U.S., allowing it to better resolve cities, mountain valleys, thunderstorms, and urban-to-rural air quality variations. The model features much improved representation of regional rainfall extremes, drought, haze and wildfire smoke, and ozone air pollution extremes in diverse U.S. air basins, including California.
Click to read the full article
Related Content
News & features, to help forecast air quality and issue timely warnings, noaa aims to answer what fires emit and how, vegetation feedbacks during drought exacerbate ozone air pollution extremes in europe, climate.gov tweet chat: talk with experts about ongoing nasa/noaa field campaign to study smoke from wildfires and agricultural burning, maps & data, what environmental data are relevant to the study of infectious diseases like covid-19, global temperature anomalies - graphing tool, searching for data, teaching climate, toolbox for teaching climate & energy, climate youth engagement, white house climate education and literacy initiative, climate resilience toolkit, climate science for water professionals, storm events database, translating climate science into hydrology.

An official website of the United States government
The .gov means it’s official. Federal government websites often end in .gov or .mil. Before sharing sensitive information, make sure you’re on a federal government site.
The site is secure. The https:// ensures that you are connecting to the official website and that any information you provide is encrypted and transmitted securely.
- Publications
- Account settings
Preview improvements coming to the PMC website in October 2024. Learn More or Try it out now .
- Advanced Search
- Journal List
- HCA Healthc J Med
- v.1(2); 2020
- PMC10324782

Introduction to Research Statistical Analysis: An Overview of the Basics
Christian vandever.
1 HCA Healthcare Graduate Medical Education
Description
This article covers many statistical ideas essential to research statistical analysis. Sample size is explained through the concepts of statistical significance level and power. Variable types and definitions are included to clarify necessities for how the analysis will be interpreted. Categorical and quantitative variable types are defined, as well as response and predictor variables. Statistical tests described include t-tests, ANOVA and chi-square tests. Multiple regression is also explored for both logistic and linear regression. Finally, the most common statistics produced by these methods are explored.
Introduction
Statistical analysis is necessary for any research project seeking to make quantitative conclusions. The following is a primer for research-based statistical analysis. It is intended to be a high-level overview of appropriate statistical testing, while not diving too deep into any specific methodology. Some of the information is more applicable to retrospective projects, where analysis is performed on data that has already been collected, but most of it will be suitable to any type of research. This primer will help the reader understand research results in coordination with a statistician, not to perform the actual analysis. Analysis is commonly performed using statistical programming software such as R, SAS or SPSS. These allow for analysis to be replicated while minimizing the risk for an error. Resources are listed later for those working on analysis without a statistician.
After coming up with a hypothesis for a study, including any variables to be used, one of the first steps is to think about the patient population to apply the question. Results are only relevant to the population that the underlying data represents. Since it is impractical to include everyone with a certain condition, a subset of the population of interest should be taken. This subset should be large enough to have power, which means there is enough data to deliver significant results and accurately reflect the study’s population.
The first statistics of interest are related to significance level and power, alpha and beta. Alpha (α) is the significance level and probability of a type I error, the rejection of the null hypothesis when it is true. The null hypothesis is generally that there is no difference between the groups compared. A type I error is also known as a false positive. An example would be an analysis that finds one medication statistically better than another, when in reality there is no difference in efficacy between the two. Beta (β) is the probability of a type II error, the failure to reject the null hypothesis when it is actually false. A type II error is also known as a false negative. This occurs when the analysis finds there is no difference in two medications when in reality one works better than the other. Power is defined as 1-β and should be calculated prior to running any sort of statistical testing. Ideally, alpha should be as small as possible while power should be as large as possible. Power generally increases with a larger sample size, but so does cost and the effect of any bias in the study design. Additionally, as the sample size gets bigger, the chance for a statistically significant result goes up even though these results can be small differences that do not matter practically. Power calculators include the magnitude of the effect in order to combat the potential for exaggeration and only give significant results that have an actual impact. The calculators take inputs like the mean, effect size and desired power, and output the required minimum sample size for analysis. Effect size is calculated using statistical information on the variables of interest. If that information is not available, most tests have commonly used values for small, medium or large effect sizes.
When the desired patient population is decided, the next step is to define the variables previously chosen to be included. Variables come in different types that determine which statistical methods are appropriate and useful. One way variables can be split is into categorical and quantitative variables. ( Table 1 ) Categorical variables place patients into groups, such as gender, race and smoking status. Quantitative variables measure or count some quantity of interest. Common quantitative variables in research include age and weight. An important note is that there can often be a choice for whether to treat a variable as quantitative or categorical. For example, in a study looking at body mass index (BMI), BMI could be defined as a quantitative variable or as a categorical variable, with each patient’s BMI listed as a category (underweight, normal, overweight, and obese) rather than the discrete value. The decision whether a variable is quantitative or categorical will affect what conclusions can be made when interpreting results from statistical tests. Keep in mind that since quantitative variables are treated on a continuous scale it would be inappropriate to transform a variable like which medication was given into a quantitative variable with values 1, 2 and 3.
Categorical vs. Quantitative Variables
Both of these types of variables can also be split into response and predictor variables. ( Table 2 ) Predictor variables are explanatory, or independent, variables that help explain changes in a response variable. Conversely, response variables are outcome, or dependent, variables whose changes can be partially explained by the predictor variables.
Response vs. Predictor Variables
Choosing the correct statistical test depends on the types of variables defined and the question being answered. The appropriate test is determined by the variables being compared. Some common statistical tests include t-tests, ANOVA and chi-square tests.
T-tests compare whether there are differences in a quantitative variable between two values of a categorical variable. For example, a t-test could be useful to compare the length of stay for knee replacement surgery patients between those that took apixaban and those that took rivaroxaban. A t-test could examine whether there is a statistically significant difference in the length of stay between the two groups. The t-test will output a p-value, a number between zero and one, which represents the probability that the two groups could be as different as they are in the data, if they were actually the same. A value closer to zero suggests that the difference, in this case for length of stay, is more statistically significant than a number closer to one. Prior to collecting the data, set a significance level, the previously defined alpha. Alpha is typically set at 0.05, but is commonly reduced in order to limit the chance of a type I error, or false positive. Going back to the example above, if alpha is set at 0.05 and the analysis gives a p-value of 0.039, then a statistically significant difference in length of stay is observed between apixaban and rivaroxaban patients. If the analysis gives a p-value of 0.91, then there was no statistical evidence of a difference in length of stay between the two medications. Other statistical summaries or methods examine how big of a difference that might be. These other summaries are known as post-hoc analysis since they are performed after the original test to provide additional context to the results.
Analysis of variance, or ANOVA, tests can observe mean differences in a quantitative variable between values of a categorical variable, typically with three or more values to distinguish from a t-test. ANOVA could add patients given dabigatran to the previous population and evaluate whether the length of stay was significantly different across the three medications. If the p-value is lower than the designated significance level then the hypothesis that length of stay was the same across the three medications is rejected. Summaries and post-hoc tests also could be performed to look at the differences between length of stay and which individual medications may have observed statistically significant differences in length of stay from the other medications. A chi-square test examines the association between two categorical variables. An example would be to consider whether the rate of having a post-operative bleed is the same across patients provided with apixaban, rivaroxaban and dabigatran. A chi-square test can compute a p-value determining whether the bleeding rates were significantly different or not. Post-hoc tests could then give the bleeding rate for each medication, as well as a breakdown as to which specific medications may have a significantly different bleeding rate from each other.
A slightly more advanced way of examining a question can come through multiple regression. Regression allows more predictor variables to be analyzed and can act as a control when looking at associations between variables. Common control variables are age, sex and any comorbidities likely to affect the outcome variable that are not closely related to the other explanatory variables. Control variables can be especially important in reducing the effect of bias in a retrospective population. Since retrospective data was not built with the research question in mind, it is important to eliminate threats to the validity of the analysis. Testing that controls for confounding variables, such as regression, is often more valuable with retrospective data because it can ease these concerns. The two main types of regression are linear and logistic. Linear regression is used to predict differences in a quantitative, continuous response variable, such as length of stay. Logistic regression predicts differences in a dichotomous, categorical response variable, such as 90-day readmission. So whether the outcome variable is categorical or quantitative, regression can be appropriate. An example for each of these types could be found in two similar cases. For both examples define the predictor variables as age, gender and anticoagulant usage. In the first, use the predictor variables in a linear regression to evaluate their individual effects on length of stay, a quantitative variable. For the second, use the same predictor variables in a logistic regression to evaluate their individual effects on whether the patient had a 90-day readmission, a dichotomous categorical variable. Analysis can compute a p-value for each included predictor variable to determine whether they are significantly associated. The statistical tests in this article generate an associated test statistic which determines the probability the results could be acquired given that there is no association between the compared variables. These results often come with coefficients which can give the degree of the association and the degree to which one variable changes with another. Most tests, including all listed in this article, also have confidence intervals, which give a range for the correlation with a specified level of confidence. Even if these tests do not give statistically significant results, the results are still important. Not reporting statistically insignificant findings creates a bias in research. Ideas can be repeated enough times that eventually statistically significant results are reached, even though there is no true significance. In some cases with very large sample sizes, p-values will almost always be significant. In this case the effect size is critical as even the smallest, meaningless differences can be found to be statistically significant.
These variables and tests are just some things to keep in mind before, during and after the analysis process in order to make sure that the statistical reports are supporting the questions being answered. The patient population, types of variables and statistical tests are all important things to consider in the process of statistical analysis. Any results are only as useful as the process used to obtain them. This primer can be used as a reference to help ensure appropriate statistical analysis.
Funding Statement
This research was supported (in whole or in part) by HCA Healthcare and/or an HCA Healthcare affiliated entity.
Conflicts of Interest
The author declares he has no conflicts of interest.
Christian Vandever is an employee of HCA Healthcare Graduate Medical Education, an organization affiliated with the journal’s publisher.
This research was supported (in whole or in part) by HCA Healthcare and/or an HCA Healthcare affiliated entity. The views expressed in this publication represent those of the author(s) and do not necessarily represent the official views of HCA Healthcare or any of its affiliated entities.

05-01-2024 · Interview
New research into the stock-bond correlation shows when they correlate – and when they don’t
Recent research investigates the impact of macroeconomic variables like inflation, real interest rates and government creditworthiness on the correlation between stocks and bonds.

- Laurens Swinkels
Head of Quant Strategy
Top keywords
- Academic journal article
- Asset allocation
- Government bonds
- Macro economy
- Quantitative investing
Robeco’s Laurens Swinkels and his co-authors, Roderick Molenaar, Edouard Sénéchal, 1 and Zhenping Wang, 2 conducted a comprehensive study on the correlation between stock and bond returns, crucial for asset allocation decisions. The findings, which indicate significant shifts in correlation across different periods, offer valuable insights for managing multi-asset portfolios and understanding bond risk premiums in various macroeconomic contexts. We sat down with Laurens to discuss the findings.
Could you tell us about what motivated the research and its importance for asset owners and managers?
“As a multi-asset investor, understanding the total risk of your portfolio requires knowledge of how the asset classes you invest in correlate with each other. The primary asset classes for multi-asset investors are bonds and equities, so understanding the correlation between these two is crucial. We’ve observed periods where this correlation is negative, which is beneficial because one asset class can act as a hedge against the other, but there are also periods where the correlation is positive, meaning both asset classes move in the same direction simultaneously. These situations increase the risk of a multi-asset portfolio.” “Because we manage multi-asset strategies, we need to manage risks effectively. This includes both the total benchmark risk and the risks associated with taking active positions for tactical asset allocation. It's important to know whether being long in equities and short in bonds is partially hedging or reinforcing the position – understanding the factors influencing the stock-bond correlation was a key driver for our research.” “Another question we explored was related to the expected return of bonds. If bonds are an effective hedge against equity risk, then owning bonds should be favorable, as they protect your portfolio from the primary risk faced by many investors. Consequently, the demand for bonds would increase, potentially raising their price, but conversely this could imply lower forward returns for bonds. In scenarios where the stock-bond correlation is negative, theory suggests that one shouldn’t expect too high excess returns from investing in bonds, as they become an attractive asset class for mitigating risk.”
What were your key findings?
“We had data going back to 1875 for the US and even further for the UK, with our findings showing different regimes in stock-bond correlation over time. Before 1952, we found limited explanatory power but post-1952, inflation and real interest rates emerged as significantly determining the correlation between the two asset classes. This is likely driven by more counter-cyclical central bank monetary policies. High real interest rates or inflation levels tend to coincide with positive stock-bond correlation, which is not favorable for multi-asset investors looking to reduce risk by investing in both asset classes.” “During our actual research, which began before the inflation spike in 2020-2023, we saw prolonged periods where stock and bond markets declined simultaneously, making bonds ineffective as a hedge. This real-time observation backed up our findings from historical data, showing how important these factors are. While our research was initially historical, our research took on a practical dimension through experiencing these inflationary periods, which really underlines the relevance of these discoveries.” “Importantly, instead of trying to directly predict the stock-bond correlation, our approach focuses more on understanding the macroeconomic situation, where inflation and interest rates play significant roles. If our predictions about the macroeconomic environment are incorrect, then our assumptions about the stock-bond correlation will likely be incorrect as well. However, in scenarios where we anticipate inflation to be under 3% and real interest rates to be normal, we expect the stock-bond correlation to be negative.” “We explored the implications of the correlations on multi-asset portfolio risks (see Figure 1 below) and found that the 60/40 stock/bond portfolio's volatility fluctuates significantly with these correlations—showing a decrease from about 10.5% to 8.4% as correlations shifted from positive (+0.35) in the period 1970–1999 to negative (-0.29) in 2000–2023. This change suggests that investors might need to adjust their equity holdings downwards during periods of positive correlations to maintain a consistent risk level.”
Figure 1: Multi-asset portfolio risk and return for different stock-bond correlations
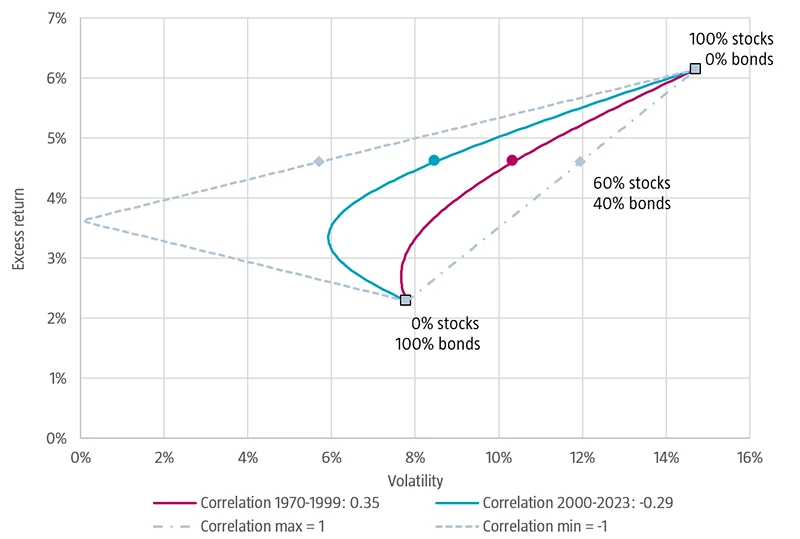
Source: Authors. Note: Authors’ average standard deviation and excess returns from January 1970 to June 2023. Pearson correlation coefficient of monthly returns computed between January 1970 and December 1999 and between January 2000 and June 2023.
“Our findings also reinforce the importance of macroeconomic variables in forecasting stock-bond correlations, crucial for managing cross-asset risks. For example, our empirical model indicates that a 1% increase in both inflation and real rates results in a 0.17 increase in the correlation between stocks and bonds. In turn, this can lead to an increase of 0.8% to 1.7% in the risk of a 60/40 portfolio, depending on the starting stock–bond correlation (see Figure 2).”
Figure 2: Relation between stock-bond correlation and portfolio risk
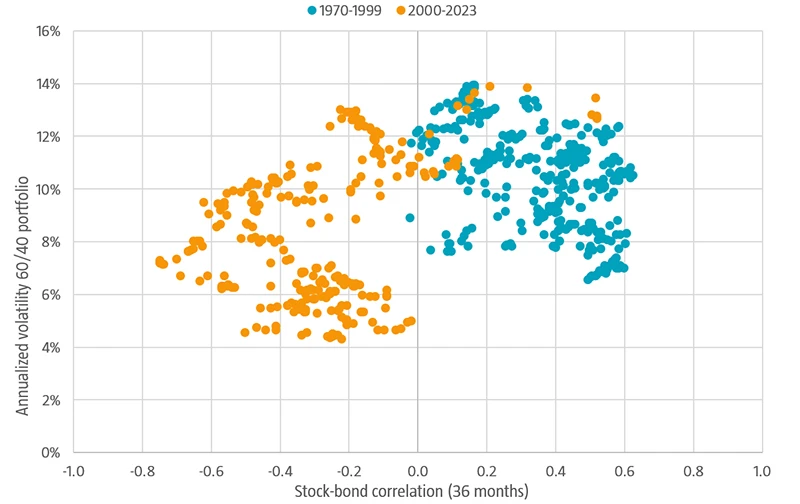
Source: Authors. Note: Average of standard deviation and Pearson correlation coefficient of monthly returns computer over 36-month rolling windows ending January 19070 to June 2023.
“In addition, we discuss how a higher stock-bond correlation could necessitate increased bond risk premiums, as demonstrated by historical data and regression analyses linking higher premiums to periods of stronger stock-bond co-movements. This may be an indication that the CAPM has a stronger empirical basis across than within asset classes.” “Based on this understanding, you can then estimate your portfolio risk. We also take these findings into account when we construct our macro scenarios in our Five-year Expected Returns. ”
Did your findings differ across markets?
“We wanted to explore how the stock-bond correlation behaves across different countries, particularly in relation to the concept of 'safe havens'. And we found that in countries considered safe havens, stock and bond correlations can fluctuate between positive and negative regimes. However, in countries not regarded as safe havens, the correlation tends to be more consistently positive. This comes from the fact that the 'flight to safety' effect, often observed in bonds during periods of market stress, is not as pronounced. Much of the existing research is US-based and highlights this effect, but our study shows that this phenomenon doesn't automatically apply to all countries.” “For instance, during the Eurozone crisis, Italian stocks and government bonds showed a positive correlation, and in emerging markets, we often observed a positive correlation, suggesting the absence of a significant flight to safety effect from government bonds. This international dimension to our research challenges the assumption that findings based on US markets are universally applicable.”
Can you share how your collaboration with the analysts at State of Wisconsin Investment Board (SWIB) came about and evolved into the project it is today?
“Through serendipity and mutual interest in financial research! It's important to note that our primary aim wasn’t the publication of a paper: the project team, comprising Roderick Molenaar, myself, and Edouard and Zhenping, two analysts from SWIB, happened to want to answer the same question. This led to an ongoing exchange of ideas, data collection and analyses, and critical questions from each side of the ocean to the other.” “As the project progressed, we recognized the broader value of our findings and decided to distill those insights into a comprehensive paper. In the end our manuscript was accepted for publication in the Financial Analysts Journal, underscoring for me the pleasure and importance of collaboration.” “It was fascinating that, despite potential differences in perspectives between asset managers and other investors like pension funds, we shared the same research question in this instance. The partnership encouraged us to question whether our standard methods were indeed the only way, or if there could be alternative, perhaps more effective approaches.” “While we focused more on the risk aspect of our total portfolio and SWIB on expected bond returns—the underlying question remained constant: What drives the stock-bond correlation?”
1 From the State of Wisconsin Investment Board 2 From the State of Wisconsin Investment Board
Related topics

Clarifying the cellular mechanisms underlying periodontitis with an improved animal model
Periodontal disease, represented by periodontitis, is the leading cause of tooth loss and affects close to one in five adults worldwide. In most cases, this condition occurs as a result of an inflammatory response to bacterial infection of the tissue around teeth. As the condition worsens, the gums begin to pull away, exposing teeth roots and bone. Notably, the incidence of periodontitis becomes more prevalent with age and with populations worldwide living longer, developing a solid understanding of its underlying causes and progression is important.
In a study recently published in Nature Communications on March 28, 2024, researchers from Tokyo Medical and Dental University (TMDU) found a way to achieve this by improving upon a widely used animal model to study periodontitis.
Studying periodontitis directly in humans is challenging. As a result, scientists often resort to animal models for preclinical research. For instance, the "mouse ligature-induced periodontitis model," since its inception in 2012, has enabled researchers to study the cellular mechanisms underlying this condition. Simply put, with this model, periodontal disease is artificially induced by ligating silk threads onto the molars of mice models, which induces plaque accumulation. While convenient and effective, this model, however, fails to capture the complete picture of periodontitis. "Even though the periodontal tissue is composed of gingiva, periodontal ligament, alveolar bone, and cementum, analyses are usually performed exclusively on gingival samples due to technical and quantitative limitations," remarks lead author Mr. Anhao Liu. "This sampling strategy limits the conclusions that may be drawn from these studies, so methods that allow for the simultaneous analysis of all tissue components are needed."
To address this limitation, the research team developed a modified ligature-induced periodontitis model. Instead of the classic single ligature, they used a triple ligature approach on the upper left molar of male mice. This strategy expanded the range of bone loss without causing severe bone destruction around the second molar, increasing the yield of the different types of periodontal tissue. "We isolated the three main tissue types and evaluated the RNA yield between the two models. The results showed that the triple-ligature model effectively increased the yield, achieving four times the yield of normal peri-root tissue and supporting the high-resolution analysis of different tissue types," explains senior author Dr. Mikihito Hayashi.
After confirming the efficacy of their modified model, the researchers proceeded to investigate the effects of periodontitis on gene expression among the different tissue types over time, focusing on genes related to inflammation and osteoclast differentiation. One of their main findings was that the expression of the Il1rl1 gene was markedly higher in peri-root tissue five days after ligation. This gene encodes the protein ST2 in both receptor and decoy isoform, which binds to a cytokine called IL-33 that is involved in inflammatory and immunoregulation processes.
To gain further insights into the role of this gene, the team induced periodontitis in genetically modified mice that lacked the Il1rl1 or Il33 genes. These mice exhibited accelerated inflammatory bone destruction, highlighting the protective role of the IL-33/ST2 pathway. Further analysis of cells containing the ST2 protein in its receptor form, mST2, revealed that most of them were of macrophage lineage. "Macrophages are typically classified into two main types, pro-inflammatory and anti-inflammatory, based on their activation process. We found that mST2-expressing cells were unique in that they expressed some markers of both types of macrophages simultaneously," comments senior author Dr. Takanori Iwata. "These cells were present in the peri-root tissue before inflammation was triggered, so we named them 'periodontal tissue-resident macrophages.'"
Together, the findings of this study showcase the power of this modified animal model to study the full scope of periodontitis in greater detail, right down to the biomolecular level. "We suggest the possibility that a novel IL-33/ST2 molecular pathway regulating inflammation and bone destruction in periodontal disease, alongside specific macrophages in peri-root tissue, is deeply involved in periodontal disease. This will hopefully lead to the development of new treatment strategies and prevention methods," concludes senior author Dr. Tomoki Nakashima.
- Bone and Spine
- Biotechnology and Bioengineering
- Microbiology
- Periodontal disease
- Adipose tissue
- House mouse
- Biological tissue
- Blood vessel
- Global climate model
Story Source:
Materials provided by Tokyo Medical and Dental University . Note: Content may be edited for style and length.
Journal Reference :
- Anhao Liu, Mikihito Hayashi, Yujin Ohsugi, Sayaka Katagiri, Shizuo Akira, Takanori Iwata, Tomoki Nakashima. The IL-33/ST2 axis is protective against acute inflammation during the course of periodontitis . Nature Communications , 2024; 15 (1) DOI: 10.1038/s41467-024-46746-2
Cite This Page :
Explore More
- Stopping Flu Before It Takes Hold
- Cosmic Rays Illuminate the Past
- Star Suddenly Vanish from the Night Sky
- Dinosaur Feather Evolution
- Warming Climate: Flash Droughts Worldwide
- Record Low Antarctic Sea Ice: Climate Change
- Brain 'Assembloids' Mimic Blood-Brain Barrier
- 'Doomsday' Glacier: Catastrophic Melting
- Blueprints of Self-Assembly
- Meerkat Chit-Chat
Trending Topics
Strange & offbeat.
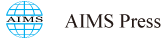
- {{subColumn.name}}
AIMS Mathematics

- {{newsColumn.name}}
- Share facebook twitter google linkedin
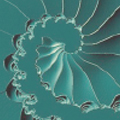
Almost sure convergence for a class of dependent random variables under sub-linear expectations
- Baozhen Wang 1,2 ,
- Qunying Wu 1,2 , ,
- 1. School of Mathematics and Statistics, Guilin University of Technology, Guilin 541006, China
- 2. Guangxi Colleges and Universities Key Laboratory of Applied Statistics, Guilin 541004, China
- Received: 06 February 2024 Revised: 05 April 2024 Accepted: 14 May 2024 Published: 20 May 2024
MSC : 60F15
- Full Text(HTML)
- Download PDF
This article aimed to investigate the almost sure convergence theorem of widely negative orthant dependent (WNOD) random variables under sub-linear expectation space. The conclusions in this essay are an extension of the corresponding conclusions in the classical probability space.
- widely negative orthant dependent ,
- almost sure convergence ,
- sub-linear expectation
Citation: Baozhen Wang, Qunying Wu. Almost sure convergence for a class of dependent random variables under sub-linear expectations[J]. AIMS Mathematics, 2024, 9(7): 17259-17275. doi: 10.3934/math.2024838
Related Papers:
- This work is licensed under a Creative Commons Attribution-NonCommercial-Share Alike 4.0 Unported License. To view a copy of this license, visit http://creativecommons.org/licenses/by-nc-sa/4.0/ -->
Supplements
Access history.
- Corresponding author: Email: [email protected] ; Tel: +86-15877031406
Reader Comments
- © 2024 the Author(s), licensee AIMS Press. This is an open access article distributed under the terms of the Creative Commons Attribution License ( http://creativecommons.org/licenses/by/4.0 )
通讯作者: 陈斌, [email protected]
沈阳化工大学材料科学与工程学院 沈阳 110142
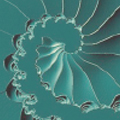
Article views( 53 ) PDF downloads( 5 ) Cited by( 0 )
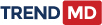
Associated material
Other articles by authors.
- Baozhen Wang
Related pages
- on Google Scholar
- Email to a friend
- Order reprints
Export File


IMAGES
VIDEO
COMMENTS
Generate the conclusion outline: After entering all necessary details, click on 'generate'. Paperpal will then create a structured outline for your conclusion, to help you start writing and build upon the outline. Write your conclusion: Use the generated outline to build your conclusion.
The conclusion is intended to help the reader understand why your research should matter to them after they have finished reading the paper. A conclusion is not merely a summary of the main topics covered or a re-statement of your research problem, but a synthesis of key points derived from the findings of your study and, if applicable, where you recommend new areas for future research.
Table of contents. Step 1: Restate the problem. Step 2: Sum up the paper. Step 3: Discuss the implications. Research paper conclusion examples. Frequently asked questions about research paper conclusions.
Here are some steps you can follow to write an effective research paper conclusion: Restate the research problem or question: Begin by restating the research problem or question that you aimed to answer in your research. This will remind the reader of the purpose of your study. Summarize the main points: Summarize the key findings and results ...
Dependent Variable The variable that depends on other factors that are measured. These variables are expected to change as a result of an experimental manipulation of the independent variable or variables. It is the presumed effect. Independent Variable The variable that is stable and unaffected by the other variables you are trying to measure.
The Role of Variables in Research. In scientific research, variables serve several key functions: Define Relationships: Variables allow researchers to investigate the relationships between different factors and characteristics, providing insights into the underlying mechanisms that drive phenomena and outcomes. Establish Comparisons: By manipulating and comparing variables, scientists can ...
These are precise and typically linked to the subject population, dependent and independent variables, and research design.1 Research questions may also attempt to describe the behavior of a population in relation to one or more variables, ... CONCLUSION. Research questions and hypotheses are crucial components to any type of research, whether ...
Drawing Conclusions. Since statistics are probabilistic in nature and findings can reflect type I or type II errors, we cannot use the results of a single study to conclude with certainty that a theory is true. Rather theories are supported, refuted, or modified based on the results of research. If the results are statistically significant and ...
Begin with a clear statement of the principal findings. This will reinforce the main take-away for the reader and set up the rest of the discussion. Explain why the outcomes of your study are important to the reader. Discuss the implications of your findings realistically based on previous literature, highlighting both the strengths and ...
This structure is commonly adopted and accepted in the scientific fields. The research report starts with a general idea. The report then leads the reader to a discussion on a specific research area. It then ends with applicability to a bigger area. The last section, Conclusion, is the focus of this lesson.
Statistical thinking involves the careful design of a study to collect meaningful data to answer a focused research question, detailed analysis of patterns in the data, and drawing conclusions that go beyond the observed data. Random sampling is paramount to generalizing results from our sample to a larger population, and random assignment is ...
So, it is usual for research protocols to include many independent variables and many dependent variables in the generation of many hypotheses, as shown in Table 1. Pairing each variable in the "independent variable" column with each variable in the "dependent variable" column would result in the generation of these hypotheses.
The independent variable is the cause. Its value is independent of other variables in your study. The dependent variable is the effect. Its value depends on changes in the independent variable. Example: Independent and dependent variables. You design a study to test whether changes in room temperature have an effect on math test scores.
The quality of research designs can be defined in terms of four key design attributes: internal validity, external validity, construct validity, and statistical conclusion validity. Internal validity, also called causality, examines whether the observed change in a dependent variable is indeed caused by a corresponding change in hypothesized ...
Siddharth Kalla 140.9K reads. It is very important to understand relationship between variables to draw the right conclusion from a statistical analysis. The relationship between variables determines how the right conclusions are reached. Without an understanding of this, you can fall into many pitfalls that accompany statistical analysis and ...
Most Conclusions were found to restate purpose, consolidate research space with a varied array of steps, recommend future research and cover practical applications, implications or recommendations. ... variables , research design process, types of sampling, data collection process, interviews, questionnaires, data analysis techniques and so on ...
The fourth aspect of research validity, which Cook and Campbell called statistical conclusion validity (SCV), is the subject of this paper. Cook and Campbell, 1979 , pp. 39-50) discussed that SCV pertains to the extent to which data from a research study can reasonably be regarded as revealing a link (or lack thereof) between independent and ...
Extraneous variables can lead to erroneous conclusions and can be controlled through random assignment or statistical techniques. ... Scientific research: Variables are used in scientific research to understand the relationships between different factors and to make predictions about future outcomes. For example, scientists may study the ...
Understanding and identifying categorical variables is crucial in research as it influences the choice of statistical analysis methods. Since these variables represent categories without numerical significance, researchers employ specific statistical tests designed for a nominal or ordinal variable to draw meaningful conclusions.
A research design is a strategy for answering your research question using empirical data. Creating a research design means making decisions about: Your overall research objectives and approach. Whether you'll rely on primary research or secondary research. Your sampling methods or criteria for selecting subjects. Your data collection methods.
The purpose of research is to describe and explain variance in the world, that is, variance that. occurs naturally in the world or chang e that we create due to manipulation. Variables are ...
Conclusion. In conclusion, variables play a crucial role in research across different fields and disciplines. They help to define research questions, design studies, analyze data, and draw conclusions. By understanding the importance of variables in research, researchers can design studies that are relevant, accurate, and reliable, and can ...
Qualitative research is a method focused on understanding human behavior and experiences through non-numerical data. Examples of qualitative research include: One-on-one interviews, Focus groups, Ethnographic research, Case studies, Record keeping, Qualitative observations. In this article, we'll provide tips and tricks on how to use ...
Background and aims Obesity and overweight have become significant health concerns worldwide, leading to an increased interest in finding natural remedies for weight reduction. One such remedy that has gained popularity is apple cider vinegar (ACV). Objective To investigate the effects of ACV consumption on weight, blood glucose, triglyceride and cholesterol levels in a sample of the Lebanese ...
Background: Persons with disabilities often face various forms of victimization, yet there is limited research exploring this phenomenon in Bangladesh. This study aims to investigate the victimization status among persons with disabilities and identify its predictors. Methods: Data of 4293 persons with disabilities analyzed in this study were extracted from the 2021 National Survey on Persons ...
Scientists at GFDL have developed a novel variable-resolution global chemistry-climate model, known as AM4VR, for research at the nexus of U.S. climate and air quality extremes. In contrast with the global models contributing to the latest Intergovernmental Panel on Climate Change Report, this model features more than 10 times finer spatial ...
Introduction. Statistical analysis is necessary for any research project seeking to make quantitative conclusions. The following is a primer for research-based statistical analysis. It is intended to be a high-level overview of appropriate statistical testing, while not diving too deep into any specific methodology.
Recent research investigates the impact of macroeconomic variables like inflation, real interest rates and government creditworthiness on the correlation between stocks and bonds. Insights ... "During our actual research, which began before the inflation spike in 2020-2023, we saw prolonged periods where stock and bond markets declined ...
from research organizations. ... "This sampling strategy limits the conclusions that may be drawn from these studies, so methods that allow for the simultaneous analysis of all tissue components ...
2. This article aimed to investigate the almost sure convergence theorem of widely negative orthant dependent (WNOD) random variables under sub-linear expectation space. The conclusions in this essay are an extension of the corresponding conclusions in the classical probability space. Citation: Baozhen Wang, Qunying Wu.