

Textual Analysis: Definition, Types & 10 Examples
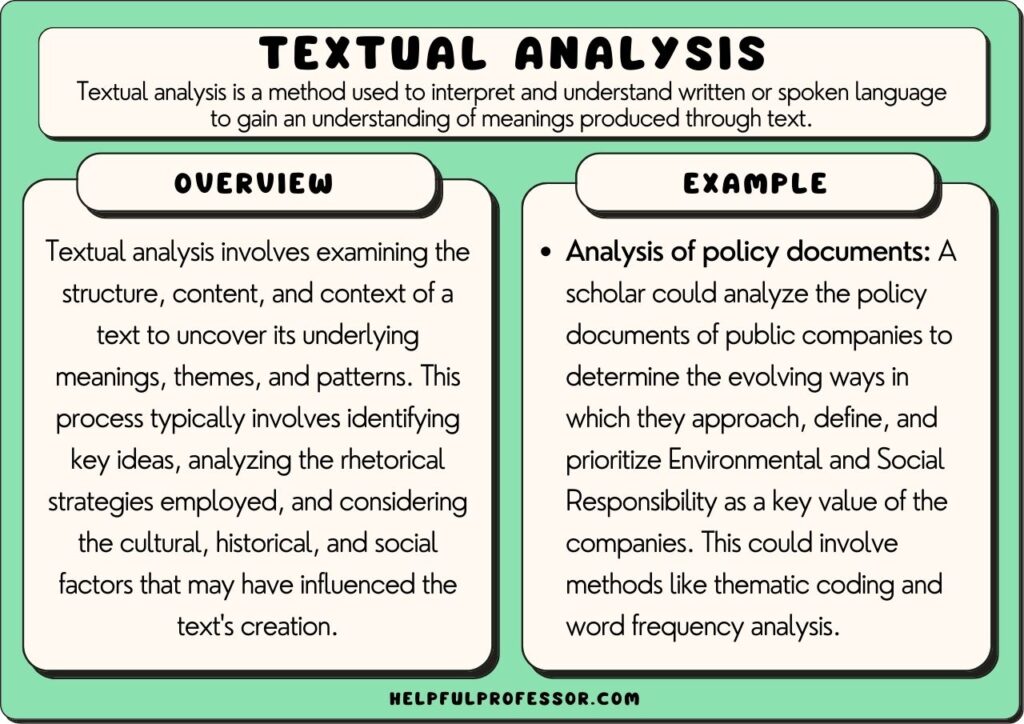
Textual analysis is a research methodology that involves exploring written text as empirical data. Scholars explore both the content and structure of texts, and attempt to discern key themes and statistics emergent from them.
This method of research is used in various academic disciplines, including cultural studies, literature, bilical studies, anthropology , sociology, and others (Dearing, 2022; McKee, 2003).
This method of analysis involves breaking down a text into its constituent parts for close reading and making inferences about its context, underlying themes, and the intentions of its author.
Textual Analysis Definition
Alan McKee is one of the preeminent scholars of textual analysis. He provides a clear and approachable definition in his book Textual Analysis: A Beginner’s Guide (2003) where he writes:
“When we perform textual analysis on a text we make an educated guess at some of the most likely interpretations that might be made of the text […] in order to try and obtain a sense of the ways in which, in particular cultures at particular times, people make sense of the world around them.”
A key insight worth extracting from this definition is that textual analysis can reveal what cultural groups value, how they create meaning, and how they interpret reality.
This is invaluable in situations where scholars are seeking to more deeply understand cultural groups and civilizations – both past and present (Metoyer et al., 2018).
As such, it may be beneficial for a range of different types of studies, such as:
- Studies of Historical Texts: A study of how certain concepts are framed, described, and approached in historical texts, such as the Bible.
- Studies of Industry Reports: A study of how industry reports frame and discuss concepts such as environmental and social responsibility.
- Studies of Literature: A study of how a particular text or group of texts within a genre define and frame concepts. For example, you could explore how great American literature mythologizes the concept of the ‘The American Dream’.
- Studies of Speeches: A study of how certain politicians position national identities in their appeals for votes.
- Studies of Newspapers: A study of the biases within newspapers toward or against certain groups of people.
- Etc. (For more, see: Dearing, 2022)
McKee uses the term ‘textual analysis’ to also refer to text types that are not just written, but multimodal. For a dive into the analysis of multimodal texts, I recommend my article on content analysis , where I explore the study of texts like television advertisements and movies in detail.
Features of a Textual Analysis
When conducting a textual analysis, you’ll need to consider a range of factors within the text that are worthy of close examination to infer meaning. Features worthy of considering include:
- Content: What is being said or conveyed in the text, including explicit and implicit meanings, themes, or ideas.
- Context: When and where the text was created, the culture and society it reflects, and the circumstances surrounding its creation and distribution.
- Audience: Who the text is intended for, how it’s received, and the effect it has on its audience.
- Authorship: Who created the text, their background and perspectives, and how these might influence the text.
- Form and structure: The layout, sequence, and organization of the text and how these elements contribute to its meanings (Metoyer et al., 2018).
Textual Analysis Coding Methods
The above features may be examined through quantitative or qualitative research designs , or a mixed-methods angle.
1. Quantitative Approaches
You could analyze several of the above features, namely, content, form, and structure, from a quantitative perspective using computational linguistics and natural language processing (NLP) analysis.
From this approach, you would use algorithms to extract useful information or insights about frequency of word and phrase usage, etc. This can include techniques like sentiment analysis, topic modeling, named entity recognition, and more.
2. Qualitative Approaches
In many ways, textual analysis lends itself best to qualitative analysis. When identifying words and phrases, you’re also going to want to look at the surrounding context and possibly cultural interpretations of what is going on (Mayring, 2015).
Generally, humans are far more perceptive at teasing out these contextual factors than machines (although, AI is giving us a run for our money).
One qualitative approach to textual analysis that I regularly use is inductive coding, a step-by-step methodology that can help you extract themes from texts. If you’re interested in using this step-by-step method, read my guide on inductive coding here .
See more Qualitative Research Approaches Here
Textual Analysis Examples
Title: “Discourses on Gender, Patriarchy and Resolution 1325: A Textual Analysis of UN Documents” Author: Nadine Puechguirbal Year: 2010 APA Citation: Puechguirbal, N. (2010). Discourses on Gender, Patriarchy and Resolution 1325: A Textual Analysis of UN Documents, International Peacekeeping, 17 (2): 172-187. doi: 10.1080/13533311003625068
Summary: The article discusses the language used in UN documents related to peace operations and analyzes how it perpetuates stereotypical portrayals of women as vulnerable individuals. The author argues that this language removes women’s agency and keeps them in a subordinate position as victims, instead of recognizing them as active participants and agents of change in post-conflict environments. Despite the adoption of UN Security Council Resolution 1325, which aims to address the role of women in peace and security, the author suggests that the UN’s male-dominated power structure remains unchallenged, and gender mainstreaming is often presented as a non-political activity.
Title: “Racism and the Media: A Textual Analysis” Author: Kassia E. Kulaszewicz Year: 2015 APA Citation: Kulaszewicz, K. E. (2015). Racism and the Media: A Textual Analysis . Dissertation. Retrieved from: https://sophia.stkate.edu/msw_papers/477
Summary: This study delves into the significant role media plays in fostering explicit racial bias. Using Bandura’s Learning Theory, it investigates how media content influences our beliefs through ‘observational learning’. Conducting a textual analysis, it finds differences in representation of black and white people, stereotyping of black people, and ostensibly micro-aggressions toward black people. The research highlights how media often criminalizes Black men, portraying them as violent, while justifying or supporting the actions of White officers, regardless of their potential criminality. The study concludes that news media likely continues to reinforce racism, whether consciously or unconsciously.
Title: “On the metaphorical nature of intellectual capital: a textual analysis” Author: Daniel Andriessen Year: 2006 APA Citation: Andriessen, D. (2006). On the metaphorical nature of intellectual capital: a textual analysis. Journal of Intellectual capital , 7 (1), 93-110.
Summary: This article delves into the metaphorical underpinnings of intellectual capital (IC) and knowledge management, examining how knowledge is conceptualized through metaphors. The researchers employed a textual analysis methodology, scrutinizing key texts in the field to identify prevalent metaphors. They found that over 95% of statements about knowledge are metaphor-based, with “knowledge as a resource” and “knowledge as capital” being the most dominant. This study demonstrates how textual analysis helps us to understand current understandings and ways of speaking about a topic.
Title: “Race in Rhetoric: A Textual Analysis of Barack Obama’s Campaign Discourse Regarding His Race” Author: Andrea Dawn Andrews Year: 2011 APA Citation: Andrew, A. D. (2011) Race in Rhetoric: A Textual Analysis of Barack Obama’s Campaign Discourse Regarding His Race. Undergraduate Honors Thesis Collection. 120 . https://digitalcommons.butler.edu/ugtheses/120
This undergraduate honors thesis is a textual analysis of Barack Obama’s speeches that explores how Obama frames the concept of race. The student’s capstone project found that Obama tended to frame racial inequality as something that could be overcome, and that this was a positive and uplifting project. Here, the student breaks-down times when Obama utilizes the concept of race in his speeches, and examines the surrounding content to see the connotations associated with race and race-relations embedded in the text. Here, we see a decidedly qualitative approach to textual analysis which can deliver contextualized and in-depth insights.
Sub-Types of Textual Analysis
While above I have focused on a generalized textual analysis approach, a range of sub-types and offshoots have emerged that focus on specific concepts, often within their own specific theoretical paradigms. Each are outlined below, and where I’ve got a guide, I’ve linked to it in blue:
- Content Analysis : Content analysis is similar to textual analysis, and I would consider it a type of textual analysis, where it’s got a broader understanding of the term ‘text’. In this type, a text is any type of ‘content’, and could be multimodal in nature, such as television advertisements, movies, posters, and so forth. Content analysis can be both qualitative and quantitative, depending on whether it focuses more on the meaning of the content or the frequency of certain words or concepts (Chung & Pennebaker, 2018).
- Discourse Analysis : Emergent specifically from critical and postmodern/ poststructural theories, discourse analysis focuses closely on the use of language within a social context, with the goal of revealing how repeated framing of terms and concepts has the effect of shaping how cultures understand social categories. It considers how texts interact with and shape social norms, power dynamics, ideologies, etc. For example, it might examine how gender is socially constructed as a distinct social category through Disney films. It may also be called ‘critical discourse analysis’.
- Narrative Analysis: This approach is used for analyzing stories and narratives within text. It looks at elements like plot, characters, themes, and the sequence of events to understand how narratives construct meaning.
- Frame Analysis: This approach looks at how events, ideas, and themes are presented or “framed” within a text. It explores how these frames can shape our understanding of the information being presented. While similar to discourse analysis, a frame analysis tends to be less associated with the loaded concept of ‘discourse’ that exists specifically within postmodern paradigms (Smith, 2017).
- Semiotic Analysis: This approach studies signs and symbols, both visual and textual, and could be a good compliment to a content analysis, as it provides the language and understandings necessary to describe how signs make meaning in cultural contexts that we might find with the fields of semantics and pragmatics . It’s based on the theory of semiotics, which is concerned with how meaning is created and communicated through signs and symbols.
- Computational Textual Analysis: In the context of data science or artificial intelligence, this type of analysis involves using algorithms to process large amounts of text. Techniques can include topic modeling, sentiment analysis, word frequency analysis, and others. While being extremely useful for a quantitative analysis of a large dataset of text, it falls short in its ability to provide deep contextualized understandings of words-in-context.
Each of these methods has its strengths and weaknesses, and the choice of method depends on the research question, the type of text being analyzed, and the broader context of the research.
See More Examples of Analysis Here
Strengths and Weaknesses of Textual Analysis
When writing your methodology for your textual analysis, make sure to define not only what textual analysis is, but (if applicable) the type of textual analysis, the features of the text you’re analyzing, and the ways you will code the data. It’s also worth actively reflecting on the potential weaknesses of a textual analysis approach, but also explaining why, despite those weaknesses, you believe this to be the most appropriate methodology for your study.
Chung, C. K., & Pennebaker, J. W. (2018). Textual analysis. In Measurement in social psychology (pp. 153-173). Routledge.
Dearing, V. A. (2022). Manual of textual analysis . Univ of California Press.
McKee, A. (2003). Textual analysis: A beginner’s guide. Textual analysis , 1-160.
Mayring, P. (2015). Qualitative content analysis: Theoretical background and procedures. Approaches to qualitative research in mathematics education: Examples of methodology and methods , 365-380. doi: https://doi.org/10.1007/978-94-017-9181-6_13
Metoyer, R., Zhi, Q., Janczuk, B., & Scheirer, W. (2018, March). Coupling story to visualization: Using textual analysis as a bridge between data and interpretation. In 23rd International Conference on Intelligent User Interfaces (pp. 503-507). doi: https://doi.org/10.1145/3172944.3173007
Smith, J. A. (2017). Textual analysis. The international encyclopedia of communication research methods , 1-7.
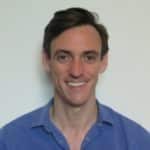
Chris Drew (PhD)
Dr. Chris Drew is the founder of the Helpful Professor. He holds a PhD in education and has published over 20 articles in scholarly journals. He is the former editor of the Journal of Learning Development in Higher Education. [Image Descriptor: Photo of Chris]
- Chris Drew (PhD) https://helpfulprofessor.com/author/chris-drew-phd/ 15 Animism Examples
- Chris Drew (PhD) https://helpfulprofessor.com/author/chris-drew-phd/ 10 Magical Thinking Examples
- Chris Drew (PhD) https://helpfulprofessor.com/author/chris-drew-phd/ Social-Emotional Learning (Definition, Examples, Pros & Cons)
- Chris Drew (PhD) https://helpfulprofessor.com/author/chris-drew-phd/ What is Educational Psychology?
Leave a Comment Cancel Reply
Your email address will not be published. Required fields are marked *
Have a language expert improve your writing
Run a free plagiarism check in 10 minutes, automatically generate references for free.
- Knowledge Base
- Dissertation
- What Is a Research Methodology? | Steps & Tips
What Is a Research Methodology? | Steps & Tips
Published on 25 February 2019 by Shona McCombes . Revised on 10 October 2022.
Your research methodology discusses and explains the data collection and analysis methods you used in your research. A key part of your thesis, dissertation, or research paper, the methodology chapter explains what you did and how you did it, allowing readers to evaluate the reliability and validity of your research.
It should include:
- The type of research you conducted
- How you collected and analysed your data
- Any tools or materials you used in the research
- Why you chose these methods
- Your methodology section should generally be written in the past tense .
- Academic style guides in your field may provide detailed guidelines on what to include for different types of studies.
- Your citation style might provide guidelines for your methodology section (e.g., an APA Style methods section ).
Instantly correct all language mistakes in your text
Be assured that you'll submit flawless writing. Upload your document to correct all your mistakes.
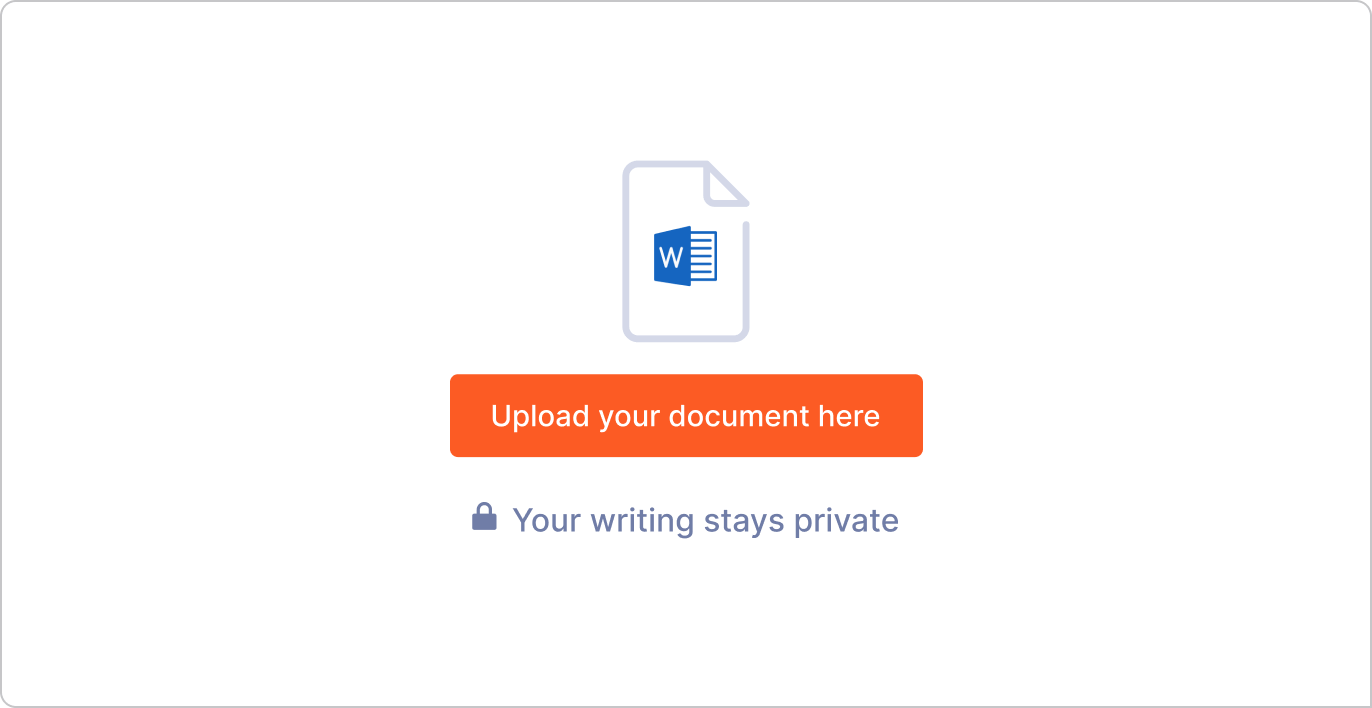
Table of contents
How to write a research methodology, why is a methods section important, step 1: explain your methodological approach, step 2: describe your data collection methods, step 3: describe your analysis method, step 4: evaluate and justify the methodological choices you made, tips for writing a strong methodology chapter, frequently asked questions about methodology.
The only proofreading tool specialized in correcting academic writing
The academic proofreading tool has been trained on 1000s of academic texts and by native English editors. Making it the most accurate and reliable proofreading tool for students.
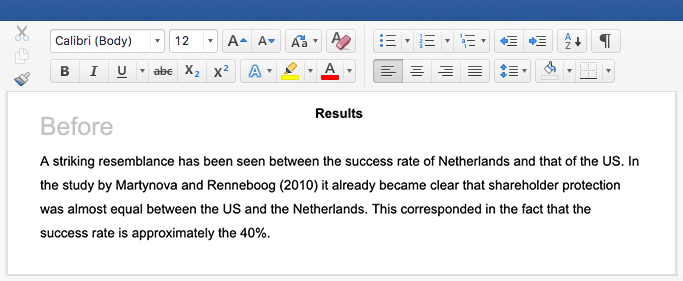
Correct my document today
Your methods section is your opportunity to share how you conducted your research and why you chose the methods you chose. It’s also the place to show that your research was rigorously conducted and can be replicated .
It gives your research legitimacy and situates it within your field, and also gives your readers a place to refer to if they have any questions or critiques in other sections.
You can start by introducing your overall approach to your research. You have two options here.
Option 1: Start with your “what”
What research problem or question did you investigate?
- Aim to describe the characteristics of something?
- Explore an under-researched topic?
- Establish a causal relationship?
And what type of data did you need to achieve this aim?
- Quantitative data , qualitative data , or a mix of both?
- Primary data collected yourself, or secondary data collected by someone else?
- Experimental data gathered by controlling and manipulating variables, or descriptive data gathered via observations?
Option 2: Start with your “why”
Depending on your discipline, you can also start with a discussion of the rationale and assumptions underpinning your methodology. In other words, why did you choose these methods for your study?
- Why is this the best way to answer your research question?
- Is this a standard methodology in your field, or does it require justification?
- Were there any ethical considerations involved in your choices?
- What are the criteria for validity and reliability in this type of research ?
Once you have introduced your reader to your methodological approach, you should share full details about your data collection methods .
Quantitative methods
In order to be considered generalisable, you should describe quantitative research methods in enough detail for another researcher to replicate your study.
Here, explain how you operationalised your concepts and measured your variables. Discuss your sampling method or inclusion/exclusion criteria, as well as any tools, procedures, and materials you used to gather your data.
Surveys Describe where, when, and how the survey was conducted.
- How did you design the questionnaire?
- What form did your questions take (e.g., multiple choice, Likert scale )?
- Were your surveys conducted in-person or virtually?
- What sampling method did you use to select participants?
- What was your sample size and response rate?
Experiments Share full details of the tools, techniques, and procedures you used to conduct your experiment.
- How did you design the experiment ?
- How did you recruit participants?
- How did you manipulate and measure the variables ?
- What tools did you use?
Existing data Explain how you gathered and selected the material (such as datasets or archival data) that you used in your analysis.
- Where did you source the material?
- How was the data originally produced?
- What criteria did you use to select material (e.g., date range)?
The survey consisted of 5 multiple-choice questions and 10 questions measured on a 7-point Likert scale.
The goal was to collect survey responses from 350 customers visiting the fitness apparel company’s brick-and-mortar location in Boston on 4–8 July 2022, between 11:00 and 15:00.
Here, a customer was defined as a person who had purchased a product from the company on the day they took the survey. Participants were given 5 minutes to fill in the survey anonymously. In total, 408 customers responded, but not all surveys were fully completed. Due to this, 371 survey results were included in the analysis.
Qualitative methods
In qualitative research , methods are often more flexible and subjective. For this reason, it’s crucial to robustly explain the methodology choices you made.
Be sure to discuss the criteria you used to select your data, the context in which your research was conducted, and the role you played in collecting your data (e.g., were you an active participant, or a passive observer?)
Interviews or focus groups Describe where, when, and how the interviews were conducted.
- How did you find and select participants?
- How many participants took part?
- What form did the interviews take ( structured , semi-structured , or unstructured )?
- How long were the interviews?
- How were they recorded?
Participant observation Describe where, when, and how you conducted the observation or ethnography .
- What group or community did you observe? How long did you spend there?
- How did you gain access to this group? What role did you play in the community?
- How long did you spend conducting the research? Where was it located?
- How did you record your data (e.g., audiovisual recordings, note-taking)?
Existing data Explain how you selected case study materials for your analysis.
- What type of materials did you analyse?
- How did you select them?
In order to gain better insight into possibilities for future improvement of the fitness shop’s product range, semi-structured interviews were conducted with 8 returning customers.
Here, a returning customer was defined as someone who usually bought products at least twice a week from the store.
Surveys were used to select participants. Interviews were conducted in a small office next to the cash register and lasted approximately 20 minutes each. Answers were recorded by note-taking, and seven interviews were also filmed with consent. One interviewee preferred not to be filmed.
Mixed methods
Mixed methods research combines quantitative and qualitative approaches. If a standalone quantitative or qualitative study is insufficient to answer your research question, mixed methods may be a good fit for you.
Mixed methods are less common than standalone analyses, largely because they require a great deal of effort to pull off successfully. If you choose to pursue mixed methods, it’s especially important to robustly justify your methods here.
Prevent plagiarism, run a free check.
Next, you should indicate how you processed and analysed your data. Avoid going into too much detail: you should not start introducing or discussing any of your results at this stage.
In quantitative research , your analysis will be based on numbers. In your methods section, you can include:
- How you prepared the data before analysing it (e.g., checking for missing data , removing outliers , transforming variables)
- Which software you used (e.g., SPSS, Stata or R)
- Which statistical tests you used (e.g., two-tailed t test , simple linear regression )
In qualitative research, your analysis will be based on language, images, and observations (often involving some form of textual analysis ).
Specific methods might include:
- Content analysis : Categorising and discussing the meaning of words, phrases and sentences
- Thematic analysis : Coding and closely examining the data to identify broad themes and patterns
- Discourse analysis : Studying communication and meaning in relation to their social context
Mixed methods combine the above two research methods, integrating both qualitative and quantitative approaches into one coherent analytical process.
Above all, your methodology section should clearly make the case for why you chose the methods you did. This is especially true if you did not take the most standard approach to your topic. In this case, discuss why other methods were not suitable for your objectives, and show how this approach contributes new knowledge or understanding.
In any case, it should be overwhelmingly clear to your reader that you set yourself up for success in terms of your methodology’s design. Show how your methods should lead to results that are valid and reliable, while leaving the analysis of the meaning, importance, and relevance of your results for your discussion section .
- Quantitative: Lab-based experiments cannot always accurately simulate real-life situations and behaviours, but they are effective for testing causal relationships between variables .
- Qualitative: Unstructured interviews usually produce results that cannot be generalised beyond the sample group , but they provide a more in-depth understanding of participants’ perceptions, motivations, and emotions.
- Mixed methods: Despite issues systematically comparing differing types of data, a solely quantitative study would not sufficiently incorporate the lived experience of each participant, while a solely qualitative study would be insufficiently generalisable.
Remember that your aim is not just to describe your methods, but to show how and why you applied them. Again, it’s critical to demonstrate that your research was rigorously conducted and can be replicated.
1. Focus on your objectives and research questions
The methodology section should clearly show why your methods suit your objectives and convince the reader that you chose the best possible approach to answering your problem statement and research questions .
2. Cite relevant sources
Your methodology can be strengthened by referencing existing research in your field. This can help you to:
- Show that you followed established practice for your type of research
- Discuss how you decided on your approach by evaluating existing research
- Present a novel methodological approach to address a gap in the literature
3. Write for your audience
Consider how much information you need to give, and avoid getting too lengthy. If you are using methods that are standard for your discipline, you probably don’t need to give a lot of background or justification.
Regardless, your methodology should be a clear, well-structured text that makes an argument for your approach, not just a list of technical details and procedures.
Methodology refers to the overarching strategy and rationale of your research. Developing your methodology involves studying the research methods used in your field and the theories or principles that underpin them, in order to choose the approach that best matches your objectives.
Methods are the specific tools and procedures you use to collect and analyse data (e.g. interviews, experiments , surveys , statistical tests ).
In a dissertation or scientific paper, the methodology chapter or methods section comes after the introduction and before the results , discussion and conclusion .
Depending on the length and type of document, you might also include a literature review or theoretical framework before the methodology.
Quantitative research deals with numbers and statistics, while qualitative research deals with words and meanings.
Quantitative methods allow you to test a hypothesis by systematically collecting and analysing data, while qualitative methods allow you to explore ideas and experiences in depth.
A sample is a subset of individuals from a larger population. Sampling means selecting the group that you will actually collect data from in your research.
For example, if you are researching the opinions of students in your university, you could survey a sample of 100 students.
Statistical sampling allows you to test a hypothesis about the characteristics of a population. There are various sampling methods you can use to ensure that your sample is representative of the population as a whole.
Cite this Scribbr article
If you want to cite this source, you can copy and paste the citation or click the ‘Cite this Scribbr article’ button to automatically add the citation to our free Reference Generator.
McCombes, S. (2022, October 10). What Is a Research Methodology? | Steps & Tips. Scribbr. Retrieved 14 May 2024, from https://www.scribbr.co.uk/thesis-dissertation/methodology/
Is this article helpful?
Shona McCombes
Other students also liked, how to write a dissertation proposal | a step-by-step guide, what is a literature review | guide, template, & examples, what is a theoretical framework | a step-by-step guide.
- Subject List
- Take a Tour
- For Authors
- Subscriber Services
- Publications
- African American Studies
- African Studies
- American Literature
- Anthropology
- Architecture Planning and Preservation
- Art History
- Atlantic History
- Biblical Studies
- British and Irish Literature
- Childhood Studies
- Chinese Studies
- Cinema and Media Studies
Communication
- Criminology
- Environmental Science
- Evolutionary Biology
- International Law
- International Relations
- Islamic Studies
- Jewish Studies
- Latin American Studies
- Latino Studies
- Linguistics
- Literary and Critical Theory
- Medieval Studies
- Military History
- Political Science
- Public Health
- Renaissance and Reformation
- Social Work
- Urban Studies
- Victorian Literature
- Browse All Subjects
How to Subscribe
- Free Trials
In This Article Expand or collapse the "in this article" section Textual Analysis and Communication
Introduction, theoretical background.
- General Introductions
- Analytical Strategies
- Methodological Antecedents
- Methodological Debate
- Types of Textual Analysis
- Qualitative Research in Media and Communication Studies
Related Articles Expand or collapse the "related articles" section about
About related articles close popup.
Lorem Ipsum Sit Dolor Amet
Vestibulum ante ipsum primis in faucibus orci luctus et ultrices posuere cubilia Curae; Aliquam ligula odio, euismod ut aliquam et, vestibulum nec risus. Nulla viverra, arcu et iaculis consequat, justo diam ornare tellus, semper ultrices tellus nunc eu tellus.
- Approaches to Multimodal Discourse Analysis
- Critical and Cultural Studies
- Feminist Theory
- Food Studies and Communication
- Gender and the Media
- Rhetoric and Communication
- Symbolic Interactionism in Communication
Other Subject Areas
Forthcoming articles expand or collapse the "forthcoming articles" section.
- Culture Shock and Communication
- LGBTQ+ Family Communication
- Queerbaiting
- Find more forthcoming titles...
- Export Citations
- Share This Facebook LinkedIn Twitter
Textual Analysis and Communication by Elfriede Fürsich LAST REVIEWED: 25 September 2018 LAST MODIFIED: 25 September 2018 DOI: 10.1093/obo/9780199756841-0216
Textual analysis is a qualitative method used to examine content in media and popular culture, such as newspaper articles, television shows, websites, games, videos, and advertising. The method is linked closely to cultural studies. Based on semiotic and interpretive approaches, textual analysis is a type of qualitative analysis that focuses on the underlying ideological and cultural assumptions of a text. In contrast to systematic quantitative content analysis, textual analysis reaches beyond manifest content to understand the prevailing ideologies of a particular historical and cultural moment that make a specific coverage possible. Critical-cultural scholars understand media content and other cultural artifacts as indicators of how realities are constructed and which ideas are accepted as normal. Following the French cultural philosopher Roland Barthes, content is understood as “text,” i.e., not as a fixed entity but as a complex set of discursive strategies that is generated in a special social, political, historic, and cultural context ( Barthes 2013 , cited under Theoretical Background ). Any text can be interpreted in multiple ways; the possibility of multiple meanings within a text is called “polysemy.” The goal of textual analysis is not to find one “true” interpretation—in contrast to traditional hermeneutic approaches to text exegesis—but to explain the variety of possible meanings inscribed in the text. Researchers who use textual analysis do not follow a single established approach but employ a variety of analysis types, such as ideological, genre, narrative, rhetorical, gender, or discourse analysis. Therefore, the term “textual analysis” could also be understood as a collective term for a variety of qualitative, interpretive, and critical content analysis techniques of popular culture artifacts. This method, just as cultural studies itself, draws on an eclectic mix of disciplines, such as anthropology, literary studies, rhetorical criticism, and cultural sociology, along with intellectual traditions, such as semiotics, (post)structuralism, and deconstruction. What distinguishes textual analysis from other forms of qualitative content analysis in the sociological tradition is its critical-cultural focus on power and ideology. Moreover, textual analysts normally do not use linguistic aspects as central evidence (such as in critical discourse analysis), nor do they use a pre-established code book, such as some traditional qualitative content methods. Textual analysis follows an inductive, interpretive approach by finding patterns in the material that lead to “readings” grounded in the back and forth between observation and contextual analysis. Of central interest is the deconstruction of representations (especially but not always of Others with regard to race, class, gender, sexuality, and ability) because these highlight the relationship of media and content to overall ideologies. The method is based in a constructionist framework. For textual analysts, media content does not simply reflect reality; instead, media, popular culture, and society are mutually constituted. Media and popular culture are arenas in which representations and ideas about reality are produced, maintained, and also challenged.
Central to textual analysis is the idea that content as “text” is a coming together of multiple meanings in a specific moment. Barthes 2013 and Barthes 1977 discuss this idea in detail and provide groundbreaking analysis of cultural phenomena in postwar France. Fiske 1987 , Fiske 2010 , and Fiske 2011 provide the standard on how popular culture can be “read,” i.e., interpreted for its ideological assumptions. Because the central aim of textual analysis is to understand how representations are produced in media content, Stuart Hall’s chapter 1 “The Work of Representation” in the renowned textbook Hall, et al. 2013 delivers a compact but comprehensive explanation of representation as a concept. To understand the shift to post-structuralism and concepts, such as discourse, hegemony, and the relationship between language and power, that are central to textual work, one can turn to the works of original theorists such as Foucault 1972 as well as Best and Kellner 1991 for contextualized clarification. Moreover, Deleuze and Guattari 2004 is a foundational post-structural text that radically rethinks the relationship between meaning and practice.
Barthes, Roland. 2013. Mythologies . New York: Hill and Wang.
English translation by Richard Howard and Annette Lavers of the original book published in 1957. Part 1: “Mythologies” consists of a series of short essayistic analyses of cultural phenomena, such as the Blue Guide travel books or advertising for detergents. Part 2: “Myth Today” lays out Barthes’s semiotic-structural approach. Although Barthes later acknowledged the historic contingencies of his interpretations, they remain important as they provided relevant perspective and methodological vocabulary for textual analysis for years to come.
Barthes, Roland. 1977. Image, music, text . Essays selected and translated by Stephen Heath. New York: Hill and Wang.
Classic collection of Barthes’s writing on semiotics and structuralism. For methodological considerations, the chapters “Introduction to the Structural Analysis of Narrative” and “The Death of the Author” are especially relevant.
Best, Steven, and Douglas Kellner. 1991. Postmodern theory: Critical interventions . New York: Guilford.
DOI: 10.1007/978-1-349-21718-2
Accessible introduction to leading postmodern and post-structuralist theorists. For textual analysis, especially the chapter 2 “Foucault and the Critique of Modernity,” chapter 4 “Baudrillard en route to Postmodernity,” and chapter 5 “Lyotard and Postmodern Gaming” provide relevant context for understanding post-structural and postmodern principles.
Deleuze, Gilles, and Félix Guattari. 2004. A thousand plateaus . Translated by Brian Massumi. London and New York: Continuum.
This book is the second part of Deleuze and Guattari’s groundbreaking philosophical project, “Capitalism and Schizophrenia.” Originally published in 1980, it explains central post-structural concepts such as rhizomes, multiplicity, and nomadic thought. Foundational for understanding the production of knowledge and meaning, these ideas have stood the test of time and resonate in networked and digitalized societies in the early 21st century.
Fiske, John. 1987. Television culture . New York: Routledge.
A classic book by Fiske. His “codes of television” (pp. 4–20) explain even for beginning researchers the important relationship among reality, representation, and ideology that is foundational for the textual analysis of any media content even beyond television.
Fiske, John. 2010. Understanding popular culture . 2d ed. London and New York: Routledge.
Important work by Fiske, originally published in 1989, that lays out the theoretical foundations for cultural analysis.
Fiske, John. 2011. Reading the popular . 2d ed. London and New York: Routledge.
Recently reissued companion book to Fiske 2010 . Provides a variety of examples for cultural analysis ranging from Madonna and shopping malls to news and quiz shows.
Foucault, Michel. 1972. The discourse on language. In The archeology of knowledge and the discourse of language . By Michel Foucault, 215–237. Translated by A. M. Sheridan Smith. New York: Pantheon.
Based on the author’s inaugural lecture at the Collège de France in 1970, this appendix provides a fairly succinct introduction to Foucault’s scholarly program and outlines his specific concepts of “discourse.” The author begins to connect discourses to structures of power and knowledge, an argument that becomes more central in his later writings.
Hall, Stuart, Jessica Evans, and Sean Nixon, eds. 2013. Representation: Cultural representations and signifying practices . 2d ed. London and Thousand Oaks, CA: SAGE.
One of the central goals of textual analysis is to understand and interpret media representations. Chapter 1 “The Work of Representation,” by Stuart Hall, is the most comprehensive introduction to this central post-structural cultural studies concept.
back to top
Users without a subscription are not able to see the full content on this page. Please subscribe or login .
Oxford Bibliographies Online is available by subscription and perpetual access to institutions. For more information or to contact an Oxford Sales Representative click here .
- About Communication »
- Meet the Editorial Board »
- Accounting Communication
- Acculturation Processes and Communication
- Action Assembly Theory
- Action-Implicative Discourse Analysis
- Activist Media
- Adherence and Communication
- Adolescence and the Media
- Advertisements, Televised Political
- Advertising
- Advertising, Children and
- Advertising, International
- Advocacy Journalism
- Agenda Setting
- Annenberg, Walter H.
- Apologies and Accounts
- Applied Communication Research Methods
- Argumentation
- Artificial Intelligence (AI) Advertising
- Attitude-Behavior Consistency
- Audience Fragmentation
- Audience Studies
- Authoritarian Societies, Journalism in
- Bakhtin, Mikhail
- Bandwagon Effect
- Baudrillard, Jean
- Blockchain and Communication
- Bourdieu, Pierre
- Brand Equity
- British and Irish Magazine, History of the
- Broadcasting, Public Service
- Capture, Media
- Castells, Manuel
- Celebrity and Public Persona
- Civil Rights Movement and the Media, The
- Co-Cultural Theory and Communication
- Codes and Cultural Discourse Analysis
- Cognitive Dissonance
- Collective Memory, Communication and
- Comedic News
- Communication Apprehension
- Communication Campaigns
- Communication, Definitions and Concepts of
- Communication History
- Communication Law
- Communication Management
- Communication Networks
- Communication, Philosophy of
- Community Attachment
- Community Journalism
- Community Structure Approach
- Computational Journalism
- Computer-Mediated Communication
- Content Analysis
- Corporate Social Responsibility and Communication
- Crisis Communication
- Critical Race Theory and Communication
- Cross-tools and Cross-media Effects
- Cultivation
- Cultural and Creative Industries
- Cultural Imperialism Theories
- Cultural Mapping
- Cultural Persuadables
- Cultural Pluralism and Communication
- Cyberpolitics
- Death, Dying, and Communication
- Debates, Televised
- Deliberation
- Developmental Communication
- Diffusion of Innovations
- Digital Divide
- Digital Gender Diversity
- Digital Intimacies
- Digital Literacy
- Diplomacy, Public
- Distributed Work, Comunication and
- Documentary and Communication
- E-democracy/E-participation
- E-Government
- Elaboration Likelihood Model
- Electronic Word-of-Mouth (eWOM)
- Embedded Coverage
- Entertainment
- Entertainment-Education
- Environmental Communication
- Ethnic Media
- Ethnography of Communication
- Experiments
- Families, Multicultural
- Family Communication
- Federal Communications Commission
- Feminist and Queer Game Studies
- Feminist Data Studies
- Feminist Journalism
- Focus Groups
- Freedom of the Press
- Friendships, Intercultural
- Gatekeeping
- Global Englishes
- Global Media, History of
- Global Media Organizations
- Glocalization
- Goffman, Erving
- Habermas, Jürgen
- Habituation and Communication
- Health Communication
- Hermeneutic Communication Studies
- Homelessness and Communication
- Hook-Up and Dating Apps
- Hostile Media Effect
- Identification with Media Characters
- Identity, Cultural
- Image Repair Theory
- Implicit Measurement
- Impression Management
- Infographics
- Information and Communication Technology for Development
- Information Management
- Information Overload
- Information Processing
- Infotainment
- Innis, Harold
- Instructional Communication
- Integrated Marketing Communications
- Interactivity
- Intercultural Capital
- Intercultural Communication
- Intercultural Communication, Tourism and
- Intercultural Communication, Worldview in
- Intercultural Competence
- Intercultural Conflict Mediation
- Intercultural Dialogue
- Intercultural New Media
- Intergenerational Communication
- Intergroup Communication
- International Communications
- Interpersonal Communication
- Interpersonal LGBTQ Communication
- Interpretation/Reception
- Interpretive Communities
- Journalism, Accuracy in
- Journalism, Alternative
- Journalism and Trauma
- Journalism, Citizen
- Journalism, Citizen, History of
- Journalism Ethics
- Journalism, Interpretive
- Journalism, Peace
- Journalism, Tabloid
- Journalists, Violence against
- Knowledge Gap
- Lazarsfeld, Paul
- Leadership and Communication
- Mass Communication
- McLuhan, Marshall
- Media Activism
- Media Aesthetics
- Media and Time
- Media Convergence
- Media Credibility
- Media Dependency
- Media Ecology
- Media Economics
- Media Economics, Theories of
- Media, Educational
- Media Effects
- Media Ethics
- Media Events
- Media Exposure Measurement
- Media, Gays and Lesbians in the
- Media Literacy
- Media Logic
- Media Management
- Media Policy and Governance
- Media Regulation
- Media, Social
- Media Sociology
- Media Systems Theory
- Merton, Robert K.
- Message Characteristics and Persuasion
- Mobile Communication Studies
- Multimodal Discourse Analysis, Approaches to
- Multinational Organizations, Communication and Culture in
- Murdoch, Rupert
- Narrative Engagement
- Narrative Persuasion
- Net Neutrality
- News Framing
- News Media Coverage of Women
- NGOs, Communication and
- Online Campaigning
- Open Access
- Organizational Change and Organizational Change Communicat...
- Organizational Communication
- Organizational Communication, Aging and
- Parasocial Theory in Communication
- Participation, Civic/Political
- Participatory Action Research
- Patient-Provider Communication
- Peacebuilding and Communication
- Perceived Realism
- Personalized Communication
- Persuasion and Social Influence
- Persuasion, Resisting
- Photojournalism
- Political Advertising
- Political Communication, Normative Analysis of
- Political Economy
- Political Knowledge
- Political Marketing
- Political Scandals
- Political Socialization
- Polls, Opinion
- Product Placement
- Public Interest Communication
- Public Opinion
- Public Relations
- Public Sphere
- Queer Intercultural Communication
- Queer Migration and Digital Media
- Race and Communication
- Racism and Communication
- Radio Studies
- Reality Television
- Reasoned Action Frameworks
- Religion and the Media
- Reporting, Investigative
- Rhetoric and Intercultural Communication
- Rhetoric and Social Movements
- Rhetoric, Religious
- Rhetoric, Visual
- Risk Communication
- Rumor and Communication
- Schramm, Wilbur
- Science Communication
- Scripps, E. W.
- Selective Exposure
- Sense-Making/Sensemaking
- Sesame Street
- Sex in the Media
- Small-Group Communication
- Social Capital
- Social Change
- Social Cognition
- Social Construction
- Social Identity Theory and Communication
- Social Interaction
- Social Movements
- Social Network Analysis
- Social Protest
- Sports Communication
- Stereotypes
- Strategic Communication
- Superdiversity
- Surveillance and Communication
- Synchrony in Intercultural Communication
- Tabloidization
- Telecommunications History/Policy
- Television, Cable
- Textual Analysis and Communication
- Third Culture Kids
- Third-Person Effect
- Time Warner
- Transgender Media Studies
- Transmedia Storytelling
- Two-Step Flow
- United Nations and Communication
- Urban Communication
- Uses and Gratifications
- Video Deficit
- Video Games and Communication
- Violence in the Media
- Virtual Reality and Communication
- Visual Communication
- Web Archiving
- Whistleblowing
- Whiteness Theory in Intercultural Communication
- Youth and Media
- Zines and Communication
- Privacy Policy
- Cookie Policy
- Legal Notice
- Accessibility
Powered by:
- [66.249.64.20|185.66.14.236]
- 185.66.14.236
Table of Contents
Ai, ethics & human agency, collaboration, information literacy, writing process, textual analysis – how to engage in textual analysis.
- © 2023 by Jennifer Janechek - IBM Quantum

As a reader, a developing writer, and an informed student and citizen, you need to be able to locate, understand, and critically analyze others’ purposes in communicating information. Being able to identify and articulate the meaning of other writers’ arguments and theses enables you to engage in intelligent, meaningful, and critical knowledge exchanges. Ultimately, regardless of the discipline you choose to participate in, textual analysis —the summary, contextualization, and interpretation of a writer’s effective or ineffective delivery of their perspective on a topic, statement of thesis, and development of an argument—will be an invaluable skill. Your ability to critically engage in knowledge exchanges—through the analysis of others’ communication—is integral to your success as a student and as a citizen.
Step 1: What Is The Thesis?
In order to learn how to better recognize a thesis in a written text, let’s consider the following argument:
So far, [Google+] does seem better than Facebook, though I’m still a rookie and don’t know how to do even some basic things.
It’s better in design terms, and also much better with its “circles” allowing you to target posts to various groups.
Example: following that high school reunion, the overwhelming majority of my Facebook friends list (which I’m barely rebuilding after my rejoin) are people from my own hometown. None of these people are going to care too much when my new book comes out from Edinburgh. Likewise, not too many of you would care to hear inside jokes about our old high school teachers, or whatever it is we banter about.
Another example: people I know only from exchanging a couple of professional emails with them ask to be Facebook friends. I’ve never met these people and have no idea what they’re really like, even if they seem nice enough on email. Do I really want to add them to my friends list on the same level as my closest friends, brothers, valued colleagues, etc.? Not yet. But then there’s the risk of offending people if you don’t add them. On Google+ you can just drop them in the “acquaintances” circle, and they’ll never know how they’re classified.
But they won’t be getting any highly treasured personal information there, which is exactly the restriction you probably want for someone you’ve never met before.
I also don’t like too many family members on my Facebook friends list, because frankly they don’t need to know everything I’m doing or chatting about with people. But on Google+ this problem will be easily manageable. (Harman)
The first sentence, “[Google+] does seem better than Facebook” (Harman), doesn’t communicate the writer’s position on the topic; it is merely an observation . A position, also called a “claim,” often includes the conjunction “because,” providing a reason why the writer’s observation is unique, meaningful, and critical.https://www.youtube.com/embed/rwSFfnlwtjY?rel=0&feature=youtu.beTherefore, if the writer’s sentence, “[Google+] does seem better than Facebook” (Harman), is simply an observation, then in order to identify the writer’s position, we must find the answer to “because, why?” One such answer can be found in the author’s rhetorical question/answer, “Do I really want to add them to my friends list on the same level as my closest friends, brothers, valued colleagues, etc.? Not yet” (Harman). The writer’s “because, why?” could be “because Google+ allows me to manage old, new, and potential friends and acquaintances using separate circles, so that I’m targeting posts to various, separate groups.” Therefore, the writer’s thesis—their position—could be something like, “Google+ is better than Facebook because its design enables me to manage my friends using separate circles, so that I’m targeting posts to various, separate groups instead of posting the same information for everyone I’ve added to my network.”
In addition to communicating a position on a particular topic, a writer’s thesis outlines what aspects of the topic they will address. Outlining intentions within a thesis is not only acceptable, but also one of a writer’s primary obligations, since the thesis relates their general argument. In a sense, you could think of the thesis as a responsibility to answer the question, “What will you/won’t you be claiming and why?”
To explain this further, let’s consider another example. If someone were to ask you what change you want to see in the world, you probably wouldn’t readily answer “world peace,” even though you (and many others) may want that. Why wouldn’t you answer that way? Because such an answer is far too broad and ambiguous to be logically argued. Although world peace may be your goal, for logic’s sake, you would be better off articulating your answer as “a peaceful solution to the violence currently occurring on the border of southern Texas and Mexico,” or something similarly specific. The distinction between the two answers should be clear: the first answer, “world peace,” is broad, ambiguous, and not a fully developed claim (there wouldn’t be many, if any, people who would disagree with this statement); the second answer is narrower, more specific, and a fully developed claim. It confines the argument to a particular example of violence, but still allows you to address what you want, “world peace,” on a smaller, more manageable, and more logical scale.
Since a writer’s thesis functions as an outline of what they will address in an argument, it is often organized in the same manner as the argument itself. Let’s return to the argument about Google+ for an example. If the author stated their position as suggested—“Google+ is better than Facebook because its design enables me to manage my friends using separate circles, so that I’m targeting posts to various, separate groups instead of posting the same information I’ve added to my network”—we would expect them to first address the similarities and differences between the designs of Google+ and Facebook, and then the reasons why they believe Google+ is a more effective way of sharing information. The organization of their thesis should reflect the overall order of their argument. Such a well-organized thesis builds the foundation for a cohesive and persuasive argument.
Textual Analysis: How is the Argument Structured?
“Textual analysis” is the term writers use to describe a reader’s written explanation of a text. The reader’s textual analysis ought to include a summary of the author’s topic, an analysis or explanation of how the author’s perspective relates to the ongoing conversation about that particular topic, an interpretation of the effectiveness of the author’s argument and thesis , and references to specific components of the text that support his or her analysis or explanation.
An effective argument generally consists of the following components:
- A thesis. Communicates the writer’s position on a particular topic.
- Acknowledgement of opposition. Explains existing objections to the writer’s position.
- Clearly defined premises outlining reasoning. Details the logic of the writer’s position.
- Evidence of validating premises. Proves the writer’s thorough research of the topic.
- A conclusion convincing the audience of the argument’s soundness/persuasiveness. Argues the writer’s position is relevant, logical, and thoroughly researched and communicated.
An effective argument also is specifically concerned with the components involved in researching, framing, and communicating evidence:
- The credibility and breadth of the writer’s research
- The techniques (like rhetorical appeals) used to communicate the evidence (see “The Rhetorical Appeals”)
- The relevance of the evidence as it reflects the concerns and interests of the author’s targeted audience
To identify and analyze a writer’s argument, you must critically read and understand the text in question. Focus and take notes as you read, highlighting what you believe are key words or important phrases. Once you are confident in your general understanding of the text, you’ll need to explain the author’s argument in a condensed summary. One way of accomplishing this is to ask yourself the following questions:
- What topic has the author written about? (Explain in as few words as possible.)
- What is the author’s point of view concerning their topic?
- What has the author written about the opposing point of view? (Where does it appear as though the author is “giving credit” to the opposition?)
- Does the author offer proof (either in reference to another published source or from personal experience) supporting their stance on the topic?
- As a reader, would you say that the argument is persuasive? Can you think of ways to strengthen the argument? Using which evidence or techniques?
Your articulation of the author’s argument will most likely derive from your answers to these questions. Let’s reconsider the argument about Google+ and answer the reflection questions listed above:
The author’s topic is two social networks—Google+ and Facebook.
The author is “for” the new social network Google+.
The author makes a loose allusion to the opposing point of view in the explanation, “I’m still a rookie and don’t know how to do even some basic things” (Harman). (The author alludes to his inexperience and, therefore, the potential for the opposing argument to have more merit.)
Yes, the author offers proof from personal experience, particularly through their first example: “following that high school reunion, the overwhelming majority of my Facebook friends list (which I’m barely rebuilding after my rejoin) are people from my hometown” (Harman). In their second example, they cite that “[o]n Google+ you can just drop [individuals] in the ‘acquaintances’ circle, and they’ll never even know how they’re classified” (Harman) in order to offer even more credible proof, based on the way Google+ operates instead of personal experience.
Yes, I would say that this argument is persuasive, although if I wanted to make it even stronger, I would include more detailed information about the opposing point of view. A balanced argument—one that fairly and thoroughly articulates both sides—is often more respected and better received because it proves to the audience that the writer has thoroughly researched the topic prior to making a judgment in favor of one perspective or another.
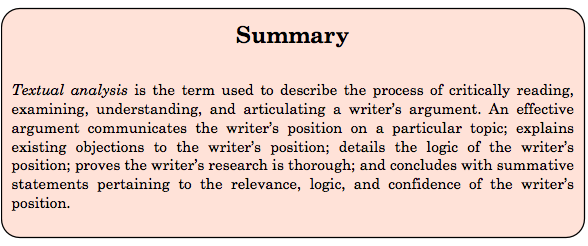
Works Cited
Harman, Graham. Object-Oriented Philosophy. WordPress, n.d. Web. 15 May 2012.
Related Articles:
Annotating the margins, textual analysis - how to analyze ads, suggested edits.
- Please select the purpose of your message. * - Corrections, Typos, or Edits Technical Support/Problems using the site Advertising with Writing Commons Copyright Issues I am contacting you about something else
- Your full name
- Your email address *
- Page URL needing edits *
- Email This field is for validation purposes and should be left unchanged.
- Jennifer Janechek
Featured Articles
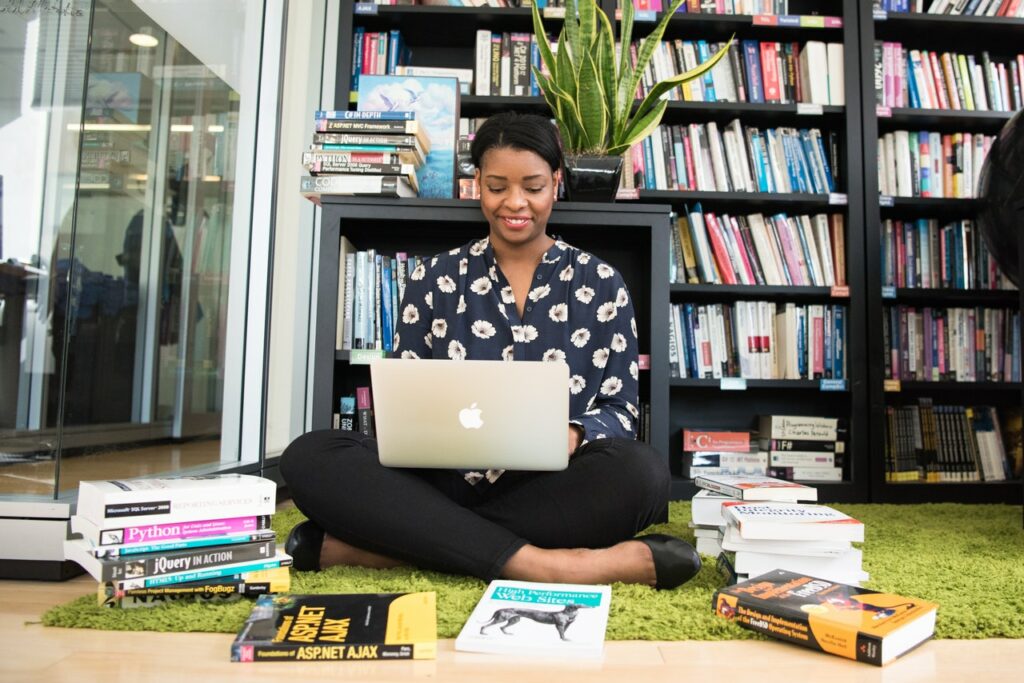
Academic Writing – How to Write for the Academic Community
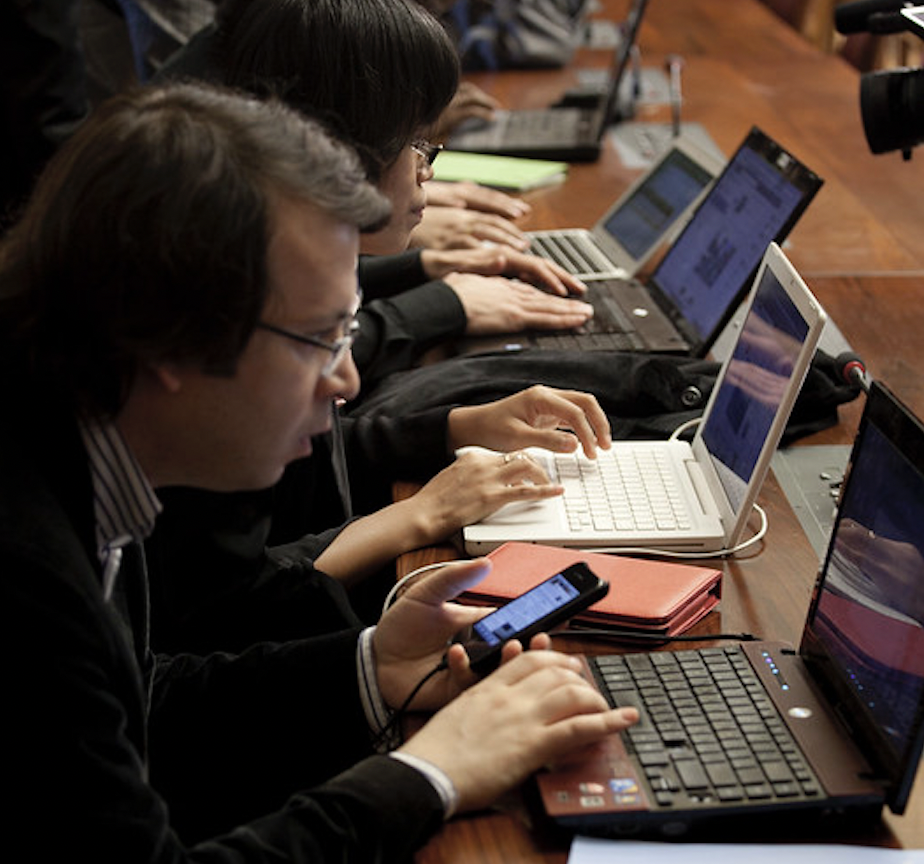
Professional Writing – How to Write for the Professional World
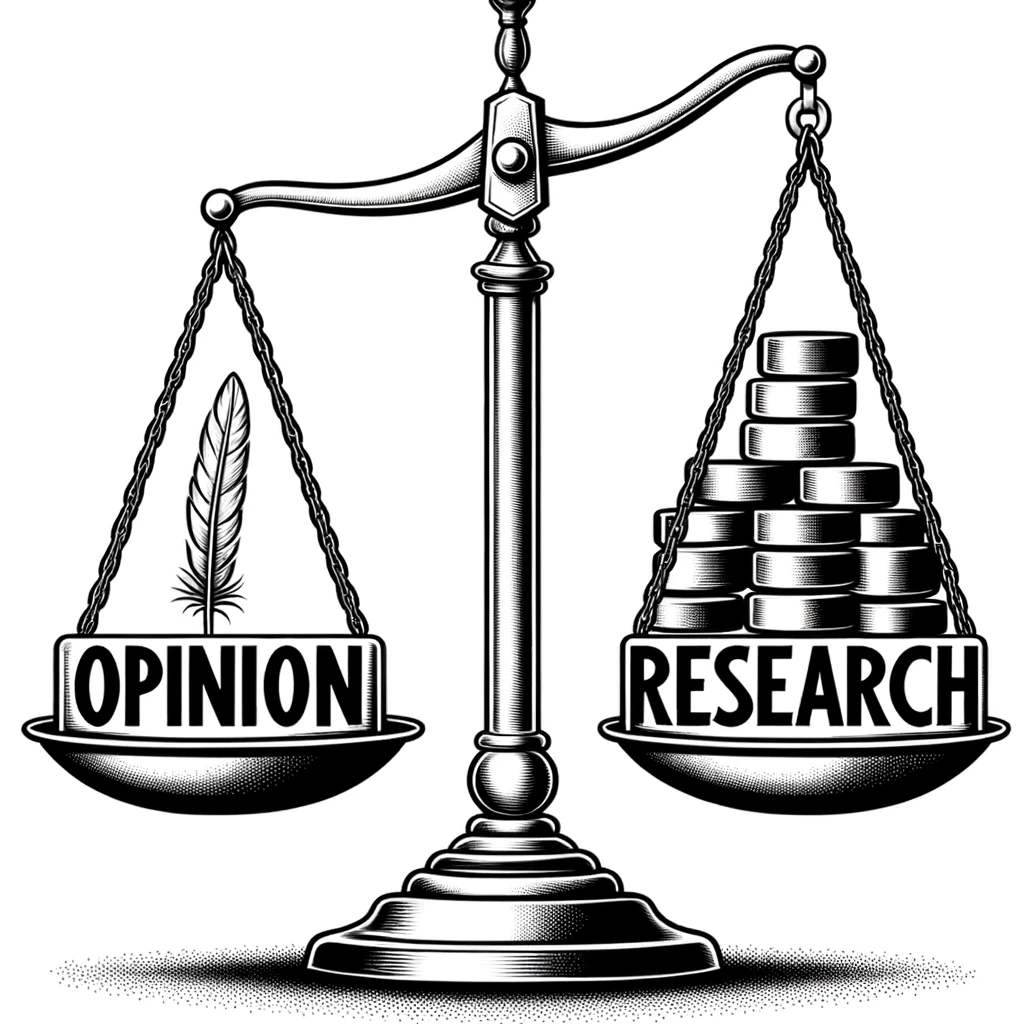
Credibility & Authority – How to Be Credible & Authoritative in Speech & Writing
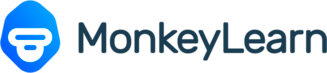
The Practical Guide to Textual Analysis
- Getting Started
- How Does It Work?
- Use Cases & Applications
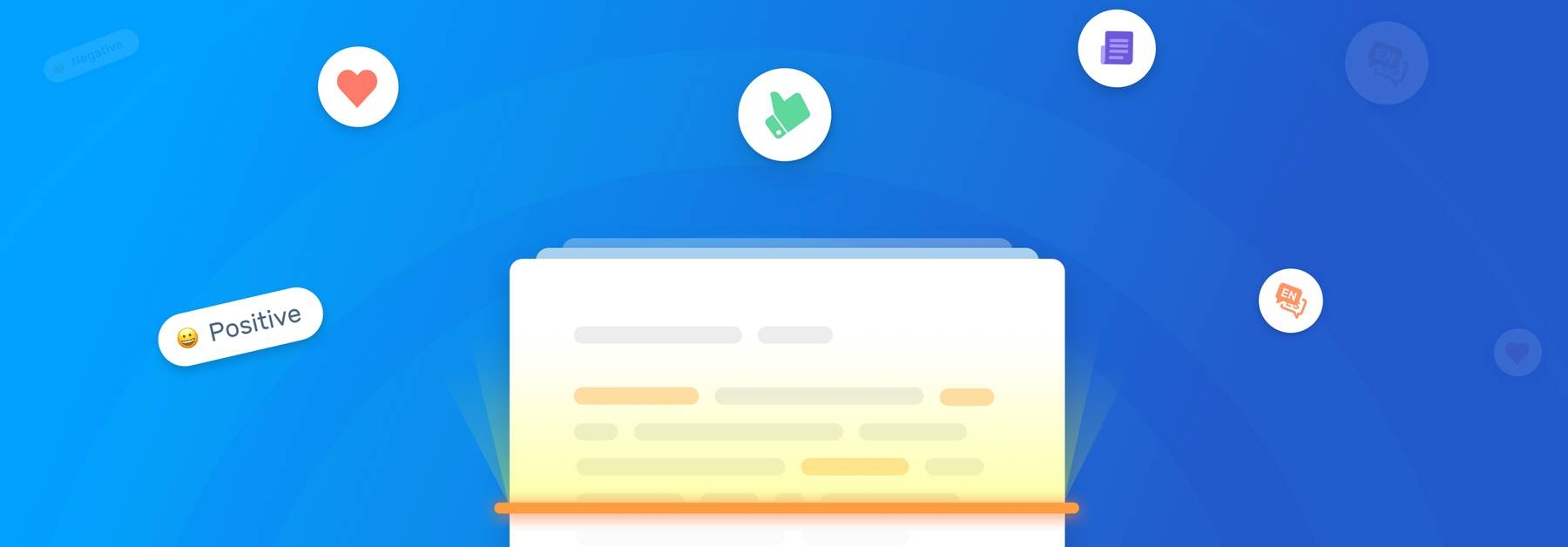
Textual analysis is the process of gathering and examining qualitative data to understand what it’s about.
But making sense of qualitative information is a major challenge. Whether analyzing data in business or performing academic research, manually reading, analyzing, and tagging text is no longer effective – it’s time-consuming, results are often inaccurate, and the process far from scalable.
Fortunately, developments in the sub-fields of Artificial Intelligence (AI) like machine learning and natural language processing (NLP) are creating unprecedented opportunities to process and analyze large collections of text data.
Thanks to algorithms trained with machine learning it is possible to perform a myriad of tasks that involve analyzing text, like topic classification (automatically tagging texts by topic), feature extraction (identifying specific characteristics in a text) and sentiment analysis (recognizing the emotions that underlie a given text).
Below, we’ll dive into textual analysis with machine learning, what it is and how it works, and reveal its most important applications in business and academic research:
Getting started with textual analysis
- What is textual analysis?
- Difference between textual analysis and content analysis?
- What is computer-assisted textual analysis?
- Methods and techniques
- Why is it important?
How does textual analysis work?
- Text classification
- Text extraction
Use cases and applications
- Customer service
- Customer feedback
- Academic research
Let’s start with the basics!
Getting Started With Textual Analysis
What is textual analysis.
While similar to text analysis , textual analysis is mainly used in academic research to analyze content related to media and communication studies, popular culture, sociology, and philosophy.
In this case, the purpose of textual analysis is to understand the cultural and ideological aspects that underlie a text and how they are connected with the particular context in which the text has been produced. In short, textual analysis consists of describing the characteristics of a text and making interpretations to answer specific questions.
One of the challenges of textual analysis resides in how to turn complex, large-scale data into manageable information. Computer-assisted textual analysis can be instrumental at this point, as it allows you to perform certain tasks automatically (without having to read all the data) and makes it simple to observe patterns and get unexpected insights. For example, you could perform automated textual analysis on a large set of data and easily tag all the information according to a series of previously defined categories. You could also use it to extract specific pieces of data, like names, countries, emails, or any other features.
Companies are using computer-assisted textual analysis to make sense of unstructured business data , and find relevant insights that lead to data-driven decisions. It’s being used to automate everyday tasks like ticket tagging and routing, improving productivity, and saving valuable time.
Difference Between Textual Analysis and Content Analysis?
When we talk about textual analysis we refer to a data-gathering process for analyzing text data. This qualitative methodology examines the structure, content, and meaning of a text, and how it relates to the historical and cultural context in which it was produced. To do so, textual analysis combines knowledge from different disciplines, like linguistics and semiotics.
Content analysis can be considered a subcategory of textual analysis, which intends to systematically analyze text, by coding the elements of the text to get quantitative insights. By coding text (that is, establishing different categories for the analysis), content analysis makes it possible to examine large sets of data and make replicable and valid inferences.
Sitting at the intersection between qualitative and quantitative approaches, content analysis has proved to be very useful to study a wide array of text data ― from newspaper articles to social media messages ― within many different fields, that range from academic research to organizational or business studies.
What is Computer-Assisted Textual Analysis?
Computer-assisted textual analysis involves using a software, digital platform, or computational tools to perform tasks related to text analysis automatically.
The developments in machine learning make it possible to create algorithms that can be trained with examples and learn a series of tasks, from identifying topics on a given text to extracting relevant information from an extensive collection of data. Natural Language Processing (NLP), another sub-field of AI, helps machines process unstructured data and transform it into manageable information that’s ready to analyze.
Automated textual analysis enables you to analyze large amounts of data that would require a significant amount of time and resources if done manually. Not only is automated textual analysis fast and straightforward, but it’s also scalable and provides consistent results.
Let’s look at an example. During the US elections 2016, we used MonkeyLearn to analyze millions of tweets referring to Donald Trump and Hillary Clinton . A text classification model allowed us to tag each Tweet into the two predefined categories: Trump and Hillary. The results showed that, on an average day, Donald Trump was getting around 450,000 Twitter mentions while Hillary Clinton was only getting about 250,000. And that was just the tip of the iceberg! What was really interesting was the nuances of those mentions: were they favorable or unfavorable? By performing sentiment analysis , we were able to discover the feelings behind those messages and gain some interesting insights about the polarity of those opinions.
For example, this is how Trump’s Tweets looked like when counted by sentiment:
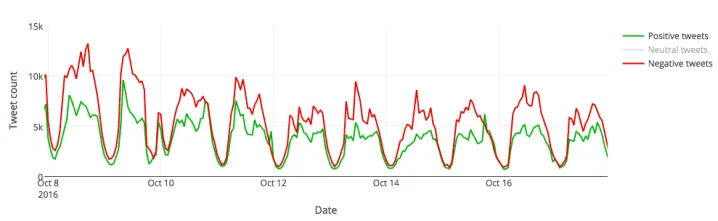
And this graphic shows the same for Hillary Clinton:
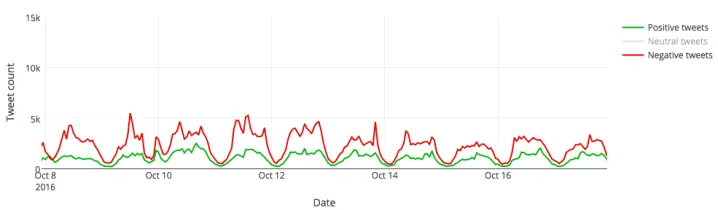
There are many methods and techniques for automated textual analysis. In the following section, we’ll take a closer look at each of them so that you have a better idea of what you can do with computer-assisted textual analysis.
Textual Analysis Methods & Techniques
- Word frequency
Collocation
Concordance, basic methods, word frequency.
Word frequency helps you find the most recurrent terms or expressions within a set of data. Counting the times a word is mentioned in a group of texts can lead you to interesting insights, for example, when analyzing customer feedback responses. If the terms ‘hard to use’ or ‘complex’ often appear in comments about your product, it may indicate you need to make UI/UX adjustments.
By ‘collocation’ we mean a sequence of words that frequently occur together. Collocations are usually bigrams (a pair of words) and trigrams (a combination of three words). ‘Average salary’ , ‘global market’ , ‘close a deal’ , ‘make an appointment’ , ‘attend a meeting’ are examples of collocations related to business.
In textual analysis, identifying collocations is useful to understand the semantic structure of a text. Counting bigrams and trigrams as one word improves the accuracy of the analysis.
Human language is ambiguous: depending on the context, the same word can mean different things. Concordance is used to identify instances in which a word or a series of words appear, to understand its exact meaning. For example, here are a few sentences from product reviews containing the word ‘time’:
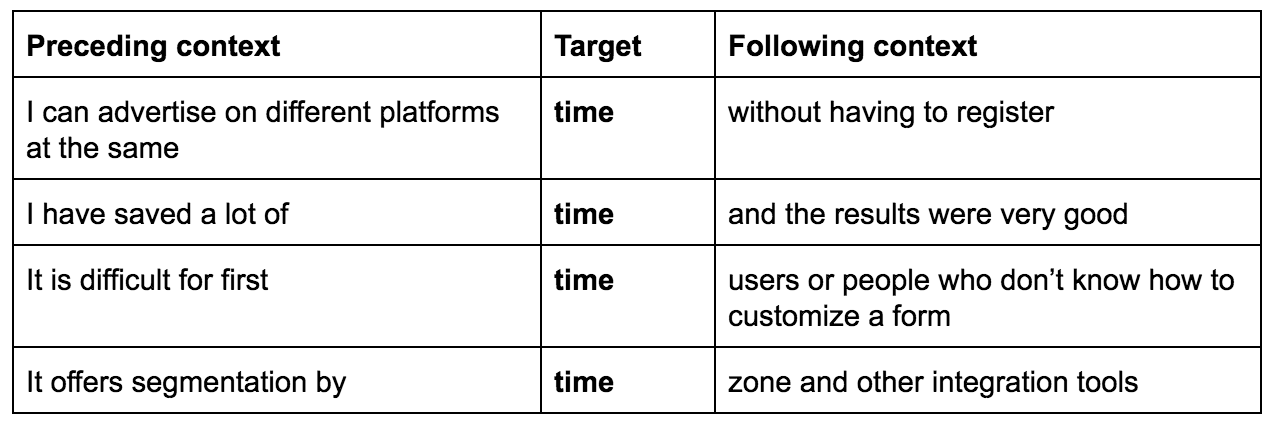
Advanced Methods
Text classification.
Text classification is the process of assigning tags or categories to unstructured data based on its content.
When we talk about unstructured data we refer to all sorts of text-based information that is unorganized, and therefore complex to sort and manage. For businesses, unstructured data may include emails, social media posts, chats, online reviews, support tickets, among many others. Text classification ― one of the essential tasks of Natural Language Processing (NLP) ― makes it possible to analyze text in a simple and cost-efficient way, organizing the data according to topic, urgency, sentiment or intent. We’ll take a closer look at each of these applications below:
Topic Analysis consists of assigning predefined tags to an extensive collection of text data, based on its topics or themes. Let’s say you want to analyze a series of product reviews to understand what aspects of your product are being discussed, and a review reads ‘the customer service is very responsive, they are always ready to help’ . This piece of feedback will be tagged under the topic ‘Customer Service’ .
Sentiment Analysis , also known as ‘opinion mining’, is the automated process of understanding the attributes of an opinion, that is, the emotions that underlie a text (e.g. positive, negative, and neutral). Sentiment analysis provides exciting opportunities in all kinds of fields. In business, you can use it to analyze customer feedback, social media posts, emails, support tickets, and chats. For instance, you could analyze support tickets to identify angry customers and solve their issues as a priority. You may also combine topic analysis with sentiment analysis (it is called aspect-based sentiment analysis ) to identify the topics being discussed about your product, and also, how people are reacting towards those topics. For example, take the product review we mentioned earlier for topic analysis: ‘the customer service is very responsive, they are always ready to help’ . This statement would be classified as both Positive and Customer Service .
Language detection : this allows you to classify a text based on its language. It’s particularly useful for routing purposes. For example, if you get a support ticket in Spanish, it could be automatically routed to a Spanish-speaking customer support team.
Intent detection : text classifiers can also be used to recognize the intent of a given text. What is the purpose behind a specific message? This can be helpful if you need to analyze customer support conversations or the results of a sales email campaign. For example, you could analyze email responses and classify your prospects based on their level of interest in your product.
Text Extraction
Text extraction is a textual analysis technique which consists of extracting specific terms or expressions from a collection of text data. Unlike text classification, the result is not a predefined tag but a piece of information that is already present in the text. For example, if you have a large collection of emails to analyze, you could easily pull out specific information such as email addresses, company names or any keyword that you need to retrieve. In some cases, you can combine text classification and text extraction in the same analysis.
The most useful text extraction tasks include:
Named-entity recognition : used to extract the names of companies , people , or organizations from a set of data.
Keyword extraction : allows you to extract the most relevant terms within a text. You can use keyword extraction to index data to be searched, create tags clouds, summarize the content of a text, among many other things.
Feature extraction : used to identify specific characteristics within a text. For example, if you are analyzing a series of product descriptions, you could create customized extractors to retrieve information like brand, model, color, etc .
Why is Textual Analysis Important?
Every day, we create a colossal amount of digital data. In fact, in the last two years alone we generated 90% percent of all the data in the world . That includes social media messages, emails, Google searches, and every other source of online data.
At the same time, books, media libraries, reports, and other types of databases are now available in digital format, providing researchers of all disciplines opportunities that didn’t exist before.
But the problem is that most of this data is unstructured. Since it doesn’t follow any organizational criteria, unstructured text is hard to search, manage, and examine. In this scenario, automated textual analysis tools are essential, as they help make sense of text data and find meaningful insights in a sea of information.
Text analysis enables businesses to go through massive collections of data with minimum human effort, saving precious time and resources, and allowing people to focus on areas where they can add more value. Here are some of the advantages of automated textual analysis:
Scalability
You can analyze as much data as you need in just seconds. Not only will you save valuable time, but you’ll also make your teams much more productive.
Real-time analysis
For businesses, it is key to detect angry customers on time or be warned of a potential PR crisis. By creating customized machine learning models for text analysis, you can easily monitor chats, reviews, social media channels, support tickets and all sorts of crucial data sources in real time, so you’re ready to take action when needed.
Academic researchers, especially in the political science field , may find real-time analysis with machine learning particularly useful to analyze polls, Twitter data, and election results.
Consistent criteria
Routine manual tasks (like tagging incoming tickets or processing customer feedback, for example) often end up being tedious and time-consuming. There are more chances of making mistakes and the criteria applied within team members often turns out to be inconsistent and subjective. Machine learning algorithms, on the other hand, learn from previous examples and always use the same criteria to analyze data .
How does Textual Analysis Work?
Computer-assisted textual analysis makes it easy to analyze large collections of text data and find meaningful information. Thanks to machine learning, it is possible to create models that learn from examples and can be trained to classify or extract relevant data.
But how easy is to get started with textual analysis?
As with most things related to artificial intelligence (AI), automated text analysis is perceived as a complex tool, only accessible to those with programming skills. Fortunately, that’s no longer the case. AI platforms like MonkeyLearn are actually very simple to use and don’t require any previous machine learning expertise. First-time users can try different pre-trained text analysis models right away, and use them for specific purposes even if they don’t have coding skills or have never studied machine learning.
However, if you want to take full advantage of textual analysis and create your own customized models, you should understand how it works.
There are two steps you need to follow before running an automated analysis: data gathering and data preparation. Here, we’ll explain them more in detail:
Data gathering : when we think of a topic we want to analyze, we should first make sure that we can obtain the data we need. Let’s say you want to analyze all the customer support tickets your company has received over a designated period of time. You should be able to export that information from your software and create a CSV or an Excel file. The data can be either internal (that is, data that’s only available to your business, like emails, support tickets, chats, spreadsheets, surveys, databases, etc) or external (like review sites, social media, news outlets or other websites).
Data preparation : before performing automated text analysis it’s necessary to prepare the data that you are going to use. This is done by applying a series of Natural Language Processing (NLP) techniques. Tokenization , parsing , lemmatization , stemming and stopword removal are just a few of them.
Once these steps are complete, you will be all set up for the data analysis itself. In this section, we’ll refer to how the most common textual analysis methods work: text classification and text extraction.
Text classification is the process of assigning tags to a collection of data based on its content.
When done manually, text categorization is a time-consuming task that often leads to mistakes and inaccuracies. By doing this automatically, it is possible to obtain very good results while spending less time and resources. Automatic text classification consists of three main approaches: rule-based, machine learning and hybrid.
Rule-based systems
Rule-based systems follow an ‘if-then’ (condition-action) structure based on linguistic rules. Basically, rules are human-made associations between a linguistic pattern on a text and a predefined tag. These linguistic patterns often refer to morphological, syntactic, lexical, semantic, or phonological aspects.
For instance, this could be a rule to classify a series of laptop descriptions:
( Lenovo | Sony | Hewlett Packard | Apple ) → Brand
In this case, when the text classification model detects any of those words within a text (the ‘if’ portion), it will assign the predefined tag ‘brand’ to them (the ‘then’ portion).
One of the main advantages of rule-based systems is that they are easy to understand by humans. On the downside, creating complex systems is quite tricky, because you need to have good knowledge of linguistics and of the topics present in the text that you want to analyze. Besides, adding new rules can be tough as it requires several tests, making rule-based systems hard to scale.
Machine learning-based systems
Machine learning-based systems are trained to make predictions based on examples. This means that a person needs to provide representative and consistent samples and assign the expected tags manually so that the system learns to make its own predictions from those past observations. The collection of manually tagged data is called training data .
But how does machine learning actually work?
Suppose you are training a machine learning-based classifier. The system needs to transform the training data into something it can understand: in this case, vectors (an array of numbers with encoded data). Vectors contain a set of relevant features from the given text, and use them to learn and make predictions on future data.
One of the most common methods for text vectorization is called bag of words and consists of counting how many times a particular word (from a predetermined list of words) appears in the text you want to analyze.
So, the text is transformed into vectors and fed into a machine learning algorithm along with its expected tags, creating a text classification model:
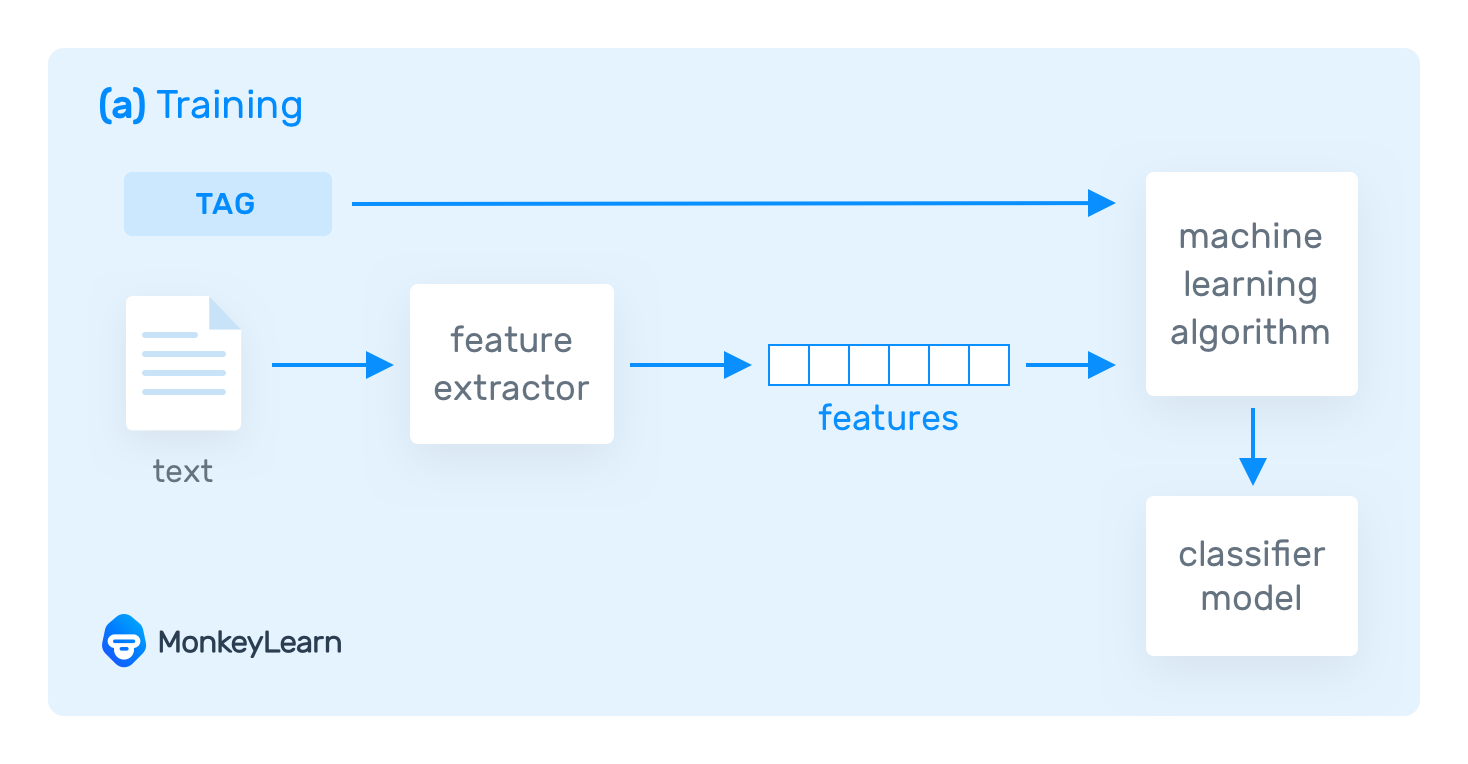
After being trained, the model can make predictions over unseen data:
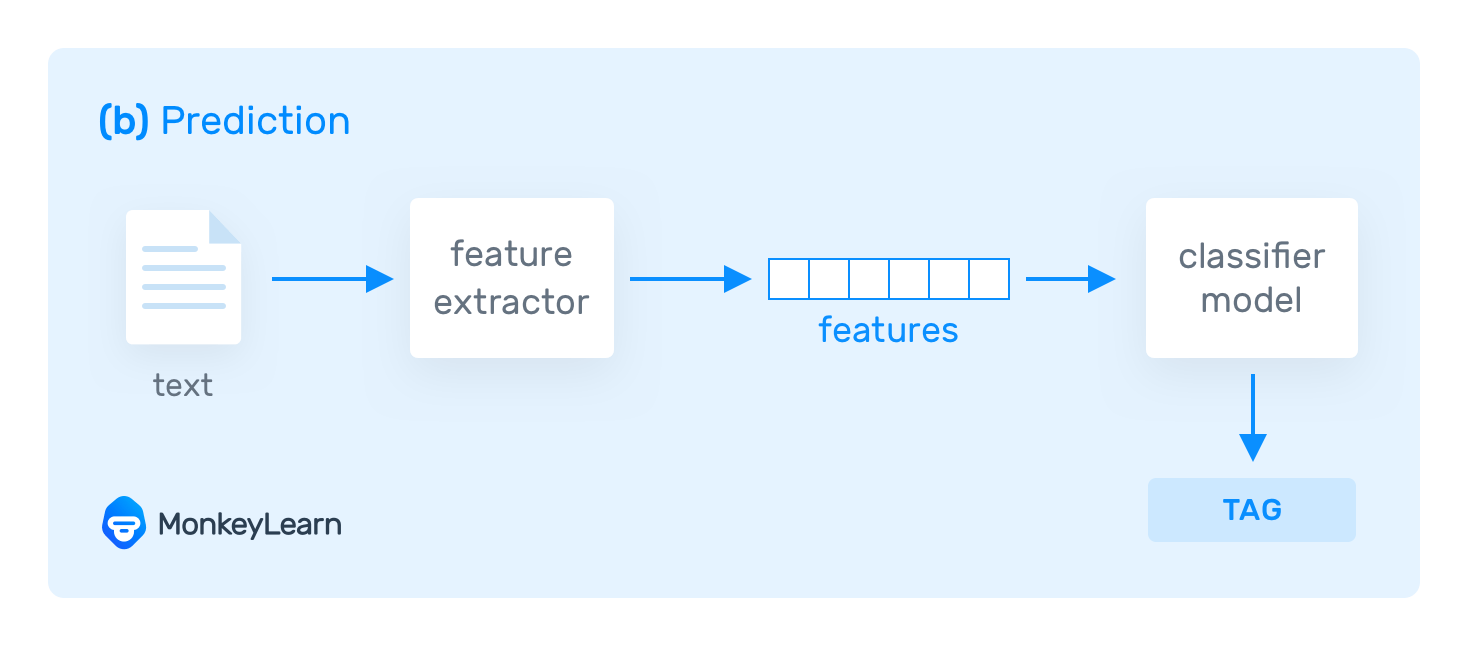
Machine learning algorithms
The most common algorithms used in text classification are Naive Bayes family of algorithms (NB), Support Vector Machines (SVM), and deep learning algorithms .
Naive Bayes family of algorithms (NB) is a probabilistic algorithm that uses Bayes’ theorem to calculate the probability of each tag for a given text. It then provides the tag with the highest likelihood of occurrence. This algorithm provides good results as long as the training data is scarce.
Support Vector Machines (SVM) is a machine learning algorithm that divides vectors into two different groups within a three-dimensional space. In one group, you have vectors that belong to a given tag, and in the other group vectors that don’t belong to that tag. Using this algorithm requires more coding skills, but the results are better than the ones with Naive Bayes.
Deep learning algorithms try to emulate the way the human brain thinks. They use millions of training examples and generate very rich representations of texts, leading to much more accurate predictions than other machine learning algorithms. The downside is that they need vast amounts of training data to provide accurate results and require intensive coding.
Hybrid systems
These systems combine rule-based systems and machine learning-based systems to obtain more accurate predictions.
There are different parameters to evaluate the performance of a text classifier: accuracy , precision , recall , and F1 score .
You can measure how your text classifier works by comparing it to a fixed testing set (that is, a group of data that already includes its expected tags) or by using cross-validation, a process that divides your training data into two groups – one used to train the model, and the other used to test the results.
Let’s go into more detail about each of these parameters:
Accuracy : this is the number of correct predictions that the text classifier makes divided by the total number of predictions. However, accuracy alone is not the best parameter to analyze the performance of a text classifier. When the number of examples is imbalanced (for example, a lot of the data belongs to one of the categories) you may experience an accuracy paradox , that is, a model with high accuracy, but one that’s not necessarily able to make accurate predictions for all tags. In this case, it’s better to look at precision and recall, and F1 score.
Precision : this metric indicates the number of correct predictions for a given tag, divided by the total number of correct and incorrect predictions for that tag. In this case, a high precision level indicates there were less false positives. For some tasks ― like sending automated email responses ― you will need text classification models with a high level of precision, that will only deliver an answer when it’s highly likely that the recipient belongs to a given tag.
Recall : it shows the number of correct predictions for a given tag, over the number of predictions that should have been predicted as belonging to that tag. High recall metrics indicate there were less false negatives and, if routing support tickets for example, it means that tickets will be sent to the right teams.
F1 score : this metric considers both precision and recall results, and provides an idea of how well your text classifier is working. It allows you to see how accurate is your model for all the tags you’re using.
Cross-validation
Cross-validation is a method used to measure the accuracy of a text classifier model. It consists of splitting the training dataset into a number of equal-length subsets, in a random way. For instance, let’s imagine you have four subsets and each of them contains 25% of your training data.
All of those subsets except one are used to train the text classifier. Then, the classifier is used to make predictions over the remaining subset. After this, you need to compile all the metrics we mentioned before (accuracy, precision, recall, and F1 score), and start the process all over again, until all the subsets have been used for testing. Finally, all the results are compiled to obtain the average performance of each metric.
Text extraction is the process of identifying specific pieces of text from unstructured data. This is very useful for a variety of purposes, from extracting company names from a Linkedin dataset to pulling out prices on product descriptions.
Text extraction allows to automatically visualize where the relevant terms or expressions are, without needing to read or scan all the text by yourself. And that is particularly relevant when you have massive databases, which would otherwise take ages to analyze manually.
There are different approaches to text extraction. Here, we’ll refer to the most commonly used and reliable:
Regular expressions
Regular expressions are similar to rules for text classification models. They can be defined as a series of characters that define a pattern.
Every time the text extractor detects a coincidence with a pattern, it assigns the corresponding tag.
This approach allows you to create text extractors quickly and with good results, as long as you find the right patterns for the data you want to analyze. However, as it gets more complex, it can be hard to manage and scale.
Conditional Random Fields
Conditional Random Fields (CRF) is a statistical approach used for text extraction with machine learning. It identifies different patterns by assigning a weight to each of the word sequences within a text. CRF’s also allow you to create additional parameters related to the patterns, based on syntactic or semantic information.
This approach creates more complex and richer patterns than regular expressions and can encode a large volume of information. However, if you want to train the text extractor properly, you will need to have in-depth NLP and computing knowledge.
You can use the same performance metrics that we mentioned for text classification (accuracy, precision, recall, and F1 score), although these metrics only consider exact matches as positive results, leaving partial matches aside.
If you want partial matches to be included in the results, you should use a performance metric called ROUGE (Recall-Oriented Understudy for Gisting Evaluation). This group of metrics measures lengths and numbers of sequences to make a match between the source text and the extraction performed by the model.
The parameters used to compare these two texts need to be defined manually. You may define ROUGE-n metrics (n is the length of the units you want to measure) or ROUGE-L metrics (to compare the longest common sentence).
Use Cases and Applications
Automated textual analysis is the process of obtaining meaningful information out of raw data. Considering unstructured data is getting closer to 80% of the existing information in the digital world , it’s easy to understand why this brings outstanding opportunities for businesses, organizations, and academic researchers.
For companies, it is now possible to obtain real-time insights on how their users feel about their products and make better business decisions based on data. Shifting to a data-driven approach is one of the main challenges of businesses today.
Textual analysis has many exciting applications across different areas of a company, like customer service, marketing, product, or sales. By allowing the automation of specific tasks that used to be manual, textual analysis is helping teams become more productive and efficient, and allowing them to focus on areas where they can add real value.
In the academic research field, computer-assisted textual analysis (and mainly, machine learning-based models) are expanding the horizons of investigation, by providing new ways of processing, classifying, and obtaining relevant data.
In this section, we’ll describe the most significant applications related to customer service, customer feedback, and academic research.
Customer Service
It’s not all about having an amazing product or investing a lot of money on advertising. What really tips the balance when it comes to business success is to provide high-quality customer service. Stats claim that 70% of the customer journey is defined by how people feel they are being treated .
So, how can textual analysis help companies deliver a better customer service experience?
Automatically tag support tickets
Every time a customer sends a request, comment, or complain, there’s a new support ticket to be processed. Customer support teams need to categorize every incoming message based on its content, a routine task that can be boring, time-consuming, and inconsistent if done manually.
Textual analysis with machine learning allows you automatically identify the topic of each support ticket and tag it accordingly. How does it work?
- First, a person defines a set of categories and trains a classifier model by applying the appropriate tags to a number of representative samples.
- The model analyzes the words and expressions used in each ticket. For example: ‘I’m having problems when paying with my credit card’ , and it compares it with previous examples.
- Finally, it automatically tags the ticket according to its content. In this case, the ticket would be tagged as Payment Issues .
Automatically route and triage support tickets
Once support tickets are tagged, they need to be routed to the appropriate team in charge to deal with that issue. Machine learning enables teams to send a ticket to the right person in real-time , based on the ticket’s topic, language or complexity. For example, a ticket previously tagged as Payment Issues will be automatically routed to the Billing Area .
Detect the urgency of a ticket
A simple task, like being able to prioritize tickets based on their urgency, can have a substantial positive impact on your customer service. By analyzing the content of each ticket, a textual analysis model can let you assess which of them are more critical and prioritize accordingly . For instance, a ticket containing the words or expressions ‘as soon as possible’ or ‘immediately’ would be automatically classified as Urgent .
Get insights from ticket analytics
The performance of customer service teams is usually measured by KPI’s, like first response time, the average time of resolution, and customer satisfaction (CSAT).
Textual analysis algorithms can be used to analyze the different interactions between customers and the customer service area, like chats, support tickets, emails, and customer satisfaction surveys.
You can use aspect-based sentiment analysis to understand the main topics discussed by your customers and how they are feeling about those topics. For example, you may have a lot of mentions referring to the topic ‘UI/UX’ . But, are all those customers’ opinions positive, negative, or neutral? This type of analysis can provide a more accurate perspective of what they think about your product and get a deeper understanding the overall customer satisfaction.
Customer Feedback
Listening to the Voice of Customer (VoC) is critical to understand the customers’ expectations, experience and opinion about your brand. Two of the most common tools to monitor and examine customer feedback are customer surveys and product reviews.
By analyzing customer feedback data, companies can detect topics for improvement, spot product flaws, get a better understanding of your customer’s needs and measure their level of satisfaction, among many other things.
But how do you process and analyze tons of reviews or thousands of customer surveys? Here are some ideas of how you can use textual analysis algorithms to analyze different kinds of customer feedback:
Analyze NPS Responses
Net Promoter Score (NPS) is the most popular tool to measure customer satisfaction. The first part of the survey involves giving the brand a score from 0 to 10 based on the question: 'How likely is it that you would recommend [brand] to a friend or colleague?' . The results allow you to classify your customers as promoters , passives , and detractors .
Then, there’s a follow-up question, inquiring about the reasons for your previous score. These open-ended responses often provide the most insightful information about your company. At the same time, it’s the most complex data to process. Yes, you could read and tag each of the responses manually, but what if there are thousands of them?
Textual analysis with machine learning enables you to detect the main topics that your customers are referring to, and even extract the most relevant keywords related to those topics . To make the most of your data, you could also perform sentiment analysis and find out if your customers are talking about a given topic positively or negatively.
Analyze Customer Surveys
Besides NPS, textual analysis algorithms can help you analyze all sorts of customer surveys. Using a text classification model to tag your responses can make you save a lot of valuable time and resources while allowing you to obtain consistent results.
Analyze Product Reviews
Product reviews are a significant factor when buying a product. Prospective buyers read at least 10 reviews before feeling they can trust a local business and that’s just one of the (many) reasons why you should keep a close eye on what people are saying about your brand online.
Analyzing product reviews can give you an idea of what people love and hate the most about your product and service. It can provide useful insights and opportunities for improvement. And it can show you what to do to get one step ahead of your competition.
The truth is that going through pages and pages of product reviews is not a very exciting task. Categorizing all those opinions can take teams hours and in the end, it becomes an expensive and unproductive process. That’s why automated textual analysis is a game-changer.
Imagine you want to analyze a set of product reviews from your SaaS company in G2 Crowd. A textual analysis model will allow you to tag g each review based on topic, like Ease of Use , Price , UI/UX , Integrations . You could also run a sentiment analysis to discover how your customers feel about those topics: do they think the price is suitable or too expensive? Do they find it too complex or easy to use?
Thanks to textual analysis algorithms, you can get powerful information to help you make data-driven decisions, and empower your teams to be more productive by reducing manual tasks to a minimum.
Academic Research
What if you were able to sift through tons of papers and journals, and discover data that is relevant to your research in just seconds? Just imagine if you could easily classify years of news articles and extract meaningful keywords from them, or analyze thousands of tweets after a significant political change .
Even though machine learning applications in business and science seem to be more frequent, social science research is also benefiting from ML to perform tasks related to the academic world.
Social science researchers need to deal with vast volumes of unstructured data. Therefore, one of the major opportunities provided by computer-assisted textual analysis is being able to classify data, extract relevant information, or identify different groups in extensive collections of data.
Another application of textual analysis with machine learning is supporting the coding process . Coding is one of the early steps of any qualitative textual analysis. It involves a detailed examination of what you want to analyze to become familiar with the data. When done manually, this task can be very time consuming and often inaccurate or inconsistent. Fortunately, machine learning algorithms (like text classifier models) can help you do this in very little time and allow you to scale up the coding process easily.
Finally, using machine learning algorithms to scan large amounts of papers, databases, and journal articles can lead to new investigation hypotheses .
Final Words
In a world overloaded with data, textual analysis with machine learning is a powerful tool that enables you to make sense of unstructured information and find what’s relevant in just seconds.
With promising use cases across many fields from marketing to social science research, machine learning algorithms are far from being a niche technology only available for a few. Moreover, they are turning into user-friendly applications that are dominated by workers with little or no coding skills.
Thanks to text analysis models, teams are becoming more productive by being released from manual and routine tasks that used to take valuable time from them. At the same time, companies can make better decisions based on valuable, real-time insights obtained from data.
By now, you probably have an idea of what textual analysis is with machine learning and how you can use it to make your everyday tasks more efficient and straightforward. Ready to get started? MonkeyLearn makes it very simple to take your first steps. Just contact us and get a personalized demo from one of our experts!
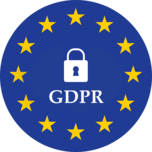
MonkeyLearn Inc. All rights reserved 2024
- Privacy Policy

Home » Textual Analysis – Types, Examples and Guide
Textual Analysis – Types, Examples and Guide
Table of Contents

Textual Analysis
Textual analysis is the process of examining a text in order to understand its meaning. It can be used to analyze any type of text, including literature , poetry, speeches, and scientific papers. Textual analysis involves analyzing the structure, content, and style of a text.
Textual analysis can be used to understand a text’s author, date, and audience. It can also reveal how a text was constructed and how it functions as a piece of communication.
Textual Analysis in Research
Textual analysis is a valuable tool in research because it allows researchers to examine and interpret text data in a systematic and rigorous way. Here are some ways that textual analysis can be used in research:
- To explore research questions: Textual analysis can be used to explore research questions in various fields, such as literature, media studies, and social sciences. It can provide insight into the meaning, interpretation, and communication patterns of text.
- To identify patterns and themes: Textual analysis can help identify patterns and themes within a set of text data, such as analyzing the representation of gender or race in media.
- To evaluate interventions: Textual analysis can be used to evaluate the effectiveness of interventions, such as analyzing the language and messaging of public health campaigns.
- To inform policy and practice: Textual analysis can provide insights that inform policy and practice, such as analyzing legal documents to inform policy decisions.
- To analyze historical data: Textual analysis can be used to analyze historical data, such as letters, diaries, and newspapers, to provide insights into historical events and social contexts.
Textual Analysis in Cultural and Media Studies
Textual analysis is a key tool in cultural and media studies as it enables researchers to analyze the meanings, representations, and discourses present in cultural and media texts. Here are some ways that textual analysis is used in cultural and media studies:
- To analyze representation: Textual analysis can be used to analyze the representation of different social groups, such as gender, race, and sexuality, in media and cultural texts. This analysis can provide insights into how these groups are constructed and represented in society.
- To analyze cultural meanings: Textual analysis can be used to analyze the cultural meanings and symbols present in media and cultural texts. This analysis can provide insights into how culture and society are constructed and understood.
- To analyze discourse: Textual analysis can be used to analyze the discourse present in cultural and media texts. This analysis can provide insights into how language is used to construct meaning and power relations.
- To analyze media content: Textual analysis can be used to analyze media content, such as news articles, TV shows, and films, to understand how they shape our understanding of the world around us.
- To analyze advertising : Textual analysis can be used to analyze advertising campaigns to understand how they construct meanings, identities, and desires.
Textual Analysis in the Social Sciences
Textual analysis is a valuable tool in the social sciences as it enables researchers to analyze and interpret text data in a systematic and rigorous way. Here are some ways that textual analysis is used in the social sciences:
- To analyze interview data: Textual analysis can be used to analyze interview data, such as transcribed interviews, to identify patterns and themes in the data.
- To analyze survey responses: Textual analysis can be used to analyze survey responses to identify patterns and themes in the data.
- To analyze social media data: Textual analysis can be used to analyze social media data, such as tweets and Facebook posts, to identify patterns and themes in the data.
- To analyze policy documents: Textual analysis can be used to analyze policy documents, such as government reports and legislation, to identify discourses and power relations present in the policy.
- To analyze historical data: Textual analysis can be used to analyze historical data, such as letters and diaries, to provide insights into historical events and social contexts.
Textual Analysis in Literary Studies
Textual analysis is a key tool in literary studies as it enables researchers to analyze and interpret literary texts in a systematic and rigorous way. Here are some ways that textual analysis is used in literary studies:
- To analyze narrative structure: Textual analysis can be used to analyze the narrative structure of a literary text, such as identifying the plot, character development, and point of view.
- To analyze language and style: Textual analysis can be used to analyze the language and style used in a literary text, such as identifying figurative language, symbolism, and rhetorical devices.
- To analyze themes and motifs: Textual analysis can be used to analyze the themes and motifs present in a literary text, such as identifying recurring symbols, themes, and motifs.
- To analyze historical and cultural context: Textual analysis can be used to analyze the historical and cultural context of a literary text, such as identifying how the text reflects the social and political context of its time.
- To analyze intertextuality: Textual analysis can be used to analyze the intertextuality of a literary text, such as identifying how the text references or is influenced by other literary works.
Textual Analysis Methods
Textual analysis methods are techniques used to analyze and interpret various types of text, including written documents, audio and video recordings, and online content. These methods are commonly used in fields such as linguistics, communication studies, sociology, psychology, and literature.
Some common textual analysis methods include:
Content Analysis
This involves identifying patterns and themes within a set of text data. This method is often used to analyze media content or other types of written materials, such as policy documents or legal briefs.
Discourse Analysis
This involves examining how language is used to construct meaning in social contexts. This method is often used to analyze political speeches or other types of public discourse.
Critical Discourse Analysis
This involves examining how power and social relations are constructed through language use, particularly in political and social contexts.
Narrative Analysis
This involves examining the structure and content of stories or narratives within a set of text data. This method is often used to analyze literary texts or oral histories.
This involves analyzing the meaning of signs and symbols within a set of text data. This method is often used to analyze advertising or other types of visual media.
Text mining
This involves using computational techniques to extract patterns and insights from large sets of text data. This method is often used in fields such as marketing and social media analysis.
Close Reading
This involves a detailed and in-depth analysis of a particular text, focusing on the language, style, and literary techniques used by the author.
How to Conduct Textual Analysis
Here are some general steps to conduct textual analysis:
- Choose your research question: Define your research question and identify the text or set of texts that you want to analyze.
- F amiliarize yourself with the text: Read and re-read the text, paying close attention to its language, structure, and content. Take notes on key themes, patterns, and ideas that emerge.
- Choose your analytical approach: Select the appropriate analytical approach for your research question, such as close reading, thematic analysis, content analysis, or discourse analysis.
- Create a coding scheme: If you are conducting content analysis, create a coding scheme to categorize and analyze the content of the text. This may involve identifying specific words, themes, or ideas to code.
- Code the text: Apply your coding scheme to the text and systematically categorize the content based on the identified themes or patterns.
- Analyze the data: Once you have coded the text, analyze the data to identify key patterns, themes, or trends. Use appropriate software or tools to help with this process if needed.
- Draw conclusions: Draw conclusions based on your analysis and answer your research question. Present your findings and provide evidence to support your conclusions.
- R eflect on limitations and implications: Reflect on the limitations of your analysis, such as any biases or limitations of the selected method. Also, discuss the implications of your findings and their relevance to the broader research field.
When to use Textual Analysis
Textual analysis can be used in various research fields and contexts. Here are some situations when textual analysis can be useful:
- Understanding meaning and interpretation: Textual analysis can help understand the meaning and interpretation of text, such as literature, media, and social media.
- Analyzing communication patterns: Textual analysis can be used to analyze communication patterns in different contexts, such as political speeches, social media conversations, and legal documents.
- Exploring cultural and social contexts: Textual analysis can be used to explore cultural and social contexts, such as the representation of gender, race, and identity in media.
- Examining historical documents: Textual analysis can be used to examine historical documents, such as letters, diaries, and newspapers.
- Evaluating marketing and advertising campaigns: Textual analysis can be used to evaluate marketing and advertising campaigns, such as analyzing the language, symbols, and imagery used.
Examples of Textual Analysis
Here are a few examples:
- Media Analysis: Textual analysis is frequently used in media studies to examine how news outlets and social media platforms frame and present news stories. Researchers can use textual analysis to examine the language and images used in news articles, tweets, and other forms of media to identify patterns and biases.
- Customer Feedback Analysis: Textual analysis is often used by businesses to analyze customer feedback, such as online reviews or social media posts, to identify common themes and areas for improvement. This allows companies to make data-driven decisions and improve their products or services.
- Political Discourse Analysis: Textual analysis is commonly used in political science to analyze political speeches, debates, and other forms of political communication. Researchers can use this method to identify the language and rhetoric used by politicians, as well as the strategies they employ to appeal to different audiences.
- Literary Analysis: Textual analysis is a fundamental tool in literary criticism, allowing scholars to examine the language, structure, and themes of literary works. This can involve close reading of individual texts or analysis of larger literary movements.
- Sentiment Analysis: Textual analysis is used to analyze social media posts, customer feedback, or other sources of text data to determine the sentiment of the text. This can be useful for businesses or organizations to understand how their brand or product is perceived in the market.
Purpose of Textual Analysis
There are several specific purposes for using textual analysis, including:
- To identify and interpret patterns in language use: Textual analysis can help researchers identify patterns in language use, such as common themes, recurring phrases, and rhetorical devices. This can provide insights into the values and beliefs that underpin the text.
- To explore the cultural context of the text: Textual analysis can help researchers understand the cultural context in which the text was produced, including the historical, social, and political factors that shaped the language and messages.
- To examine the intended and unintended meanings of the text: Textual analysis can help researchers uncover both the intended and unintended meanings of the text, and to explore how the language is used to convey certain messages or values.
- To understand how texts create and reinforce social and cultural identities: Textual analysis can help researchers understand how texts contribute to the creation and reinforcement of social and cultural identities, such as gender, race, ethnicity, and nationality.
Applications of Textual Analysis
Here are some common applications of textual analysis:
Media Studies
Textual analysis is frequently used in media studies to analyze news articles, advertisements, and social media posts to identify patterns and biases in media representation.
Literary Criticism
Textual analysis is a fundamental tool in literary criticism, allowing scholars to examine the language, structure, and themes of literary works.
Political Science
Textual analysis is commonly used in political science to analyze political speeches, debates, and other forms of political communication.
Marketing and Consumer Research
Textual analysis is used to analyze customer feedback, such as online reviews or social media posts, to identify common themes and areas for improvement.
Healthcare Research
Textual analysis is used to analyze patient feedback and medical records to identify patterns in patient experiences and improve healthcare services.
Social Sciences
Textual analysis is used in various fields within social sciences, such as sociology, anthropology, and psychology, to analyze various forms of data, including interviews, field notes, and documents.
Linguistics
Textual analysis is used in linguistics to study language use and its relationship to social and cultural contexts.
Advantages of Textual Analysis
There are several advantages of textual analysis in research. Here are some of the key advantages:
- Systematic and objective: Textual analysis is a systematic and objective method of analyzing text data. It enables researchers to analyze text data in a consistent and rigorous way, minimizing the risk of bias or subjectivity.
- Versatile : Textual analysis can be used to analyze a wide range of text data, including interview transcripts, survey responses, social media data, policy documents, and literary texts.
- Efficient : Textual analysis can be a more efficient method of data analysis compared to manual coding or other methods of qualitative analysis. With the help of software tools, researchers can process large volumes of text data more quickly and accurately.
- Allows for in-depth analysis: Textual analysis enables researchers to conduct in-depth analysis of text data, uncovering patterns and themes that may not be visible through other methods of data analysis.
- Can provide rich insights: Textual analysis can provide rich and detailed insights into complex social phenomena. It can uncover subtle nuances in language use, reveal underlying meanings and discourses, and shed light on the ways in which social structures and power relations are constructed and maintained.
Limitations of Textual Analysis
While textual analysis can provide valuable insights into the ways in which language is used to convey meaning and create social and cultural identities, it also has several limitations. Some of these limitations include:
- Limited Scope : Textual analysis is only able to analyze the content of written or spoken language, and does not provide insights into non-verbal communication such as facial expressions or body language.
- Subjectivity: Textual analysis is subject to the biases and interpretations of the researcher, as well as the context in which the language was produced. Different researchers may interpret the same text in different ways, leading to inconsistencies in the findings.
- Time-consuming: Textual analysis can be a time-consuming process, particularly if the researcher is analyzing a large amount of text. This can be a limitation in situations where quick analysis is necessary.
- Lack of Generalizability: Textual analysis is often used in qualitative research, which means that its findings cannot be generalized to larger populations. This limits the ability to draw conclusions that are applicable to a wider range of contexts.
- Limited Accessibility: Textual analysis requires specialized skills and training, which may limit its accessibility to researchers who are not trained in this method.
About the author
Muhammad Hassan
Researcher, Academic Writer, Web developer
You may also like

Cluster Analysis – Types, Methods and Examples

Questionnaire – Definition, Types, and Examples

Discriminant Analysis – Methods, Types and...

Case Study – Methods, Examples and Guide

MANOVA (Multivariate Analysis of Variance) –...

Observational Research – Methods and Guide
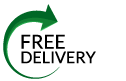
The Plagiarism Checker Online For Your Academic Work
Start Plagiarism Check
Editing & Proofreading for Your Research Paper
Get it proofread now
Online Printing & Binding with Free Express Delivery
Configure binding now
- Academic essay overview
- The writing process
- Structuring academic essays
- Types of academic essays
- Academic writing overview
- Sentence structure
- Academic writing process
- Improving your academic writing
- Titles and headings
- APA style overview
- APA citation & referencing
- APA structure & sections
- Citation & referencing
- Structure and sections
- APA examples overview
- Commonly used citations
- Other examples
- British English vs. American English
- Chicago style overview
- Chicago citation & referencing
- Chicago structure & sections
- Chicago style examples
- Citing sources overview
- Citation format
- Citation examples
- College essay overview
- Application
- How to write a college essay
- Types of college essays
- Commonly confused words
- Definitions
- Dissertation overview
- Dissertation structure & sections
- Dissertation writing process
- Graduate school overview
- Application & admission
- Study abroad
- Master degree
- Harvard referencing overview
- Language rules overview
- Grammatical rules & structures
- Parts of speech
- Punctuation
- Methodology overview
- Analyzing data
- Experiments
- Observations
- Inductive vs. Deductive
- Qualitative vs. Quantitative
- Types of validity
- Types of reliability
- Sampling methods
- Theories & Concepts
- Types of research studies
- Types of variables
- MLA style overview
- MLA examples
- MLA citation & referencing
- MLA structure & sections
- Plagiarism overview
- Plagiarism checker
- Types of plagiarism
- Printing production overview
- Research bias overview
- Types of research bias
- Example sections
- Types of research papers
- Research process overview
- Problem statement
- Research proposal
- Research topic
- Statistics overview
- Levels of measurment
- Frequency distribution
- Measures of central tendency
- Measures of variability
- Hypothesis testing
- Parameters & test statistics
- Types of distributions
- Correlation
- Effect size
- Hypothesis testing assumptions
- Types of ANOVAs
- Types of chi-square
- Statistical data
- Statistical models
- Spelling mistakes
- Tips overview
- Academic writing tips
- Dissertation tips
- Sources tips
- Working with sources overview
- Evaluating sources
- Finding sources
- Including sources
- Types of sources
Your Step to Success
Plagiarism Check within 10min
Printing & Binding with 3D Live Preview
Textual Analysis – Definition, Approaches & Fields
How do you like this article cancel reply.
Save my name, email, and website in this browser for the next time I comment.
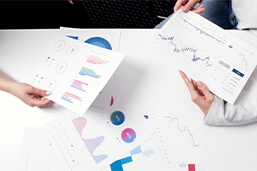
Textual communication surrounds a large part of the methodology used by researchers to analyze and interpret texts by studying their style, content, structure, purpose, and underlying meaning. Besides analyzing the text, textual analysis gives us a better and more detailed idea of how people communicate and represent their experiences and perspectives through writing. This article will outline the different approaches to textual analysis and in what fields of study it is commonly used.
Inhaltsverzeichnis
- 1 Textual Analysis – In a Nutshell
- 2 Definition: Textual analysis
- 3 What are the approaches to textual analysis?
- 4 Different fields of textual analysis
Textual Analysis – In a Nutshell
- Text can be defined simply as any instance of language in any medium that makes sense to someone who knows the language, e.g., newspaper articles and transcripts of spoken interviews and observations.
- The purpose of textual analysis is to describe the functions, content, and structure of messages contained in a text.
- This type of analysis is used in different fields, including literary studies (where it is most common), social sciences, and cultural and media studies.
Definition: Textual analysis
Textual analysis is a broad term that entails various research methods, which allow to analyze, describe, and interpret the characteristics of a recorded message. There are four main approaches typically used in academic writing. Nevertheless, textual analysis is also used in other fields, such as in cultural and media studies, social sciences, and literary studies.
An example of textual analysis is brand monitoring to determine social opinion on your brand in various contexts.
What are the approaches to textual analysis?
There are four main approaches to textual analysis, which are listed and discussed below:
1. Rhetorical criticism
Rhetorical criticism is the systematic method of describing, analyzing, interpreting, and evaluating the persuasive force of messages within texts. At its core, rhetorical criticism is simply analyzing the use of rhetoric.
The process of rhetorical criticism has four steps :
- Select the text to study
- Choose the type of rhetorical criticism
- Analyze the chosen text using the chosen method
- Write a critical essay
The process of rhetorical criticism serves essential functions , including:
- Helping in understanding the historical, cultural, and social contexts
- Researchers can use it to evaluate society as a form of social criticism
- Helps illuminate the purpose of persuasive messages
- Teaching people what makes effective persuasion and how persuasion works
2. Content analysis
Content analysis is the analysis of specific message characteristics in a piece of text. It is usually considered unobtrusive since the analyzed texts already exist and are not being produced on request.
Content analysis can be both qualitative and quantitative, as described below:
- Quantitative – A systematic step-by-step procedure used to test hypotheses and answer research questions
- Qualitative – An approach that is more interested in the meanings of messages than the frequency of the occurrence of message variables.
The goals of content analysis include:
- Understanding the intentions of groups and individuals
- Identifying bias and propaganda
- Finding connections in how concepts are communicated
- Identifying communication differences in different contexts
3. Interaction analysis
Interaction analysis is the process of evaluating processes, determining the data requirements of each, and generating a matrix of what data is used by what process.
An interaction analysis involves two general tasks:
- Obtain a sample of interaction : When gathering a sample, the researchers’ choices affect the quality and type of data collected. Their choices determine if the interaction will be natural or structured, whether the location will be in a laboratory or another area, and the exact methods used to collect data.
- Analyzing the sample : Specific analysis of the collected samples will depend on the researchers’ goals and the form of the collected data.
4. Performance Studies
Performance studies is an approach that uses performance as the lens to study the world. There are six primary steps to undertaking a performance study:

Different fields of textual analysis
Textual analysis is a critical component of research in various fields of study, as discussed below.
Textual analysis in cultural and media studies
Researchers in cultural and media studies take media and cultural objects and treat them as texts to be analyzed.
- Media content
- Music videos
- Social media content.
Researchers in this field usually seek to connect contemporary culture and politics with text elements. In this context, the analysis is usually qualitative and creative. The different aspects of a text that a researcher may analyze include:
- The relationship with other relevant texts
- The design elements
- The word choice
- The location of the text
- The intended audience
Textual analysis in social sciences
Textual analysis in the social sciences is usually applied to texts like surveys, interview transcripts, and different media types to draw conclusions about social relations.
In this field, textual analysis usually takes a more quantitative approach, where certain text features’ frequency is measured numerically.
A researcher may investigate which words in a particular language are used most commonly, or which colors feature most in different advertisements aimed at different demographics.
Common methods of analyzing text in this field include discourse analysis, content analysis , and thematic analysis .
Textual analysis in literary studies
One of the most common uses of textual analysis is found in literary studies. Almost all works in this field – poems, plays, stories, novels – involve a comprehensive analysis. As this field deals with literary texts, a greater emphasis is usually placed on deliberately constructed elements such as the narrator’s voice and the rhyming scheme. In understanding these elements, the researcher lends more meaning to the text.
Textual analysis in this field also explores what the text reveals from the perspective through which the text was written, and finds new and unexpected ways to analyze classic pieces of text.
How do you write a textual analysis?
To write this analysis, the writer should analyze the structure, characters, setting, citations of a text, and central idea and themes. Consider the what, who, why, and where of the text you are analyzing.
What are the common approaches to textual analysis?
There are four primary textual analysis approaches:
- Rhetorical criticism
- Content analysis
- Interaction analysis
- Performance studies
What are the key features of textual analysis?
The key features of a textual analysis essay include:
- The summary and context of a text
- A statement of intent
- An explanation of the text’s continuing relevance
Why do we need to analyze texts?
The analysis helps extract a deeper meaning from a specific text and discover different perspectives in context.
We use cookies on our website. Some of them are essential, while others help us to improve this website and your experience.
- External Media
Individual Privacy Preferences
Cookie Details Privacy Policy Imprint
Here you will find an overview of all cookies used. You can give your consent to whole categories or display further information and select certain cookies.
Accept all Save
Essential cookies enable basic functions and are necessary for the proper function of the website.
Show Cookie Information Hide Cookie Information
Statistics cookies collect information anonymously. This information helps us to understand how our visitors use our website.
Content from video platforms and social media platforms is blocked by default. If External Media cookies are accepted, access to those contents no longer requires manual consent.
Privacy Policy Imprint
Literature Review: What Is Textual Analysis, and Can We Use It to Measure Corporate Culture?
- First Online: 25 April 2023
Cite this chapter
- Terry Harris 2
327 Accesses
The textual analysis approach also sometimes referred to as content analysis, computational linguistics, information retrieval, natural language processing, etc., refers to the systematic and objective quantification of the semantic content contained in a body of text. This notion of the parsing text to discover patterns allows for the unearthing of valuable information in text and has a long history and has been applied in many different contexts and disciplines.
This is a preview of subscription content, log in via an institution to check access.
Access this chapter
- Available as EPUB and PDF
- Read on any device
- Instant download
- Own it forever
- Compact, lightweight edition
- Dispatched in 3 to 5 business days
- Free shipping worldwide - see info
- Durable hardcover edition
Tax calculation will be finalised at checkout
Purchases are for personal use only
Institutional subscriptions
In later work, Bodnaruk et al. ( 2015 ) adopt a similar methodology to that used in Loughran and McDonald ( 2011 ) and propose “constraining” words, which are those words that suggest some financial constraint, for inclusion in the Loughran and McDonald word list.
Ahern, K. R., & Sosyura, D. (2014). Who Writes the News? Corporate Press Releases During Merger Negotiations. The Journal of Finance, 69 , 241–291.
Article Google Scholar
Andreou, P. C., Fiordelisi, F., Harris, T., & Philip, D. (2021). Institutional Ownership and Firms’ Thrust to Compete. British Journal of Management, 33 , 1346–1370.
Andreou, P. C., Harris, T., & Philip, D. (2020). Measuring Firms’ Market Orientation Using Textual Analysis of 10-K Filings. British Journal of Management, 31 , 872–895.
Antweiler, W., & Frank, M. Z. (2004). Is All That Talk Just Noise? The Information Content of Internet Stock Message Boards. The Journal of Finance, 59 , 1259–1294.
Audi, R., Loughran, T., & McDonald, B. (2016). Trust, but Verify: MD&A Language and the Role of Trust in Corporate Culture. Journal of Business Ethics, 139 , 551–561.
Barth, A. (2016). The Role of Corporate Culture in the Financial Industry . Available at SSRN 2707078.
Google Scholar
Bellstam, G., Bhagat, S., & Cookson, J. A. (2019). A Text-Based Analysis of Corporate Innovation . Available at SSRN 2803232.
Bhandari, A., Mammadov, B., Thevenot, M., & Vakilzadeh, S. H. (2017). The Invisible Hand: Corporate Culture and Its Implications for Earnings Management (Working Paper). Warrington College of Business, Gainesville, FL.
Biddle, G. C., Hilary, G., & Verdi, R. S. (2009). How does Financial Reporting Quality Relate to Investment Efficiency? Journal of Accounting and Economics, 48 , 112–131.
Blei, D. M., Ng, A. Y., & Jordan, M. I. (2003). Latent Dirichlet Allocation. Journal of Machine Learning Research, 3 , 993–1022.
Bodnaruk, A., Loughran, T., & McDonald, B. (2015). Using 10-K Text to Gauge Financial Constraints. Journal of Financial and Quantitative Analysis, 50 , 623–646.
Bonsall, S. B., IV., Leone, A. J., Miller, B. P., & Rennekamp, K. (2017). A Plain English Measure of Financial Reporting Readability. Journal of Accounting and Economics, 63 , 329–357.
Boukus, E., & Rosenberg, J. V. (2006). The Information Content of FOMC Minutes . Available at SSRN 922312.
Bushman, R. M., Hendricks, B. E., & Williams, C. D. (2016). Bank Competition: Measurement, Decision-Making, and Risk-Taking. Journal of Accounting Research, 54 , 777–826.
Chen, H., De, P., Hu, Y. J., & Hwang, B. H. (2014). Wisdom of Crowds: The Value of Stock Opinions Transmitted Through Social Media. The Review of Financial Studies , 27 , 1367–1403.
Cohen, L., Malloy, C., & Nguyen, Q. (2020). Lazy prices. The Journal of Finance, 75 , 1371–1415.
Da, Z., Engelberg, J., & Gao, P. (2015). The Sum of All FEARS Investor Sentiment and Asset Prices. The Review of Financial Studies, 28 , 1–32.
Davis, A. K., Piger, J. M., & Sedor, L. M. (2012). Beyond the Numbers: Measuring the Information Content of Earnings Press Release Language. Contemporary Accounting Research, 29 , 845–868.
Davis, A. K., & Tama-Sweet, I. (2012). Managers’ Use of Language Across Alternative Disclosure Outlets: Earnings Press Releases Versus MD&A. Contemporary Accounting Research, 29 , 804–837.
Das, S. R., & Chen, M. Y. (2007). Yahoo! for Amazon: Sentiment Extraction from Small Talk on the Web. Management Science, 53 , 1375–1388.
Doran, J. S., Peterson, D. R., & Price, S. M. (2012). Earnings Conference Call Content and Stock Price: The Case of REITs. The Journal of Real Estate Finance and Economics, 45 , 402–434.
Dougal, C., Engelberg, J., Garcia, D., & Parsons, C. A. (2012). Journalists and the Stock Market. The Review of Financial Studies, 25 , 639–679.
Dyer, T., Lang, M., & Stice-Lawrence, L. (2017). The Evolution of 10-K Textual Disclosure: Evidence from Latent Dirichlet Allocation. Journal of Accounting and Economics, 64 , 221–245.
Feldman, R., Govindaraj, S., Livnat, J., & Segal, B. (2010). Management’s Tone Change, Post Earnings Announcement Drift and Accruals. Review of Accounting Studies, 15 , 915–953.
Ferris, S. P., Hao, Q., & Liao, M. Y. (2013). The Effect of Issuer Conservatism on IPO Pricing and Performance. Review of Finance, 17 , 993–1027.
Fiordelisi, F., & Ricci, O. (2014). Corporate Culture and CEO Turnover. Journal of Corporate Finance, 28 , 66–82.
Fiordelisi, F., & Ricci, O. (2021). Corrigendum to “Corporate Culture and CEO Turnover”. Journal of Corporate Finance, 70 , 102064.
Fiordelisi, F., Renneboog, L., Ricci, O., & Lopes, S. S. (2019). Creative Corporate Culture and Innovation. Journal of International Financial Markets, Institutions and Money, 63 , 101137.
Garcia, D. (2013). Sentiment During Recessions. The Journal of Finance, 68 , 1267–1300.
Graham, J. R., Harvey, C. R., Grennen, J., & Rajgopal, S. (2022). Corporate Culture: Evidence from the Field. Journal of Financial Economics, 146 , 552–593.
Grennan, J. (2019). A Corporate Culture Channel: How Increased Shareholder Governance Reduces Firm Value . Available at SSRN 2345384.
Guiso, L., Sapienza, P., & Zingales, L. (2015a). Corporate Culture, Societal Culture, and Institutions. American Economic Review, 105 , 336–339.
Guiso, L., Sapienza, P., & Zingales, L. (2015b). The Value of Corporate Culture. Journal of Financial Economics, 117 , 60–76.
Guo, H., Yin, C., & Zeng, Y. (2022). How Does Firm‐Specific Investor Sentiment Affect the Value of Corporate Cash Holdings? British Journal of Management .
Hanley, K. W., & Hoberg, G. (2010). The Information Content of IPO Prospectuses. The Review of Financial Studies, 23 , 2821–2864.
Henry, E. (2008). Are Investors Influenced by How Earnings Press Releases Are Written? The Journal of Business Communication, 45 , 363–407.
Heston, S. L., & Sinha, N. R. (2015). News Versus Sentiment: Predicting Stock Returns from News Stories . Available at SSRN 2311310.
Hillert, A., Niessen-Ruenzi, A., & Ruenzi, S. (2014). Mutual Fund Shareholder Letter Tone-Do Investors Listen? (Working Paper). University of Mannheim, Mannheim, Germany.
Hoberg, G., & Phillips, G. (2010). Product Market Synergies and Competition in Mergers and Acquisitions: A Text-Based Analysis. Review of Financial Studies, 23 , 3773–3811.
Hoberg, G., & Phillips, G. (2016). Text-Based Network Industries and Endogenous Product Differentiation. Journal of Political Economy, 124 , 1423–1465.
Hoberg, G., Phillips, G., & Prabhala, N. (2014). Product Market Threats, Payouts, and Financial Flexibility. The Journal of Finance, 69 , 293–324.
Huang, A. H., Zang, A. Y., & Zheng, R. (2014a). Evidence on the Information Content of Text in Analyst Reports. The Accounting Review, 89 , 2151–2180.
Huang, X., Teoh, S. H., & Zhang, Y. (2014b). Tone Management. The Accounting Review, 89 , 1083–1113.
Kearney, C., & Liu, S. (2014). Textual Sentiment in Finance: A survey of Methods and Models. International Review of Financial Analysis, 33 , 171–185.
Kothari, S. P., Li, X., & Short, J. E. (2009). The Effect of Disclosures by Management, Analysts, and Business Press on Cost of Capital, Return Volatility, and Analyst Forecasts: A Study Using Content Analysis. The Accounting Review, 84 , 1639–1670.
Lawrence, A. (2013). Individual Investors and Financial Disclosure. Journal of Accounting and Economics, 56 , 130–147.
Lehavy, R., Li, F., & Merkley, K. (2011). The Effect of Annual Report Readability on Analyst Following and the Properties of Their Earnings Forecasts. The Accounting Review, 86 , 1087–1115.
Li, E. X., & Ramesh, K. (2009). Market Reaction Surrounding the Filing of Periodic SEC Reports. The Accounting Review, 84 , 1171–1208.
Li, F. (2008). Annual Report Readability, Current Earnings, and Earnings Persistence. Journal of Accounting and Economics, 45 , 221–247.
Li, F. (2010a). Textual Analysis of Corporate Disclosures: A Survey of the Literature. Journal of Accounting Literature, 29 , 143.
Li, F. (2010b). The Information Content of Forward-Looking Statements in Corporate Filings: A Naive Bayesian Machine Learning Approach. Journal of Accounting Research, 48 , 1049–1102.
Li, F. (2011). Managers’ Self-serving Attribution Bias, Overconfidence, and Corporate Financial Policies (Working Paper). University of Michigan, Ann Arbor, MI.
Li, F., Lundholm, R., & Minnis, M. (2013a). A Measure of Competition Based on 10-K Filings. Journal of Accounting Research, 51 , 399–436.
Li, K., Griffin, D., Yue, H., & Zhao, L. (2013b). How Does Culture Influence Corporate Risk-Taking? Journal of Corporate Finance, 23 , 1–22.
Li, K., Liu, X., Mai, F., & Zhang, T. (2020). The Role of Corporate Culture in Bad Times, Evidence from the COVID-19 Pandemic. Journal of Financial and Quantitative Analysis , 1–68.
Li, X. (2010c). The Impacts of Product Market Competition on the Quantity and Quality of Voluntary Disclosures. Review of Accounting Studies, 15 , 663–711.
Loughran, T., McDonald, B., & Yun, H. (2009). A Wolf in Sheep’s Clothing: The Use of Ethics-Related Terms in 10-K Reports. Journal of Business Ethics, 89 , 39–49.
Loughran, T., & McDonald, B. (2011). When Is a Liability Not a Liability? Textual Analysis, Dictionaries, and 10-Ks. The Journal of Finance, 66 , 35–65.
Loughran, T., & McDonald, B. (2013). IPO First-Day Returns, Offer Price Revisions, Volatility, and Form S-1 Language. Journal of Financial Economics, 109 , 307–326.
Loughran, T., & McDonald, B. (2014). Measuring Readability in Financial Disclosures. The Journal of Finance, 69 , 1643–1671.
Loughran, T., & McDonald, B. (2016). Textual Analysis in Accounting and Finance: A Survey. Journal of Accounting Research, 54 , 1187–1230.
Luu, H., Nguyen, L., & Wilson, J. O. S. (2022). Organizational Culture, Competition and Bank Loan Loss Provisions . Available at SSRN 3921043.
Mayew, W. J., & Venkatachalam, M. (2012). The Power of Voice: Managerial Affective States and Future Firm Performance. The Journal of Finance, 67 , 1–43.
Miller, B. P. (2010). The Effects of Reporting Complexity on Small and Large Investor Trading. The Accounting Review, 85 , 2107–2143.
Mosteller, F., & Wallace, D. (1964). Inference and Disputed Authorship: The Federalist . Addison-Wesley.
Nelson, K. K., & Pritchard, A. C. (2007). Litigation Risk and Voluntary Disclosure: The Use of Meaningful Cautionary Language. Available at SSRN 998590.
Nguyen, D. D., Hagendorff, J., & Eshraghi, A. (2018). Does a CEO’s Cultural Heritage Affect Performance Under Competitive Pressure? The Review of Financial Studies, 31 , 97–141.
Nguyen, D. D., Nguyen, L., & Sila, V. (2019). Does Corporate Culture Affect Bank Risk-Taking? Evidence from Loan-Level Data. British Journal of Management, 30 , 106–133.
Price, S. M., Doran, J. S., Peterson, D. R., & Bliss, B. A. (2012). Earnings Conference Calls and Stock Returns: The Incremental Informativeness of Textual Tone. Journal of Banking & Finance, 36 , 992–1011.
Rogers, J. L., Van Buskirk, A., & Zechman, S. L. (2011). Disclosure Tone and Shareholder Litigation. The Accounting Review, 86 , 2155–2183.
Simon, A. (1997). Administrative Behaviour (4th ed.). The Free Press.
Solomon, D. H., Soltes, E., & Sosyura, D. (2014). Winners in the Spotlight: Media Coverage of Fund Holdings as a Driver of Flows. Journal of Financial Economics, 113 , 53–72.
Tetlock, P. C. (2007). Giving Content to Investor Sentiment: The Role of Media in the Stock Market. The Journal of Finance, 62 , 1139–1168.
Tetlock, P. C., Saar-Tsechansky, M., & Macskassy, S. (2008). More Than Words: Quantifying Language to Measure Firms’ Fundamentals. The Journal of Finance, 63 , 1437–1467.
Download references
Author information
Authors and affiliations.
Accounting Department, Durham University, Durham, UK
Terry Harris
You can also search for this author in PubMed Google Scholar
Corresponding author
Correspondence to Terry Harris .
Rights and permissions
Reprints and permissions
Copyright information
© 2023 The Author(s), under exclusive license to Springer Nature Switzerland AG
About this chapter
Harris, T. (2023). Literature Review: What Is Textual Analysis, and Can We Use It to Measure Corporate Culture?. In: Competition Culture and Corporate Finance. Palgrave Macmillan, Cham. https://doi.org/10.1007/978-3-031-30156-8_3
Download citation
DOI : https://doi.org/10.1007/978-3-031-30156-8_3
Published : 25 April 2023
Publisher Name : Palgrave Macmillan, Cham
Print ISBN : 978-3-031-30155-1
Online ISBN : 978-3-031-30156-8
eBook Packages : Economics and Finance Economics and Finance (R0)
Share this chapter
Anyone you share the following link with will be able to read this content:
Sorry, a shareable link is not currently available for this article.
Provided by the Springer Nature SharedIt content-sharing initiative
- Publish with us
Policies and ethics
- Find a journal
- Track your research
- How it works
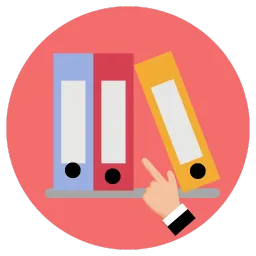
A Quick Guide to Textual Analysis – Definition & Steps
Published by Alvin Nicolas at August 16th, 2021 , Revised On August 29, 2023
Textual analysis is the method of analysing and understanding the text. It includes the close reading of fictional texts, images symbols, and all forms of written literary texts. A researcher needs to look keenly at the text to identify the writer’s context and its message.
What is a Text?
- A text can be anything from which you can extract any meaning or information.
- A text can be a written book, records, journals, newspapers, magazines, ornaments, objects, emails, or transcribed conversations.
- It also includes analysing events, places, videos, images, or movies to get in-depth knowledge about its creation and purpose.
Why do we Call it a Text?
You might be thinking, why don’t we call it a book, video, or magazine? Why do we call it a text ?
The word text means the post-structuralist approach to thinking about the development of meaning.
The Purpose of Textual Analysis
The purpose of your textual analysis depends on the object of your analysis, such as:
- Analysis of a video or movie may aim to determine the target audience, dialogues, cinematography, visual and sound effects, and the message conveyed through his work.
- Analysis of texts and short stories focus on the language, narrative, choice of words, imagery, perception of the writer, and organisation of the text.
- You can analyse a monument based on its architectural history, stories related to it, and visitors’ navigation.
- You can analyse images in terms of the photographer’s creativity, use of a camera, sense of selecting locations, skills of capturing images, etc.
- You can analyse paintings in terms of their artistic level, use of colours, perception of the painters, the message hidden in the image, etc.
Confused between qualitative and quantitative methods of data analysis? No idea what discourse and content analysis are?
We hear you.
- Whether you want a full dissertation written or need help forming a dissertation proposal, we can help you with both.
- Get different dissertation services at ResearchProspect and score amazing grades!
Textual Analysis of Cultural and Media Studies
A researcher uses videos, social media content, newspapers, maps, buildings, advertisements, and images. Textual analysis plays a key role in the fields of cultural and media studies. A researcher aims to analyse, interpret, and analyse the controversial issues and messages embedded within the text and tries to connect them with other texts or events.
These kinds of texts are analysed from the following aspects:
- Content of the texts
- Language and word choice
- Elements of creativity
- Target audience
- Association with other texts or events
It focuses primarily on the creative and qualitative approach of the text. A researcher identifies the context and hidden message of the text.
Textual Analysis in the Social Sciences
It includes the textual analysis of the interview transcripts and surveys, and other media, including TV programs, chats, social media content. It takes a quantitative approach to measure the characteristics and organisation of the text. You can measure the text’s repetitions, types of colours displayed, tone of the speakers, etc.
Content analysis , thematic analysis , and discourse analysis are the most frequent ways to analyse these texts.
Literary Text Analysis
The literary text analysis is breaking a text’s structure and content into smaller parts to seek in-depth understanding. A researcher It includes stories, poetry, plays, novels, plays, and books.
Also read about: Correlational research , a comprehensive guide on its types with examples
It focuses on identifying:
- The association between various individual texts.
- The similarities between the concepts of the two separate texts.
- The relationship between the texts and political, social, aesthetic, economic, or religious contexts.
Frequently Asked Questions
How to describe textual analysis.
Textual analysis examines written, visual, or spoken material to uncover layers of meaning, themes, and patterns. It dissects language, structure, and context to reveal how elements convey messages, ideologies, and cultural insights, often contributing to a deeper understanding of the content’s significance.
You May Also Like
A survey includes questions relevant to the research topic. The participants are selected, and the questionnaire is distributed to collect the data.
Descriptive research is carried out to describe current issues, programs, and provides information about the issue through surveys and various fact-finding methods.
A meta-analysis is a formal, epidemiological, quantitative study design that uses statistical methods to generalise the findings of the selected independent studies.
USEFUL LINKS
LEARNING RESOURCES
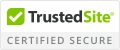
COMPANY DETAILS
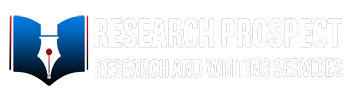
- How It Works
- Architecture and Design
- Asian and Pacific Studies
- Business and Economics
- Classical and Ancient Near Eastern Studies
- Computer Sciences
- Cultural Studies
- Engineering
- General Interest
- Geosciences
- Industrial Chemistry
- Islamic and Middle Eastern Studies
- Jewish Studies
- Library and Information Science, Book Studies
- Life Sciences
- Linguistics and Semiotics
- Literary Studies
- Materials Sciences
- Mathematics
- Social Sciences
- Sports and Recreation
- Theology and Religion
- Publish your article
- The role of authors
- Promoting your article
- Abstracting & indexing
- Publishing Ethics
- Why publish with De Gruyter
- How to publish with De Gruyter
- Our book series
- Our subject areas
- Your digital product at De Gruyter
- Contribute to our reference works
- Product information
- Tools & resources
- Product Information
- Promotional Materials
- Orders and Inquiries
- FAQ for Library Suppliers and Book Sellers
- Repository Policy
- Free access policy
- Open Access agreements
- Database portals
- For Authors
- Customer service
- People + Culture
- Journal Management
- How to join us
- Working at De Gruyter
- Mission & Vision
- De Gruyter Foundation
- De Gruyter Ebound
- Our Responsibility
- Partner publishers

Your purchase has been completed. Your documents are now available to view.
9. Textual Analysis as a Research Method
From the book research methods for english studies.
- Catherine Belsey
- X / Twitter
Supplementary Materials
Please login or register with De Gruyter to order this product.
Chapters in this book (17)

- Copley Library
- Legal Research Center
- < Previous
Home > Theses and Dissertations > Dissertations > 1024
Dissertations
Ennobling each other through collaborative inquiry: exploring music as a provocation for leadership development.
Ihan Ip , University of San Diego Follow
Date of Award
Degree name.
PhD Leadership Studies
Dissertation Committee
Cheryl Getz, EdD, Chair René Molenkamp, PhD, Member Donna Ladkin, PhD, Member
leadership development, arts-based methods, music, aesthetic knowing, facilitation, holding environment, safety, group process, collaboration, collective leadership, dialectics, paradigm shift, identity, inclusivity, belonging
Amid the challenges in a global village, leadership education needs to surpass traditional methods, nurturing creativity, flexibility, and adaptability. This study is a collaborative action inquiry that considers music as an arts-based method in service of leadership development. The study unfolded over five cycles, in which 14 coinquirers collaborated in a process of exploration. The study illuminates the strong potential of music as a provocation for leadership development and reveals crucial realizations in the area of facilitation in collective processes.
This dissertation tells the story of the inquiry with the voices of its coinquirers and offers insights on facilitation through my reflections as the researcher. It honored the fluidity of the process by gathering data through a combination of questionnaires, workshops, and individual conversations. Akin to the process of birthing or composition, both data collection and analysis occurred iteratively, accessing emergent, musically informed methods of sense-making.
This study found the intersection of music and leadership development emerged as a novel exploration. The findings underscore the importance of cocreating a holding environment, an awareness of the dynamics within collective leadership situations, scaffolding, and the profound value of experiential learning. Further, the findings reveal the necessity of strong mutual trust among group members. Music was shown to catalyze meaningful leadership education along the dimensions of identity, inclusivity, and belonging. The study also demonstrated music facilitates leadership learning at multiple levels—embodiment, awareness, and engagement in emergent processes—underscoring its potential as a transformative tool in leadership development.
Despite successfully designing musical leadership workshops, however, coinquirers experienced concern about taking their learning outside of the study. Implications for future research and practice include investigating the use of music in leadership in organizational contexts, finding ways to support leadership development facilitators to creatively use arts-based methods for their work, applying the use of music in social justice spaces, and developing more applied methods of leadership and facilitation through inquiry to deepen our ways of engaging with each other in our world.
Document Type
Dissertation: Open Access
Leadership Studies
Digital USD Citation
Ip, Ihan, "Ennobling Each Other Through Collaborative Inquiry: Exploring Music as a Provocation for Leadership Development" (2024). Dissertations . 1024. https://digital.sandiego.edu/dissertations/1024
Copyright held by the author
Since May 13, 2024
Included in
Adult and Continuing Education Commons , Curriculum and Instruction Commons , Curriculum and Social Inquiry Commons , Leadership Studies Commons , Organization Development Commons
https://doi.org/10.22371/05.2024.015
Advanced Search
- Notify me via email or RSS
- Collections
- Disciplines
Author Corner
- Submit Dissertation
- Site Policies
- Author Deposit Agreement
Home | About | FAQ | My Account | Accessibility Statement
Privacy Copyright
- Systematic Review
- Open access
- Published: 12 May 2024
Association between problematic social networking use and anxiety symptoms: a systematic review and meta-analysis
- Mingxuan Du 1 ,
- Chengjia Zhao 2 ,
- Haiyan Hu 1 ,
- Ningning Ding 1 ,
- Jiankang He 1 ,
- Wenwen Tian 1 ,
- Wenqian Zhao 1 ,
- Xiujian Lin 1 ,
- Gaoyang Liu 1 ,
- Wendan Chen 1 ,
- ShuangLiu Wang 1 ,
- Pengcheng Wang 3 ,
- Dongwu Xu 1 ,
- Xinhua Shen 4 &
- Guohua Zhang 1
BMC Psychology volume 12 , Article number: 263 ( 2024 ) Cite this article
186 Accesses
Metrics details
A growing number of studies have reported that problematic social networking use (PSNU) is strongly associated with anxiety symptoms. However, due to the presence of multiple anxiety subtypes, existing research findings on the extent of this association vary widely, leading to a lack of consensus. The current meta-analysis aimed to summarize studies exploring the relationship between PSNU levels and anxiety symptoms, including generalized anxiety, social anxiety, attachment anxiety, and fear of missing out. 209 studies with a total of 172 articles were included in the meta-analysis, involving 252,337 participants from 28 countries. The results showed a moderately positive association between PSNU and generalized anxiety (GA), social anxiety (SA), attachment anxiety (AA), and fear of missing out (FoMO) respectively (GA: r = 0.388, 95% CI [0.362, 0.413]; SA: r = 0.437, 95% CI [0.395, 0.478]; AA: r = 0.345, 95% CI [0.286, 0.402]; FoMO: r = 0.496, 95% CI [0.461, 0.529]), and there were different regulatory factors between PSNU and different anxiety subtypes. This study provides the first comprehensive estimate of the association of PSNU with multiple anxiety subtypes, which vary by time of measurement, region, gender, and measurement tool.
Peer Review reports
Introduction
Social network refers to online platforms that allow users to create, share, and exchange information, encompassing text, images, audio, and video [ 1 ]. The use of social network, a term encompassing various activities on these platforms, has been measured from angles such as frequency, duration, intensity, and addictive behavior, all indicative of the extent of social networking usage [ 2 ]. As of April 2023, there are 4.8 billion social network users globally, representing 59.9% of the world’s population [ 3 ]. The usage of social network is considered a normal behavior and a part of everyday life [ 4 , 5 ]. Although social network offers convenience in daily life, excessive use can lead to PSNU [ 6 , 7 ], posing potential threats to mental health, particularly anxiety symptoms (Rasmussen et al., 2020). Empirical research has shown that anxiety symptoms, including generalized anxiety (GA), social anxiety (SA), attachment anxiety (AA), and fear of missing out (FoMO), are closely related to PSNU [ 8 , 9 , 10 , 11 , 12 ]. While some empirical studies have explored the relationship between PSNU and anxiety symptoms, their conclusions are not consistent. Some studies have found a significant positive correlation [ 13 , 14 , 15 ], while others have found no significant correlation [ 16 , 17 , 18 , 19 ]. Furthermore, the degree of correlation varies widely in existing research, with reported r-values ranging from 0.12 to 0.80 [ 20 , 21 ]. Therefore, a systematic meta-analysis is necessary to clarify the impact of PSNU on individual anxiety symptoms.
Previous research lacks a unified concept of PSNU, primarily due to differing theoretical interpretations by various authors, and the use of varied standards and diagnostic tools. Currently, this phenomenon is referred to by several terms, including compulsive social networking use, problematic social networking use, excessive social networking use, social networking dependency, and social networking addiction [ 22 , 23 , 24 , 25 , 26 ]. These conceptual differences hinder the development of a cohesive and systematic research framework, as it remains unclear whether these definitions and tools capture the same underlying construct [ 27 ]. To address this lack of uniformity, this paper will use the term “problematic use” to encompass all the aforementioned nomenclatures (i.e., compulsive, excessive, dependent, and addictive use).
Regarding the relationship between PSNU and anxiety symptoms, two main perspectives exist: the first suggests a positive correlation, while the second proposes a U-shaped relationship. The former perspective, advocating a positive correlation, aligns with the social cognitive theory of mass communication. It posits that PSNU can reinforce certain cognitions, emotions, attitudes, and behaviors [ 28 , 29 ], potentially elevating individuals’ anxiety levels [ 30 ]. Additionally, the cognitive-behavioral model of pathological use, a primary framework for explaining factors related to internet-based addictions, indicates that psychiatric symptoms like depression or anxiety may precede internet addiction, implying that individuals experiencing anxiety may turn to social networking platforms as a coping mechanism [ 31 ]. Empirical research also suggests that highly anxious individuals prefer computer-mediated communication due to the control and social liberation it offers and are more likely to have maladaptive emotional regulation, potentially leading to problematic social network service use [ 32 ]. Turning to the alternate perspective, it proposes a U-shaped relationship as per the digital Goldilocks hypothesis. In this view, moderate social networking usage is considered beneficial for psychosocial adaptation, providing individuals with opportunities for social connection and support. Conversely, both excessive use and abstinence can negatively impact psychosocial adaptation [ 33 ]. In summary, both perspectives offer plausible explanations.
Incorporating findings from previous meta-analyses, we identified seven systematic reviews and two meta-analyses that investigated the association between PSNU and anxiety. The results of these meta-analyses indicated a significant positive correlation between PSNU and anxiety (ranging from 0.33 to 0.38). However, it is evident that these previous meta-analyses had certain limitations. Firstly, they focused only on specific subtypes of anxiety; secondly, they were limited to adolescents and emerging adults in terms of age. In summary, this systematic review aims to ascertain which theoretical perspective more effectively explains the relationship between PSNU and anxiety, addressing the gaps in previous meta-analyses. Additionally, the association between PSNU and anxiety could be moderated by various factors. Drawing from a broad research perspective, any individual study is influenced by researcher-specific designs and associated sample estimates. These may lead to bias compared to the broader population. Considering the selection criteria for moderating variables in empirical studies and meta-analyses [ 34 , 35 ], the heterogeneity of findings on problematic social network usage and anxiety symptoms could be driven by divergence in sample characteristics (e.g., gender, age, region) and research characteristics (measurement instrument of study variables). Since the 2019 coronavirus pandemic, heightened public anxiety may be attributed to the fear of the virus or heightened real life stress. The increased use of electronic devices, particularly smartphones during the pandemic, also instigates the prevalence of problematic social networking. Thus, our analysis focuses on three moderators: sample characteristics (participants’ gender, age, region), measurement tools (for PSNU and anxiety symptoms) and the time of measurement (before COVID-19 vs. during COVID-19).
The present study was conducted in accordance with the 2020 statement on Preferred Reporting Items for Systematic Reviews and Meta-Analyses (PRISMA) [ 36 ]. To facilitate transparency and to avoid unnecessary duplication of research, this study was registered on PROSPERO, and the number is CRD42022350902.
Literature search
Studies on the relationship between the PSNU and anxiety symptoms from 2000 to 2023 were retrieved from seven databases. These databases included China National Knowledge Infrastructure (CNKI), Wanfang Data, Chongqing VIP Information Co. Ltd. (VIP), Web of Science, ScienceDirect, PubMed, and PsycARTICLES. The search strings consisted of (a) anxiety symptoms, (b) social network, and (c) Problematic use. As shown in Table 1 , the keywords for anxiety are as follows: anxiety, generalized anxiety, social anxiety, attachment anxiety, fear of missing out, and FoMO. The keywords for social network are as follows: social network, social media, social networking site, Instagram, and Facebook. The keywords for addiction are as follows: addiction, dependence, problem/problematic use, excessive use. The search deadline was March 19, 2023. A total of 2078 studies were initially retrieved and all were identified ultimately.
Inclusion and exclusion criteria
Retrieved studies were eligible for the present meta-analysis if they met the following inclusion criteria: (a) the study provided Pearson correlation coefficients used to measure the relationship between PSNU and anxiety symptoms; (b) the study reported the sample size and the measurement instruments for the variables; (c) the study was written in English and Chinese; (d) the study provided sufficient statistics to calculate the effect sizes; (e) effect sizes were extracted from independent samples. If multiple independent samples were investigated in the same study, they were coded separately; if the study was a longitudinal study, they were coded by the first measurement. In addition, studies were excluded if they: (a) examined non-problematic social network use; (b) had an abnormal sample population; (c) the results of the same sample were included in another study and (d) were case reports or review articles. Two evaluators with master’s degrees independently assessed the eligibility of the articles. A third evaluator with a PhD examined the results and resolved dissenting views.
Data extraction and quality assessment
Two evaluators independently coded the selected articles according to the following characteristics: literature information, time of measurement (before the COVID-19 vs. during the COVID-19), sample source (developed country vs. developing country), sample size, proportion of males, mean age, type of anxiety, and measurement instruments for PSNU and anxiety symptoms. The following principles needed to be adhered to in the coding process: (a) effect sizes were extracted from independent samples. If multiple independent samples were investigated in the same study, they were coded separately; if the study was a longitudinal study, it was coded by the first measurement; (b) if multiple studies used the same data, the one with the most complete information was selected; (c) If studies reported t or F values rather than r , the following formula \( r=\sqrt{\frac{{t}^{2}}{{t}^{2}+df}}\) ; \( r=\sqrt{\frac{F}{F+d{f}_{e}}}\) was used to convert them into r values [ 37 , 38 ]. Additionally, if some studies only reported the correlation matrix between each dimension of PSNU and anxiety symptoms, the following formula \( {r}_{xy}=\frac{\sum {r}_{xi}{r}_{yj}}{\sqrt{n+n(n-1){r}_{xixj}}\sqrt{m+m(m-1){r}_{yiyj}}}\) was used to synthesize the r values [ 39 ], where n or m is the number of dimensions of variable x or variable y, respectively, and \( {r}_{xixj} \) or \( {r}_{yiyj}\) represents the mean of the correlation coefficients between the dimensions of variable x or variable y, respectively.
Literature quality was determined according to the meta-analysis quality evaluation scale developed [ 40 ]. The quality of the post-screening studies was assessed by five dimensions: sampling method, efficiency of sample collection, level of publication, and reliability of PSNU and anxiety symptom measurement instruments. The total score of the scale ranged from 0 to 10; higher scores indicated better quality of the literature.
Data analysis
All data were performed using Comprehensive Meta Analysis 3.3 (CMA 3.3). Pearson’s product-moment coefficient r was selected as the effect size index in this meta-analysis. Firstly, \( {\text{F}\text{i}\text{s}\text{h}\text{e}\text{r}}^{{\prime }}\text{s} Z=\frac{1}{2}\times \text{ln}\left(\frac{1+r}{1-r}\right)\) was used to convert the correlation coefficient to Fisher Z . Then the formula \( SE=\sqrt{\frac{1}{n-3}}\) was used to calculate the standard error ( SE ). Finally, the summary of r was obtained from the formula \( r=\frac{{e}^{2z}-1}{{e}^{2z}+1}\) for a comprehensive measure of the relationship between PSNU and anxiety symptoms [ 37 , 41 ].
Although the effect sizes estimated by the included studies may be similar, considering the actual differences between studies (e.g., region and gender), the random effects model was a better choice for data analysis for the current meta-analysis. The heterogeneity of the included study effect sizes was measured for significance by Cochran’s Q test and estimated quantitatively by the I 2 statistic [ 42 ]. If the results indicate there is a significant heterogeneity (the Q test: p -value < 0.05, I 2 > 75) and the results of different studies are significantly different from the overall effect size. Conversely, it indicates there are no differences between the studies and the overall effect size. And significant heterogeneity tends to indicate the possible presence of potential moderating variables. Subgroup analysis and meta-regression analysis were used to examine the moderating effect of categorical and continuous variables, respectively.
Funnel plots, fail-safe number (Nfs) and Egger linear regression were utilized to evaluate the publication bias [ 43 , 44 , 45 ]. The likelihood of publication bias was considered low if the intercept obtained from Egger linear regression was not significant. A larger Nfs indicated a lower risk of publication bias, and if Nfs < 5k + 10 (k representing the original number of studies), publication bias should be a concern [ 46 ]. When Egger’s linear regression was significant, the Duval and Tweedie’s trim-and-fill was performed to correct the effect size. If there was no significant change in the effect size, it was assumed that there was no serious publication bias [ 47 ].
A significance level of P < 0.05 was deemed applicable in this study.
Sample characteristics
The PRISMA search process is depicted in Fig. 1 . The database search yielded 2078 records. After removing duplicate records and screening the title and abstract, the full text was subject to further evaluation. Ultimately, 172 records fit the inclusion criteria, including 209 independent effect sizes. The present meta-analysis included 68 studies on generalized anxiety, 44 on social anxiety, 22 on attachment anxiety, and 75 on fear of missing out. The characteristics of the selected studies are summarized in Table 2 . The majority of the sample group were adults. Quality scores for selected studies ranged from 0 to 10, with only 34 effect sizes below the theoretical mean, indicating high quality for the included studies. The literature included utilized BSMAS as the primary tool to measure PSNU, DASS-21-A to measure GA, IAS to measure SA, ECR to measure AA, and FoMOS to measure FoMO.
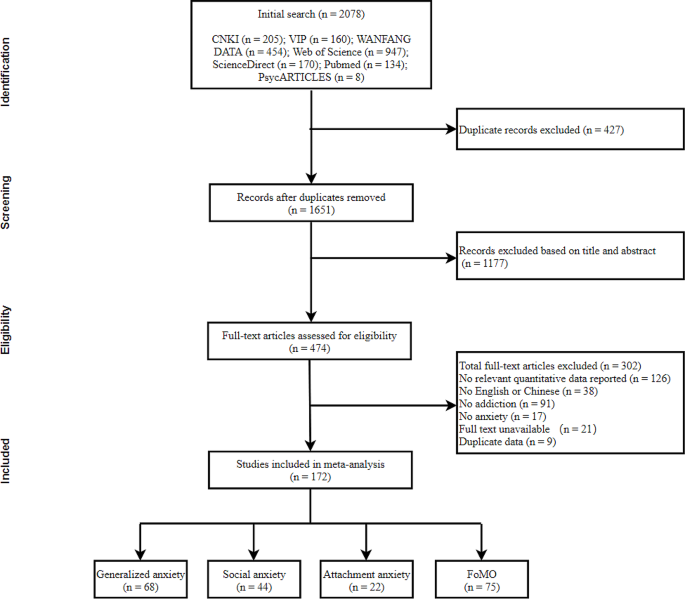
Flow chart of the search and selection strategy
Overall analysis, homogeneity tests and publication bias
As shown in Table 3 , there was significant heterogeneity between PSNU and all four anxiety symptoms (GA: Q = 1623.090, I 2 = 95.872%; SA: Q = 1396.828, I 2 = 96.922%; AA: Q = 264.899, I 2 = 92.072%; FoMO: Q = 1847.110, I 2 = 95.994%), so a random effects model was chosen. The results of the random effects model indicate a moderate positive correlation between PSNU and anxiety symptoms (GA: r = 0.350, 95% CI [0.323, 0.378]; SA: r = 0.390, 95% CI [0.347, 0.431]; AA: r = 0.345, 95% CI [0.286, 0.402]; FoMO: r = 0.496, 95% CI [0.461, 0.529]).
Figure 2 shows the funnel plot of the relationship between PSNU and anxiety symptoms. No significant symmetry was seen in the funnel plot of the relationship between PSNU and GA and between PSNU and SA. And the Egger’s regression results also indicated that there might be publication bias ( t = 3.775, p < 0.001; t = 2.309, p < 0.05). Therefore, it was necessary to use fail-safe number (Nfs) and the trim and fill method for further examination and correction. The Nfs for PSNU and GA as well as PSNU and SA are 4591 and 7568, respectively. Both Nfs were much larger than the standard 5 k + 10. After performing the trim and fill method, 14 effect sizes were added to the right side of the funnel plat (Fig. 2 .a), the correlation coefficient between PSNU and GA changed to ( r = 0.388, 95% CI [0.362, 0.413]); 10 effect sizes were added to the right side of the funnel plat (Fig. 2 .b), the correlation coefficient between PSNU and SA changed to ( r = 0.437, 95% CI [0.395, 0.478]). The correlation coefficients did not change significantly, indicating that there was no significant publication bias associated with the relationship between PSNU and these two anxiety symptoms (GA and SA).
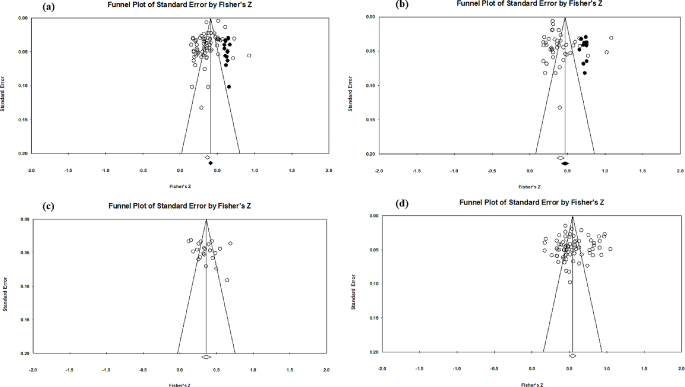
Funnel plot of the relationship between PSNU and anxiety symptoms. Note: Black dots indicated additional studies after using trim and fill method; ( a ) = Funnel plot of the PSNU and GA; ( b ) = Funnel plot of the PSNU and SA; ( c ) = Funnel plot of the PSNU and AA; ( d ) = Funnel plot of the PSNU and FoMO
Sensitivity analyses
Initially, the findings obtained through the one-study-removed approach indicated that the heterogeneities in the relationship between PSNU and anxiety symptoms were not attributed to any individual study. Nevertheless, it is important to note that sensitivity analysis should be performed based on literature quality [ 223 ] since low-quality literature could potentially impact result stability. In the relationship between PSNU and GA, the 10 effect sizes below the theoretical mean scores were excluded from analysis, and the sensitivity analysis results were recalculated ( r = 0.402, 95% CI [0.375, 0.428]); In the relationship between PSNU and SA, the 8 effect sizes below the theoretical mean scores were excluded from analysis, and the sensitivity analysis results were recalculated ( r = 0.431, 95% CI [0.387, 0.472]); In the relationship between PSNU and AA, the 5 effect sizes below the theoretical mean scores were excluded from analysis, and the sensitivity analysis results were recalculated ( r = 0.367, 95% CI [0.298, 0.433]); In the relationship between PSNU and FoMO, the 11 effect sizes below the theoretical mean scores were excluded from analysis, and the sensitivity analysis results were recalculated ( r = 0.508, 95% CI [0.470, 0.544]). The revised estimates indicate that meta-analysis results were stable.
Moderator analysis
The impact of moderator variables on the relation between psnu and ga.
The results of subgroup analysis and meta-regression are shown in Table 4 , the time of measurement significantly moderated the correlation between PSNU and GA ( Q between = 19.268, df = 2, p < 0.001). The relation between the two variables was significantly higher during the COVID-19 ( r = 0.392, 95% CI [0.357, 0.425]) than before the COVID-19 ( r = 0.270, 95% CI [0.227, 0.313]) or measurement time uncertain ( r = 0.352, 95% CI [0.285, 0.415]).
The moderating effect of the PSNU measurement was significant ( Q between = 6.852, df = 1, p = 0.009). The relation was significantly higher when PSNU was measured with the BSMAS ( r = 0.373, 95% CI [0.341, 0.404]) compared to others ( r = 0.301, 95% CI [0.256, 0.344]).
The moderating effect of the GA measurement was significant ( Q between = 60.061, df = 5, p < 0.001). Specifically, when GA measured by the GAD ( r = 0.398, 95% CI [0.356, 0.438]) and the DASS-21-A ( r = 0.433, 95% CI [0.389, 0.475]), a moderate positive correlation was observed. However, the correlation was less significant when measured using the STAI ( r = 0.232, 95% CI [0.187, 0.276]).
For the relation between PSNU and GA, the moderating effect of region, gender and age were not significant.
The impact of moderator variables on the relation between PSNU and SA
The effects of the moderating variables in the relation between PSNU and SA were shown in Table 5 . The results revealed a gender-moderated variances between the two variables (b = 0.601, 95% CI [ 0.041, 1.161], Q model (1, k = 41) = 4.705, p = 0.036).
For the relation between PSNU and SA, the moderating effects of time of measurement, region, measurement of PSNU and SA, and age were not significant.

The impact of moderator variables on the relation between PSNU and AA
The effects of the moderating variables in the relation between PSNU and AA were shown in Table 6 , region significantly moderated the correlation between PSNU and AA ( Q between = 6.410, df = 2, p = 0.041). The correlation between the two variables was significantly higher in developing country ( r = 0.378, 95% CI [0.304, 0.448]) than in developed country ( r = 0.242, 95% CI [0.162, 0.319]).
The moderating effect of the PSNU measurement was significant ( Q between = 6.852, df = 1, p = 0.009). Specifically, when AA was measured by the GPIUS-2 ( r = 0.484, 95% CI [0.200, 0.692]) and the PMSMUAQ ( r = 0.443, 95% CI [0.381, 0.501]), a moderate positive correlation was observed. However, the correlation was less significant when measured using the BSMAS ( r = 0.248, 95% CI [0.161, 0.331]) and others ( r = 0.313, 95% CI [0.250, 0.372]).
The moderating effect of the AA measurement was significant ( Q between = 17.283, df = 2, p < 0.001). The correlation was significantly higher when measured using the ECR ( r = 0.386, 95% CI [0.338, 0.432]) compared to the RQ ( r = 0.200, 95% CI [0.123, 0.275]).
For the relation between PSNU and AA, the moderating effects of time of measurement, region, gender, and age were not significant.
The impact of moderator variables on the relation between PSNU and FoMO
The effects of the moderating variables in the relation between PSNU and FoMO were shown in Table 7 , the moderating effect of the PSNU measurement was significant ( Q between = 8.170, df = 2, p = 0.017). Among the sub-dimensions, the others was excluded because there was only one sample. Specifically, when measured using the FoMOS-MSME ( r = 0.630, 95% CI [0.513, 0.725]), a moderate positive correlation was observed. However, the correlation was less significant when measured using the FoMOS ( r = 0.472, 95% CI [0.432, 0.509]) and the T-S FoMOS ( r = 0.557, 95% CI [0.463, 0.639]).
For the relationship between PSNU and FoMO, the moderating effects of time of measurement, region, measurement of PSNU, gender and age were not significant.
Through systematic review and meta-analysis, this study established a positive correlation between PSNU and anxiety symptoms (i.e., generalized anxiety, social anxiety, attachment anxiety, and fear of missing out), confirming a linear relationship and partially supporting the Social Cognitive Theory of Mass Communication [ 28 ] and the Cognitive Behavioral Model of Pathological Use [ 31 ]. Specifically, a significant positive correlation between PSNU and GA was observed, implying that GA sufferers might resort to social network for validation or as an escape from reality, potentially alleviating their anxiety. Similarly, the meta-analysis demonstrated a strong positive correlation between PSNU and SA, suggesting a preference for computer-mediated communication among those with high social anxiety due to perceived control and liberation offered by social network. This preference is often accompanied by maladaptive emotional regulation, predisposing them to problematic use. In AA, a robust positive correlation was found with PSNU, indicating a higher propensity for such use among individuals with attachment anxiety. Notably, the study identified the strongest correlation in the context of FoMO. FoMO’s significant association with PSNU is multifaceted, stemming from the real-time nature of social networks that engenders a continuous concern about missing crucial updates or events. This drives frequent engagement with social network, thereby establishing a direct link to problematic usage patterns. Additionally, social network’s feedback loops amplify this effect, intensifying FoMO. The culture of social comparison on these platforms further exacerbates FoMO, as users frequently compare their lives with others’ selectively curated portrayals, enhancing both their social networking usage frequency and the pursuit for social validation. Furthermore, the integral role of social network in modern life broadens FoMO’s scope, encompassing anxieties about staying informed and connected.
The notable correlation between FoMO and PSNU can be comprehensively understood through various perspectives. FoMO is inherently linked to the real-time nature of social networks, which cultivates an ongoing concern about missing significant updates or events in one’s social circle [ 221 ]. This anxiety prompts frequent engagement with social network, leading to patterns of problematic use. Moreover, the feedback loops in social network algorithms, designed to enhance user engagement, further intensify this fear [ 224 ]. Additionally, social comparison, a common phenomenon on these platforms, exacerbates FoMO as users continuously compare their lives with the idealized representations of others, amplifying feelings of missing out on key social experiences [ 225 ]. This behavior not only increases social networking usage but also is closely linked to the quest for social validation and identity construction on these platforms. The extensive role of social network in modern life further amplifies FoMO, as these platforms are crucial for information exchange and maintaining social ties. FoMO thus encompasses more than social concerns, extending to anxieties about staying informed with trends and dynamics within social networks [ 226 ]. The multifaceted nature of FoMO in relation to social network underscores its pronounced correlation with problematic social networking usage. In essence, the combination of social network’s intrinsic characteristics, psychological drivers of user behavior, the culture of social comparison, and the pervasiveness of social network in everyday life collectively make FoMO the most pronouncedly correlated anxiety type with PSNU.
Additionally, we conducted subgroup analyses on the timing of measurement (before COVID-19 vs. during COVID-19), measurement tools (for PSNU and anxiety symptoms), sample characteristics (participants’ region), and performed a meta-regression analysis on gender and age in the context of PSNU and anxiety symptoms. It was found that the timing of measurement, tools used for assessing PSNU and anxiety, region, and gender had a moderating effect, whereas age did not show a significant moderating impact.
Firstly, the relationship between PSNU and anxiety symptoms was significantly higher during the COVID-19 period than before, especially between PSNU and GA. However, the moderating effect of measurement timing was not significant in the relationship between PSNU and other types of anxiety. This could be attributed to the increased uncertainty and stress during the pandemic, leading to heightened levels of general anxiety [ 227 ]. The overuse of social network for information seeking and anxiety alleviation might have paradoxically exacerbated anxiety symptoms, particularly among individuals with broad future-related worries [ 228 ]. While the COVID-19 pandemic altered the relationship between PSNU and GA, its impact on other types of anxiety (such as SA and AA) may not have been significant, likely due to these anxiety types being more influenced by other factors like social skills and attachment styles, which were minimally impacted by the epidemic.
Secondly, the observed variance in the relationship between PSNU and AA across different economic contexts, notably between developing and developed countries, underscores the multifaceted influence of socio-economic, cultural, and technological factors on this dynamic. The amplified connection in developing countries may be attributed to greater socio-economic challenges, distinct cultural norms regarding social support and interaction, rising social network penetration, especially among younger demographics, and technological disparities influencing accessibility and user experience [ 229 , 230 ]. Moreover, the role of social network as a coping mechanism for emotional distress, potentially fostering insecure attachment patterns, is more pronounced in these settings [ 231 ]. These findings highlight the necessity of considering contextual variations in assessing the psychological impacts of social network, advocating for a nuanced understanding of how socio-economic and cultural backgrounds mediate the relationship between PSNU and mental health outcomes [ 232 ]. Additionally, the relationship between PSNU and other types of anxiety (such as GA and SA) presents uniform characteristics across different economic contexts.
Thirdly, the significant moderating effects of measurement tools in the context of PSNU and its correlation with various forms of anxiety, including GA, and AA, are crucial in interpreting the research findings. Specifically, the study reveals that the Bergen Social Media Addiction Scale (BSMAS) demonstrates a stronger correlation between PSNU and GA, compared to other tools. Similarly, for AA, the Griffiths’ Problematic Internet Use Scale 2 (GPIUS2) and the Problematic Media Social Media Use Assessment Questionnaire (PMSMUAQ) show a more pronounced correlation with AA than the BSMAS or other instruments, but for SA and FoMO, the PSNU instrument doesn’t significantly moderate the correlation. The PSNU measurement tool typically contains an emotional change dimension. SA and FoMO, due to their specific conditional stimuli triggers and correlation with social networks [ 233 , 234 ], are likely to yield more consistent scores in this dimension, while GA and AA may be less reliable due to their lesser sensitivity to specific conditional stimuli. Consequently, the adjustment effects of PSNU measurements vary across anxiety symptoms. Regarding the measurement tools for anxiety, different scales exhibit varying degrees of sensitivity in detecting the relationship with PSNU. The Generalized Anxiety Disorder Scale (GAD) and the Depression Anxiety Stress Scales 21 (DASS-21) are more effective in illustrating a strong relationship between GA and PSNU than the State-Trait Anxiety Inventory (STAI). In the case of AA, the Experiences in Close Relationships-21 (ECR-21) provides a more substantial correlation than the Relationship Questionnaire (RQ). Furthermore, for FoMO, the Fear of Missing Out Scale - Multi-Social Media Environment (FoMOS-MSME) is more indicative of a strong relationship with PSNU compared to the standard FoMOS or the T-S FoMOS. These findings underscore the importance of the selection of appropriate measurement tools in research. Different tools, due to their unique design, focus, and sensitivity, can reveal varying degrees of correlation between PSNU and anxiety disorders. This highlights the need for careful consideration of tool characteristics and their potential impact on research outcomes. It also cautions against drawing direct comparisons between studies without acknowledging the possible variances introduced by the use of different measurement instruments.
Fourthly, the significant moderating role of gender in the relationship between PSNU and SA, particularly pronounced in samples with a higher proportion of females. Women tend to engage more actively and emotionally with social network, potentially leading to an increased dependency on these platforms when confronting social anxiety [ 235 ]. This intensified use might amplify the association between PSNU and SA. Societal and cultural pressures, especially those related to appearance and social status, are known to disproportionately affect women, possibly exacerbating their experience of social anxiety and prompting a greater reliance on social network for validation and support [ 236 ]. Furthermore, women’s propensity to seek emotional support and express themselves on social network platforms [ 237 ] could strengthen this link, particularly in the context of managing social anxiety. Consequently, the observed gender differences in the relationship between PSNU and SA underscore the importance of considering gender-specific dynamics and cultural influences in psychological research related to social network use. In addition, gender consistency was observed in the association between PSNU and other types of anxiety, indicating no significant gender disparities.
Fifthly, the absence of a significant moderating effect of age on the relationship between PSNU and various forms of anxiety suggests a pervasive influence of social network across different age groups. This finding indicates that the impact of PSNU on anxiety is relatively consistent, irrespective of age, highlighting the universal nature of social network’s psychological implications [ 238 ]. Furthermore, this uniformity suggests that other factors, such as individual psychological traits or socio-cultural influences, might play a more crucial role in the development of anxiety related to social networking usage than age [ 239 ]. The non-significant role of age also points towards a potential generational overlap in social networking usage patterns and their psychological effects, challenging the notion that younger individuals are uniquely susceptible to the adverse effects of social network on mental health [ 240 ]. Therefore, this insight necessitates a broader perspective in understanding the dynamics of social network and mental health, one that transcends age-based assumptions.
Limitations
There are some limitations in this research. First, most of the studies were cross-sectional surveys, resulting in difficulties in inferring causality of variables, longitudinal study data will be needed to evaluate causal interactions in the future. Second, considerable heterogeneity was found in the estimated results, although heterogeneity can be partially explained by differences in study design (e.g., Time of measurement, region, gender, and measurement tools), but this can introduce some uncertainty in the aggregation and generalization of the estimated results. Third, most studies were based on Asian samples, which limits the generality of the results. Fourth, to minimize potential sources of heterogeneity, some less frequently used measurement tools were not included in the classification of measurement tools, which may have some impact on the results of heterogeneity interpretation. Finally, since most of the included studies used self-reported scales, it is possible to get results that deviate from the actual situation to some extent.
This meta-analysis aims to quantifies the correlations between PSNU and four specific types of anxiety symptoms (i.e., generalized anxiety, social anxiety, attachment anxiety, and fear of missing out). The results revealed a significant moderate positive association between PSNU and each of these anxiety symptoms. Furthermore, Subgroup analysis and meta-regression analysis indicated that gender, region, time of measurement, and instrument of measurement significantly influenced the relationship between PSNU and specific anxiety symptoms. Specifically, the measurement time and GA measurement tools significantly influenced the relationship between PSNU and GA. Gender significantly influenced the relationship between PSNU and SA. Region, PSNU measurement tools, and AA measurement tools all significantly influenced the relationship between PSNU and AA. The FoMO measurement tool significantly influenced the relationship between PSNU and FoMO. Regarding these findings, prevention interventions for PSNU and anxiety symptoms are important.
Data availability
The datasets are available from the corresponding author on reasonable request.
Abbreviations
- Problematic social networking use
- Generalized anxiety
- Social anxiety
- Attachment anxiety
Fear of miss out
Bergen Social Media Addiction Scale
Facebook Addiction Scale
Facebook Intrusion Questionnaire
Generalized Problematic Internet Use Scale 2
Problematic Mobile Social Media Usage Assessment Questionnaire
Social Network Addiction Tendency Scale
Brief Symptom Inventory
The anxiety subscale of the Depression Anxiety Stress Scales
Generalized Anxiety Disorder
The anxiety subscale of the Hospital Anxiety and Depression Scale
State-Trait Anxiety Inventory
Interaction Anxiousness Scale
Liebowitz Social Anxiety Scale
Social Anxiety Scale for Social Media Users
Social Anxiety for Adolescents
Social Anxiety Subscale of the Self-Consciousness Scale
Social Interaction Anxiety Scale
Experiences in Close Relationship Scale
Relationship questionnaire
Fear of Missing Out Scale
FoMO Measurement Scale in the Mobile Social Media Environment
Trait-State Fear of missing Out Scale
Rozgonjuk D, Sindermann C, Elhai JD, Montag C. Fear of missing out (FoMO) and social media’s impact on daily-life and productivity at work: do WhatsApp, Facebook, Instagram, and Snapchat Use disorders mediate that association? Addict Behav. 2020;110:106487.
Article PubMed Google Scholar
Mieczkowski H, Lee AY, Hancock JT. Priming effects of social media use scales on well-being outcomes: the influence of intensity and addiction scales on self-reported depression. Social Media + Soc. 2020;6(4):2056305120961784.
Article Google Scholar
Global digital population as of April. 2023 [ https://www.statista.com/statistics/617136/digital-population-worldwide/ ].
Marengo D, Settanni M, Fabris MA, Longobardi C. Alone, together: fear of missing out mediates the link between peer exclusion in WhatsApp classmate groups and psychological adjustment in early-adolescent teens. J Social Personal Relationships. 2021;38(4):1371–9.
Marengo D, Fabris MA, Longobardi C, Settanni M. Smartphone and social media use contributed to individual tendencies towards social media addiction in Italian adolescents during the COVID-19 pandemic. Addict Behav. 2022;126:107204.
Müller SM, Wegmann E, Stolze D, Brand M. Maximizing social outcomes? Social zapping and fear of missing out mediate the effects of maximization and procrastination on problematic social networks use. Comput Hum Behav. 2020;107:106296.
Sun Y, Zhang Y. A review of theories and models applied in studies of social media addiction and implications for future research. Addict Behav. 2021;114:106699.
Boustead R, Flack M. Moderated-mediation analysis of problematic social networking use: the role of anxious attachment orientation, fear of missing out and satisfaction with life. Addict Behav 2021, 119.
Hussain Z, Griffiths MD. The associations between problematic social networking Site Use and Sleep Quality, attention-deficit hyperactivity disorder, Depression, anxiety and stress. Int J Mental Health Addict. 2021;19(3):686–700.
Gori A, Topino E, Griffiths MD. The associations between attachment, self-esteem, fear of missing out, daily time expenditure, and problematic social media use: a path analysis model. Addict Behav. 2023;141:107633.
Marino C, Manari T, Vieno A, Imperato C, Spada MM, Franceschini C, Musetti A. Problematic social networking sites use and online social anxiety: the role of attachment, emotion dysregulation and motives. Addict Behav. 2023;138:107572.
Tobin SJ, Graham S. Feedback sensitivity as a mediator of the relationship between attachment anxiety and problematic Facebook Use. Cyberpsychology Behav Social Netw. 2020;23(8):562–6.
Brailovskaia J, Rohmann E, Bierhoff H-W, Margraf J. The anxious addictive narcissist: the relationship between grandiose and vulnerable narcissism, anxiety symptoms and Facebook Addiction. PLoS ONE 2020, 15(11).
Kim S-S, Bae S-M. Social Anxiety and Social Networking Service Addiction Proneness in University students: the Mediating effects of Experiential Avoidance and interpersonal problems. Psychiatry Invest. 2022;19(8):702–702.
Zhao J, Ye B, Yu L, Xia F. Effects of Stressors of COVID-19 on Chinese College Students’ Problematic Social Media Use: A Mediated Moderation Model. Front Psychiatry 2022, 13.
Astolfi Cury GS, Takannune DM, Prates Herrerias GS, Rivera-Sequeiros A, de Barros JR, Baima JP, Saad-Hossne R, Sassaki LY. Clinical and Psychological Factors Associated with Addiction and Compensatory Use of Facebook among patients with inflammatory bowel disease: a cross-sectional study. Int J Gen Med. 2022;15:1447–57.
Balta S, Emirtekin E, Kircaburun K, Griffiths MD. Neuroticism, trait fear of missing out, and Phubbing: the mediating role of state fear of missing out and problematic Instagram Use. Int J Mental Health Addict. 2020;18(3):628–39.
Boursier V, Gioia F, Griffiths MD. Do selfie-expectancies and social appearance anxiety predict adolescents’ problematic social media use? Comput Hum Behav. 2020;110:106395.
Worsley JD, McIntyre JC, Bentall RP, Corcoran R. Childhood maltreatment and problematic social media use: the role of attachment and depression. Psychiatry Res. 2018;267:88–93.
de Bérail P, Guillon M, Bungener C. The relations between YouTube addiction, social anxiety and parasocial relationships with YouTubers: a moderated-mediation model based on a cognitive-behavioral framework. Comput Hum Behav. 2019;99:190–204.
Naidu S, Chand A, Pandaram A, Patel A. Problematic internet and social network site use in young adults: the role of emotional intelligence and fear of negative evaluation. Pers Indiv Differ. 2023;200:111915.
Apaolaza V, Hartmann P, D’Souza C, Gilsanz A. Mindfulness, compulsive Mobile Social Media Use, and derived stress: the mediating roles of self-esteem and social anxiety. Cyberpsychology Behav Social Netw. 2019;22(6):388–96.
Demircioglu ZI, Goncu-Kose A. Antecedents of problematic social media use and cyberbullying among adolescents: attachment, the dark triad and rejection sensitivity. Curr Psychol (New Brunsw NJ) 2022:1–19.
Gao Q, Li Y, Zhu Z, Fu E, Bu X, Peng S, Xiang Y. What links to psychological needs satisfaction and excessive WeChat use? The mediating role of anxiety, depression and WeChat use intensity. BMC Psychol. 2021;9(1):105–105.
Article PubMed PubMed Central Google Scholar
Malak MZ, Shuhaiber AH, Al-amer RM, Abuadas MH, Aburoomi RJ. Correlation between psychological factors, academic performance and social media addiction: model-based testing. Behav Inform Technol. 2022;41(8):1583–95.
Song C. The effect of the need to belong on mobile phone social media dependence of middle school students: Chain mediating roles of fear of missing out and maladaptive cognition. Sichuan Normal University; 2022.
Tokunaga RS, Rains SA. A review and meta-analysis examining conceptual and operational definitions of problematic internet use. Hum Commun Res. 2016;42(2):165–99.
Bandura A. Social cognitive theory of mass communication. Media effects. edn.: Routledge; 2009. pp. 110–40.
Valkenburg PM, Peter J, Walther JB. Media effects: theory and research. Ann Rev Psychol. 2016;67:315–38.
Slater MD. Reinforcing spirals: the mutual influence of media selectivity and media effects and their impact on individual behavior and social identity. Communication Theory. 2007;17(3):281–303.
Ahmed E, Vaghefi I. Social media addiction: A systematic review through cognitive-behavior model of pathological use. 2021.
She R, han Mo PK, Li J, Liu X, Jiang H, Chen Y, Ma L, fai Lau JT. The double-edged sword effect of social networking use intensity on problematic social networking use among college students: the role of social skills and social anxiety. Comput Hum Behav. 2023;140:107555.
Przybylski AK, Weinstein N. A large-scale test of the goldilocks hypothesis: quantifying the relations between digital-screen use and the mental well-being of adolescents. Psychol Sci. 2017;28(2):204–15.
Ran G, Li J, Zhang Q, Niu X. The association between social anxiety and mobile phone addiction: a three-level meta-analysis. Comput Hum Behav. 2022;130:107198.
Fioravanti G, Casale S, Benucci SB, Prostamo A, Falone A, Ricca V, Rotella F. Fear of missing out and social networking sites use and abuse: a meta-analysis. Comput Hum Behav. 2021;122:106839.
Moher D, Liberati A, Tetzlaff J, Altman DG, Group* P. Preferred reporting items for systematic reviews and meta-analyses: the PRISMA statement. Ann Intern Med. 2009;151(4):264–9.
Card NA. Applied meta-analysis for social science research. Guilford; 2015.
Peterson RA, Brown SP. On the use of beta coefficients in meta-analysis. J Appl Psychol. 2005;90(1):175.
Hunter JE, Schmidt FL. Methods of meta-analysis: correcting error and bias in research findings. Sage; 2004.
Zhang Y, Li S, Yu G. The relationship between self-esteem and social anxiety: a meta-analysis with Chinese students. Adv Psychol Sci. 2019;27(6):1005–18.
Borenstein M, Hedges LV, Higgins JP, Rothstein HR. Introduction to meta-analysis. Wiley; 2021.
Higgins JP, Thompson SG. Quantifying heterogeneity in a meta-analysis. Stat Med. 2002;21(11):1539–58.
Egger M, Smith GD, Schneider M, Minder C. Bias in meta-analysis detected by a simple, graphical test. BMJ. 1997;315(7109):629–34.
Light RJ, Pillemer DB. Summing up: the science of reviewing research. Harvard University Press; 1984.
Rosenthal R. Meta-Analytic Procedures for Social Science Research Sage Publications: Beverly Hills, 1984, 148 pp. Educational Researcher 1986;15(8):18–20.
Rothstein HR, Sutton AJ, Borenstein M. Publication bias in meta-analysis. Publication bias meta‐analysis: Prev Assess Adjustments 2005:1–7.
Duval S, Tweedie R. Trim and fill: a simple funnel-plot–based method of testing and adjusting for publication bias in meta‐analysis. Biometrics. 2000;56(2):455–63.
Al-Mamun F, Hosen I, Griffiths MD, Mamun MA. Facebook use and its predictive factors among students: evidence from a lower- and middle-income country, Bangladesh. Front Psychiatry 2022, 13.
Schou Andreassen C, Billieux J, Griffiths MD, Kuss DJ, Demetrovics Z, Mazzoni E, Pallesen S. The relationship between addictive use of social media and video games and symptoms of psychiatric disorders: a large-scale cross-sectional study. Psychol Addict Behaviors: J Soc Psychologists Addict Behav. 2016;30(2):252–62.
Arikan G, Acar IH, Ustundag-Budak AM. A two-generation study: The transmission of attachment and young adults’ depression, anxiety, and social media addiction. Addict Behav 2022, 124.
Arpaci I, Karatas K, Kiran F, Kusci I, Topcu A. Mediating role of positivity in the relationship between state anxiety and problematic social media use during the COVID-19 pandemic. Death Stud. 2022;46(10):2287–97.
Brailovskaia J, Margraf J. Facebook Addiction Disorder (FAD) among German students-A longitudinal approach. PLoS ONE 2017, 12(12).
Brailovskaia J, Margraf J. The relationship between burden caused by coronavirus (Covid-19), addictive social media use, sense of control and anxiety. Comput Hum Behav. 2021;119:106720–106720.
Brailovskaia J, Margraf J. Addictive social media use during Covid-19 outbreak: validation of the Bergen Social Media Addiction Scale (BSMAS) and investigation of protective factors in nine countries. Curr Psychol (New Brunsw NJ) 2022:1–19.
Brailovskaia J, Krasavtseva Y, Kochetkov Y, Tour P, Margraf J. Social media use, mental health, and suicide-related outcomes in Russian women: a cross-sectional comparison between two age groups. Women’s Health (London England). 2022;18:17455057221141292–17455057221141292.
PubMed Google Scholar
Chang C-W, Huang R-Y, Strong C, Lin Y-C, Tsai M-C, Chen IH, Lin C-Y, Pakpour AHH, Griffiths MDD. Reciprocal relationships between Problematic Social Media Use, problematic gaming, and psychological distress among University students: a 9-Month Longitudinal Study. Front Public Health 2022, 10.
Charzynska E, Sussman S, Atroszko PA. Profiles of potential behavioral addictions’ severity and their associations with gender, personality, and well-being: A person-centered approach. Addict Behav 2021, 119.
Chen C-Y, Chen IH, Pakpour AH, Lin C-Y, Griffiths MD. Internet-related behaviors and psychological distress among Schoolchildren during the COVID-19 School Hiatus. Cyberpsychology Behav Social Netw. 2021;24(10):654–63.
Da Veiga GF, Sotero L, Pontes HM, Cunha D, Portugal A, Relvas AP. Emerging adults and Facebook Use: the validation of the Bergen Facebook Addiction Scale (BFAS). Int J Mental Health Addict. 2019;17(2):279–94.
Dadiotis A, Bacopoulou F, Kokka I, Vlachakis D, Chrousos GP, Darviri C, Roussos P. Validation of the Greek version of the Bergen Social Media Addiction Scale in Undergraduate Students. EMBnetjournal 2021, 26.
Fekih-Romdhane F, Jahrami H, Away R, Trabelsi K, Pandi-Perumal SR, Seeman MV, Hallit S, Cheour M. The relationship between technology addictions and schizotypal traits: mediating roles of depression, anxiety, and stress. BMC Psychiatry 2023, 23(1).
Flynn S, Noone C, Sarma KM. An exploration of the link between adult attachment and problematic Facebook use. BMC Psychol. 2018;6(1):34–34.
Fung XCC, Siu AMH, Potenza MN, O’Brien KS, Latner JD, Chen C-Y, Chen IH, Lin C-Y. Problematic use of internet-related activities and Perceived Weight Stigma in Schoolchildren: a longitudinal study across different epidemic periods of COVID-19 in China. Front Psychiatry 2021, 12.
Gonzalez-Nuevo C, Cuesta M, Muniz J, Postigo A, Menendez-Aller A, Kuss DJ. Problematic Use of Social Networks during the First Lockdown: User Profiles and the Protective Effect of Resilience and Optimism. Journal of Clinical Medicine 2022, 11(24).
Hou X-L, Wang H-Z, Hu T-Q, Gentile DA, Gaskin J, Wang J-L. The relationship between perceived stress and problematic social networking site use among Chinese college students. J Behav Addictions. 2019;8(2):306–17.
Hussain Z, Wegmann E. Problematic social networking site use and associations with anxiety, attention deficit hyperactivity disorder, and resilience. Computers Hum Behav Rep. 2021;4:100125.
Imani V, Ahorsu DK, Taghizadeh N, Parsapour Z, Nejati B, Chen H-P, Pakpour AH. The mediating roles of anxiety, Depression, Sleepiness, Insomnia, and Sleep Quality in the Association between Problematic Social Media Use and Quality of Life among patients with Cancer. Healthcare 2022, 10(9).
Islam MS, Sujan MSH, Tasnim R, Mohona RA, Ferdous MZ, Kamruzzaman S, Toma TY, Sakib MN, Pinky KN, Islam MR et al. Problematic smartphone and Social Media Use among Bangladeshi College and University students amid COVID-19: the role of Psychological Well-Being and Pandemic related factors. Front Psychiatry 2021, 12.
Islam MS, Jahan I, Dewan MAA, Pontes HM, Koly KN, Sikder MT, Rahman M. Psychometric properties of three online-related addictive behavior instruments among Bangladeshi school-going adolescents. PLoS ONE 2022, 17(12).
Jahan I, Hosen I, Al Mamun F, Kaggwa MM, Griffiths MD, Mamun MA. How has the COVID-19 pandemic impacted Internet Use behaviors and facilitated problematic internet use? A Bangladeshi study. Psychol Res Behav Manage. 2021;14:1127–38.
Jiang Y. Problematic social media usage and anxiety among University Students during the COVID-19 pandemic: the mediating role of Psychological Capital and the moderating role of academic burnout. Front Psychol. 2021;12:612007–612007.
Kim M-R, Oh J-W, Huh B-Y. Analysis of factors related to Social Network Service Addiction among Korean High School Students. J Addictions Nurs. 2020;31(3):203–12.
Koc M, Gulyagci S. Facebook addiction among Turkish college students: the role of psychological health, demographic, and usage characteristics. Cyberpsychology Behav Social Netw. 2013;16(4):279–84.
Lin C-Y, Namdar P, Griffiths MD, Pakpour AH. Mediated roles of generalized trust and perceived social support in the effects of problematic social media use on mental health: a cross-sectional study. Health Expect. 2021;24(1):165–73.
Lin C-Y, Imani V, Griffiths MD, Brostrom A, Nygardh A, Demetrovics Z, Pakpour AH. Temporal associations between morningness/eveningness, problematic social media use, psychological distress and daytime sleepiness: mediated roles of sleep quality and insomnia among young adults. J Sleep Res 2021, 30(1).
Lozano Blasco R, Latorre Cosculluela C, Quilez Robres A. Social Network Addiction and its impact on anxiety level among University students. Sustainability 2020, 12(13).
Marino C, Musetti A, Vieno A, Manari T, Franceschini C. Is psychological distress the key factor in the association between problematic social networking sites and poor sleep quality? Addict Behav 2022, 133.
Meshi D, Ellithorpe ME. Problematic social media use and social support received in real-life versus on social media: associations with depression, anxiety and social isolation. Addict Behav 2021, 119.
Mitropoulou EM, Karagianni M, Thomadakis C. Social Media Addiction, Self-Compassion, and Psychological Well-Being: a structural equation Model. Alpha Psychiatry. 2022;23(6):298–304.
Ozimek P, Brailovskaia J, Bierhoff H-W. Active and passive behavior in social media: validating the Social Media Activity Questionnaire (SMAQ). Telematics Inf Rep. 2023;10:100048.
Phillips WJ, Wisniewski AT. Self-compassion moderates the predictive effects of social media use profiles on depression and anxiety. Computers Hum Behav Rep. 2021;4:100128.
Reer F, Festl R, Quandt T. Investigating problematic social media and game use in a nationally representative sample of adolescents and younger adults. Behav Inform Technol. 2021;40(8):776–89.
Satici B, Kayis AR, Griffiths MD. Exploring the Association between Social Media Addiction and relationship satisfaction: psychological distress as a Mediator. Int J Mental Health Addict 2021.
Sediri S, Zgueb Y, Ouanes S, Ouali U, Bourgou S, Jomli R, Nacef F. Women’s mental health: acute impact of COVID-19 pandemic on domestic violence. Archives Womens Mental Health. 2020;23(6):749–56.
Shabahang R, Shim H, Aruguete MS, Zsila A. Oversharing on Social Media: anxiety, Attention-Seeking, and Social Media Addiction Predict the breadth and depth of sharing. Psychol Rep 2022:332941221122861–332941221122861.
Sotero L, Ferreira Da Veiga G, Carreira D, Portugal A, Relvas AP. Facebook Addiction and emerging adults: the influence of sociodemographic variables, family communication, and differentiation of self. Escritos De Psicología - Psychol Writings. 2019;12(2):81–92.
Stockdale LA, Coyne SM. Bored and online: reasons for using social media, problematic social networking site use, and behavioral outcomes across the transition from adolescence to emerging adulthood. J Adolesc. 2020;79:173–83.
Wang Z, Yang H, Elhai JD. Are there gender differences in comorbidity symptoms networks of problematic social media use, anxiety and depression symptoms? Evidence from network analysis. Pers Indiv Differ. 2022;195:111705.
White-Gosselin C-E, Poulin F. Associations Between Young Adults’ Social Media Addiction, Relationship Quality With Parents, and Internalizing Problems: A Path Analysis Model. 2022.
Wong HY, Mo HY, Potenza MN, Chan MNM, Lau WM, Chui TK, Pakpour AH, Lin C-Y. Relationships between Severity of Internet Gaming Disorder, Severity of Problematic Social Media Use, Sleep Quality and Psychological Distress. Int J Environ Res Public Health 2020, 17(6).
Yam C-W, Pakpour AH, Griffiths MD, Yau W-Y, Lo C-LM, Ng JMT, Lin C-Y, Leung H. Psychometric testing of three Chinese online-related addictive Behavior instruments among Hong Kong University students. Psychiatr Q. 2019;90(1):117–28.
Yuan Y, Zhong Y. A survey on the use of social networks and mental health of college students during the COVID-19 pandemic. J Campus Life Mental Health\. 2021;19(3):209–12.
Google Scholar
Yurdagul C, Kircaburun K, Emirtekin E, Wang P, Griffiths MD. Psychopathological consequences related to problematic Instagram Use among adolescents: the mediating role of body image dissatisfaction and moderating role of gender. Int J Mental Health Addict. 2021;19(5):1385–97.
Zhang W, Pu J, He R, Yu M, Xu L, He X, Chen Z, Gan Z, Liu K, Tan Y, et al. Demographic characteristics, family environment and psychosocial factors affecting internet addiction in Chinese adolescents. J Affect Disord. 2022;315:130–8.
Zhang L, Wu Y, Jin T, Jia Y. Revision and validation of the Chinese short version of social media disorder. Mod Prev Med. 2021;48(8):1350–3.
Zhang X, Fan L. The influence of anxiety on colleges’ life satisfaction. Chin J Health Educ. 2021;37(5):469–72.
Zhao M, Wang H, Dong Y, Niu Y, Fang Y. The relationship between self-esteem and wechat addiction among undergraduate students: the multiple mediating roles of state anxiety and online interpersonal trust. J Psychol Sci. 2021;44(1):104–10.
Zhao J, Zhou Z, Sun B, Zhang X, Zhang L, Fu S. Attentional Bias is Associated with negative emotions in problematic users of Social Media as measured by a dot-probe Task. Int J Environ Res Public Health 2022, 19(24).
Atroszko PA, Balcerowska JM, Bereznowski P, Biernatowska A, Pallesen S, Schou Andreassen C. Facebook addiction among Polish undergraduate students: validity of measurement and relationship with personality and well-being. Comput Hum Behav. 2018;85:329–38.
Chen Y, Li R, Zhang P, Liu X. The moderating role of state attachment anxiety and avoidance between social anxiety and social networking sites Addiction. Psychol Rep. 2020;123(3):633–47.
Chen B, Zheng X, Sun X. The relationship between problematic social media use and online social anxiety: the roles of social media cognitive overload and dispositional mindfulness. Psychol Dev Educ. 2023;39(5):743–51.
Chentsova VO, Bravo AJ, Mezquita L, Pilatti A, Hogarth L, Cross-Cultural AS. Internalizing symptoms, rumination, and problematic social networking site use: a cross national examination among young adults in seven countries. Addict Behav 2023, 136.
Chu X, Ji S, Wang X, Yu J, Chen Y, Lei L. Peer phubbing and social networking site addiction: the mediating role of social anxiety and the moderating role of Family Financial Difficulty. Front Psychol. 2021;12:670065–670065.
Dempsey AE, O’Brien KD, Tiamiyu MF, Elhai JD. Fear of missing out (FoMO) and rumination mediate relations between social anxiety and problematic Facebook use. Addict Behav Rep. 2019;9:100150–100150.
PubMed PubMed Central Google Scholar
Yildiz Durak H, Seferoglu SS. Modeling of variables related to problematic social media usage: Social desirability tendency example. Scand J Psychol. 2019;60(3):277–88.
Ekinci N, Akat M. The relationship between anxious-ambivalent attachment and social appearance anxiety in adolescents: the serial mediation of positive Youth Development and Instagram Addiction. Psychol Rep 2023:332941231159600–332941231159600.
Foroughi B, Griffiths MD, Iranmanesh M, Salamzadeh Y. Associations between Instagram Addiction, academic performance, social anxiety, Depression, and life satisfaction among University students. Int J Mental Health Addict. 2022;20(4):2221–42.
He L. Influence mechanism and intervention suggestions on addiction of social network addiction. Gannan Normal University; 2021.
Hu Y. The influencing mechanism of type D personality on problematic social networking sites use among adolescents and intervention research. Central China Normal University; 2020.
Jia L. A study of the relationship between neuroticism, perceived social support, social anxiety and problematic social network use in high school students. Harbin Normal University; 2022.
Lee-Won RJ, Herzog L, Park SG. Hooked on Facebook: the role of social anxiety and need for Social Assurance in Problematic Use of Facebook. Cyberpsychology Behav Social Netw. 2015;18(10):567–74.
Li H. Social anxiety and internet interpersonal addiction in adolescents and countermeasures. Central China Normal University; 2022.
Lin W-S, Chen H-R, Lee TS-H, Feng JY. Role of social anxiety on high engagement and addictive behavior in the context of social networking sites. Data Technol Appl. 2019;53(2):156–70.
Liu Y. The influence of family function on social media addiction in adolescents: the chain mediation effect of social anxiety and resilience. Hunan Normal University; 2021.
Lyvers M, Salviani A, Costan S, Thorberg FA. Alexithymia, narcissism and social anxiety in relation to social media and internet addiction symptoms. Int J Psychology: J Int De Psychologie. 2022;57(5):606–12.
Majid A, Yasir M, Javed A, Ali P. From envy to social anxiety and rumination: how social media site addiction triggers task distraction amongst nurses. J Nurs Adm Manag. 2020;28(3):504–13.
Mou Q, Zhuang J, Gao Y, Zhong Y, Lu Q, Gao F, Zhao M. The relationship between social anxiety and academic engagement among Chinese college students: a serial mediation model. J Affect Disord. 2022;311:247–53.
Ruggieri S, Santoro G, Pace U, Passanisi A, Schimmenti A. Problematic Facebook use and anxiety concerning use of social media in mothers and their offspring: an actor-partner interdependence model. Addict Behav Rep. 2020;11:100256–100256.
Ruiz MJ, Saez G, Villanueva-Moya L, Exposito F. Adolescent sexting: the role of body shame, Social Physique anxiety, and social networking site addiction. Cyberpsychology Behav Social Netw. 2021;24(12):799–805.
She R, Kit Han Mo P, Li J, Liu X, Jiang H, Chen Y, Ma L, Tak Fai Lau J. The double-edged sword effect of social networking use intensity on problematic social networking use among college students: the role of social skills and social anxiety. Comput Hum Behav. 2023;140:107555.
Stănculescu E. The Bergen Social Media Addiction Scale Validity in a Romanian sample using item response theory and network analysis. Int J Mental Health Addict 2022.
Teng X, Lei H, Li J, Wen Z. The influence of social anxiety on social network site addiction of college students: the moderator of intentional self-regulation. Chin J Clin Psychol. 2021;29(3):514–7.
Tong W. Influence of boredom on the problematic mobile social networks usage in adolescents: multiple chain mediator. Chin J Clin Psychol. 2019;27(5):932–6.
Tu W, Jiang H, Liu Q. Peer victimization and adolescent Mobile Social Addiction: mediation of social anxiety and gender differences. Int J Environ Res Public Health 2022, 19(17).
Wang S. The influence of college students self-esteem, social anxiety and fear of missing out on the problematic mobile social networks usage. Huaibei Normal University; 2021.
Wang X. The impact of peer relationship and social anxiety on secondary vocational school students’ problematic social network use and intervention study. Huaibei Normal University; 2022.
Wegmann E, Stodt B, Brand M. Addictive use of social networking sites can be explained by the interaction of internet use expectancies, internet literacy, and psychopathological symptoms. J Behav Addictions. 2015;4(3):155–62.
Yang W. The relationship between the type of internet addiction and the personality traits in college students. Huazhong University of Science and Technology; 2004.
Yang Z. The relationship between social variables and social networking usage among shanghai working population. East China Normal University; 2013.
Zhang C. The relationship between perceived social support and problematic social network use among junior high school students: a chain mediation model and an intervention study. Hebei University; 2022.
Zhang J, Chang F, Huang D, Wen X. The relationship between neuroticism and the problematic mobile social networks use in adolescents: the mediating role of anxiety and positive self-presentation. Chin J Clin Psychol. 2021;29(3):598–602.
Zhang Z. College students’ loneliness and problematic social networking use: Chain mediation of social self-efficacy and social anxiety. Shanghai Normal University; 2019.
Zhu B. Discussion on mechanism of social networking addiction——Social anxiety, craving and excitability. Liaoning Normal University; 2017.
Blackwell D, Leaman C, Tramposch R, Osborne C, Liss M. Extraversion, neuroticism, attachment style and fear of missing out as predictors of social media use and addiction. Pers Indiv Differ. 2017;116:69–72.
Chen A. From attachment to addiction: the mediating role of need satisfaction on social networking sites. Comput Hum Behav. 2019;98:80–92.
Chen Y, Zhong S, Dai L, Deng Y, Liu X. Attachment anxiety and social networking sites addiction in college students: a moderated mediating model. Chin J Clin Psychol. 2019;27(3):497–500.
Li J. The relations among problematic social networks usage behavior, Childhood Trauma and adult attachment in University students. Hunan Agricultural University; 2020.
Liu C, Ma J-L. Adult attachment orientations and social networking site addiction: the Mediating effects of Online Social Support and the fear of missing out. Front Psychol. 2019;10:2629–2629.
Mo S, Huang W, Xu Y, Tang Z, Nie G. The impact of medical students’ attachment anxiety on the use of problematic social networking sites during the epidemic. Psychol Monthly. 2022;17(9):1–4.
Teng X. The effect of attachment anxiety on problematic mobile social network use: the role of loneliness and self-control. Harbin Normal University; 2021.
Worsley JD, Mansfield R, Corcoran R. Attachment anxiety and problematic social media use: the Mediating Role of Well-Being. Cyberpsychology Behav Social Netw. 2018;21(9):563–8.
Wu Z. The effect of adult attachment on problematic social network use: the chain mediating effect of loneliness and fear of missing out. Jilin University; 2022.
Xia N. The impact of attachment anxiety on adolescent problem social networking site use: a moderated mediation model. Shihezi University; 2022.
Young L, Kolubinski DC, Frings D. Attachment style moderates the relationship between social media use and user mental health and wellbeing. Heliyon 2020, 6(6).
Bakioglu F, Deniz M, Griffiths MD, Pakpour AH. Adaptation and validation of the online-fear of missing out inventory into Turkish and the association with social media addiction, smartphone addiction, and life satisfaction. BMC Psychol. 2022;10(1):154–154.
Bendayan R, Blanca MJ. Spanish version of the Facebook Intrusion Questionnaire (FIQ-S). Psicothema. 2019;31(2):204–9.
Blachnio A, Przepiorka A. Facebook intrusion, fear of missing out, narcissism, and life satisfaction: a cross-sectional study. Psychiatry Res. 2018;259:514–9.
Casale S, Rugai L, Fioravanti G. Exploring the role of positive metacognitions in explaining the association between the fear of missing out and social media addiction. Addict Behav. 2018;85:83–7.
Chen Y, Zhang Y, Zhang S, Wang K. Effect of fear of’ missing out on college students negative social adaptation: Chain¬ - mediating effect of rumination and problematic social media use. China J Health Psychol. 2022;30(4):581–6.
Cheng S, Zhang X, Han Y. Relationship between fear of missing out and phubbing on college students: the chain intermediary effect of intolerance of uncertainty and problematic social media use. China J Health Psychol. 2022;30(9):1296–300.
Cui Q, Wang J, Zhang J, Li W, Li Q. The relationship between loneliness and negative emotion in college students: the chain-mediating role of fear of missing out and social network sites addiction. J Jining Med Univ. 2022;45(4):248–51.
Ding Q, Wang Z, Zhang Y, Zhou Z. The more gossip, the more addicted: the relationship between interpersonal curiosity and social networking sites addiction tendencies in college students. Psychol Dev Educ. 2022;38(1):118–25.
Fabris MA, Marengo D, Longobardi C, Settanni M. Investigating the links between fear of missing out, social media addiction, and emotional symptoms in adolescence: the role of stress associated with neglect and negative reactions on social media. Addict Behav. 2020;106:106364.
Fang J, Wang X, Wen Z, Zhou J. Fear of missing out and problematic social media use as mediators between emotional support from social media and phubbing behavior. Addict Behav. 2020;107:106430.
Gao Z. The study on the relationship and intervention among fear of missing out self-differentiation and problematic social media use of college students. Yunnan Normal University; 2021.
Gioia F, Fioravanti G, Casale S, Boursier V. The Effects of the Fear of Missing Out on People’s Social Networking Sites Use During the COVID-19 Pandemic: The Mediating Role of Online Relational Closeness and Individuals’ Online Communication Attitude. Front Psychiatry 2021, 12.
Gu X. Study on the Inhibitory Effect of Mindfulness Training on Social Media Addiction of College Students. Wuhan University; 2020.
Gugushvili N, Taht K, Schruff-Lim EM, Ruiter RA, Verduyn P. The Association between Neuroticism and problematic social networking sites Use: the role of fear of missing out and Self-Control. Psychol Rep 2022:332941221142003–332941221142003.
Hou J. The study on FoMO and content social media addiction among young people. Huazhong University of Science and Technology; 2021.
Hu R, Zhang B, Yang Y, Mao H, Peng Y, Xiong S. Relationship between college students’ fear of missing and wechat addiction: a cross-lagged analysis. J Bio-education. 2022;10(5):369–73.
Hu G. The relationship between basic psychological needs satisfaction and the use of problematic social networks by college students: a moderated mediation model and online intervention studies. Jiangxi Normal University; 2020.
Jiang Y, Jin T. The relationship between adolescents’ narcissistic personality and their use of problematic mobile social networks: the effects of fear of missing out and positive self-presentation. Chin J Special Educ 2018(11):64–70.
Li J. The effect of positive self-presentation on social networking sites on problematic use of social networking sites: a moderated mediation model. Henan University; 2020.
Li J, Zhang Y, Zhang X. The impact of Freshmen Social Exclusion on problematic Social Network Use: a Moderated Mediation Model. J Heilongjiang Vocat Inst Ecol Eng. 2023;36(1):118–22.
Li M. The relationship between fear of missing out and social media addiction among middle school students——The moderating role of self-control. Kashi University; 2022.
Li R, Dong X, Wang M, Wang R. A study on the relationship between fear of missing out and social network addiction. New Educ Era 2021(52):122–3.
Li Y. Fear of missing out or social avoidance? The influence of peer exclusion on problematic social media use among adolescents in Guangdong Province and Macao. Guangzhou University; 2020.
Ma J, Liu C. The effect of fear of missing out on social networking sites addiction among college students: the mediating roles of social networking site integration use and social support. Psychol Dev Educ. 2019;35(5):605–14.
Mao H. A follow-up study on the mechanism of the influence of university students’ Qi deficiency quality on WeChat addiction. Hunan University of Chinese Medicine; 2021.
Mao Y. The effect of dual filial piety to the college students ’internet social dependence: the mediation of maladaptive cognition and fear of missing out. Huazhong University of Science and Technology; 2021.
Moore K, Craciun G. Fear of missing out and personality as predictors of Social networking sites usage: the Instagram Case. Psychol Rep. 2021;124(4):1761–87.
Niu J. The relationship of college students’ basic psychological needs and social media dependence: the mediating role of fear of missing out. Huazhong University of Science and Technology; 2021.
Pi L, Li X. Research on the relationship between loneliness and problematic mobile social media usage: evidence from variable-oriented and person-oriented analyses. China J Health Psychol. 2023;31(6):936–42.
Pontes HM, Taylor M, Stavropoulos V. Beyond Facebook Addiction: the role of cognitive-related factors and Psychiatric Distress in Social networking site addiction. Cyberpsychol Behav Soc Netw. 2018;21(4):240–7.
Quaglieri A, Biondi S, Roma P, Varchetta M, Fraschetti A, Burrai J, Lausi G, Marti-Vilar M, Gonzalez-Sala F, Di Domenico A et al. From Emotional (Dys) Regulation to Internet Addiction: A Mediation Model of Problematic Social Media Use among Italian Young Adults. Journal of Clinical Medicine 2022, 11(1).
Servidio R, Koronczai B, Griffiths MD, Demetrovics Z. Problematic smartphone Use and Problematic Social Media Use: the predictive role of Self-Construal and the Mediating Effect of Fear Missing Out. Front Public Health 2022, 10.
Sheldon P, Antony MG, Sykes B. Predictors of problematic social media use: personality and life-position indicators. Psychol Rep. 2021;124(3):1110–33.
Sun C, Li Y, Kwok SYCL, Mu W. The relationship between intolerance of uncertainty and problematic Social Media Use during the COVID-19 pandemic: a serial mediation model. Int J Environ Res Public Health 2022, 19(22).
Tang Z. The relationship between loneliness and problematic social networks use among college students: the mediation of fear of missing out and the moderation of social support. Jilin University; 2022.
Tomczyk Ł, Selmanagic-Lizde E. Fear of missing out (FOMO) among youth in Bosnia and Herzegovina — Scale and selected mechanisms. Child Youth Serv Rev. 2018;88:541–9.
Unal-Aydin P, Ozkan Y, Ozturk M, Aydin O, Spada MM. The role of metacognitions in cyberbullying and cybervictimization among adolescents diagnosed with major depressive disorder and anxiety disorders: a case-control study. Clinical Psychology & Psychotherapy; 2023.
Uram P, Skalski S. Still logged in? The Link between Facebook Addiction, FoMO, Self-Esteem, Life satisfaction and loneliness in social media users. Psychol Rep. 2022;125(1):218–31.
Varchetta M, Fraschetti A, Mari E, Giannini AM. Social Media Addiction, fear of missing out (FoMO) and online vulnerability in university students. Revista Digit De Investigación en Docencia Universitaria. 2020;14(1):e1187.
Wang H. Study on the relationship and intervention between fear of missing and social network addiction in college students. Yunnan Normal University; 2021.
Wang M, Yin Z, Xu Q, Niu G. The relationship between shyness and adolescents’ social network sites addiction: Moderated mediation model. Chin J Clin Psychol. 2020;28(5):906–9.
Wegmann E, Oberst U, Stodt B, Brand M. Online-specific fear of missing out and internet-use expectancies contribute to symptoms of internet-communication disorder. Addict Behav Rep. 2017;5:33–42.
Wegmann E, Brandtner A, Brand M. Perceived strain due to COVID-19-Related restrictions mediates the Effect of Social needs and fear of missing out on the risk of a problematic use of Social Networks. Front Psychiatry 2021, 12.
Wei Q. Negative emotions and problematic social network sites usage: the mediating role of fear of missing out and the moderating role of gender. Central China Normal University; 2018.
Xiong L. Effect of social network site use on college students’ social network site addiction: A moderated mediation model and attention bias training intervention study. Jiangxi Normal University; 2022.
Yan H. The influence of college students’ basic psychological needs on social network addiction: The intermediary role of fear of missing out. Wuhan University; 2020.
Yan H. The status and factors associated with social media addiction among young people——Evidence from WeChat. Chongqing University; 2021.
Yang L. Research on the relationship of fear of missing out, excessive use of Wechat and life satisfaction. Beijing Forestry University; 2020.
Yin Y, Cai X, Ouyang M, Li S, Li X, Wang P. FoMO and the brain: loneliness and problematic social networking site use mediate the association between the topology of the resting-state EEG brain network and fear of missing out. Comput Hum Behav. 2023;141:107624.
Zhang C. The parental rejection and problematic social network sites with adolescents: the chain mediating effect of basic psychological needs and fear of missing out. Central China Normal University; 2022.
Zhang J. The influence of basic psychological needs on problematic mobile social networks usage of adolescent: a moderated mediation model. Liaocheng University; 2020.
Zhang Y, Chen Y, Jin J, Yu G. The relationship between fear of missing out and social media addiction: a cross-lagged analysis. Chin J Clin Psychol. 2021;29(5):1082–5.
Zhang Y, Jiang W, Ding Q, Hong M. Social comparison orientation and social network sites addiction in college students: the mediating role of fear of missing out. Chin J Clin Psychol. 2019;27(5):928–31.
Zhou J, Fang J. Social network sites support and addiction among college students: a moderated mediation model. Psychology: Techniques Appl. 2021;9(5):293–9.
Andreassen CS, Torsheim T, Brunborg GS, Pallesen S. Development of a Facebook addiction scale. Psychol Rep. 2012;110(2):501–17.
Andreassen CS, Billieux J, Griffiths MD, Kuss DJ, Demetrovics Z, Mazzoni E, Pallesen S. The relationship between addictive use of social media and video games and symptoms of psychiatric disorders: a large-scale cross-sectional study. Psychol Addict Behav. 2016;30(2):252.
Elphinston RA, Noller P. Time to face it! Facebook intrusion and the implications for romantic jealousy and relationship satisfaction. Cyberpsychology Behav Social Netw. 2011;14(11):631–5.
Caplan SE. Theory and measurement of generalized problematic internet use: a two-step approach. Comput Hum Behav. 2010;26(5):1089–97.
Jiang Y. Development of problematic mobile social media usage assessment questionnaire for adolescents. Psychology: Techniques Appl. 2018;6(10):613–21.
Wang X. College students’ social network addiction tendency: Questionnaire construction and correlation research. Master’s thesis Southwest University; 2016.
Derogatis LR. Brief symptom inventory 18. Johns Hopkins University Baltimore; 2001.
Lovibond PF, Lovibond SH. The structure of negative emotional states: comparison of the Depression anxiety stress scales (DASS) with the Beck Depression and anxiety inventories. Behav Res Ther. 1995;33(3):335–43.
Spitzer RL, Kroenke K, Williams JB, Löwe B. A brief measure for assessing generalized anxiety disorder: the GAD-7. Arch Intern Med. 2006;166(10):1092–7.
Zigmond AS, Snaith RP. The hospital anxiety and depression scale. Acta Psychiatrica Scandinavica. 1983;67(6):361–70.
Spielberger CD, Gonzalez-Reigosa F, Martinez-Urrutia A, Natalicio LF, Natalicio DS. The state-trait anxiety inventory. Revista Interamericana de Psicologia/Interamerican Journal of Psychology 1971, 5(3&4).
Marteau TM, Bekker H. The development of a six-item short‐form of the state scale of the Spielberger State—trait anxiety inventory (STAI). Br J Clin Psychol. 1992;31(3):301–6.
Leary MR. Social anxiousness: the construct and its measurement. J Pers Assess. 1983;47(1):66–75.
Liebowitz MR. Social phobia. Modern problems of pharmacopsychiatry 1987.
Alkis Y, Kadirhan Z, Sat M. Development and validation of social anxiety scale for social media users. Comput Hum Behav. 2017;72:296–303.
La Greca AM, Stone WL. Social anxiety scale for children-revised: factor structure and concurrent validity. J Clin Child Psychol. 1993;22(1):17–27.
Fenigstein A, Scheier MF, Buss AH. Public and private self-consciousness: Assessment and theory. J Consult Clin Psychol. 1975;43(4):522.
Mattick RP, Clarke JC. Development and validation of measures of social phobia scrutiny fear and social interaction anxiety. Behav Res Ther. 1998;36(4):455–70.
Peters L, Sunderland M, Andrews G, Rapee RM, Mattick RP. Development of a short form Social Interaction anxiety (SIAS) and Social Phobia Scale (SPS) using nonparametric item response theory: the SIAS-6 and the SPS-6. Psychol Assess. 2012;24(1):66.
Brennan KA, Clark CL, Shaver PR. Self-report measurement of adult attachment: an integrative overview. Attachment Theory Close Relationships. 1998;46:76.
Wei M, Russell DW, Mallinckrodt B, Vogel DL. The experiences in Close Relationship Scale (ECR)-short form: reliability, validity, and factor structure. J Pers Assess. 2007;88(2):187–204.
Bartholomew K, Horowitz LM. Attachment styles among young adults: a test of a four-category model. J Personal Soc Psychol. 1991;61(2):226.
Przybylski AK, Murayama K, DeHaan CR, Gladwell V. Motivational, emotional, and behavioral correlates of fear of missing out. Comput Hum Behav. 2013;29(4):1841–8.
Xiaokang S, Yuxiang Z, Xuanhui Z. Developing a fear of missing out (FoMO) measurement scale in the mobile social media environment. Libr Inform Service. 2017;61(11):96.
Bown M, Sutton A. Quality control in systematic reviews and meta-analyses. Eur J Vasc Endovasc Surg. 2010;40(5):669–77.
Turel O, Qahri-Saremi H. Problematic use of social networking sites: antecedents and consequence from a dual-system theory perspective. J Manage Inform Syst. 2016;33(4):1087–116.
Chou H-TG, Edge N. They are happier and having better lives than I am: the impact of using Facebook on perceptions of others’ lives. Cyberpsychology Behav Social Netw. 2012;15(2):117–21.
Beyens I, Frison E, Eggermont S. I don’t want to miss a thing: adolescents’ fear of missing out and its relationship to adolescents’ social needs, Facebook use, and Facebook related stress. Comput Hum Behav. 2016;64:1–8.
Di Blasi M, Gullo S, Mancinelli E, Freda MF, Esposito G, Gelo OCG, Lagetto G, Giordano C, Mazzeschi C, Pazzagli C. Psychological distress associated with the COVID-19 lockdown: a two-wave network analysis. J Affect Disord. 2021;284:18–26.
Yang X, Hu H, Zhao C, Xu H, Tu X, Zhang G. A longitudinal study of changes in smart phone addiction and depressive symptoms and potential risk factors among Chinese college students. BMC Psychiatry. 2021;21(1):252.
Kuss DJ, Griffiths MD. Social networking sites and addiction: ten lessons learned. Int J Environ Res Public Health. 2017;14(3):311.
Ryan T, Chester A, Reece J, Xenos S. The uses and abuses of Facebook: a review of Facebook addiction. J Behav Addictions. 2014;3(3):133–48.
Elhai JD, Levine JC, Dvorak RD, Hall BJ. Non-social features of smartphone use are most related to depression, anxiety and problematic smartphone use. Comput Hum Behav. 2017;69:75–82.
Jackson LA, Wang J-L. Cultural differences in social networking site use: a comparative study of China and the United States. Comput Hum Behav. 2013;29(3):910–21.
Ahrens LM, Mühlberger A, Pauli P, Wieser MJ. Impaired visuocortical discrimination learning of socially conditioned stimuli in social anxiety. Soc Cognit Affect Neurosci. 2014;10(7):929–37.
Elhai JD, Yang H, Montag C. Fear of missing out (FOMO): overview, theoretical underpinnings, and literature review on relations with severity of negative affectivity and problematic technology use. Brazilian J Psychiatry. 2020;43:203–9.
Barker V. Older adolescents’ motivations for social network site use: the influence of gender, group identity, and collective self-esteem. Cyberpsychology Behav. 2009;12(2):209–13.
Krasnova H, Veltri NF, Eling N, Buxmann P. Why men and women continue to use social networking sites: the role of gender differences. J Strateg Inf Syst. 2017;26(4):261–84.
Palmer J. The role of gender on social network websites. Stylus Knights Write Showc 2012:35–46.
Vannucci A, Flannery KM, Ohannessian CM. Social media use and anxiety in emerging adults. J Affect Disord. 2017;207:163–6.
Primack BA, Shensa A, Sidani JE, Whaite EO, yi Lin L, Rosen D, Colditz JB, Radovic A, Miller E. Social media use and perceived social isolation among young adults in the US. Am J Prev Med. 2017;53(1):1–8.
Twenge JM, Campbell WK. Associations between screen time and lower psychological well-being among children and adolescents: evidence from a population-based study. Prev Med Rep. 2018;12:271–83.
Download references
This research was supported by the Social Science Foundation of China (Grant Number: 23BSH135).
Author information
Authors and affiliations.
School of Mental Health, Wenzhou Medical University, 325035, Wenzhou, China
Mingxuan Du, Haiyan Hu, Ningning Ding, Jiankang He, Wenwen Tian, Wenqian Zhao, Xiujian Lin, Gaoyang Liu, Wendan Chen, ShuangLiu Wang, Dongwu Xu & Guohua Zhang
School of Education, Renmin University of China, 100872, Beijing, China
Chengjia Zhao
School of Media and Communication, Shanghai Jiao Tong University, Dongchuan Road 800, 200240, Shanghai, China
Pengcheng Wang
Department of Neurosis and Psychosomatic Diseases, Huzhou Third Municipal Hospital, 313002, Huzhou, China
Xinhua Shen
You can also search for this author in PubMed Google Scholar
Contributions
GZ, XS, XL and MD prepared the study design, writing - review and editing. MD and CZ wrote the main manuscript text. MD and HH analyzed data and edited the draft. ND, JH, WT, WZ, GL, WC, SW, PW and DX conducted resources and data curation. All authors have approved the final version of the manuscript.
Corresponding authors
Correspondence to Xinhua Shen or Guohua Zhang .
Ethics declarations
Ethics approval and consent to participate.
Not applicable.
Consent for publication
Competing interests.
The authors declare no competing interests.
Additional information
Publisher’s note.
Springer Nature remains neutral with regard to jurisdictional claims in published maps and institutional affiliations.
Rights and permissions
Open Access This article is licensed under a Creative Commons Attribution 4.0 International License, which permits use, sharing, adaptation, distribution and reproduction in any medium or format, as long as you give appropriate credit to the original author(s) and the source, provide a link to the Creative Commons licence, and indicate if changes were made. The images or other third party material in this article are included in the article’s Creative Commons licence, unless indicated otherwise in a credit line to the material. If material is not included in the article’s Creative Commons licence and your intended use is not permitted by statutory regulation or exceeds the permitted use, you will need to obtain permission directly from the copyright holder. To view a copy of this licence, visit http://creativecommons.org/licenses/by/4.0/ . The Creative Commons Public Domain Dedication waiver ( http://creativecommons.org/publicdomain/zero/1.0/ ) applies to the data made available in this article, unless otherwise stated in a credit line to the data.
Reprints and permissions
About this article
Cite this article.
Du, M., Zhao, C., Hu, H. et al. Association between problematic social networking use and anxiety symptoms: a systematic review and meta-analysis. BMC Psychol 12 , 263 (2024). https://doi.org/10.1186/s40359-024-01705-w
Download citation
Received : 25 January 2024
Accepted : 03 April 2024
Published : 12 May 2024
DOI : https://doi.org/10.1186/s40359-024-01705-w
Share this article
Anyone you share the following link with will be able to read this content:
Sorry, a shareable link is not currently available for this article.
Provided by the Springer Nature SharedIt content-sharing initiative
- Fear of missing out
- Meta-analysis
BMC Psychology
ISSN: 2050-7283
- General enquiries: [email protected]
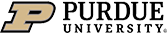
Comparison of Soil Carbon Dynamics Between Restored Prairie and Agricultural Soils in the U.S. Midwest
Globally, soils hold more carbon than both the atmosphere and aboveground terrestrial biosphere combined. Changes in land use and land cover have the potential to alter soil carbon cycling throughout the soil profile, from the surface to meters deep, yet most studies focus only on the near surface impact ( 3 and C 4 photosynthetic pathway plant community composition. Comparative analysis of edaphic properties and soil carbon suggests that deep loess deposits in Nebraska permit enhanced water infiltration and SOC deposition to depths of ~100 cm in 60 years of prairie restoration. In Illinois, poorly drained, clay/lime rich soils on glacial till and a younger restored prairie age (15 years) restricted the influence of prairie restoration to the upper 30 cm. Comparing the δ 13 C values of SOC and SIC in each system demonstrated that SIC at each site is likely of lithogenic origin. This work indicates that the magnitude of influence of restoration management is dependent on edaphic properties inherited from geological and geomorphological controls. Future work should quantify root structures and redox properties to better understand the influence of rooting depth on soil carbon concentrations. Fast-cycling C dynamics can be assessed using continuous, in-situ CO 2 and O 2 soil gas concentration changes. The secondary objective of my thesis was to determine if manual, low temporal resolution gas sampling and analysis are a low cost and effective means of measuring soil O 2 and CO 2 , by comparing it with data from in-situ continuous (hourly) sensors. Manual analysis of soil CO 2 and O 2 from field replicates of buried gas collection cups resulted in measurement differences from the continuous sensors. Measuring CO2 concentration with manual methods often resulted in higher concentrations than hourly, continuous measurements across all sites. Additionally, O 2 concentrations measured by manual methods were higher than hourly values in the restored prairie and less in agricultural sites. A variety of spatial variability, pressure perturbations, calibration offsets, and system leakage influences on both analysis methods could cause the discrepancy.
NSF Grant 1331906
Degree type.
- Master of Science
- Earth, Atmospheric and Planetary Sciences
Campus location
- West Lafayette
Advisor/Supervisor/Committee Chair
Additional committee member 2, additional committee member 3, additional committee member 4, additional committee member 5, usage metrics.
- Environmental biogeochemistry
- Soil chemistry and soil carbon sequestration (excl. carbon sequestration science)

- Open access
- Published: 14 May 2024
Multi-class boosting for the analysis of multiple incomplete views on microbiome data
- Andrea Simeon 1 na1 ,
- Miloš Radovanović 2 ,
- Tatjana Lončar-Turukalo 3 ,
- Michelangelo Ceci 4 , 5 , 6 ,
- Sanja Brdar 1 &
- Gianvito Pio 4 , 5 na1
BMC Bioinformatics volume 25 , Article number: 188 ( 2024 ) Cite this article
56 Accesses
6 Altmetric
Metrics details
Microbiome dysbiosis has recently been associated with different diseases and disorders. In this context, machine learning (ML) approaches can be useful either to identify new patterns or learn predictive models. However, data to be fed to ML methods can be subject to different sampling, sequencing and preprocessing techniques. Each different choice in the pipeline can lead to a different view (i.e., feature set) of the same individuals, that classical (single-view) ML approaches may fail to simultaneously consider. Moreover, some views may be incomplete, i.e., some individuals may be missing in some views, possibly due to the absence of some measurements or to the fact that some features are not available/applicable for all the individuals. Multi-view learning methods can represent a possible solution to consider multiple feature sets for the same individuals, but most existing multi-view learning methods are limited to binary classification tasks or cannot work with incomplete views.
We propose irBoost.SH, an extension of the multi-view boosting algorithm rBoost.SH, based on multi-armed bandits. irBoost.SH solves multi-class classification tasks and can analyze incomplete views. At each iteration, it identifies one winning view using adversarial multi-armed bandits and uses its predictions to update a shared instance weight distribution in a learning process based on boosting. In our experiments, performed on 5 multi-view microbiome datasets, the model learned by irBoost.SH always outperforms the best model learned from a single view, its closest competitor rBoost.SH, and the model learned by a multi-view approach based on feature concatenation, reaching an improvement of 11.8% of the F1-score in the prediction of the Autism Spectrum disorder and of 114% in the prediction of the Colorectal Cancer disease.
Conclusions
The proposed method irBoost.SH exhibited outstanding performances in our experiments, also compared to competitor approaches. The obtained results confirm that irBoost.SH can fruitfully be adopted for the analysis of microbiome data, due to its capability to simultaneously exploit multiple feature sets obtained through different sequencing and preprocessing pipelines.
Peer Review reports
Introduction
Over the last few years, microbiome dysbiosis has been associated with many diseases, since it tends to occur as an accompanying symptom with a higher prevalence than usual in disease conditions. Microbiome alterations can be related to some types of cancer [ 1 , 2 , 3 ] or, through the so-called microbiota-gut-brain axis, to neurodevelopmental conditions such as the Autism Spectrum Disorder (ASD) [ 4 , 5 ].
Sequencing of microbiome samples is considered convenient, especially when other tests are impossible, hard or invasive to perform. However, despite the reduction of sequencing costs, they can still be problematic, specifically for large microbiome studies or whole genome studies. In this context, the Next Generation Sequencing (NGS) technology has revolutionized microbiome sequence analysis. Indeed, while initially the research targeted only hypervariable regions of conserved genes such as 16S ribosomal RNA (rRNA) gene, more recently, it has been expanded on longer reads [ 6 , 7 ] and on the whole genome. In 16S ( amplicon ) metagenomic sequencing, short chains (< 500 bp) of nucleotides from 16S rRNA gene are amplified by PCR and then read out. This is further used to identify and differentiate multiple microbial species from multiple samples at once. In spite of simplification, this approach suffers from several drawbacks: poor resolution (only up to the level of species); poor diversity detection; sample source dependency, specifically on sampling, sequencing and analysis protocols, such DNA extraction kits and alignment algorithms [ 6 , 7 , 8 ]; poor reproducibility [ 6 ].
The sequencing of the whole genome solved some of the above-mentioned limitations, among which the most important is identification accuracy. This sequencing technique, called shotgun , involves randomly breaking up the genome into small overlapping DNA fragments, that are sequenced individually and virtually reassembled. Even if more precise, it also exhibits some drawbacks: the issue about the sample source dependency is not solved, and it is generally more expensive.
16S amplicon sequencing was initially very popular, and the amount of available data collected with this technique is currently much larger than that of the shotgun counterpart. However, several studies now aim to adopt both approaches [ 4 ], even if their simultaneous exploitation in a pipeline of analysis is challenging.
In the literature, we can find several attempts involving the adoption of machine learning (ML) techniques for the analysis of microbiome data [ 9 , 10 , 11 ]. However, to the best of the authors’ knowledge, there is no work aiming to consider simultaneously 16S and shotgun data in the construction of ML models. A simple solution would consist in the concatenation of the features representing the same individual, obtained through 16S and shotgun techniques. However, this approach can exacerbate the curse of dimensionality [ 12 ], which is already present in microbiome data due to the high unbalancing between the (usually low) number of individuals and the (usually high) number of features. In this context, multi-view learning approaches [ 12 , 13 , 14 ] represent a possible solution, since they mainly follow a decision level fusion, based on average/voting approaches, similarly to ensemble techniques. In these methods, different feature sets of the same instances (e.g., pictures of the same objects taken from different perspectives, features representing conceptually different aspects, or features obtained after the application of different preprocessing techniques) are called views , and a ML model is learned on each view separately. Subsequently, their output is combined and iteratively exploited to boost the performance of the learned models.
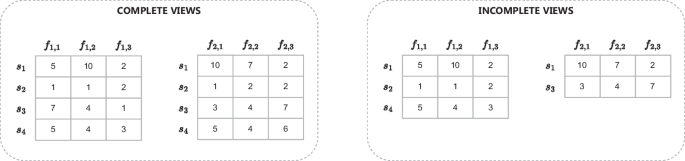
An example showing the difference between complete (on the left) and incomplete (on the right) views: rows correspond to the instances (individuals, in the case of microbiome studies); each view represents a different viewpoint of the instances (e.g., 16S and shotgun data, or features obtained through different preprocessing pipelines). In the case of incomplete views, we can observe that not all instances are represented according to both views (e.g., \(s_3\) is not represented in the first view, while \(s_2\) and \(s_4\) are not represented in the second view
Among the pioneering techniques, we can mention the co-training framework [ 15 ], that works on two views in the semi-supervised setting. In this framework, unlabeled examples are labeled by each of the two classifiers, and the most reliable predictions are fed as new training examples to the classifier working on the other view, for the subsequent iteration(s). This approach was originally limited to working on exactly two views. Different variants have been proposed in the literature, such as the co-regularization technique [ 16 , 17 ], that was mainly adopted for reducing overfitting issues. In [ 16 ], the authors extended it to work with more than two views, while in the [ 18 ], the authors proposed a multi-view learning approach able to work in the positive-unlabeled learning setting for gene network reconstruction.
In the work [ 19 ], the authors extended the well-known boosting algorithm Adaboost [ 20 ] to the multi-view learning setting. In [ 21 ], the authors proposed an efficient version using adversarial multi-armed bandits for selecting one of the views based on its usefulness, instead of repeatedly considering all views. Other approaches for multi-view learning exploit kernel-based algorithms. Relevant examples include the system proposed in [ 22 ], which uses within- and between-view regularization, and the work [ 23 ], where the authors formulate the learning problem on each view as a Multiple Kernel Learning (MKL) problem. Both approaches were compared with four different methods proposed in [ 12 ], along with the original Adaboost on concatenated views, and the system Mumbo [ 24 ], a multi-view algorithm for multi-class classification that exploits a separate instance weight distribution for each view. Among the considered competitors and the variants proposed in [ 12 ], rBoost.SH, which is based on partial information games, obtained the best results.
One of the strongest limitations of the aforementioned approaches is that they can only work when samples are fully observed on all the considered views. However, in real-world situations, some of the available observations may not be represented by all the views (i.e., they are partially observed). A relevant example in the context of microbiome data can be represented by individuals for whom we have a 16S sample but not a shotgun sample, or vice versa. In this scenario, the above-mentioned approaches cannot be adopted without a preprocessing step aimed to fill missing values, possibly introducing excessive approximations in the data. In [ 25 ], the authors proposed a multi-view model to complete missing values using a SOR-like optimization algorithm. The issue about incomplete views in multi-view learning has also been tackled in two recent papers: [ 14 ] considered data incompleteness for the multi-view clustering task, while [ 26 ] adopted a graph-based learning approach for classification with incomplete views.
Another limitation of most of multi-view methods comes from their inability to solve multi-class classification tasks. While some approaches like Mumbo [ 24 ] tackled this issue, to the best of the authors’ knowledge, none of them can simultaneously solve multi-class classification tasks and work on incomplete views.
In this paper, we aim to fill this gap by proposing a novel multi-view approach that is able to work on incomplete views (see an example in Fig. 1 ) to solve multi-class classification problems. We adopt the proposed method in the context of microbiome studies for two different scenarios: (i) to classify individuals according to 16S and/or shotgun microbiome data, which represent two, possibly incomplete, different views; (ii) to classify individuals considering multiple preprocessing pipelines to generate different views from the same 16S data. Through the former scenario we demonstrate the ability of our approach to fully exploit both views of the microbiome data, while in the latter we show the applicability of our approach to solve one of the critical issues of the analysis of microbiome data, namely, the selection of the best preprocessing pipeline(s), which in our case is fully automated.
The remainder of the paper is structured as follows: in ‘ Methods ’ section we describe the proposed approach in detail, starting from the background concepts it is based on; ‘ Experimental setting ’ section presents the considered tasks, together with the adopted datasets and the experimental setting considered; in ‘ Results and discussion ’ section we show and discuss the obtained results. Finally, in ‘ Conclusion ’ section we conclude the paper and present some avenues for future work.
The approach we propose in this paper is based on the method rBoost.SH [ 12 ]. Specifically, we significantly extend it to make it able to solve multi-class classification tasks and to work with incomplete views. We call our approach irBoost.SH. In ‘ Boost.SH and rBoost.SH: binary classifier via multi-view boosting from complete views ’ section, we first briefly describe the methods Boost.SH and rBoost.SH, together with the main concepts they rely on. Then, in ‘ The proposed method irBoost.SH: multi-class classifier via multi-view boosting from incomplete views ’ section we provide the details about our method irBoost.SH.
Boost.SH and rBoost.SH: binary classifier via multi-view boosting from complete views
In this subsection, we provide a quick overview of Boost.SH , which is the simplest version among the multi-view algorithms proposed in [ 12 , 27 ], and then we describe its extension rBoost.SH which is based on adversarial multi-armed bandits. Both Boost.SH and rBoost.SH adopt a single instance weight distribution that is shared among the views, unlike the multi-armed version of AdaBoost [ 21 ], which allows each view to adopt its own weight distribution. We remind that the approach we propose in this paper is based on rBoost.SH , which can originally solve only binary classification tasks from complete views.
Boost.SH starts from training data \(S = \{({\textbf{x}}_i, y_i)\}_{i=1}^{n}\) , where \({\textbf{x}}_i = \{x_i^1, x_i^2,..., x_i^K\}\) is a training instance, \(x_i^j \in {\mathbb {R}}^{q_j}\) is the instance \(x_i\) represented according to the j -th view in its \(q_j\) -dimensional feature space, and \(y_i \in {\mathcal {Y}}=\{-1, +1\}\) is the label of the instance \(x_i\) . Instances \(({\textbf{x}}_i, y_i)\) are assumed to be independently and identically distributed according to a probability distribution D over \({\mathcal {X}} \times {\mathcal {Y}}\) , where \({\mathcal {X}} \subseteq {\mathbb {R}}^q\) and \(q=\sum _{j=1}^{K}q_j\) . At the beginning, the shared instance weights are uniformly distributed.
For each iteration of the algorithm and for each view, a weak classifier is trained and, on the basis of the predictions on training data, an edge is computed, that somehow represents the current predictive accuracy of such a view. Afterwards, the algorithm selects the view with the largest edge as the winning view. The edge of the winning view and the corresponding classifier are used to update the shared instance weights for the subsequent iterations. Finally, after T iterations, Boost.SH builds a combined classifier as a weighted sum of the winning classifiers over all the T iterations.
Despite optimizing the consistency through the shared weight distribution, Boost.SH does not promote diversity [ 12 ]. In general, the algorithm is limited to learn only from the views with the maximal edge. The extreme situation happens when a view wins for all the iterations, that would degenerate to learning from one single view, discarding the possible contribution provided by the other(s).
This limitation has been overcome by its randomized version, called rBoost.SH , that is formalized in Algorithm 1. It incorporates adversarial multi-armed bandits (AMB) into a boosting process by computing probabilities \({\textbf{p}}_{1 \times K}\) for choosing the actions to take (i.e., the views to select as winner).
Auer et al. [ 28 , 29 ] proposed different versions of AMB. The one of interest here is a partial information game algorithm, called EXP3.P, in which a player (an algorithm) and an adversary (views) compete, and the reward is the only information propagated to the player. Unlike other problems based on bandits, in this case, no statistical assumptions are made about the process followed to generate the rewards (stochastic bandits, for instance, use the assumption that rewards are generated from a given, pre-determined, distribution). In rBoost.SH , the AMB problem is addressed using the exponentially weighted average (EWA) forecaster algorithm [ 12 , 30 ]. The forecaster algorithm updates the probability distribution over actions/views, such that the probability of choosing an action exponentially depends on the average rewards associated with it [ 12 ]. The update of this probability distribution is controlled by two parameters, namely \(\sigma\) and \(\gamma\) .
Contrary to Boost.SH, rBoost.SH computes the predictions only along the selected view j (see line 5 in Algorithm 1). The reward for such a view is divided by the probability of selecting it (see line 9 in Algorithm 1), which encourages the views with low probability to be selected, promoting diversity.
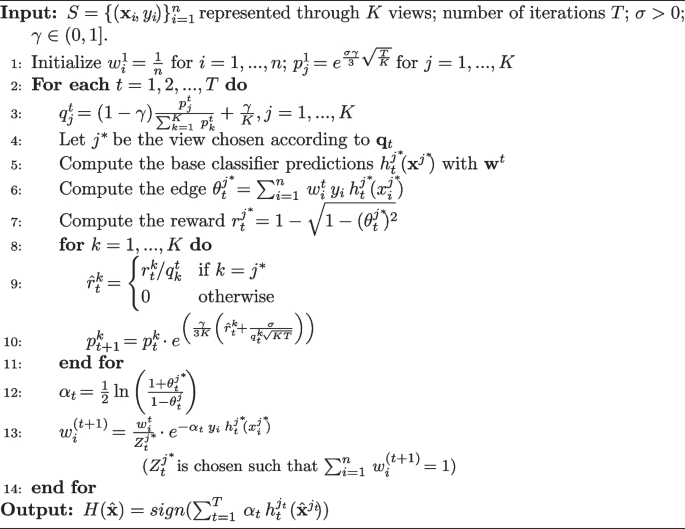
The method rBoost.SH
The proposed method irBoost.SH: multi-class classifier via multi-view boosting from incomplete views
In this subsection, we present our method irBoost.SH, which overcomes the main limitations of rBoost.SH, namely, it can solve multi-class classification tasks and can also work with incomplete views, i.e., when some instances are not represented according to all the views.
The proposed method irBoost.SH is formalized in Algorithm 2. In this case, the set S consists of views of possibly different shapes: each instance can be present in one or more views, but not necessarily in all the views, and the number of features in each view can be different.
For each view j , with \(j=1, 2,...,K\) , we denote with \({\mathcal {N}}_j\) the set of instances that are present in view j , and with \({\mathcal {N}} = \bigcup _{j=1}^{K}\ {\mathcal {N}}_j\) the set of all the instances. Accordingly, \(|{\mathcal {N}}|\) denotes the total number of instances. Every instance is associated with a class label \(y_i \in {\mathcal {Y}} \subset \mathbb {N^+}\) , which corresponds to a multi-class classification task, with each different integer representing a different class.
The algorithm starts by initializing the weights \(w_i\) for each instance \(i \in {\mathcal {N}}\) , and probabilities \(p_j\) for each view \(j=1,2,...,K\) (Algorithm 2, line 1). Lines 3 and 4 of Algorithm 2 are the same as lines 3 and 4 of Algorithm 1 (rBoost.SH). Besides computing the predicted label, the weak classifier in irBoost.SH outputs a class probability vector \({\textbf{v}}_i\) for each instance (Algorithm 2, line 5), that is a confidence vector with components in [0, 1] for each class \(l\in {\mathcal {Y}}\) . The predicted label is used in the computation of the edge (Algorithm 2, line 7), thus indirectly also for the computation of the reward (Algorithm 2, line 8) and for the update of the shared weights (Algorithm 2, line 14). Unlike rBoost.SH, irBoost.SH uses class probabilities in the output prediction.
Because of the possibility to have incomplete views, we define the modified predicted label \({\hat{h}}^j(x_i^j)\) and the modified class probability vector \(\hat{{\textbf{v}}}^j(x_i^j)\) for \(i \in {\mathcal {N}}\) , as follows:
Note that 0 in Eq. ( 1 ) does not correspond to a class, but it is an artificial label (note that \({\mathcal {Y}} \subset {\mathbb {N}}^+\) ) for unknown class label prediction for instances not contained in that view j . In that case, the artificial prediction, and hence unknown instance representation, will not contribute to the computation of the edge nor to the update of the weights. Accordingly, in Eq. ( 2 ), \({0}\) represents the unknown probability vector, which is, however, used in the prediction phase.
We also update the computation of the edge towards the multi-class classification task. In the original rBoost.SH, the multiplication of real and predicted labels \(y_i \cdot h_t^j\) leads to 1 when they agree, i.e., \(1 \cdot 1\) or \((-1) \cdot (-1)\) , and to \(-1\) when they disagree, i.e., \((-1) \cdot 1\) or \(1 \cdot (-1)\) . Analogously, we define the function \({\mathbb {I}}\) , that generalizes such agreement, as follows:
Accordingly, we also modify the sum of multiplications of the original rBoost.SH ( \(\sum _{i\in {\mathcal {N}}} y_i \cdot h(x_i)\) , where \(y_i,\ h(x_i) \in \{-1, +1\}\) ) as \(2 \cdot \sum _{i\in {\mathcal {N}}} \left( {\mathbb {I}}[y_i=h(x_i)] - 0.5\right)\) , where \({\mathbb {I}}\) is computed through Eq. ( 3 ), and \(y_i,\ h(x_i) \in {\mathbb {N}}\) . More formally, we derive the updated sum of multiplications as follows:
The above term is almost the same used in the computation of the edge (Algorithm 2, line 7), except for the fact that it is weighted. Since \({\mathbb {I}}\) compares real and predicted values, and outputs values in \(\{0,1\}\) , now the class label can be any positive natural number. In the case of incomplete views, the predicted and the real label for an instance that is absent in the chosen view would never be the same, because of the definition given in Eq. ( 1 ) and the assumption that class labels are from a subset of positive natural numbers. Accordingly, it will not contribute to the total sum. Formally:
As a final remark, we want to point out that the approach followed by irBoost.SH (but also its predecessor rBoost.SH) exhibits an interesting advantage: the number of times each view is selected as the winning view can be seen as an indicator of its relevance. As emphasized in the introduction, this aspect is very useful when views correspond to different preprocessing pipelines in order to identify the most useful ones for the predictive task at hand.
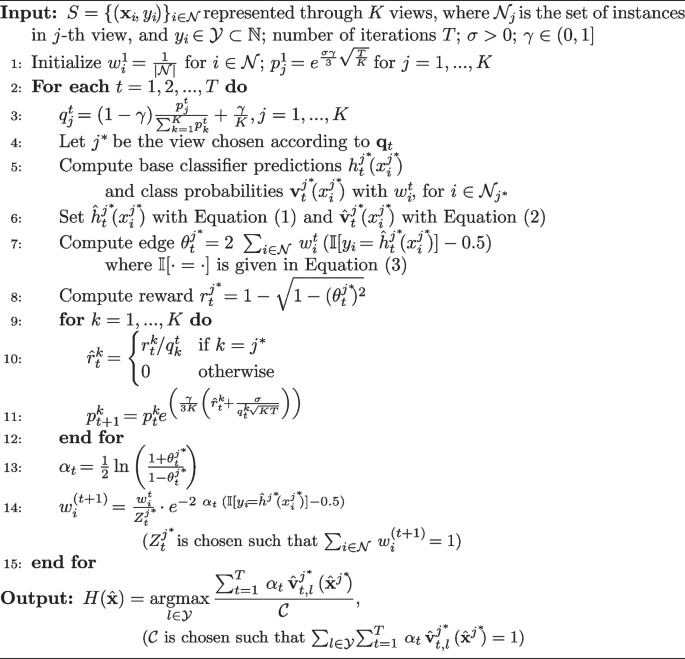
The proposed method irBoost.SH
Experimental setting
In this section, we describe the experimental setting of the evaluation we performed to assess the effectiveness of the proposed method irBoost.SH. As introduced at the end of section ‘Introduction’, we considered two different scenarios: i) the classification of individuals according to 16S and/or shotgun microbiome data, which represent two, possibly incomplete, different views; ii) the classification of individuals considering multiple preprocessing pipelines and hyper-parameter settings to generate multiple views from the same 16S data. For the former, we consider data related to the (binary) classification of individuals in terms of presence or absence of Autism Spectrum Disorders (ASD); for the latter, we consider 16S microbiome data related to ASD and 16S microbiome data for the (multi-class) classification of individuals according to the degree (or absence, in case of healthy individuals) of the Colorectal Cancer (CRC) disease.
In the following subsections, we first describe the contexts and the datasets; then we describe the pipelines followed to define the views; finally, we describe the evaluation setting and the considered competitors.
Autism spectrum disorder (ASD)
The Autism Spectrum Disorder (ASD) is a severe neurodevelopmental disorder that is primarily characterized by abnormal behavioral symptoms: social interaction impairment, stereotyped behavior, and restricted interests. Recent studies have shown a significant association between this disease and gut microbiome through the so-called microbiota-gut-brain axis [ 4 , 31 , 32 ].
The considered dataset is publicly available at the NCBI repository Footnote 1 and consists of a cohort of 143 children with clinical diagnosis of ASD, aged 2–13 years old, and age- and sex-matched typically developing (TD) individuals (average age \(5.189 \pm 0.170\) ; 127 males and 16 females), who attended annual physical examinations. 16S rRNA sequencing of feces samples was performed for all individuals, while shotgun metagenomic sequencing was performed only for 30 ASD and 30 TD individuals. We considered two multi-view versions of the dataset:
MV-ASD , that contains pre-computed 16S rRNA and shotgun Operational Taxonomic Unit (OTU) tables as available at the Kaggle repository, Footnote 2 where 16S data represent the first view and shotgun data represent the second view (see quantitative information in Table 1 Footnote 3 );
ASD-16S , that we constructed by downloading the raw 16S rRNA sequences from NCBI and by preprocessing them using the pipelines described in ‘ Data preprocessing pipelines ’ section, obtaining 40 different views for all children in the study (143 ASD + 143 TD).
It is noteworthy that MV-ASD is inherently incomplete: primarily, incompleteness comes from a much lower number of individuals represented through the shotgun view with respect to those represented through the 16S view; moreover, some 16S samples were discarded during the preprocessing of the dataset (see footnote 3). Therefore, in the dataset we have individuals that are represented with (i) only 16S features, (ii) only shotgun features, or (iii) both 16S and shotgun features. Accordingly, we defined three different experimental settings, that are summarized in Table 2 . The first setting ( MV-ASD-1 ) considers only individuals that are represented according to (at least) the 16S view: the representation according to the shotgun view is considered only for those individuals that also have a representation in the 16S view. The second setting ( MV-ASD-2 ) considers only individuals that are represented according to (at least) the shotgun view: the representation according to the 16S view is considered only for those individuals that also have a representation in the shotgun view. Finally, the third setting ( MV-ASD-3 ) only focuses on those individuals who are represented according to both 16S and shotgun views. Therefore, in this setting, views are complete.
Colorectal cancer (CRC)
Colorectal cancer (CRC) is the second leading cause of death among cancers in the USA [ 1 ]. Several studies suggest that gut microbiota may represent a reservoir of biomarkers that would complement existing non-invasive methods, such as the fecal immunochemical test (FIT) [ 1 , 2 , 3 ].
The considered dataset, hereafter called CRC , consists of OTU tables computed from 16S rRNA sequences for the prediction of the CRC condition between CRC (191 samples), Adenoma Footnote 4 (241 samples) or Control (277 healthy samples). Sequences were downloaded and merged from three repositories Footnote 5 \(^{,}\) Footnote 6 \(^{,}\) Footnote 7 selected according to the guidelines Footnote 8 produced within ML4Microbiome COST Action CA18131, Footnote 9 and preprocessed following the pipelines described in ‘ Data preprocessing pipelines ’ section, leading to a total of 40 different views.
Data preprocessing pipelines
Amplicon sequence data preprocessing was performed using QIIME 2 [ 33 ], version 2021.2. Forward sequences were denoised with DADA2 [ 34 ], truncated at 240 bp and closed-reference clustered against SILVA database by varying the similarity threshold in \(\{ 90\%, 94\%, 97\%, 99\% \}\) . Each of the produced OTU tables after clustering was than subjected to feature filtering, that has been performed according to the following criteria:
by frequency , i.e., on the minimum number of individuals for whom a given feature must be present, in \(\{ 2, 5, 10, 50 \}\) ;
by samples , i.e., on the minimum percentage of the individuals for whom a given feature must be present, in \(\{ 2\%, 5\%, 10\%, 20\%, 50\% \}\) .
Moreover, we also considered unfiltered OTU tables. In total, we obtained 40 different views, considering 4 different similarity thresholds in the clustering phase, multiplied by 10 different filtering configurations, i.e., 4 (filtering by frequency) + 5 (filtering by samples) + 1 (unfiltered).
For the ASD-16S dataset (‘Autism spectrum disorder (ASD)’ section), average reads quality scores were very good (higher than 30), except for one point in the region between 240 and 260 bp. This was the reason for truncating at 240bp in DADA2 denoising. On average, 88% of input ASD-16S sequences passed denosing and, in total 2517 of features were identified in Amplicon Sequence Variant (ASV) table, subsequently used for the clustering phase. The final number of features ranged from 219 to 1737, depending on the pipeline.
For the CRC dataset (‘Colorectal cancer (CRC)’ section), the quality of the paired-end sequences (both forward and reverse) was not particularly high (especially after 240bp). On average, around 70% of input sequences passed DADA2 denoising (trimmed at 240bp) and merging for paired-end reads. After denoising, 14055 ASV features were found, but this number was reduced by the clustering into OTUs and by the filtering step, leading to a final number of features ranging from 69 to 4402.
A complete overview of the workflow followed for sequence preprocessing is provided in the Fig. 2 .
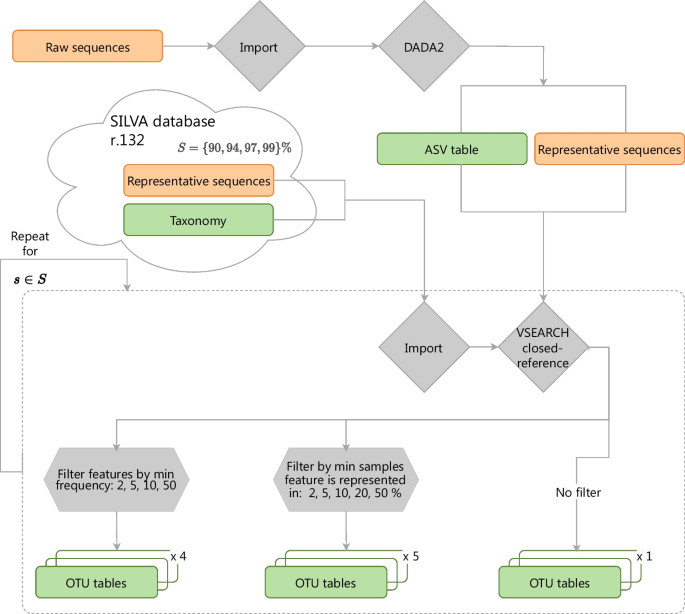
QIIME 2 data preprocessing pipeline
Model evaluation and comparison
For irBoost.SH, we set the parameters \(\sigma\) and \(\gamma\) as suggested in [ 12 ] for rBoost.SH, namely, \(\sigma =0.15\) and \(\gamma =0.3\) . As base classifiers we considered single decision trees (DT) and random forests (RF) with 50 trees. Since after 50 iterations the results appeared to be comparable, in the following, we only show those obtained through RF.
In order to actually assess the contribution provided by irBoost.SH in capturing the information conveyed by multiple views, we also performed the experiments with some competitor approaches. Specifically, we adopted the standard (single-view) version of RF, hereafter denoted with RF (SV) , for MV-ASD-1 and MV-ASD-2, as well as for all the 40 views of ASD-16S and CRC, that are considered separately. This comparison allows us to understand if the multi-view model learned by irBoost.SH outperforms all the models (2 in the case of MV-ASD-1 and MV-ASD-2, 40 in the case of ASD-16S and CRC) learned from a single view.
We also ran the experiments with the original version of rBoost.SH (hereafter denoted with rBoost.SH (MV) ), on all the considered datasets except for the CRC dataset, on which it cannot be run due to the presence of multiple classes. Moreover, to overcome the incompleteness of the views for MV-ASD-1 and MV-ASD-2, we adopted a standard approach based on filling missing values with the mean value observed for each feature. Finally, we ran a baseline multi-view version of RF, hereafter denoted with Concat-RF (MV) , where the features coming from all the views are concatenated; also in this case, to manage the possible incompleteness of the views, we replaced missing values with the mean value observed for each feature.
We want to remark that, although other base learners could have been considered in our experiments (either plugged into our framework or as standalone single-view systems), they were not included since the primary focus was on the assessment of the contribution provided by the multiple available (possibly incomplete) views, rather than on the comparison of the performance achieved through different base learners.
As the evaluation strategy we adopted 10-fold cross-validation. In the case of incomplete views, the partitioning was made such that each view is split into 10 equally-sized folds. The adopted performance measures are precision, recall, accuracy, F1-score and area under the ROC curve (AUC). Note that it was not possible to collect the AUC for rBoost.SH (MV) since it does neither return a probability distribution for labels nor a score/confidence, but directly the predicted label.
Results and discussion
In Table 3 , we show the results of the considered approaches on all the datasets in terms of all the evaluation measures, while in Fig. 3 we graphically summarize the results in terms of F1-scores. In Table 3 and Fig. 3 , RF (SV) Worst , RF (SV) Average and RF (SV) Best denote, respectively, the worst, the average and the best result achieved among all the constructed views, by the single-view Random Forest model. The whole set of results obtained by each single view is reported in Additional file 1 , while some confusion matrices are shown in Additional file 2 . In the following, we mainly discuss the results in terms of F1-scores, but similar conclusions can be drawn considering the other evaluation measures.
Focusing on the MV-ASD datasets, we can observe that simultaneously considering both 16S and shotgun views is generally beneficial, even if they are exploited through a simple concatenation strategy, as done by Concat-RF (MV). However, in this case, the improvement obtained by adding shotgun data about 60 individuals over 254 total individuals represented through 16S data (MV-ASD-1) led to a less sensible improvement with respect to adding 16S data about 56 individuals over 60 total individuals represented through shotgun data (MV-ASD-2). The results obtained by rBoost.SH exhibit this phenomenon in a much more evident manner: on MV-ASD-1, rBoost.SH obtains worse results than RF (SV), while on MV-ASD-2 it exhibits interesting improvements. This phenomenon is possibly due to the fact that the number of missing values (replaced by the mean value of each feature) is, in the case of MV-ASD-1, much higher than in MV-ASD-2. This is supported by the fact that on MV-ASD-3, where views are complete, rBoost.SH obtains results that are comparable with those achieved by our method. This observation confirms the effectiveness of irBoost.SH in handling the incompleteness of the views.
In summary, we achieved an improvement of 8% over RF (SV), 6.1% over Concat-RF (MV), and 10.8% over rBoost.SH on MV-ASD-1; an improvement of 30.5% over RF (SV), 15.5% over Concat-RF (MV), and 1.7% over rBoost.SH on MV-ASD-2; an improvement of 10% over Concat-RF (MV) and a tie with rBoost.SH on MV-ASD-3.
The exploitation of the complementarity of the information conveyed by 16S and shotgun views, performed by irBoost.SH, is confirmed by the fact that the 16S view was selected as the winner in 51% of the iterations, while the shotgun view was selected as the winner in 49% of the iterations, emphasizing an almost equal importance and contribution of the views.
Focusing on the results on the other datasets, we can observe that the selection of the preprocessing pipeline in a single-view setting influences the results. Indeed, for the ASD-16S dataset, the F1-score ranges from about 89% (in the worst case) to about 94% (in the best case), while for the CRC dataset, it ranges from about 35% (in the worst case) to 47% (in the best case). The multi-view approach based on the concatenation, i.e., Concat-RF (MV), always performed better than the worst single-view pipeline, but was not able to reach the best single-view configuration. On the contrary, irBoost.SH provided a significant boost, with irBoost.SH reaching a F1-score of more than 99% on ASD-16S and more than 95% on CRC, achieving an improvement over Concat-RF (MV) of 9% and 114%, respectively. rBoost.SH was able to obtain good results on ASD-16S, with a disadvantage of about 0.3% over our method irBoost.SH. Like MV-ASD-3, this dataset was naturally complete, therefore, in these scenarios, the only difference with respect to irBoost.SH is the different way they combine the output. On the other hand, as already stated in ‘Model evaluation and comparison’ section, rBoost.SH cannot be run at all on the CRC dataset since it is multi-class.
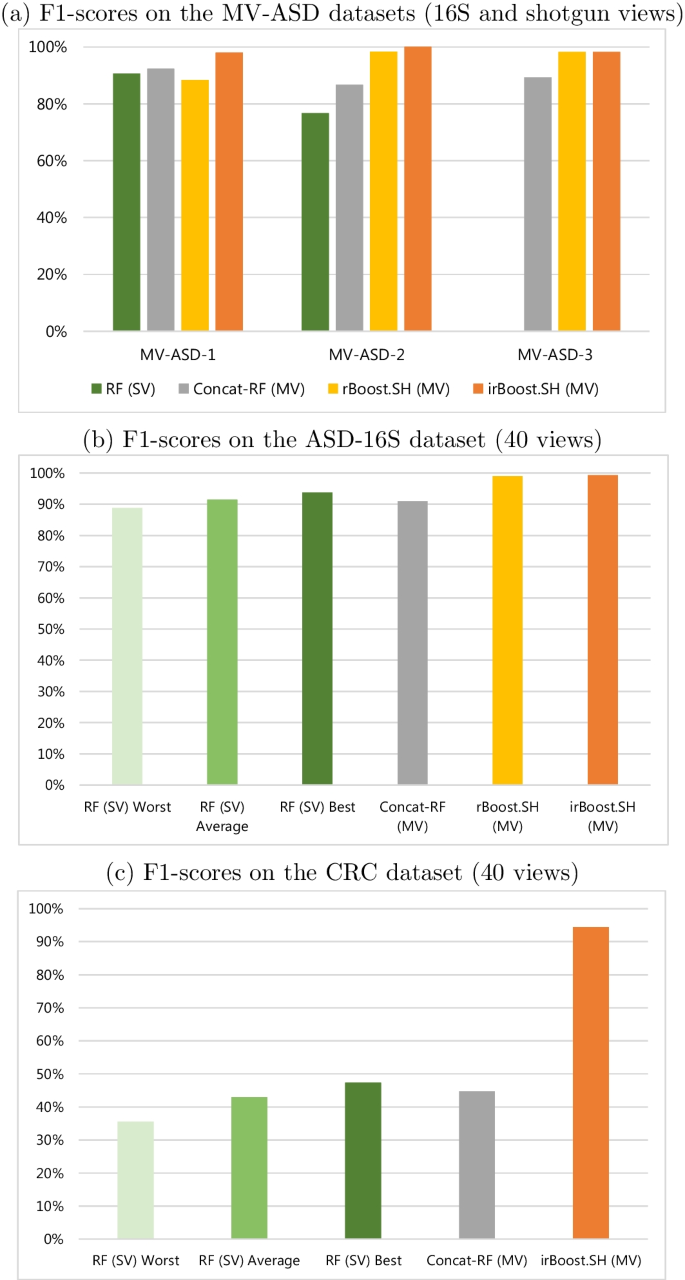
F1-scores obtained on all the analyzed datasets by irBoost.SH, and by competitor single-view (SV) and multi-view (MV) approaches
In order to further confirm the significance of the obtained results, we computed the following three Wilcoxon signed-rank tests:
irBoost.SH versus RF (SV) Best , on all the datasets except for MV-ASD-3, on which RF (SV) Best is not applicable;
irBoost.SH versus Concat-RF (MV) , on all the datasets;
irBoost.SH versus rBoost.SH (MV) , on all the datasets except for CRC, on which rBoost.SH (MV) cannot be run since it is multi-class.
Considering that we ran multiple tests, we corrected the obtained p values with the False Discovery Rate (FDR) correction proposed by Benjamini and Hochberg [ 35 ], obtaining the results reported in Table 4 . As it can be observed from the table, irBoost.SH outperforms all the considered competitors at a significance level \(\alpha = 0.01\) .
For the ASD-16S dataset, on average, each view built through a frequency-based filtering, sample-based filtering, and no filtering was selected as the winner in 2.8%, 2.3%, and 2.1% of the iterations, respectively. For the CRC dataset, on average, each view built through a frequency-based filtering, sample-based filtering, and no filtering was selected as the winner in 2.5%, 2.75%, and 2.44% of the iterations, respectively. An overview of the percentage of iterations for which each view was selected is provided in Additional file 3 .
The huge improvement in terms of all the evaluation measures provided by irBoost.SH, together with the (almost) equal distribution of the times each type of views was selected, confirms that the features constructed by multiple pipelines describe the phenomenon from different, complimentary, viewpoints, that are fruitfully captured by irBoost.SH.
In this paper, we proposed a novel method, called irBoost.SH, to solve multi-class classification tasks from multiple, possibly incomplete, views. Instead of discarding the information conveyed by incomplete views or filling in missing values, irBoost.SH exploits the available information of all the views, without introducing excessive approximation in the data, through a boosting process based on multi-armed bandits.
The proposed algorithm is motivated by the challenges that arise in the analysis of microbiome data, where the presence of multiple, possibly incomplete, views is very common. The obtained experimental results emphasized that the models learned by irBoost.SH were able to outperform all the models learned from each single view independently, as well as a baseline multi-view approach based on the concatenation of the features of all the views. The superiority of irBoost.SH was observed in two different tasks, i.e., in the prediction of the presence of Autism Spectrum Disorders (ASD) and in the prediction of the presence of the Colorectal Cancer (CRC) disease, where views were constructed considering 16S and shotgun data (2 views) or by applying several preprocessing pipelines (40 views). The obtained results confirmed that irBoost.SH can fruitfully be adopted for the analysis of microbiome data, to simultaneously exploit 16S and shotgun data, and to solve the issues coming from the identification of the most proper preprocessing pipeline, that in this case is fully automated.
As future work, we will adapt irBoost.SH to solve other tasks, such as regression and multi-target classification/regression. Moreover, we will investigate the possibility to make it able to work in the semi-supervised learning setting, where the class label is not available for all the training instances. Finally, we will evaluate the effectiveness of irBoost.SH in capturing the information conveyed by multiple, possibly incomplete, views also in other application domains.
Availability of data and materials
The datasets considered in this study for the prediction of the Autism Spectrum Disorder are available at the following links: https://www.ncbi.nlm.nih.gov/bioproject/?term=PRJNA453621 and https://www.kaggle.com/datasets/antaresnyc/human-gut-microbiome-with-asd . The datasets considered in this study for the prediction of the Colorectal Cancer Disease are available at the following links: https://www.ncbi.nlm.nih.gov/bioproject/PRJNA290926 , https://www.ncbi.nlm.nih.gov/bioproject/PRJEB6070 , and http://mothur.org/MicrobiomeBiomarkerCRC . All the datasets in the pre-processed form are publicly available at: https://figshare.com/collections/Processed_16S_rRNA_sequencing_multi-view_datasets/7066355 . The source code of the proposed method irBoost.SH is publicly available at: https://github.com/AndreaMSBios/Multi-class-boosting-for-the-analysis-of-multiple-incomplete-views-on-microbiome-data .
https://www.ncbi.nlm.nih.gov/bioproject/?term=PRJNA453621
https://www.kaggle.com/datasets/antaresnyc/human-gut-microbiome-with-asd
Note that the number of available TD individuals in the dataset available at the Kaggle repository is slightly less than the original dataset available at NCBI (28 missing TD individuals). This is possibly due to some preprocessing steps applied by the authors of the dataset available at the Kaggle repository.
A tumor that is not cancer. It starts in gland-like cells of the epithelial tissue (thin layer of tissue that covers organs, glands, and other structures within the body).
https://www.ncbi.nlm.nih.gov/bioproject/PRJNA290926 .
https://www.ncbi.nlm.nih.gov/bioproject/PRJEB6070 .
http://mothur.org/MicrobiomeBiomarkerCRC/ .
https://doi.org/10.5281/zenodo.7382814 .
https://www.cost.eu/actions/CA18131/ .
Baxter NT, Ruffin MT, Rogers MAM, Schloss PD. Microbiota-based model improves the sensitivity of fecal immunochemical test for detecting colonic lesions. Genome Med. 2016;8(1):37.
Article PubMed PubMed Central Google Scholar
...Zeller G, Tap J, Voigt AY, Sunagawa S, Kultima JR, Costea PI, Amiot A, Böhm J, Brunetti F, Habermann N, Hercog R, Koch M, Luciani A, Mende DR, Schneider MA, Schrotz-King P, Tournigand C, Nhieu JTV, Yamada T, Zimmermann J, Benes V, Kloor M, Ulrich CM, Doeberitz MK, Sobhani I, Bork P. Potential of fecal microbiota for early-stage detection of colorectal cancer. Mol Syst Biol. 2014;10:766.
Zackular JP, Rogers MA, Ruffin MT, Schloss PD. The human gut microbiome as a screening tool for colorectal cancer. Cancer Prev Res. 2014;7:1112–21.
Article CAS Google Scholar
Dan Z, Mao X, Liu Q, Guo M, Zhuang Y, Liu Z, Chen K, Chen J, Xu R, Tang J, Qin L, Gu B, Liu K, Su C, Zhang F, Xia Y, Hu Z, Liu X. Altered gut microbial profile is associated with abnormal metabolism activity of autism spectrum disorder. Gut Microbes. 2020;11:1246–67.
Article CAS PubMed PubMed Central Google Scholar
Rosenfeld CS. Microbiome disturbances and autism spectrum disorders. Drug Metab Dispos. 2015;43:1557–71.
Article CAS PubMed Google Scholar
Ranjan R, Rani A, Metwally A, McGee HS, Perkins DL. Analysis of the microbiome: advantages of whole genome shotgun versus 16s amplicon sequencing. Biochem Biophys Res Commun. 2016;469:967–77.
Mas-Lloret J, Obón-Santacana M, Ibáñez-Sanz G, Guinó E, Pato ML, Rodriguez-Moranta F, Mata A, García-Rodríguez A, Moreno V, Pimenoff VN. Gut microbiome diversity detected by high-coverage 16s and shotgun sequencing of paired stool and colon sample. Sci Data. 2020;7(1):92.
Shaffer JP, Carpenter CS, Martino C, Salido RA, Minich JJ, Bryant M, Sanders K, Schwartz T, Humphrey G, Swafford AD, Knight R. A comparison of six DNA extraction protocols for 16s, ITS and shotgun metagenomic sequencing of microbial communities. BioTechniques. 2022;73:34–46.
...Moreno-Indias I, Lahti L, Nedyalkova M, Elbere I, Roshchupkin G, Adilovic M, Aydemir O, Bakir-Gungor B, Santa Pau ECD, D’Elia D, Desai MS, Falquet L, Gundogdu A, Hron K, Klammsteiner T, Lopes MB, Marcos-Zambrano LJ, Marques C, Mason M, May P, Pašic L, Pio G, Pongor S, Promponas VJ, Przymus P, Saez-Rodriguez J, Sampri A, Shigdel R, Stres B, Suharoschi R, Truu J, Truica CO, Vilne B, Vlachakis D, Yilmaz E, Zeller G, Zomer AL, Gómez-Cabrero D, Claesson MJ. Statistical and machine learning techniques in human microbiome studies: contemporary challenges and solutions. Front Microbiol. 2021. https://doi.org/10.3389/fmicb.2021.635781 .
...D’Elia D, Truu J, Lahti L, Berland M, Papoutsoglou G, Ceci M, Zomer A, Lopes MB, Ibrahimi E, Gruca A, Nechyporenko A, Frohme M, Klammsteiner T, Pau ECDS, Marcos-Zambrano LJ, Hron K, Pio G, Simeon A, Suharoschi R, Moreno-Indias I, Temko A, Nedyalkova M, Apostol ES, Truica CO, Shigdel R, Telalovic JH, Bongcam-Rudloff E, Przymus P, Jordamovic NB, Falquet L, Tarazona S, Sampri A, Isola G, Pérez-Serrano D, Trajkovik V, Klucar L, Loncar-Turukalo T, Havulinna AS, Jansen C, Bertelsen RJ, Claesson MJ. Advancing microbiome research with machine learning: key findings from the ml4microbiome cost action. Front Microbiol. 2023. https://doi.org/10.3389/fmicb.2023.1257002 .
...Marcos-Zambrano LJ, López-Molina VM, Bakir-Gungor B, Frohme M, Karaduzovic-Hadziabdic K, Klammsteiner T, Ibrahimi E, Lahti L, Loncar-Turukalo T, Dhamo X, Simeon A, Nechyporenko A, Pio G, Przymus P, Sampri A, Trajkovik V, Lacruz-Pleguezuelos B, Aasmets O, Araujo R, Anagnostopoulos I, Aydemir O, Berland M, Calle ML, Ceci M, Duman H, Gündogdu A, Havulinna AS, Kaka Bra KHN, Kalluci E, Karav S, Lode D, Lopes MB, May P, Nap B, Nedyalkova M, Paciência I, Pasic L, Pujolassos M, Shigdel R, Susín A, Thiele I, Truica CO, Wilmes P, Yilmaz E, Yousef M, Claesson MJ, Truu J, de Santa Pau EC. A toolbox of machine learning software to support microbiome analysis. Front Microbiol. 2023. https://doi.org/10.3389/fmicb.2023.1250806 .
Peng J, Aved AJ, Seetharaman G, Palaniappan K. Multiview boosting with information propagation for classification. IEEE Trans Neural Netw Learn Syst. 2018;29:657–69.
Article PubMed Google Scholar
Nguyen ND, Wang D. Multiview learning for understanding functional multiomics. PLOS Comput Biol. 2020;16: e1007677.
Yang M, Li Y, Hu P, Bai J, Lv J, Peng X. Robust multi-view clustering with incomplete information. IEEE Trans Pattern Anal Mach Intell. 2023;45(1):1055–69.
Blum A, Mitchell T, in Proc. of the 11th annual conference on Computational learning theory - COLT’ 98 (ACM Press, 1998), pp. 92–100
Sindhwani V, Niyogi P, Belkin M. In: Proceedings of the workshop on learning with multiple views, 22nd (ICML, Bonn, 2005)
Wang J, Luo S, Li Y. Advances in Neural Networks - ISNN 2010. Berlin: Springer; 2010. p. 444–9.
Book Google Scholar
Ceci M, Pio G, Kuzmanovski V, Džeroski S. Semi-supervised multi-view learning for gene network reconstruction. PLoS ONE. 2015;10(12):1–27.
Article Google Scholar
Xu Z, Sun S. Neural information processing. Theory and algorithms. Berlin: Springer; 2010. p. 355–62.
Freund Y, Schapire RE. Lecture notes in computer science. Berlin: Springer; 1995. p. 23–37.
Google Scholar
Busa-Fekete R, Kegl B. In: Proceedings of the 27th international conference on machine learning, Haifa, Israel. 2010, pp 143–150
Hà Quang M, Bazzani L, Murino V. In: Proceedings of the 30th international conference on machine learning. 2013; vol 28, pp 100–108
Xu X, Li W, Xu D, Tsang IW. Co-labeling for multi-view weakly labeled learning. IEEE Trans Pattern Anal Mach Intell. 2016;38:1113–25.
Koco S, Capponi C. Machine learning and knowledge discovery in databases. Berlin: Springer; 2011. p. 209–28.
Xu C, Tao D, Xu C. Multi-view learning with incomplete views. IEEE Trans Image Process. 2015;24:5812–25.
Zhu P, Yao X, Wang Y, Cao M, Hui B, Zhao S, Hu Q, Latent heterogeneous graph network for incomplete multi-view learning. IEEE Trans Multimed. 2022.
Peng J, Barbu C, Seetharaman G, Fan W, Wu X, Palaniappan K. Machine learning and knowledge discovery in databases. Berlin: Springer; 2011. p. 597–612.
Peter Auer YF, Cesa-Bianchi Nicolo, Schapire RE, Gambling in a rigged casino:the adversarial multi-armed bandit problem. Tech. rep., University of Technology Graz/Universita di Milano/AT &T Labs, Florham Park, NJ, 1998
Auer P, Cesa-Bianchi N, Freund Y, Schapire RE. The nonstochastic multiarmed bandit problem. SIAM J Comput. 2002;32:48–77.
Cesa-Bianchi N. Prediction, learning, and games. London: Cambridge University Press; 2010.
Liu Z, Mao X, Dan Z, Pei Y, Xu R, Guo M, Liu K, Zhang F, Chen J, Su C, Zhuang Y, Tang J, Xia Y, Qin L, Hu Z, Liu X. Gene variations in autism spectrum disorder are associated with alternation of gut microbiota, metabolites and cytokines. Gut Microbes. 2021;13:1854967.
Pulikkan J, Mazumder A, Grace T. Advances in experimental medicine and biology. Berlin: Springer; 2019. p. 253–69.
...Bolyen E, Rideout JR, Dillon MR, Bokulich NA, Abnet CC, Al-Ghalith GA, Alexander H, Alm EJ, Arumugam M, Asnicar F, Bai Y, Bisanz JE, Bittinger K, Brejnrod A, Brislawn CJ, Brown CT, Callahan BJ, Caraballo-Rodríguez AM, Chase J, Cope EK, Silva RD, Diener C, Dorrestein PC, Douglas GM, Durall DM, Duvallet C, Edwardson CF, Ernst M, Estaki M, Fouquier J, Gauglitz JM, Gibbons SM, Gibson DL, Gonzalez A, Gorlick K, Guo J, Hillmann B, Holmes S, Holste H, Huttenhower C, Huttley GA, Janssen S, Jarmusch AK, Jiang L, Kaehler BD, Kang KB, Keefe CR, Keim P, Kelley ST, Knights D, Koester I, Kosciolek T, Kreps J, Langille MGI, Lee J, Ley R, Liu YX, Loftfield E, Lozupone C, Maher M, Marotz C, Martin BD, McDonald D, McIver LJ, Melnik AV, Metcalf JL, Morgan SC, Morton JT, Naimey AT, Navas-Molina JA, Nothias LF, Orchanian SB, Pearson T, Peoples SL, Petras D, Preuss ML, Pruesse E, Rasmussen LB, Rivers A, Robeson MS, Rosenthal P, Segata N, Shaffer M, Shiffer A, Sinha R, Song SJ, Spear JR, Swafford AD, Thompson LR, Torres PJ, Trinh P, Tripathi A, Turnbaugh PJ, Ul-Hasan S, van der Hooft JJJ, Vargas F, Vázquez-Baeza Y, Vogtmann E, von Hippel M, Walters W, Wan Y, Wang M, Warren J, Weber KC, Williamson CHD, Willis AD, Xu ZZ, Zaneveld JR, Zhang Y, Zhu Q, Knight R, Caporaso JG. Reproducible, interactive, scalable and extensible microbiome data science using QIIME 2. Nat Biotechnol. 2019;37:852–7.
Callahan BJ, McMurdie PJ, Rosen MJ, Han AW, Johnson AJA, Holmes SP. DADA2: high-resolution sample inference from illumina amplicon data. Nat Methods. 2016;13:581–3.
Benjamini Y, Hochberg Y. Controlling the false discovery rate: a practical and powerful approach to multiple testing. J R Stat Soc Ser B. 1995;57(1):289–300.
Download references
Acknowledgements
The research on the topic started during Short-term Scientific Mission (STSM) within ML4Microbiome COST Action. The first author wishes to thank Gianvito Pio, PhD for his warm patience and immense help during her visit to Bari, Italy, and for the support during the whole process concluding with this paper. This endeavor would not be possible without Tatjana Lončar-Turukalo, PhD and Michelangelo Ceci, PhD. Warmest thanks to Sanja Brdar, PhD and Miloš Radovanović, PhD for continuous support throughout all these years.
This publication is based upon work from COST Action CA18131 “Statistical and machine learning techniques in human microbiome studies”, supported by COST (European Cooperation in Science and Technology). This work was also partially supported by the following projects under the NRRP MUR program funded by the NextGenerationEU: Future AI Research (PE00000013), Spoke 6 - Symbiotic AI; PRIN 2022 "BA-PHERD: Big Data Analytics Pipeline for the Identification of Heterogeneous Extracellular non-coding RNAs as Disease Biomarkers", grant n. 2022XABBMA, CUP: H53D23003690006; CN3 RNA - "National Center for Gene Therapy and Drugs based on RNA Technology", CUP: H93C22000430007.
Author information
Andrea Simeon and Gianvito Pio have contributed equally to this work.
Authors and Affiliations
BioSense Institute, University of Novi Sad, dr Zorana Djindjića 1, Novi Sad, 21000, Serbia
Andrea Simeon & Sanja Brdar
Faculty of Sciences, University of Novi Sad, Trg Dositeja Obradovića 3, Novi Sad, 21000, Serbia
Miloš Radovanović
Faculty of Technical Sciences, University of Novi Sad, Trg Dositeja Obradovića 6, Novi Sad, 21000, Serbia
Tatjana Lončar-Turukalo
Department of Computer Science, University Bari Aldo Moro, Via Orabona 4, 70125, Bari, Italy
Michelangelo Ceci & Gianvito Pio
Big Data Laboratory, National Interuniversity Consortium for Informatics (CINI), Via Ariosto 25, 00185, Rome, Italy
Department of Knowledge Technologies, Jožef Stefan Institute, Jamova cesta 39, 1000, Ljubljana, Slovenia
Michelangelo Ceci
You can also search for this author in PubMed Google Scholar
Contributions
AS and GP conceived the task and designed the solution from a methodological point of view. AS, SB and TLT developed the workflow for microbiome data preprocessing. AS implemented the model, ran the experiments and collected the results. AS, GP, MC and MR interpreted and discussed the results. GP, MC and MR supervised the whole research. All the authors contributed to the manuscript drafting and approved the final version of the manuscript.
Corresponding authors
Correspondence to Andrea Simeon or Gianvito Pio .
Ethics declarations
Ethics approval and consent to participate.
The data used in this study was publicly available for research purposes and collected during previous studies. Prior consent to the use of such data for research purposes was already collected in the original studies.
Consent for publication
Not applicable.
Competing interests
The authors declare that they have no conflict of interest.
Additional information
Publisher's note.
Springer Nature remains neutral with regard to jurisdictional claims in published maps and institutional affiliations.
Supplementary information
Additional file 1..
Full set of results. Description: Complete results in terms of precision, recall, F1-score, accuracy and AUROC for all the considered datasets and classifiers
Additional file 2.
Confusion matrices. Description: Confusion matrices for all the considered datasets and classifiers
Additional file 3.
Selected views. Description: Overview of the percentage of chosen views over iterations in irBoost.SH for all the considered datasets
Rights and permissions
Open Access This article is licensed under a Creative Commons Attribution 4.0 International License, which permits use, sharing, adaptation, distribution and reproduction in any medium or format, as long as you give appropriate credit to the original author(s) and the source, provide a link to the Creative Commons licence, and indicate if changes were made. The images or other third party material in this article are included in the article's Creative Commons licence, unless indicated otherwise in a credit line to the material. If material is not included in the article's Creative Commons licence and your intended use is not permitted by statutory regulation or exceeds the permitted use, you will need to obtain permission directly from the copyright holder. To view a copy of this licence, visit http://creativecommons.org/licenses/by/4.0/ . The Creative Commons Public Domain Dedication waiver ( http://creativecommons.org/publicdomain/zero/1.0/ ) applies to the data made available in this article, unless otherwise stated in a credit line to the data.
Reprints and permissions
About this article
Cite this article.
Simeon, A., Radovanović, M., Lončar-Turukalo, T. et al. Multi-class boosting for the analysis of multiple incomplete views on microbiome data. BMC Bioinformatics 25 , 188 (2024). https://doi.org/10.1186/s12859-024-05767-w
Download citation
Received : 16 August 2023
Accepted : 04 April 2024
Published : 14 May 2024
DOI : https://doi.org/10.1186/s12859-024-05767-w
Share this article
Anyone you share the following link with will be able to read this content:
Sorry, a shareable link is not currently available for this article.
Provided by the Springer Nature SharedIt content-sharing initiative
- Multi-view learning
- Incomplete views
- Multi-armed bandits
- Microbiome analysis
BMC Bioinformatics
ISSN: 1471-2105
- General enquiries: [email protected]
Thank you for visiting nature.com. You are using a browser version with limited support for CSS. To obtain the best experience, we recommend you use a more up to date browser (or turn off compatibility mode in Internet Explorer). In the meantime, to ensure continued support, we are displaying the site without styles and JavaScript.
- View all journals
- My Account Login
- Explore content
- About the journal
- Publish with us
- Sign up for alerts
- Technical Report
- Open access
- Published: 06 May 2024
Dimensionality reduction beyond neural subspaces with slice tensor component analysis
- Arthur Pellegrino ORCID: orcid.org/0009-0007-1849-575X 1 , 2 na1 ,
- Heike Stein ORCID: orcid.org/0000-0002-0871-6076 1 na1 &
- N. Alex Cayco-Gajic ORCID: orcid.org/0000-0003-3593-5773 1
Nature Neuroscience ( 2024 ) Cite this article
6604 Accesses
38 Altmetric
Metrics details
- Neural circuits
- Neural decoding
Recent work has argued that large-scale neural recordings are often well described by patterns of coactivation across neurons. Yet the view that neural variability is constrained to a fixed, low-dimensional subspace may overlook higher-dimensional structure, including stereotyped neural sequences or slowly evolving latent spaces. Here we argue that task-relevant variability in neural data can also cofluctuate over trials or time, defining distinct ‘covariability classes’ that may co-occur within the same dataset. To demix these covariability classes, we develop sliceTCA (slice tensor component analysis), a new unsupervised dimensionality reduction method for neural data tensors. In three example datasets, including motor cortical activity during a classic reaching task in primates and recent multiregion recordings in mice, we show that sliceTCA can capture more task-relevant structure in neural data using fewer components than traditional methods. Overall, our theoretical framework extends the classic view of low-dimensional population activity by incorporating additional classes of latent variables capturing higher-dimensional structure.
Similar content being viewed by others
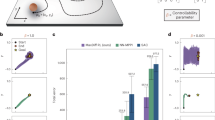
Maximum diffusion reinforcement learning
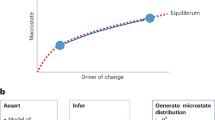
Entropy, irreversibility and inference at the foundations of statistical physics
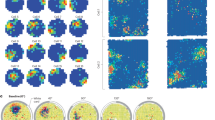
Remapping revisited: how the hippocampus represents different spaces
Neural activity varies in relation to fluctuations in the environment, changes in synaptic input, learning or adaptation, and heterogeneous cell properties, creating variability across neurons, time and trials. Recent work has emphasized that trial-to-trial variability is often correlated across populations of neurons 1 , generating low-dimensional representations of sensory or behavioral variables. Indeed, analyzing the structure of neural covariances has led to key insights into the information encoded and computations performed by neural circuits 2 , 3 . Such findings have driven an increase in the popularity of dimensionality reduction methods (for example, principal component analysis (PCA)), which seek to capture structure in neural data by identifying population-wide patterns of covariance. More recent work has advocated instead for applying tensor-based methods (for example, tensor component analysis (TCA)) that distinguish between changes in neural trajectories that occur over fast (within-trial) and slow (between-trial) timescales 4 , 5 , 6 . In these approaches, neural activity is assumed to be constrained to a low-dimensional neural subspace (defined by a set of latent variables) that is fixed over the course of an experiment.
However, this picture of low-dimensional latent variables fails to account for some forms of structure in neural datasets. First, not all population activity is described by covariance patterns across neurons. For example, many brain areas produce temporal sequences in which the latency of activation varies from neuron to neuron but that are highly stereotyped across conditions 7 , 8 , 9 , 10 , 11 . Second, the neural encoding weights for a given sensory stimulus may change over trials due to adaptation, learning 12 , 13 or representational drift 14 , 15 , 16 . These examples demonstrate three different types (or ‘classes’) of ‘covariability’, by which we mean structure in neural population recordings that can be described by stereotyped patterns across neurons, trials or time. Yet, because common neural dimensionality reduction methods typically look for covarying population-wide patterns, they may miss these additional forms of covariability in neural data.
Here, we propose that neural circuits are likely to encode task-relevant information in multiple co-occurring covariability classes. To demonstrate this, we introduce sliceTCA, a new unsupervised dimensionality reduction method able to identify and disentangle components belonging to different covariability classes that are mixed within the same dataset. This property contrasts sliceTCA from matrix factorization methods (such as PCA), which capture a single covariability class at a time, and from TCA, which identifies components constrained to all of them simultaneously. As a result, we show that sliceTCA can capture more structure in fewer components than either of these methods. Based on theoretical and practical considerations of the sliceTCA decomposition, we develop an analysis pipeline for model selection, optimization and visualization that is implemented in a readily applicable Python library.
After validating our method on simulated data, we illustrate the advantages of the mixed covariability framework in three large-scale neural datasets. First, we demonstrate that different covariability classes encode distinct behaviorally relevant signals in motor cortical recordings in nonhuman primates 17 . Next, in simultaneous imaging data from cortical and cerebellar populations during a cued motor task 18 , we show that sliceTCA uncovers task-relevant manifolds by considering covariability across trials. Finally, we analyze a recent dataset from the International Brain Laboratory (IBL) 19 and show that sliceTCA disentangles region-specific covariability classes across the visual cortex, hippocampus, thalamus and midbrain. We then provide a geometric intuition for how neural population activity is shaped by latent variables belonging to the three different covariability classes. Together, these results demonstrate the necessity of extending the traditional view of latent variables and neural covariability to uncover higher-dimensional latent structure. With sliceTCA, we propose a new unsupervised dimensionality reduction method that uncovers coexisting classes of behaviorally relevant covariability in neural datasets.
Multiple covariability classes
Neural activity often displays correlated fluctuations 1 . This form of covariability in neural data is usually determined by the neuron-by-neuron covariance matrix. Classic methods such as PCA capture the neural covariance matrix to identify characteristic patterns of neural weights whose time course of activation can vary freely from trial to trial (Fig. 1a ). Each of these patterns is represented by a different component. However, there are other forms of structure in population activity that are not captured by the neural covariance matrix and that would be discarded within this framework. Heterogeneous latencies or timescales in different neurons (Fig. 1b ) have been widely reported across brain regions, including in neural sequences 7 , 8 , 9 , 10 , 11 . Such temporal patterns are often characteristic for trials of the same task condition. Hence, such patterns represent a distinct kind of covariability in neural data, in which population activity covaries over trials, whereas the time courses of activation are heterogeneous across neurons (Fig. 1b and Extended Data Fig. 1 ).
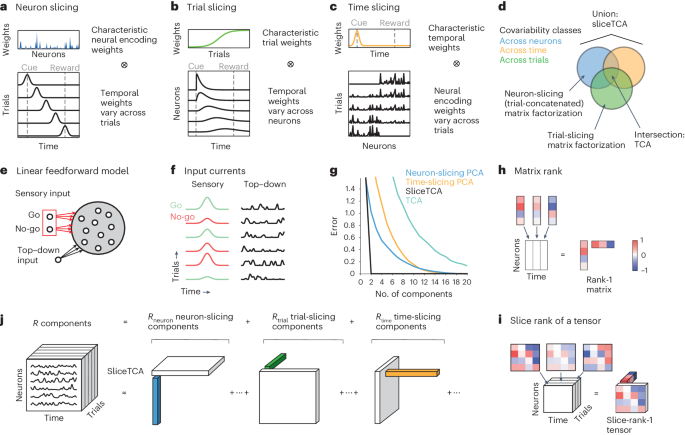
a , Example of a latent variable that represents a fixed neural encoding but whose temporal profile changes from trial to trial. b , Example of a latent variable that scales in amplitude over trials but has a neuron-specific time course within a trial. c , Example of a latent variable with a characteristic temporal profile within a trial but whose neural encoding weights change over trials. d , Schematic of the three covariability classes captured by sliceTCA. Matrix factorization methods such as PCA capture only a single covariability class at a time depending on how the data tensor is unfolded into matrix form. Because TCA treats neurons, trials and time symmetrically, it requires each component to lie in the intersection of the three classes. In contrast, sliceTCA represents the union of these three classes. e , Toy model of perceptual learning during a go/no-go task. On each trial, a population of linear neurons receives (1) a sensory input from one of two upstream sources representing the go/no-go stimuli and (2) top–down modulation representing stimulus-independent factors. Red indicates plastic weights. f , Evolution of inputs over trials. Go/no-go inputs increase/decrease in strength over trials due to synaptic potentiation/depression, whereas top–down inputs vary from trial to trial but are nonplastic. g , Error as a function of the number of components for different methods. h , Schematic of a rank-1 matrix. Each column of the matrix is a scaled version of the same vector. Equivalently, the matrix can be written as the outer product of that same column vector and a row vector representing the scaling weights. i , Schematic of a slice-rank-1 tensor. Each ‘slice’ of the tensor is a scaled version of the same matrix. The tensor can be written as an outer product of that matrix (a ‘slice’) and a vector representing the scaling weights. j , Schematic illustrating that sliceTCA approximates the data tensor as a low-slice-rank approximation. Each component is a slice-rank-1 tensor, which can be one of three types: neuron slicing, trial slicing or time slicing, corresponding to the examples in a – c .
These two examples illustrate that population covariability falls into multiple classes, which we call ‘neural covariability’ and ‘trial covariability’. Similar to neural covariability, trial covariability can be analyzed using the trial-by-trial covariance matrix, each element of which describes the similarity between the time courses of the full population response on two distinct trials. Indeed, using this approach, previous work has argued that different cortical regions are better described by trial covariability or neural covariability 7 . An additional form of structure in population activity might follow a characteristic temporal profile (say, locked to the time of stimulus presentation), whereas its neural encoding profile might change from trial to trial (Fig. 1c ) due to adaptation or representational drift 12 , 14 , 15 , 16 . This gives rise to a third covariability class corresponding to ‘temporal covariability’, which is captured by the time-by-time covariance matrix.
In this Article, we argue that neural population activity is likely to exhibit multiple covariability classes that are intermixed (Fig. 1d ). To provide intuition on how mixed covariability could arise at the level of neural circuits, we first built a toy feedforward model of the sensory cortex during a go/no-go task (Fig. 1e ). In this model, a population of linear cortical neurons received two sources of input in the context of a go/no-go task (Fig. 1f and Extended Data Fig. 2 ). First, all neurons received a sensory input that was time-locked to the stimulus. The projection weights were stimulus specific (either go or no-go) and plastic (potentiation/depression for go/no-go stimuli, respectively), in line with enhanced sensitivity to target stimuli in the sensory cortex during perceptual learning 20 , 21 . Potentiation and depression rates were stochastic and heterogeneous across neurons (Extended Data Fig. 2b,c and Methods ). Second, all neurons also received a top–down modulatory input unrelated to task events, for example, due to temporal fluctuations of arousal 22 . In this linear model, each neuron’s activity is simply the summation of its sensory and top–down input currents (Fig. 1f ).
From these minimal assumptions, the two input sources represent two different classes of covariability. First, stimulus-locked sensory inputs share the same characteristic temporal profile, with neural encoding weights that vary over neurons and trials due to heterogeneity in potentiation and depression rates. This type of structure corresponds to time covariability (Fig. 1c ). In contrast, the top–down input is fed through a static pattern of neural weights (as these synapses are nonplastic) but fluctuates in strength over time and trials due to variability in the modulatory signals. Therefore, this second type of structure falls into the class of neural covariability (Fig. 1a ). In principle, the resulting population activity can be described by two components capturing the sensory and top–down inputs ( Methods ). Yet, despite the simplicity of this model, PCA, which relies on a single covariability class, requires many components to capture the resulting population activity (Fig. 1g ). This toy model illustrates how mixed covariability classes can emerge from minimal assumptions regarding different sources of heterogeneity in neural circuits and that they cannot be disentangled using traditional dimensionality reduction methods.
SliceTCA disentangles mixed covariability
To disentangle mixed covariability in neural data, we must first return to the mathematical formulation of PCA. Matrix factorization methods, including PCA and non-negative matrix factorization (NMF), approximate a data matrix X as a sum of R components:
In neuroscience, X is generally a matrix of size N × K T containing the activity of N neurons recorded over K trials, each containing T time points. Each component X ( r ) is a rank-1 matrix defined by a vector of neural weights describing different activation patterns across the population and a vector of temporal weights describing how the strength of these patterns changes in amplitude over the course of the experiment (Fig. 1h ). Through this low-rank constraint, these methods are typically used to capture dominant patterns of neural covariability.
However, arranging neural data into matrix form limits the structure that can be captured, as matrix factorizations do not distinguish between rapid fluctuations within a trial and slower variations across trials 4 . This limitation can be addressed by structuring the data into an N × T × K tensor, which can be similarly decomposed following equation ( 1 ) into a low-rank tensor approximation. For this, we must generalize the concept of a rank-1 matrix to tensors. Different definitions of the tensor rank will capture different forms of structure in the data.
Here, we present sliceTCA, a new tensor decomposition method based on the slice rank 23 ( Methods ). A rank-1 matrix is defined as the outer product of two vectors so that each column of the matrix is a scaled version of the same column vector (Fig. 1h ). Similarly, a slice-rank-1 tensor is defined as the outer product of a vector and a matrix (or ‘slice’; Fig. 1i ). Depending on how the tensor is sliced, these components can capture any of the three covariability classes.
To see this, we may consider each slice type separately. First, a neuron-slicing component is described by a vector of characteristic neural weights and a matrix describing the time course for that component over trials (Fig. 1a ). This is the same class of neural covariability captured by common applications of matrix factorizations in which the data tensor is reshaped or ‘unfolded’ into an N × K T matrix (sometimes referred to as ‘trial-concatenated’ matrix factorization; Extended Data Fig. 1a ). Similarly, the trial-slicing components capture trial covariability: stereotyped neuron-specific temporal profiles that vary together in amplitude over trials (Fig. 1b ). Meanwhile, the time-slicing components identify time covariability: a common temporal profile whose neural encoding weights change from trial to trial, for example, due to learning, adaptation or drift (Fig. 1c ).
If only one of these three slice types were fitted, sliceTCA would be equivalent to a matrix factorization on the respective unfolding of the data tensor (Extended Data Fig. 1a–c ). Indeed, previous work has argued for performing PCA on different unfoldings of the data tensor to identify the slice type that provides the best approximation 7 . Crucially, sliceTCA differs from this approach by fitting all three slice types simultaneously, thereby demixing different covariability classes that may be combined within the same dataset (Fig. 1j ). SliceTCA is also related to, yet distinct from, TCA (that is, the CP (canonical polyadic) decomposition) 4 , 5 , 6 . TCA constrains each component to be described by the outer product of three vectors of neural, trial and temporal factors, which requires that each component lies in the intersection of all three covariability classes (Fig. 1d and Methods ). To demonstrate the conceptual difference between these methods, we applied sliceTCA to our toy model (Fig. 1e ). Indeed, sliceTCA was able to decompose the activity into its two ground-truth components (Extended Data Fig. 2c ), whereas PCA and TCA required substantially more components to capture the data (Fig. 1g ). SliceTCA also outperformed PCA and TCA in the presence of noise (Extended Data Fig. 2d,e and Supplementary Fig. 1 ). These results demonstrate that, by disentangling mixed covariability classes, sliceTCA is able to capture more structure in the data with fewer components as compared to other methods.
While we were able to explore neural and temporal covariability in the feedforward model, it neglected trial covariability, which can capture temporally rich population dynamics such as neural sequences. Toward this end, we built a linear recurrent neural network (RNN) model to generate high-dimensional, condition-specific sequences 24 while additionally integrating low-dimensional, condition-independent inputs ( Methods and Extended Data Fig. 3a–c ). As designed, the RNN activity could be decomposed into a few trial-slicing components corresponding to the embedded sequences and a few neural-slicing components corresponding to the inputs (Extended Data Fig. 3d ). Through this model, we were able to systematically examine the effects of three different sources of noise: low-dimensional input noise, intrinsic noise in the circuit dynamics and observation noise ( Methods ). As expected, sliceTCA was able to achieve near-optimal denoising of observation noise (Extended Data Fig. 3g ). Conversely, variability in the RNN activity coming from input noise was entirely retained by sliceTCA; this is because any variability in the inputs pushes the activity along a low-dimensional subspace determined by the input projection. Finally, sliceTCA performed well for intrinsic noise with a tendency to overfit for higher noise levels. These results clarify the robustness of sliceTCA to different sources and amounts of noise and provide insight into the relationship between the slice rank and neural circuit dynamics.
Task-relevant information is distributed across slice types
Based on the results of our toy model, we predicted that different slice types could capture different kinds of behaviorally relevant signals in neural data. We tested this hypothesis in a dataset comprising population recordings of the primary motor cortex (M1) and dorsal premotor cortex (PMd) during maze reaching and classic center-out (no-maze) reaching tasks (Fig. 2a , hand position). To quantify decoding performance, we linearly mapped population activity onto hand velocity ( Methods ). As a benchmark, we first mapped trial-averaged raw neural data onto kinematic trajectories, revealing a close match between behavior and neural activity (Fig. 2a , trial-averaged raw data). However, when we attempted to decode hand trajectories based on individual trials, we observed considerable trial-to-trial variability that corresponded poorly to kinematic data (Fig. 2a , raw data).
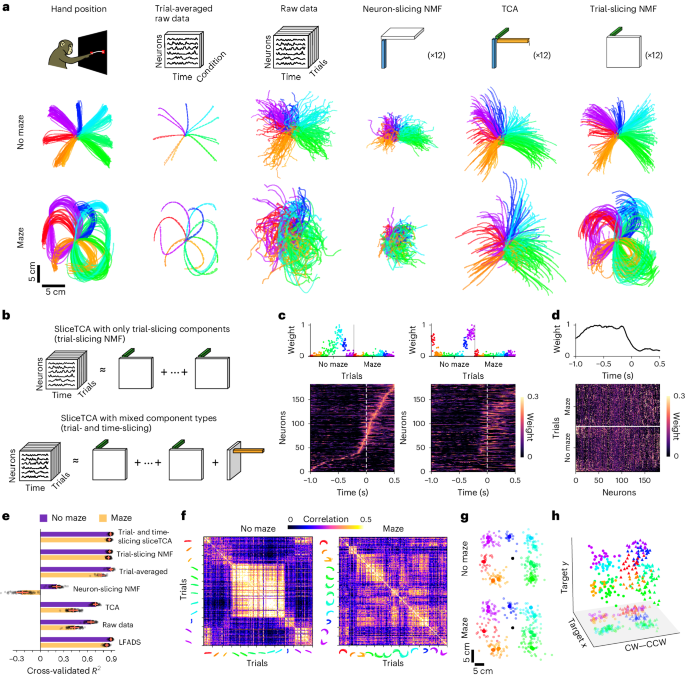
a , Behavioral and motor cortical trajectories ( n = 182 neurons from M1 and PMd) during a classic center-out reaching task with straight reaches (top) and curved maze reaches (bottom; modified from ref. 52 ). Different colors indicate different reach directions. Hand position: hand positions during the experiment. Trial-averaged raw data: condition-wise trial-averaged reaches (dashed lines) versus neural population activity (solid lines), projected onto the two-dimensional (2D) subspace that best matches hand trajectories. Raw data: raw population activity mapped onto hand trajectories at single-trial resolution. Neuron-slicing NMF: denoised population activity mapped onto hand trajectories (neuron-slicing NMF, 12 components; equivalent to NMF performed on the trial-concatenated data matrix). TCA: denoised population activity (TCA, 12 components) mapped onto hand trajectories. Trial-slicing NMF: denoised population activity (trial-slicing NMF, 12 components) mapped onto hand trajectories. b , Schematic of a sliceTCA model with multiple components of the same slice type versus a model with mixed slice types. c , Two example trial-slicing components, with neurons ordered by peak activation times of the first component. Sequential patterns distinguish specific reach conditions (here, upper left versus upper right straight reaches). d , The single time-slicing component, which displays a high temporal weight preceding movement onset. Condition-specific neural weights are shown in the slice. e , R 2 of fivefold cross-validated velocity decoding in each model (error bars represent the s.e.m. over n = 49 and n = 53 test trials for the maze and no-maze conditions, respectively, averaged over a fivefold cross-validation of 20 permutations of the trials). f , Correlations between neural weights on the time-slicing component in the PMd. Correlations were high for pairs of trials with similar reach direction and curvature and low for dissimilar reaches. g , Mapping of average activity in the time-slicing component before movement onset (from 0.75 to 0 s before onset) onto reach targets, revealing a strong association ( R 2 = 0.95 and R 2 = 0.91, center-out versus curved reaches). h , Partially reconstructed activity from the time-slicing component, projected into a 3D subspace identified to maximally separate clockwise (CW) versus counterclockwise (CCW) movements and target x and y positions. Data points are clustered according to both reach direction and curvature, indicating that the time-slicing component encodes information about the dynamics of the upcoming movement (dots, clockwise reaches; triangles, counterclockwise reaches).
We reasoned that single-trial kinematic information might be present in the data but obscured by behaviorally irrelevant neural variability. If true, then the decoder should perform significantly better on properly denoised data. To test this, we first used a common approach of fitting a low-rank approximation using NMF ( R = 12 components) to the N × ( T K ) matrix of trial-concatenated neural activity (‘neuron-unfolded’ data). Surprisingly, this decreased the performance of the decoder (Fig. 2a , neuron-slicing NMF), suggesting that the discarded variability contained information about hand kinematics. We wondered whether a better performance could be obtained with a method that explicitly identifies covariability across trials. Indeed, TCA-denoised data displayed a better match to the hand kinematics ( R = 12 components; Fig. 2a , TCA). Yet, by constraining the decomposition to be low tensor rank (and thus also discarding temporal variability across neurons), TCA is unable to reconstruct neural sequences at a sufficiently high temporal resolution to allow for precise behavioral readout.
By performing TCA and NMF on the neuron-unfolded data tensor, we have assumed that behaviorally relevant information in the data is represented by neural covariability (Fig. 1d ). However, previous work has emphasized that neural activity in motor regions is better described by stereotyped sequences that are distinct for each task condition 7 , 25 . Following this intuition, we performed the same decoding analysis on denoised trial-unfolded data, in which a T × ( N K ) matrix is approximated using NMF ( R = 12 components). Remarkably, this simple change in the denoising strategy resulted in a significantly better match between trial-to-trial variability in the data and the hand kinematics (Fig. 2a , trial-slicing NMF). We further validated that the components obtained by trial-slicing NMF corresponded to reach-tuned sequences whose temporal orderings were reproducible across held-out data (Supplementary Fig. 2 ). These results reveal that, in this dataset, behaviorally relevant information was encoded by trial covariability (specifically, neural sequences) rather than by neural covariability.
Trial- and neuron-concatenated NMFs constitute two special cases of non-negative sliceTCA in which either neuron-slicing components or trial-slicing components exclusively are fitted. Therefore, we next asked whether we could identify additional information in the data by demixing different classes of covariability with sliceTCA. Previous work has identified preparatory signals in the PMd that indicate the dynamics of the upcoming movement 26 . Therefore, we hypothesized that we could capture preparatory signals in a time-slicing component with a stereotyped ramping profile and neural weights encoding reach targets and curvature on a trial-by-trial basis.
Toward this end, we used sliceTCA to add a single time-slicing component to the previous model with 12 trial-slicing components (Fig. 2b and Supplementary Fig. 3 ; R neuron = 0, R trial = 12, R time = 1 selected based on the elbow of the cross-validated loss (Extended Data Fig. 4b )). In both the trial-slicing NMF model and the mixed covariability sliceTCA model, the trial-slicing components identified sequential neural activations for similar reach conditions that seemed to be continuously tuned to target angles (Fig. 2c and Supplementary Fig. 3 ). Decoding from these trial-slicing components (in either the mixed or unmixed model) led to significantly better performance as compared to the neuron-slicing and TCA models (Fig. 2e , Extended Data Fig. 4a and Supplementary Fig. 4 ). Furthermore, despite being a (multi)linear method, sliceTCA had a decoding performance on par with that of LFADS (latent factor analysis via dynamical systems) 27 for straight reaches and performed better for the maze condition ( P = 1.907 × 10 −6 , two-sided Wilcoxon signed-rank test; Fig. 2e and Extended Data Fig. 4a ). We additionally noted that the trial-slicing partial reconstruction from sliceTCA mapped onto hand kinematics slightly better in the mixed model than in the trial-slicing-only model (Fig. 2e ; P = 1.907 × 10 −6 , two-sided Wilcoxon signed-rank test). Intriguingly, while the single time-slicing component mapped poorly onto hand kinematics (Extended Data Fig. 4a ), its time course displayed a peak around 100 ms before movement onset followed by a drop in amplitude (Fig. 2d ), consistent with a motor preparatory signal.
If the time-slicing component contains motor preparatory information, we would further expect it to contain information regarding the parameters of the upcoming movement 26 . Indeed, the neural encoding weights in the PMd (but not M1; Extended Data Fig. 4c,d ) were correlated across similar conditions and encoded both reach direction and curvature (Fig. 2f–h ). Therefore, while the trial-slicing components directly encoded motor sequences governing hand kinematics, the time-slicing component contained primarily preparatory information about movement parameters. Interestingly, simply applying NMF without explicitly demixing covariability classes was not able to recover this preparatory signal (Extended Data Fig. 4e and Supplementary Fig. 5 ). Together, these results show that behaviorally relevant information in neural data can be spread across different slice types, motivating the need to demix covariability with sliceTCA.
Pipeline for sliceTCA model selection and optimization
Dimensionality reduction methods, while powerful, can prove challenging in practice. First, robustly identifying the optimal number of components is a crucial yet challenging step in interpreting the dimensionality of neural representations 28 , 29 . Even after the rank is fixed, invariances in the decomposition may lead to multiple possible solutions (for example, matrix factorizations are known to be invariant to invertible linear transformations such as rotations), although adding a non-negativity constraint (as in the case of NMF) confers better uniqueness properties compared to unconstrained matrix factorizations 30 . Thanks to the tractability of sliceTCA, we were able to characterize its mathematical invariances (Extended Data Figs. 5 and 6 ). To provide objective criteria for model selection and uniqueness, we developed a full analysis pipeline for sliceTCA, including data preprocessing, model selection, model optimization and visualization (Fig. 3 ). First, trials must be time-warped, trimmed or masked for the data to be shaped into a tensor. Alignment to key events is an important consideration to remove additional sources of variability that are not incorporated into sliceTCA assumptions, such as sequences that are jittered or warped in time 31 (Extended Data Fig. 7 ). We have taken the approach of piecewise linearly warping trials to task-relevant variables, but unsupervised warping is a promising alternative 32 . Second, to choose the optimal rank, we developed a rigorous cross-validation procedure to identify the number of components of each slice type, which we validated on ground-truth data (Extended Data Fig. 8a,b ). Third, we identified the two invariance classes leading to equivalent sliceTCA decompositions (that is, for which different sets of weights of the components yield the same reconstructed tensor approximation) ( Methods and Extended Data Figs. 5 and 6 ) and developed a hierarchical model optimization that adds additional constraints in the form of ‘sub-losses’ that must be minimized at three stages ( Methods and Extended Data Fig. 8c,d ). Model similarity analysis across different parameter initializations quantified the nonuniqueness of sliceTCA solutions (Extended Data Fig. 8e–h and Supplementary Fig. 6 ) and confirmed that the hierarchical optimization procedure leads to unique solutions. We further prove mathematically that a unique solution is guaranteed if each of the sub-losses is unique ( Supplementary mathematical notes ). Using a rigorous and standardized pipeline for model selection, fitting and optimization allows the user to make a robust, principled choice of sliceTCA decomposition for further interpretation.
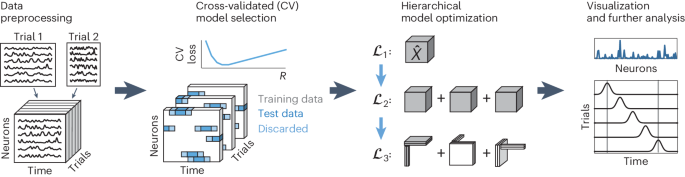
First, neural data are preprocessed to form a data tensor. In experiments with variable trial lengths, this could include temporal warping, exclusion of outlier trials and/or trimming to the time period of interest. Second, model selection is performed to choose the number of components of each slice type ( R neuron , R trial , R time ) based on the cross-validated mean-squared error (MSE) loss (blue curve). For cross-validation, we randomly assign blocks of consecutive time points (blue) within the data tensor as held-out data. The remaining entries of the tensor are used as training data (white). Specifically, the held-out entries are masked when computing the loss used to optimize the model parameters. To reduce temporal correlations between the training and testing data, we discard a brief period from the ends of the held-out blocks (light blue) from both training and testing. We use only the interiors of these blocks as test data (dark blue). Note that, because there are three slice types, the optimization is a 3D grid search on the cross-validated loss. Third, a hierarchical model optimization procedure is performed to identify a unique solution to the two mathematically identified invariance classes by optimizing the MSE loss \({{{{\mathcal{L}}}}}_{1}\) followed by secondary and tertiary losses \({{{{\mathcal{L}}}}}_{2}\) and \({{{{\mathcal{L}}}}}_{3}\) ( Methods ). After this procedure, the resulting loading vectors and slices can be analyzed.
Denoising task-relevant manifolds
With a standardized data analysis pipeline established, we next applied unconstrained sliceTCA to a new dataset consisting of the z -scored fluorescence traces of simultaneously imaged granule cells in the cerebellum and pyramidal neurons in the premotor cortex of mice performing a motor task (Fig. 4a ) 18 . Using the sliceTCA analysis pipeline, we selected a model with three trial-slicing components and three neuron-slicing components at the elbow of the cross-validated loss function (Fig. 4b,c and Extended Data Fig. 9a ; similar components were observed in the optimal model (Supplementary Fig. 7 )). By comparison, TCA required 18 components to attain the same performance and displayed redundancy in the fitted components (Extended Data Fig. 9c,d ). The cross-validation procedure resulted in no time-slicing components, as they consistently led to an increased test loss for this dataset (Extended Data Fig. 9a ). The first trial-slicing component captured temporally distributed cerebellar and cortical time courses that were common to both left and right correct reaches but distinct from error reaches (Fig. 4b,d ). In contrast, the second trial-slicing component accounted for the differential activation in left versus right trials (Fig. 4b,d ). A third component decayed slowly over trials, possibly representing adaptation over the course of the session (Fig. 4b ).
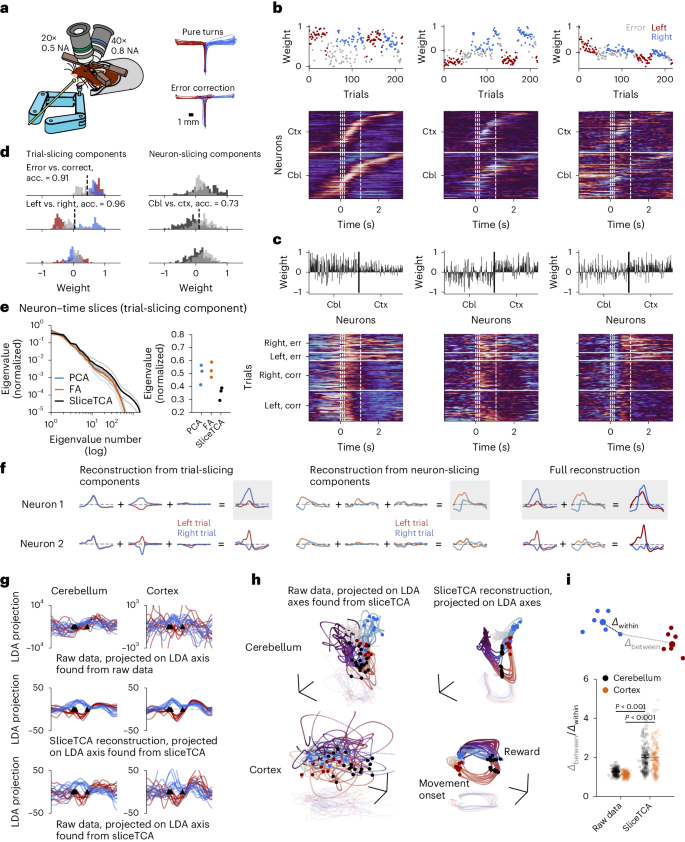
a , Schematic of the experimental setup. Image modified from ref. 18 . NA, numerical aperture. b , Trial-slicing components. Loading vector weights are colored according to trial type. In the slices, neurons are sorted within each region (Cbl, cerebellum; Ctx, premotor cortex) by the latency of the maximum activation in the first component. Dashed lines indicate movement onset, mid-turn, movement end and reward. c , Neuron-slicing components. In the slices, trials are separated by left/right and correct (corr)/error (err). Within blocks, trials are plotted in increasing order (ascending). d , Histograms of loading weights, colored by trial type and region. We classified weight vectors (correct versus incorrect, left versus right correct trials, cerebellum versus cortex). acc., accuracy. e , Left: eigenspectra of the covariance matrices of the slices of the trial-slicing components identified by PCA, factor analysis (FA) or sliceTCA, averaged over components (thick lines; transparent lines indicate individual components). Right: leading eigenvalue for each component. f , Single-neuron reconstructions of low-slice-rank approximations. The full sliceTCA reconstruction (right) is obtained by summing the contributions of all components from both slice types. g , Data from ten example trials per condition, projected onto an axis that maximally separates left and right correct trials between movement onset and reward. LDA, linear discriminant analysis. h , Neural manifolds in an orthonormalized neural subspace found with LDA (axis 1, same as g ; axis 2 separates movement onset versus reward; axis 3 separates reward expectation versus post-reward) from raw data and sliceTCA reconstruction. i , Separation of left versus right trajectories from full data and data denoised with sliceTCA. Δ within ( Δ between ) indicates the distance of the population vector around the time of movement onset to the center of the cluster of data points in its same (the opposite) trial class. Each dot represents a different trial for n = 151 correct left and right trials. Error bars represent the bootstrapped 95% confidence intervals of the mean. Left and right trajectories are more separable after sliceTCA denoising (two-sided Wilcoxon signed-rank test, P < 0.001 for both the cerebellum and premotor cortex).
In addition, the three neuron-slicing components captured trial-specific activations of population modes localized around the time of movement or reward (dashed lines, Fig. 4c ), with prolonged (and enhanced) activity in error trials, compared to correct trials, in the first and third components (two-sided Mann–Whitney U test, P < 0.001 for both components). Interestingly, the second neuron-slicing component captured differences between cerebellar and cortical activity (Fig. 4c,d ). We next asked how sliceTCA compares to matrix factorization methods that do not demix neural and trial covariability. To test this, we performed PCA and factor analysis on the neuron and trial unfoldings of the data tensor (Fig. 4e and Supplementary Fig. 8 ). Demixing covariability classes with sliceTCA resulted in components with higher-dimensional structure in the slices (Fig. 4e and Supplementary Fig. 8c,d ). This suggests that simply applying PCA to the tensor unfoldings cannot capture as much variability in the data (for example, in timing for individual neurons or trials) because it may be obscured by other dominant covariability types. Together, these results show that sliceTCA identifies both task-specific (left, right, error trials) and region-specific (cerebellum versus cortex) variables by capturing the structure of neural data across multiple covariability classes.
We next examined how applying sliceTCA affects reconstructed neural activity (Fig. 4f ). Toward this end, we compared the neural representations of the raw data in neural space to the reconstructed data from the sliceTCA model. The sliceTCA reconstruction captured the same top principal components as the raw data, confirming that it faithfully captured its overall structure (Supplementary Fig. 9 ). The advantage of including both neural covariability and trial covariability was reflected in the increased behavioral interpretability of the neural representations. For this, we projected the data onto the dimension that best separated left versus right correct trials during the period between movement and reward. The axis found from the sliceTCA reconstruction revealed more interpretable, denoised representations as compared to the dimension found from raw data (Fig. 4g ). Similarly, the task-relevant neural manifolds, found by projecting neural trajectories onto a subspace that separates activity along three task-relevant dimensions ( Methods ), appeared substantially denoised when sliceTCA was applied compared to a direct projection of the raw data (Fig. 4h and Supplementary Fig. 9 ). We confirmed this denoising effect by measuring the distance between left and right trials around the time of movement onset in sliceTCA reconstructions as compared to the raw data (Fig. 4i ). Our results indicate that sliceTCA, by grouping behaviorally similar trajectories in an unsupervised manner, increases the distance between trajectories of behaviorally distinct trials. Together, these findings show that sliceTCA is able to denoise task-relevant representations in neural data in an unsupervised fashion.
Identifying region-specific covariability patterns
Thus far, we have shown that mixed covariability occurs within the same neural population. However, the need to consider multiple covariability classes becomes even more crucial in multiregion recordings, as different brain areas are better described by different classes 7 . Yet, relying on different data tensor unfoldings for each region would require that they be analyzed separately without leveraging the simultaneous nature of such data. Therefore, we asked whether sliceTCA could demix area-specific representations in distinct slice types.
To test this idea, we took advantage of a recently published dataset consisting of Neuropixels recordings across six brain regions during a perceptual decision-making task (Fig. 5a ) 19 . Our cross-validation procedure selected a model with eight components: two trial-slicing components, three neuron-slicing components and three time-slicing components (Extended Data Fig. 10a and Supplementary Fig. 10 ). The two trial-slicing components identified variables related to behavioral performance (Fig. 5b ). The first trial-slicing component separated correct from incorrect trials (two-sided Mann–Whitney U test, P < 0.001), and the corresponding slice was characterized by reward-locked temporal response profiles in midbrain nuclei (anterior pretectal nucleus and midbrain reticular nucleus), which we validated in single-neuron peristimulus time histograms (PSTHs) (Fig. 5c ) and in nonwarped data (Extended Data Fig. 10b,c ). The second trial-slicing component instead featured temporally heterogeneous responses in all regions and correlated inversely with the log reaction times (Pearson’s r = −0.35, P < 0.001, n = 831 trials; Fig. 5b ). We next asked how these components contributed to the activity of different regions. The full sliceTCA reconstruction explained 33–49% of neural activity, depending on the region (Fig. 5d ). Of this reconstructed activity, the two trial-slicing components contributed considerably to neurons in the anterior pretectal nucleus, midbrain reticular nucleus and thalamus (19 ± 10%, mean ± s.d., n = 75 neurons; Fig. 5e ). Thus, the trial-slicing components identified stereotyped activations in subcortical regions (thalamus, anterior pretectal nucleus and midbrain reticular nucleus) that were linked to behavioral performance across trials.
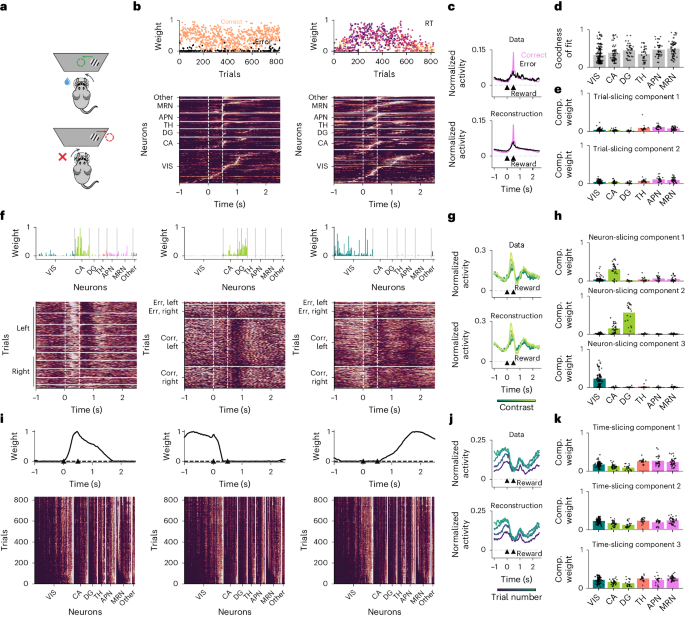
a , Schematic of the perceptual decision-making task from the IBL. Image modified from ref. 53 . Green (red) arrows and circles indicate correct (incorrect) actions. b , Trial-slicing components: the loading vector of component 1 shows a separation between correct (orange) and error (black) trials. In component 2, the color scale in the loading vector indicates the log reaction time (RT). In the corresponding slices: VIS, visual cortex; CA, hippocampus; DG, dentate gyrus; TH, thalamus; APN, anterior pretectal nucleus; MRN, midbrain reticular nucleus. White lines indicate stimulus onset and reward or timeout onset. Slice weights are normalized to [0, 1] for each neuron separately and sorted by the latency of the peak activation within each region (separately for each component). c , Top: PSTH of an example neuron from the anterior pretectal nucleus showing reward-locked activation for correct/error trials (pink/black). Bottom: PSTH built from the full sliceTCA reconstruction. Arrowheads indicate stimulus onset and reward. d , Reconstruction performance ( Methods ) of the full sliceTCA model, separated by region. Black dots indicate individual neurons. e , Contribution of each trial-slicing component to the overall reconstruction. Comp. weight, component weight. f , Neuron-slicing components: trials are grouped into blocks separately for different components. In component 1 (hippocampal region CA1 related), trials are grouped by contrast separately for left/right trials (within left/right, contrast increases from bottom to top). In components 2 (dentate gyrus related) and 3 (visual cortex related), trials are grouped into blocks by left/right and correct/error. For all slices, within each block, trials are sorted in increasing order (ascending). Each slice is normalized to [0, 1]. g , Top: PSTH of an example hippocampal neuron for low to high contrast (dark to light green). Bottom: PSTH built from the full sliceTCA reconstruction. h , Contribution of each neuron-slicing component to the overall reconstruction. i , Time-slicing components: in the slices, neurons are sorted within each region according to increasing activation in early trials after normalizing weights for each neuron to [0, 1] (same sorting across components). j , Top: PSTH of an example visual cortical neuron for early to late trials (indigo to teal). Bottom, PSTH built from the full sliceTCA reconstruction. k , Contribution of each time-slicing component to the overall reconstruction.
In contrast, the three neuron-slicing components identified three distinct clusters of neurons corresponding to cortical regions: the hippocampus, dentate gyrus and visual cortex (Fig. 5f ). These components, therefore, represented population-wide covariability patterns that were specific to each of these regions. The slice of the hippocampus-preferring component was characterized by a contrast-dependent activation between the sensory cue and reward (correlation of stimulus-evoked responses with contrast, Pearson’s r = 0.40, P < 0.001; Fig. 5f,g ), a feature that was less prominent in the dentate gyrus and not observed in visual cortex-preferring components ( r = 0.11, P = 0.002 for dentate gyrus, r = −0.05, P = 0.14 for visual cortex). In the dentate gyrus-preferring component, we observed post-reward suppression on correct (rewarded) trials, which was significantly shorter on error trials (two-sided Mann–Whitney U test, P < 0.001; Fig. 5f ). The final visual cortex-preferring component revealed pre-stimulus activation that increased in strength over trials (Pearson’s r = 0.55, P < 0.001; Fig. 5f ), possibly indicating the emergence of a predictive signal of cue onset over the course of the experiment. Each component contributed to a large fraction of the sliceTCA reconstruction in its respective region (37 ± 21%, n = 138 neurons; Fig. 5h ). Therefore, the three neuron-slicing components represented different task-relevant features that were separately encoded in hippocampal, dentate gyrus and visual cortical population responses.
Finally, the remaining time-slicing components partitioned the task duration into three distinct periods: early (pre-stimulus and stimulus onset), late (post-reward) and reward periods (Fig. 5i ). The corresponding slices revealed smooth variations of the strength of each of these components in single neurons over the course of the experiment. While these changes appeared low-rank, simply replacing them with a TCA component led to a drop in the reconstruction error (Supplementary Fig. 12 ). Furthermore, given the strong similarity of the three slices, we asked whether the components could sum to a flat trial-varying baseline for each neuron. However, we observed examples of a broad range of modulation patterns of PSTHs, with slowly varying activity that changed heterogeneously over trials for the three task periods (for example, in Fig. 5j ). Indeed, a substantial proportion of neurons across all regions showed significantly different rates of change in trial weights across the three components (analysis of variance, P < 0.05 with Bonferroni correction, n = 221 neurons; Extended Data Fig. 10d ). Moreover, these three components contributed substantially to the sliceTCA reconstruction across all recorded regions (62 ± 18%, n = 213 neurons; Fig. 5k ), demonstrating that the dataset was dominated by time covariability. Therefore, we asked whether the task-relevant and region-specific information observed in the trial and neural slice-type components would be visible without explicitly demixing the covariability classes with sliceTCA. However, simply applying NMF to the relevant unfoldings led to neural loadings that were not clustered by region and trial loadings that were not correlated with behavior (Supplementary Fig. 13 ). In contrast to sliceTCA, TCA components were less region-specific (Extended Data Fig. 10e ). Together, these results show that, by accounting for different classes of covariability, sliceTCA is able to demix multiregion recording data into brain-wide representations of task period, behaviorally relevant stereotyped activity and population-wide patterns of covariability encoded by individual regions.
Geometric interpretation of mixed covariability
Dimensionality reduction methods such as PCA allow for the interpretation of neural representations as trajectories embedded in a low-dimensional latent subspace within the full neural activity space. In sliceTCA, the neuron-slicing components can be interpreted in the same way owing to their relationship to standard matrix factorizations. However, the time- and trial-slicing components have different interpretations, as their loading vectors form bases of subspaces of the time and trial spaces. How, then, can we grasp the time- and trial-slicing components’ contributions to latent representations in neural activity space?
We can answer this question by considering the contribution from each slice type separately. First, note that while the neuron-slicing components are constrained to an R neuron -dimensional subspace, their trajectories within that subspace are unconstrained over trials (Fig. 6 , neuron-slicing component). Conversely, the trajectories of the R time time-slicing components are constrained to be a linear combination of a few common temporal profiles, but the neural weight vectors can instead vary from trial to trial. Geometrically, this means that the reconstruction from these components lies within an R time -dimensional subspace that can now vary on each trial, but the embedded low-dimensional trajectories will have similar shapes (Fig. 6 , time-slicing component). Finally, the R trial trial-slicing components’ neural weights change at every time point, whereas trial weights are fixed. This corresponds to trajectories that are no longer embedded in a low-dimensional subspace but that are instead constrained to be linear combinations of stereotyped, potentially high-dimensional trajectories (Fig. 6 , trial-slicing component; see Supplementary Fig. 14 for an example with multiple components). In this way, the three covariability classes that we have described can also be seen as three classes of latent activity in neural state space. All three classes combine to form the full reconstruction, which may appear more complex than any one component type (Fig. 6 , reconstruction).
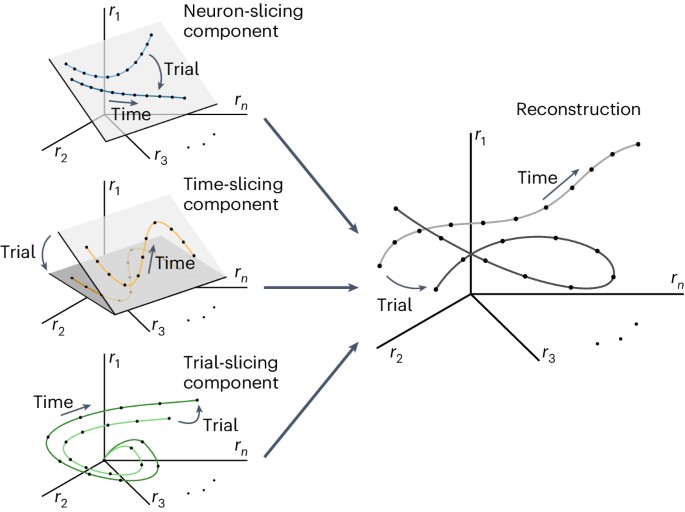
Neuron-slicing component: example of two neuron-slicing components visualized in neural activity space. The latent activity is embedded in a 2D subspace, but their trajectories within that subspace are unconstrained. Time-slicing component: example of two time-slicing components. These are also embedded within a 2D subspace, but that subspace may vary over trials. Within each latent subspace, the latent trajectories have similar shape as they are constrained to linear combinations of a few characteristic temporal weights. Trial-slicing component: the trial-slicing components are not constrained to any subspace, as the neural encodings may change at every time point. These components describe linear combinations of a few potentially high-dimensional latent trajectories such as neural sequences. Note that, here, only one component is shown for clarity. In this case, the latent trajectory is simply re-scaled on each trial. Reconstruction: after summing these components, the full latent trajectories are not necessarily limited by any of the geometric constraints that characterize individual slice types.
This geometric view illustrates that, by fitting different covariability classes, sliceTCA is able to capture latent trajectories that are no longer confined to a linear subspace despite still being a multilinear method. In contrast, traditional matrix factorization methods that capture only a single covariability class are restricted to one of the three geometric classes of latent activity in neural state space shown in Fig. 6 , whereas TCA constrains its components to obey the geometrical constraints of all three classes simultaneously (Supplementary Fig. 15 ). In sum, sliceTCA is able to capture a broader range of covariability structure in neural data (and a broader range of latent representations in neural space) than related methods, all while remaining easily interpretable.
Neural activity is often interpreted as low-dimensional population modes representing patterns of covariation across neurons. We have advocated for an expansion of this view to describe three distinct classes of covariability: across neurons, across time and across trials. We further introduced sliceTCA, a new tensor decomposition that demixes these covariability classes in large-scale neural data. Through several example datasets, we demonstrated that sliceTCA captures more task-relevant covariability in fewer components, allowing for the discovery of intricate latent structure in population activity. Thus, sliceTCA expands the classic view of neural representations toward latent variables that are not constrained to a fixed low-dimensional subspace.
Our framework of multiple covariability classes addresses key limitations of the classic view on latent variables, which is unable to identify several types of structure commonly found in neural data (for example, neural sequences) 7 , 25 . Indeed, task-relevant sequences are a widespread phenomenon observed across brain regions 11 , 33 , 34 . While we emphasized the ability of trial covariability to capture condition-specific neural sequences, we note that this class can capture more complex forms of stereotyped temporal patterning across neurons 35 , 36 , 37 . In contrast, population modes characterized by variable timing on different trials (for example, in temporal difference learning 38 ) are captured by neural covariability. Lastly, temporal covariability captures stereotyped trajectories embedded in reaching direction-specific subspaces within the neural state space 39 . We speculate that temporal covariability could also capture latent subspaces that evolve slowly due to learning or drift 12 , 14 . Our results support previous work arguing that different brain regions are better described by different covariability classes 7 . Importantly, we further show that, without demixing covariability, task-relevant variability can be obscured by components of the dominant slice type (Supplementary Figs. 5 , 8 and 13 ). Therefore, demixing covariability classes may be a crucial step when considering multiregion recordings that may contain qualitatively distinct computations in different populations.
A long-standing challenge in systems neuroscience is the difficulty of mapping neural variability to changes in behavior 40 . Despite being unsupervised, sliceTCA was able to disentangle behavioral and task information in each of the datasets presented. This may be due to two factors: first, demixing covariability effectively ‘denoises’ components representing task variables that would have otherwise been occluded by other covariability classes. Second, trial-slicing components identify changes that are common across trials, which tend to be defined by task variables or behavioral outcomes. Indeed, we found that trial-slicing components often correlated with behavioral variables. Moreover, using feedforward and recurrent circuit models, we demonstrated how sliceTCA could offer a window into the computational roles of variables modeled by different slice types. Hence, we argue that the classical view on latent neural representations, which assumes that behaviorally relevant neural variability is correlated across neurons, is overly reductionist and may miss many types of neural dynamics underlying behavior.
A key advantage of matrix and tensor decompositions is their simplicity. (Multi)linear methods can perform as well as nonlinear methods in specific applications while remaining considerably more interpretable (Extended Data Fig. 4a ). Indeed, the analytical tractability of sliceTCA enabled us to characterize its invariance classes and to propose a method to identify a unique solution in the unconstrained case (Extended Data Fig. 8c ). Identifying invariances is crucial for reproducibility and interpretation, as nonunique solutions may prohibit clear comparisons across datasets 41 , 42 . This issue is ever more important with the trend toward comparisons of neural data to task-trained neural networks, whose representations are known to be sensitive to model specifications 43 , 44 . Going forward, matrix and tensor decompositions could prove useful for comparing latent representations by virtue of their tractability.
SliceTCA is closely related to both TCA and PCA while offering more flexibility by capturing multiple covariability classes. However, in some cases, TCA or PCA may be sufficient to capture the data while also offering other advantages. If no demixing is required, PCA has the benefit of having a closed-form solution (but we note that PCA is a special case of sliceTCA that could be identified through our pipeline). In contrast, the stronger constraints imposed by TCA mean that it generally requires more components than PCA or sliceTCA yet has fewer parameters per component. As such, TCA and sliceTCA represent two sides of a trade-off between model parsimony and expressivity, which could be balanced by combining their respective strengths (Supplementary Fig. 12 ). Future work could generalize sliceTCA and TCA using the partition rank 45 . However, careful consideration would be required to fully understand the implications of a low-partition-rank decomposition, including potentially new invariances.
While tensor decompositions can be viewed as generalizations of matrix factorizations, they have specific limitations (for example, they are generally more computationally expensive 46 , 47 ). Data tensors also require trimming, masking or warping trials to the same length. These preprocessing steps make implicit assumptions about the temporal structure of latent variables: warping assumes that latent variables are simply rescaled in time on different trials 48 , whereas trimming is more suitable when latent variables have a fixed intrinsic temporal structure independent of trial length (for example, background oscillations) 32 . Because of these considerations, we note that sliceTCA may not be a good fit for datasets in which these two kinds of temporal structure are mixed, datasets that lack a systematic trial structure or datasets in which activity is dominated by chaotic dynamics rather than patterns of covariation. More generally, time warping is a thorny issue for tensor decomposition when key events for alignment are unknown (Supplementary Fig. 11 ). Toward this end, unsupervised time-warping methods could help identify unlabeled events in the data, whether as a preprocessing step 32 or performed simultaneously with dimensionality reduction 31 , 49 .
Together, tensor decompositions are useful for neural data, as they allow for the discovery of patterns in trial-structured data. While we focused on third-order tensors, data tensors of even higher order could be imagined by adding legs corresponding to days, conditions or even individuals 50 , 51 . Going forward, our framework of mixed covariability could, therefore, help advance our understanding of behaviorally relevant latent structure in high-dimensional neural data across brain regions and subjects.
No original data were collected for this study. We analyzed data from three previous datasets 17 , 18 , 19 . All experiments were approved by the relevant bodies: the Institutional Animal Care and Use Committee of Stanford University (dataset 1), the Administrative Panel on Laboratory Animal Care and Administrative Panel on Biosafety of Stanford University (dataset 2), and the Institutional Animal Care and Use Committees of Cold Spring Harbor Laboratory (dataset 3). Additional experimental details can be found below.
Definition of the sliceTCA model
Matrix rank and matrix factorization.
Consider a data matrix consisting of N neurons recorded over T samples (time points): \({{{{X}}}}\in {{\mathbb{R}}}^{N\times T}\) . Matrix factorization methods find a low-rank approximation \(\hat{{{{{X}}}}}\) following equation ( 1 ), in which each component is a rank-1 matrix: X ( r ) = u ( r ) ⊗ v ( r ) , where \({{{{\bf{u}}}}}^{(r)}\in {{\mathbb{R}}}^{N}\) and \({{{{\bf{v}}}}}^{(r)}\in {{\mathbb{R}}}^{T}\) are vectors representing the neural and temporal coefficients, which are chosen to minimize a loss function. In other words, the activity of neuron n at time t is given by
A common choice of loss function is the MSE:
Constraints may be added to the minimization of the loss, such as non-negativity of the coefficients in NMF.
Slice rank and sliceTCA
A d -tensor is a generalization of a matrix to d legs (that is, a data matrix is a 2-tensor). Here, we are specifically concerned with 3-tensors typically used in neuroscience, in which the three legs represent neurons, time and trial/condition: \({{{{X}}}}\in {{\mathbb{R}}}^{N\times T\times K}\) . SliceTCA extends the matrix factorization in equation ( 1 ) by fitting X with a low ‘slice rank’ approximation 23 . A slice-rank-1 d -tensor is an outer product of a vector and a ( d − 1)-tensor. For the 3-tensors that we have been considering, this corresponds to the outer product of a ‘loading’ vector and a 2-tensor, thus making this 2-tensor a ‘slice’ of this slice-rank-1 tensor up to a scalar multiple determined by the loading vector.
Each sliceTCA component can be one of three different slice types. For example, a neuron-slicing component can be written as X ( r ) = u ( r ) ⊗ A ( r ) , where \({{{{{A}}}}}^{(r)}\in {{\mathbb{R}}}^{T\times K}\) is the time-by-trial slice representing the weights of the component across both time and trials and the vector u ( r ) represents the neural loading vector. Components of other slice types can be constructed similarly with their respective loading vectors and slices: \({{{{\bf{v}}}}}^{(r)}\in {{\mathbb{R}}}^{T},\;{{{{{B}}}}}^{(r)}\in {{\mathbb{R}}}^{N\times K}\) for the time-slicing components and \({{{{\bf{w}}}}}^{(r)}\in {{\mathbb{R}}}^{K},\;{{{{{C}}}}}^{(r)}\in {{\mathbb{R}}}^{N\times T}\) for the trial-slicing components. Put together, this results in a decomposition of the following form:
Because of the different slice types, each sliceTCA model can be described by the hyperparameter three-tuple R = ( R neuron , R trial , R time ), defining the number of neuron-, trial- and time-slicing components, for a total of R neuron + R trial + R time components.
Relationship to TCA
The extension of matrix factorizations to TCA is based on a different definition of tensor rank, in which a rank-1 tensor is as an outer product of d vectors. Each component is defined by a set of vectors corresponding to neuron, time and trial coefficients \({{{{\bf{u}}}}}^{(r)}\in {{\mathbb{R}}}^{N}, {{{{\bf{v}}}}}^{(r)}\in {{\mathbb{R}}}^{T},{{{{\bf{w}}}}}^{(r)}\in {{\mathbb{R}}}^{K}\) for each component: X ( r ) = u ( r ) ⊗ v ( r ) ⊗ w ( r ) . Then, each element of the approximated data tensor can be written as
In other words, a TCA component is a special case of a sliceTCA component in which the slice is a rank-1 matrix. In this way, sliceTCA is more flexible than TCA, as it has fewer constraints on the type of structure that is identified in the data. However, this increase in flexibility comes with the cost of an increased number of parameters, as sliceTCA fits all the entries of each slice. The flexibility of sliceTCA also leads to different invariance classes as discussed below. Finally, we note that the two methods can, in principle, be merged by incorporating TCA components into equation ( 4 ).
SliceTCA invariance classes
Transformations within a slice type.
Matrix factorization methods are known to be invariant to invertible linear transformations, including, but not limited to, rotations of the loading vectors. For example, suppose we decompose a matrix \({{{{Y}}}}\in {{\mathbb{R}}}^{N\times T}\) into a product of a matrix of weights, \({{{{W}}}}\in {{\mathbb{R}}}^{N\times R}\) , and a matrix of scores, \({{{{S}}}}\in {{\mathbb{R}}}^{R\times T}\) . Consider any invertible linear transformation \({{{{F}}}}\in {{\mathbb{R}}}^{R\times R}\) . Then, Y can be rewritten as
where \(\tilde{{{{{W}}}}}={{{{WF}}}}\) and \(\tilde{{{{{S}}}}}={{{{{F}}}}}^{-{{{{1}}}}}{{{{S}}}}\) . As a result, matrix decompositions, such as factor analysis, lead to not one solution but rather an invariance class of equivalent solutions. Note that PCA avoids this problem by aligning the first component to the direction of the maximum projected variance, as long as the eigenvalues of the covariance matrix are distinct. However, other methods that do not have a ranking of components are not able to use the same alignment. SliceTCA inherits this same invariance class, as all the loading vectors within a given slice type can be transformed in the same way as equation ( 6 ) to yield the same partially reconstructed tensor for each slice type (Extended Data Fig. 5a ).
Transformations between slice types
SliceTCA has an additional invariance class due to the fundamental properties of multilinear addition. For example, consider a slice-rank-2 tensor \({{{{Y}}}}\in {{\mathbb{R}}}^{N\times T\times K}\) , which is made of two components of different slice types. We will assume without loss of generality that these are neuron- and time-slicing components with corresponding slices V and U , such that
Then, the following transformation can be performed for the arbitrary vector \({{{\bf{z}}}}\in {{\mathbb{R}}}^{K}\) :
where \(\tilde{{{{{V}}}}}={{{{V}}}}-{{{\bf{v}}}}\otimes {{{\bf{z}}}}\) and \(\tilde{{{{{U}}}}}={{{{U}}}}+{{{\bf{u}}}}\otimes {{{\bf{z}}}}\) are transformations of the original slices. This invariance class, therefore, corresponds to passing a tensor-rank-1 tensor between two slices of differing slice types (Extended Data Fig. 5b ).
Note that two classes of transformations (within slice type and between slice type) commute (see proposition 2.1 of Supplementary mathematical notes ); therefore, one cannot obtain a new transformation by, for example, applying the first transformation, followed by the second and then the first again.
Identification of a unique sliceTCA decomposition
To find a uniquely defined solution, we can take advantage of the natural hierarchy between the two invariance classes. Specifically, let us first define the partial reconstruction \({\hat{{{{{X}}}}}}{\,}^{{{{\rm{neuron}}}}}\) of the low-slice-rank approximation \(\hat{{{{{X}}}}}\) based on the neuron-slicing components; that is
and let \({\hat{{{{{X}}}}}}{\,}^{{{{\rm{time}}}}}\) and \({\hat{{{{{X}}}}}}{\,}^{{{{\rm{trial}}}}}\) be similarly defined, so that \(\hat{{{{{X}}}}}={\hat{{{{{X}}}}}}{\,}^{{{{\rm{neuron}}}}}+{\hat{{{{{X}}}}}}{\,}^{{{{\rm{time}}}}}+{\hat{{{{{X}}}}}}{\,}^{{{{\rm{trial}}}}}\) . Now, note that the within-slice-type transformations change the weights of the loading vectors and slices of all components of a given slice type without changing the partial reconstructions for each slice type. For example, applying these transformations to the neuron-slicing components would change u ( r ) and A ( r ) but not \({\hat{{{{{X}}}}}}{\,}^{{{{\rm{neuron}}}}}\) . On the contrary, the between-slice-type transformations change the partial reconstructions \({\hat{{{{{X}}}}}}{\,}^{{{{\rm{neuron}}}}},\;{\hat{{{{{X}}}}}}{\,}^{{{{\rm{time}}}}}\) and \({\hat{{{{{X}}}}}}{\,}^{{{{\rm{trial}}}}}\) , but not the full reconstruction \(\hat{{{{{X}}}}}\) . Therefore, the key to identifying a unique solution is first to perform the between-slice-type transformations to identify the unique partial reconstructions \({\hat{{{{{X}}}}}}{\,}^{{{{\rm{neuron}}}}},\;{\hat{{{{{X}}}}}}{\,}^{{{{\rm{time}}}}}\) and \({\hat{{{{{X}}}}}}{\,}^{{{{\rm{trial}}}}}\) and then perform the within-slice-type transformations to identify the unique loading vectors and components.
We leveraged this hierarchy to develop a post hoc model optimization into three steps, each with a distinct loss function. The first step identifies a model that minimizes a loss function \({{{{\mathcal{L}}}}}_{1}\) defined on the full reconstruction (Extended Data Fig. 8c (i)), resulting in the approximation \(\hat{{{{{X}}}}}\) . Next, because of the two invariance classes, there is a continuous manifold of solutions with different parameters (loading vectors and slices) that, after being recombined, all result in the same \(\hat{{{{{X}}}}}\) and, therefore, have the same loss. Next, we use stochastic gradient descent to identify the between-slice-type transformation that minimizes a secondary loss function \({{{{\mathcal{L}}}}}_{2}\) , which fixes \({\hat{{{{{X}}}}}}{\,}^{{{{\rm{neuron}}}}},\;{\hat{{{{{X}}}}}}{\,}^{{{{\rm{time}}}}}\) and \({\hat{{{{{X}}}}}}{\,}^{{{{\rm{trial}}}}}\) without affecting \(\hat{{{{{X}}}}}\) (Extended Data Fig. 8c (ii)). Finally, we identify the within-slice-type transformation that minimizes a tertiary loss function \({{{{\mathcal{L}}}}}_{3}\) to arrive at the final components (loading vectors u ( r ) , v ( r ) , w ( r ) and slices A ( r ) , B ( r ) , C ( r ) ) without affecting \({\hat{{{{{X}}}}}}{\,}^{{{{\rm{neuron}}}}},\;{\hat{{{{{X}}}}}}{\,}^{{{{\rm{trial}}}}}\) and \({\hat{{{{{X}}}}}}{\,}^{{{{\rm{time}}}}}\) (Extended Data Fig. 8c (iii)). Each of the three loss functions can, in principle, be chosen according to the constraints or normative assumptions most relevant to the question at hand.
We note that, if we performed only the \({{{{\mathcal{L}}}}}_{1}\) optimization step, then different initializations would lead to different solutions for the coefficients. Both the \({{{{\mathcal{L}}}}}_{2}\) and \({{{{\mathcal{L}}}}}_{3}\) steps are necessary to identify a unique solution across the two invariance classes. If we applied only \({{{{\mathcal{L}}}}}_{3}\) after \({{{{\mathcal{L}}}}}_{1}\) , there would be no guarantee that \({\hat{{{{{X}}}}}}{\,}^{{{{\rm{neuron}}}}}\) would be the same for two seeds, as they could differ by more than just a rotation due to the between-slice-type invariances; therefore, it would not necessarily be possible to identify a unique solution. If we then applied \({{{{\mathcal{L}}}}}_{2}\) to correct this, we would need to reapply \({{{{\mathcal{L}}}}}_{3}\) to come up with a unique set of coefficients. Therefore, the most natural way to identify a unique solution is to exploit the hierarchical structure of the invariances by optimizing the invariances in the proposed order: \({{{{\mathcal{L}}}}}_{1}\) , then \({{{{\mathcal{L}}}}}_{2}\) , then \({{{{\mathcal{L}}}}}_{3}\) . More precisely, we prove that, if each of these objective functions leads to a unique solution, the decomposition is unique under weak conditions (see theorem 2.7 in Supplementary mathematical notes ).
This procedure can also be understood more intuitively by considering the case in which there is only a single component type, in which case sliceTCA reduces to a matrix factorization. Even then, minimizing \({{{{{{{{\mathcal{L}}}}}}}}}_{{{{{1}}}}}\) is not sufficient to determine a unique model due to there being a continuum of factor rotations that yield the same \(\hat{{{{{X}}}}}\) . PCA solves these invariances by constraining the factors to be orthogonal and ranking them by variance explained, resulting in a unique solution (under certain weak conditions, for example, up to sign reversals if all singular values are unique). This can be written through an additional loss function (equivalent to \({{{{\mathcal{L}}}}}_{3}\) in our framework). When considering mixed slice types, the second step (minimizing \({{{{\mathcal{L}}}}}_{2}\) ) becomes necessary owing to the invariant transformations between slice types.
Model selection, optimization and fitting
To fit sliceTCA for a given dataset arranged as a 3-tensor, we followed the data analysis pipeline described in the main text. Below, we provide details and hyperparameters for the steps involved in the pipeline.
Fitting sliceTCA with stochastic gradient descent
For a fixed choice of R , model parameters (that is, the loading vectors and slices of all components) were fitted using the optimizer Adam 54 in Pytorch. Initial parameters were randomly drawn from a uniform distribution over [−1, 1] or [0, 1] for unconstrained and non-negative sliceTCA, respectively. Throughout, we optimized the MSE loss in equation ( 3 ) with a learning rate of 0.02. Note that, during model selection, some of these entries will be masked (that is, not be summed in the loss) for cross-validation (see the next section). To introduce stochasticity in the computation of the gradient, and thus avoid local minima, we additionally masked a fraction of tensor entries so that they are not included in the calculation of the loss. This fraction starts at 80% and decreases exponentially during training with a decay factor of 0.5 over three (Fig. 2 ) or five blocks of iterations (Figs. 4 and 5 ). Within each block, the mask indices are randomly reinitialized every 20 of a total of 150 (Fig. 2 ), 200 (Fig. 4 ) or 100 iterations per block (Fig. 5 ). Run time scales approximately linearly with the number of components (Supplementary Fig. 16 ). To obtain an optimal model under a given R , we repeated the fitting procedure ten times with different random seeds and chose the model with the lowest loss.
Cross-validated model selection
To choose the number of components in each slice type, we run a 3D grid search to optimize the cross-validated loss. In addition to the decaying mask used during model fitting, we masked 20% of the entries throughout the fitting procedure as held-out data. These masked entries were chosen in randomly selected 1-s (Fig. 4 ) or 150-ms blocks (Fig. 5 ) of consecutive time points in random neurons and trials. Blocked masking of held-out data (rather than salt-and-pepper masking) was necessary to avoid temporal correlations between the training and testing data due to the slow timescale of the calcium indicator or due to smoothing effects in electrophysiological data. To protect further against spuriously high cross-validation performance due to temporal correlations, we trimmed the first and last 250 ms (Fig. 4 ) or 40 ms (Fig. 5 ) from each block; these data were discarded from the test set, and only the remaining interior of each block was used to calculate the cross-validated loss. We repeated the grid search ten times with different random seeds for train–test split and parameter initialization while keeping a constant seed for different R . Once the cross-validated grid search was complete, we selected R * by identifying the model with a minimum or near-optimal average test loss across seeds. Admissible models are defined as those achieving a minimum of 80% of the optimal performance for nonconstrained sliceTCA and 95% of the optimal model performance for non-negative sliceTCA, as compared to root-mean-squared entries of the raw data.
Hierarchical model optimization
For the first step of the model optimization procedure, we chose the MSE loss for \({{{{\mathcal{L}}}}}_{1}\) :
as in the model selection (essentially refitting the model with the specific ranks identified with the cross-validation procedure on the entire data). For \({{{{\mathcal{L}}}}}_{2}\) , we used the sum of the squared entries of the three partial reconstructions from each slice type:
where \({{{\bf{x}}}}\in {{\mathbb{R}}}^{{R}_{{{{\rm{time}}}}}\times {R}_{{{{\rm{trial}}}}}\times N},\;{{{\bf{y}}}}\in {{\mathbb{R}}}^{{R}_{{{{\rm{neuron}}}}}\times {R}_{{{{\rm{trial}}}}}\times T}\) and \({{{\bf{z}}}}\in {{\mathbb{R}}}^{{R}_{{{{\rm{neuron}}}}}\times {R}_{{{{\rm{time}}}}}\times K}\) . This can be thought as a form of L2 regularization. For \({{{{\mathcal{L}}}}}_{3}\) , we chose orthogonalization and variance explained ordering through singular value decomposition (SVD).
We stress that the losses \({{{{\mathcal{L}}}}}_{1},\;{{{{\mathcal{L}}}}}_{2}\) and \({{{{\mathcal{L}}}}}_{3}\) may be chosen according to the specific problem at hand. For example, different factor rotations could be easily implemented into the hierarchical model optimization, including varimax or even oblique (that is, nonorthogonal) rotations. Therefore, while we chose an \({{{{\mathcal{L}}}}}_{3}\) that constrained components to be orthogonal, in general, sliceTCA does not necessarily need to return orthogonal components. Finally, we remark that the hierarchical model optimization procedure is valid only for unconstrained sliceTCA, as adding a non-negativity constraint restricts the possible space of solutions. This also explains why non-negative factorizations (for example, NMF) are known to suffer less from uniqueness issues but also require more complex conditions to guarantee uniqueness 30 . Future work could borrow from existing methods for factor rotations specifically designed for NMF to extend to non-negative sliceTCA 55 .
Model similarity
To estimate whether solutions found with sliceTCA are unique in practice, we adopted a measure of the model similarity of the solutions found from different random initializations 4 , 56 . This score is based on computing the angle between a pair of vectors corresponding to the loading factors of two models after components are matched according to the Hungarian algorithm. For each pair of sliceTCA components, we unfolded the slice of each component into a vector. Then, we computed the angle between the loading vectors, the angle between the vectors resulting from unfolded slices, and their average values.
Following previous work 4 , we computed this modified similarity score for each of the ten randomly initialized models against the model that achieved the lowest MSE loss. We calculated (1) the overall model similarity and (2) the model similarity for each slice type, which could be an informative diagnostic tool for model optimization in future work. To establish a baseline chance level of similarity, we also computed a shuffled model similarity score: for each slice type and component, we shuffled the elements of the weight vectors of one of the two models within the respective weight vectors before computing their similarity score. We then calculated the mean similarity over 100 shuffle repetitions for each slice type.
Feedforward model of perceptual learning
We modeled a population of linear neurons receiving a sensory input from upstream sources representing a go stimulus and a no-go stimulus, as well as an input representing a top–down modulation that varied from trial to trial. On each trial k , either the go or no-go stimulus was activated, with probability P = 0.5 of presenting the same stimulus as in the previous trial. Go/no-go inputs ( x go , x no ) were assumed to follow the same bell-shaped activation function \({s}_{t}={e}^{-{(t-4)}^{2}}\) on the trials during which their corresponding stimulus was presented, that is, \({x}_{t,k}^{\,{\textrm{go}}}={s}_{t}\) if k was a go trial and \({x}_{t,k}^{\textrm{go}}=0\) otherwise (and vice versa for the no-go input).
The stochastic learning process of the go and no-go weights \({{{{\bf{w}}}}}_{k}^{\textrm{go}},{{{{\bf{w}}}}}_{k}^{\textrm {no}}\in {{\mathbb{R}}}^{N}\) over trials was modeled as an Ornstein–Uhlenbeck process, which was initialized at \({{{{\bf{w}}}}}_{0}^{\textrm{go}}={{{{\bf{w}}}}}_{0}^{\textrm{no}}=\bf{1}\) and evolved independently across neurons:
where \({\alpha }_{n} \sim {{{\mathcal{U}}}}([0.2,0.8])\) are the neuron-specific learning rates, and μ go = 2, μ no = 0, σ = 1.3. Furthermore, to keep weights non-negative and simulate their saturation, we clamped them to [0, 2]. The process was evaluated using a stochastic differential equation solver and sampled at K evenly spaced points in [0, 10] representing K trials.
Top–down modulation was modeled as a rectified Gaussian process:
with the temporal kernel:
where \(l=\sqrt{0.5}\) . Top–down weights were nonplastic and distributed as \({w}_{n}^{\textrm{TD}} \sim {{{\mathcal{U}}}}([0,1])\) .
The activity of each neuron was thus given by
where the sensory input is combined into \({w}_{n,k}^{\textrm{S}}={w}_{n,k}^{\textrm{go}}{{\mathbb{1}}}_{k}^{\textrm{go}}+{w}_{n,k}^{\textrm{no}}(1-{{\mathbb{1}}}_{k}^{\textrm{go}})\) , where \({{\mathbb{1}}}^{\textrm{go}}\) is an indicator function that is 1 when trial k is a go trial and 0 if it is a no-go trial. By construction, the tensor X has a slice rank of 2, as it can be written in the following form:
where \({I}_{n,t,k}^{\,{\textrm{S}}}={w}_{n,k}^{\textrm{S}}{s}_{t}\) is a time-slicing component representing the weighted, trial-specific sensory input and \({I}_{n,t,k}^{\textrm{TD}}={w}_{n}^{\textrm{TD}}{x}_{t,k}^{\textrm{TD}}\) is a neuron-slicing component representing top–down modulatory factors that vary over trials. In our simulations, we used K = 100, T = 90, N = 80.
We fitted sliceTCA with non-negativity constraints to the synthetic dataset, using five blocks of 200 iterations each with a learning rate that decayed exponentially over blocks from 0.2 to 0.0125 and a mask that decayed exponentially over blocks from 0.8 to 0.05. Masked entries changed randomly every iteration. Initial parameters were drawn uniformly over [0, 1].
RNN model of condition-dependent neural sequences
Model description.
We built a model of a linear RNN that produces recurrently generated sequences for different task conditions while also receiving condition-independent inputs. To generate sequences, we parameterize the connectivity matrix \(W\in {{\mathbb{R}}}^{N\times N}\) by a Schur decomposition 24 . Additionally, we let the central matrix have a block-diagonal structure to embed multiple sequences into the dynamics. Formally, we let W = U S U T , where U is a unitary matrix and S is defined in block structure as
where \(I\in {{\mathbb{R}}}^{N/2\times N/2}\) is the identity matrix and \({I}_{-}\in {{\mathbb{R}}}^{N/2\times N/2}\) is the matrix with ones along its subdiagonal. The unitary matrix U was generated as the left singular vector matrix of a random normal matrix.
Each block of S corresponds to the sequential dynamics for one of the two noninterfering sequences. The specific sequence is selected by the initial state of the network. This is parameterized through the first and ( N /2 + 1)th columns of U (that is, U 1 and U N /2+1 ), which correspond to the beginning of each sequence. The RNN also receives a 2D input that is condition independent. To avoid interference with the sequences, we mapped the input through the ( N /2)th and the N th columns of U (that is, U N /2 and U N ), as these are the elements corresponding to the end of the sequence. In this way, we were able to generate RNN dynamics that produce condition-specific sequences while also being influenced by condition-independent inputs.
To test the effects of different sources of noise, we considered RNN dynamics that are governed by stochastic differential equations. On trial k , the population activity \({{{{\bf{x}}}}}^{(k)}(t)\in {{\mathbb{R}}}^{N}\) and inputs \({{{{\bf{u}}}}}^{(k)}(t)\in {{\mathbb{R}}}^{2}\) evolve according to
where \(B=[{U}_{N/2},{U}_{N}],\;{A}_{ij} \sim {{{\mathcal{N}}}}(0,1/2)\) and d W i are infinitesimal increments of a Wiener process. Furthermore, we took \([{c}_{1}^{(k)},{c}_{1}^{(k)}]=[\cos ({\theta }^{(k)}),\sin ({\theta }^{(k)})]\) , where θ ( k ) represents the angle of the task variable. In our simulations, we used K = T = N = 200 and took ϕ = id.
RNNs have three natural sources of noise: (1) noise at the level of the dynamics of each neuron, σ 1 d W 1 , which we call intrinsic noise; (2) input noise, σ 2 d W 2 ; and (3) observation noise added to the full tensor, Y = X + η , where \({{{{{\eta }}}}}_{ijk} \sim {{{\mathcal{N}}}}(0,{\sigma }_{3})\) . Thus, by systematically varying σ 1 , σ 2 , σ 3 , we can vary the magnitude of different sources of noise in the data. Importantly, they have the property that for ϕ = id, \({\mathbb{E}}[{{{{Y}}}}\;]={{{{X}}}}\) , where y ( k ) ( t ) is the activity with σ i ≠ 0 for at least one i and x ( k ) ( t ) is the activity with σ i = 0 for all i .
To evaluate the effect of these different sources of noise on sliceTCA, we considered the variance explained \(\kappa =1-| | \hat{{{{{Y}}}}}-{{{{Y}}}}| {| }_{F}^{2}/| | {{{{Y}}}}-\bar{y}| {| }_{F}^{2}\) as a function of the noise level \(\zeta =| | {{{{Y}}}}-{{{{X}}}}| {| }_{F}^{2}/| | {{{{Y}}}}-\bar{y}| {| }_{F}^{2}\) , where \(\hat{{{{{Y}}}}}\) is the reconstruction from sliceTCA fit on Y . In the normalization term above, \(\bar{y}\in {\mathbb{R}}\) is the average over all N T K entries of Y (but we note that different marginalizations are possible 57 ). An optimal denoiser (that is, for which \(\hat{{{{{Y}}}}}={{{{X}}}}\) ) would yield κ = 1 − ζ . Meanwhile, a model that fully captures the variability (including noise) in the data (that is, \(\hat{{{{{Y}}}}}={{{{Y}}}}\) ) would have κ = 1.
Statistics and reproducibility
As we reanalyzed existing data, no statistical method was used to predetermine sample sizes. Instead, we demonstrated the utility of sliceTCA by choosing three previously published datasets representing a typical range of numbers of recorded neurons, time points and trials. For the application of sliceTCA to these example datasets and subsequent analyses, we randomly selected an example session and animal for each dataset. General trends were confirmed by fitting sliceTCA on other example sessions of the same dataset (not shown). To ensure reproducibility, we have made available the datasets for the sessions analyzed in this paper, along with the analysis code (see the ‘Data availability’ and ‘Code availability’ sections below). Model selection was performed as described in the ‘ Model selection, optimization and fitting ’ section above. During cross-validation, tensor entries (indexed by neurons, trials and blocks of time) were randomly allocated (80–20%) into training versus held-out data using a pseudo-random number generator. No blinding was performed, as our method is unsupervised and was applied to the full dataset. The investigators were not blinded to outcome assessment. Unless otherwise specified, we performed two-sided nonparametric statistical tests. In Extended Data Fig. 10d , model assumptions were not tested before performing analyses of variance. In dataset 3, we excluded neurons with low firing rates (<0.2 Hz); otherwise, no data were excluded from the analyses.
Dataset 1 of motor cortical recordings during a center-out and maze reaching task
Description of the dataset.
We analyzed a dataset of motor cortical (M1, n = 90) and premotor cortical (PMd, n = 92) electrophysiological recordings 17 . The dataset is curated and publicly available as part of the ‘Neural Latents Benchmark’ project 58 . Briefly, monkeys were trained to perform a delayed center-out reach task to one of 27 locations in both the maze condition (in which barriers were placed on the screen, leading to curved optimal reach trajectories) and the no-maze condition with matched target locations (classic center-out task leading to straight optimal reach trajectories). The go signal for movement initiation appeared 0–1,000 ms after target onset and 1,000–2,600 ms after the trial started with a fixation cue. We analyzed data from one animal (monkey J) in a single session and randomly subselected 12 target locations, resulting in K = 246 single-target trials in the maze reach conditions and K = 265 single-target trials in the 12 center-out reach conditions with matched target locations.
Additional preprocessing
We calculated firing rates for bins of 10 ms, which we then smoothed with a Gaussian filter with σ = 20 ms and rescaled to minimum and maximum values of 0 and 1 over the course of the experiment for each neuron separately. We selected a time period starting 1 s before movement onset (thus including a substantial part of the motor preparation period) and ending 0.5 s after movement onset when the monkey had successfully reached the target position in most trials. We did not time-warp the data. The resulting data tensor had dimensions of N = 182, T = 150 and K = 511.
Supervised mapping of neural population activity onto kinematic data
To identify the neural subspace from which 2D hand trajectories could be read out (Fig. 2a ), we used ordinary least squares (OLS). Specifically, we found weights that project the neuron-unfolded data from the full neural space onto a 2D subspace that best maps onto ( x , y ) hand velocity with a time delay of 100 ms to account for the lag between neural activity and movement. When testing the decoding analysis after dimensionality reduction, we instead applied OLS to the reconstruction (or partial reconstruction (that is, from a single slice type)) after reshaping it into an N × K T matrix. We also used OLS to project time-averaged pre-movement activity onto target locations (Fig. 2g ). For Fig. 2h , we used LDA to identify the dimension that best separates pre-movement averaged activity in clockwise versus counterclockwise curved reaches in the maze condition. To plot activity in a 3D neural subspace that contained information about the upcoming movement, we then orthogonalized the two axes that map neural activity onto target locations to the axis that distinguishes clockwise and counterclockwise movements.
For all decoding analyses, we calculated R 2 values on left-out trials in a fivefold cross-validation procedure performed on 20 permutations of the trials. Decoding was performed on data from the period spanning 250 ms before to 450 ms after movement onset. For trial-resolved data (Fig. 2a , raw data, neuron-slicing NMF, TCA, trial-slicing NMF), we averaged trial-wise R 2 values; for pre-movement information on target positions, we calculated a single R 2 value across trials for center-out and maze reaching conditions. For trial-averaged data (Fig. 2a , trial-averaged raw data), we performed twofold cross-validation by averaging hand and neural trajectories separately for each fold and then calculating R 2 values averaged over conditions and folds.
Visualization of sliceTCA weights
The results of fitting non-negative sliceTCA are shown in Fig. 2c,d and Supplementary Fig. 3 . Each component consists of a weight vector and a slice of corresponding weights on the other two variables. Along the trial dimension, we sorted trials by the angle of the target position and whether trials belonged to center-out or maze reaching conditions. Along the neuron dimension of trial-slicing components, neurons were sorted by the peak latency of neural activity in the first component. For the time-slicing component, neurons were sorted according to their mean activity in the first reaching condition.
Correlation matrices
To assess the encoding similarity of movement preparation in the time-slicing component, we calculated the K × K correlation matrix of the neural encoding weights (that is, the rows of the slice in Fig. 2d ) for different pairs of trials, separately for center-out and maze reach conditions, and for the PMd (Fig. 2f ) and M1 (Extended Data Fig. 4c ). We sorted the resulting correlation matrices by the angle of the target location (Fig. 2f ).
Dataset 2 of cortico-cerebellar calcium imaging during a motor task
We analyzed recently published calcium imaging data consisting of simultaneously recorded cerebellar granule cells ( n = 134) and premotor cortical L5 pyramidal cells ( n = 152) from a head-fixed mouse performing a motor task in which a manipulandum had to be moved forward and leftward or rightward for a reward 18 . After a correct movement was completed, a water reward was delivered with a 1-s delay, followed by an additional 3.5-s intertrial interval. Left versus right rewarded turn directions were alternated without a cue after 40 successful trials. We analyzed data from one session of a mouse in an advanced stage of learning, comprising a total of K = 218 trials. The data were sampled at a 30-Hz frame rate. Calcium traces were corrected for slow drifts, z -scored and low-pass filtered 18 .
Owing to the freely timed movement period, we piecewise linearly warped data to the median interval lengths between movement onset, turn and movement end. The remaining trial periods were left unwarped and cut to include data from 1.5 s before movement onset until 2.5 s after reward delivery, resulting in a preprocessed N × T × K data tensor with N = 286, T = 150 and K = 218.
In Fig. 4b,c , we show the results of a fitted sliceTCA model. We further reordered trials in the trial–time slices according to trial type and neurons in the neuron–time slices according to the peak activity in the first trial-loading component. This allows for a visual comparison of the tiling structure across components. We used Mann–Whitney U tests on the time-averaged activity between reward and trial end in the trial–time slices. We used LDA to determine the classification accuracy for neuron identity (cerebellum versus cortex) based on the loading vector weights of the three neuron-slicing components found by sliceTCA. We similarly reported the classification accuracy of trial identity (error versus correct, left versus right) based on the loading vector weights of the trial-slicing components.
Matrix rank of slices
To determine whether sliceTCA finds components with higher matrix ranks compared to methods that do not demix slice types (neuron-slicing PCA and factor analysis with neuron loadings, neuron- and time-concatenated PCA and factor analysis with trial loadings), we performed SVD on the six slices (after centering) of the sliceTCA model shown in Fig. 4b , as well as on the scores of either trial-slicing or neuron-slicing PCA and factor analysis, after refolding the resulting scores into N × T or K × T matrices, respectively. We then compared these to the spectra of squared singular values obtained from the slices of the trial-slicing (Fig. 4e ) or neuron-slicing components (Supplementary Fig. 8 ). Factor analysis was performed using the ‘sklearn’ Python package 59 , which uses an SVD-based solver. For comparability with PCA and sliceTCA solutions, no factor rotations were performed.
Manifolds from sliceTCA reconstructions
To analyze the geometry of neural data, we reconstructed the low-slice-rank approximation of neural activity from the sliceTCA model separately for the cerebellum and premotor cortex. We then used LDA on both raw and reconstructed data to find the three axes that maximally separate left versus right correct trials between movement onset and reward (axis 1, Fig. 4g ), movement onset time versus the time of reward in all correct trials (axis 2), and the time of reward versus post-reward (axis 3). We orthonormalized the three axes and projected raw and reconstructed data onto the new, 3D basis (Fig. 4h ).
We then measured the distance ratio to compare the distance between trials of the same class versus the distance between trials of distinct classes (left versus right) in the full neural space. For the reconstructed versus the full dataset, we averaged neural activity over a 650-ms window centered at movement onset and measured the Euclidean distance of the population response in each trial to the trial-averaged population response in its own trial type, compared to the Euclidean distance to the average population response of the respective other trial type: Δ between / Δ within , where \({\varDelta}_{{{{\rm{within}}}}}={d}({x}_{k}^{\,{L}},{\bar{x}}^{\,{L}})\) is the Euclidean distance between population vectors in each left trial to the mean population vector across all left trials (and vice versa for right trials), and \({\varDelta }_{{{{\rm{between}}}}}={{d}}({x}_{k}^{\,{L}},{\bar{x}}{\,}^{R})\) is the Euclidean distance of population vectors in each left trial to the mean population vector across all right trials (and vice versa for right trials).
Dataset 3 of electrophysiology across many brain regions during perceptual decision-making
The third analyzed dataset comprised recently published multiregion Neuropixels recordings ( n = 303) in mice performing a perceptual decision-making task 53 . In the task, mice were presented a grating patch image with varying contrast (0%, 25%, 35%, 50% or 100%), shown on the left or right sides of a screen. The mice were trained to move the image to the center of the screen using a steering wheel within a 60-s period to receive a sugary water reward. A correct response was registered if the stimulus was moved to the center, whereas an incorrect response was recorded if the stimulus was moved to the border of the screen. We selected a single example mouse (subject CSHL049 from the openly released electrophysiology data repository).
We binned single-neuron spiking events in 10-ms windows. Owing to the variable response times across trials, we piecewise linearly warped data between stimulus onset and reward delivery or timeout onset to correspond to the median interval length and clipped the trial period to start 1 s before stimulus onset and to end 2 s after reward delivery or timeout onset. We smoothed data with a Gaussian filter with σ = 20 ms and rescaled the activity of each neuron to a minimal and maximal value of 0 and 1 over all trials. We excluded neurons with mean firing rates below 0.2 Hz, leading to a total of n = 221 neurons analyzed of n = 303 neurons recorded. Brain regions included the visual cortex (anterior layers 2/3, 4, 5, 6a and 6b as well as anteromedial layers 2/3, 4, 5 and 6a; n = 85 neurons), hippocampal regions CA1 ( n = 32 neurons) and dentate gyrus (molecular, polymorph and granule cell layers; n = 21 neurons), thalamus (including the posterior limiting nucleus and lateral posterior nucleus; n = 18 neurons) and the anterior pretectal and midbrain reticular nucleus (anterior pretectal nucleus, n = 22 neurons; midbrain reticular nucleus, n = 35 neurons) of the midbrain. In total, the resulting data tensor had dimensions of N = 221, T = 350 and K = 831.
In Fig. 5b , we scaled the rows of the neuron–time slices to a [0, 1] interval to highlight differences in the timing of peak activity between neurons. We then reordered neuron–time slices by the peak activity within each region for each slice type separately to show characteristic differences between neural correlates of behavioral variables. Trial–time slices were regrouped by trial type to show region-specific representations of task variables. Finally, neuron–trial slices were reordered by the average weights across the first 100 trials for each neuron within a region.
Reconstruction performance and component weights
For each neuron, we estimated the goodness of fit of the sliceTCA reconstruction as
We then quantified the contribution of the neuron-slicing components on the total sliceTCA reconstruction for each neuron n as the following ratio:
where \({\hat{{{{{X}}}}}}{\,}^{{{{\rm{neuron}}}}}\) describes the partial reconstruction of the data tensor from only the neuron-slicing components. We similarly defined the contributions of the time- and trial-slicing components to the sliceTCA reconstruction of each neuron n as \({f}{\,}_{\!n}^{{{{\rm{time}}}}}\) and \({f}{\,}_{\!n}^{{{{\rm{trial}}}}}\) .
Reporting summary
Further information on research design is available in the Nature Portfolio Reporting Summary linked to this article.
Data availability
The datasets presented in this paper are available via figshare at https://doi.org/10.6084/m9.figshare.24961917.v1 (ref. 60 ). Source data are available via GitHub at https://github.com/caycogajiclab/sliceTCA_paper (ref. 61 ).
Code availability
A GPU-accelerated Python library for the sliceTCA data analysis pipeline (including preprocessing, model selection, model optimization and visualization of components) is available as a pip installable package at https://github.com/arthur-pe/slicetca (ref. 62 ). Additionally, code for the analyses in this paper is available at https://github.com/caycogajiclab/sliceTCA_paper (ref. 61 ).
Cunningham, J. P. & Yu, B. M. Dimensionality reduction for large-scale neural recordings. Nat. Neurosci. 17 , 1500–1509 (2014).
Article CAS PubMed PubMed Central Google Scholar
Panzeri, S., Moroni, M., Safaai, H. & Harvey, C. D. The structures and functions of correlations in neural population codes. Nat. Rev. Neurosci. 23 , 551–567 (2022).
Article CAS PubMed Google Scholar
Jazayeri, M. & Ostojic, S. Interpreting neural computations by examining intrinsic and embedding dimensionality of neural activity. Curr. Opin. Neurobiol. 70 , 113–120 (2021).
Williams, A. H. et al. Unsupervised discovery of demixed, low-dimensional neural dynamics across multiple timescales through tensor component analysis. Neuron 98 , 1099–1115 (2018).
Harshman, R. A. et al. Foundations of the PARAFAC procedure: models and conditions for an ‘explanatory’ multimodal factor analysis. UCLA Work. Paper Phonet. 16 , 1–84 (1970).
Google Scholar
Carroll, J. D. & Chang, J.-J. Analysis of individual differences in multidimensional scaling via an n -way generalization of ‘Eckart–Young’ decomposition. Psychometrika 35 , 283–319 (1970).
Article Google Scholar
Seely, J. S. et al. Tensor analysis reveals distinct population structure that parallels the different computational roles of areas M1 and V1. PLoS Comput. Biol. 12 , e1005164 (2016).
Article PubMed PubMed Central Google Scholar
Pastalkova, E., Itskov, V., Amarasingham, A. & Buzsáki, G. Internally generated cell assembly sequences in the rat hippocampus. Science 321 , 1322–1327 (2008).
Peters, A. J., Chen, S. X. & Komiyama, T. Emergence of reproducible spatiotemporal activity during motor learning. Nature 510 , 263–267 (2014).
Okubo, T. S., Mackevicius, E. L., Payne, H. L., Lynch, G. F. & Fee, M. S. Growth and splitting of neural sequences in songbird vocal development. Nature 528 , 352–357 (2015).
Harvey, C. D., Coen, P. & Tank, D. W. Choice-specific sequences in parietal cortex during a virtual-navigation decision task. Nature 484 , 62–68 (2012).
Hennig, J. A. et al. How learning unfolds in the brain: toward an optimization view. Neuron 109 , 3720–3735 (2021).
Gurnani, H. & Cayco Gajic, N. A. Signatures of task learning in neural representations. Curr. Opin. Neurobiol. 83 , 102759 (2023).
Rule, M. E., O’Leary, T. & Harvey, C. D. Causes and consequences of representational drift. Curr. Opin. Neurobiol. 58 , 141–147 (2019).
Driscoll, L. N., Pettit, N. L., Minderer, M., Chettih, S. N. & Harvey, C. D. Dynamic reorganization of neuronal activity patterns in parietal cortex. Cell 170 , 986–999 (2017).
Schoonover, C. E., Ohashi, S. N., Axel, R. & Fink, A. J. Representational drift in primary olfactory cortex. Nature 594 , 541–546 (2021).
Churchland, M. M. et al. Neural population dynamics during reaching. Nature 487 , 51–56 (2012).
Wagner, M. J. et al. Shared cortex–cerebellum dynamics in the execution and learning of a motor task. Cell 177 , 669–682 (2019).
International Brain Laboratory et al. Reproducibility of in-vivo electrophysiological measurements in mice. Preprint at bioRxiv https://doi.org/10.1101/2022.05.09.491042 (2022).
Poort, J. et al. Learning enhances sensory and multiple non-sensory representations in primary visual cortex. Neuron 86 , 1478–1490 (2015).
Chadwick, A. et al. Learning shapes cortical dynamics to enhance integration of relevant sensory input. Neuron 111 , 106–120 (2023).
Vinck, M., Batista-Brito, R., Knoblich, U. & Cardin, J. A. Arousal and locomotion make distinct contributions to cortical activity patterns and visual encoding. Neuron 86 , 740–754 (2015).
Tao, T. & Sawin, W. Notes on the ‘slice rank’ of tensors. WordPress terrytao.wordpress.com/2016/08/24/notes-on-the-slice-rank-of-tensors/ (2016).
Goldman, M. S. Memory without feedback in a neural network. Neuron 61 , 621–634 (2009).
Mackevicius, E. L. et al. Unsupervised discovery of temporal sequences in high-dimensional datasets, with applications to neuroscience. eLife 8 , e38471 (2019).
Shenoy, K. V., Sahani, M. & Churchland, M. M. Cortical control of arm movements: a dynamical systems perspective. Annu. Rev. Neurosci. 36 , 337–359 (2013).
Keshtkaran, M. R. et al. A large-scale neural network training framework for generalized estimation of single-trial population dynamics. Nat. Methods 19 , 1572–1577 (2022).
Stringer, C., Pachitariu, M., Steinmetz, N., Carandini, M. & Harris, K. D. High-dimensional geometry of population responses in visual cortex. Nature 571 , 361–365 (2019).
Lanore, F., Cayco-Gajic, N. A., Gurnani, H., Coyle, D. & Silver, R. A. Cerebellar granule cell axons support high-dimensional representations. Nat. Neurosci. 24 , 1142–1150 (2021).
Gillis, N. Nonnegative Matrix Factorization (SIAM, 2020).
Williams, A. H., Degleris, A., Wang, Y. & Linderman, S. W. Point process models for sequence detection in high-dimensional neural spike trains. Adv. Neural Inf. Process. Syst. 33 , 14350–14361 (2020).
PubMed PubMed Central Google Scholar
Williams, A. H. et al. Discovering precise temporal patterns in large-scale neural recordings through robust and interpretable time warping. Neuron 105 , 246–259 (2020).
Parker, N. F. et al. Choice-selective sequences dominate in cortical relative to thalamic inputs to NAc to support reinforcement learning. Cell Rep. 39 , 110756 (2022).
Zhou, S., Masmanidis, S. C. & Buonomano, D. V. Neural sequences as an optimal dynamical regime for the readout of time. Neuron 108 , 651–658 (2020).
Feng, T., Silva, D. & Foster, D. J. Dissociation between the experience-dependent development of hippocampal theta sequences and single-trial phase precession. J. Neurosci. 35 , 4890–4902 (2015).
Lakshmanan, K. C., Sadtler, P. T., Tyler-Kabara, E. C., Batista, A. P. & Yu, B. M. Extracting low-dimensional latent structure from time series in the presence of delays. Neural Comput. 27 , 1825–1856 (2015).
Koay, S. A., Charles, A. S., Thiberge, S. Y., Brody, C. D. & Tank, D. W. Sequential and efficient neural-population coding of complex task information. Neuron 110 , 328–349 (2022).
Amo, R. et al. A gradual temporal shift of dopamine responses mirrors the progression of temporal difference error in machine learning. Nat. Neurosci. 25 , 1082–1092 (2022).
Sabatini, D. A. & Kaufman, M. T. A curved manifold orients rotational dynamics in motor cortex. Preprint at bioRxiv https://doi.org/10.1101/2021.09.09.459647 (2021).
Renart, A. & Machens, C. K. Variability in neural activity and behavior. Curr. Opin. Neurobiol. 25 , 211–220 (2014).
Dyer, E. L. et al. A cryptography-based approach for movement decoding. Nat. Biomed. Eng. 1 , 967–976 (2017).
Gallego, J. A., Perich, M. G., Chowdhury, R. H., Solla, S. A. & Miller, L. E. Long-term stability of cortical population dynamics underlying consistent behavior. Nat. Neurosci. 23 , 260–270 (2020).
Lindsay, G. W., Mrsic-Flogel, T. D. & Sahani, M. Bio-inspired neural networks implement different recurrent visual processing strategies than task-trained ones do. Preprint at bioRxiv https://doi.org/10.1101/2022.03.07.483196 (2022).
Williams, A. H., Kunz, E., Kornblith, S. & Linderman, S. Generalized shape metrics on neural representations. In Proc. 35th Conference on Neural Information Processing Systems (NeurIPS 2021) (eds Ranzato, M. et al.) 4738–4750 (Curran Associates, 2021); proceedings.neurips.cc/paper/2021/file/252a3dbaeb32e7690242ad3b556e626b-Paper.pdf
Naslund, E. The partition rank of a tensor and k -right corners in \({{\mathbb{F}}}_{q}^{n}\) . J. Comb. Theory Ser. A 174 , 105190 (2019).
Kolda, T. G. & Bader, B. W. Tensor decompositions and applications. SIAM Rev. 51 , 455–500 (2009).
Bläser, M., Ikenmeyer, C., Lysikov, V., Pandey, A. & Schreyer, F. Variety membership testing, algebraic natural proofs, and geometric complexity theory. Preprint at https://arxiv.org/abs/1911.02534 (2019).
Wang, J., Narain, D., Hosseini, E. A. & Jazayeri, M. Flexible timing by temporal scaling of cortical responses. Nat. Neurosci. 21 , 102–110 (2018).
Williams, A. H. Combining tensor decomposition and time warping models for multi-neuronal spike train analysis. Preprint at bioRxiv https://doi.org/10.1101/2020.03.02.974014 (2020).
Kuchibhotla, K. V. et al. Dissociating task acquisition from expression during learning reveals latent knowledge. Nat. Commun. 10 , 2151 (2019).
Smith, M. A.-Y., Honegger, K. S., Turner, G. & de Bivort, B. Idiosyncratic learning performance in flies. Biol. Lett. 18 , 20210424 (2022).
Churchland, M. M., Cunningham, J. P., Kaufman, M. T., Ryu, S. I. & Shenoy, K. V. Cortical preparatory activity: representation of movement or first cog in a dynamical machine? Neuron 68 , 387–400 (2010).
International Brain Laboratory et al. Standardized and reproducible measurement of decision-making in mice. eLife 10 , e63711 (2021).
Kingma, D. P. & Ba, J. Adam: a method for stochastic optimization. Preprint at https://arxiv.org/abs/1412.6980 (2014).
Gillis, N. Sparse and unique nonnegative matrix factorization through data preprocessing. J. Mach. Learn. Res. 13 , 3349–3386 (2012).
Tomasi, G. & Bro, R. A comparison of algorithms for fitting the PARAFAC model. Comput. Stat. Data Anal. 50 , 1700–1734 (2006).
Kobak, D. et al. Demixed principal component analysis of neural population data. eLife 5 , e10989 (2016).
Pei, F. et al. Neural Latents Benchmark ’21: evaluating latent variable models of neural population activity. In Proc. Neural Information Processing Systems Track on Datasets and Benchmarks 1 (NeurIPS Datasets and Benchmarks 2021) (eds Vanschoren, J. & Yeung, S.) (Curran Associates, 2021); datasets-benchmarks-proceedings.neurips.cc/paper_files/paper/2021/file/979d472a84804b9f647bc185a877a8b5-Paper-round2.pdf
Pedregosa, F. et al. Scikit-learn: machine learning in Python. J. Mach. Learn. Res. 12 , 2825–2830 (2011).
Stein, H. Pellegrino_Stein_Cayco-Gajic_2024_Nat-Neuro. figshare https://doi.org/10.6084/m9.figshare.24961917.v1 (2024).
Stein, H. & Pellegrino, A. caycogajiclab/sliceTCA_paper. GitHub github.com/caycogajiclab/sliceTCA_paper (2023).
Stein, H. & Pellegrino, A. arthur-pe/slicetca. GitHub github.com/arthur-pe/slicetca (2023).
Download references
Acknowledgements
We thank J. Barbosa, M. Hennig, K. Kuchibhotla, A. Litwin-Kumar, A. Onken, Y. Sweeney and the members of the Cayco-Gajic laboratory for comments on the paper. We additionally thank A. Chadwick for helpful discussions on an early stage of the paper and M. Wagner for sharing his data. We are also grateful to the IBL, the Churchland/Shenoy laboratories and the Neural Latents Benchmark project for making their processed and curated data freely available. We thank the NeuroCAAS team for helping with fitting and assessing autoLFADS. This work was funded by the European Molecular Biology Organization (ALTF 471-2021 to H.S.) and the Agence Nationale de la Recherche (ANR-20-CE37-0004 and ANR-17-EURE-0017 to N.A.C.-G.). The funders had no role in study design, data collection and analysis, decision to publish or preparation of the manuscript.
Author information
These authors contributed equally: Arthur Pellegrino, Heike Stein.
Authors and Affiliations
Laboratoire de Neurosciences Cognitives et Computationnelles, INSERM U960, Département D’Etudes Cognitives, Ecole Normale Supérieure, PSL University, Paris, France
Arthur Pellegrino, Heike Stein & N. Alex Cayco-Gajic
Institute for Adaptive and Neural Computation, School of Informatics, University of Edinburgh, Edinburgh, UK
Arthur Pellegrino
You can also search for this author in PubMed Google Scholar
Contributions
A.P., H.S. and N.A.C.-G. conceptualized the project. A.P. and H.S. designed the data analysis pipeline. A.P. and H.S. performed data analysis investigations. A.P., H.S. and N.A.C.-G. designed the feedforward model. A.P. wrote the mathematical notes. N.A.C.-G. wrote an initial draft of the paper, which all authors reviewed and revised. N.A.C.-G. supervised the project.
Corresponding authors
Correspondence to Arthur Pellegrino or N. Alex Cayco-Gajic .
Ethics declarations
Competing interests.
The authors declare no competing interests.
Peer review
Peer review information.
Nature Neuroscience thanks Alex Williams and the other, anonymous, reviewer(s) for their contribution to the peer review of this work.
Additional information
Publisher’s note Springer Nature remains neutral with regard to jurisdictional claims in published maps and institutional affiliations.
Extended data
Extended data fig. 1 tensor decomposition, matrix factorization, and covariability classes..
a . A neuron-slicing component can be converted into a rank-1 matrix by unfolding the slice into a row vector (or equivalently, by unfolding the reconstructed 3-tensor into a matrix). A sliceTCA model comprised only of neuron-slicing components (that is, R time = R trial = 0) is equivalent to applying matrix factorization after unfolding the data tensor into an N × K T matrix (for example, as in ‘trial-concatenated PCA’). Therefore, a neuron-slicing component can be interpreted as a latent trajectory that varies over trials but which has fixed neural encoding weights. b-c . The trial-slicing ( b ) and time-slicing ( c ) components can similarly be converted to rank-1 matrices. These components represent covariability across trials and time points, respectively. d . A TCA component can be represented as any of the three sliceTCA components by defining the slice to be the outer product of two of the loading vectors. This means that a single TCA component simultaneously corresponds to covariability across neurons, time, and trials.
Extended Data Fig. 2 Feedforward model of perceptual learning.
a . Sample of the activity of a single neuron over six example trials (grey) as well as trial-averaged activity (red). b . Evolution of the mean Go and No-go weight over learning. Shading represents the standard error of the mean. c . Recovered neuron-slicing (left) and time-slicing (right) components, plotted alongside ground truth values (grey). Weights for each neuron in the slice of the time-slicing component are plotted separately for Go (green) and No-go (red) trials. The sliceTCA decomposition captures the ground truth exactly. d . Single neuron activity is better captured by sliceTCA with (bottom) and without (top) white noise added to the data tensor. e . Mean squared error of decomposing the activity of the feedforward model with added white noise for sliceTCA, TCA, and PCA as a function of the number of components used in the model. The dashed line represents the mean squared deviation between the noisy and the noiseless model. Adding white noise does not affect the performance of sliceTCA relative to TCA or PCA.
Extended Data Fig. 3 Linear RNN model of condition-specific neural sequences.
a . Top: Two sequences are encoded into neural weights using the Schur decomposition (24). Bottom right: The initial condition continuously parametrizes which sequence is activated. Bottom left: In addition, the RNN receives a two-dimensional task-irrelevant stochastic input that varies from trial to trial. b . Sequential activity during a single trial, neurons sorted by peak activity. c . Singular values of the activity matrix. The two dominant singular values come from the two-dimensional input. Nevertheless, as expected from sequential activity, the singular value spectrum has a long, slowly-decaying tail (participation ratio of 7.57). In comparison, the singular values of the partial reconstruction from the two trial slicing components (which capture the neural sequences, see panel d) retain the long tail, confirming that the two dominant modes in the data are input-driven. d . SliceTCA components for R trial = R neuron = 2, R time = 0. Neurons are sorted according to their peak activity in the slice of the first component. e . Example of single-neuron activity in the raw data and the SliceTCA reconstruction. f . Comparison of the variance of the activity captured by sliceTCA, TCA, and PCA with or without intrinsic noise (mean variance plus/minus standard deviation over n = 10 simulations of the RNN). g . Cross-validated variance explained by fitting sliceTCA ( R trial = R neuron = 2, R time = 0) to the RNN activity while systematically varying the level of noise added at the level of the circuit dynamics (intrinsic noise, red), low-dimensional inputs (input noise, blue), or neural activity itself (observation noise, green).
Extended Data Fig. 4 Analysis of the mixed covariability model used to decode reaching kinematics from motor cortical recordings (Dataset 1).
a . Ordinary least squares decoding of the hand velocity from different decompositions. For comparison, hand position and the mapping from the reconstruction of the trial-slicing only NMF model ( R neuron = 0, R time = 0, R trial = 12) are reproduced from Fig. 2 . Also shown are the decoding performances using the fit of the mixed covariability model ( R neuron = 0, R time = 1, R trial = 12), based on the full reconstruction of all components in the model, as well as the partial reconstruction of the data tensor from only the time-slicing component or only the trial-slicing components. Note that adding the single time component to the mixed model before decoding decreases performance, indicating that it captures variability in the neural data that is not directly relevant to movement. Finally, we show the decoding performance based on the reconstruction from autoLFADS (27, fitted on the cloud-based NeuroCAAS platform; https://neurocaas.org/analysis/20 ). b . Cross-validated model selection. Top: The cross-validated loss is displayed as a function of the number of time- and trial-slicing components (for 0 neuron-slicing components). Bottom: Cross-validated loss as a function of the number of neuron- and trial-slicing components (for 0 time-slicing components). For 10 random seeds, data tensors were split in train- and test-data 80% and 20%, respectively), following a blocked masking procedure (Methods). Full black star marks the optimal model, and hollow black star marks the selected model analyzed here and in Fig. 2 . Black thresholds represents the 95% loss elbow. c . Reach condition-specific structure in motor preparatory information is observed in time-slicing weights of PMd, but not of M1. Top: Correlation matrix between neural encoding weights for pairs of trials in the time-slicing matrix, showing similarity of PMd neuron weights for trials with similar reach direction and curvature (left/right indicate no maze/maze conditions, cf. Fig. 2f) . Bottom: In contrast, M1 neuron weights reveal a lack of structure in its correlation matrices. d . Average fraction of the variance of PMd and M1 neuron activity explained by the single time-slicing component, separated by maze and no maze conditions. e . Comparison of sliceTCA and time-slicing NMF. Left: SliceTCA time-slicing component ( R trial = 12, R time = 1, R neuron = 0), cf. components of NMF applied to the time-unfolded data tensor for R time = 1 (middle) and R time = 13 (right). Neither time-slicing NMF model appears to capture pre-movement preparatory activity.
Extended Data Fig. 5 Schematic of the two sliceTCA invariant transformations.
a . Example of a within-slice-type invariant transformation in a slice-rank-2 tensor formed by adding and subtracting a slice-rank-1 component with the same loading vector as component 1 and the same slice as component 2. These terms can be absorbed into the original two components, resulting in two equivalent decompositions. b . Example of a between-slice-type invariant transformation in a slice-rank-2 tensor. Here, a rank-1 tensor constructed by the two components’ loading vectors (green and yellow) and a third free vector (blue) is added and subtracted. These terms can be absorbed into the original two components, resulting in two equivalent decompositions.
Extended Data Fig. 6 Between-slice-type invariance in the feedforward model.
a . The activity of the feedforward model (Extended Data Figure 2 ) can be decomposed into the sum of a time-slicing component (top) and a neuron-slicing component (bottom). In this example, the Gaussian Process is not rectified and the data is not non-negative, leading to bleedthrough between the two components due to this invariance class (bottom, red rectangle shows discrepancy between model and ground truth). This invariance class was not observed in the original non-negative model (Fig. 1e–g , Extended Data Figure 2 ) because there are fewer permissible transformations when the factors are more constrained. b . Example of a rank-1 tensor that can be passed between the two components. Two of the loading vectors are identical to the loading vectors of the components in a. The third is unconstrained. c . By choosing the trial loading that minimizes a specified objective function, a unique solution can be found. Here the correlation between the activity of trial-neuron pairs was minimized in the neuron slicing component, resulting in a fit that matches the ground-truth values.
Extended Data Fig. 7 Drifting neural sequences have high slice rank.
a . Over trials, the time point at which a neural sequence starts continuously drifts later and later within the trial. In the model, the activity at trial i ∈ {1, …, K } for neuron j ∈ {1, …, N } and time point k ∈ {1, …, T } is given by \({X}_{ijk}={{{\exp}}}\left({-{\left(\frac{i+j-k}{\sigma T}\right)}^{2}}\right)\) . Here we used T = 2 N = 2 K , N = 100, σ = 0.1. b . Left: A neuron that activates early in the sequence begins to be activated later and later in time, for increasing trials. Right: similar drift for a neuron that fires late in the sequence. Therefore, in addition to there being a sequence of neural activation whose timing drifts from trial to trial (as in panel a), there is equivalently a sequence of activations over trials whose timing drifts from neuron to neuron. This symmetry of the sequences in the data tensor means that it can neither be captured by few neuron slicing components nor by few trial slicing components. c . SliceTCA gridsearch for the optimal number of components (fit on the full tensor, not cross-validated). The loss continues to decrease indefinitely as the number of components increases. d . Cross-validated sliceTCA gridsearch for the optimal number of components. Retaining blocks of trial x neuron x time creates a test set that is nearly completely decorrelated (in neuron, trial or time) from the train set; thus the test loss is close to random and larger in magnitude than in panel c due to overfitting.
Extended Data Fig. 8 SliceTCA model selection and optimization.
a . Cross-validated model selection procedure. We randomly assign blocks of consecutive time points (blue) within the data tensor as held out data. The remaining entries of the tensor are used as training data (white). To reduce correlations between the training and testing data, we discard a brief period from the ends of the held-out blocks (light blue) from both training and testing. We use only the interiors of these blocks as test data (dark blue). We run a 3D grid search on the cross-validated loss (right). We either choose the optimal model (red star) or a model at the ‘elbow’ of the loss function (red circle). b . Validation of the cross-validation procedure. For ten random seeds, data tensors were split in training data and held out data (80% and 20%, respectively), following a blocked masking procedure as described in Methods. Grid shows mean squared error loss for different numbers of components of each slice type, averaged across ten cross-validation folds. The ground truth model was a tensor formed by approximating the cortico-cerebellar dataset tensor using a decomposition of R neuron = 3, R time = 1, R trial = 2 and subsequently adding independent Gaussian noise ( σ = 0.1) to each tensor entry. The grid search recovers the correct number of components of each slice type (black star indicates optimal model). c . Hierarchical model optimization procedure. Schematic illustrates the three optimization stages to be performed in sequence (gray represents the entries which change at each stage). i. We first fit the model on all data (optimizing the MSE or \({{{{\mathcal{L}}}}}_{1}\) loss), resulting in the approximation \(\hat{{{{{X}}}}}\) to the data tensor X. ii. Next, we optimize the auxiliary loss \({{{{\mathcal{L}}}}}_{2}\) (a function of the partial reconstructions \({\hat{{{{{X}}}}}}{\,}^{{{{\rm{neuron}}}}}\) , etc.) in order to find a solution to the between-slice-type invariances. This can be considered as a regularization step that affects the relative weighting of each slice type. iii. Finally, the auxiliary loss function \({{{{\mathcal{L}}}}}_{3}\) (a function of the loading vectors and slices, for example, u ( r ) and A ( r ) ) is optimized to solve the factor rotation problem for each slice type separately (here, shown for the neuron slice type components). d . Results of running the tensor-passing ( \({{{{\mathcal{L}}}}}_{2}\) ) optimization step on the decomposition shown in Fig. 4 , with 10 random seeds, so that the free vector of the rank-1 tensor was initialized differently each time. During optimization, the free vector is optimized to minimize the L2-norm of slices. Over 250 iterations, the optimization procedure lead to a unique solution, observable in a perfect correlation between slice weights both for trial- (green, top) and neuron-slicing components (blue, bottom). Each transparent line is the pairwise correlation between two solutions found based on 10 different initialization seeds. e-h . Model similarity scores for all three datasets. For a given number of components, we fitted ten sliceTCA models from different parameter initializations. We then compared the similarity between the model with the lowest mean squared error (MSE) and all other models using a metric originally proposed in 56, and adapted from 4. For this, we unfolded each component’s slice into a vector and computed two angles per component: one corresponding to the loading vectors of the two models, and another corresponding to the unfolded slices. Components were matched using the Hungarian algorithm (as in 4). We report a similarity score that is averaged over all components (‘full model’) as well as slice type-specific similarity scores. Single small dots are scores for models from different initializations, color-coded by MSE (brighter colors indicate higher MSE). Lines denote average chance level over 100 shuffle repetitions, for which the elements of vectors of one component were permuted before computing the similarity score. e . Similarity scores for reaching dataset (Dataset 1, shown in Fig. 2 ). See Supplementary Figure 6 to see components of the lowest-similarity model. f . Similarity scores for multi-region dataset during decision-making (Dataset 3, shown in Fig. 5 ). g-h . Similarity scores for cerebello-cortical imaging data (Dataset 2, shown in Fig. 4 ) before ( g ) and after ( h ) hierarchical optimization of \({{{{\mathcal{L}}}}}_{2}\) and \({{{{\mathcal{L}}}}}_{3}\) to identify a unique solution.
Extended Data Fig. 9 Cross-validated model selection and comparison to TCA for cerebello-cortical imaging data (Dataset 2).
a . The cross-validated loss as a function of the number of time-slicing components. Transparent grey lines show different combinations of 0-5 neuron- and 0-5 trial-slicing components. This indicates that adding any time-slicing components leads to an overfitting of the data. b . The cross-validated loss as a function of the number of neuron- and trial-slicing components (for 0 time-slicing components). The filled star indicates the minimum loss (that is, the optimal model), while the black line indicates the 80% loss elbow. The hollow star indicates the model selected for further analysis in Fig. 4 . c . TCA applied to the cerebello-cortical dataset. Cross-validated loss as a function of the number of TCA components (grey curves indicate ten different cross-validation folds, with the mean shown in black). The solid red line shows the performance of the sliceTCA model shown in Fig. 4 (that is, at the elbow of the sliceTCA loss), while the dashed red line shows the optimal sliceTCA model (components shown in Supplementary Figure 7 , dashed red line). d . Factors of the 6-component TCA model. Note that the first three and last three components show redundancy in the trial factors, while the corresponding time factors cover different periods of the task.
Extended Data Fig. 10 Cross-validated model selection, effects of temporal warping, and comparison to TCA for multi-region recordings during decision making (Dataset 3).
a . Cross-validated model selection. For 10 random seeds, data tensors were split in train- and test-data (80% and 20%, respectively), following a blocked masking procedure as described in Methods. Full black star marks the optimal model, and hollow black star marks the selected model analyzed in Fig. 5 . Black thresholds describe a 95% loss elbow. b . Effect of piecewise linear warping on the example APN neuron shown in Fig. 5c . Trials are ordered by the time of reward (white line; for visibility of neural responses, line has been shifted left by 100 ms). c . Single-neuron response of the same ARN neuron in correct vs. incorrect trials without time-warping (c.f. Fig. 5c ). d . To determine whether the three time-slicing components found by sliceTCA reconstructed an increasing baseline for most neurons or whether responses in single task periods changed more strongly than others, we compared the slope of linear trends over trials (for each neuron separately) across the three time-slicing components. Specifically, we fitted linear models with a multiplicative term for each neuron: w k , r = β 0 + β 1 k + β 2 δ r =2 + β 3 δ r =3 + β 4 k δ r =2 + β 5 k δ r =3 + ε k , r where w k , r is the weight in trial k for component r , and δ r =2 = 1 if the weight belongs to component 2, δ r =2 = 0 otherwise (same for δ r =3 , whereas component 1 is the reference class). We performed analyses of variance for each neuron to test whether multiplicative terms explained a significant part of variance (at a Bonferroni-corrected significance level α = 0.05 with n = 221 neurons). We discarded neurons with non-significant multiplicative terms (grey). For neurons with significant differences in the rate of change across time-slicing, we compared rates of change between components. Each neuron was classified by the highest absolute change coefficient and its sign, leading to six classes for three time-slicing components. e . 8-component TCA model on multi-region Neuropixel dataset. Trial loading vectors are color-coded by the behavioral or task variable most correlated with the weights (if any). Similarly to sliceTCA, component 1 was correlated with trial outcome and component 2 with the reaction time. However, components 3-8 tile the sequence of trials but have little correlation with trial outcome, reaction time or the block switching structure of the IBL task.
Supplementary information
Supplementary information.
Supplementary Figs. 1–16 and mathematical notes.
Reporting Summary
Rights and permissions.
Open Access This article is licensed under a Creative Commons Attribution 4.0 International License, which permits use, sharing, adaptation, distribution and reproduction in any medium or format, as long as you give appropriate credit to the original author(s) and the source, provide a link to the Creative Commons licence, and indicate if changes were made. The images or other third party material in this article are included in the article’s Creative Commons licence, unless indicated otherwise in a credit line to the material. If material is not included in the article’s Creative Commons licence and your intended use is not permitted by statutory regulation or exceeds the permitted use, you will need to obtain permission directly from the copyright holder. To view a copy of this licence, visit http://creativecommons.org/licenses/by/4.0/ .
Reprints and permissions
About this article
Cite this article.
Pellegrino, A., Stein, H. & Cayco-Gajic, N.A. Dimensionality reduction beyond neural subspaces with slice tensor component analysis. Nat Neurosci (2024). https://doi.org/10.1038/s41593-024-01626-2
Download citation
Received : 06 December 2022
Accepted : 20 March 2024
Published : 06 May 2024
DOI : https://doi.org/10.1038/s41593-024-01626-2
Share this article
Anyone you share the following link with will be able to read this content:
Sorry, a shareable link is not currently available for this article.
Provided by the Springer Nature SharedIt content-sharing initiative
Quick links
- Explore articles by subject
- Guide to authors
- Editorial policies
Sign up for the Nature Briefing newsletter — what matters in science, free to your inbox daily.

- Systematic Review
- Open access
- Published: 15 May 2024
A systematic review and meta-analysis on mortality rate following total pelvic exenteration in cancer patients
- Arezoo Esmailzadeh ORCID: orcid.org/0000-0001-5999-9590 1 na1 ,
- Mohammad Sadegh Fakhari ORCID: orcid.org/0000-0002-0350-447X 2 na1 ,
- Nafise Saedi ORCID: orcid.org/0000-0001-8187-5738 3 ,
- Nasim Shokouhi ORCID: orcid.org/0000-0002-4746-8087 4 &
- Amir Almasi-Hashiani ORCID: orcid.org/0000-0003-4434-561X 5 , 6
BMC Cancer volume 24 , Article number: 593 ( 2024 ) Cite this article
Metrics details
Total pelvic exenteration (TPE), an en bloc resection is an ultraradical operation for malignancies, and refers to the removal of organs inside the pelvis, including female reproductive organs, lower urological organs and involved parts of the digestive system. The aim of this meta-analysis is to estimate the intra-operative mortality, in-hospital mortality, 30- and 90-day mortality rate and overall mortality rate (MR) following TPE in colorectal, gynecological, urological, and miscellaneous cancers.
This is a systematic review and meta-analysis in which three international databases including Medline through PubMed, Scopus and Web of Science on November 2023 were searched. To screen and select relevant studies, retrieved articles were entered into Endnote software. The required information was extracted from the full text of the retrieved articles by the authors. Effect measures in this study was the intra-operative, in-hospital, and 90-day and overall MR following TPE. All analyzes are performed using Stata software version 16 (Stata Corp, College Station, TX).
In this systematic review, 1751 primary studies retrieved, of which 98 articles (5343 cases) entered into this systematic review. The overall mortality rate was 30.57% in colorectal cancers, 25.5% in gynecological cancers and 12.42% in Miscellaneous. The highest rate of mortality is related to the overall mortality rate of colorectal cancers. The MR in open surgeries was higher than in minimally invasive surgeries, and also in primary advanced cancers, it was higher than in recurrent cancers.
In conclusion, it can be said that performing TPE in a specialized surgical center with careful patient eligibility evaluation is a viable option for advanced malignancies of the pelvic organs.
Peer Review reports
Introduction
Total pelvic exenteration (TPE), an en bloc resection is an ultraradical operation for malignancies which was performed for the first time in 1946 by Alexander Brunschwig [ 1 ], and refers to the removal of organs inside the pelvis, including female reproductive organs, lower urological organs and involved parts of the digestive system (rectosigmoid) [ 2 , 3 , 4 ].
TPE procedure is used in the treatment of advanced gynecological cancers as well as primary advanced and recurrent rectal cancers [ 3 , 5 ]. Even though TPE is infrequently performed, it may be considered as the last hope for the treatment of recurrent or advanced cancers [ 6 , 7 ].
TPE technique was associated with significant complications and mortality in the first decades, but in recent decades due to the improvement of preoperative planning (whole-body positron emission tomography), intraoperative and postoperative care, the survival rate, surgical complications and mortality of candidate patients has improved significantly [ 4 , 8 , 9 ].
Overall survival and disease-free survival rate significantly improved following TPE, especially in well-selected patients [ 3 ]. To the best of our knowledge, the highest 5 years overall survival rate was reported as 65.8% [ 10 ] in cervical cancer patients following pelvic exenteration and in colorectal cancer patients, one year survival rate was more than 80% in several previous studies [ 11 , 12 , 13 , 14 ] and the highest five year survival rate was reported as 92.9% in a study by Mark Katory et al. in the United Kingdom [ 14 ].
Considering that this surgical technique is considered a rare and advanced technique, significant complications and mortality rate (MR) have been reported for it. Intra-operative mortality, in-hospital mortality, 30- and 90-day mortality are important consequences that are reported for the management of the complications of this surgery. In addition to the survival rate, mortality and complications are also changing over time and depend on the equipment of the surgical center as well as the experience of the surgical team, and different studies have reported different mortality rates and there is no comprehensive review in this regard. The aim of this meta-analysis is to estimate the intra-operative mortality, in-hospital mortality, 30- and 90-day mortality rate and overall mortality rate following TPE in colorectal, gynecological, urological, and miscellaneous.
Study design
This is a systematic review and meta-analysis in which international databases were searched to find the relevant studies. Standard guideline of “Preferred Reporting Items for Systematic Reviews and Meta-Analyses (PRISMA) was followed to prepared the report. This study was registered in the PROSPERO (CRD42023467479).
Eligibility criteria
In this study, all observational studies related to the MR after TPE surgery with English full-text were included in the study. There was no time limit for entering the articles, and also in terms of the study design, all the articles that reported the MR including cohort studies, cross-sectional studies and case series studies were included. However, studies which had not defined the surgical procedure of TPE routinely were excluded. Additionally, we excluded case reports, letters to the editor, and review studies from our analysis. Although, we thoroughly screened the full texts of these articles to ensure that any relevant studies that were initially overlooked, were included in our primary search. Further details of the excluded articles are defined in Fig. 1 .
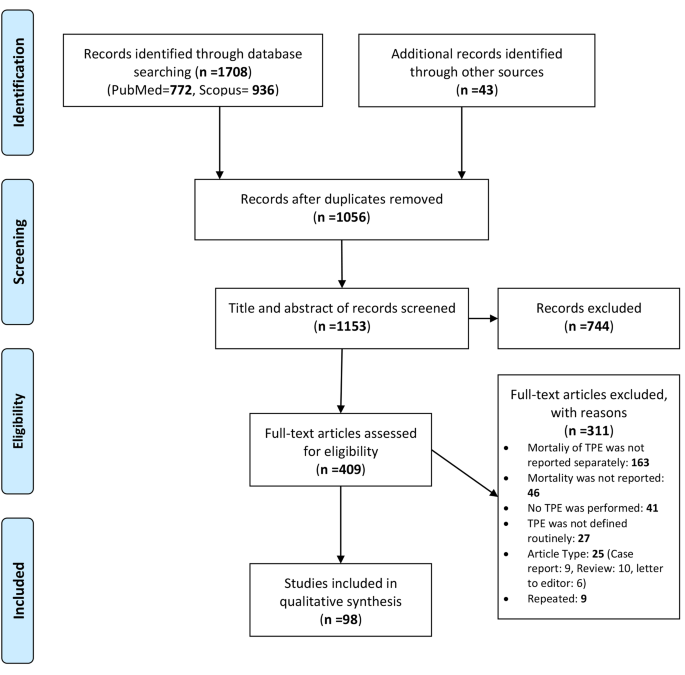
Flow diagram of the literature search for studies included in meta-analysis. TPE: Total Pelvic Exenteration
Information sources and search strategy
Articles published in English were searched. To retrieved relevant articles, the search was carried out using keywords for three international databases including Medline through PubMed, Scopus and Web of Science on November 2023. Different keywords were used to search the databases, and the search strategy in PubMed is given as an example.
((((“Survival“[Mesh] OR “Mortality“[Mesh] OR “mortality” [Subheading] OR “Disease-Free Survival“[Mesh] OR “Survival Analysis“[Mesh] OR “Survival Rate“[Mesh]) OR (“Survival“[tw])) OR (“Mortality“[tw])) OR (“Disease-Free Survival“[tw])) AND (((((“Pelvic Exenteration“[Mesh]) OR (“Pelvic Exenteration“[tw])) OR (“Pelvic Exenteration“[tiab])) OR (“total Pelvic Exenteration“[tw])) OR (“total Pelvic Exenteration“[tiab]))
Data collection process
To select relevant studies, retrieved articles were entered into Endnote software and duplicate articles were removed at this stage. Then the titles and abstracts of the remaining articles were screened and irrelevant articles were discarded. After that, the full text of the remaining articles was evaluated and irrelevant articles were removed. Finally, the required information was extracted from the remaining related articles.
The required information was extracted from the full text of the retrieved articles by the authors, and in cases of disagreement, decisions were made in consultation with other authors. The data extracted from each article included the name of the first author, year of publication, type of study design, sample size, type of cancer, location of the study, MR, included sample size, quality score of studies and the study population.
Our results were divided into four groups based on type of cancer: colorectal, gynecological, urological, and miscellaneous. The miscellaneous category included data on MR of TPE regardless of cancer type. Other cancers indicated for TPE in this study included squamous cell carcinoma, soft tissue sarcoma, perineal skin cancer, anal cancer, leiomyosarcoma, etc.
MR for intra-operative mortality, in-hospital mortality, 30-and 90-day mortality, was defined as reported deaths due to the surgical procedure and MR for overall mortality included death of the patients during the follow-up period due to surgery or cancer. Notably, patients who died due to other causes or were lost to follow-up were omitted from the analysis.
Study risk of bias assessment
The Joanna Briggs Institute Critical Appraisal (JBI) checklists were used to assess the quality of the included studies [ 15 ]. For each type of article, either cohort studies or case series, we utilized the relevant checklists provided by JBI. Each item on the checklist was assigned a score of 1 if the response was “yes”, and 0 if the response was “no”, “unclear”, or “not applicable”. The quality indicators were converted to 100%, Studies addressing ≥ 75% of the checklist items were considered as having a low risk of bias [ 16 ]. One author (MSF) carried out the quality assessment.
Effect measures
Effect measures in this study was the intra-operative, in-hospital, 30-day and 90-day and overall MR following TPE. The included sample size and the number of dead people were extracted from the studies, and the MR and 95% confidence interval were calculated.
Synthesis methods and statistical analysis
To check the heterogeneity among the studies, the I 2 statistic was used and it was tested using the chi-square test, and if there was significant heterogeneity between the studies, the random-effects model was used to merge the data. Although, based on the heterogeneity between the studies, from a methodological point of view, the fixed effects model was used, but considering that the mortality rate may be different based on the center expertise, surgeon experience, and postoperative care, in addition to the fixed effects model, random effects model was also performed and its results were reported. Egger’s linear regression test, Begg’s test and funnel plot were used to check publication bias. All analyzes are performed using Stata software version 16 (Stata Corp, College Station, TX).
Study selection
In this systematic review, 1751 primary studies (772 papers via Medline, 936 via Scopus and 43 papers via additional search) retrieved. Of the total articles, 695 duplicate articles were identified and removed. Then, the titles and abstracts of the remaining 1153 articles were screened and at this stage, 744 articles were excluded due to the lack of fulfilling the inclusion criteria and the full text of 409 remained articles was evaluated, of which 98 articles entered into this systematic review. All the process was presented in Fig. 1 .
Study characteristics
As it was shown in Tables 1 and 98 studies [ 6 , 7 , 12 , 13 , 14 , 17 , 18 , 19 , 20 , 21 , 22 , 23 , 24 , 25 , 26 , 27 , 28 , 29 , 30 , 31 , 32 , 33 , 34 , 35 , 36 , 37 , 38 , 39 , 40 , 41 , 42 , 43 , 44 , 45 , 46 , 47 , 48 , 49 , 50 , 51 , 52 , 53 , 54 , 55 , 56 , 57 , 58 , 59 , 60 , 61 , 62 , 63 , 64 , 65 , 66 , 67 , 68 , 69 , 70 , 71 , 72 , 73 , 74 , 75 , 76 , 77 , 78 , 79 , 80 , 81 , 82 , 83 , 84 , 85 , 86 , 87 , 88 , 89 , 90 , 91 , 92 , 93 , 94 , 95 , 96 , 97 , 98 , 99 , 100 , 101 , 102 ] (5343 cases) were included in the analysis. The oldest one was published in 1967 and the most recent in 2023. Both case series (23 studies) and cohort studies (75 studies) were included in the analysis. The sample size of included studies ranged from 2 to 2305 cases and colorectal, gynecological, urological and miscellaneous cancers were included in the analysis. More details in this regard were presented in Table 1 .
Risk of bias within studies
All the articles we reviewed met over 80% of the criteria in the JBI checklists and were thus included in the study. Tables 2 and 3 describe the details of evaluating the included studies according to JBI checklist for cohort studies and case series, respectively.
Quantitative data synthesis and heterogeneity across studies
Colorectal cancers mortality rate.
The MR following TPE in colorectal cancers was estimated and the results of meta-analysis suggested that intra-operative MR is 0.2% ( n = 27, 95%CI = 0.07–1.11%, I-square = 0.0%), in-hospital MR is 3.11% ( n = 31, 95%CI = 2.15–4.46%, I-square = 9.02%), 30-day MR is estimated as 2.61% ( n = 35, 95%CI = 1.95–3.48%, I-square = 15.18%), 90-day MR is 6.22% ( n = 12, 95%CI = 4.17–9.18%, I-square = 16.87%) and overall MR is estimated as 30.57% ( n = 13, 95%CI = 26.9–34.4%, I-square = 60.6%), respectively (Table 4 ). All analysis was done by fixed-effects model because of no significant heterogeneity among studies. In addition, the overall MR in open surgery was 30.57%, in primary cancer 2.44%, and in primary and recurrent cancers 31.6%. There were not enough studies to perform meta-analysis for recurrent cancer.
Gynecological cancers mortality rate
Regarding MR following TPE in gynecological cancers, the obtained results showed that intra-operative MR is 0.21% ( n = 40, 95%CI = 0.05–0.85%, I-square = 0.0%), in-hospital MR is 2.65% ( n = 34, 95%CI = 1.61–4.36%, I-square = 1.35%), 30-day MR is estimated as 5.89% ( n = 37, 95%CI = 4.65–7.43%, I-square = 0.39%), 90-day MR is 2.74% ( n = 7, 95%CI = 1.03–7.07%, I-square = 0.0%) and overall MR is estimated as 25.5% ( n = 12, 95%CI = 19.8–32.1%, I-square = 46.6%), respectively (Table 5 ). All analysis was done by fixed-effects model because of no significant heterogeneity among studies. The overall MR in open surgery was 25.5%, in minimally invasive surgery was 25.0%, and in primary, recurrent and both of them together was 53.8%, 12.7% and 55.5%, respectively.
Urological cancers mortality rate
In the case of urological cancers, there have been fewer studies, but still, the results showed that 30-day MR is estimated as 2.07% ( n = 4, 95%CI = 1.37–3.13%, I-square = 0.0%).
Miscellaneous cancers mortality rate
The results of meta-analysis revealed that following TPE in Miscellaneous cancers, MR of intra-operative MR is 0.16% ( n = 16, 95%CI = 0.02–1.1%, I-square = 56.9%), in-hospital MR is 0.8% ( n = 17, 95%CI = 0.3–2.12%, I-square = 57.6%), 30-day MR is estimated as 1.59% ( n = 18, 95%CI = 1.23–2.04%, I-square = 6.01%), 90-day MR is 2.33% ( n = 4, 95%CI = 1.11–4.8%, I-square = 0.0%), and overall MR is estimated as 12.42% ( n = 3, 95%CI = 9.2–16.6%, I-square = 39.7%) (Table 6 ). These rates for surgeries are reported in Table 6 , but for other cases, due to the lack of sufficient studies, meta-analysis was not performed.
In this study, we investigated the MR after TPE using meta-analysis method, which included different types of cancers such as colorectal, gynecological, urological and miscellaneous cancers. The main findings of this study showed that the highest mortality rate was related to overall mortality. The overall mortality rate was 30.57% in colorectal cancers, 25.5% in gynecological cancers and 12.42% in Miscellaneous. In fact, the highest rate of mortality is related to the overall mortality rate of colorectal cancers. Naturally, the MR in open surgeries was higher than in minimally invasive surgeries, and also in primary advanced cancers, it was higher than in recurrent cancers.
Generally, TPE is used in the treatment of advanced gynecological cancers as well as primary advanced and recurrent rectal cancers, so it is mostly used in cases where conventional treatment modalities do not have a suitable prognosis. Due to the fact that the stage of cancer is higher and the prognosis is worse in patients who are candidates for this surgery, it is expected that the MR will be higher, on the other hand, this surgery is considered as an advanced surgery, and its success rate depends on the experience of the surgeon and the equipment of the surgical center.
In a study by Vigneswaran et al. [ 94 ] with the largest sample size conducted in the USA, 2305 cases of TPE between 2005 and 2016 were evaluated. Of these, 45% were urological malignancies, 33% colorectal, 15% gynecological and 9% other cancers. The authors have stated that despite the common complications in this surgery, the mortality rate is relatively low and the outcomes during and after the operation are dissimilar in different types of cancer. Also, the prevalence of major complications is 15%, 30-day mortality is 2%, the duration of hospitalization after surgery is 9 days, and blood transfusion is reported in 50% of cases. The results of the present meta-analysis estimated the 30-day mortality rate to be 2.61%, 5.89%, 1.59% and 2.07% in colorectal, gynecological, miscellaneous and urological cancer which is higher than the value reported in the aforementioned study in most cases. Part of this difference can be related to better equipment and care in USA medical centers and part of it to more experience of medical centers and surgical teams. In our study, the results showed that the overall mortality rate in gynecological malignancies is lower than that in colorectal cancers (25.5% vs. 30.57%). Although in the study of Vigneswaran et al. [ 94 ], no significant difference was reported in the 30-day mortality rate of different cancers, but the prevalence of complications was higher in gynecological cancers, and the return to the operating room due to complications was also higher in gynecological cancers than in colorectal cancer (12.8% vs. 8.7%), while it was 4.8% for urological cancers.
Intra-operative mortality rate in colorectal cancers with rate of 0.21% showed the highest rate among studied cancers and its value in all other cancers were 0.2% or less. In terms of in-hospital mortality, this rate was estimated at 3.11% for colorectal cancer, and the highest rate of in-hospital mortality rate was related to colorectal cancer. Therefore, the results of our study showed that in performing TPE for colorectal cancers, intraoperative, in-hospital, 30-day, 90-day and overall mortality rate is more than gynecological, urological, and miscellaneous cancers.
It is important to note that while recent advancements in surgical techniques and well-equipped surgical centers have improved mortality rates for TPE, the main rationale for such an aggressive surgery is the potential chance for a cure, which has been reported in up to 63% of patients [ 103 ]. However, the effectiveness of alternative options such as robotic-assisted or laparoscopic surgeries in achieving this goal has not been thoroughly studied [ 104 ]. One notable study by Bizzarri et al. [ 83 ] reported a 30-day mortality rate of 0% following minimally invasive TPE, demonstrating its feasibility in a small group of 5 patients. More research is needed to fully understand the outcomes of minimally invasive TPE compared to conventional surgical method.
The complexity of the TPE procedure makes it challenging to predict outcomes. Factors such as the purpose of surgery (curative or palliative), cancer type, patient comorbidities, and the expertise of the surgical team and center are known to be associated with morbidity and mortality [ 94 , 104 , 105 ]. Patients undergoing TPE also require strong physical and emotional support. Therefore, a skilled multi-disciplinary team is essential for evaluating patient eligibility and performing the surgery. Previous studies have emphasized the use of specific guidelines, such as the enhanced recovery after surgery (ERAS) guideline, to reduce complications [ 94 , 106 ]. Ultimately, individualized patient selection is recommended before performing TPE.
To the best of our knowledge, this is the largest meta-analysis of MR following TPE. However, several limitations should be acknowledged. Our data may be biased towards reporting more studies with a 0% MR. This is mainly because if a study reported a 0% MR for a specific time period, the MR for previous periods would be assumed to be 0% as well, even if it wasn’t reported in detail. However, if a study reported a MR higher than 0% for a specific time period and didn’t report the previous MRs, those data points were labeled as missing. Furthermore, in this study we included as much studies as possible, to create a holistic picture of MR following TPE. Therefore, it might be subject to bias as all TPE performed since 1976 with proper definition of TPE were included in our analysis. Further studies are required to investigate the impact of surgical intention, surgical center expertise, post-operation care, and patients’ comorbidities on MR following TPE.
Data availability
All data generated or analyzed during this study are included in the article.
Brunschwig A. Complete excision of pelvic viscera for advanced carcinoma. A one-stage abdominoperineal operation with end colostomy and bilateral ureteral implantation into the colon above the colostomy. Cancer. 1948;1(2):177–83.
Article CAS PubMed Google Scholar
Pleth Nielsen CK, Sørensen MM, Christensen HK, Funder JA. Complications and survival after total pelvic exenteration. Eur J Surg Oncol. 2022;48(6):1362–7.
Article PubMed Google Scholar
Miri SR, Akhavan S, Mousavi AS, Hashemi SR, Sheikhhasan S, Almasi-Hashiani A, Sadegh Fakhari M, Esmailzadeh A. A systematic review on overall survival and disease-free survival following total pelvic exenteration. Asian Pac J cancer Prevention: APJCP. 2022;23(4):1137–45.
Article Google Scholar
Straubhar AM, Chi AJ, Zhou QC, Iasonos A, Filippova OT, Leitao MM Jr., Awowole IO, Abu-Rustum NR, Broach VA, Jewell EL, et al. Pelvic exenteration for recurrent or persistent gynecologic malignancies: clinical and histopathologic factors predicting recurrence and survival in a modern cohort. Gynecol Oncol. 2021;163(2):294–8.
Article PubMed PubMed Central Google Scholar
Shaikh I, Nasir I, Gani A, Mureb A, Dowsett D, Haywood R, Rosich Medina A, Al Kadhi O, Parvaiz A, Kapur S. Robotic total pelvic exenteration – a video vignette. Colorectal Dis. 2021;23(7):1946–1946.
Berek JS, Howe C, Lagasse LD, Hacker NF. Pelvic exenteration for recurrent gynecologic malignancy: survival and morbidity analysis of the 45-year experience at UCLA. Gynecol Oncol. 2005;99(1):153–9.
Goldberg GL, Sukumvanich P, Einstein MH, Smith HO, Anderson PS, Fields AL. Total pelvic exenteration: the Albert Einstein College of Medicine/Montefiore Medical Center Experience (1987 to 2003). Gynecol Oncol. 2006;101(2):261–8.
Lambrou NC, Pearson JM, Averette HE. Pelvic exenteration of gynecologic malignancy: indications, and technical and reconstructive considerations. Surg Oncol Clin N Am. 2005;14(2):289–300.
Lakhman Y, Nougaret S, Miccò M, Scelzo C, Vargas HA, Sosa RE, Sutton EJ, Chi DS, Hricak H, Sala E. Role of MR Imaging and FDG PET/CT in selection and follow-up of patients treated with pelvic exenteration for gynecologic malignancies. Radiographics: Rev Publication Radiological Soc North Am Inc. 2015;35(4):1295–313.
Terán-Porcayo MA, Zeichner-Gancz I, del-Castillo RA, Beltrán-Ortega A, Solorza-Luna G. Pelvic exenteration for recurrent or persistent cervical cancer: experience of five years at the National Cancer Institute in Mexico. Med Oncol (Northwood Lond Engl). 2006;23(2):219–23.
Luna-Perez P, Rodriguez DF, Flores D, Delgado S, Labastida S. Morbidity and mortality following preoperative radiation therapy and total pelvic exenteration for primary rectal adenocarcinoma. Surg Oncol. 1995;4(6):295–301.
Russo P, Ravindran B, Katz J, Paty P, Guillem J, Cohen AM. Urinary diversion after total pelvic exenteration for rectal cancer. Ann Surg Oncol. 1999;6(8):732–8.
Kamat AM, Huang SF, Bermejo CE, Rosser CJ, Pettaway CA, Pisters PW, Guitreau D, Pisters LL. Total pelvic exenteration: effective palliation of perineal pain in patients with locally recurrent prostate cancer. J Urol. 2003;170(5):1868–71.
Katory M, McLean R, Paez E, Kucukmetin A, Naik R. Short- and long-term outcomes following pelvic exenteration for gynae-oncological and colorectal cancers: a 9 year consecutive single-centre cohort study. Int J Surg (London England). 2017;43:38–45.
Institute JB, The Joanna Briggs Institute Levels of Evidence and Grades of Recommendation Working Party*. Supporting Document for the Joanna Briggs Institute Levels of Evidence and Grades of Recommendation Austrália: Joanna Briggs Institute 2014:2019 – 2005.
Peters MD, Godfrey CM, McInerney P, Soares CB, Khalil H, Parker D. The Joanna Briggs Institute reviewers’ manual 2015: methodology for JBI scoping reviews. 2015.
Ingiulla W, Cosmi EV. Pelvic exenteration for advanced carcinoma of the cervix. Some reflections on 241 cases. Am J Obstet Gynecol. 1967;99(8):1083–6.
Thornton WN Jr, Flanagan WC Jr. Pelvic exenteration in the treatment of advanced malignancy of the vulva. Am J Obstet Gynecol. 1973;117(6):774–81.
Karlen JR, Piver MS. Reduction of mortality and morbidity associated with pelvic exenteration. Gynecol Oncol. 1975;3(2):164–7.
Eckhauser FE, Lindenauer SM, Morley GW. Pelvic exenteration for advanced rectal carcinoma. Am J Surg. 1979;138(3):412–4.
Ledesma EJ, Bruno S, Mittelman A. Total pelvic exenteration in colorectal disease: a 20-year experience. Ann Surg. 1981;194(6):701–3.
Article CAS PubMed PubMed Central Google Scholar
Mori T, Tominaga T, Itoh I. Results of pelvic exenteration. World J Surg. 1981;5(5):749–50.
Boey J, Wong J, Ong GB. Pelvic exenteration for locally advanced colorectal carcinoma. Ann Surg. 1982;195(4):513–8.
Takagi H, Morimoto T, Yasue M, Kato K, Yamada E, Suzuki R. Total pelvic exenteration for advanced carcinoma of the lower colon. J Surg Oncol. 1985;28(1):59–62.
Cuevas HR, Torres A, Garza MDL, Hernandez D, Herrera L. Pelvic exenteration for carcinoma of the cervix: analysis of 252 cases. J Surg Oncol. 1988;38(2):121–5.
Yeung RS, Moffat FL, Falk RE. Pelvic exenteration for recurrent and extensive primary colorectal adenocarcinoma. Cancer. 1993;72(6):1853–8.
Liu SY, Wang YN, Zhu WQ, Gu WL, Fu H. Total pelvic exenteration for locally advanced rectal carcinoma. Dis Colon Rectum. 1994;37(2):172–4.
Lopez MJ, Standiford SB, Skibba JL. Total pelvic exenteration. A 50-year experience at the Ellis Fischel Cancer Center. Archives of surgery (Chicago, Ill: 1960) 1994, 129(4):390–395; discussion 395–396.
Sardi A, Bolton JS, Hicks TC, Skenderis BS. 2nd: total pelvic exenteration with or without sacral resection in patients with recurrent colorectal cancer. South Med J. 1994;87(3):363–9.
Woodhouse CR, Plail RO, Schlesinger PE, Shepherd JE, Hendry WF, Breach NM. Exenteration as palliation for patients with advanced pelvic malignancy. Br J Urol. 1995;76(3):315–20.
Luna-Perez P. Patterns of recurrence following pelvic exenteration and external radiotherapy for locally advanced primary rectal adenocarcinoma. Ann Surg Oncol. 1996;3(6):526–33.
Shirouzu K, Isomoto H, Kakegawa T. Total pelvic exenteration for locally advanced colorectal carcinoma. Br J Surg. 1996;83(1):32–5.
Law WL, Chu KW, Choi HK. Total pelvic exenteration for locally advanced rectal cancer. J Am Coll Surg. 2000;190(1):78–83.
Chen HS, Sheen-Chen SM. Total pelvic exenteration for primary local advanced colorectal cancer. World J Surg. 2001;25(12):1546–9.
Wiig JN, Poulsen JP, Larsen S, Brændengen M, Waehre H, Giercksky KE. Total pelvic exenteration with preoperative irradiation for advanced primary and recurrent rectal cancer. Eur J Surg. 2002;168(1):42–8.
Ike H, Shimada H, Yamaguchi S, Ichikawa Y, Fujii S, Ohki S. Outcome of total pelvic exenteration for primary rectal cancer. Dis Colon Rectum. 2003;46(4):474–80.
Jimenez RE, Shoup M, Cohen AM, Paty PB, Guillem J, Wong WD. Contemporary outcomes of total pelvic exenteration in the treatment of colorectal cancer. Dis Colon Rectum. 2003;46(12):1619–25.
Kakuda JT, Lamont JP, Chu DZ, Paz IB. The role of pelvic exenteration in the management of recurrent rectal cancer. Am J Surg. 2003;186(6):660–4.
Vitelli CE, Crenca F, Fortunato L, Di Nardo A, Farina M, Mustacciuoli G. Pelvic exenterative procedures for locally advanced or recurrent colorectal carcinoma in a community hospital. Tech Coloproctol. 2003;7(3):159–63.
Houvenaeghel G, Moutardier V, Karsenty G, Bladou F, Lelong B, Buttarelli M, Delpero JR. Major complications of urinary diversion after pelvic exenteration for gynecologic malignancies: a 23-year mono-institutional experience in 124 patients. Gynecol Oncol. 2004;92(2):680–3.
Moriya Y, Akasu T, Fujita S, Yamamoto S. Total pelvic exenteration with distal sacrectomy for fixed recurrent rectal cancer in the pelvis. Dis Colon Rectum. 2004;47(12):2047–53. discussion 2053 – 2044.
Leibovici D, Pagliaro L, Rosser CJ, Pisters LL. Salvage surgery for bulky local recurrence of prostate cancer following radical prostatectomy. J Urol. 2005;173(3):781–3.
Nguyen DQ, McGregor AD, Freites O, Carr ND, Beynon J, El-Sharkawi AM, Lucas MG. Exenterative pelvic surgery–eleven year experience of the Swansea Pelvic Oncology Group. Eur J Surg Oncology: J Eur Soc Surg Oncol Br Association Surg Oncol. 2005;31(10):1180–4.
Article CAS Google Scholar
de Wilt JH, van Leeuwen DH, Logmans A, Verhoef C, Kirkels WJ, Vermaas M, Ansink AC. Pelvic exenteration for primary and recurrent gynaecological malignancies. Eur J Obstet Gynecol Reprod Biol. 2007;134(2):243–8.
Park JY, Choi HJ, Jeong SY, Chung J, Park JK, Park SY. The role of pelvic exenteration and reconstruction for treatment of advanced or recurrent gynecologic malignancies: analysis of risk factors predicting recurrence and survival. J Surg Oncol. 2007;96(7):560–8.
Vermaas M, Ferenschild FT, Verhoef C, Nuyttens JJ, Marinelli AW, Wiggers T, Kirkels WJ, Eggermont AM, de Wilt JH. Total pelvic exenteration for primary locally advanced and locally recurrent rectal cancer. Eur J Surg Oncology: J Eur Soc Surg Oncol Br Association Surg Oncol. 2007;33(4):452–8.
Wells BJ, Stotland P, Ko MA, Al-Sukhni W, Wunder J, Ferguson P, Lipa J, Last L, Smith AJ, Swallow CJ. Results of an aggressive approach to resection of locally recurrent rectal cancer. Ann Surg Oncol. 2007;14(2):390–5.
Ungar L, Palfalvi L, Novak Z. Primary pelvic exenteration in cervical cancer patients. Gynecol Oncol. 2008;111(2 Suppl):S9–12.
Ferenschild FT, Vermaas M, Verhoef C, Ansink AC, Kirkels WJ, Eggermont AM, de Wilt JH. Total pelvic exenteration for primary and recurrent malignancies. World J Surg. 2009;33(7):1502–8.
Maggioni A, Roviglione G, Landoni F, Zanagnolo V, Peiretti M, Colombo N, Bocciolone L, Biffi R, Minig L, Morrow CP. Pelvic exenteration: ten-year experience at the European Institute of Oncology in Milan. Gynecol Oncol. 2009;114(1):64–8.
Puntambekar S, Agarwal G, Puntambekar S, Sathe R, Patil A. Stretching the limits of laparoscopy in gynecological oncology: technical feasibility of doing a laparoscopic total pelvic exenteration for palliation in advanced cervical cancer. Int J Biomedical Science: IJBS. 2009;5(1):17.
Spahn M, Weiss C, Bader P, Frohneberg D, Studer UE, Burkhard FC. The role of exenterative surgery and urinary diversion in persistent or locally recurrent gynecological malignancy: complications and survival. Urol Int. 2010;85(1):16–22.
Zoucas E, Frederiksen S, Lydrup ML, Månsson W, Gustafson P, Alberius P. Pelvic exenteration for advanced and recurrent malignancy. World J Surg. 2010;34(9):2177–84.
Chokshi RJ, Fowler J, Cohn D, Bahnson R, Lumbley J, Martin EW Jr. A single-institution approach to total pelvic exenteration. Am Surg. 2011;77(12):1629–39.
Domes TS, Colquhoun PH, Taylor B, Izawa JI, House AA, Luke PP, Izawa JI. Total pelvic exenteration for rectal cancer: outcomes and prognostic factors. Can J Surg J canadien de chirurgie. 2011;54(6):387–93.
Guimarães GC, Baiocchi G, Ferreira FO, Kumagai LY, Fallopa CC, Aguiar S, Rossi BM, Soares FA, Lopes A. Palliative pelvic exenteration for patients with gynecological malignancies. Arch Gynecol Obstet. 2011;283(5):1107–12.
Mitulescu G, Gluck G, Stîngu C. Radical surgical treatement in pelvic advanced cancer. Annals Fundeni Hosp. 2011;16(3–4):87–106.
Google Scholar
Baiocchi G, Guimaraes GC, Rosa Oliveira RA, Kumagai LY, Faloppa CC, Aguiar S, Begnami MD, Soares FA, Lopes A. Prognostic factors in pelvic exenteration for gynecological malignancies. Eur J Surg Oncology: J Eur Soc Surg Oncol Br Association Surg Oncol. 2012;38(10):948–54.
Kuhrt MP, Chokshi RJ, Arrese D, Martin EW Jr. Retrospective review of pelvic malignancies undergoing total pelvic exenteration. World J Surg Oncol. 2012;10:110.
Ramamurthy R, Duraipandian A. Morbidity and outcome of pelvic exenteration in locally advanced pelvic malignancies. Indian J Surg Oncol. 2012;3(3):231–5.
Yoo HJ, Lim MC, Seo SS, Kang S, Yoo CW, Kim JY, Park SY. Pelvic exenteration for recurrent cervical cancer: ten-year experience at national cancer center in Korea. J Gynecologic Oncol. 2012;23(4):242–50.
Jäger L, Nilsson PJ, Rådestad AF. Pelvic exenteration for recurrent gynecologic malignancy: a study of 28 consecutive patients at a single institution. Int J Gynecol cancer: Official J Int Gynecol Cancer Soc. 2013;23(4):755–62.
Tan KK, Pal S, Lee PJ, Rodwell L, Solomon MJ. Pelvic exenteration for recurrent squamous cell carcinoma of the pelvic organs arising from the cloaca–a single institution’s experience over 16 years. Colorectal Disease: Official J Association Coloproctology Great Br Irel. 2013;15(10):1227–31.
Ueda T, Koyama F, Nakagawa T, Nakamura S, Nishigori N, Inoue T, Kawasaki K, Obara S, Nakamoto T, Fujii H, et al. Clinical outcomes of pelvic exenteration for locally advanced primary or recurrent non-colorectal pelvic malignancies. Gan Kagaku Ryoho Cancer Chemother. 2013;40(12):2433–6.
Milne T, Solomon MJ, Lee P, Young JM, Stalley P, Harrison JD, Austin KK. Sacral resection with pelvic exenteration for advanced primary and recurrent pelvic cancer: a single-institution experience of 100 sacrectomies. Dis Colon Rectum. 2014;57(10):1153–61.
Pathiraja P, Sandhu H, Instone M, Haldar K, Kehoe S. Should pelvic exenteration for symptomatic relief in gynaecology malignancies be offered? Arch Gynecol Obstet. 2014;289:657–62.
Petruzziello A, Kondo W, Hatschback SB, Guerreiro JA, Filho FP, Vendrame C, Luz M, Ribeiro R. Surgical results of pelvic exenteration in the treatment of gynecologic cancer. World J Surg Oncol. 2014;12:279.
Tanaka S, Nagase S, Kaiho-Sakuma M, Nagai T, Kurosawa H, Toyoshima M, Tokunaga H, Otsuki T, Utsunomiya H, Takano T, et al. Clinical outcome of pelvic exenteration in patients with advanced or recurrent uterine cervical cancer. Int J Clin Oncol. 2014;19(1):133–8.
Xin KY, Ng DW, Tan GH, Teo MC. Role of pelvic exenteration in the management of locally advanced primary and recurrent rectal cancer. J Gastrointest cancer. 2014;45(3):291–7.
Cəpîlna ME, Moldovan B, Szabo B. Pelvic exenteration - our initial experience in 15 cases. Eur J Gynaecol Oncol. 2015;36(2):142–5.
PubMed Google Scholar
Ghouti L, Pereira P, Filleron T, Humeau M, Guimbaud R, Selves J, Carrere N. Pelvic exenterations for specific extraluminal recurrences in the era of total mesorectal excision: is there still a chance for cure? A single-center review of patients with extraluminal pelvic recurrence for rectal cancer from March 2004 to November 2010. Am J Surg. 2015;209(2):352–62.
Kusters M, Austin KK, Solomon MJ, Lee PJ, Nieuwenhuijzen GA, Rutten HJ. Survival after pelvic exenteration for T4 rectal cancer. Br J Surg. 2015;102(1):125–31.
Moreno-Palacios E, Diestro MD, De Santiago J, Hernández A, Zapardiel I. Pelvic exenteration in Gynecologic Cancer: La Paz University Hospital Experience. Int J Gynecol cancer: Official J Int Gynecol Cancer Soc. 2015;25(6):1109–14.
Rombouts AJ, Koh CE, Young JM, Masya L, Roberts R, De-Loyde K, de Wilt JH, Solomon MJ. Does radiotherapy of the primary rectal cancer affect prognosis after pelvic exenteration for recurrent rectal cancer? Dis Colon Rectum. 2015;58(1):65–73.
Koda K, Shuto K, Matsuo K, Kosugi C, Mori M, Hirano A, Hiroshima Y, Tanaka K. Layer-oriented total pelvic exenteration for locally advanced primary colorectal cancer. Int J Colorectal Dis. 2016;31(1):59–66.
Schmidt AM, Imesch P, Fink D, Egger H. Pelvic exenterations for Advanced and recurrent endometrial Cancer: clinical outcomes of 40 patients. Int J Gynecol cancer: Official J Int Gynecol Cancer Soc. 2016;26(4):716–21.
Chew MH, Yeh YT, Toh EL, Sumarli SA, Chew GK, Lee LS, Tan MH, Hennedige TP, Ng SY, Lee SK, et al. Critical evaluation of contemporary management in a new pelvic exenteration unit: the first 25 consecutive cases. World J Gastrointest Oncol. 2017;9(5):218–27.
Aslim EJ, Chew MH, Chew GK, Lee LS. Urological outcomes following pelvic exenteration for advanced pelvic cancer are not inferior to those following radical cystectomy. ANZ J Surg. 2018;88(9):896–900.
Hagemans JAW, Rothbarth J, Kirkels WJ, Boormans JL, van Meerten E, Nuyttens J, Madsen EVE, Verhoef C, Burger JWA. Total pelvic exenteration for locally advanced and locally recurrent rectal cancer in the elderly. Eur J Surg Oncology: J Eur Soc Surg Oncol Br Association Surg Oncol. 2018;44(10):1548–54.
Li L, Ma SQ, Tan XJ, Zhong S, Wu M. Pelvic exenteration for recurrent and persistent cervical Cancer. Chin Med J. 2018;131(13):1541–8.
Mehta AM, Hellawell G, Burling D, Littler S, Antoniou A, Jenkins JT. Transperineal retropubic approach in total pelvic exenteration for advanced and recurrent colorectal and anal cancer involving the penile base: technique and outcomes. Tech Coloproctol. 2018;22(9):663–71.
Romeo A, Gonzalez MI, Jaunarena J, Zubieta ME, Favre G, Tejerizo JC. Pelvic exenteration for gynecologic malignancies: postoperative complications and oncologic outcomes. Actas Urol Esp. 2018;42(2):121–5.
Bizzarri N, Chiantera V, Ercoli A, Fagotti A, Tortorella L, Conte C, Cappuccio S, Di Donna MC, Gallotta V, Scambia G, et al. Minimally invasive pelvic exenteration for gynecologic malignancies: a multi-institutional Case Series and Review of the literature. J Minim Invasive Gynecol. 2019;26(7):1316–26.
de Gregorio N, de Gregorio A, Ebner F, Friedl TWP, Huober J, Hefty R, Wittau M, Janni W, Widschwendter P. Pelvic exenteration as ultimate ratio for gynecologic cancers: single-center analyses of 37 cases. Arch Gynecol Obstet. 2019;300(1):161–8.
Kiiski J, Räikkönen K, Vuento MH, Hyöty MK, Kallio J, Kuokkanen HO, Kaartinen IS. Transverse myocutaneous Gracilis flap reconstruction is feasible after pelvic exenteration: 12-year surgical and oncological results. Eur J Surg Oncology: J Eur Soc Surg Oncol Br Association Surg Oncol. 2019;45(9):1632–7.
Lago V, Poveda I, Padilla-Iserte P, Simón-Sanz E, García-Granero Á, Pontones JL, Matute L, Domingo S. Pelvic exenteration in gynecologic cancer: complications and oncological outcome. Gynecol Surg 2019, 16(1).
Lee P, Tan WJ, Brown KGM, Solomon MJ. Addressing the empty pelvic syndrome following total pelvic exenteration: does mesh reconstruction help? Colorectal Disease: Official J Association Coloproctology Great Br Irel. 2019;21(3):365–9.
Nedyalkov K, Magunska N, Bechev B, Kostov I. Survival rate and complications after different types of pelvic exenteration for gynecological cancer. Eur J Gynaecol Oncol. 2019;40(1):69–73.
Rema P, Suchetha S, Mathew AP, George P, Mathew A, Thomas S. Pelvic exenterations for Cervical Cancer Recurrences—a safe option in Indian scenario. Indian J Surg. 2019;81(6):537–42.
Soeda S, Furukawa S, Sato T, Ueda M, Kamo N, Endo Y, Kojima M, Nomura S, Kataoka M, Fujita S, et al. Pelvic exenteration as potential cure and Symptom Relief in Advanced and recurrent Gynaecological Cancer. Anticancer Res. 2019;39(10):5631–7.
Tortorella L, Casarin J, Mara KC, Weaver AL, Multinu F, Glaser GE, Cliby WA, Scambia G, Mariani A, Kumar A. Prediction of short-term surgical complications in women undergoing pelvic exenteration for gynecological malignancies. Gynecol Oncol. 2019;152(1):151–6.
Lewandowska A, Szubert S, Koper K, Koper A, Cwynar G, Wicherek L. Analysis of long-term outcomes in 44 patients following pelvic exenteration due to cervical cancer. World J Surg Oncol 2020, 18(1).
Tuech JJ, Pinson J, Nouhaud FX, Wood G, Clavier T, Sabourin JC, Di Fiore F, Monge M, Papet E, Coget J. Total pelvic exenteration, cytoreductive surgery, and hyperthermic intraperitoneal chemotherapy for rectal cancer with associate peritoneal metastases: Surgical strategies to optimize safety. Cancers. 2020;12(11):1–14.
Vigneswaran HT, Schwarzman LS, Madueke IC, David SML, Nordenstam J, Moreira D, Abern MR. Morbidity and Mortality of Total Pelvic Exenteration for Malignancy in the US. Annals of surgical oncology 2020.
Bogner A, Fritzmann J, Müssle B, Huber J, Dobroschke J, Bork U, Wolk S, Distler M, Weitz J, Welsch T et al. Pelvic exenteration for colorectal and non-colorectal cancer: a comparison of perioperative and oncological outcome. Int J Colorectal Dis 2021.
Brown KGM, Ansari N, Solomon MJ, Austin KKS, Hamilton AER, Young CJ. Pelvic exenteration combined with cytoreductive surgery and hyperthermic intraperitoneal chemotherapy for advanced primary or recurrent colorectal cancer with peritoneal metastases. Colorectal Dis. 2021;23(1):186–91.
Kanao H, Aoki Y, Omi M, Nomura H, Tanigawa T, Okamoto S, Chang EJ, Kurita T, Netsu S, Matoda M et al. Laparoscopic pelvic exenteration and laterally extended endopelvic resection for postradiation recurrent cervical carcinoma: Technical feasibility and short-term oncologic outcome. Gynecologic oncology 2021.
Ter Glane L, Hegele A, Wagner U, Boekhoff J. Pelvic exenteration for recurrent or advanced gynecologic malignancies–analysis of outcome and complications. Gynecologic Oncol Rep. 2021;36:100757.
Abdulrahman GO, Das N, Chandrasekaran TV, Khot U, Drew PJ, Bose P, Vet JN, Tofazzal N, Roberts S, Lutchman Singh K. Pelvic exenteration for the treatment of locally advanced vulvar cancer in south west wales. Cancers. 2022;14(7):1767.
Bouraoui I, Bouaziz H, Tounsi N, Ben Romdhane R, Hechiche M, Slimane M, Rahal K. Survival after pelvic exenteration for cervical cancer. J Obstet Gynecol India. 2022;72(1):66–71.
Haidopoulos D, Pergialiotis V, Aggelou K, Thomakos N, Alexakis N, Stamatakis E, Rodolakis A. Pelvic exenteration for gynecologic malignancies: the experience of a tertiary center from Greece. Surg Oncol. 2022;40:101702.
Nielsen CKP, Sørensen MM, Christensen HK, Funder JA. Complications and survival after total pelvic exenteration. Eur J Surg Oncol. 2022;48(6):1362–7.
Brown KG, Solomon MJ, Koh CE. Pelvic exenteration surgery: the evolution of radical surgical techniques for advanced and recurrent pelvic malignancy. Dis Colon Rectum. 2017;60(7):745–54.
Diver EJ, Rauh-Hain JA, Del Carmen MG. Total pelvic exenteration for gynecologic malignancies. International Journal of Surgical Oncology 2012, 2012.
Kroon HM, Dudi-Venkata N, Bedrikovetski S, Thomas M, Kelly M, Aalbers A, Aziz NA, Abraham-Nordling M, Akiyoshi T, Alberda W. Palliative pelvic exenteration: a systematic review of patient-centered outcomes. Eur J Surg Oncol. 2019;45(10):1787–95.
Nelson G, Bakkum-Gamez J, Kalogera E, Glaser G, Altman A, Meyer LA, Taylor JS, Iniesta M, Lasala J, Mena G. Guidelines for perioperative care in gynecologic/oncology: enhanced recovery after surgery (ERAS) society recommendations—2019 update. Int J Gynecologic Cancer 2019, 29(4).
Ferron G, Querleu D, Martel P, Letourneur B, Soulié M. Laparoscopy-assisted vaginal pelvic exenteration. Gynecol Oncol. 2006;100(3):551–5.
Ogura A, Akiyoshi T, Konishi T, Fujimoto Y, Nagayama S, Fukunaga Y, Ueno M. Safety of laparoscopic pelvic exenteration with urinary diversion for colorectal malignancies. World J Surg. 2016;40:1236–43.
Yang K, Cai L, Yao L, Zhang Z, Zhang C, Wang X, Tang J, Li X, He Z, Zhou L. Laparoscopic total pelvic exenteration for pelvic malignancies: the technique and short-time outcome of 11 cases. World J Surg Oncol. 2015;13(1):1–9.
Pokharkar A, Kammar P, D’Souza A, Bhamre R, Sugoor P, Saklani A. Laparoscopic pelvic exenteration for locally advanced rectal Cancer, technique and short-term outcomes. J Laparoendoscopic Adv Surg Techniques. 2018;28(12):1489–94.
Ichihara M, Uemura M, Ikeda M, Miyake M, Kato T, Hamakawa T, Maeda S, Hama N, Nishikawa K, Miyamoto A. Safety and feasibility of laparoscopic pelvic exenteration for locally advanced or recurrent colorectal cancer. Surg Laparosc Endosc Percutan Tech. 2019;29(5):389–92.
Nonaka T, Tominaga T, Akazawa Y, Sawai T, Nagayasu T. Feasibility of laparoscopic-assisted transanal pelvic exenteration in locally advanced rectal cancer with anterior invasion. Tech Coloproctol. 2021;25:69–74.
Rios-Doria E, Filippova OT, Straubhar AM, Chi A, Awowole I, Sandhu J, Broach V, Mueller JJ, Gardner GJ, Jewell EL. A modern-day experience with Brunschwig’s operation: outcomes associated with pelvic exenteration. Gynecol Oncol. 2022;167(2):277–82.
Karkia R, Tailor A, Ellis P, Madhuri T, Scala A, Read J, Perry M, Patil K, Blackburn A, Butler-Manuel S. Minimally invasive pelvic exenteration for gynaecological malignancy: a single-centre case series and review of the literature. Eur J Obstet Gynecol Reproductive Biology. 2022;274:56–61.
Quyn AJ, Murthy S, Gould L, Said H, Tiernan J, Sagar P, Antoniou A, Jenkins I, Burns EM. Clinical and oncological outcomes of pelvic exenteration surgery for anal squamous cell carcinoma. Colorectal Dis. 2023;25(11):2131–8.
Naha U, Khurshudyan A, Vigneswaran HT, Mima M, Abern MR, Moreira DM. Perioperative outcomes in male patients undergoing cystectomy, radical colorectal procedure or total pelvic exenteration. Translational Androl Urol. 2023;12(11):1631.
Ralston C, Hainsworth A, de Naurois J, Schizas A, George M. Is an uncomplicated postoperative recovery following total pelvic exenteration a more important prognostic factor than achieving R0 in the first 2 years? Colorectal Dis 2023.
Saqib SU, Raza MZ, Twigg J, Altan O, Bajwa AA. Feasibility of robotic platform to perform R0 resection for locally advanced multi-visceral pelvic malignancy: an institutional experience on outcomes of robotic pelvic exenteration. Langenbeck’s Archives Surg. 2023;409(1):9.
Beppu N, Ito K, Otani M, Imada A, Matsubara T, Song J, Kimura K, Kataoka K, Kuwahara R, Horio Y. Feasibility of transanal minimally invasive surgery for total pelvic exenteration for advanced primary and recurrent pelvic malignancies. Tech Coloproctol 2023:1–9.
Valstad H, Eyjolfsdottir B, Wang Y, Kristensen G, Skeie-Jensen T, Lindemann K. Pelvic exenteration for vulvar cancer: postoperative morbidity and oncologic outcome–A single center retrospective analysis. Eur J Surg Oncol. 2023;49(9):106958.
Download references
Not Funded.
Author information
Arezoo Esmailzadeh and Mohammad Sadegh Fakhari contributed equally to this work.
Authors and Affiliations
Department of Obstetrics & Gynecology, Trauma Research Center, Baqiyatallah University of Medical Sciences, Tehran, Iran
Arezoo Esmailzadeh
Student Research Committee, Arak University of Medical Sciences, Arak, Iran
Mohammad Sadegh Fakhari
Fellowship of Perinatology, Department of Gynecologic Oncology, Tehran University of Medical Sciences, Tehran, Iran
Nafise Saedi
Fellowship of Female Pelvic Medicine and Reconstructive Surgery, Yas Women Hospital, Tehran University of Medical Sciences, Tehran, Iran
Nasim Shokouhi
Department of Epidemiology, Arak University of Medical Sciences, Arak, Iran
Amir Almasi-Hashiani
Traditional and Complementary Medicine Research Center, Arak University of Medical Sciences, Arak, Iran
You can also search for this author in PubMed Google Scholar
Contributions
AAH, AE, MSF, NS and NS conceived the study. AAH, AE, MSF, NS and NS contributed to the title, abstract and full-text screening. Data extraction was done by MSF, AE and AAH, and AAH and MSF analyzed the data. All authors contributed equally to the initial draft of the manuscript. All authors have read and approved the final version of the manuscript.
Corresponding author
Correspondence to Amir Almasi-Hashiani .
Ethics declarations
Ethics approval and consent to participate.
Not applicable.
Consent for publication
Competing interests.
The authors declare no competing interests.
Additional information
Publisher’s note.
Springer Nature remains neutral with regard to jurisdictional claims in published maps and institutional affiliations.
Rights and permissions
Open Access This article is licensed under a Creative Commons Attribution 4.0 International License, which permits use, sharing, adaptation, distribution and reproduction in any medium or format, as long as you give appropriate credit to the original author(s) and the source, provide a link to the Creative Commons licence, and indicate if changes were made. The images or other third party material in this article are included in the article’s Creative Commons licence, unless indicated otherwise in a credit line to the material. If material is not included in the article’s Creative Commons licence and your intended use is not permitted by statutory regulation or exceeds the permitted use, you will need to obtain permission directly from the copyright holder. To view a copy of this licence, visit http://creativecommons.org/licenses/by/4.0/ . The Creative Commons Public Domain Dedication waiver ( http://creativecommons.org/publicdomain/zero/1.0/ ) applies to the data made available in this article, unless otherwise stated in a credit line to the data.
Reprints and permissions
About this article
Cite this article.
Esmailzadeh, A., Fakhari, M.S., Saedi, N. et al. A systematic review and meta-analysis on mortality rate following total pelvic exenteration in cancer patients. BMC Cancer 24 , 593 (2024). https://doi.org/10.1186/s12885-024-12377-5
Download citation
Received : 19 February 2024
Accepted : 13 May 2024
Published : 15 May 2024
DOI : https://doi.org/10.1186/s12885-024-12377-5
Share this article
Anyone you share the following link with will be able to read this content:
Sorry, a shareable link is not currently available for this article.
Provided by the Springer Nature SharedIt content-sharing initiative
- Total pelvic exenteration
- Colorectal neoplasms
- Gynecological neoplasms
- Urologic neoplasms
ISSN: 1471-2407
- Submission enquiries: [email protected]
- General enquiries: [email protected]
- Open access
- Published: 14 May 2024
Comparative analysis of GoPro and digital cameras in head and neck flap harvesting surgery video documentation: an innovative and efficient method for surgical education
- Xin-Yue Huang 1 na1 ,
- Zhe Shao 1 , 2 na1 ,
- Nian-Nian Zhong 1 ,
- Yuan-Hao Wen 1 ,
- Tian-Fu Wu 1 , 2 ,
- Bing Liu 1 , 2 ,
- Si-Rui Ma 1 , 2 &
- Lin-Lin Bu 1 , 2
BMC Medical Education volume 24 , Article number: 531 ( 2024 ) Cite this article
90 Accesses
Metrics details
An urgent need exists for innovative surgical video recording techniques in head and neck reconstructive surgeries, particularly in low- and middle-income countries where a surge in surgical procedures necessitates more skilled surgeons. This demand, significantly intensified by the COVID-19 pandemic, highlights the critical role of surgical videos in medical education. We aimed to identify a straightforward, high-quality approach to recording surgical videos at a low economic cost in the operating room, thereby contributing to enhanced patient care.
The recording was comprised of six head and neck flap harvesting surgeries using GoPro or two types of digital cameras. Data were extracted from the recorded videos and their subsequent editing process. Some of the participants were subsequently interviewed.
Both cameras, set at 4 K resolution and 30 frames per second (fps), produced satisfactory results. The GoPro, worn on the surgeon’s head, moves in sync with the surgeon, offering a unique first-person perspective of the operation without needing an additional assistant. Though cost-effective and efficient, it lacks a zoom feature essential for close-up views. In contrast, while requiring occasional repositioning, the digital camera captures finer anatomical details due to its superior image quality and zoom capabilities.
Merging these two systems could significantly advance the field of surgical video recording. This innovation holds promise for enhancing technical communication and bolstering video-based medical education, potentially addressing the global shortage of specialized surgeons.
1) The GoPro camera offers stable vision and does not require an assistant for operation.
2) A digital camera provides images of higher quality and better anatomical detail.
3) Combining the two could result in a highly efficient and innovative method.
4) Trainees provided positive feedback on the educational impact of the videos.
Peer Review reports
Introduction
Innovation often occurs when knowledge from different disciplines converges and new ideas emerge or merge to foster progress [ 1 ]. Technological advancements have introduced innovations and tools that have entered head and neck surgical practice, ranging from the operating microscope and robotic, imaging-based navigation to computer-assisted design and perfusion monitoring technologies, providing precision care and better patient prognoses [ 1 , 2 , 3 , 4 ]. The combination of video recording and streaming with head and neck reconstructive surgery enables recording the surgeon’s view, allowing others to see exactly what the surgeon observes and does. Video recording technology can also be beneficial in various areas, such as technical communication, research, case data backup, and clinical education. As the saying goes, “A picture is worth a thousand words,” but video holds more convincing power than pictures alone. In the field of head and neck surgery, medical students and junior surgical trainees often do not acquire the full range of surgical skills during their operating room clerkships [ 5 ]. Simultaneously, the global shortage and uneven distribution of the surgical workforce are gaining recognition, with low- and middle-income countries (LMICs) in dire need of skilled surgeons [ 6 ]. There is significant demand for surgical videos in surgical education and surgeon training, especially as COVID-19 ravaged the world, affecting many residents’ clinical practice schedules to varying degrees [ 7 , 8 ]. Consequently, teaching surgical skills has become more challenging.
Digital video capture during surgical procedures is an essential technology in modern-day surgical education [ 9 , 10 , 11 , 12 ]. The advent of fifth-generation mobile technology (5G) has facilitated the distribution of video formats, making it as effortless as sharing text and picture formats in the past, no longer constrained by mobile devices or network bandwidth. Recording surgeries in video format is employed across various domains, such as open surgery, microsurgery, laryngoscopy, and laparoscopy, yielding excellent outcomes regarding video quality and educational purposes [ 13 , 14 , 15 , 16 , 17 ]. Its benefits include 1) assisting medical students in their training, 2) enhancing comprehension of the surgical procedure and the patient’s clinical condition, 3) visualizing crucial routine manual operations, such as flap harvesting, 4) aiding in the preservation of legal evidence, and 5) providing a more precise anatomical description of body regions [ 18 ]. These critical aspects are challenging to convey effectively through descriptions, even with the support of photographs and other media.
The high construction costs associated with a dedicated medical recording system in the operating room can be prohibitive for some hospitals and medical institutions in LMICs and developed countries. Fortunately, due to the rapid advancement of technological innovation in recent years, personal digital video technologies have become more affordable and offer good image quality. Previous studies have also demonstrated that these technologies when applied to surgical video recording, can yield positive results [ 19 , 20 ]. However, few studies have compared different types of camera systems for surgical recordings.
Our study compared the GoPro (Hero 8 Black), a low-cost commercially available action camera, with two higher-priced commercial digital cameras (Canon EOS R5 and EOS 850D). We preliminarily explored other types of surgical video recording (Figure S1), as flap harvesting is a crucial operation in head and neck reconstructive surgery with significant teaching values. Our research focused on comparing the video recording outcomes of these two camera systems during flap harvesting procedures. This study aimed to identify a straightforward, high-quality approach to recording surgical videos at a low economic cost in the operating room, thereby contributing to enhanced patient care.
Materials and methods
The recordings were taken in the Department of Oral & Maxillofacial—Head Neck Oncology at the Hospital of Stomatology, Wuhan University, from November to December 2021. A total of six operations were prospectively recorded. All patients signed informed consent forms before surgery, and the recordings did not involve any parts of the patients’ bodies outside the operative areas.
GoPro is a brand of action camera that can be attached to the body with simple accessories, enabling hands-free recording and first-person perspectives, especially in extreme sports. The GoPro HERO 8 Black (GoPro Inc, San Mateo, CA), used in this study, is currently a widely recognized product. This camera is exceptionally compact and portable, measuring 62*33.7*44.6 mm and weighing 450 g. The GoPro 8 supports stabilized 4 K video recording at 30 or 60 frames per second (fps) and slow-motion 1080P video at 240 fps. It is equipped with the HyperSmooth system, which stabilizes the video image without the need for external stabilizers, even when the surgeon wearing the device is moving. It can also connect to a smart device via a wireless network during filming to monitor the shot or even broadcast live using the GoPro Quik app. The fixed focus setting on this device maintains consistent focus, regardless of whether the subject gets closer or moves further away within a certain distance.
Generally, the term “digital camera” may also refer to the camera systems integrated into smartphones (such as an iPhone). However, surgical videos require precise documentation of operations on delicate anatomical structures, and our previous pilot study found that the images captured by smartphones (iPhone X) did not meet the requirements for teaching or technical communication. Therefore, the “digital camera” referenced in this article pertains specifically to professional digital cameras. We utilized two relatively recent models on the market, the EOS R5, Canon’s flagship product, and the EOS 850D, its entry-level counterpart.
A total of six operations were prospectively studied, involving three surgeons, seven circulating nurses, and ten surgical residents.
The surgeon wore the GoPro 8 camera attached to a unique headband (Fig. 1 ), with no additional loupes or head-mounted lighting systems to physically interfere with the camera. An iPad, connected to the GoPro and equipped with the GoPro Quik app, served as a viewfinder and remote control for recording the six operations.
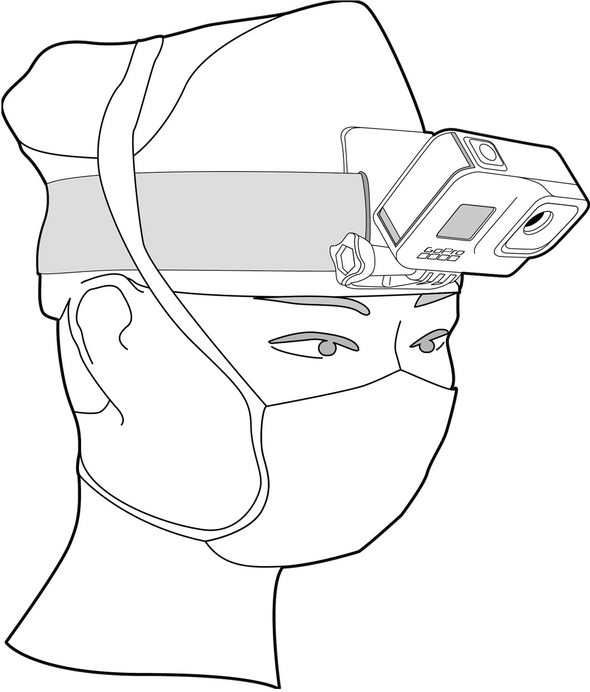
The surgeon with the head-mounted camera in place to record the surgery
The digital cameras were mounted on an external tripod for recording and were set to manual mode with manual focus. The first three recordings observed that the surgical team members occasionally obscured the surgical area. Therefore, the tripod setup was modified in the subsequent three recordings, drawing on previous studies’ methods to attain a better field of view (FOV) (Fig. 2 ) [ 21 ]. The sixth surgery was recorded using an EOS 850D, while the others were documented with an EOS R5.
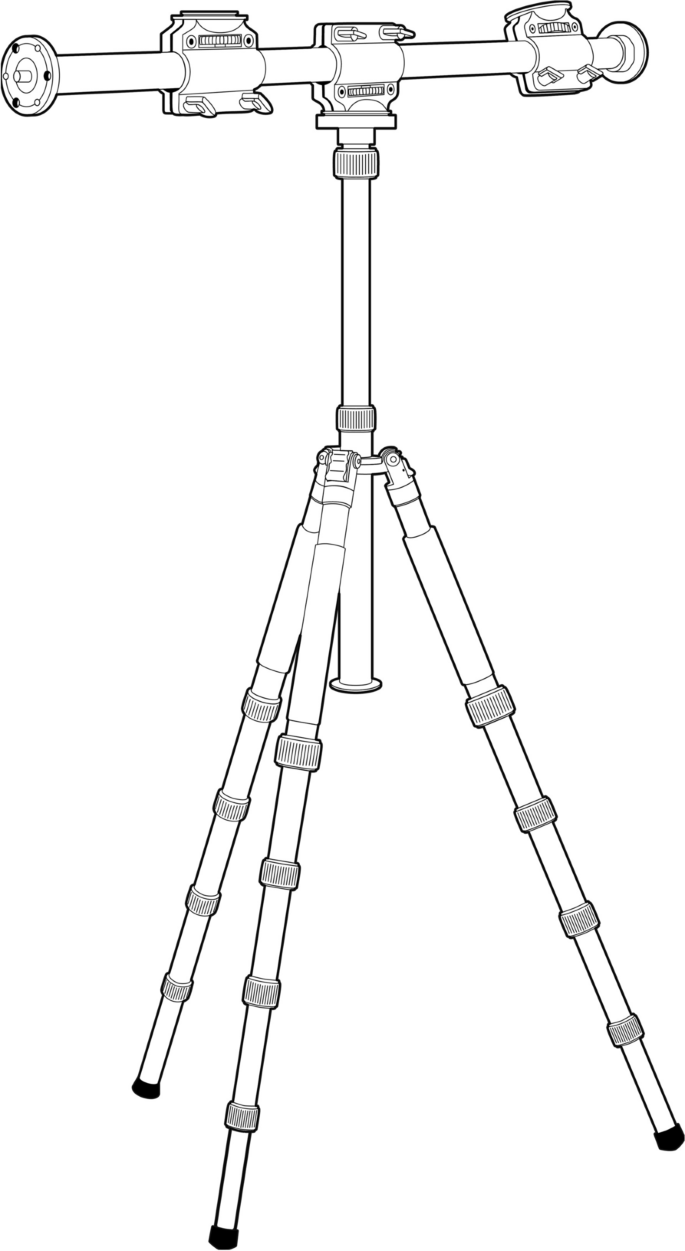
Post-assembly view of the modified tripod
Characterization of videos
Data for this study were extracted from the recorded videos and their subsequent editing process. Selected variables were entered into a Microsoft Excel database. The evaluated variables included: 1) the total recording time for each device during all surgeries; 2) the duration of all surgical procedures; 3) the duration of unavailable video (including obscured surgical areas, inaccurate focus, and overexposure) and their percentage of the entire surgical procedure; and 4) the file size of the original videos.
Educational impact analysis
To assess the quality of the recorded surgical videos and their applicability in teaching, we designed a questionnaire to gather opinions from three surgeons and nine resident physicians (students) on the edited videos. The surgeons’ questionnaire primarily assessed whether the videos clearly conveyed the surgeon’s operational concepts and specific details. Trainees were queried whether they could clearly view the procedures and derive educational benefits from it.
To further analyze the educational impact of the surgical videos, we established an Expert Review Panel (ERP) comprising five experts with over ten years of experience in clinical surgery and medical student education. We also created an assessment table for evaluating surgical education video (Table 1 ). The ERP reviewed six edited surgical videos and evaluated their instructional quality, clarity, stability, and effectiveness in conveying surgical techniques. Subsequently, the table was completed to categorize the overall quality of each video.
It took approximately 10 min to prepare the video equipment before surgery, including setting up the tripod, determining the recording settings, and adjusting the camera’s position. All surgeons reported that the head-mounted recording device did not interfere with the operation. The operations involved different types of flap harvesting, including ilium flap harvesting ( n = 3), fibula flap harvesting ( n = 1), anterolateral thigh flap harvesting ( n = 1), and forearm flap harvesting ( n = 1). The average duration of the operations was one and a half hours. Six surgical procedures were recorded simultaneously using both the GoPro and digital cameras. The characteristics of each surgical video are shown in Table 2 . The technical details of the two types of cameras used in the study (GoPro HERO 8 Black, EOS R5, and EOS 850D) are summarized in Table 3 . A video of the sample can be found in the Supplementary Video.
Video settings
While filming surgeries, we consulted previous studies for camera settings. Graves et al. conducted research using a GoPro camera in the operating room [ 20 ]. They selected an earlier type—the GoPro HERO 3 + Black—and concluded that with a narrow FOV, automatic white balance, 1080P resolution, and 48 fps, one could achieve high-quality, low-cost video recordings of surgical procedures. In our study, we initially tried a 1080P resolution with a narrow field and obtained relatively good results (Fig. 3 A). However, a 1080P resolution HD video consists of two million pixels (1920 × 1080), whereas a 4 K (Ultra HD) video comprises over eight million pixels (3840 × 2160). Thus, 4 K video produces a sharper image with four times the resolution of 1080P. Given that the GoPro does not support narrow-field shooting at 4 K resolution, we discovered that setting it to a “linear” FOV with 4 K resolution provided more precise and crisper imagery (Fig. 3 B). In most of our recordings, we used 4 K resolution and a frame rate of 30 fps for both the GoPro and digital cameras.
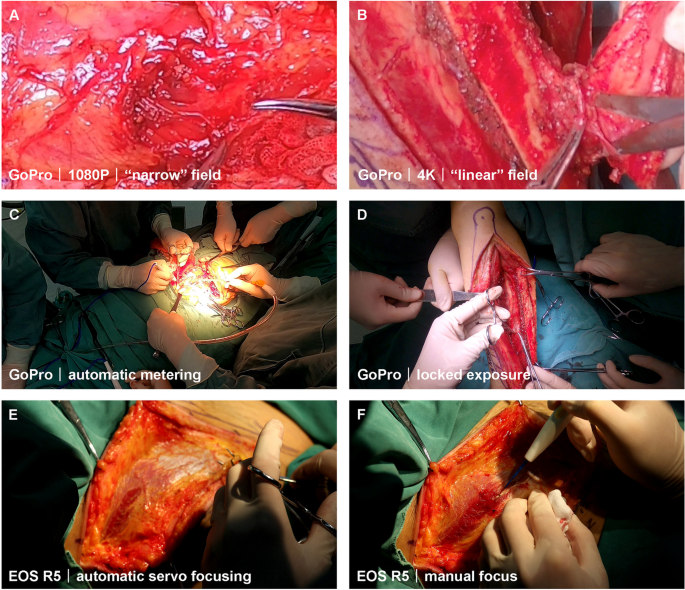
Image quality comparison of video screenshots with the magnification of 500% obtained from ( A ) 1080P narrow field and ( B ) 4K linear field. C When set to automatic metering, the intraoperative area was overexposed, and ( D ) the area was normally exposed after locked exposure. E In automatic servo focusing mode, the focal plane is not in the area but in the operator’s hand. F Manual focus keeps the focus on the area, even if the operator’s hand is covered
Light metering involves determining the necessary exposure based on environmental conditions, which can be manually adjusted by the photographer with different exposure settings or automatically by the camera’s program. The light environment of the operating room is complex, as the surgical field illuminated by a shadowless lamp is typically brighter than the surrounding area. When recording the surgical area with a digital camera that permits manual operation, it is recommended to use a smaller aperture (an opening that allows light to reach a lens) to reduce light intake and increase the depth of field (DOF), which is the range within which objects appear sharp. Although the GoPro was set to automatic metering mode due to the difficulty of manual operation, its FOV shifted with the surgeon’s head movements. As bright lights continually focused on the surgical area, rapid changes in the FOV easily caused overexposure in the operating area (Fig. 3 C). This issue was later addressed by locking the exposure before recording (Fig. 3 D).
The first recorded video revealed that the digital camera’s automatic servo focusing caused instability in the focal plane within the operational area due to various instruments and the surgeon’s hands in the surgical field (Fig. 3 E). This issue was addressed by manually focusing and locking the focal plane before recording (Fig. 3 F). However, when the position changed during the procedure, an assistant without surgical hand disinfection was required to adjust the camera promptly.
Quality of videos
The image quality of videos from three cameras was sufficient for depicting static and moving objects. However, the operating room is a unique environment where multiple factors influence the cameras’ effectiveness. These factors include the distance from the operating area, obstruction by surgical team members [ 22 ], the lens’s FOV, light overexposure, and reflection from metal instruments.
To more clearly compare the video quality of the three devices across six different head and neck reconstructive procedures, we extracted images from the video files of all devices and assessed their clarity at magnifications of 100% and 300%. Fig. 4 A-C show 100% images alongside detailed 300% magnified images captured from videos recorded by the GoPro8, EOS 850D, and EOS R5, respectively. All three devices provided precise and reliable output under various circumstances and lighting conditions.
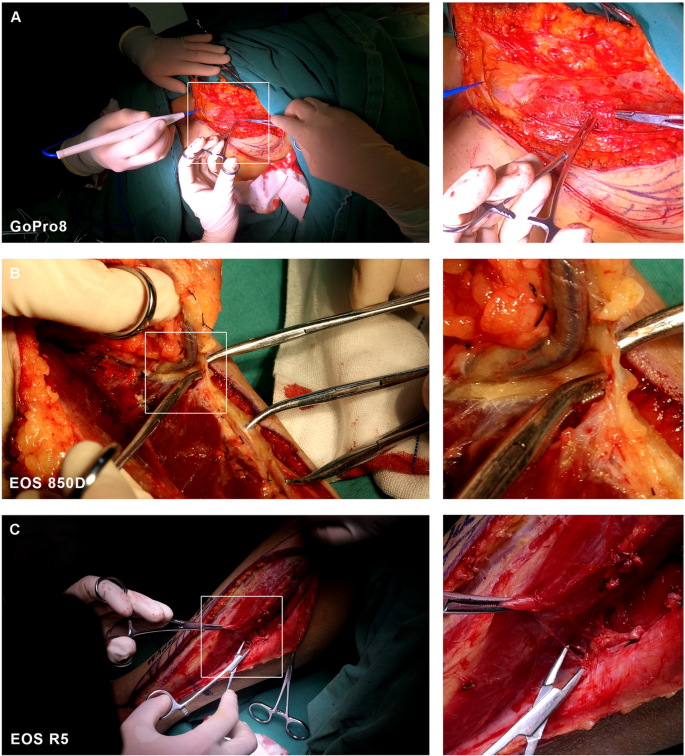
A 100% image from uncompressed file video of GoPro8, compared with magnified 300% video image in detail. B 100% image from uncompressed file video of EOS 850D, compared with magnified 300% video image in detail. C 100% image from uncompressed file video of EOS R5, compared with magnified 300% video image in detail
Positioning in the operative room
Digital cameras capture high-definition images during surgery with accurate focus. However, there were instances when the lens was obscured by the bodies of surgical team members or instruments, missing critical moments. As shown in Table 2 , videos recorded with a digital camera placed on an unmodified tripod had a higher rate of unavailable video duration, primarily due to obscuration. The tripod was modified to position the camera’s FOV more perpendicular to the surgical area. Consequently, the average duration of unusable video recorded by the digital camera using the modified tripod was 11.3%, a significant decrease from the 37.55% average without modification.
Figure 5 depicts the positioning of the modified tripod, head-mounted camera, and surgical field used for the recording.
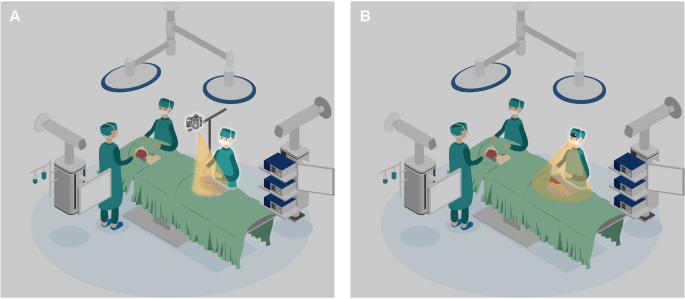
The position used for the recording with the surgical field and surgeon. A Ilium flap harvesting with digital camera. B Ilium flap harvesting with GoPro
Field of view
In the recordings of Anterolateral thigh flap harvesting, Ilium flap harvesting 2, and Fibula flap harvesting surgeries, the use of the GoPro resulted in a lower duration of unavailable video (1.9%, 2.5%, and 2.0%, respectively) compared to digital cameras (29.5%, 14.7%, and 5.5%), even with the tripod modified for the latter two recordings. This outcome is primarily because the surgeon’s hand often blocked the digital camera, positioned for a third-person perspective. In contrast, the GoPro, attached to the surgeon’s head, offered a viewpoint closer to the surgeon’s own eyes, thereby capturing a better visual field. The surgeon’s perspective is arguably the most advantageous, as corroborated by many previous studies that placed the camera on the surgeon’s forehead for procedural recording [ 13 , 20 , 23 ]. Rafael et al. reported that the head camera position was well-received by volunteers [ 24 ]. Though we obtained valuable images this way, there were limitations. The angle from the eye to the target point varies with the surgical techniques. Digital cameras can easily shift focus by adjusting the tripod’s position and angle to maintain the view of the surgical area; however, the GoPro’s FOV and focus are fixed upon installation, allowing horizontal adjustments, occasionally resulting in the surgical area going out of frame. Nevertheless, the GoPro’s wide FOV in both “wide” and “linear” modes generally ensures that the area remains within the shot without continuous monitoring.
Based on the images extracted from the videos, the digital camera can achieve a more detailed view of the surgical area with its zoom capabilities compared to the GoPro’s wider FOV. Although the GoPro’s images reveal clear anatomical structures upon magnification, they are not as sharp as those from the digital camera. This limitation, however, had unexpected benefits, as it could record the surgeon’s hand movements between the patient’s tissues and the instruments, providing insights into surgical hand positioning and instrument ergonomics that are crucial for training but often overlooked [ 23 ]. Experienced surgeons efficiently organize their workspace, holding instruments currently in use while preparing others for subsequent steps. On-site trainees, focusing primarily on the operative site, may miss these subtle ergonomic maneuvers. When used in education, surgical recordings simultaneously displaying the operative site and hand positioning can offer learners vital insights previously unnoticed [ 25 ].
Connectivity
All three devices possess the capability for wireless connectivity via Wi-Fi or Bluetooth systems. Video captured by these devices can be streamed in real-time to nearby mobile devices or monitors and can even be broadcast online. This feature forms the foundation for remote tele-proctoring and education purposes in surgery, a method proven to be innovative for enhancing surgical education in high-resource settings [ 26 ]. Fig. 6 illustrates the connectivity scheme, which includes a wireless link between the cameras and mobile devices through Wi-Fi or Bluetooth, facilitating further dissemination by these devices.
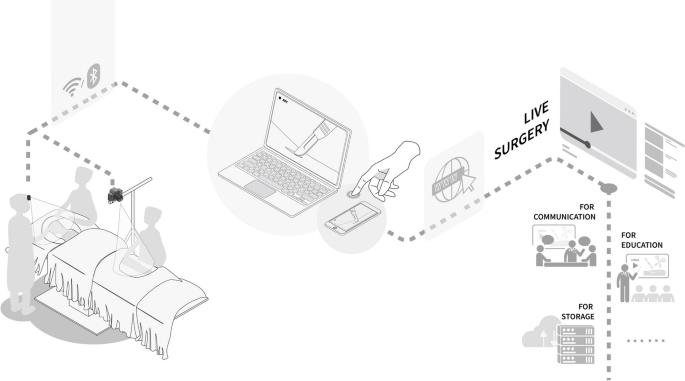
The camera can be connected to a mobile phone or laptop via Wi-Fi or Bluetooth or even broadcast live via the Internet for more purposes
In addition, the GoPro device itself comes equipped with a Livestream function in full 1080P HD mode. However, the video quality of the Webcast is not as high as the recordings due to limitations imposed by wireless connection speeds and bandwidth. The "choppy" nature of the video presentation during streaming can be mitigated by using a direct cable for live broadcasts, allowing direct streaming onto a monitor for local presentation or broader live broadcasts, and offering a quality superior to Wi-Fi or Bluetooth options. The downside is the cumbersome nature of the required cables.
Editing of video
Benefiting from the high resolution of 4K video, high definition is maintained even after the original video clip is magnified. Structural details are well-preserved, and the clarity of the operation remains evident in the magnified version, which can be further saved or shared. GoPro Quik, an application developed by the GoPro company, facilitates customized video editing. It can be used to edit original clips shot by the GoPro camera, re-adjust the field of interest, and conveniently export the video in the appropriate format. High-resolution video has its pros and cons. The extensive data involved makes storing and editing raw video files challenging. Future technologies should enable surgeons to ensure real-time recording of the area of interest, allowing for more manageable data acquisition without the need for zooming or cropping post-capture.
Videos for education
The results of the questionnaire were as follows. In the surgeons’ group, 100% ( n = 3) confirmed that the videos well represented the details of their operations. In the students’ group, 66.7% of respondents ( n = 6) rated the image quality with GoPro as excellent, and 33.3% ( n = 3) found it fine, while for the digital camera, 88.9% of respondents ( n = 8) rated it as excellent and 11.1% ( n = 1) as fine (Fig. 7 ). All respondents ( n = 9) positively affirmed that they could learn professional skills from the videos. In the evaluation conducted by the Expert Review Panel, of the six videos, four were considered suitable for clinical teaching applications, one was also suitable but required a better replacement, and one was deemed unsuitable for clinical teaching applications. However, these results must be interpreted with caution due to the small sample size.
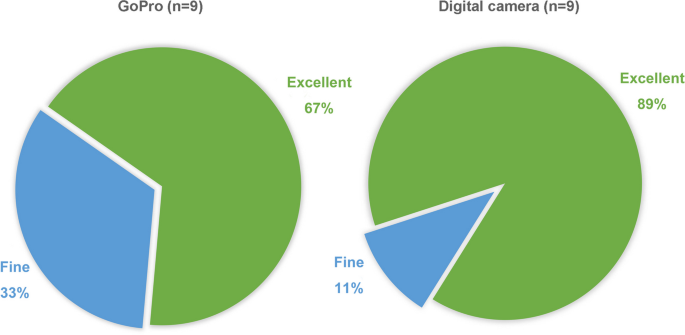
Trainees reported satisfaction degrees from surgical videos, according to whether they can see the procedure clearly and learn from it—comparison of GoPro versus digital camera
With the increasing demand for technical communication, medical teaching, surgical procedure recording, and so on, surgical video has become a popular multimedia mode. It is a powerful medium that can enhance patient safety in several ways: education, real-time consultation, research, process improvement, and workflow coordination [ 27 ]. Operation videos can be transmitted through the internet in real-time, providing a platform for communication and cooperation between hospitals. Experienced surgeons can assess trainees’ surgical competency in an unbiased fashion through the trainees’ intraoperative video [ 28 ]. Experienced individual surgeons hope to share their professional knowledge and skills through surgical videos and achieve the purpose of self-publicity. Regarding privacy protection, Turnbull et al. emphasized that video documentation has significant ethical and legal considerations as it contains personal information and infringes on patients’ privacy [ 29 ]. The patient’s privacy should be carefully considered to avoid potential ethical and legal conflicts brought about by filming operations.
Pros and cons of two camera systems
The introduction of video technology into surgical procedures is becoming more common, and high-resolution camera technology has been integrated into surgical instrumentation for laparoscopic and minimally invasive procedures [ 30 ]. Although technology continuously evolves, leading to the adoption of many new technologies in intraoperative video recording, there are still limitations in devices for capturing open surgery. Due to economic conditions and space constraints, operating rooms are not routinely equipped with video recording equipment, making personal recording equipment a more viable solution. This study compared two technologies (GoPro and digital camera) used for intraoperative video capture in open surgeries and summarized their advantages and disadvantages (Table 4 ).
GoPro cameras are designed for extreme sports, featuring high resolution, high frame rates, small image sensors, and a lack of complete manual control. They are light and portable enough to be worn on a surgeon’s head, providing an image that approximates the natural field of vision without hindering the operation. Simultaneously, their built-in stabilizer function ensures the output image remains stable and visible. Being waterproof, they can be soaked in povidone-iodine for disinfection, facilitating hand-held shooting [ 31 ]. Existing studies confirm that this disinfection method does not compromise asepsis [ 32 ]. The built-in Wi-Fi and Bluetooth allow for remote monitoring and real-time transmission of intraoperative video. The affordability of GoPro enables doctors wanting to record surgeries to do so cost-effectively, making them accessible to surgeons from LMICs. However, the downsides are clear: users in the operating room are more likely to obtain a narrower FOV aimed at the surgical area, but the GoPro, as an action camera, is designed to capture as comprehensive a panoramic view as possible. Due to the absence of manual controls, it does not adapt well to frequent brightness changes caused by bright overhead operating room lights. Additionally, the battery capacity of the GoPro lasts approximately 60 min and may shut down if its body temperature reaches an upper limit during prolonged sessions. For extened recording times, spare batteries are necessary, and consideration of the device temperature is essential.
Digital cameras, due to their optimal optical performance and excellent zoom capabilities, can capture specific areas of interest in high quality. They are typically more durable and are generally equipped with larger image sensors, better adapting to unfavorable lighting conditions. Their robust maneuverability makes them suitable for the complex operating room environment. Digital cameras were not widely used for surgical video recording due to their high cost. However, this study shows that even inexpensive digital cameras, such as the EOS 850D, can produce adequate surgical videos. The picture quality is not significantly different from the much pricier EOS R5 when using the same 4 K 30 fps model. Of course, more expensive cameras like the EOS R5, which supports 8 K quality video, allow for a better representation of delicate anatomy.
Nevertheless, these cameras’ drawback is that they always require supports like tripods or rocker arms for steady recording. The positioning, height, and relationship with the surgical team determine the final video quality. Furthermore, an additional assistant is needed to adjust camera positions and video settings to maintain the appropriate shooting angle during the procedure. This camera operator might need to direct the surgeon to stop and start at different points throughout the surgery, potentially interfering with the surgical team. The risk of breaking sterility should also be considered when introducing an extra individual into the operating room. This cumbersome and time-consuming shooting method does not lend itself to daily, routine intraoperative videotaping.
Using either a GoPro or a digital camera is a commendable choice. According to our research, the GoPro is a highly efficient option that is better suited for personal recording and can be operated easily without an assistant. Digital cameras, though requiring additional assistance, deliver higher output quality. If the two are innovatively combined, images from different fields of vision can be captured to produce rich, comprehensive, and high-quality videos.
Application of surgical video in education and other aspects
Surgical video holds broad application prospects in medical teaching, technical communication, patient safety, workflow coordination, case data backup, research, real-time consulting, and skill improvement. With the advancement of communication facilities, real-time video recording during surgery presents extensive development prospects akin to digital twin technology [ 33 , 34 ]. Mentoring through this medium can enhance quality and patient safety throughout a medical student’s career. Future developments may involve coaching sessions or honing non-technical skills, such as optimizing teamwork in the operating room to elevate patient care.
Medical students’ journey to becoming surgeons critically requires specific technical feedback while developing foundational skills during their internships. Despite the importance of targeted feedback, medical students often endure inconsistent, fragmented, and stressful experiences in the operating room [ 5 ]. Compounding these challenges, a study on oral and maxillofacial surgery trainees in the United States revealed that the COVID-19 pandemic disrupted the scheduling of non-urgent and elective operations [ 35 ]. With approximately 2.28 million more skilled medical professionals needed to meet the global demand for surgical procedures [ 6 ], training a substantial cohort of future surgeons is a pressing, worldwide challenge.
In addition to traditional book learning and clinical practice, watching surgical videos can help medical students acquire technical details related to surgical operations more precisely, and some critical but fleeting points can be repeated during video playback. Video-based interventions to enhance surgical skills are gaining attention for their educational applications and related research [ 12 ]. The use of video technology in teaching is relatively common in other fields, including sports. In head and neck surgery, some advantages of utilizing high-quality surgical recordings as educational tools are as follows: 1) They provide clear, sharp images that depict fine anatomical structure; 2) Learning through videos offers a more intuitive experience, as viewing surgery footage from a first-person perspective affords residents a more immersive sensation, encouraging them to conceptualize the surgery from the surgeon’s viewpoint; 3) Video recordings of resident physicians’ operations facilitate the assessment of their skill levels, paving the way for enhanced performance; 4) Essential intraoperative findings can be documented and elucidated; 5) The zoom feature enables close-up, detailed recording of surgical procedures and anatomical nuances.
Leveraging the Wi-Fi and Bluetooth capabilities of recording devices, real-time videos can be streamed to mobile phones or laptops or even broadcast live over the internet for tele-proctoring. This emerging technology allows instructors to provide real-time guidance and technical support through audio and video interactions from various geographical locations. This method effectively circumvents the additional logistical costs, time constraints, and challenges posed by distance that are inherent when instructors physically travel to the field [ 36 ]. McCullough et al. [ 26 ] previously explored the feasibility of wearable recording technology in expanding the reach and availability of specialized surgical training in LMICs, using Mozambique as a case study. Their research suggests that this educational model connects surgeons globally and fosters advanced mentoring in regions where surgical trainees have limited opportunities.
Limitations
The findings of this study must be considered within the context of certain limitations. The research was single-centered with a limited number of surgeons involved, and only a single brand of digital camera was selected, which may lead to a lack of diversity and overlook ergonomic differences between types of surgeries and the subtle imaging details between different camera manufacturers. The assessment of the impact of video on teaching also had a small sample size, so potential biases in questionnaire feedback should be considered. Furthermore, there is a persistent need for objective and repeatable metrics to conclusively demonstrate the efficacy of camera technology in clinical education, continuous performance improvement, and quality enhancement initiatives.
Considering that the primary aim of this study was to compare and recommend a high-quality approach for recording surgical videos, future research will focus on conducting multi-centered studies with larger sample sizes and emphasis on the diversity of surgical specialties and camera brands. It is also essential to assess its application more effectively in a learning experience in surgical education, not only in head and neck surgery but also in other surgical areas. Future studies will improve the evaluation of skill levels through practical techniques and written exams, study learning curves in relation to surgical timing, analyze cost-effectiveness, and gather evaluations from the trainer’s perspective.
The field of head and neck surgery has consistently welcomed innovation, embracing the introduction of new techniques into surgical practice. There is a substantial demand and room for development in the domain of open surgical recordings. Surgical video recording serves the purpose of technical communication and accomplishes the objective of medical education through real-time connectivity, addressing the current global shortage of specialized surgeons. The two systems examined in this study, the GoPro and the digital camera, each have distinct features and advantages. The GoPro, an affordable and physician-independent solution, offers a stable and continuous view of the surgical area, though it lacks a medical-specific design and a zoom function. On the other hand, despite requiring periodic repositioning and potentially distracting the surgical team, the digital camera delivers superior visibility of anatomical details and higher image quality.
Availability of data and materials
The data supporting the findings of this study are available within the article and its supplementary materials.
Suchyta M, Mardini S. Innovations and future directions in head and neck microsurgical reconstruction. Clin Plast Surg. 2017;44(2):325–44. https://doi.org/10.1016/j.cps.2016.11.009 .
Article Google Scholar
Tarsitano A, Battaglia S, Ciocca L, Scotti R, Cipriani R, Marchetti C. Surgical reconstruction of maxillary defects using a computer-assisted design/computer-assisted manufacturing-produced titanium mesh supporting a free flap. J Craniomaxillofac Surg. 2016;44(9):1320–6. https://doi.org/10.1016/j.jcms.2016.07.013 .
Rana M, Essig H, Eckardt AM, et al. Advances and innovations in computer-assisted head and neck oncologic surgery. J Craniofac Surg. 2012;23(1):272–8. https://doi.org/10.1097/SCS.0b013e318241bac7 .
Poon H, Li C, Gao W, Ren H, Lim CM. Evolution of robotic systems for transoral head and neck surgery. Oral Oncol. 2018;87:82–8. https://doi.org/10.1016/j.oraloncology.2018.10.020 .
Alameddine MB, Englesbe MJ, Waits SA. A video-based coaching intervention to improve surgical skill in fourth-year medical students. J Surg Educ. 2018;75(6):1475–9. https://doi.org/10.1016/j.jsurg.2018.04.003 .
Meara JG, Leather AJM, Hagander L, et al. Global surgery 2030: evidence and solutions for achieving health, welfare, and economic development. Lancet. 2015;386(9993):569–624. https://doi.org/10.1016/s0140-6736(15)60160-x .
Huntley RE, Ludwig DC, Dillon JK. Early effects of COVID-19 on oral and maxillofacial surgery residency training-results from a national survey. J Oral Maxillofac Surg. 2020;78(8):1257–67. https://doi.org/10.1016/j.joms.2020.05.026 .
Shah JP. The impact of COVID-19 on head and neck surgery, education, and training. Head Neck. 2020;42(6):1344–7. https://doi.org/10.1002/hed.26188 .
Augestad KM, Lindsetmo RO. Overcoming distance: video-conferencing as a clinical and educational tool among surgeons. World J Surg. 2009;33(7):1356–65. https://doi.org/10.1007/s00268-009-0036-0 .
Hu YY, Mazer LM, Yule SJ, et al. Complementing operating room teaching with video-based coaching. JAMA Surg. 2017;152(4):318–25. https://doi.org/10.1001/jamasurg.2016.4619 .
Augestad KM, Butt K, Ignjatovic D, Keller DS, Kiran R. Video-based coaching in surgical education: a systematic review and meta-analysis. Surg Endosc. 2020;34(2):521–35. https://doi.org/10.1007/s00464-019-07265-0 .
Greenberg CC, Dombrowski J, Dimick JB. Video-based surgical coaching: an emerging approach to performance improvement. JAMA Surg. 2016;151(3):282–3. https://doi.org/10.1001/jamasurg.2015.4442 .
Bizzotto N, Sandri A, Lavini F, Dall’Oca C, Regis D. Video in operating room: GoPro HERO3 camera on surgeon’s head to film operations–a test. Surg Innov. 2014;21(3):338–40. https://doi.org/10.1177/1553350613513514 .
Matsumoto S, Sekine K, Yamazaki M, et al. Digital video recording in trauma surgery using commercially available equipment. Scand J Trauma Resusc Emerg Med. 2013;21:27. https://doi.org/10.1186/1757-7241-21-27 .
Knight H, Gajendragadkar P, Bokhari A. Wearable technology: using Google Glass as a teaching tool. BMJ Case Rep. 2015;2015:bcr2014208768. https://doi.org/10.1136/bcr-2014-208768 .
Liao CH, Ooyang CH, Chen CC, et al. Video coaching improving contemporary technical and nontechnical ability in laparoscopic education. J Surg Educ. 2020;77(3):652–60. https://doi.org/10.1016/j.jsurg.2019.11.012 .
Volz S, Stevens TP, Dadiz R. A randomized controlled trial: does coaching using video during direct laryngoscopy improve residents’ success in neonatal intubations? J Perinatol. 2018;38(8):1074–80. https://doi.org/10.1038/s41372-018-0134-7 .
Giusto G, Caramello V, Comino F, Gandini M. The surgeon’s view: comparison of two digital video recording systems in veterinary surgery. J Vet Med Educ. 2015;42(2):161–5. https://doi.org/10.3138/jvme.0814-088R1 .
Silberthau KR, Chao TN, Newman JG. Innovating surgical education using video in the otolaryngology operating room. JAMA Otolaryngol Head Neck Surg. 2020;146(4):321–2. https://doi.org/10.1001/jamaoto.2019.4862 .
Graves SN, Shenaq DS, Langerman AJ, Song DH. Video capture of plastic surgery procedures using the GoPro HERO 3+. Plast Reconstr Surg Glob Open. 2015;3(2):e312. https://doi.org/10.1097/gox.0000000000000242 .
Kapi E. Surgeon-manipulated live surgery video recording apparatuses: personal experience and review of literature. Aesthetic Plast Surg. 2017;41(3):738–46. https://doi.org/10.1007/s00266-017-0826-y .
Kajita H, Takatsume Y, Shimizu T, Saito H, Kishi K. Overhead multiview camera system for recording open surgery. Plastic and reconstructive surgery Global open. 2020;8(4):e2765. https://doi.org/10.1097/GOX.0000000000002765 .
Warrian KJ, Ashenhurst M, Gooi A, Gooi P. A novel combination point-of-view (POV) action camera recording to capture the surgical field and instrument ergonomics in oculoplastic surgery. Ophthalmic Plast Reconstr Surg. 2015;31(4):321–2. https://doi.org/10.1097/iop.0000000000000465 .
Chaves RO, de Oliveira PAV, Rocha LC, et al. An innovative streaming video system with a point-of-view head camera transmission of surgeries to smartphones and tablets: an educational utility. Surg Innov. 2017;24(5):462–70. https://doi.org/10.1177/1553350617715162 .
Wentzell D, Dort J, Gooi A, Gooi P, Warrian K. Surgeon and assistant point of view simultaneous video recording. Studies in health technology and informatics. 2019;257:489–93.
Google Scholar
McCullough MC, Kulber L, Sammons P, Santos P, Kulber DA. Google glass for remote surgical tele-proctoring in low- and middle-income countries: a feasibility study from Mozambique. Plastic and reconstructive surgery Global open. 2018;6(12):e1999. https://doi.org/10.1097/GOX.0000000000001999 .
Xiao Y, Schimpff S, Mackenzie C, et al. Video technology to advance safety in the operating room and perioperative environment. Surg Innov. 2007;14(1):52–61. https://doi.org/10.1177/1553350607299777 .
Berger AJ, Gaster RS, Lee GK. Development of an affordable system for personalized video-documented surgical skill analysis for surgical residency training. Ann Plast Surg. 2013;70(4):442–6. https://doi.org/10.1097/SAP.0b013e31827e513c .
Turnbull AM, Emsley ES. Video recording of ophthalmic surgery–ethical and legal considerations. Surv Ophthalmol. 2014;59(5):553–8. https://doi.org/10.1016/j.survophthal.2014.01.006 .
Rassweiler JJ, Teber D. Advances in laparoscopic surgery in urology. Nat Rev Urol. 2016;13(7):387–99. https://doi.org/10.1038/nrurol.2016.70 .
Navia A, Parada L, Urbina G, Vidal C, Morovic CG. Optimizing intraoral surgery video recording for residents’ training during the COVID-19 pandemic: Comparison of 3 point of views using a GoPro. J Plast Reconstr Aesthet Surg. 2021;74(5):1101–60. https://doi.org/10.1016/j.bjps.2020.10.068 .
Purnell CA, Alkureishi LWT, Koranda C, Patel PK. Use of a waterproof camera immersed in povidone-iodine to improve intraoperative photography. Plastic and reconstructive surgery. 2019;143(3):962–5. https://doi.org/10.1097/prs.0000000000005327 .
Ahmed H, Devoto L. The potential of a digital twin in surgery. Surg Innov. 2021;28(4):509–10. https://doi.org/10.1177/1553350620975896 .
Laaki H, Miche Y, Tammi K. Prototyping a digital twin for real time remote control over mobile networks: application of remote surgery. IEEE Access. 2019;7:20325–36. https://doi.org/10.1109/access.2019.2897018 .
Hope C, Reilly JJ, Griffiths G, Lund J, Humes D. The impact of COVID-19 on surgical training: a systematic review. Tech Coloproctol. 2021;25(5):505–20. https://doi.org/10.1007/s10151-020-02404-5 .
Ereso AQ, Garcia P, Tseng E, et al. Live transference of surgical subspecialty skills using telerobotic proctoring to remote general surgeons. J Am Coll Surg. 2010;211(3):400–11. https://doi.org/10.1016/j.jamcollsurg.2010.05.014 .
Download references
Acknowledgements
The authors express their gratitude to the surgeons and students who participated in this study. Special thanks are also extended to Dr. Jun Jia and Dr. Kun Lv from the Department of Oral and Maxillofacial Surgery at the School & Hospital of Stomatology, Wuhan University, for their assistance with the surgical cases.
This study was supported by Wuhan University Undergraduate Education Quality Improvement and Comprehensive Reform Project (1607–413200072), Fundamental Research Funds for the Central Universities (Wuhan University, Clinical Medicine + X) (2042024YXB017), Postdoctoral Science Foundation of China (2018M630883& 2019T120688), Hubei Province Chinese Medicine Research Project (ZY20230015), Natural Science Foundation of Hubei Province (2023AFB665), and Medical Young Talents Program of Hubei Province, and Wuhan Young Medical Talents Training Project to L.-L. Bu.
Author information
Xin-Yue Huang and Zhe Shao contributed equally to this work.
Authors and Affiliations
State Key Laboratory of Oral & Maxillofacial Reconstruction and Regeneration, Key Laboratory of Oral Biomedicine Ministry of Education, Hubei Key Laboratory of Stomatology, School & Hospital of Stomatology, Wuhan University, Wuhan, China
Xin-Yue Huang, Zhe Shao, Nian-Nian Zhong, Yuan-Hao Wen, Tian-Fu Wu, Bing Liu, Si-Rui Ma & Lin-Lin Bu
Department of Oral & Maxillofacial - Head Neck Oncology, School & Hospital of Stomatology, Wuhan University, Wuhan, China
Zhe Shao, Tian-Fu Wu, Bing Liu, Si-Rui Ma & Lin-Lin Bu
You can also search for this author in PubMed Google Scholar
Contributions
XYH: Methodology, Investigation, Visualization, Writing—Original Draft, Writing—Review & Editing. ZS: Conceptualization, Investigation, Writing—Original Draft. NNZ: Methodology, Investigation, Writing—Review & Editing. YHW: Visualization, Writing—Review & Editing. TFW: Investigation, Writing—Review & Editing. BL: Writing—Review & Editing, Supervision, Funding acquisition. SRM: Conceptualization, Writing—Review & Editing, Supervision. LLB: Conceptualization, Methodology, Writing—Review & Editing, Supervision, Funding acquisition. All authors have reviewed and approved the final version of this manuscript for publication. Each author agrees to be accountable for all aspects of the work in ensuring that questions related to the accuracy or integrity of any part of the work are appropriately investigated and resolved.
Corresponding authors
Correspondence to Si-Rui Ma or Lin-Lin Bu .
Ethics declarations
Ethics approval and consent to participate.
This study was approved by the Ethics Committee of the School of Stomatology of Wuhan University (Approval No. 2022B11), and followed the guidelines of the Declaration of Helsinki of the World Medical Association. Informed consent was obtained fom all subjects involved in the study.
Consent for publication
Not applicable.
Competing interests
The authors declare no competing interests.
Additional information
Publisher’s note.
Springer Nature remains neutral with regard to jurisdictional claims in published maps and institutional affiliations.
Supplementary Information
Supplementary material 1..
Supplementary Material 2.
Rights and permissions
Open Access This article is licensed under a Creative Commons Attribution 4.0 International License, which permits use, sharing, adaptation, distribution and reproduction in any medium or format, as long as you give appropriate credit to the original author(s) and the source, provide a link to the Creative Commons licence, and indicate if changes were made. The images or other third party material in this article are included in the article's Creative Commons licence, unless indicated otherwise in a credit line to the material. If material is not included in the article's Creative Commons licence and your intended use is not permitted by statutory regulation or exceeds the permitted use, you will need to obtain permission directly from the copyright holder. To view a copy of this licence, visit http://creativecommons.org/licenses/by/4.0/ . The Creative Commons Public Domain Dedication waiver ( http://creativecommons.org/publicdomain/zero/1.0/ ) applies to the data made available in this article, unless otherwise stated in a credit line to the data.
Reprints and permissions
About this article
Cite this article.
Huang, XY., Shao, Z., Zhong, NN. et al. Comparative analysis of GoPro and digital cameras in head and neck flap harvesting surgery video documentation: an innovative and efficient method for surgical education. BMC Med Educ 24 , 531 (2024). https://doi.org/10.1186/s12909-024-05510-2
Download citation
Received : 18 November 2023
Accepted : 02 May 2024
Published : 14 May 2024
DOI : https://doi.org/10.1186/s12909-024-05510-2
Share this article
Anyone you share the following link with will be able to read this content:
Sorry, a shareable link is not currently available for this article.
Provided by the Springer Nature SharedIt content-sharing initiative
- Head and neck surgery
- Surgery video recording
- Video-based education
- Medical education
BMC Medical Education
ISSN: 1472-6920
- Submission enquiries: [email protected]
- General enquiries: [email protected]

COMMENTS
Textual analysis is a broad term for various research methods used to describe, interpret and understand texts. All kinds of information can be gleaned from a text - from its literal meaning to the subtext, symbolism, assumptions, and values it reveals. The methods used to conduct textual analysis depend on the field and the aims of the ...
Textual analysis is a research methodology that involves exploring written text as empirical data. Scholars explore both the content and structure of texts, and attempt to discern key themes and statistics emergent from them. This method of research is used in various academic disciplines, including cultural studies, literature, bilical studies ...
Thematic analysis, often called Qualitative Content Analysis (QCA) in Europe, is one of the most commonly used methods for analyzing qualitative data (Guest et al. 2012; Kuckartz 2014; Mayring 2014, 2015; Schreier 2012).This chapter presents the basics of this systematic method of qualitative data analysis, highlights its key characteristics, and describes a typical workflow.
Revised on 10 October 2022. Your research methodology discusses and explains the data collection and analysis methods you used in your research. A key part of your thesis, dissertation, or research paper, the methodology chapter explains what you did and how you did it, allowing readers to evaluate the reliability and validity of your research.
Qualitative researchers have developed a wide range of methods of analysis to make sense of textual data, one of the most common forms of data used in qualitative research (Attride-Stirling, 2001; Cho & Trent, 2006; Stenvoll & Svensson, 2011).As a result, qualitative text and discourse analysis (QTDA) has become a thriving methodological space characterized by the diversity of its approaches ...
Textual analysis is a method of reading in which a researcher engages with the potential meanings of a text based on some kind of cultural contextthe culture in which the text was written, the ...
Textual analysis is a way for researchers to gather information about how other human beings make sense of the world. It is a method-ology ± a data-gathering process ± for those researchers who want to understand the ways in which members of various cultures and subcultures make sense of who they are, and of how they ®t into the world in ...
Introduction. Textual analysis is a qualitative method used to examine content in media and popular culture, such as newspaper articles, television shows, websites, games, videos, and advertising. The method is linked closely to cultural studies. Based on semiotic and interpretive approaches, textual analysis is a type of qualitative analysis ...
Textual Analysis - How to Engage in Textual Analysis. As a reader, a developing writer, and an informed student and citizen, you need to be able to locate, understand, and critically analyze others' purposes in communicating information. Being able to identify and articulate the meaning of other writers' arguments and theses enables you ...
Sentiment Analysis, also known as 'opinion mining', is the automated process of understanding the attributes of an opinion, that is, the emotions that underlie a text (e.g. positive, negative, and neutral). Sentiment analysis provides exciting opportunities in all kinds of fields.
Textual analysis is the process of examining a text in order to understand its meaning. It can be used to analyze any type of text, including literature, poetry, speeches, and scientific papers. Textual analysis involves analyzing the structure, content, and style of a text. Textual analysis can be used to understand a text's author, date ...
Thematic analysis is a research method used to identify and interpret patterns or themes in a data set; it often leads to new insights and understanding (Boyatzis, 1998; Elliott, 2018; Thomas, 2006).However, it is critical that researchers avoid letting their own preconceptions interfere with the identification of key themes (Morse & Mitcham, 2002; Patton, 2015).
How to Do Thematic Analysis | Step-by-Step Guide & Examples. Published on September 6, 2019 by Jack Caulfield.Revised on June 22, 2023. Thematic analysis is a method of analyzing qualitative data.It is usually applied to a set of texts, such as an interview or transcripts.The researcher closely examines the data to identify common themes - topics, ideas and patterns of meaning that come up ...
Textual analysis is a broad term that entails various research methods, which allow to analyze, describe, and interpret the characteristics of a recorded message. There are four main approaches typically used in academic writing. Nevertheless, textual analysis is also used in other fields, such as in cultural and media studies, social sciences ...
Chapter 9: Textual Analysis. I. Introduction. Textual analysis is the method communication researchers use to describe and interpret the characteristics of a recorded or visual message. The purpose of textual analysis is to describe the content, structure, and functions of the messages contained in texts. The important considerations in textual ...
In summary, textual analysis has recently been proven to be a valuable tool in finance to measure relevant information from various texts (see, e.g., Antweiler & Frank, 2004; Audi et al., 2016; Hoberg & Phillips, 2010; Kearney & Liu, 2014; Li, 2008; Loughran & McDonald, 2011; Tetlock, 2007; Tetlock et al., 2008).Further, we have discussed the relevance of this approach for quantifying ...
2. TEXTUAL ANALYSIS AS A RESEARCH METHOD 2.1. Textual Analysis: Textual analysis is a term familiar to every researcher related to qualitative research. It is one of the key methods of research methodology being used in cultural studies. It relies on complex aspects like originality,
Published by Alvin Nicolas at August 16th, 2021 , Revised On August 29, 2023. Textual analysis is the method of analysing and understanding the text. It includes the close reading of fictional texts, images symbols, and all forms of written literary texts. A researcher needs to look keenly at the text to identify the writer's context and its ...
9. Textual Analysis as a Research Method was published in Research Methods for English Studies on page 160.
Amid the challenges in a global village, leadership education needs to surpass traditional methods, nurturing creativity, flexibility, and adaptability. This study is a collaborative action inquiry that considers music as an arts-based method in service of leadership development. The study unfolded over five cycles, in which 14 coinquirers collaborated in a process of exploration. The study ...
A growing number of studies have reported that problematic social networking use (PSNU) is strongly associated with anxiety symptoms. However, due to the presence of multiple anxiety subtypes, existing research findings on the extent of this association vary widely, leading to a lack of consensus. The current meta-analysis aimed to summarize studies exploring the relationship between PSNU ...
Additionally, O 2 concentrations measured by manual methods were higher than hourly values in the restored prairie and less in agricultural sites. A variety of spatial variability, pressure perturbations, calibration offsets, and system leakage influences on both analysis methods could cause the discrepancy.
The proposed approaches have been validated on both synthetic and real data, and compared against other methods from the literature. It results that neighborhood analysis allows to outperform competitors, and when it is combined with collaborative filtering the prediction accuracy further improves, scoring a value of AUC equal to 0966.
Background Microbiome dysbiosis has recently been associated with different diseases and disorders. In this context, machine learning (ML) approaches can be useful either to identify new patterns or learn predictive models. However, data to be fed to ML methods can be subject to different sampling, sequencing and preprocessing techniques. Each different choice in the pipeline can lead to a ...
To demix these covariability classes, we develop sliceTCA (slice tensor component analysis), a new unsupervised dimensionality reduction method for neural data tensors.
Background Total pelvic exenteration (TPE), an en bloc resection is an ultraradical operation for malignancies, and refers to the removal of organs inside the pelvis, including female reproductive organs, lower urological organs and involved parts of the digestive system. The aim of this meta-analysis is to estimate the intra-operative mortality, in-hospital mortality, 30- and 90-day mortality ...
A total of six operations were prospectively studied, involving three surgeons, seven circulating nurses, and ten surgical residents. The surgeon wore the GoPro 8 camera attached to a unique headband (Fig. 1), with no additional loupes or head-mounted lighting systems to physically interfere with the camera.An iPad, connected to the GoPro and equipped with the GoPro Quik app, served as a ...