The Church-Turing Thesis Explained: A Deep Dive into the Foundations of Computation
- by history tools
- March 28, 2024
The Church-Turing thesis is a fundamental tenet of computer science that provides the very definition of what it means to be "computable." In essence, it claims that any function that is intuitively "computable" by an effective procedure can be computed by a Turing machine. While this may sound simple, the implications are profound, touching everything from the limits of logical proof to the nature of human cognition.
As a digital technology expert, I find the Church-Turing thesis endlessly fascinating, both for its elegance as an idea and its relevance to the technology we use every day. Far from an archaic piece of mathematical trivia, it remains the beating heart of theoretical computer science nearly a century after its inception. Let‘s dive in and explore the origins, meaning, and implications of this landmark idea in the history of human knowledge.

Origins of Computability Theory in the 1930s
Independently, a young British mathematician named Alan Turing was exploring similar questions. In 1936, Turing published a groundbreaking paper introducing what he called "a-machines," later known as Turing machines.[^3] A Turing machine is an abstract device that can perform any computation that can be done by a human following a finite set of explicit instructions. It consists of:
- An infinite tape divided into cells that can each hold a symbol from a finite alphabet
- A read/write head that can move left or right on the tape and read or write symbols
- A finite set of states the machine can be in, with transition rules that specify how the state and tape contents change based on the current state and symbol being read
Turing showed that any function computable by a Turing machine was also expressible in the lambda calculus, and vice versa. This established the equivalence of the two formalisms and led Church to propose what became known as "Church‘s thesis" or the "Church-Turing thesis":
"A function is effectively calculable if and only if it is computable by a Turing machine or expressible in the lambda calculus."
While Church and Turing could not prove that their formalisms captured all possible notions of computability, the thesis has stood the test of time remarkably well. In the 85 years since it was proposed, no one has found a convincing counterexample of a function that is intuitively computable but not Turing computable. The Church-Turing thesis has become a foundational axiom of computer science.
Computable and Uncomputable Functions
To get a concrete sense of what the Church-Turing thesis means, let‘s look at some examples of computable and uncomputable functions. A classic computable function is primality testing: given a natural number n, determine whether n is prime (i.e. evenly divisible only by 1 and itself). Here‘s a simple Python program that implements a primality test:
This function takes a number n as input, checks if it‘s evenly divisible by any number between 2 and its square root, and returns True if no such divisor is found (meaning n is prime) or False otherwise. It‘s easy to see that this function is computable by a Turing machine: we can specify a finite set of rules for updating the machine‘s state and tape contents to implement the same logic as the Python code. Primality testing is a computable problem.
Turing‘s proof is a clever use of diagonalization and self-reference. Suppose a halting solver Turing machine H existed. We could then construct a machine M that takes a program P as input and does the following:
- Run H on P and P itself as input
- If H says P halts on itself, go into an infinite loop; otherwise, halt immediately
Now, what happens if we run M on itself? If M halts on itself, then H would have determined that M does not halt on itself, in which case the second step would cause M to halt. But if M doesn‘t halt on itself, then H would have determined that M halts on itself, in which case the second step would cause M to go into an infinite loop! This contradiction means our original assumption that H exists must have been false. The halting problem is uncomputable.
This kind of self-referential paradox crops up often in computability theory. It‘s reminiscent of Gödel‘s incompleteness theorems, and in a sense, establishes a fundamental limit on what can be algorithmically decided. Not every well-defined mathematical question has a computable solution.
Variations and Extensions to the Church-Turing Thesis
While the Church-Turing thesis is widely accepted, there have been various philosophical and mathematical challenges to it over the years. Some researchers have proposed notions of "hypercomputation" that go beyond the limits of Turing machines, such as:
- Oracle machines: Turing machines equipped with a black box "oracle" that can magically solve the halting problem or other uncomputable tasks
- Analog computers: Machines that perform computation using continuous physical quantities like voltages or fluid pressures instead of discrete symbols
- Quantum computers: Devices that harness quantum superposition and entanglement to perform computations, potentially offering exponential speedups over classical computers for certain problems
Personally, I find the Church-Turing thesis compelling both as a mathematical foundation and an empirical claim. The fact that nearly a century of research has failed to produce a convincing counterexample suggests that Turing machines really do capture something fundamental about the nature of computation. At the same time, I‘m excited by theoretical and technological developments that probe the boundaries of the computable, and I try to keep an open mind about the potential for new computational models.
The Computational Lens on Mind and Universe
Beyond its central role in computer science, the Church-Turing thesis provides a powerful conceptual framework for viewing the world at large through a computational lens. The notion that any effective procedure can be realized by a Turing machine suggests a kind of universal computability to the cosmos. And if the universe itself is a computer, might the human mind simply be an embodied subprogram?
The strong form of this view is that human cognition is Turing-computable – that everything from perception to reasoning to consciousness can in principle be implemented by a sufficiently advanced AI system. If this is true, then the Church-Turing thesis places ultimate limits on the nature of intelligence. No matter how sophisticated our technology becomes, the space of possible minds will be constrained by the space of Turing-computable functions.
As a computer scientist, I lean towards a computational view of mind, but I also recognize the difficulty of reducing something as complex and subjective as human experience to the cut-and-dried formalisms of Turing machines. While I believe artificial general intelligence is possible in principle, I suspect the Church-Turing thesis alone is too crude a tool to fully delineate the space of possible minds. We likely need a richer theory of computation that can account for context, embodiment, and interaction with the environment.
This connects to perhaps the grandest application of the Church-Turing lens: viewing the physical universe itself as a computation. Digital physics, as championed by thinkers like Konrad Zuse, Edward Fredkin, and Stephen Wolfram, models the cosmos as a giant (quantum) computer, with the physics constrained by the Church-Turing thesis.[^12] In this view, spacetime is the hardware, particles are the software, and the speed of light is the clock rate.
While a compelling metaphor, digital physics remains highly speculative. We have no empirical evidence that the universe is discretized at the Planck scale or that physical dynamics are bounded by Turing computability. In fact, some have argued that a discrete, computable universe would violate locality and Lorentz invariance.[^13] For now, digital physics is more of a philosophical stance than a scientific theory.
The Church-Turing thesis is a profound and enduring idea that has shaped the foundations of computer science and our philosophical understanding of the nature of mind and cosmos. By precisely defining what it means for a function to be "computable," Church and Turing gave us a powerful mathematical framework for reasoning about the limits of algorithmic problem-solving.
While the thesis remains unproven in a formal sense, its remarkable resilience over nearly a century attests to its conceptual power. No one has yet found a convincing example of an intuitively computable function that is not Turing computable. The Church-Turing thesis has become a bedrock assumption of modern computability theory.
At the same time, the thesis raises deep questions about the nature of computation in the physical universe and human minds. Are there forms of hypercomputation that transcend the Church-Turing limit? Is the brain itself bounded by Turing computability? Might the universe be a vast digital computer constrained by the laws of Church and Turing? These are heady philosophical questions that have inspired much debate and speculation.
As our digital technologies continue to advance at a dizzying pace, it‘s worth reflecting on the Church-Turing foundations that make it all possible. The smartphones in our pockets and the supercomputers in the cloud are all in a sense instantiations of Turing‘s original vision – an astoundingly general model of mechanical computation. Every time you run a program, send an email, or do a web search, you‘re implicitly relying on the Church-Turing thesis. That is the mark of a truly deep idea.
Moving forward, I believe the Church-Turing thesis will remain a vital touchstone for anyone seeking to understand the nature of computation – in silicon, in carbon, and in the cosmos. While it may not be the final word on computability, it is a crucial piece of the puzzle, and one that will continue to inspire and inform our thinking about the algorithmic universe we inhabit. As a digital technology expert, I find that an endlessly exciting prospect.
Related posts:
- Hello Reader, Here is the Complete Guide to Understanding the Turing Test
- The Origins and Evolution of STEM Education: An Exploration of Its History, Significance and Future
- Unlocking the Power of Z-Scores: An Essential Guide for the Tech-Savvy Data Analyst
- Precision vs Recall: Understanding the Key Differences
- The Complete Guide to Cybernetics
- A Complete History of Code-Breaking and Cryptanalysis
- Converting Between Celsius and Fahrenheit: A Digital Technology Perspective
- Hashing 101: A Comprehensive Guide to Understanding Hash Functions
The Church-Turing Thesis: Logical Limit or Breachable Barrier?
- Hacker News
- Download PDF
- Join the Discussion
- View in the ACM Digital Library
- Introduction
Key Insights
History of the thesis, what is an algorithm, what justifies the church-turing thesis, is the thesis mathematically provable, complexity: the extended church-turing thesis, physical computability, ctt-p and quantum mechanics, weaker physical computability theses, physical computation thesis, relativistic computation, ctt-a and computation in the broad.
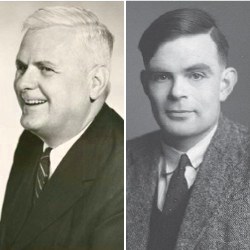
The Church-Turing thesis (CTT) underlies tantalizing open questions concerning the fundamental place of computing in the physical universe. For example, is every physical system computable? Is the universe essentially computational in nature? What are the implications for computer science of recent speculation about physical uncomputability? Does CTT place a fundamental logical limit on what can be computed, a computational “barrier” that cannot be broken, no matter how far and in what multitude of ways computers develop? Or could new types of hardware, based perhaps on quantum or relativistic phenomena, lead to radically new computing paradigms that do breach the Church-Turing barrier, in which the uncomputable becomes computable, in an upgraded sense of “computable”? Before addressing these questions, we first look back to the 1930s to consider how Alonzo Church and Alan Turing formulated, and sought to justify, their versions of CTT. With this necessary history under our belts, we then turn to today’s dramatically more powerful versions of CTT.
Back to Top
- The term “Church-Turing thesis” is used today for numerous theses that diverge significantly from the one Alonzo Church and Alan Turing conceived in 1936,
- The range of algorithmic processes studied in modern computer science far transcends the range of processes a “human cornputer” could possibly carry out.
- There are at least three forms of the “physical Church-Turing thesis”— modest, bold, and super-bold—though, at the present stage of physical inquiry, it is unknown whether any of them is true.
Turing stated what we will call “Turing’s thesis” in various places and with varying degrees of rigor. The following formulation is one of his most accessible.
Turing’s thesis. “L.C.M.s [logical computing machines, Turing’s expression for Turing machines] can do anything that could be described as … ‘purely mechanical’.” 38
Turing also formulated his thesis in terms of numbers. For example, he said, “It is my contention that these operations [the operations of an L.C.M.] include all those which are used in the computation of a number.” 36 and “[T]he ‘computable numbers’ include all numbers which would naturally be regarded as computable.” 36
Church (who, like Turing, was working on the German mathematician David Hubert’s Entscheidungsproblem ) advanced “Church’s thesis,” which he expressed in terms of definability in his lambda calculus.
Church’s thesis. “We now define the notion … of an effectively calculable function of positive integers by identifying it with the notion of a recursive function of positive integers (or of a λ-definable function of positive integers).” 5

Church chose to call this a definition. American mathematician Emil Post, on the other hand, referred to Church’s thesis as a “working hypothesis” and criticized Church for masking it in the guise of a definition. 33
Upon learning of Church’s “definition,” Turing quickly proved that λ-definability and his own concept of computability (over positive integers) are equivalent. Church’s thesis and Turing’s thesis are thus equivalent, if attention is restricted to functions of positive integers. (Turing’s thesis, more general than Church’s, also encompassed computable real numbers.) However, it is important for a computer scientist to appreciate that despite this extensional equivalence, Turing’s thesis and Church’s thesis have distinct meanings and so are different theses, since they are not intensionally equivalent. A leading difference in their meanings is that Church’s thesis contains no reference to computing machinery, whereas Turing’s thesis is expressed in terms of the “Turing machine,” as Church dubbed it in his 1937 review of Turing’s paper.
It is now widely understood that Turing introduced his machines with the intention of providing an idealized description of a certain human activity—numerical computation; in Turing’s day computation was carried out by rote workers called “computers,” or, sometimes, “computors”; see, for example, Turing. 37 The Church-Turing thesis is about computation as the term was used in 1936—human computation. Church’s term “effectively calculable function” was intended to refer to functions that are calculable by an idealized human computer; and, likewise, Turing’s phrase “numbers which would naturally be regarded as computable” was intended to refer to those numbers that could be churned out, digit by digit, by an idealized human computer working ceaselessly.
Here, then, is our formulation of the historical version of the Church-Turing thesis, as informed by Turing’s proof of the equivalence of his and Church’s theses:
CTT-Original (CTT-O). Every function that can be computed by the idealized human computer, which is to say, can be effectively computed, is Turing-computable.
Some mathematical logicians view CTT-O as subject ultimately to either mathematical proof or mathematical refutation, like open mathematical conjectures, as in the Riemann hypothesis, while others regard CTT-O as not amenable to mathematical proof but supported by philosophical arguments and an accumulation of mathematical evidence. Few logicians today follow Church in regarding CTT-O as a definition. We subscribe to Turing’s view of the status of CTT-O, as we outline later.
In computer science today, algorithms and effective procedures are, of course, associated not primarily with humans but with machines. (Note, while some expositors might distinguish between the terms “algorithm” and “effective procedure,” we use the terms interchangeably.) Many computer science textbooks formulate the Church-Turing thesis without mentioning human computers at all; examples include the well-known books by Hopcroft and Ullman 24 and Lewis and Papadimitriou. 29 This is despite the fact that the concept of human computation was at the heart of both Turing’s and Church’s analysis of computation.
We discuss several important modern forms of the Church-Turing thesis, each going far beyond CTT-O. First, we look more closely at the algorithmic form of thesis, as stated to a first approximation by Lewis and Papadimitriou 29 : “[W]e take the Turing machine to be a precise formal equivalent of the intuitive notion of ‘algorithm’.”
The range of algorithmic processes studied in modern computer science far transcends the range of processes a Turing machine is able to carry out. The Turing machine is restricted to, say, changing at most one bounded part at each sequential step of a computation. As Yuri Gurevich pointed out, the concept of an algorithm keeps evolving: “We have now parallel, interactive, distributed, real-time, analog, hybrid, quantum, etc. algorithms.” 22 There are enzymatic algorithms, bacterial foraging algorithms, slime-mold algorithms, and more. The Turing machine is incapable of performing the atomic steps of algorithms carried out by, say, an enzymatic system (such as selective enzyme binding) or a slime mold (such as pseudopod extension). The Turing machine is similarly unable to duplicate (as opposed to simulate) John Conway’s Game of Life, where—unlike a Turing machine—every cell updates simultaneously.
A thesis aiming to limit the scope of algorithmic computability to Turing computability should thus not state that every possible algorithmic process can be performed by a Turing machine. The way to express the thesis is to say the extensional input-output function ια associated with an algorithm α is always Turing-computable; ια is simply the extensional mapping of α ‘s inputs to α ‘s outputs. The algorithm the Turing machine uses to compute ια might be very different from α itself. A question then naturally arises: If an algorithmic process need not be one a Turing machine can carry out, save in the weak sense just mentioned, then where do the boundaries of this concept lie? What indeed is an algorithm?
The dominant view in computer science is that, ontologically speaking, algorithms are abstract entities; however, there is debate about what abstract entities algorithms are. Gurevich defined the concept in terms of abstract-state machines, Yiannis Moschovakis in terms of abstract recursion, and Noson Yanofsky in terms of equivalence classes of programs, while Moshe Vardi has speculated that an algorithm is both abstract-state machine and recursor. It is also debated whether an algorithm must be physically implementable. Moschovakis and Vasilis Paschalis (among others) adopt a concept of algorithm “so wide as to admit ‘non-implementable’ algorithms,” 30 while other approaches do impose a requirement of physical implementability, even if only a very mild one. David Harel, for instance, writes: [A]ny algorithmic problem for which we can find an algorithm that can be programmed in some programming language, any language, running on some computer, any computer, even one that has not been built yet but can be built … is also solvable by a Turing machine. This statement is one version of the so-called Church/Turing thesis.” 23
Steering between these debates—and following Harel’s suggestion that the algorithms of interest to computer science are always expressible in programming languages—we arrive at the following program-oriented formulation of the algorithmic thesis:
CTT-Algorithm (CTT-A). Every algorithm can be expressed by means of a program in some (not necessarily currently existing) Turing-equivalent programming language.
There is an option to narrow CTT-A by adding “physically implementable” before “program,” although in our view this would be to lump together two distinct computational issues that are better treated separately.
The evolving nature and open-endedness of the concept of an algorithm is matched by a corresponding open-endedness in the concept of a programming language. But this open-endedness notwithstanding, CTT-A requires that all algorithms be bounded by Turing computability.
Later in this article we examine complexity-theoretic and physical versions of the Church-Turing thesis but first turn to the question of the justification of the theses introduced so far. Are CTT-O and CTT-A correct?
Stephen Kleene—who coined the term “Church-Turing thesis”—catalogued four types of argument for CTT-O: First, the argument from non-refutation points out the thesis has never been refuted, despite sustained (and ongoing) attempts to find a counterexample (such as the attempts by László Kalmár and, more recently, by Doukas Kapantais). Second, the argument from confluence points to the fact that the various characterizations of computability, while differing in their approaches and formal details, turn out to encompass the very same class of computable functions. Four such characterizations were presented (independently) in 1936 and immediately proved to be extensionally equivalent: Turing computability, Church’s λ-definability, Kleene’s recursive functions, and Post’s finitary combinatory processes.
Third is an argument usually referred to nowadays as “Turing’s analysis.” Turing called it simply argument “I,” stating five very general and intuitive constraints—or axioms—the human computer may be assumed to satisfy: “The behavior of the computer at any moment is determined by the symbols which he is observing, and his ‘state of mind’ at that moment”; “[ T ] here is a bound B to the number of symbols or squares which the computer can observe at one moment”; “[E]ach of the new observed squares is within L squares of an immediately previously observed square”; “[I]n a simple operation not more than one symbol is altered”; and “[T]he number of states of mind which need be taken into account is finite.” Turing noted that reference to the computer’s states of mind can be avoided by talking instead about configurations of symbols, these being “a more definite and physical counterpart” of states of mind. 36
The second part of Turing’s argument I is a demonstration that each function computed by any human computer subject to these constraints is also computable by a Turing machine; it is not difficult to see that each of the computer’s steps can be mimicked by the Turing machine, either in a single step or by means of a series of steps. In short, Turing’s five axioms entail CTT-O. (Turing’s axiomatic approach to computability was in fact foreshadowed by Kurt Gödel in a suggestion to Church a year or so earlier. 15 Some more recent axiomatic approaches to computability proceed differently; for example, Erwin Engeler employs the Schönfinkel-Curry idea of “combinators” in order to axiomatize the concept of an algorithmic function.)
The Turing machine is restricted to, say, changing at most one bounded part at each sequential step of a computation.
Fourth in this catalog of considerations supporting CTT-O are arguments from first-order logic. They are typified by a 1936 argument of Church’s and by Turing’s argument II, from Section 9 of Turing’s 1936 paper. In 2013, Saul Kripke 28 presented a reconstruction of Turing’s argument II, which goes as follows: Computation is a special form of mathematical deduction; and every mathematical deduction—and therefore every computation—can be formalized as a valid deduction in the language of first-order predicate logic with identity (a step Kripke referred to as “Hilbert’s thesis”); following Gödel’s completeness theorem, each computation is thus formalized by a provable formula of first-order logic; and every computation can therefore be carried out by the universal Turing machine. This last step regarding the universal Turing machine is secured by a theorem proved by Turing: Every provable formula of first-order logic can be proved by the universal Turing machine.
The third and fourth of these arguments provide justification for CTT-O but not for CTT-A. As Robin Gandy 20 pointed out, the third argument—Turing’s I—contains “crucial steps … where he [Turing] appeals to the fact that the calculation is being carried out by a human being.” 20 For example, Turing assumed “a human being can only write one symbol at a time,” and Gandy noted this assumption cannot be carried over to a parallel machine that “prints an arbitrary number of symbols simultaneously.” 20 In Conway’s Game of Life, for instance, there is no upper bound on the number of cells that make up the grid, yet the symbols in all the cells are updated simultaneously. Likewise, the fourth argument (Turing’s II) involves the claim that computation is a special form of formal proof, but the notion of proof is intrinsically related to what a human mathematician—and not some oracle—can prove.
It is thus perhaps not too surprising that the third and fourth arguments in this catalog seldom if ever appear in logic and computer science textbooks. The two arguments that are always given for the Church-Turing thesis (in, for example, Lewis and Papadimitriou 29 ) are confluence and non-refutation. Yet both those arguments are merely inductive, whereas the third and fourth arguments are deductive in nature.
However, a number of attempts have sought to extend Turing’s axiomatic analysis to machine computation; for example, Gandy 20 broadened Turing’s analysis in such a way that parallel computation is included, while Dershowitz and Gurevich 16 gave a more general analysis in terms of abstract state machines. We return to the topic of extending the analysis to machine computation later in this article but first address the important question of whether CTT-O is mathematically provable.
It used to be thought by mathematical logicians and others that CTT-O is not amenable to formal proof, since it is not a mathematically precise statement. This is because it pairs an informal concept—a “vague intuitive notion,” Church called it 5 —with a precise concept. However, Elliott Mendelson gave a powerful critique of this general argument; and today the view that CTT-O is formally provable seems to be gaining acceptance; see, for example, Dershowitz and Gurevich. 16 Inspired by Gandy, 20 Wilfried Sieg 35 stated that a tightened form of Turing’s argument I proves the thesis; and Kripke 28 entertained the same claim for Turing’s argument II.
Turing’s own view was that, on the contrary, his thesis is not susceptible to mathematical proof. He thought his arguments I and II, and indeed “[a]ll arguments which can be given” for the thesis, are “fundamentally, appeals to intuition, and for this reason rather unsatisfactory mathematically.” 36 Hilbert’s thesis is another example of a proposition that can be justified only by appeal to intuition, and so Kripke’s 28 tightened form of argument II, far from proving CTT-O, merely deduced it from another thesis that is also not amenable to mathematical proof.
Much the same can be said about argument I. If axioms 1–5 are formulated in precise mathematical terms, then it is certainly provable from them that computation is bounded by Turing computability; this is probably what Gandy 20 meant when he said Turing’s argument I proves a “theorem.” But the real issue is whether these axioms completely capture the concept of a computational or algorithmic process, and, so far as we see, no one has ever given a rigorous mathematical justification of that claim. The axioms may be supported by informal arguments, but the whole edifice then falls short of mathematical proof. This is most apparent when the informal arguments offered for the axioms invoke limitations in the cognitive capacities of human computers, as we point out elsewhere. 13 A justification of the second axiom may, for instance, refer to the limitations of human observation. The axioms most certainly lie beyond the scope of mathematical demonstration if their truth depends on contingent human limitations. Turing himself cheerfully appealed to cognitive limitations in the course of his analysis, saying, for example, “[j]ustification lies in the fact that the human memory is necessarily limited.” 36
Turing’s own view was that, on the contrary, his thesis is not susceptible to mathematical proof.
In summary, our answer to “Is CTT-O mathematically provable?” is: Turing thought not and we have found no reason to disagree with him. The various historical arguments seem more than sufficient to establish CTT-O, but these arguments do indeed fall short of mathematical proof.
We next address complexity theoretic forms of the Church-Turing thesis, then turn to the question of whether CTT-A is justified in the context of physically realistic computations.
It is striking that the Turing machine holds a central place not only in computability theory but also in complexity theory, where it is viewed as a universal model for complexity classes.
In complexity theory, the time complexities of any two general and reasonable models of computation are assumed to be polynomially related. But what counts as “reasonable”? Aharonov and Vazirani 1 glossover “reasonable” as “physically realizable in principle”; see also Bernstein and Vazirani. 3 If a computational problem’s time complexity is t in some (general and reasonable) model, then its time complexity is assumed to be poly( t ) in the single-tape Turing machine model; see also Goldreich. 21 This assumption has different names in the literature; Goldreich 21 called it the Cobham-Edmonds thesis, while Yao 40 introduced the term “Extended Church-Turing thesis.” The thesis is of interest only if P ≠ NP , since otherwise it is trivial.
Quantum-computation researchers also use a variant of this thesis, as expressed in terms of probabilistic Turing machines. Bernstein and Vazirani 3 said: “[C]omputational complexity theory rests upon a modern strengthening of [the Church-Turing] thesis, which asserts that any ‘reasonable’ model of computation can be efficiently simulated on a probabilistic Turing machine.” 3
Aharonov and Vazirani 1 give the following formulation of this assumption, naming it the “Extended Church-Turing thesis”—though it is not quite the same as Yao’s earlier thesis of the same name, which did not refer to probabilistic Turing machines:
CTT-Extended (CTT-E). “[A]ny reasonable computational model can be simulated efficiently by the standard model of classical computation, namely, a probabilistic Turing machine.” 1
As is well known in computer science, Peter Shor’s quantum algorithm for prime factorization is a potential counterexample to CTT-E; the algorithm runs on a quantum computer in polynomial time and is much faster than the most-efficient known “classical” algorithm for the task. But the counterexample is controversial. Some computer scientists think the quantum computer invoked is not a physically reasonable model of computation, while others think accommodating these results might require further modifications to complexity theory.
We turn now to extensions of the Church-Turing thesis into physics.
The issue of whether every aspect of the physical world is Turing-computable was broached by several authors in the 1960s and 1970s, and the topic rose to prominence in the mid-1980s.
In 1985, Stephen Wolfram formulated a thesis he described as “a physical form of the Church-Turing hypothesis,” saying, “[U]niversal computers are as powerful in their computational capacities as any physically realizable system can be, so that they can simulate any physical system.” 39 In the same year, David Deutsch, who laid the foundations of quantum computation, independently stated a similar thesis, describing it as “the physical version of the Church-Turing principle.” 17 The thesis is now known as the Church-Turing-Deutsch thesis and the Church-Turing-Deutsch-Wolfram thesis.
Church-Turing-Deutsch-Wolfram thesis (CTDW). Every finite physical system can be simulated to any specified degree of accuracy by a universal Turing machine.
Deutsch pointed out that if “simulated” is understood as “perfectly simulated,” then the thesis is falsified by continuous classical systems, since such classical systems necessarily involve uncomputable real numbers, and went on to introduce the concept of a universal quantum computer, saying such a computer is “capable of perfectly simulating every finite, realizable physical system.” Other physical formulations were advanced by Lenore Blum et al., John Earman, Itamar Pitowsky, Marian Pour-El, and Ian Richards, among others.
We next formulate a strong version of the physical Church-Turing thesis we call the “total physical computability thesis.” (We consider some weaker versions later in the article.) By “physical system” we mean any system whose behavior is in accordance with the actual laws of physics, including non-actual and idealized systems.
Total physical computability thesis (CTT-P). Every physical aspect of the behavior of any physical system can be calculated (to any specified degree of accuracy) by a universal Turing machine.
As with CTT-E, there is also a probabilistic version of CTT-P, formulated in terms of a probabilistic Turing machine.
Arguably, the phrase “physical version of the Church-Turing thesis” is an inappropriate name for this and related theses, since CTT-O concerns a form of effective or algorithmic activity and asserts the activity is always bounded by Turing computability, while CTT-P and CTDW, on the other hand, entail that the activity of every physical system is bounded by Turing computability; the system’s activity need not be algorithmic/effective at all. Nevertheless, in our “CTT-” nomenclature, we follow the Deutsch-Wolfram tradition throughout this article.
Is CTT-P true? Not if physical systems include systems capable of producing unboundedly many digits of a random binary sequence; Church showed such sequences are uncomputable, as we discussed elsewhere. 8 Moreover, speculation that there may be deterministic physical processes whose behavior cannot be calculated by the universal Turing machine stretches back over several decades; for a review, see Copeland. 9 In 1981, Pour-El and Richards 34 showed that a system evolving from computable initial conditions in accordance with the familiar three-dimensional wave equation is capable of exhibiting behavior that falsifies CTT-P; even today, however, it is an open question whether these initial conditions are physically possible. Earlier papers, from the 1960s, by Bruno Scarpellini, Arthur Komar, and Georg Kreisel, in effect questioned CTT-P, with Kreisel stating: “There is no evidence that even present-day quantum theory is a mechanistic, i.e., recursive theory in the sense that a recursively described system has recursive behavior.” 27 Other potential counterexamples to CTT-P have been described by a number of authors, including what are called “relativistic” machines. First introduced by Pitowsky, 32 they will be examined in the section called “Relativistic Computation.”
There are a number of theoretical countermodels to CTT-P arising from quantum mechanics. For example, in 1964, Komar 26 raised “the issue of the macroscopic distinguishability of quantum states,” asserting there is no effective procedure “for determining whether two arbitrarily given physical states can be superposed to show interference effects.” In 2012, Eisert et al. 19 showed “[T]he very natural physical problem of determining whether certain outcome sequences cannot occur in repeated quantum measurements is undecidable, even though the same problem for classical measurements is readily decidable.” This is an example of a problem that refers unboundedly to the future but not to any specific time. Other typical physical problems take the same form; Pitowsky gave as examples “Is the solar system stable?” and “Is the motion of a given system, in a known initial state, periodic?”
Cubitt et al. 14 described another such undecidability result in a 2015 Nature article, outlining their proof that “[T]he spectral gap problem is algorithmically undecidable: There cannot exist any algorithm that, given a description of the local interactions, determines whether the resultant model is gapped or gapless.” Cubitt et al. also said this is the “first undecidability result for a major physics problem that people would really try to solve.”
The spectral gap, an important determinant of a material’s properties, refers to the energy spectrum immediately above the ground-energy level of a quantum many-body system, assuming a well-defined least-energy level of the system exists; the system is said to be “gapless” if this spectrum is continuous and “gapped” if there is a well-defined next-least energy level. The spectral gap problem for a quantum many-body system is the problem of determining whether the system is gapped or gapless, given the finite matrices (at most three) describing the local interactions of the system.
In their proof, Cubitt et al. 14 encoded the halting problem in the spectral gap problem, showing the latter is at least as hard as the former. The proof involves an infinite family of two-dimensional lattices of atoms. But they pointed out their result also applies to finite systems whose size increases, saying, “Not only can the lattice size at which the system switches from gapless to gapped be arbitrarily large, the threshold at which this transition occurs is uncomputable.” Their proof offers an interesting countermodel to CTT-P, involving a physically relevant example of a finite system of increasing size. There exists no effective method for extrapolating the system’s future behavior from (complete descriptions of) its current and past states.
It is debatable whether any of these quantum models correspond to real-world quantum systems. Cubitt et al. 14 admitted the model invoked in their proof is highly artificial, saying, “Whether the results can be extended to more natural models is yet to be determined.” There is also the question of whether the spectral gap problem becomes computable when only local Hilbert spaces of realistically low dimensionality are considered. Nevertheless, these results are certainly suggestive: CTT-P cannot be taken for granted, even in a finite quantum universe.
Summarizing the current situation with respect to CTT-P, we can say, although theoretical countermodels in which CTT-P is false have been described, there is at present—so far as we know—not a shred of evidence that CTT-P is false in the actual universe. Yet it would seem most premature to assert that CTT-P is true.
Piccinini 31 has distinguished between two different types of physical versions of the Church-Turing thesis, both commonly found in the literature, describing them as “bold” and “modest” versions of the thesis, respectively. The bold and modest versions are weaker than our “super-bold” version just discussed (CTT-P). Bold versions of the thesis state, roughly, that “Any physical process can be simulated by some Turing machine.” 31 The Church-Turing-Deutsch-Wolfram thesis (CTDW) is an example, though Piccinini emphasized that the bold versions proposed by different researchers are often “logically independent of one another” and that, unlike the different formulations of CTT-O, which exhibit confluence, the different bold formulations in fact exhibit “lack of confluence.” 31
CTDW and other bold forms are too weak to rule out the uncomputability scenarios described by Cubitt et al. 14 and by Eisert et al. 19 This is because the physical processes involved in these scenarios may, so far as we know, be Turing-computable; it is possible that each process can be simulated by a Turing machine, to any required degree of accuracy, and yet the answers to certain physical questions about the processes are, in general, uncomputable. The situation is similar in the case of the universal Turing machine itself. The machine’s behavior (consisting of the physical actions of the read/write head) is always Turing-computable since it is produced by the Turing machine’s program, yet the answers to some questions about the behavior (such as whether or not the machine halts given certain inputs) are not computable.

Nevertheless, bold forms (such as CTDW) are interesting empirical hypotheses in their own right and the world might confute them. For instance, CTDW fails in the wave-equation countermodel due to Pour-El and Richards 34 where the mapping between the wave equation’s “inputs” and “outputs” is not a Turing-computable (real) function; although, as noted earlier, the physicality of this countermodel can readily be challenged. We discuss some other potential countermodels later in the article, but turn first to what Piccinini termed “modest” versions of the thesis.
Modest versions maintain in essence that every physical computing process is Turing-computable; for two detailed formulations, see Gandy 20 and Copeland. 8 Even if CTT-P and CTDW are in general false, the behavior of the subset of physical systems that are appropriately described as computing systems may nevertheless be bounded by Turing-computability. An illustration of the difference between modest versions on the one hand and CTT-P and CTDW on the other is given by the fact that the wave-equation example is not a counter-model to the modest thesis, assuming, as seems reasonable, that the physical dynamics described by the equation do not constitute a computing process.
Here, we formulate a modest version of the physical Church-Turing thesis we call the “Physical Computation” thesis, then turn to the question of whether it is true.
This form of the thesis maintains that physical computation is bounded by Turing-computability.
Physical computation thesis (CTT-P-C). Every function computed by any physical computing system is Turing-computable.
Is CTT-P-C true? As with the stronger physical computability theses, it seems too early to say. CTT-P-C could be false only if CTT-P and CTDW turn out to be false, since each of them entails CTT-P-C (see the figure here, which outlines the relationships among CTT-P, CTDW, and CTT-P-C). If all physical computation is effective in the 1930s sense of Turing and Church, then CTT-P-C is certainly true. If, however, the door is open to a broadened sense of computation, where physical computation is not necessarily effective in the sense of being bounded by Turing-computability, then CTT-P-C makes a substantive claim.
There is, in fact, heated debate among computer scientists and philosophers about what counts as physical computation. Moreover, a number of attempts have sought to describe a broadened sense of computation in which computation is not bounded by Turing-computability; see, for example, Copeland. 6 Computing machines that compute “beyond the Turing limit” are known collectively as “hypercomputers,” a term introduced in Copeland and Proudfoot. 11 Some of the most thought-provoking examples of notional machines that compute in the broad sense are called “supertask” machines. These “Zeno computers” squeeze infinitely many computational steps into a finite span of time. Examples include accelerating machines, 7 , 12 shrinking machines, and the intriguing relativistic computers described in the next section.
Notional machines all constitute rather theoretical countermodels to CTT-P-C, so long as it is agreed that they compute in a broadened sense, but none has been shown to be physically realistic, although, as we explain, relativistic computers come close. In short, the truth or falsity of CTT-P-C remains unsettled.
Relativistic machines operate in space-time structures with the property that the entire endless lifetime of one component of the machine is included in the finite chronological past of another component, called “the observer.” The first component could thus carry out an infinite computation (such as calculating every digit of π) in what is, from the observer’s point of view, a finite timespan of, say, one hour. (Such machines are in accord with Einstein’s general theory of relativity, hence the term “relativistic.”) Examples of relativistic computation have been detailed by Pitowsky, Mark Hogarth, and Istvan Németi.
In this section we outline a relativistic machine RM consisting of a pair of communicating Turing machines, T E and T o , in relative motion. T E is a universal machine, and T o is the observer. RM is able to compute the halting function, in a broad sense of computation. Speaking of computation here seems appropriate, since RM consists of nothing but two communicating Turing machines.
Here is how RM works. When the input ( m,n ), asking whether the m th Turing machine (in some enumeration of the Turing machines) halts or not when started on input n , enters T o , T o first prints 0 (meaning “never halts”) in its designated output cell and then transmits ( m,n ) to T E . T E simulates the computation performed by the m th Turing machine when started on input n and sends a signal back to T o if and only if the simulation terminates. If T o receives a signal from T E , T o deletes the 0 it previously wrote in its output cell and writes 1 in its place (meaning “halts”). After one hour, T o ‘s output cell shows 1 if the m th Turing machine halts on input n and shows 0 if the m th machine does not halt on n.
The most physically realistic version of this setup to date is due to Németi and his collaborators in Budapest. T E , an ordinary computer, remains on Earth, while the observer T o travels toward and enters a slowly rotating Kerr black hole. T o approaches the outer event horizon, a bubble-like hypersurface surrounding the black hole. Németi theorized that the closer T o gets to the event horizon, the faster T E ‘s clock runs relative to T o due to Einsteinian gravitational time dilation, and this speeding up continues with no upper limit. T o motion proceeds until, relative to a time t on T o clock, the entire span of T E ‘s computing is over. If any signal was emitted by T E , the signal will have been received by T o before time t. So T o will fall into the black hole with 1 in its output cell if T E halted and 0 if T E never halted. Fortunately, T o can escape annihilation if its trajectory is carefully chosen in advance, says Németi; the rotational forces of the Kerr hole counterbalance the gravitational forces that would otherwise “spaghettify” T o . T o thus emerges unscathed from the hole and goes on to use the computed value of the halting function in further computations.
Németi and colleagues emphasize their machine is physical in the sense it is “not in conflict with presently accepted scientific principles” and, in particular, “the principles of quantum mechanics are not violated.” 2 They suggest humans might “even build” a relativistic computer “sometime in the future.” 2 This is, of course, highly controversial. However, our point is that Németi’s theoretical countermodel, which counters not only CTT-P-C but also CTT-P and CTDW, helps underscore that the “physical version of the Church-Turing thesis” is quite independent of CTT-O, since the countermodel stands whether or not CTT-O is endorsed. We next reconsider CTT-A.
The continuing expansion of the concept of an algorithm is akin to the extension of the concept of number from integers to signed integers to rational, real, and complex numbers. Even the concept of human computation underwent an expansion; before 1936, computation was conceived of in terms of total functions, and it was Kleene in 1938 who explicitly extended the conception to also cover partial functions.
Gurevich argued in 2012 that formal methods cannot capture the algorithm concept in its full generality due to the concept’s open-ended nature; at best, formal methods provide treatments of “strata of algorithms” that “have matured enough to support rigorous definitions.” 22 An important question for computer science is whether CTT-A is a reasonable constraint on the growth of new strata. Perhaps not. In 1982, Jon Doyle 18 suggested equilibrating systems with discrete spectra (such as molecules and other quantum many-body systems) illustrate a concept of effectiveness that is broader than the classical concept, saying, “[E]quilibrating can be so easily, reproducibly, and mindlessly accomplished” that we may “take the operation of equilibrating as an effective one,” even if “the functions computable in principle given Turing’s operations and equilibrating include non-recursive functions.”
Over the years, there have been several departures from Turing’s 1936 analysis, as the needs of computer science led to a broadening of the algorithm concept. For example, Turing’s fourth axiom, which bounds the number of parts of a system that can be changed simultaneously, became irrelevant when the algorithm concept broadened to cover parallel computations. The future computational landscape might conceivably include more extensive revisions of the concept, if, for example, physicists were to discover that hardware effective in Doyle’s extended sense is a realistic possibility.
If such hardware were to be developed—hardware in which operations are effective in the sense of being “easily, reproducibly, and mindlessly accomplished” but not bounded by Turing computability—then would the appropriate response by computer scientists be to free the algorithm concept from CTT-A? Or should CTT-A remain as a constraint on algorithms, with instead two different species of computation being recognized, called, say, algorithmic computation and non-algorithmic computation? Not much rides on a word, but we note we prefer “effective computation” for computation that is bounded by Turing computability and “neo-effective computation” for computation that is effective in Doyle’s sense and not bounded by Turing computability, with “neo” indicating a new concept related to an older one.
The numerous examples of notional “hypercomputers” (see Copeland 9 for a review) prompt similar questions. Interestingly, a study of the expanding literature about the concept of an infinite-time Turing machine, introduced by Joel Hamkins and Andy Lewis in 2000, shows that a number of computer scientists are prepared to describe the infinite-time machine as computing the halting function. Perhaps this indicates the concept of computation is already in the process of bifurcating into “effective” and “neo-effective” computation.
In the computational literature the term “Church-Turing thesis” is applied to a variety of different propositions usually not equivalent to the original the-sis—CTT-O; some even go far beyond anything either Church or Turing wrote. Several but not all are fundamental assumptions of computer science. Others (such as the various physical computability theses we have discussed) are important in the philosophy of computing and the philosophy of physics but are highly contentious; indeed, the label “Church-Turing thesis” should not mislead computer scientists or anyone else into thinking they are established fact or even that Church or Turing endorsed them.
Submit an Article to CACM
CACM welcomes unsolicited submissions on topics of relevance and value to the computing community.
You Just Read
Copyright held by the authors. Publication rights licensed to ACM. Request permission to publish from [email protected]
The Digital Library is published by the Association for Computing Machinery. Copyright © 2019 ACM, Inc.
January 2019 Issue
Published: January 1, 2019
Vol. 62 No. 1
Pages: 66-74
Advertisement
Join the Discussion (0)
Become a member or sign in to post a comment, the latest from cacm.
When Colorless Green DNNs Sleep Furiously in an Unexplainable Fantasy
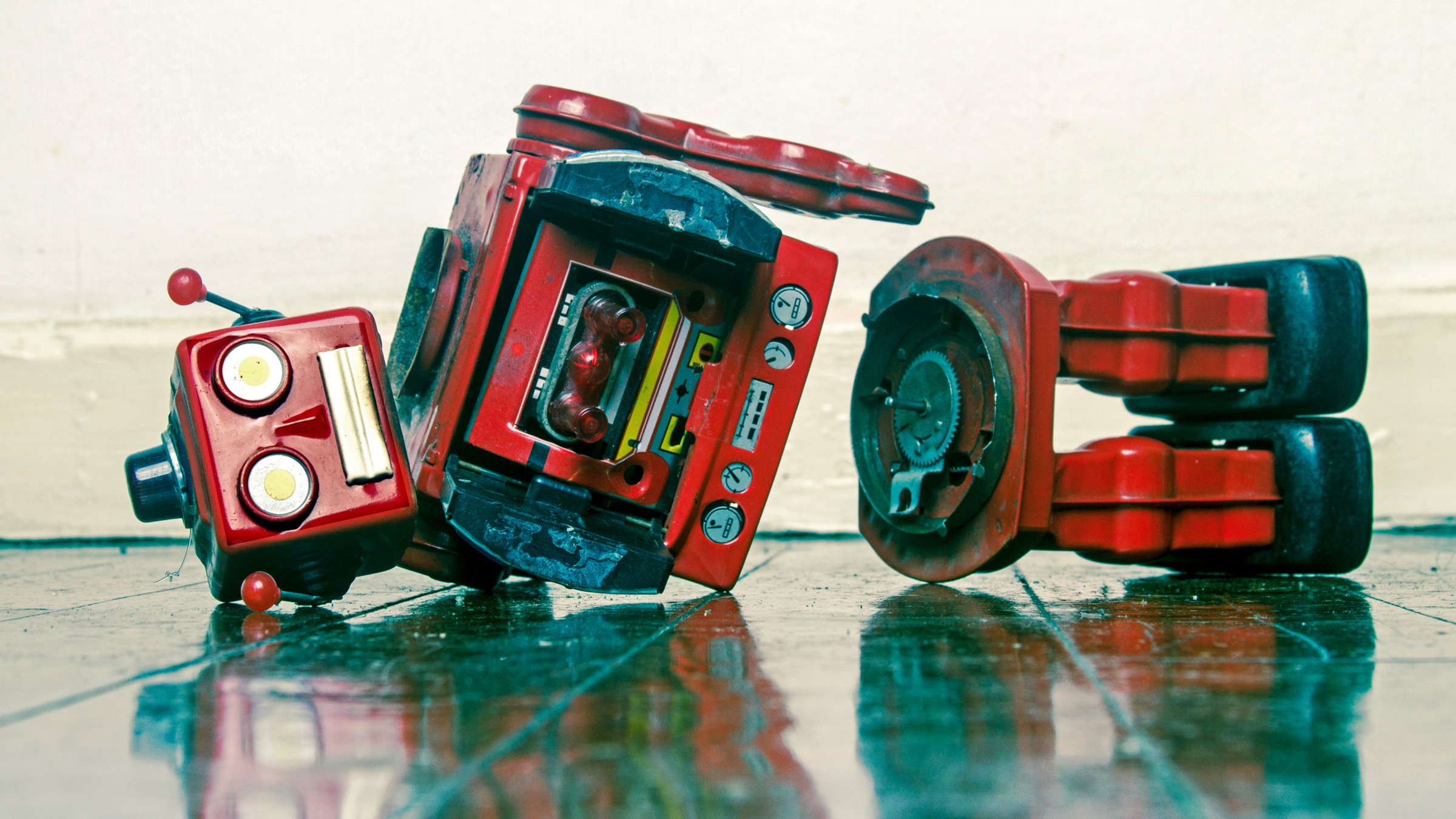
Assistive Robotics Takes a Step Forward
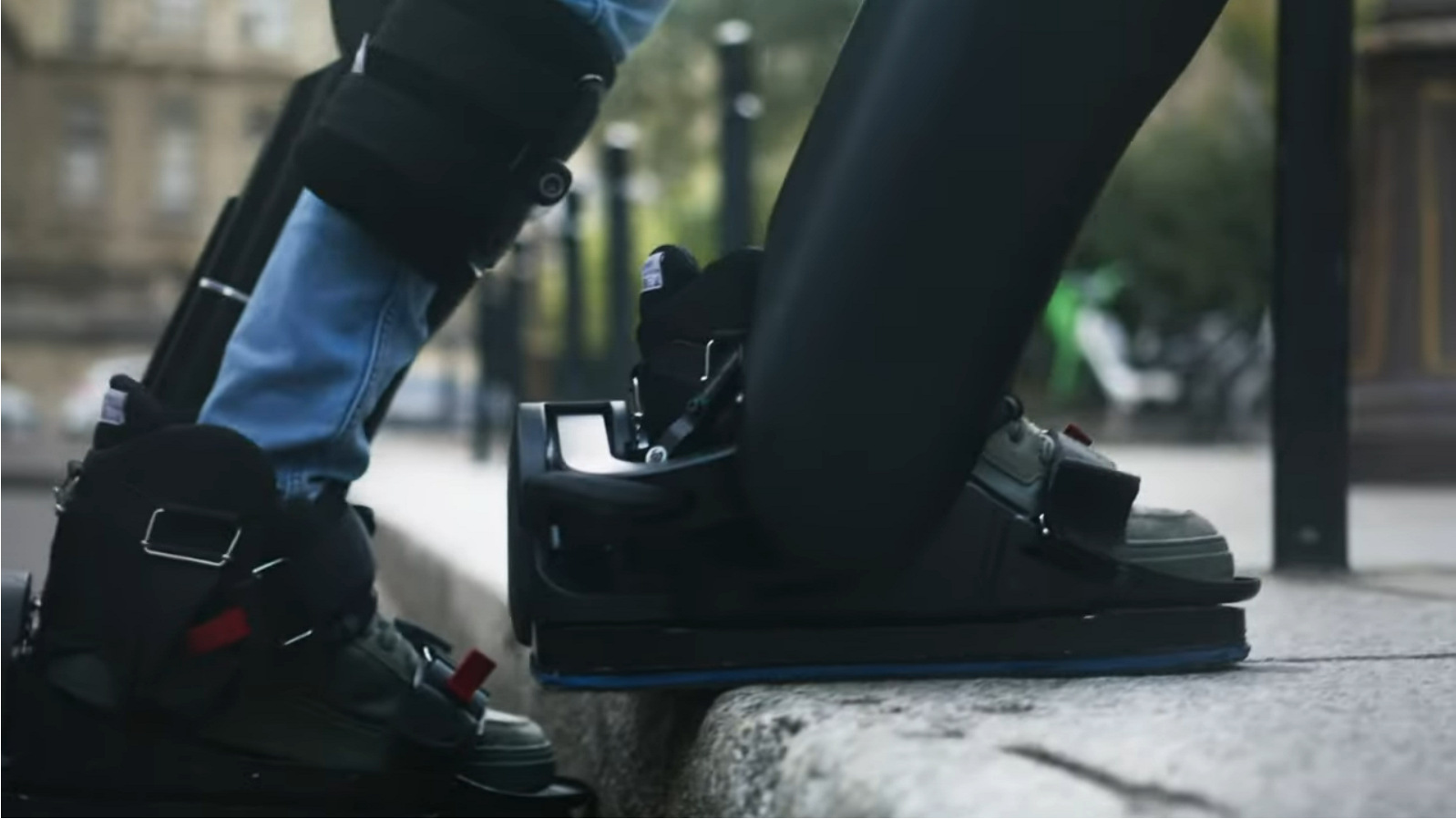
How We Lost the Internet
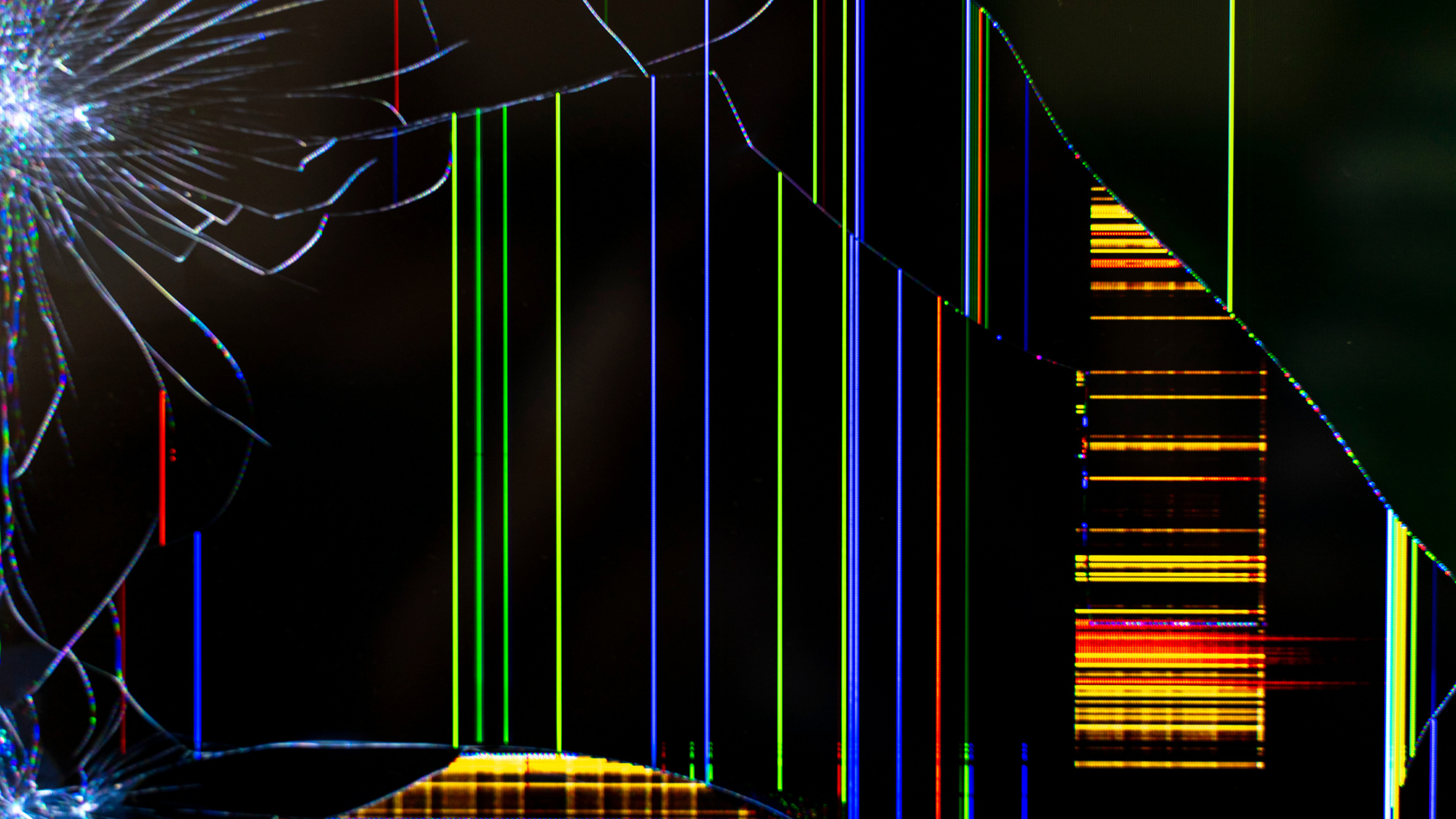
Shape the Future of Computing
ACM encourages its members to take a direct hand in shaping the future of the association. There are more ways than ever to get involved.
Communications of the ACM (CACM) is now a fully Open Access publication.
By opening CACM to the world, we hope to increase engagement among the broader computer science community and encourage non-members to discover the rich resources ACM has to offer.
University of Notre Dame
Notre Dame Philosophical Reviews
- Home ›
- Reviews ›
Computability: Turing, Gödel, Church, and Beyond
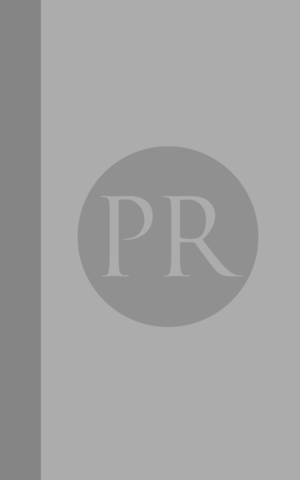
B. Jack Copeland, Carl J. Posy, and Oron Shagrir (eds.), Computability: Turing, Gödel, Church, and Beyond , MIT Press, 2013, 362pp., $20.00 (pbk), ISBN 9780262527484.
Reviewed by Andrew Arana, University of Illinois at Urbana-Champaign/Aix-Marseille University
It would be no exaggeration to say that computation changed the world in the twentieth century. Implicated in nearly all contemporary technology, today's computers empower so-called "intelligent systems" to negotiate the world of human reasoners, even driving cars. At the root of all these advances is computation, and in particular, the pioneering work of Kurt Gödel and Alan Turing in the 1930s. This work and its legacy is the focus of the volume under review. A reader of this volume will acquire a broad acquaintance with the history of the theory of computation in the twentieth century, and with ways in which this theory will continue to develop in the twenty-first century.
At the heart of the twentieth-century revolution of computation are Gödel's incompleteness theorems of 1931, which assert the existence of arithmetic sentences that are true in the standard natural numbers but unprovable in any formalized axiomatic theory of those natural numbers. The content of these results was revolutionary for the foundations of mathematics, but their proof is more directly relevant to the theory of computation. Gödel's method for demonstrating these theorems involves coding the syntax of formal theory using purely arithmetic resources. This coding takes two steps: firstly, Gödel shows that syntactic relations of a formal theory (such as "x is a proof of y") can be defined by a recursive relation, where "recursivity" is a condition codified by Hilbert and Ackermann to capture finite stepwise definition; secondly, he shows that every recursively-defined relation can be expressed by an arithmetic relation (in this volume, Martin Davis's article presents a proof of this second step that Davis judges more direct than Gödel's). Gödel recognized that the generality of his results depended upon the details of this coding, since its applicability to other formal theories requires a correspondingly general means of mechanizing syntactic derivation. He was unsure that recursivity provided such a means.
In the years after 1931 Gödel would seize instead upon Turing's analysis of computability, published in 1936, as providing this generality. Turing identified a class of conditions that he took to capture idealized human computation; these conditions could be taken to define a class of machines now called "Turing machines". The class of functions computable by a Turing machine was soon shown to be extensionally equivalent to the class of recursive functions. Other models of computation were offered around the same time, such as Alonzo Church's lambda calculus, and these classes of functions were also shown to be extensionally equivalent to the class of Turing computable functions. That these classes of functions capture the informal notion of computability has been asserted in what is known as the Church-Turing thesis, which states that a function is computable in an informal sense if and only if it is Turing computable (or computable by one of the many other extensionally equivalent models of computation). Many have wondered whether this thesis is mathematically provable, or whether it is too imprecise to admit of mathematical proof. This question is the subject of two of this volume's articles, to which we now turn.
Saul Kripke's article contends that the Church-Turing thesis is provable, arguing in a way he says resembles arguments given by Turing and Church. In particular, Kripke wants to prove that every intuitively computable function is recursive. He claims that computations are specific forms of mathematical deductions, since they are sets of instructions whose output is supposed to follow deductively from those instructions. Suppose, Kripke says, that the steps of a given deduction are fully expressible in first-order logic (he calls this supposition "Hilbert's thesis"). The Gödel completeness theorem for first-order logic entails that P is a first-order consequence of a first-order theory T if and only if P can be deduced from T by first-order logic. Taking P as the conclusion of the given computation/deduction and T as the premises of this deduction, the completeness theorem yields that there is a first-order deduction of P from T. Gödel showed moreover that the proof relation of first-order logic is recursive. It follows, Kripke contends, that the inferences of the formalized deduction are recursive, and thus the computation so expressed is recursive, as he wanted to show. The cogency of this argument comes down, of course, to whether one accepts Hilbert's thesis. One might hold that an informal deduction cannot be fully expressed in a first-order way, since informal notions are too rich for their meanings to be settled by any single finite expression. Such a view seems to have been part of Brouwer's belief that mathematical thought is essentially unformalizable. One might instead maintain the need for higher-order logic to formalize adequately the concepts and inferences of branches of mathematics that implicate the infinite, such as real analysis. Kripke holds that even if his thesis is only understood as a reduction of Church's thesis to Hilbert's thesis, he has amplified the Church-Turing thesis in a substantive way.
Stewart Shapiro's article makes the case, in contrast to Kripke's, that the Church-Turing thesis cannot be proved. Extending the case made in his 2006 article on the same subject, Shapiro articulates a view of Friedrich Waismann that our pre-theoretical mathematical concepts are generally not determined in all possible ways, so that there are typically many ways to sharpen concepts further, rather than just one "correct" way (as the Platonist would have it). He illustrates the "open texture" of mathematical concepts by drawing on Lakatos' famous dialogue on the concept of polyhedra, in which a series of proposed definitions of polyhedra are confronted with confounding cases that suggest a series of revisions to these proposals. The question is whether these sharpenings were somehow "tacit" in our original pre-theoretic concept, or whether these revisions are instead replacing the original concept with something new. The advocate of open texture holds that the original concept was not precise enough to fix any particular revision as being right. Shapiro continues by arguing that the notion of informal computability at issue in the Church-Turing thesis is subject to open texture in this way. He recounts the sharpenings of computability during the twentieth century, noting that there were other options along the way, other idealizations of computation, that could have been chosen (such as computable in polynomial time or space). That the notion chosen seemed "right" hinges upon choices that the advocate of open texture stresses are not determined by the pre-theoretic concept. We could come across procedures in the future that we want to count as computations that we do not right now: Dorit Aharonov and Umesh Vazirani discuss one such possibility, quantum computation, in their chapter. To the extent that today's concept of computability is settled, as the widespread acceptance of the Church-Turing thesis suggests, Shapiro urges us to see that settling as a sharpening, the result of human decisions, rather than the discovery of the "true" contours of the concept of computability.
A brief word is in order concerning the opposing positions of Kripke's and Shapiro's articles. Kripke's alleged proof of the Church-Turing thesis hinges on what he calls "Hilbert's thesis", that every computation can be fully expressed in a first-order way. If pre-theoretic computation is subject to open texture, then no particular expression of it fully captures its content, and hence no first-order expression does so. Thus the open texture of computability would undermine the cogency of Kripke's proof by contradicting Hilbert's thesis. Thus, as Kripke recognizes, Hilbert's thesis will be a locus of disagreement with his proof. A point more relevant to Shapiro's argument is what to make of all the proved equivalences between different models of computation: anything computable by a Turing machine is computable by a partial recursive function, is computable in the lambda calculus, and so on. One might hold that these equivalences are evidence that we have found the "real" concept of computability, because they indicate the "inevitability" of their analyses. No matter how we try to sharpen our concept of computability, we keep finding the same class of computations, extensionally speaking. Thus the original concept was precise enough to fix this class of computations, and this is evidence that these equivalent sharpenings are "right". The normativity at play here between concept and analysis demands clarification, but such clarification is needed to spell out open texture as well, since open texture is at root a negative view that no single analysis of a (mathematical) concept can be, or alternately can be shown to be, adequate for capturing the full content of that concept.
Questions of computability have often been linked to questions about the nature of the human mind, since one may wonder if the mind is a computational machine. Gödel was moved by this question, asking in his Gibbs lecture to the American Mathematical Society in 1951 if "the human mind . . . infinitely surpasses the powers of any finite machine". Three articles in this volume bear on this question and, taking Gödel's lead, on its relation to Gödel's logical work. In a paper previously published in 2006 but included in this volume, Putnam argues that if the mind (more precisely, the linguistic and scientific faculties of the mind, in Chomsky's terms) were representable by a Turing machine, then we could never know (by mathematical or empirical means) which Turing machine it is.
The articles of B. Jack Copeland and Oron Shagrir, and of Wilfried Sieg, take a more historical approach to Gödel's question, contrasting Gödel and Turing's views on this matter. As these articles point out, both Gödel and Turing see the incompleteness theorems as setting limits on our ability to mechanize mathematical reasoning. Gödel singles out the search for new axioms of infinity as transcending mechanization, though he also acknowledges, enigmatically in Sieg's judgment, that humans could augment their search for these axioms using mechanical processes. Turing, by contrast, identifies mechanized reasoning as "discipline" that human reasoners exceed in exercising what he calls "initiative", deployed when discipline fails in tackling problems. As Sieg notes, Turing suggested that new theories resulting from such "initiative" could themselves be mechanized once settled. The resulting conception of the dynamics of mathematical theory change is also the focus of Copeland and Shagrir's article. Copeland and Shagrir emphasize what they call Turing's "multi-machine theory of mind", in which human minds are Turing machines at each stage of development, but which machine they are changes during a lifetime rather than remaining fixed from birth to death. These changes occur when minds break the "discipline" of one mechanization and learn new processes, resulting in a new machine. While computational models of mind are not in vogue at present, Turing's view seems worthy of interest to contemporary investigators in cognitive science and the philosophy of mind.
Solomon Feferman's article is an engrossing discussion of computation over the real numbers, a key component of contemporary science and engineering in their use of numerical methods for simulations, modeling, optimization, and statistical analysis. He presents two recent models of computation on the reals: the BSS model developed by Lenore Blum, Michael Shub and Stephen Smale (1989/1997); and a model given by Mark Braverman and Stephen Cook (2006). Both are claimed by their creators to be well-suited as foundations for scientific computation and its numerical methods. Feferman notes that these two models of computation are incompatible, in that each classifies functions over the reals as computable that the other does not. His focal question is whether they can both be reasonable models of computation on the reals. To answer this, Feferman observes that one needs the right perspective, one given by thinking of computation over an arbitrary structure, since the two competing models identify the structure of the reals differently (algebraically and topologically, respectively). He then explicates three theories of computation over an arbitrary structure: the first due to Harvey Friedman; the second to John Tucker and Jeffery Zucker; and the third to himself, adapting work of Richard Platek and Yiannis Moschovakis. He affirms the adequacy of each of these three theories as suitable for implementing the particular models of both BSS and of Braverman and Cook, thus answering positively his focal question. Thus one who desires to implement scientific computations may choose either of these two models of computation, and the decision to choose between them must be made on other grounds.
Feferman notes that, in practice, users of scientific computations simply use approximations to the reals and real-valued functions, using what computer scientists call "floating-point arithmetic" (think, for instance, of Runga-Kutta methods for solving ordinary differential equations). The BSS and Braverman and Cook models, by contrast, do not take the reals as approximations, but rather as continuous structures. They are thus arguably more precise from a foundational point of view than the methods used by most practicing numerical analysts today. The developers of the BSS and Braverman and Cook models are concerned with the pragmatics of computation, but on grounds of computational complexity rather than the pragmatics of implementation in daily work. For instance, they introduce analogs of the P/NP complexity distinction from discrete computation in analyzing their models of computation, true to the developers' backgrounds in theoretical computer science. Feferman notes that there is another approach to computing over the reals, introduced by Errett Bishop's constructive analysis. Bishop works with approximations to the reals, and is thus closer to normal practice in scientific computation than the other models of computation considered here. Feferman narrates recent work in interpreting Bishop's work by logicians working in computability theory, and notes that further analysis of Bishop's constructive mathematics in this direction would be worthwhile because there are indications that such work could permit information on the computational complexity of Bishop's model to be mined. This would bring together the practical concerns of the scientific computation community and the community of complexity theorists in theoretical computer science.
Carl Posy's article also addresses computability over the reals and its applicability to empirical science, but has a rather different focus than Feferman's. Posy is motivated by an argument by Putnam that mathematical physics requires non-constructive methods. Posy's reconstruction of Putnam's argument identifies an assumption that the constructive and the computable coincide, and this article is an interrogation of this assumption. The focal question is whether the constructive and the computable coincide. To investigate this question, Posy compares and contrasts Hilbert's and Brouwer's analyses of the constructive as figureheads of the two central schools of contemporary constructivity (Bishop's analysis is also addressed but set aside as insufficiently imprecise for the investigation here). He argues that for Hilbert (and the Russian school of constructive analysis descending from Markov), recursive functions adequately represent finitely intuitive thinking, so that for Hilbert, the constructive and computable coincide. He then argues that for Brouwer (and the intuitionist school of constructivity that follows), the constructive is not necessarily recursive, since Brouwer sees indeterminacy as intrinsic to the activity of the free-creating subject at the root of his analysis. Thus Hilbert and Brouwer have opposing answers to the article's focal question. Posy then argues cogently that this clash is rooted in Hilbert's and Brouwer's differing adaptations of Kantian epistemology to the mathematics of the early twentieth century.
Robert Soare's article also illustrates how issues in the theory of computation are relevant to computation in practice. He draws attention to oracle machines, a type of Turing machine that can receive and use facts about membership in a particular set, called its oracle. Machines with non-computable oracles thus exceed the computational power of ordinary Turing machines, and we thus talk about computability relative to an oracle. For instance, an oracle machine that can ask questions of the halting set will be able to tell all ordinary Turing machines whether they will halt (though each oracle machine has its own halting problem and halting set, generating a hierarchy of halting sets). Soare notes that oracle machines can be thought of as online computers, able to draw on information external to the machine itself, like the World Wide Web; whereas ordinary Turing machines are always offline, so to speak. Soare thus contends that computability theory is relevant to computer science today.
Mathematical logicians and philosophers during the twentieth century focused largely on computability in an idealization where practical constraints did not apply. A computation that takes as many seconds to finish as there are elementary particles in the universe is as computable as one that takes five seconds, from the point of view of the analyses of Turing, Church, and so on. But feasibility matters very much for computations in our daily lives. An airplane autopilot that took a century to compute the plane's descent would be of little use. Naturally computer science has been more concerned with questions of feasible computability than with computability tout court , as computers have come to fill so many key roles in our lives today.
Scott Aaronson's fascinating article argues that philosophers ought to follow computer scientists by reflecting on computational complexity. In brief, complexity theory studies the amount of time needed to solve a problem computationally relative to the size of that problem; for example, how long does it take to factor an integer into its prime components, as a function of the number of digits of that integer? Complexity theorists have settled on two main classes of complexity. Feasible problems are those whose solution time grows at a polynomial rate relative to the size N of the problem, in that the time has an upper bound computed by a polynomial function on N. By contrast, an infeasible problem's solution time grows at an exponential rate relative to N, that is, this time has a lower bound computed by an exponential function on N. As Aaronson observes, there is no presently known efficient algorithm for factoring primes, and so the problem of prime factorization is currently judged infeasible, but that does not imply that there is no efficient way to factor primes. Thus membership in these complexity classes is subject to revision; hence the considerable interest in the related question of whether P, the class of problems solvable by a polynomial-time algorithm, is extensionally equivalent to NP, the class of problems for which a solution can be checked (though not necessarily solved) by a polynomial-time algorithm.
Aaronson shows the relevance of computational complexity to many areas of philosophy: to quote him,
the explanatory content of Darwinism, the nature of mathematical knowledge and proof, computationalism, syntax versus semantics, the problem of logical omniscience, debates surrounding the Turing test and Chinese Room, the problem of induction, the foundations of quantum mechanics, closed timelike curves, and economic rationality. (p. 311)
His discussions of each are overflowing with ideas; hundreds of graduate students could mine the article for distinct, highly worthwhile philosophical thesis topics. I'll present just one example to give a flavor of Aaronson's insights. In addition to metaphysical issues like the intentionality of consciousness, the possibility of artificial intelligence hinges on practical questions: the Turing test singles out human dialogue as an empirically-verifiable activity that an intelligent machine would need to be able to do. Aaronson notes that humans require relatively little empirical information to judge other humans to be conscious, and that for any given dialogue this information could be codified in a "lookup table" listing all possible histories of the dialogue and the participant actions following each such history. For a finite dialogue this lookup table would be finite, and thus a computer could access the same information that humans do in making their judgments of consciousness. Thus there is no in-principle obstacle to a computer passing the Turing test in this way. But there is, Aaronson points out, a feasibility obstacle, since an algorithm for accessing such a lookup table would be, according to our present algorithmic know-how, extraordinarily inefficient. One could cavil that merely reading off such a lookup table fails to provide the kind of intuitive understanding we take to be characteristic of human judgments. Aaronson places this issue alongside the issue of solving computationally hard problems like Sudoku puzzles: while a computer must charge through by brute force checking case after case, a human can identify high-level patterns in such problems and can thus skip most of that checking. One could, Aaronson notes, argue against the possibility of artificial intelligence by designating human pattern finding abilities as "real" intelligence, against mere brute force case checking; but then one would have to characterize precisely the high-level patterns humans are evidently so good at finding, in addition to arguing that no computer could efficiently find these patterns. Thus issues of computational complexity intensify the need for fundamental philosophical analyses of concepts like high-level patterns.
This volume sets out a plethora of topics, both looking backwards to the origins of the theory of computation, and to new settings for philosophical reflection on computation. The articles together make a sustained case for the continuing importance of computability for philosophers today, and will, I hope, stimulate compelling future research in the area.

- Table of Contents
- Random Entry
- Chronological
- Editorial Information
- About the SEP
- Editorial Board
- How to Cite the SEP
- Special Characters
- Advanced Tools
- Support the SEP
- PDFs for SEP Friends
- Make a Donation
- SEPIA for Libraries
- Entry Contents
Bibliography
Academic tools.
- Friends PDF Preview
- Author and Citation Info
- Back to Top
Turing Machines
Turing machines, first described by Alan Turing in Turing 1936–7, are simple abstract computational devices intended to help investigate the extent and limitations of what can be computed. Turing’s ‘automatic machines’, as he termed them in 1936, were specifically devised for the computing of real numbers. They were first named ‘Turing machines’ by Alonzo Church in a review of Turing’s paper (Church 1937). Today, they are considered to be one of the foundational models of computability and (theoretical) computer science. [ 1 ]
1.1 Turing’s Definition
1.2 post’s definition, 1.3 the definition formalized, 1.4 describing the behavior of a turing machine, 2.1 some (simple) examples, 2.2 computable numbers and problems, 2.3.1 interchangeability of program and behavior: a notation, 2.3.2 interchangeability of program and behavior: a basic set of functions, 2.4.1 direct and indirect proofs of uncomputable decision problems, 2.4.2 turing’s basic problem circ, print and the entscheidungsproblem, 2.4.3 the halting problem, 2.5 variations on the turing machine, 3.1 human and machine computations, 3.2 thesis, definition, axioms or theorem, 4.1 general recursive functions, 4.2 λ-definability, 4.3 post production systems, 4.4 formulation 1, 5.1 impact on theoretical computer science, 5.2 turing machines and the modern computer, 5.3 theories of programming, busy beaver, the halting problem, software simulators, hardware simulators, related entries, 1. definitions of the turing machine.
Turing introduced Turing machines in the context of research into the foundations of mathematics. More particularly, he used these abstract devices to prove that there is no effective general method or procedure to solve, calculate or compute every instance of the following problem:
Entscheidungsproblem The problem to decide for every statement in first-order logic (the so-called restricted functional calculus, see the entry on classical logic for an introduction) whether or not it is derivable in that logic.
Note that in its original form (Hilbert & Ackermann 1928), the problem was stated in terms of validity rather than derivability. Given Gödel’s completeness theorem (Gödel 1929) proving that there is an effective procedure (or not) for derivability is also a solution to the problem in its validity form. In order to tackle this problem, one needs a formalized notion of “effective procedure” and Turing’s machines were intended to do exactly that.
A Turing machine then, or a computing machine as Turing called it, in Turing’s original definition is a machine capable of a finite set of configurations \(q_{1},\ldots,q_{n}\) (the states of the machine, called m -configurations by Turing). It is supplied with a one-way infinite and one-dimensional tape divided into squares each capable of carrying exactly one symbol. At any moment, the machine is scanning the content of one square r which is either blank (symbolized by \(S_0\)) or contains a symbol \(S_{1},\ldots ,S_{m}\) with \(S_1 = 0\) and \(S_2 = 1\).
The machine is an automatic machine ( a -machine) which means that at any given moment, the behavior of the machine is completely determined by the current state and symbol (called the configuration ) being scanned. This is the so-called determinacy condition ( Section 3 ). These a -machines are contrasted with the so-called choice machines for which the next state depends on the decision of an external device or operator (Turing 1936–7: 232). A Turing machine is capable of three types of action:
- Print \(S_i\), move one square to the left ( L ) and go to state \(q_{j}\)
- Print \(S_i\), move one square to the right ( R ) and go to state \(q_{j}\)
- Print \(S_i\), do not move ( N ) and go to state \(q_{j}\)
The ‘program’ of a Turing machine can then be written as a finite set of quintuples of the form:
Where \(q_i\) is the current state, \(S_j\) the content of the square being scanned, \(S_{i,j}\) the new content of the square; \(M_{i,j}\) specifies whether the machine is to move one square to the left, to the right or to remain at the same square, and \(q_{i,j}\) is the next state of the machine. These quintuples are also called the transition rules of a given machine. The Turing machine \(T_{\textrm{Simple}}\) which, when started from a blank tape, computes the sequence \(S_0S_1S_0S_1\ldots\) is then given by Table 1 .
Table 1: Quintuple representation of \(T_{\textrm{Simple}}\)
Note that \(T_{\textrm{Simple}}\) will never enter a configuration where it is scanning \(S_1\) so that two of the four quintuples are redundant. Another typical format to represent Turing machines and which was also used by Turing is the transition table . Table 2 gives the transition table of \(T_{\textrm{Simple}}\).
Table 2: Transition table for \(T_{\textrm{Simple}}\)
Where current definitions of Turing machines usually have only one type of symbols (usually just 0 and 1; it was proven by Shannon that any Turing machine can be reduced to a binary Turing machine (Shannon 1956)) Turing, in his original definition of so-called computing machines , used two kinds of symbols: the figures which consist entirely of 0s and 1s and the so-called symbols of the second kind . These are differentiated on the Turing machine tape by using a system of alternating squares of figures and symbols of the second kind. One sequence of alternating squares contains the figures and is called the sequence of F -squares. It contains the sequence computed by the machine ; the other is called the sequence of E -squares. The latter are used to mark F -squares and are there to “assist the memory” (Turing 1936–7: 232). The content of the E -squares is liable to change. F -squares however cannot be changed which means that one cannot implement algorithms whereby earlier computed digits need to be changed. Moreover, the machine will never print a symbol on an F -square if the F -square preceding it has not been computed yet. This usage of F and E -squares can be quite useful (see Sec. 2.3 ) but, as was shown by Emil L. Post, it results in a number of complications (see Sec. 1.2 ).
There are two important things to notice about the Turing machine setup. The first concerns the definition of the machine itself, namely that the machine’s tape is potentially infinite. This corresponds to an assumption that the memory of the machine is (potentially) infinite. The second concerns the definition of Turing computable, namely that a function will be Turing computable if there exists a set of instructions that will result in a Turing machine computing the function regardless of the amount of time it takes. One can think of this as assuming the availability of potentially infinite time to complete the computation.
These two assumptions are intended to ensure that the definition of computation that results is not too narrow. This is, it ensures that no computable function will fail to be Turing-computable solely because there is insufficient time or memory to complete the computation. It follows that there may be some Turing computable functions which may not be carried out by any existing computer, perhaps because no existing machine has sufficient memory to carry out the task. Some Turing computable functions may not ever be computable in practice, since they may require more memory than can be built using all of the (finite number of) atoms in the universe. If we moreover assume that a physical computer is a finite realization of the Turing machine, and so that the Turing machine functions as a good formal model for the computer, a result which shows that a function is not Turing computable is very strong, since it implies that no computer that we could ever build could carry out the computation. In Section 2.4, it is shown that there are functions which are not Turing-computable.
Turing’s definition was standardized through (some of) Post’s modifications of it in Post 1947. In that paper Post proves that a certain problem from mathematics known as Thue’s problem or the word problem for semi-groups is not Turing computable (or, in Post’s words, recursively unsolvable). Post’s main strategy was to show that if it were decidable then the following decision problem from Turing 1936–7 would also be decidable:
PRINT? The problem to decide for every Turing machine M whether or not it will ever print some symbol (for instance, 0).
It was however proven by Turing that PRINT? is not Turing computable and so the same is true of Thue’s problem.
While the uncomputability of PRINT? plays a central role in Post’s proof, Post believed that Turing’s proof of that was affected by the “spurious Turing convention” (Post 1947: 9), viz. the system of F and E -squares. Thus, Post introduced a modified version of the Turing machine. The most important differences between Post’s and Turing’s definition are:
Post’s Turing machine, when in a given state, either prints or moves and so its transition rules are more ‘atomic’ (it does not have the composite operation of moving and printing). This results in the quadruple notation of Turing machines, where each quadruple is in one of the three forms of Table 3 :
Table 3: Post’s Quadruple notation
- Post’s Turing machine has only one kind of symbol and so does not rely on the Turing system of F and E -squares.
- Post’s Turing machine has a two-way infinite tape.
- Post’s Turing machine halts when it reaches a state for which no actions are defined.
Note that Post’s reformulation of the Turing machine is very much rooted in his Post 1936. (Some of) Post’s modifications of Turing’s definition became part of the definition of the Turing machine in standard works such as Kleene 1952 and Davis 1958. Since that time, several (logically equivalent) definitions have been introduced. Today, standard definitions of Turing machines are, in some respects, closer to Post’s Turing machines than to Turing’s machines. In what follows we will use a variant on the standard definition from Minsky 1967 which uses the quintuple notation but has no E and F -squares and includes a special halting state H . It also has only two move operations, viz., L and R and so the action whereby the machine merely prints is not used. When the machine is started, the tape is blank except for some finite portion of the tape. Note that the blank square can also be represented as a square containing the symbol \(S_0\) or simply 0. The finite content of the tape will also be called the dataword on the tape.
Talk of “tape” and a “read-write head” is intended to aid the intuition (and reveals something of the time in which Turing was writing) but plays no important role in the definition of Turing machines. In situations where a formal analysis of Turing machines is required, it is appropriate to spell out the definition of the machinery and program in more mathematical terms. Purely formally a Turing machine can be specified as a quadruple \(T = (Q,\Sigma, s, \delta)\) where:
- Q is a finite set of states q
- \(\Sigma\) is a finite set of symbols
- s is the initial state \(s \in Q\)
\(\delta\) is a transition function determining the next move:
The transition function for the machine T is a function from computation states to computation states. If \(\delta(q_i,S_j) = (S_{i,j},D,q_{i,j})\), then when the machine’s state is \(q_j\), reading the symbol \(S_j\), \(T\) replaces \(S_j\) by \(S_{i,j}\), moves in direction \(D \in \{L,R\}\) and goes to state \(q_{i,j}\).
We introduce a representation which allows us to describe the behavior or dynamics of a Turing machine \(T_n\), relying on the notation of the complete configuration (Turing 1936–7: 232) also known today as instantaneous description (ID) (Davis 1982: 6). At any stage of the computation of \(T_{i}\) its ID is given by:
- (1) the content of the tape, that is, its data word
- (2) the location of the reading head
- (3) the machine’s internal state
So, given some Turing machine T which is in state \(q_{i}\) scanning the symbol \(S_{j}\), its ID is given by \(Pq_{i}S_{j}Q\) where P and Q are the finite words to the left and right hand side of the square containing the symbol \(S_{j}\). Figure 1 gives a visual representation of an ID of some Turing machine T in state \(q_i\) scanning the tape.
Figure 1: A complete configuration of some Turing machine T
The notation thus allows us to capture the developing behavior of the machine and its tape through its consecutive IDs. Figure 2 gives the first few consecutive IDs of \(T_{\textrm{Simple}}\) using a graphical representation.
Figure 2: The dynamics of \(T_{\textrm{Simple}}\) graphical representation
The animation can be started by clicking on the picture. One can also explicitly print the consecutive IDs, using their symbolic representations. This results in a state-space diagram of the behavior of a Turing machine. So, for \(T_{\textrm{Simple}}\) we get (Note that \(\overline{0}\) means the infinite repetition of 0s):
2. Computing with Turing Machines
As explained in Sec. 1.1 , Turing machines were originally intended to formalize the notion of computability in order to tackle a fundamental problem of mathematics. Independently of Turing, Alonzo Church gave a different but logically equivalent formulation (see Sec. 4 ). Today, most computer scientists agree that Turing’s, or any other logically equivalent, formal notion captures all computable problems, viz. for any computable problem, there is a Turing machine which computes it. This is known as the Church-Turing thesis , Turing’s thesis (when the reference is only to Turing’s work) or Church’s thesis (when the reference is only to Church’s work).
It implies that, if accepted, any problem not computable by a Turing machine is not computable by any finite means whatsoever. Indeed, since it was Turing’s ambition to capture “[all] the possible processes which can be carried out in computing a number” (Turing 1936–7: 249), it follows that, if we accept Turing’s analysis:
- Any problem not computable by a Turing machine is not “computable” in the absolute sense (at least, absolute relative to humans, see Section 3 ).
It is my contention that [the] operations [of a computing machine] include all those which are used in the computation of a number. (Turing 1936–7: 231)
In this section, examples will be given which illustrate the computational power and boundaries of the Turing machine model. Section 3 then discusses some philosophical issues related to Turing’s thesis.
In order to speak about a Turing machine that does something useful from the human perspective, we will have to provide an interpretation of the symbols recorded on the tape. For example, if we want to design a machine which will compute some mathematical function, addition say, then we will need to describe how to interpret the ones and zeros appearing on the tape as numbers.
In the examples that follow we will represent the number n as a block of \(n+1\) copies of the symbol ‘1’ on the tape. Thus we will represent the number 0 as a single ‘1’ and the number 3 as a block of four ‘1’s. This is called unary notation .
We will also have to make some assumptions about the configuration of the tape when the machine is started, and when it finishes, in order to interpret the computation. We will assume that if the function to be computed requires n arguments, then the Turing machine will start with its head scanning the leftmost ‘1’ of a sequence of n blocks of ‘1’s. The blocks of ‘1’s representing the arguments must be separated by a single occurrence of the symbol ‘0’. For example, to compute the sum \(3+4\), a Turing machine will start in the configuration shown in Figure 3 .
Figure 3: Initial configuration for a computation over two numbers n and m
Here the supposed addition machine takes two arguments representing the numbers to be added, starting at the leftmost 1 of the first argument. The arguments are separated by a single 0 as required, and the first block contains four ‘1’s, representing the number 3, and the second contains five ‘1’s, representing the number 4.
A machine must finish in standard configuration too. There must be a single block of symbols (a sequence of 1s representing some number or a symbol representing another kind of output) and the machine must be scanning the leftmost symbol of that sequence. If the machine correctly computes the function then this block must represent the correct answer.
Adopting this convention for the terminating configuration of a Turing machine means that we can compose machines by identifying the final state of one machine with the initial state of the next.
Addition of two numbers n and m
Table 4 gives the transition table of a Turing machine \(T_{\textrm{Add}_2}\) which adds two natural numbers n and m . We assume the machine starts in state \(q_1\) scanning the leftmost 1 of \(n+1\).
Table 4: Transition table for \(T_{\textrm{Add}_2}\)
The idea of doing an addition with Turing machines when using unary representation is to shift the leftmost number n one square to the right. This is achieved by erasing the leftmost 1 of \(n +1\) (this is done in state \(q_1\)) and then setting the 0 between \(n+1\) and \(m+1\) to 1 (state \(q_2\)). We then have \(n + m + 2\) and so we still need to erase one additional 1. This is done by erasing the leftmost 1 (states \(q_3\) and \(q_4\)). Figure 4 shows this computation for \(3 + 4\).
Figure 4: The computation of \(3+4\) by \(T_{\textrm{Add}_2}\)
Addition of n numbers
We can generalize \(T_{\textrm{Add}_2}\) to a Turing machine \(T_{\textrm{Add}_i}\) for the addition of an arbitrary number i of integers \(n_1, n_2,\ldots, n_j\). We assume again that the machine starts in state \(q_1\) scanning the leftmost 1 of \(n_1+1\). The transition table for such a machine \(T_{\textrm{Add}_i}\) is given in Table 5 .
Table 5: Transition table for \(T_{\textrm{Add}_i}\)
The machine \(T_{\textrm{Add}_i}\) uses the principle of shifting the addends to the right which was also used for \(T_{\textrm{Add}_2}\). More particularly, \(T_{add_i}\) computes the sum of \(n_1 + 1\), \(n_2 + 1\),… \(n_i+1\) from left to right, viz. it computes this sum as follows:
The most important difference between \(T_{\textrm{Add}_2}\) and \(T_{\textrm{Add}_i}\) is that \(T_{\textrm{Add}_i}\) needs to verify if the leftmost addend \(N_j, 1 < j \leq i\) is equal to \(N_i\). This is achieved by checking whether the first 0 to the right of \(N_j\) is followed by another 0 or not (states \(q_2\) and \(q_3\)). If it is not the case, then there is at least one more addend \(n_{j+1}\) to be added. Note that, as was the case for \(T_{\textrm{Add}_2}\), the machine needs to erase an additional one from the addend \(n_{j+1}\) which is done via state \(q_5\). It then moves back to state \(q_1\). If, on the other hand, \(N_j = N_i\), the machine moves to the leftmost 1 of \(N_i = n_1 + n_2 + \ldots + n_i + 1 \) and halts.
Turing’s original paper is concerned with computable (real) numbers . A (real) number is Turing computable if there exists a Turing machine which computes an arbitrarily precise approximation to that number. All of the algebraic numbers (roots of polynomials with algebraic coefficients) and many transcendental mathematical constants, such as e and \(\pi\) are Turing-computable. Turing gave several examples of classes of numbers computable by Turing machines (see section 10 Examples of large classes of numbers which are computable of Turing 1936–7) as a heuristic argument showing that a wide diversity of classes of numbers can be computed by Turing machines.
One might wonder however in what sense computation with numbers, viz. calculation, captures non-numerical but computable problems and so how Turing machines capture all general and effective procedures which determine whether something is the case or not. Examples of such problems are:
- “decide for any given x whether or not x denotes a prime”
- “decide for any given x whether or not x is the description of a Turing machine”.
In general, these problems are of the form:
- “decide for any given x whether or not x has property X ”
An important challenge of both theoretical and concrete advances in computing (often at the interface with other disciplines) has become the problem of providing an interpretation of X such that it can be tackled computationally. To give just one concrete example, in daily computational practices it might be important to have a method to decide for any digital “source” whether or not it can be trusted and so one needs a computational interpretation of trust.
The characteristic function of a predicate is a function which has the value TRUE or FALSE when given appropriate arguments. In order for such functions to be computable, Turing relied on Gödel’s insight that these kind of problems can be encoded as a problem about numbers (See Gödel’s incompleteness theorem and the next Sec. 2.3 ) In Turing’s wording:
The expression “there is a general process for determining …” has been used [here] […] as equivalent to “there is a machine which will determine …”. This usage can be justified if and only if we can justify our definition of “computable”. For each of these “general process” problems can be expressed as a problem concerning a general process for determining whether a given integer n has a property \(G(n)\) [e.g. \(G(n)\) might mean “ n is satisfactory” or “ n is the Gödel representation of a provable formula”], and this is equivalent to computing a number whose n -th figure is 1 if \(G(n)\) is true and 0 if it is false. (1936–7: 248)
It is the possibility of coding the “general process” problems as numerical problems that is essential to Turing’s construction of the universal Turing machine and its use within a proof that shows there are problems that cannot be computed by a Turing machine.
2.3 Turing’s Universal Machine
The universal Turing machine which was constructed to prove the uncomputability of certain problems, is, roughly speaking, a Turing machine that is able to compute what any other Turing machine computes. Assuming that the Turing machine notion fully captures computability (and so that Turing’s thesis is valid), it is implied that anything which can be “computed”, can also be computed by that one universal machine. Conversely, any problem that is not computable by the universal machine is considered to be uncomputable.
This is the rhetorical and theoretical power of the universal machine concept, viz. that one relatively simple formal device captures all “ the possible processes which can be carried out in computing a number ” (Turing 1936–7). It is also one of the main reasons why Turing has been retrospectively identified as one of the founding fathers of computer science (see Section 5 ).
So how to construct a universal machine U out of the set of basic operations we have at our disposal? Turing’s approach is the construction of a machine U which is able to (1) ‘understand’ the program of any other machine \(T_{n}\) and, based on that “understanding”, (2) ‘mimic’ the behavior of \(T_{n}\). To this end, a method is needed which allows to treat the program and the behavior of \(T_n\) interchangeably since both aspects are manipulated on the same tape and by the same machine. This is achieved by Turing in two basic steps: the development of (1) a notational method (2) a set of elementary functions which treats that notation—independent of whether it is formalizing the program or the behavior of \(T_n\)—as text to be compared, copied down, erased, etc. In other words, Turing develops a technique that allows to treat program and behavior on the same level.
Given some machine \(T_n\), Turing’s basic idea is to construct a machine \(T_n'\) which, rather than directly printing the output of \(T_n\), prints out the successive complete configurations or instantaneous descriptions of \(T_n\). In order to achieve this, \(T_n'\):
[…] could be made to depend on having the rules of operation […] of [\(T_n\)] written somewhere within itself […] each step could be carried out by referring to these rules. (Turing 1936–7: 242)
In other words, \(T_n'\) prints out the successive complete configurations of \(T_n\) by having the program of \(T_n\) written on its tape. Thus, Turing needs a notational method which makes it possible to ‘capture’ two different aspects of a Turing machine on one and the same tape in such a way they can be treated by the same machine , viz.:
- (1) its description in terms of what it should do —the quintuple notation
- (2) its description in terms of what it is doing —the complete configuration notation
Thus, a first and perhaps most essential step, in the construction of U are the quintuple and complete configuration notation and the idea of putting them on the same tape. More particularly, the tape is divided into two regions which we will call the A and B region here. The A region contains a notation of the ‘program’ of \(T_n\) and the B region a notation for the successive complete configurations of \(T_n\). In Turing’s paper they are separated by an additional symbol “::”.
To simplify the construction of U and in order to encode any Turing machine as a unique number, Turing develops a third notation which permits to express the quintuples and complete configurations with letters only. This is determined by [Note that we use Turing’s original encoding. Of course, there is a broad variety of possible encodings, including binary encodings]:
- Replacing each state \(q_i\) in a quintuple of \(T_n\) by \[D\underbrace{A\ldots A}_i,\] so, for instance \(q_3\) becomes \(DAAA\).
- Replacing each symbol \(S_{j}\) in a quintuple of \(T_n\) by \[D\underbrace{C\ldots C}_j,\] so, for instance, \(S_1\) becomes \(DC\).
Using this method, each quintuple of some Turing machine \(T_n\) can be expressed in terms of a sequence of capital letters and so the ‘program’ of any machine \(T_{n}\) can be expressed by the set of symbols A, C, D, R, L, N and ;. This is the so-called Standard Description (S.D.) of a Turing machine. Thus, for instance, the S.D. of \(T_{\textrm{Simple}}\) is:
This is, essentially, Turing’s version of Gödel numbering . Indeed, as Turing shows, one can easily get a numerical description representation or Description Number (D.N.) of a Turing machine \(T_{n}\) by replacing:
- “A” by “1”
- “C” by “2”
- “D” by “3”
- “L” by “4”
- “R” by “5”
- “N” by “6”
- “;” by “7”
Thus, the D.N. of \(T_{\textrm{Simple}}\) is:
Note that every machine \(T_n\) has a unique D.N.; a D.N. represents one and one machine only.
Clearly, the method used to determine the \(S.D.\) of some machine \(T_n\) can also be used to write out the successive complete configurations of \(T_n\). Using “:” as a separator between successive complete configurations, the first few complete configurations of \(T_{\textrm{Simple}}\) are:
Having a notational method to write the program and successive complete configurations of some machine \(T_n\) on one and the same tape of some other machine \(T_n'\) is the first step in Turing’s construction of U . However, U should also be able to “emulate” the program of \(T_n\) as written in region A so that it can actually write out its successive complete configurations in region B . Moreover it should be possible to “take out and exchange[…] [the rules of operations of some Turing machine] for others” (Turing 1936–7: 242). Viz., it should be able not just to calculate but also to compute, an issue that was also dealt with by others such as Church, Gödel and Post using their own formal devices. It should, for instance, be able to “recognize” whether it is in region A or B and it should be able to determine whether or not a certain sequence of symbols is the next state \(q_i\) which needs to be executed.
This is achieved by Turing through the construction of a sequence of Turing computable problems such as:
- Finding the leftmost or rightmost occurrence of a sequence of symbols
- Marking a sequence of symbols by some symbol a (remember that Turing uses two kinds of alternating squares)
- Comparing two symbol sequences
- Copying a symbol sequence
Turing develops a notational technique, called skeleton tables , for these functions which serves as a kind of shorthand notation for a complete Turing machine table but can be easily used to construct more complicated machines from previous ones. The technique is quite reminiscent of the recursive technique of composition (see: recursive functions ).
To illustrate how such functions are Turing computable, we discuss one such function in more detail, viz. the compare function. It is constructed on the basis of a number of other Turing computable functions which are built on top of each other. In order to understand how these functions work, remember that Turing used a system of alternating F and E -squares where the F -squares contain the actual quintuples and complete configurations and the E -squares are used as a way to mark off certain parts of the machine tape. For the comparing of two sequences \(S_1\) and \(S_2\), each symbol of \(S_1\) will be marked by some symbol a and each symbol of \(S_2\) will be marked by some symbol b .
Turing defined nine different functions to show how the compare function can be computed with Turing machines:
- FIND\((q_{i}, q_{j},a)\): this machine function searches for the leftmost occurrence of a . If a is found, the machine moves to state \(q_{i}\) else it moves to state \(q_{j}\). This is achieved by having the machine first move to the beginning of the tape (indicated by a special mark) and then to have it move right until it finds a or reaches the rightmost symbol on the tape.
- FINDL\((q_{i}, q_{j},a)\): the same as FIND but after a has been found, the machine moves one square to the left. This is used in functions which need to compute on the symbols in F -squares which are marked by symbols a in the E -squares.
- ERASE\((q_{i},q_{j},a)\): the machine computes FIND. If a is found, it erases a and goes to state \(q_{i}\) else it goes to state \(q_{j}\)
- ERASE_ALL\((q_j,a) = \textrm{ERASE}(\textrm{ERASE}\_\textrm{ALL}, q_j,a)\): the machines computes ERASE on a repeatedly until all a ’s have been erased. Then it moves to \(q_{j}\).
- EQUAL\((q_i,q_j,a)\): the machine checks whether or not the current symbol is a . If yes, it moves to state \(q_i\) else it moves to state \(q_j\)
- CMP_XY\((q_i,q_j,b) = \textrm{FINDL(EQUAL}(q_i,q_j,x), q_j, b)\): whatever the current symbol x , the machine computes FINDL on b (and so looks for the symbol marked by b ). If there is a symbol y marked with b , the machine computes \(\textrm{EQUAL}\) on x and y , else, the machine goes to state \(q_j\). In other words, CMP_XY\((q_i,q_j,b)\) compares whether the current symbol is the same as the leftmost symbol marked b .
- COMPARE_MARKED\((q_i,q_j,q_n,a,b)\): the machine checks whether the leftmost symbols marked a and b respectively are the same. If there is no symbol marked a nor b , the machine goes to state \(q_{n}\); if there is a symbol marked a and one marked b and they are the same, the machine goes to state \(q_i\), else the machine goes to state \(q_j\). The function is computed as \(\textrm{FINDL(CMP}\_XY(q_i,q_j,b), \textrm{FIND}(q_j,q_n,b),a)\)
- \(\textrm{COMPARE}\_\textrm{ERASE}(q_iq_j,q_n,a,b)\): the same as COMPARE_MARKED but when the symbols marked a and b are the same, the marks a and b are erased. This is achieved by computing \(\textrm{ERASE}\) first on a and then on b .
\(\textrm{COMPARE}\_\textrm{ALL}(q_j,q_n,a,b)\) The machine compares the sequences A and B marked with a and b respectively. This is done by repeatedly computing COMPARE_ERASE on a and b . If A and B are equal, all a ’s and b ’s will have been erased and the machine moves to state \(q_j\), else, it will move to state \(q_n\). It is computed by \[\textrm{COMPARE}\_\textrm{ERASE}(\textrm{COMPARE}\_\textrm{ALL}(q_j,q_n,a,b),q_j,q_n,a,b)\] and so by recursively calling \(\textrm{COMPARE}\_\textrm{ALL}\).
In a similar manner, Turing defines the following functions:
- \(\textrm{COPY}(q_i,a)\): copy the sequence of symbols marked with a ’s to the right of the last complete configuration and erase the marks.
- \(\textrm{COPY}_{n}(q_i, a_1,a_2,\ldots ,a_n)\): copy down the sequences marked \(a_1\) to \(a_n\) to the right of the last complete configuration and erase all marks \(a_i\).
- \(\textrm{REPLACE}(q_i, a,b)\): replace all letters a by b
- \(\textrm{MARK_NEXT_CONFIG}(q_i,a)\): mark the first configuration \(q_iS_j\) to the right of the machine’s head with the letter a .
- \(\textrm{FIND}\_\textrm{RIGHT}(q_i,a)\): find the rightmost symbol a .
Using the basic functions COPY, REPLACE and COMPARE, Turing constructs a universal Turing machine.
Below is an outline of the universal Turing machine indicating how these basic functions indeed make possible universal computation. It is assumed that upon initialization, U has on its tape the S.D. of some Turing machine \(T_n\). Remember that Turing uses the system of alternating F and E -squares and so, for instance, the S.D. of \(T_{\textrm{Simple}}\) will be written on the tape of U as:
where “_” indicates an unmarked E -square.
- INIT: To the right of the rightmost quintuple of T _ n , U prints ::_:_ D _ A _, where _ indicates an unmarked E -square.
FIND_NEXT_STATE: The machine first marks (1) with y the configuration \(q_{CC,i}S_{CC,j}\) of the rightmost (and so last) complete configuration computed by U in the B part of the tape and (2) with x the configuration \(q_{q,m}S_{q,n}\) of the leftmost quintuple which is not preceded by a marked (with the letter z ) semicolon in the A part of the tape. The two configurations are compared. If they are identical, the machine moves to MARK_OPERATIONS, if not, it marks the semicolon preceding \(q_{q,m}S_{q,n}\) with z and goes to FIND_NEXT_STATE. This is easily achieved using the function COMPARE_ALL which means that, whatever the outcome of the comparison, the marks x and y will be erased. For instance, suppose that \(T_n = T_{\textrm{Simple}}\) and that the last complete configuration of \(T_{\textrm{Simple}}\) as computed by U is:
Then U will move to region A and determine that the corresponding quintuple is:
MARK_OPERATIONS: The machine U marks the operations that it needs to execute in order to compute the next complete configuration of \(T_n\). The printing and move (L,R, N) operations are marked with u and the next state with y . All marks z are erased. Continuing with our example, U will mark \(\eqref{quint_univ}\) as follows:
MARK_COMPCONFIG: The last complete configuration of \(T_n\) as computed by U is marked into four regions: the configuration \(q_{CC,i}S_{CC,j}\) itself is left unmarked; the symbol just preceding it is marked with an x and the remaining symbols to the left or marked with v . Finally, all symbols to the right, if any, are marked with w and a “:” is printed to the right of the rightmost symbol in order to indicate the beginning of the next complete configuration of \(T_n\) to be computed by U . Continuing with our example, \(\eqref{CC_univ}\) will be marked as follows by U :
U then goes to PRINT
- PRINT. It is determined if, in the instructions that have been marked in MARK_OPERATIONS, there is an operation Print 0 or Print 1. If that is the case, \(0:\) respectively \(1:\) is printed to the right of the last complete configuration. This is not a necessary function but Turing insisted on having U print out not just the (coded) complete configurations computed by \(T_n\) but also the actual (binary) real number computed by \(T_n\).
PRINT_COMPLETE_CONFIGURATION. U prints the next complete configuration and erases all marks u, v, w, x, y . It then returns to FIND_NEXT_STATE. U first searches for the rightmost letter u , to check which move is needed ( R, L, N ) and erases the mark u for R, L, N . Depending on the value L, R or N will then write down the next complete configuration by applying COPY\(_5\) to u, v, w, x, y . The move operation ( L, R, N ) is accounted for by the particular combination of u, v, w, x, y :
Following our example, since \(T_{\textrm{Simple}}\) needs to move right, the new rightmost complete configursiation of \(T_{\textrm{Simple}}\) written on the tape of U is:
Since we have that for this complete configuration the square being scanned by \(T_{\textrm{Simple}}\) is one that was not included in the previous complete configuration (viz. \(T_{\textrm{Simple}}\) has reached beyond the rightmost previous point) the complete configuration as written out by U is in fact incomplete. This small defect was corrected by Post (Post 1947) by including an additional instruction in the function used to mark the complete configuration in the next round.
As is clear, Turing’s universal machine indeed requires that program and ‘data’ produced by that program are manipulated interchangeably, viz. the program and its productions are put next to each other and treated in the same manner, as sequences of letters to be copied, marked, erased and compared.
Turing’s particular construction is quite intricate with its reliance on the F and E -squares, the use of a rather large set of symbols and a rather arcane notation used to describe the different functions discussed above. Since 1936 several modifications and simplifications have been implemented. The removal of the difference between F and E -squares was already discussed in Section 1.2 and it was proven by Shannon that any Turing machine, including the universal machine, can be reduced to a binary Turing machine (Shannon 1956). Since the 1950s, there has been quite some research on what could be the smallest possible universal devices (with respect to the number of states and symbols) and quite some “small” universal Turing machines have been found. These results are usually achieved by relying on other equivalent models of computability such as, for instance, tag systems. For a survey on research into small universal devices (see Margenstern 2000; Woods & Neary 2009).
2.4 The Halting Problem and the Entscheidungsproblem
As explained, the purpose of Turing’s paper was to show that the Entscheidungsproblem for first-order logic is not computable. The same result was achieved independently by Church (1936a, 1936b) using a different kind of formal device which is logically equivalent to a Turing machine (see Sec. 4 ). The result went very much against what Hilbert had hoped to achieve with his finitary and formalist program. Indeed, next to Gödel’s incompleteness results, they broke much of Hilbert’s dream of making mathematics void of Ignorabimus and which was explicitly expressed in the following words of Hilbert:
The true reason why Comte could not find an unsolvable problem, lies in my opinion in the assertion that there exists no unsolvable problem. Instead of the stupid Ignorabimus, our solution should be: We must know. We shall know. (1930: 963) [translation by the author]
Note that the solvability Hilbert is referring to here concerns solvability of mathematical problems in general and not just mechanically solvable. It is shown however in Mancosu et al. 2009 (p. 94), that this general aim of solving every mathematical problem, underpins two particular convictions of Hilbert namely that (1) the axioms of number theory are complete and (2) that there are no undecidable problems in mathematics.
So, how can one show, for a particular decision problem \(\textrm{D}_i\), that it is not computable? There are two main methods:
- Indirect proof: take some problem \(\textrm{D}_{\textrm{uncomp}}\) which is already known to be uncomputable and show that the problem “reduces” to \(\textrm{D}_{i}\).
- Direct proof: prove the uncomputability of \(\textrm{D}_{i}\) directly by assuming some version of the Church-Turing thesis.
Today, one usually relies on the first method while it is evident that in the absence of a problem \(\textrm{D}_{\textrm{uncomp}}\), Turing but also Church and Post (see Sec. 4 ) had to rely on the direct approach.
The notion of reducibility has its origins in the work of Turing and Post who considered several variants of computability (Post 1947; Turing 1939). The concept was later appropriated in the context of computational complexity theory and is today one of the basic concepts of both computability and computational complexity theory (Odifreddi 1989; Sipser 1996). Roughly speaking, a reduction of a problem \(D_i\) to a problem \(D_j\) comes down to providing an effective procedure for translating every instance \(d_{i,m}\) of the problem \(D_i\) to an instance \(d_{j,n}\) of \(D_j\) in such a way that an effective procedure for solving \(d_{j,n}\) also yields an effective procedure for solving \(d_{i,m}\). In other words, if \(D_i\) reduces to \(D_j\) then, if \(D_i\) is uncomputable so is \(D_j\). Note that the reduction of one problem to another can also be used in decidability proofs: if \(D_i\) reduces to \(D_j\) and \(D_j\) is known to be computable then so is \(D_i\).
In the absence of D \(_{\textrm{uncomp}}\) a very different approach was required and Church, Post and Turing each used more or less the same approach to this end (Gandy 1988). First of all, one needs a formalism which captures the notion of computability. Turing proposed the Turing machine formalism to this end. A second step is to show that there are problems that are not computable within the formalism. To achieve this, a uniform process U needs to be set-up relative to the formalism which is able to compute every computable number. One can then use (some form of) diagonalization in combination with U to derive a contradiction. Diagonalization was introduced by Cantor to show that the set of real numbers is “uncountable” or not denumerable. A variant of the method was used also by Gödel in the proof of his first incompleteness theorem .
Recall that in Turing’s original version of the Turing machine, the machines are computing real numbers. This implied that a “well-behaving” Turing machine should in fact never halt and print out an infinite sequence of figures. Such machines were identified by Turing as circle-free . All other machines are called circular machines . A number n which is the D.N. of a circle-free machine is called satisfactory .
This basic difference is used in Turing’s proof of the uncomputability of:
CIRC? The problem to decide for every number n whether or not it is satisfactory
The proof of the uncomputability of CIRC? uses the construction of a hypothetical and circle-free machine \(T_{decide}\) which computes the diagonal sequence of the set of all computable numbers computed by the circle-free machines. Hence, it relies for its construction on the universal Turing machine and a hypothetical machine that is able to decide CIRC? for each number n given to it. It is shown that the machine \(T_{decide}\) becomes a circular machine when it is provided with its own description number, hence the assumption of a machine which is capable of solving CIRC? must be false.
Based on the uncomputability of CIRC? , Turing then shows that also PRINT? is not computable. More particularly he shows that if PRINT? were to be computable, also CIRC? would be decidable, viz. he rephrases PRINT? in such a way that it becomes the problem to decide for any machine whether or not it will print an infinity of symbols which would amount to deciding CIRC? .
Finally, based on the uncomputability of PRINT? Turing shows that the Entscheidungsproblem is not decidable. This is achieved by showing:
- how for each Turing machine T , it is possible to construct a corresponding formula T in first-order logic and
- if there is a general method for determining whether T is provable, then there is a general method for proving that T will ever print 0. This is the problem PRINT? and so cannot be decidable (provided we accept Turing’s thesis).
It thus follows from the uncomputability of PRINT? , that the Entscheidungsproblem is not computable.
Given Turing’s focus on computable real numbers, his base decision problem is about determining whether or not some Turing machine will not halt and so is not quite the same as the more well-known halting problem:
- HALT? The problem to decide for every Turing machine T whether or not T will halt.
Turing’s problem PRINT? is in fact very close to HALT? (see Davis 1958: Chapter 5, Theorem 2.3).
A popular proof of HALT? goes as follows. Assume that HALT? is computable. Then it should be possible to construct a Turing machine which decides, for each machine \(T_i\) and some input w for \(T_i\) whether or not \(T_i\) will halt on w . Let us call this machine \(T_{H}\). More particularly, we have:
We now define a second machine \(T_D\) which relies on the assumption that the machine \(T_H\) can be constructed. More particularly, we have:
If we now set \(T_i\) to \(T_D\) we end up with a contradiction: if \(T_D\) halts it means that \(T_D\) does not halt and vice versa. A popular but quite informal variant of this proof was given by Christopher Strachey in the context of programming (Strachey 1965).
As is clear from Sections 1.1 and 1.2 , there is a variety of definitions of the Turing machine. One can use a quintuple or quadruple notation; one can have different types of symbols or just one; one can have a two-way infinite or a one-way infinite tape; etc. Several other less obvious modifications have been considered and used in the past. These modifications can be of two kinds: generalizations or restrictions. These do not result in “stronger” or “weaker” models. Viz. these modified machines compute no more and no less than the Turing computable functions. This adds to the robustness of the Turing machine definition.
Binary machines
In his short 1936 note Post considers machines that either mark or unmark a square which means we have only two symbols \(S_0\) and \(S_1\) but he did not prove that this formulation captures exactly the Turing computable functions. It was Shannon who proved that for any Turing machine T with n symbols there is a Turing machine with two symbols that simulates T (Shannon 1956). He also showed that for any Turing machine with m states, there is a Turing machine with only two states that simulates it.
Non-erasing machines
Non-erasing machines are machines that can only overprint \(S_0\). In Moore 1952, it was mentioned that Shannon proved that non-erasing machines can compute what any Turing machine computes. This result was given in a context of actual digital computers of the 50s which relied on punched tape (and so, for which, one cannot erase). Shannon’s result however remained unpublished. It was Wang who published the result (Wang 1957).
Non-writing machines
It was shown by Minsky that for every Turing machine there is a non-writing Turing machine with two tapes that simulates it.
Multiple tapes
Instead of one tape one can consider a Turing machine with multiple tapes. This turned out the be very useful in several different contexts. For instance, Minsky, used two-tape non-writing Turing machines to prove that a certain decision problem defined by Post (the decision problem for tag systems) is non-Turing computable (Minsky 1961). Hartmanis and Stearns then, in their founding paper for computational complexity theory, proved that any n -tape Turing machine reduces to a single tape Turing machine and so anything that can be computed by an n -tape or multitape Turing machine can also be computed by a single tape Turing machine, and conversely (Hartmanis & Stearns 1965). They used multitape machines because they were considered to be closer to actual digital computers.
n -dimensional Turing machines
Another variant is to consider Turing machines where the tape is not one-dimensional but n -dimensional. This variant too reduces to the one-dimensional variant.
Non-deterministic machines
An apparently more radical reformulation of the notion of Turing machine is that of non-deterministic Turing machines. As explained in 1.1 , one fundamental condition of Turing’s machines is the so-called determinacy condition, viz. the idea that at any given moment, the machine’s behavior is completely determined by the configuration or state it is in and the symbol it is scanning. Next to these, Turing also mentions the idea of choice machines for which the next state is not completely determined by the state and symbol pair. Instead, some external device makes a random choice of what to do next. Non-deterministic Turing machines are a kind of choice machines: for each state and symbol pair, the non-deterministic machine makes an arbitrary choice between a finite (possibly zero) number of states. Thus, unlike the computation of a deterministic Turing machine, the computation of a non-deterministic machine is a tree of possible configuration paths. One way to visualize the computation of a non-deterministic Turing machine is that the machine spawns an exact copy of itself and the tape for each alternative available transition, and each machine continues the computation. If any of the machines terminates successfully, then the entire computation terminates and inherits that machine’s resulting tape. Notice the word successfully in the preceding sentence. In this formulation, some states are designated as accepting states and when the machine terminates in one of these states, then the computation is successful, otherwise the computation is unsuccessful and any other machines continue in their search for a successful outcome. The addition of non-determinism to Turing machines does not alter the extent of Turing-computability. Non-determinism was introduced for finite automata in the paper, Rabin & Scott 1959, where it is also shown that adding non-determinism does not result in more powerful automata. Non-deterministic Turing machines are an important model in the context of computational complexity theory .

Weak and semi-weak machines
Weak Turing machines are machines where some word over the alphabet is repeated infinitely often to the left and right of the input. Semi-weak machines are machines where some word is repeated infinitely often either to the left or right of the input. These machines are generalizations of the standard model in which the initial tape contains some finite word (possibly nil). They were introduced to determine smaller universal machines. Watanabe was the first to define a universal semi-weak machine with six states and five symbols (Watanabe 1961). Recently, a number of researchers have determined several small weak and semi-weak universal Turing machines (e.g., Woods & Neary 2007; Cook 2004)
Besides these variants on the Turing machine model, there are also variants that result in models which capture, in some well-defined sense, more than the (Turing)-computable functions. Examples of such models are oracle machines (Turing 1939), infinite-time Turing machines (Hamkins & Lewis 2008) and accelerating Turing machines (Copeland 2002). There are various reasons for introducing such stronger models. Some are well-known models of computability or recursion theory and are used in the theory of higher-order recursion and relative computability (oracle machines); others, like the accelerating machines, were introduced in the context of supertasks and the idea of providing physical models that “compute” functions which are not Turing-computable.
3. Philosophical Issues Related to Turing Machines
In its original context, Turing’s identification between the computable numbers and Turing machines was aimed at proving that the Entscheidungsproblem is not a computable problem and so not a so-called “general process” problem (Turing 1936–7: 248). The basic assumption to be made for this result is that our “intuitive” notion of computability can be formally defined as Turing computability and so that there are no “computable” problems that are not Turing computable. But what was Turing’s “intuitive” notion of computability and how can we be sure that it really covers all computable problems, and, more generally, all kinds of computations? This is a very basic question in the philosophy of computer science .
At the time Turing was writing his paper, the modern computer was not developed yet and so rephrasings of Turing’s thesis which identify Turing computability with computability by a modern computer are interpretations rather than historically correct statements of Turing’s thesis. The existing computing machines at the time Turing wrote his paper, such as the differential analyzer or desk calculators, were quite restricted in what they could compute and were used in a context of human computational practices (Grier 2007). It is thus not surprising that Turing did not attempt to formalize machine computation but rather human computation and so computable problems in Turing’s paper become computable by human means. This is very explicit in Section 9 of Turing 1936–7 where he shows that Turing machines are a ‘natural’ model of (human) computation by analyzing the process of human computation. The analysis results in a kind of abstract human ‘computor’ who fulfills a set of different conditions that are rooted in Turing’s recognition of a set of human limitations which restrict what we can compute (of our sensory apparatus but also of our mental apparatus). This ‘computor’ computes (real) numbers on an infinite one-dimensional tape divided into squares [Note: Turing assumed that the reduction of the 2-dimensional character of the paper a human mathematician usually works on “is not essential of computation” (Turing 1936–7: 249)]. It has the following restrictions (Gandy 1988; Sieg 1994):
- Determinacy condition D “The behaviour of the computer at any moment is determined by the symbols which he is observing and his ‘state of mind’ at that moment.” (Turing 1936–7: 250)
- Boundedness condition B1 “there is a bound B to the number of symbols or squares which the computer can observe at one moment. If he wishes to observe more, he must use successive observations.” (Turing 1936–7: 250)
- Boundedness condition B2 “the number of states of mind which need be taken into account is finite” (Turing 1936–7: 250)
- Locality condition L1 “We may […] assume that the squares whose symbols are changed are always ‘observed’ squares.” (Turing 1936–7: 250)
- Locality condition L2 “each of the new observed squares is within L squares of an immediately previously observed square.” (Turing 1936–7: 250)
It is this so-called “direct appeal to intuition” (1936–7: 249) of Turing’s analysis and resulting model that explain why the Turing machine is today considered by many as the best standard model of computability (for a strong statement of this point of view, see Soare 1996). Indeed, from the above set of conditions one can quite easily derive Turing’s machines. This is achieved basically by analyzing the restrictive conditions into “‘simple operations’ which are so elementary that it is not easy to imagine them further divided” (Turing 1936–7: 250).
Note that while Turing’s analysis focuses on human computation, the application of his identification between (human) computation and Turing machine computation to the Entscheidungsproblem suggests that he did not consider the possibility of a model of computation that somehow goes “beyond” human computation and is capable of providing an effective and general procedure which solves the Entscheidungsproblem. If that would have been the case, he would not have considered the Entscheidungsproblem to be uncomputable.
The focus on human computation in Turing’s analysis of computation, has led researchers to extend Turing’s analysis to computation by physical devices. This results in (versions of) the physical Church-Turing thesis. Robin Gandy focused on extending Turing’s analysis to discrete mechanical devices (note that he did not consider analog machines). More particularly, like Turing, Gandy starts from a basic set of restrictions of computation by discrete mechanical devices and, on that basis, develops a new model which he proved to be reducible to the Turing machine model. This work is continued by Wilfried Sieg who proposed the framework of Computable Dynamical Systems (Sieg 2008). Others have considered the possibility of “reasonable” models from physics which “compute” something that is not Turing computable. See for instance Aaronson, Bavarian, & Gueltrini 2016 (Other Internet Resources) in which it is shown that if closed timelike curves would exist, the halting problem would become solvable with finite resources. Others have proposed alternative models for computation which are inspired by the Turing machine model but capture specific aspects of current computing practices for which the Turing machine model is considered less suited. One example here are the persistent Turing machines intended to capture interactive processes. Note however that these results do not show that there are “computable” problems that are not Turing computable. These and other related proposals have been considered by some authors as reasonable models of computation that somehow compute more than Turing machines. It is the latter kind of statements that became affiliated with research on so-called hypercomputation resulting in the early 2000s in a rather fierce debate in the computer science community, see, e.g., Teuscher 2004 for various positions.
As is clear, strictly speaking, Turing’s thesis is not provable, since, in its original form, it is a claim about the relationship between a formal and a vague or intuitive concept. By consequence, many consider it as a thesis or a definition. The thesis would be refuted if one would be able to provide an intuitively acceptable effective procedure for a task that is not Turing-computable. This far, no such counterexample has been found. Other independently defined notions of computability based on alternative foundations, such as recursive functions and abacus machines have also been shown to be equivalent to Turing computability. These equivalences between quite different formulations indicate that there is a natural and robust notion of computability underlying our understanding. Given this apparent robustness of our notion of computability, some have proposed to avoid the notion of a thesis altogether and instead propose a set of axioms used to sharpen the informal notion. There are several approaches, most notably, an approach of structural axiomatization where computability itself is axiomatized (Sieg 2008) and one whereby an axiomatization is given from which the Church-Turing thesis can be derived (Dershowitz & Gurevich 2008).
4. Alternative Historical Models of Computability
Besides the Turing machine, several other models were introduced independently of Turing in the context of research into the foundation of mathematics which resulted in theses that are logically equivalent to Turing’s thesis. For each of these models it was proven that they capture the Turing computable functions. Note that the development of the modern computer stimulated the development of other models such as register machines or Markov algorithms. More recently, computational approaches in disciplines such as biology or physics, resulted in bio-inspired and physics-inspired models such as Petri nets or quantum Turing machines. A discussion of such models, however, lies beyond the scope of this entry.
The original formulation of general recursive functions can be found in Gödel 1934, which built on a suggestion by Herbrand. In Kleene 1936 a simpler definition was given and in Kleene 1943 the standard form which uses the so-called minimization or \(\mu\)-operator was introduced. For more information, see the entry on recursive functions .
Church used the definition of general recursive functions to state his thesis:
Church’s thesis Every effectively calculable function is general recursive
In the context of recursive function one uses the notion of recursive solvability and unsolvability rather than Turing computability and uncomputability. This terminology is due to Post (1944).
Church’s λ-calculus has its origin in the papers (Church 1932, 1933) and which were intended as a logical foundation for mathematics. It was Church’s conviction at that time that this different formal approach might avoid Gödel incompleteness (Sieg 1997: 177). However, the logical system proposed by Church was proven inconsistent by his two PhD students Stephen C. Kleene and Barkley Rosser and so they started to focus on a subpart of that logic which was basically the λ-calculus. Church, Kleene and Rosser started to λ-define any calculable function they could think of and quite soon Church proposed to define effective calculability in terms of λ-definability. However, it was only after Church, Kleene and Rosser had established that general recursiveness and λ-definability are equivalent that Church announced his thesis publicly and in terms of general recursive functions rather than λ-definability (Davis 1982; Sieg 1997).
In λ-calculus there are only two types of symbols. The three primitive symbols λ, (, ) also called the improper symbols, and an infinite list of variables. There are three rules to define the well-formed formulas of λ-calculus, called λ-formulas.
- The λ-formulas are first of all the variables themselves.
- If P is a λ-formula containing x as a free variable then \(\lambda x[\textbf{P}]\) is also a λ-formula. The λ-operator is used to bind variables and it thus converts an expression containing free variables into one that denotes a function
- If M and N are λ-formulas then so is { M }( N ), where { M }( N ) is to be understood as the application of the function M to N .
The λ-formulas, or well-formed formulas of λ-calculus are all and only those formulas that result from (repeated) application of these three rules.
There are three operations or rules of conversion. Let us define \(\textrm{S}_{\mathbf{N}}^{x}\mathbf{M}|\) as standing for the formula that results by substitution of N for x in M .
- Reduction . To replace any part \(\{\lambda x \mathbf{[M]}\} (\mathbf{N})\) of a formula by \(\textrm{S}_{\mathbf{N}}^{x}\mathbf{M}|\) provided that the bound variables of M are distinct both from x and from the free variables of N . For example \(\{\lambda x [x^{2}]\}(2)\) reduces to \(2^{2}\)
- Expansion To replace any part \(\textrm{S}_{\mathbf{N}}^{x}\mathbf{M}|\) of a formula by \(\{\lambda x \mathbf{[M]}\} (\mathbf{N})\) provided that \(((\lambda x \mathbf{M}) \mathbf{N})\) is well-formed and the bound variables of M are distinct both from x and from the free variables in N . For example, \(2^{2}\) can be expanded to \(\{\lambda x [x^{2}]\}(2)\)
- Change of bound variable To replace any part M of a formula by \(\textrm{S}_{\textrm{y}}^{x}\mathbf{M}|\) provided that x is not a free variable of M and y does not occur in M . For example changing \(\{\lambda x [x^{2}]\}\) to \(\{\lambda y [y^{2}]\}\)
Church introduces the following abbreviations to define the natural numbers in λ-calculus:
Using this definition, it is possible to λ -define functions over the positive integers. A function F of one positive integer is λ-definable if we can find a λ-formula F , such that if \(F(m) = n\) and m and n are λ-formulas standing for the integers m and n , then the λ-formula \(\{\mathbf{F}\} (\mathbf{m})\) can be converted to n by applying the conversion rules of λ-calculus. Thus, for example, the successor function S , first introduced by Church, can be λ-defined as follows:
To give an example, applying S to the λ-formula standing for 2, we get:
Today, λ-calculus is considered to be a basic model in the theory of programming.
Around 1920–21 Emil Post developed different but related types of production systems in order to develop a syntactical form which would allow him to tackle the decision problem for first-order logic. One of these forms are Post canonical systems C which became later known as Post production systems.
A canonical system consists of a finite alphabet \(\Sigma\), a finite set of initial words \(W_{0,0}\), \(W_{0,1}\),…, \(W_{0,n}\) and a finite set of production rules of the following form:
The symbols g are a kind of metasymbols: they correspond to actual sequences of letters in actual productions. The symbols P are the operational variables and so can represent any sequence of letters in a production. So, for instance, consider a production system over the alphabet \(\Sigma = \{a,b\}\) with initial word:
and the following production rule:
Then, starting with \(W_0\), there are three possible ways to apply the production rule and in each application the variables \(P_{1,i}\) will have different values but the values of the g’s are fixed. Any set of finite sequences of words that can be produced by a canonical system is called a canonical set .
A special class of canonical forms defined by Post are normal systems. A normal system N consists of a finite alphabet \(\Sigma\), one initial word \(W_0 \in \Sigma^{\ast}\) and a finite set of production rules, each of the following form:
Any set of finite sequences of words that can be produced by a normal system is called a normal set . Post was able to show that for any canonical set C over some alphabet \(\Sigma\) there is a normal set N over an alphabet \(\Delta\) with \(\Sigma \subseteq \Delta\) such that \(C = N \cap \Sigma^{\ast}\). It was his conviction that (1) any set of finite sequences that can be generated by finite means can be generated by canonical systems and (2) the proof that for every canonical set there is a normal set which contains it, which resulted in Post’s thesis I:
Post’s thesis I (Davis 1982) Every set of finite sequences of letters that can be generated by finite processes can also be generated by normal systems. More particularly, any set of words on an alphabet \(\Sigma\) which can be generated by a finite process is of the form \(N \cap \Sigma^{\ast}\), with N a normal set.
Post realized that “[for the thesis to obtain its full generality] a complete analysis would have to be made of all the possible ways in which the human mind could set up finite processes for generating sequences” (Post 1965: 408) and it is quite probable that the formulation 1 given in Post 1936 and which is almost identical to Turing’s machines is the result of such an analysis.
Post production systems became important formal devices in computer science and, more particularly, formal language theory (Davis 1989; Pullum 2011).
In 1936 Post published a short note from which one can derive Post’s second thesis (De Mol 2013):
Post’s thesis II Solvability of a problem in the intuitive sense coincides with solvability by formulation 1
Formulation 1 is very similar to Turing machines but the ‘program’ is given as a list of directions which a human worker needs to follow. Instead of a one-way infinite tape, Post’s ‘machine’ consists of a two-way infinite symbol space divided into boxes. The idea is that a worker is working in this symbol space, being capable of a set of five primitive acts (\(O_{1}\) mark a box, \(O_{2}\) unmark a box, \(O_{3}\) move one box to the left, \(O_{4}\) move one box to the right, \(O_{5}\) determining whether the box he is in is marked or unmarked), following a finite set of directions \(d_{1}\),…, \(d_{n}\) where each direction \(d_{i}\) always has one of the following forms:
- Perform one of the operations (\(O_{1}\)–\(O_4\)) and go to instruction \(d_{j}\)
- Perform operation \(O_{5}\) and according as the box the worker is in is marked or unmarked follow direction \(d_{j'}\) or \(d_{j''}\).
Post also defined a specific terminology for his formulation 1 in order to define the solvability of a problem in terms of formulation 1. These notions are applicability, finite-1-process, 1-solution and 1-given. Roughly speaking these notions assure that a decision problem is solvable with formulation 1 on the condition that the solution given in the formalism always terminates with a correct solution.
5. Impact of Turing Machines on Computer Science
Turing is today one of the most celebrated figures of computer science. Many consider him as the father of computer science and the fact that the main award in the computer science community is called the Turing award is a clear indication of that (Daylight 2015). This was strengthened by the Turing centenary celebrations from 2012, which were largely coordinated by S. Barry Cooper. This resulted not only in an enormous number of scientific events around Turing but also a number of initiatives that brought the idea of Turing as the father of computer science also to the broader public (Bullynck, Daylight, & De Mol 2015). Amongst Turing’s contributions which are today considered as pioneering, the 1936 paper on Turing machines stands out as the one which has the largest impact on computer science. However, recent historical research shows also that one should treat the impact of Turing machines with great care and that one should be careful in retrofitting the past into the present.
Today, the Turing machine and its theory are part of the theoretical foundations of computer science. It is a standard reference in research on foundational questions such as:
- What is an algorithm?
- What is a computation?
- What is a physical computation?
- What is an efficient computation?
It is also one of the main models for research into a broad range of subdisciplines in theoretical computer science such as: variant and minimal models of computability, higher-order computability, computational complexity theory , algorithmic information theory, etc. This significance of the Turing machine model for theoretical computer science has at least two historical roots.
First of all, there is the continuation of the work in mathematical logic from the 1920s and 1930s by people like Martin Davis—who is a student of Post and Church—and Kleene. Within that tradition, Turing’s work was of course well-known and the Turing machine was considered as the best model of computability given. Both Davis and Kleene published a book in the 1950s on these topics (Kleene 1952; Davis 1958) which soon became standard references not just for early computability theory but also for more theoretical reflections in the late 1950s and 1960s on computing.
Secondly, one sees that in the 1950s there is a need for theoretical models to reflect on the new computing machines, their abilities and limitations and this in a more systematic manner. It is in that context that the theoretical work already done was picked up. One important development is automata theory in which one can situate, amongst others, the development of other machine models like the register machine model or the Wang B machine model which are, ultimately, rooted in Turing’s and Post’s machines; there are the minimal machine designs discussed in Section 5.2 ; and there is the use of Turing machines in the context of what would become the origins of formal language theory, viz the study of different classes of machines with respect to the different “languages” they can recognize and so also their limitations and strengths. It are these more theoretical developments that contributed to the establishment of computational complexity theory in the 1960s. Of course, besides Turing machines, other models also played and play an important role in these developments. Still, within theoretical computer science it is mostly the Turing machine which remains the model, even today. Indeed, when in 1965 one of the founding papers of computational complexity theory (Hartmanis & Stearns 1965) is published, it is the multitape Turing machine which is introduced as the standard model for the computer.
In several accounts, Turing has been identified not just as the father of computer science but as the father of the modern computer. The classical story for this more or less goes as follows: the blueprint of the modern computer can be found in von Neumann’s EDVAC design and today classical computers are usually described as having a so-called von Neumann architecture. One fundamental idea of the EDVAC design is the so-called stored-program idea. Roughly speaking this means the storage of instructions and data in the same memory allowing the manipulation of programs as data. There are good reasons for assuming that von Neumann knew the main results of Turing’s paper (Davis 1988). Thus, one could argue that the stored-program concept originates in Turing’s notion of the universal Turing machine and, singling this out as the defining feature of the modern computer, some might claim that Turing is the father of the modern computer. Another related argument is that Turing was the first who “captured” the idea of a general-purpose machine through his notion of the universal machine and that in this sense he also “invented” the modern computer (Copeland & Proudfoot 2011). This argument is then strengthened by the fact that Turing was also involved with the construction of an important class of computing devices (the Bombe) used for decrypting the German Enigma code and later proposed the design of the ACE (Automatic Computing Engine) which was explicitly identified as a kind of physical realization of the universal machine by Turing himself:
Some years ago I was researching on what might now be described as an investigation of the theoretical possibilities and limitations of digital computing machines. […] Machines such as the ACE may be regarded as practical versions of this same type of machine. (Turing 1947)
Note however that Turing already knew the ENIAC and EDVAC designs and proposed the ACE as a kind of improvement on that design (amongst others, it had a simpler hardware architecture).
These claims about Turing as the inventor and/or father of the computer have been scrutinized by some historians of computing (Daylight 2014; Haigh 2013; Haigh 2014; Mounier-Kuhn 2012), mostly in the wake of the Turing centenary and this from several perspectives. Based on that research it is clear that claims about Turing being the inventor of the modern computer give a distorted and biased picture of the development of the modern computer. At best, he is one of the many who made a contribution to one of the several historical developments (scientific, political, technological, social and industrial) which resulted, ultimately, in (our concept of) the modern computer. Indeed, the “first” computers are the result of a wide number of innovations and so are rooted in the work of not just one but several people with diverse backgrounds and viewpoints.
In the 1950s then the (universal) Turing machine starts to become an accepted model in relation to actual computers and is used as a tool to reflect on the limits and potentials of general-purpose computers by both engineers, mathematicians and logicians. More particularly, with respect to machine designs, it was the insight that only a few number of operations were required to built a general-purpose machine which inspired in the 1950s reflections on minimal machine architectures. Frankel, who (partially) constructed the MINAC stated this as follows:
One remarkable result of Turing’s investigation is that he was able to describe a single computer which is able to compute any computable number. He called this machine a universal computer . It is thus the “best possible” computer mentioned. […] This surprising result shows that in examining the question of what problems are, in principle, solvable by computing machines, we do not need to consider an infinite series of computers of greater and greater complexity but may think only of a single machine. Even more surprising than the theoretical possibility of such a “best possible” computer is the fact that it need not be very complex. The description given by Turing of a universal computer is not unique. Many computers, some of quite modest complexity, satisfy the requirements for a universal computer. (Frankel 1956: 635)
The result was a series of experimental machines such as the MINAC, TX-0 (Lincoln Lab) or the ZERO machine (van der Poel) which in their turn became predecessors of a number of commercial machines. It is worth pointing out that also Turing’s ACE machine design fits into this philosophy. It was also commercialized as the BENDIX G15 machine (De Mol, Bullynck, & Daylight 2018).
Of course, by minimizing the machine instructions, coding or programming became a much more complicated task. To put it in Turing’s words who clearly realized this trade-off between code and (hard-wired) instructions when designing the ACE: “[W]e have often simplified the circuit at the expense of the code” (Turing 1947). And indeed, one sees that with these early minimal designs, much effort goes into developing more efficient coding strategies. It is here that one can also situate one historical root of making the connection between the universal Turing machine and the important principle of the interchangeability between hardware and programs.
Today, the universal Turing machine is by many still considered as the main theoretical model of the modern computer especially in relation to the so-called von Neumann architecture. Of course, other models have been introduced for other architectures such as the Bulk synchronous parallel model for parallel machines or the persistent Turing machine for modeling interactive problems.
The idea that any general-purpose machine can, in principle, be modeled as a universal Turing machine also became an important principle in the context of automatic programming in the 1950s (Daylight 2015). In the machine design context it was the minimizing of the machine instructions that was the most important consequence of that viewpoint. In the programming context then it was about the idea that one can built a machine that is able to ‘mimic’’ the behavior of any other machine and so, ultimately, the interchangeability between machine hardware and language implementations. This is introduced in several forms in the 1950s by people like John W. Carr III and Saul Gorn—who were also actively involved in the shaping of the Association for Computing Machinery (ACM) —as the unifying theoretical idea for automatic programming which indeed is about the (automatic) “translation” of higher-order to lower-level, and, ultimately, machine code. Thus, also in the context of programming, the universal Turing machine starts to take on its foundational role in the 1950s (Daylight 2015).
Whereas the Turing machine is and was a fundamental theoretical model delimiting what is possible and not on the general level, it did not have a real impact on the syntax and semantics of programming languages. In that context it were rather λ-calculus and Post production systems that had an effect (though also here one should be careful in overstating the influence of a formal model on a programming practice). In fact, Turing machines were often regarded as machine models rather than as a model for programming:
Turing machines are not conceptually different from the automatic computers in general use, but they are very poor in their control structure. […] Of course, most of the theory of computability deals with questions which are not concerned with the particular ways computations are represented. It is sufficient that computable functions be represented somehow by symbolic expressions, e.g., numbers, and that functions computable in terms of given functions be somehow represented by expressions computable in terms of the expressions representing the original functions. However, a practical theory of computation must be applicable to particular algorithms. (McCarthy 1963: 37)
Thus one sees that the role of the Turing machine for computer science should be situated rather on the theoretical level: the universal machine is today by many still considered as the model for the modern computer while its ability to mimic machines through its manipulation of programs-as-data is one of the basic principles of modern computing. Moreover, its robustness and naturalness as a model of computability have made it the main model to challenge if one is attacking versions of the so-called (physical) Church-Turing thesis.
- Barwise, Jon and John Etchemendy, 1993, Turing’s World , Stanford, CA: CSLI Publications.
- Boolos, George S. and Richard C. Jeffrey, 1974, Computability and Logic , Cambridge: Cambridge University Press; fifth edition 2007. doi:10.1017/CBO9780511804076 (fifth edition)
- Bromley, Allan G., 1985, “Stored Program Concept. The Origin of the Stored Program Concept”, Technical Report 274, Basser Department of Computer Science, November 1985. [ Bromley 1985 available online ]
- Bullynck, Maarten, Edgar G. Daylight, and Liesbeth De Mol, 2015, “Why Did Computer Science Make a Hero Out of Turing?”, Communications of the ACM , 58(3): 37–39.doi:10.1145/2658985
- Church, Alonzo, 1932, “A Set of Postulates for the Foundation of Logic”, Annals of Mathematics , 33(2): 346–366. doi:10.2307/1968337
- –––, 1933, “A Set of Postulates for the Foundation of Logic (Second Paper)”, Annals of Mathematics , 34(4): 839–864. doi:10.2307/1968702
- –––, 1936a, “An Unsolvable Problem of Elementary Number Theory”, American Journal of Mathematics , 58(2): 345–363.
- –––, 1936b, “A Note on the Entscheidungsproblem”, Journal of Symbolic Logic , 1(1): 40–41. doi:10.2307/2269326
- –––, 1937, “Review of: On Computable Numbers with An Application to the Entscheidungsproblem by A.M. Turing”, Journal of Symbolic Logic , 2(1): 42–43. doi:10.1017/S002248120003958X
- Cook, Matthew, 2004, “Universality in Elementary Cellular Automata”, Complex Systems , 15(1): 1–40.
- Cooper, S. Barry and Jan Van Leeuwen, 2013, Alan Turing: His Work and Impact , Amsterdam: Elsevier. doi:10.1016/C2010-0-66380-2
- Copeland, B. Jack, 2002, “Accelerating Turing Machines”, Minds and Machines , 12(2): 281–301. doi:10.1023/A:1015607401307
- Copeland, B. Jack and Diane Proudfoot, 2011, “Alan Turing: Father of the Modern Computer”, The Rutherford Journal , 4: 1. [ Copeland & Proudfoot 2011 available online ]
- Davis, Martin, 1958 [1982], Computability and Unsolvability , New York: McGraw-Hill. Reprinted Dover, 1982.
- –––, 1965, The Undecidable. Basic papers on undecidable propositions, unsolvable problems and computable functions , New York: Raven Press.
- –––, 1978, “What is a Computation?”, Lynn Arthur Steen (ed.), Mathematics Today: Twelve Informal Essays , New York: Springer, pp. 241–267. doi:10.1007/978-1-4613-9435-8_10
- –––, 1982, “Why Gödel Didn’t Have Church’s Thesis”, Information and Control , 54:(1–2): 3–24. doi:10.1016/S0019-9958(82)91226-8
- –––, 1988, “Mathematical Logic and the Origin of the Modern Computer”, in Herken 1988: 149–174.
- –––, 1989, “Emil Post’s Contribution to Computer Science”, Proceedings of the Fourth Annual Symposium on Logic in Computer Science , IEEE Computer Society Press, pp. 134–137. doi:10.1109/LICS.1989.39167
- Davis, Martin and Wilfried Sieg, 2015, “Conceptual Confluence in 1936: Post and Turing”, in Giovanni Sommaruga and Thomas Strahm (eds.), Turing’s Revolution: The Impact of His Ideas about Computability , Cham: Springer. doi:10.1007/978-3-319-22156-4_1
- Daylight, Edgar G., 2014, “A Turing Tale”, Communications of the ACM , 57(10): 36–38. doi:10.1145/2629499
- –––, 2015, “Towards a Historical Notion of ‘Turing—The Father of Computer Science’”, History and Philosophy of Logic , . 36(3): 205–228. doi:10.1080/01445340.2015.1082050
- De Mol, Liesbeth, 2013, “Generating, Solving and the Mathematics of Homo Sapiens. Emil Post’s Views On computation”, Hector Zenil (ed.), A Computable Universe. Understanding Computation & Exploring Nature As Computation , Hackensack, NJ: World Scientific, pp. 45–62. doi:10.1142/9789814374309_0003 [ De Mol 2013 available online ]
- De Mol, Liesbeth, Maarten Bullynck, and Edgar G. Daylight, 2018, “Less is More in the Fifties: Encounters between Logical Minimalism and Computer Design during the 1950s”, IEEE Annals of the History of Computing , 40(1): 19–45. doi:10.1109/MAHC.2018.012171265 [ De Mol et al. 2018 available online ]
- Deutsch, D., 1985, “Quantum Theory, the Church-Turing Principle and the Universal Quantum Computer”, Proceedings of the Royal Society A , 400(1818): 97–117. doi:10.1098/rspa.1985.0070
- Dershowitz, Nachum and Yuri Gurevich, 2008, “ A Natural Axiomatization of Computability and Proof of Church’s Thesis”, Bulletin of Symbolic Logic , 14(3): 299–350.
- Frankel, Stanley, 1956, “Useful Applications of a Magnetic-Drum Computer”, Electrical Engineering , 75(7): 634–39, doi:10.1109/EE.1956.6442018
- Gandy, Robin, 1980, “Church’s Thesis and Principles for Mechanism”, in Jon Barwise, H. Jerome Keisler, and Kenneth Kunen (eds.), The Kleene Symposium: Proceedings of the Symposium Held June 18–24, 1978 at Madison, Wisconsin, U.S.A. , (Studies in Logic and the Foundations of Mathematics, 101), Amsterdam: North-Holland, pp. 123–148. doi:10.1016/S0049-237X(08)71257-6
- –––, 1988, “The Confluence of Ideas in 1936”, in Herken 1988: 55–111.
- Gödel, Kurt, 1929, “Die Vollständigkeit der Axiome des logischen Funktionenkalkül”, Monatshefte für Mathematik und Physik , 37: 349–360. doi:10.1007/BF01696781
- –––, 1934, “On Undecidable Propositions of Formal Mathematical Systems”, mimeographed lecture notes by S. C. Kleene and J. B. Rosser, Institute for Advanced Study, Princeton, NJ; corrected and amplified in Davis 1965: 41–74.
- Grier, David Alan, 2007, When Computers Were Human , Princeton, NJ: Princeton University Press.
- Haigh, Thomas, 2013, “‘Stored Program Concept’ Considered Harmful: History and Historiography”, in Paola Bonizzoni, Vasco Brattka, and Benedikt Löwe, The Nature of Computation. Logic, Algorithms, Applications: 9th Conference on Computability in Europe, CiE 2013, Milan, Italy, July 1–5, 2013 Proceedings , (Lecture Notes in Computer Science, 7921), Berlin: Springer, pp. 241–251. doi:10.1007/978-3-642-39053-1_28
- –––, 2014, “Actually, Turing Did Not Invent the Computer”, Communications of the ACM , 57(1): 36–41. doi:10.1145/2542504
- Hamkins, Joel David and Andy Lewis, 2000, “Infinite Time Turing Machines”, Journal of Symbolic Logic , 65(2): 567–604. doi:10.2307/2586556
- Hartmanis, J. and R.E. Stearns, 1965, “On the Computational Complexity of Algorithms” Transactions of the American Mathematical Society , 117: 285–306. doi:10.1090/S0002-9947-1965-0170805-7
- Herken, Rolf, (ed.), 1988, The Universal Turing Machine: A Half-Century Survey , New York: Oxford University Press.
- Hilbert, David, 1930, “Naturerkennen und Logik”, Naturwissenschaften , 18(47–49): 959–963. doi:10.1007/BF01492194
- Hilbert, David and Wilhelm Ackermann, 1928, Grundzüge der Theoretischen Logik , Berlin: Springer. doi:10.1007/978-3-642-65400-8
- Hodges, Andrew, 1983, Alan Turing: The Enigma , New York: Simon and Schuster.
- Kleene, Stephen Cole, 1936, “General Recursive Functions of Natural Numbers”, Mathematische Annalen , 112: 727–742. doi:10.1007/BF01565439
- –––, 1943, “Recursive predicates and quantifiers”, Transactions of the American Mathematical Society , 53(1): 41–73. doi:10.2307/2267986
- –––, 1952, Introduction to Metamathematics , Amsterdam: North Holland.
- Lambek, Joachim, 1961, “How to Program an Infinite Abacus”, Canadian Mathematical Bulletin , 4: 295–302. doi:10.4153/CMB-1961-032-6
- Lewis, Henry R. and Christos H. Papadimitriou, 1981, Elements of the Theory of Computation , Englewood Cliffs, NJ: Prentice-Hall.
- Lin, Shen and Tibor Radó, 1965, “Computer Studies of Turing Machine Problems”, Journal of the Association for Computing Machinery , 12(2): 196–212. doi:10.1145/321264.321270
- Mancosu, Paolo, Richard Zach, and Calixto Badesa, 2009, “The Development of Mathematical Logic from Russell to Tarski, 1900–1935”, in Leila Haaparanta (ed.), The Development of Modern Logic , New York: Oxford University Press, pp. 318–470. doi:10.1093/acprof:oso/9780195137316.003.0029 [ Mancosu et al. 2009 available online ]
- Margenstern, Maurice, 2000, “Frontier Between Decidability and Undecidability: A Survey”, Theoretical Computer Science , 231(2): 217–251. doi:10.1016/S0304-3975(99)00102-4
- McCarthy, John, 1963, “A Basis for a Mathematical Theory of Computation”, in: P. Braffort and D. Hirschberg, Computer Programming and Formal Systems , Amsterdam: North-Holland, pp. 33–70. [ McCarthy 1963 available online ]
- Minsky, Marvin, 1961, “Recursive Unsolvability of Post's Problem of ‘Tag’ and other Topics in Theory of Turing Machines”, Annals of Mathematics , 74(3): 437–455. doi:10.2307/2269716
- –––, 1967, Computation: Finite and Infinite Machines , Englewood Cliffs, NJ: Prentice Hall.
- Moore, E.F., 1952, “A simplified universal Turing machine”, Proceedings of the Association of Computing Machinery (meetings at Toronto, Ontario), Washington, DC: Sauls Lithograph, 50–55. doi:10.1145/800259.808993
- Mounier-Kuhn, Pierre, 2012, “Logic and Computing in France: A Late Convergence”, in AISB/IACAP World Congress 2012: History and Philosophy of Programming , University of Birmingham, 2-6 July 2012. [ Mounier-Kuhn 2012 available online ]
- Odifreddi, P., 1989, Classical Recursion Theory , Amsterdam: Elsevier.
- Petzold, Charles, 2008, The Annotated Turing: A Guided Tour Through Alan Turing’s Historic Paper on Computability and Turing Machines , Indianapolis, IN: Wiley.
- Post, Emil L., 1936, “Finite Combinatory Processes-Formulation 1”, Journal of Symbolic Logic , 1(3): 103–105. doi:10.2307/2269031
- –––, 1944, “Recursively Enumerable Sets of Positive Integers and Their Decision Problems”, Bulletin of the American Mathematical Society , 50(5): 284–316. [ Post 1944 available online ]
- –––, 1947, “Recursive Unsolvability of a Problem of Thue”, Journal of Symbolic Logic , 12(1): 1–11. doi:10.2307/2267170
- –––, 1965, “Absolutely Unsolvable Problems and Relatively Undecidable Propositions—Account of an Anticipation”, in Martin Davis (ed.), The Undecidable: Basic Papers on Undecidable Propositions, Unsolvable Problems and Computable Functions , New York: Raven Press. Corrected republication 2004, Dover publications, New York, pp. 340–433.
- Pullum, Geoffrey K., 2011, “On the Mathematical Foundations of Syntactic Structures ”, Journal of Logic, Language and Information , 20(3): 277–296. doi:10.1007/s10849-011-9139-8
- Rabin, M.O. and D. Scott, 1959, “Finite Automata and their Decision Problems”, IBM Journal of Research and Development , 3(2): 114–125. doi:10.1147/rd.32.0114
- Radó, Tibor, 1962, “On Non-Computable Functions”, Bell System Technical Journal , 41(3/May): 877–884. doi:10.1002/j.1538-7305.1962.tb00480.x
- Shannon, Claude E., 1956, “A Universal Turing Machine with Two Internal States”, in Shannon & McCarthy 1956: 157–165. doi:10.1515/9781400882618-007
- Shannon, Claude E. and John McCarthy (eds), 1956, Automata Studies , (Annals of Mathematics Studies, 34), Princeton: Princeton University Press.
- Shapiro, Stewart, 2007, “Computability, Proof, and Open-Texture”, in Adam Olszewski, Jan Wolenski, and Robert Janusz (eds.), Church’s Thesis After 70 years , Berlin: Ontos Verlag, pp. 420–455. doi:10.1515/9783110325461.420
- Sieg, Wilfried, 1994, “Mechanical Procedures and Mathematical Experience”, in Alexander George (ed.), Mathematics and Mind , Oxford: Oxford University Press, pp. 71–117.
- –––, 1997, “Step by Recursive Step: Church’s Analysis of Effective Calculability”, The Bulletin of Symbolic Logic , 3(2): 154–180. doi:10.2307/421012
- –––, 2008, “Church without Dogma: Axioms for Computability”, in S. Barry Cooper, Benedikt Löwe, and Andrea Sorbi (eds.), New Computational Paradigms: Changing Conceptions of What is Computable , New York: Springer Verlag, pp. 139–152. doi:10.1007/978-0-387-68546-5_7
- Sipser, Michael, 1996, Introduction to the Theory of Computation , Boston: PWS Publishing.
- Soare, Robert I., 1996, “Computability and Recursion”, Bulletin for Symbolic Logic , 2(3): 284–321. doi:10.2307/420992
- Strachey, Christopher, 1965, “An Impossible Program (letter to the editor )”, The Computer Journal , 7(4): 313. doi:10.1093/comjnl/7.4.313
- Teuscher, Christof (ed.), 2004, Alan Turing: Life and Legacy of a Great Thinker , Berlin: Springer. doi:10.1007/978-3-662-05642-4
- Turing, A.M., 1936–7, “On Computable Numbers, With an Application to the Entscheidungsproblem”, Proceedings of the London Mathematical Society , s2-42: 230–265; correction ibid ., s2-43: 544–546 (1937). doi:10.1112/plms/s2-42.1.230 and doi:10.1112/plms/s2-43.6.544
- –––, 1937, “Computability and λ-Definability”, Journal of Symbolic Logic , 2(4): 153–163. doi:10.2307/2268280
- –––, 1939, “Systems of Logic Based on Ordinals”, Proceedings of the London Mathematical Society , s2-45: 161–228. doi:10.1112/plms/s2-45.1.161
- –––, 1947 [1986], “Lecture to the London Mathematical Society on 20 February 1947”, reprinted in A M. Turing's ACE Report of 1946 and Other Papers: Papers by Alan Turing and Michael Woodger , (Charles Babbage Institute Reprint, 10), B.E. Carpenter and R.W. Doran (eds.), Cambridge, MA: MIT Press, 1986.
- –––, 1954, “Solvable and Unsolvable Problems”, Science News , (February, Penguin), 31: 7–23.
- Wang, Hao, 1957, “A Variant to Turing’s Theory of Computing Machines”, Journal of the ACM , 4(1): 63–92. doi:10.1145/320856.320867
- Watanabe, Shigeru, 1961, “5-Symbol 8-State and 5-Symbol 6-State Universal Turing Machines”, Journal of the ACM , 8(4): 476–483. doi:10.1145/321088.321090
- Woods, Damien and Turlough Neary, 2007, “Small Semi-Weakly Universal Turing Machines”, in Jérôme Durand-Lose and Maurice Margenstern (eds.), Machines, Computations, and Universality: 5th International Conference, MCU 2007 Orléans, France, September 10–13, 2007 , (Lecture Notes in Computer Science, 4664), Berlin: Springer, pp. 303–315. doi:10.1007/978-3-540-74593-8_26
- –––, 2009, “The Complexity of Small Universal Turing Machines: A Survey”, Theoretical Computer Science , 410(4–5): 443–450. doi:10.1016/j.tcs.2008.09.051
How to cite this entry . Preview the PDF version of this entry at the Friends of the SEP Society . Look up topics and thinkers related to this entry at the Internet Philosophy Ontology Project (InPhO). Enhanced bibliography for this entry at PhilPapers , with links to its database.
Other Internet Resources
- “Turing Machines”, Stanford Encyclopedia of Philosophy (Fall 2018 Edition), Edward N. Zalta (ed.), URL = < http://plato.stanford.edu/archives/fall2018/entries/turing-machine/ >. [ This was the previous entry on Turing Machines in the SEP, written by David Barker-Plummer. ] .
- Aaronson, Scott, Mohammad Bavarian, and Giulio Gueltrini, 2016, “ Computability Theory of Closed Timelike Curves ”, manuscript available at arXiv.org.
- The Alan Turing Home Page , maintained by Andrew Hodges
- Bletchley Park , in the U.K., where, during the Second World War, Alan Turing was involved in code breaking activities at Station X.
- Michael Somos’ page of Busy Beaver references.
- Halting problem is solvable (funny)
Online Turing Machine Simulators
Turing machines are more powerful than any device that can actually be built, but they can be simulated both in software and hardware.
There are many Turing machine simulators available. Here are three software simulators that use different technologies to implement simulators using your browser.
- Andrew Hodges’ Turing Machine Simulator (for limited number of machines)
- Suzanne Britton’s Turing Machine Simulator (A Java Applet)
Here is an application that you can run on the desktop (no endorsement of these programs is implied).
- Visual Turing : freeware simulator for Windows 95/98/NT/2000
- Turing Machine in the Classic Style , Mike Davey’s physical Turing machine simulator.
- Lego of Doom , Turing machine simulator using Lego™.
- A purely mechanical Turing Machine , Computer Science Department, École Normal Supérieure de Lyon. (This one has no embedded microprocessor.)
Church-Turing Thesis | computability and complexity | computational complexity theory | recursive functions | Turing, Alan
Acknowledgments
The version of this entry published on September 24, 2018 is essentially a new entry, though the author would like to acknowledge the few sentences that remain from the previous version written by David Barker-Plummer. See also footnote 1 for an acknowledgment to S. Barry Cooper.
Copyright © 2018 by Liesbeth De Mol < liesbeth . demol @ univ-lille3 . fr >
- Accessibility
Support SEP
Mirror sites.
View this site from another server:
- Info about mirror sites
The Stanford Encyclopedia of Philosophy is copyright © 2023 by The Metaphysics Research Lab , Department of Philosophy, Stanford University
Library of Congress Catalog Data: ISSN 1095-5054
Physical Computability Theses
- First Online: 08 April 2020
Cite this chapter
- B. Jack Copeland 4 &
- Oron Shagrir 5
Part of the book series: Jerusalem Studies in Philosophy and History of Science ((JSPS))
470 Accesses
The Church-Turing thesis asserts that every effectively computable function is Turing computable. On the other hand, the physical Church-Turing Thesis (PCTT) concerns the computational power of physical systems, regardless of whether these perform effective computations. We distinguish three variants of PCTT – modest, bold and super-bold – and examine some objections to each. We highlight Itamar Pitowsky’s contributions to the formulation of these three variants of PCTT, and discuss his insightful remarks regarding their validity. The distinction between the modest and bold variants was originally advanced by Piccinini (Br J Philos Sci 62:733–769, 2011). The modest variant concerns the behavior of physical computing systems, while the bold variant is about the behavior of physical systems more generally. Both say that this behavior, when formulated in terms of some mathematical function, is Turing computable. We distinguish these two variants from a third – the super-bold variant – concerning decidability questions about the behavior of physical systems. This says, roughly, that every physical aspect of the behavior of physical systems – e.g., stability, periodicity – is decidable (i.e. Turing computable). We then examine some potential challenges to these three variants, drawn from relativity theory, quantum mechanics, and elsewhere. We conclude that all three variants are best viewed as open empirical hypotheses.
This is a preview of subscription content, log in via an institution to check access.
Access this chapter
- Available as PDF
- Read on any device
- Instant download
- Own it forever
- Available as EPUB and PDF
- Compact, lightweight edition
- Dispatched in 3 to 5 business days
- Free shipping worldwide - see info
- Durable hardcover edition
Tax calculation will be finalised at checkout
Purchases are for personal use only
Institutional subscriptions
Similar content being viewed by others
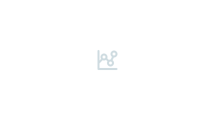
Semantics of Computable Physical Models
What Is Computable? What Is Feasibly Computable? A Physicist’s Viewpoint
Computability and Analysis, a Historical Approach
Some papers from the Workshop were published in 1990, in a special volume of Iyyun . The volume also contains papers by Avishai Margalit, Charles Parsons, Warren Goldfarb, William Tait, and Mark Steiner.
Aaronson, S. (2005). Guest column: NP-complete problems and physical reality. ACM SIGACT News, 36 (1), 30–52.
Google Scholar
Andréka, H., Németi, I., & Németi, P. (2009). General relativistic hypercomputing and foundation of mathematics. Natural Computing, 8 , 499–516.
Barrett, J. A., & Aitken, W. (2010). A note on the physical possibility of ordinal computation. The British Journal for the Philosophy of Science, 61 , 867–874.
Button, T. (2009). SAD computers and two versions of the Church-Turing thesis. The British Journal for the Philosophy of Science, 60 , 765–792.
Calude, C. S., Dinneen, M. J., Dumitrescu, M., & Svozil, K. (2010). Experimental evidence of quantum randomness incomputability. Physical Review A, 82 : 022102–1-022102–8.
Calude, C. S., & Svozil, K. (2008). Quantum randomness and value indefiniteness. Advanced Science Letters, 1 , 165–168.
Castelvecchi, D. (2015). Paradox at the heart of mathematics makes physics problem unanswerable. Nature, 528 , 207.
Chalmers, D. J. (2011). A computational foundation for the study of cognition. Journal of Cognitive Science, 12 , 323–357.
Church, A. (1936). An unsolvable problem of elementary number theory. American Journal of Mathematics, 58 , 345–363.
Church, A. (1940). On the concept of a random sequence. American Mathematical Society Bulletin, 46 , 130–135.
Copeland, B. J. (1997). The broad conception of computation. American Behavioral Scientist, 40 , 690–716.
Copeland, B. J. (1998). Even Turing machines can compute uncomputable functions. In C. Calude, J. Casti, & M. Dinneen (Eds.), Unconventional models of computation . New York: Springer.
Copeland, B. J. (2000). Narrow versus wide mechanism. Journal of Philosophy 96 , 5–32. Reprinted in M. Scheutz (Ed.) (2002). Computationalism . Cambridge, MA: MIT Press.
Copeland, B. J. (2002). Hypercomputation. Minds and Machines, 12 , 461–502.
Copeland, B. J. (2004). Hypercomputation: Philosophical issues. Theoretical Computer Science, 317 , 251–267.
Copeland, B. J. (2017). The Church-Turing thesis. In E. N. Zalta (Ed.), The Stanford encyclopedia of philosophy. https://plato.stanford.edu/archives/win2017/entries/church-turing/
Copeland, B. J., & Shagrir, O. (2007). Physical computation: How general are Gandy’s principles for mechanisms? Minds and Machines, 17 , 217–231.
Copeland, B. J., & Shagrir, O. (2011). Do accelerating Turing machines compute the uncomputable? Minds and Machines, 21 , 221–239.
Copeland, B. J., & Shagrir, O. (2019). The Church–Turing thesis—Logical limit, or breachable barrier? Communications of the ACM, 62 , 66–74.
Copeland, B. J., Shagrir, O., & Sprevak, M. (2018). Zuse’s thesis, Gandy’s thesis, and Penrose’s thesis. In M. Cuffaro & S. Fletcher (Eds.), Physical perspectives on computation, computational perspectives on physics (pp. 39–59). Cambridge: Cambridge University Press.
Cubitt, T. S., Perez-Garcia, D., & Wolf, M. M. (2015). Undecidability of the spectral gap. Nature, 528 (7581), 207–211.
Davies, B. E. (2001). Building infinite machines. The British Journal for the Philosophy of Science, 52 , 671–682.
Deutsch, D. (1985). Quantum theory, the Church-Turing principle and the universal quantum computer. Proceedings of the Royal Society of London A: Mathematical, Physical and Engineering Sciences, 400 (1818), 97–117.
Earman, J. (1986). A primer on determinism . Dordrecht: Reidel.
Earman, J., & Norton, J. D. (1993). Forever is a day: Supertasks in Pitowsky and Malament-Hogarth spacetimes. Philosophy of Science, 60 , 22–42.
Eisert, J., Müller, M. P., & Gogolin, C. (2012). Quantum measurement occurrence is undecidable. Physical Review Letters, 108 (26), 260501.
Etesi, G., & Németi, I. (2002). Non-Turing computations via Malament-Hogarth space-times. International Journal of Theoretical Physics, 41 , 341–370.
Fresco, N. (2014). Physical computation and cognitive science . Berlin: Springer.
Gandy, R. O. (1980). Church’s thesis and principles of mechanisms. In S. C. Kleene, J. Barwise, H. J. Keisler, & K. Kunen (Eds.), The Kleene symposium . Amsterdam: North-Holland.
Geroch, R., & Hartle, J. B. (1986). Computability and physical theories. Foundations of Physics, 16 , 533–550.
Grzegorczyk, A. (1955). Computable functionals. Fundamenta Mathematicae, 42 , 168–203.
Grzegorczyk, A. (1957). On the definitions of computable real continuous functions. Fundamenta Mathematicae, 44 , 61–71.
Harel, D., & Feldman, Y. A. (1992). Algorithmics: The spirit of computing (3rd ed.). Harlow: Addison-Wesley.
Hogarth, M. (1994). Non-Turing computers and non-Turing computability. Proceedings of the Biennial Meeting of the Philosophy of Science Association, 1 , 126–138.
Hogarth, M. (2004). Deciding arithmetic using SAD computers. The British Journal for the Philosophy of Science, 55 , 681–691.
Komar, A. (1964). Undecidability of macroscopically distinguishable states in quantum field theory. Physical Review , second series, 133B, 542–544.
Kreisel, G. (1965). Mathematical logic. In T. L. Saaty (Ed.), Lectures on modern mathematics (Vol. 3). New York: Wiley.
Kreisel, G. (1967). Mathematical logic: What has it done for the philosophy of mathematics? In R. Schoenman (Ed.), Bertrand Russell: Philosopher of the century . London: Allen and Unwin.
Lacombe, D. (1955). Extension de la notion de fonction récursive aux fonctions d’une ou plusieurs variables réelles III. Comptes Rendus Académie des Sciences Paris, 241 , 151–153.
Mazur, S. (1963). Computational analysis . Warsaw: Razprawy Matematyczne.
Miłkowski, M. (2013). Explaining the computational mind . Cambridge: MIT Press.
Moore, C. (1990). Unpredictability and undecidability in dynamical systems. Physical Review Letters, 64 , 2354–2357.
Németi, I., & Dávid, G. (2006). Relativistic computers and the Turing barrier. Journal of Applied Mathematics and Computation, 178 , 118–142.
Penrose, R. (1989). The emperor’s new mind: Concerning computers, minds and the laws of physics . Oxford: Oxford University Press.
Penrose, R. (1994). Shadows of the mind . Oxford: Oxford University Press.
Piccinini, G. (2011). The physical Church-Turing thesis: Modest or bold? The British Journal for the Philosophy of Science, 62 , 733–769.
Piccinini, G. (2015). Physical computation: A mechanistic account . Oxford: Oxford University Press.
Pitowsky, I. (1990). The physical Church thesis and physical computational complexity. Iyyun, 39 , 81–99.
Pitowsky, I. (1996). Laplace’s demon consults an oracle: The computational complexity of prediction. Studies in History and Philosophy of Science Part B, 27 (2), 161–180.
Pitowsky, I. (2002). Quantum speed-up of computations. Philosophy of Science, 69 , S168–S177.
Pour-El, M. B. (1974). Abstract computability and its relation to the general purpose analog computer (some connections between logic, differential equations and analog computers). Transactions of the American Mathematical Society, 199 , 1–28.
Pour-El, M. B., & Richards, I. J. (1981). The wave equation with computable initial data such that its unique solution is not computable. Advances in Mathematics, 39 , 215–239.
Pour-El, M. B., & Richards, I. J. (1989). Computability in analysis and physics . Berlin: Springer.
Scarpellini, B. (1963). Zwei unentscheidbare Probleme der Analysis. Zeitschrift für mathematische Logik und Grundlagen der Mathematik, 9, 265–289. English translation in Minds and Machines 12 (2002): 461–502.
Shagrir, O. (2006). Why we view the brain as a computer. Synthese, 153 , 393–416.
Shagrir, O., & Pitowsky, I. (2003). Physical hypercomputation and the Church–Turing thesis. Minds and Machines, 13 , 87–101.
Sprevak, M. (2010). Computation, individuation, and the received view on representation. Studies in History and Philosophy of Science, 41 , 260–270.
Turing, A. M. (1936). On computable numbers, with an application to the Entscheidungsproblem. Proceedings of the London Mathematical Society, Series, 2 (42), 230–265.
Turing, A. M. (1947). Lecture on the Automatic Computing Engine. In Copeland, B. J. (2004). The essential Turing: Seminal writings in computing, logic, philosophy, artificial intelligence, and artificial life, plus the secrets of Enigma . Oxford University Press.
Turing, A. M. (1948). Intelligent machinery. In The essential Turing . Oxford University Press.
Turing, A.M. (1950). Computing machinery and intelligence. In The essential Turing . Oxford University Press.
Welch, P. D. (2008). The extent of computation in Malament-Hogarth spacetimes. The British Journal for the Philosophy of Science, 59 , 659–674.
Wolfram, S. (1985). Undecidability and intractability in theoretical physics. Physical Review Letters, 54 , 735–738.
Download references
Acknowledgements
An early version of this chapter was presented at the Workshop on Physics and Computation (UCNC2018) in Fontainebleau, at the International Association for Computing and Philosophy meeting ( IACAP2018 ) in Warsaw, and at the research logic seminar in Tel Aviv University. Thanks to the audiences for helpful discussion. We are also grateful to Piccinini for his comments on a draft of this paper. Shagrir’s research was supported by the Israel Science Foundation grant 830/18.
Author information
Authors and affiliations.
Department of Philosophy, University of Canterbury, Christchurch, New Zealand
B. Jack Copeland
Department of Philosophy, The Hebrew University of Jerusalem, Jerusalem, Israel
Oron Shagrir
You can also search for this author in PubMed Google Scholar
Editor information
Editors and affiliations.
University of Haifa, Haifa, Israel
The Hebrew University of Jerusalem, Jerusalem, Israel
Orly Shenker
Rights and permissions
Reprints and permissions
Copyright information
© 2020 Springer Nature Switzerland AG
About this chapter
Copeland, B.J., Shagrir, O. (2020). Physical Computability Theses. In: Hemmo, M., Shenker, O. (eds) Quantum, Probability, Logic. Jerusalem Studies in Philosophy and History of Science. Springer, Cham. https://doi.org/10.1007/978-3-030-34316-3_9
Download citation
DOI : https://doi.org/10.1007/978-3-030-34316-3_9
Published : 08 April 2020
Publisher Name : Springer, Cham
Print ISBN : 978-3-030-34315-6
Online ISBN : 978-3-030-34316-3
eBook Packages : Religion and Philosophy Philosophy and Religion (R0)
Share this chapter
Anyone you share the following link with will be able to read this content:
Sorry, a shareable link is not currently available for this article.
Provided by the Springer Nature SharedIt content-sharing initiative
- Publish with us
Policies and ethics
- Find a journal
- Track your research
- Engineering Mathematics
- Discrete Mathematics
- Operating System
- Computer Networks
- Digital Logic and Design
- C Programming
- Data Structures
- Theory of Computation
- Compiler Design
- Computer Org and Architecture
Church’s Thesis for Turing Machine
- Turing machine for subtraction | Set 1
- Turing Machine for subtraction | Set 2
- Turing machine for 1's and 2’s complement
- Turing machine for multiplication
- Restricted Turing Machines
- Turing Machine in TOC
- Turing machine for copying data
- Turing Machine as Comparator
- Turing Machine for L = {a^n b^n | n>=1}
- Oracle Turing Machine
- Design a Turing Machine for equal number of a's and b's
- Turing Machine Simulator Using Python
- Design a Turing Machine to Generate 'ww' from 'w'
- Dovetailing in Turing Machines
- Turing Machine to accept maximum of two numbers
- Turing Machine Construction (Transducers Turing Machine) in Java
- Construct Turing Machine for incrementing Binary Number by 1
- Construct Turing Machine for L = {a^i b^j | i<j, i>0}
- Significance of Turing Test
In 1936, A method named as lambda-calculus was created by Alonzo Church in which the Church numerals are well defined, i.e. the encoding of natural numbers. Also in 1936, Turing machines (earlier called theoretical model for machines) was created by Alan Turing, that is used for manipulating the symbols of string with the help of tape.
Church Turing Thesis :
Turing machine is defined as an abstract representation of a computing device such as hardware in computers. Alan Turing proposed Logical Computing Machines (LCMs), i.e. Turing’s expressions for Turing Machines. This was done to define algorithms properly. So, Church made a mechanical method named as ‘M’ for manipulation of strings by using logic and mathematics. This method M must pass the following statements:
- Number of instructions in M must be finite.
- Output should be produced after performing finite number of steps.
- It should not be imaginary, i.e. can be made in real life.
- It should not require any complex understanding.
Using these statements Church proposed a hypothesis called
Church’s Turing thesis
that can be stated as: “The assumption that the intuitive notion of computable functions can be identified with partial recursive functions.”
Or in simple words we can say that “Every computation that can be carried out in the real world can be effectively performed by a Turing Machine.”
In 1930, this statement was first formulated by Alonzo Church and is usually referred to as Church’s thesis, or the Church-Turing thesis. However, this hypothesis cannot be proved. The recursive functions can be computable after taking following assumptions:
- Each and every function must be computable.
- Let ‘F’ be the computable function and after performing some elementary operations to ‘F’, it will transform a new function ‘G’ then this function ‘G’ automatically becomes the computable function.
- If any functions that follow above two assumptions must be states as computable function.
Please Login to comment...
Similar reads, improve your coding skills with practice.
What kind of Experience do you want to share?

IMAGES
VIDEO
COMMENTS
In computability theory, the Church-Turing thesis (also known as computability thesis, [1] the Turing-Church thesis, [2] the Church-Turing conjecture, Church's thesis, Church's conjecture, and Turing's thesis) is a thesis about the nature of computable functions. It states that a function on the natural numbers can be calculated by an ...
The Church-Turing Thesis. First published Wed Jan 8, 1997; substantive revision Mon Dec 18, 2023. The Church-Turing thesis (or Turing-Church thesis) is a fundamental claim in the theory of computability. It was advanced independently by Church and Turing in the mid 1930s. There are various equivalent formulations of the thesis.
notions: computability by a Turing machine, general recursiveness in the sense of Herbrand-Gödel-Kleene, and λ-definability in the sense of Kleene and the present ... Thus was born what is now called the Church-Turing Thesis, according to which the effectively computable functions are exactly those computable by a Turing machine.5 The (Church ...
tive computability. Here, in brief, is the story of what led Turing to Church, what was in his thesis, and what came after, both for him and for the subject.1 From Cambridge to Princeton As an undergraduate at King's College, Cambridge, from 1931 to 1934, Turing was attracted to many parts of mathematics, including mathematical logic.
Variants of TMs, Church-Turing Thesis 1 ¥ Reading: Sipser, ¤ 3.2, ¤ 3.3. T M Ext ensions T hat Do Not Increase It s Power ¥ TMs with a 2-way inÞ nite tape á á á ! a b a a á á á Ð Unbounded tape to left as well as right ¥ Claim: Any language recognizable (resp., decidable) by a 2-way inÞ nite tape is also recognizable
Very rapidly it was shown that the mathematical scope of Turing computability coincided with Church's definition (and also with the scope of the general recursive functions defined by Gödel). Turing wrote his own statement (Turing 1939, p. 166) of the conclusions that had been reached in 1938; it is in the Ph.D. thesis that he wrote under ...
When the thesis is expressed in terms of the formal concept proposed by Turing, it is appropriate to refer to the thesis also as 'Turing's thesis'; and mutatis mutandis in the case of Church. The formal concept proposed by Turing is that of computability by Turing machine. He argued for the claim (Turing's thesis) that whenever there is an ...
The notions of algorithm and computability as formal subjects of mathematical reasoning gained prominence in the twentieth century with the advent of symbolic logic and the discovery of inherent limitations to formal deductive reasoning. The most popular formal model of computation is due to Alan Turing. The claim that computation paradigms such as Turing's capture the intuitive notion of ...
The Computability (Church-Turing) Thesis formalized the informal notions of computation and enabled their mathematical treatment. The thesis is by many viewed as an unproved or even unprovable proposition, although it has been subjected to continuous examination. Nevertheless, the consequences of this breakthrough are immense.
Turing's famous 1936 paper introduced a formal definition of a computing machine, a Turing machine. This model led to both the development of actual computers and to computability theory, the study of what machines can and cannot compute. This book presents classical computability theory from Turing and Post to current results and methods, and ...
The Church- Turing thesis states that a function on the positive integers is effectively calculable if and only if it is computable. An informal accumulation of the tradition in S. C. Kleene (1952) has transformed it to the Computability thesis: there is an objective notion of effective computability independent of a particular formalisation ...
The Church-Turing thesis is a fundamental tenet of computer science that provides the very definition of what it means to be "computable." In essence, it claims that any function that is intuitively "computable" by an effective procedure can be computed by a Turing machine. While this may sound simple, the implications are profound, touching ...
Alan Turing argued that his model was a correct formulation of effective computability. He defended the following proposition, which has come to be called the Church-Turing thesis in acknowledgment of the contribution of the logician Alonzo Church, who proposed a parallel formalism based on ideas from logic and known as the λ-calculus ...
Thesis 3.1 (Turing's Thesis, 1936). A function on the integers is computable by a nite procedure if and only if it is computable by a Turing a-machine. Church [1936] had tried to give a similar informal argument for Church's Thesis but Gandy [1988:79] and especially Sieg [1994:80, 87] in their excel-
Turing gave two formulations of what he called "the Hilbert Entscheidungsproblem" (1936 [2004: 84, 87]): ... Given Turing's thesis, the two formulations are equivalent. ... who worked closely with Church and played a leading part in the development of computability theory in the 1930s. Kleene noted that
Publication date: 2013. Computer scientists, mathematicians, and philosophers discuss the conceptual foundations of the notion of computability as well as recent theoretical developments. In the 1930s a series of seminal works published by Alan Turing, Kurt Gödel, Alonzo Church, and others established the theoretical basis for computability.
(The Church-Turing Thesis) A function f is partial recursive iff f is Turing-computable iff f is effectively computable. Let us take the path of Church and Kleene and have a brief look at their \(\lambda \) -calculus, which later became the basis of many functional programming languages such as Lisp, ML, and Haskell.
A thesis aiming to limit the scope of algorithmic computability to Turing computability should thus not state that every possible algorithmic process can be performed by a Turing machine. The way to express the thesis is to say the extensional input-output function ια associated with an algorithm α is always Turing-computable; ια is simply ...
Shapiro continues by arguing that the notion of informal computability at issue in the Church-Turing thesis is subject to open texture in this way. He recounts the sharpenings of computability during the twentieth century, noting that there were other options along the way, other idealizations of computation, that could have been chosen (such ...
Computability theory, also known as recursion theory, is a branch of mathematical logic, computer science, and the theory of computation that originated in the 1930s with the study of computable functions and Turing degrees.The field has since expanded to include the study of generalized computability and definability.In these areas, computability theory overlaps with proof theory and ...
At the time Turing was writing his paper, the modern computer was not developed yet and so rephrasings of Turing's thesis which identify Turing computability with computability by a modern computer are interpretations rather than historically correct statements of Turing's thesis. The existing computing machines at the time Turing wrote his ...
The Church-Turing thesis asserts that every effectively computable function is Turing computable. On the other hand, the physical Church-Turing Thesis (PCTT) concerns the computational power of physical systems, regardless of whether these perform effective computations. We distinguish three variants of PCTT - modest, bold and super-bold - and examine some objections to each.
Church's Turing thesis. that can be stated as: "The assumption that the intuitive notion of computable functions can be identified with partial recursive functions.". Or in simple words we can say that "Every computation that can be carried out in the real world can be effectively performed by a Turing Machine.".