Have a language expert improve your writing
Run a free plagiarism check in 10 minutes, automatically generate references for free.
- Knowledge Base
- Methodology
- How to Write a Strong Hypothesis | Guide & Examples

How to Write a Strong Hypothesis | Guide & Examples
Published on 6 May 2022 by Shona McCombes .
A hypothesis is a statement that can be tested by scientific research. If you want to test a relationship between two or more variables, you need to write hypotheses before you start your experiment or data collection.
Table of contents
What is a hypothesis, developing a hypothesis (with example), hypothesis examples, frequently asked questions about writing hypotheses.
A hypothesis states your predictions about what your research will find. It is a tentative answer to your research question that has not yet been tested. For some research projects, you might have to write several hypotheses that address different aspects of your research question.
A hypothesis is not just a guess – it should be based on existing theories and knowledge. It also has to be testable, which means you can support or refute it through scientific research methods (such as experiments, observations, and statistical analysis of data).
Variables in hypotheses
Hypotheses propose a relationship between two or more variables . An independent variable is something the researcher changes or controls. A dependent variable is something the researcher observes and measures.
In this example, the independent variable is exposure to the sun – the assumed cause . The dependent variable is the level of happiness – the assumed effect .
Prevent plagiarism, run a free check.
Step 1: ask a question.
Writing a hypothesis begins with a research question that you want to answer. The question should be focused, specific, and researchable within the constraints of your project.
Step 2: Do some preliminary research
Your initial answer to the question should be based on what is already known about the topic. Look for theories and previous studies to help you form educated assumptions about what your research will find.
At this stage, you might construct a conceptual framework to identify which variables you will study and what you think the relationships are between them. Sometimes, you’ll have to operationalise more complex constructs.
Step 3: Formulate your hypothesis
Now you should have some idea of what you expect to find. Write your initial answer to the question in a clear, concise sentence.
Step 4: Refine your hypothesis
You need to make sure your hypothesis is specific and testable. There are various ways of phrasing a hypothesis, but all the terms you use should have clear definitions, and the hypothesis should contain:
- The relevant variables
- The specific group being studied
- The predicted outcome of the experiment or analysis
Step 5: Phrase your hypothesis in three ways
To identify the variables, you can write a simple prediction in if … then form. The first part of the sentence states the independent variable and the second part states the dependent variable.
In academic research, hypotheses are more commonly phrased in terms of correlations or effects, where you directly state the predicted relationship between variables.
If you are comparing two groups, the hypothesis can state what difference you expect to find between them.
Step 6. Write a null hypothesis
If your research involves statistical hypothesis testing , you will also have to write a null hypothesis. The null hypothesis is the default position that there is no association between the variables. The null hypothesis is written as H 0 , while the alternative hypothesis is H 1 or H a .
Hypothesis testing is a formal procedure for investigating our ideas about the world using statistics. It is used by scientists to test specific predictions, called hypotheses , by calculating how likely it is that a pattern or relationship between variables could have arisen by chance.
A hypothesis is not just a guess. It should be based on existing theories and knowledge. It also has to be testable, which means you can support or refute it through scientific research methods (such as experiments, observations, and statistical analysis of data).
A research hypothesis is your proposed answer to your research question. The research hypothesis usually includes an explanation (‘ x affects y because …’).
A statistical hypothesis, on the other hand, is a mathematical statement about a population parameter. Statistical hypotheses always come in pairs: the null and alternative hypotheses. In a well-designed study , the statistical hypotheses correspond logically to the research hypothesis.
Cite this Scribbr article
If you want to cite this source, you can copy and paste the citation or click the ‘Cite this Scribbr article’ button to automatically add the citation to our free Reference Generator.
McCombes, S. (2022, May 06). How to Write a Strong Hypothesis | Guide & Examples. Scribbr. Retrieved 31 May 2024, from https://www.scribbr.co.uk/research-methods/hypothesis-writing/
Is this article helpful?
Shona McCombes
Other students also liked, operationalisation | a guide with examples, pros & cons, what is a conceptual framework | tips & examples, a quick guide to experimental design | 5 steps & examples.
- Translators
- Graphic Designers
Please enter the email address you used for your account. Your sign in information will be sent to your email address after it has been verified.
How To Write a Strong Research Hypothesis
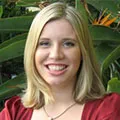
Are you looking to take your research project to the next level? Have you heard of the power of a hypothesis but need to figure out how to formulate one that will unlock potential discoveries? We can help!
So get ready; it's time to dive into unlocking the power of research! This blog post will explore what makes a well-crafted and powerful hypothesis - from identifying a research question to developing supporting evidence.
By learning how to craft a compelling hypothesis, you'll have more tremendous success in every step of your research project.
What are hypotheses, and why are they important?
A hypothesis is an educated guess or a proposition based on limited evidence as a starting point for further investigation. It provides a framework for research and allows researchers to refine their ideas, collect data, and draw conclusions. Hypotheses are essential to the process because they will enable us to organize our thoughts and test theories properly.
Hypotheses are used in many fields , from medicine to psychology to economics. In each area, developing hypotheses based on observations enable researchers to make predictions about their data and guide them toward finding meaningful results.
For example, in medicine, hypotheses can be used to predict which treatments will be most effective for particular conditions or which drugs may have adverse effects when taken together. This allows doctors to make better decisions when caring for patients.
In psychology, hypotheses are often used in experiments to determine whether certain variables influence behavior or mental processes. By testing different combinations of variables, psychologists can identify patterns and understand why people behave the way they do.
In economics, hypotheses provide economists with a framework for analyzing the relationship between economic variables such as wages and consumer spending habits. By understanding these relationships, economists can better understand how economic forces affect the economy.
Overall, hypotheses play an essential role in helping scientists develop new ideas and draw meaningful conclusions from the collected data. Without taking the step to create hypotheses, it would be difficult for researchers to make sense of the vast amounts of information available today and use it effectively in their investigations.
How to determine an effective research question to form your hypothesis
When conducting research, having a compelling research question is critical . Properly formulating this question will allow the researcher to develop their hypothesis. A research question provides a clear and focused goal for your research study and also gives direction on how to get there. A compelling research question should be specific, answerable in the context of your field of study, significant, novel (not already answered by previous studies), and timely – that is, relevant to current events or trends.
Before determining the best research question, you must first understand your topic. Think about the area of knowledge that interests you most and narrow it down to a single theme or concept within this topic. Focus on what interests you most within this theme, and make sure there is room for further exploration and analysis. Once you have chosen a specific topic and narrowed down your focus, you can begin formulating questions related to your project.
To ensure relevance and impact to your field of study, choose questions that address essential issues in the literature or suggest solutions to existing problems. Avoid overly broad topics with unclear objectives; instead, opt for focused questions to enable targeted data collection and analysis with concrete results.
Additionally, consider time frames when formulating questions. If the issue has been discussed extensively in the past but has not been revisited recently, then it's likely not worthy of a new investigation.
Once you have developed some potential questions related to your topic, review them carefully and decide which question best captures the essence of what you want to learn through researching this topic.
Ask yourself:
- Is this question answerable?
- Does it fit within my field of study?
- Is it significant enough?
- Would its findings be novel?
If so, then congratulations! You have identified a compelling research question.
Tips for crafting a well-crafted hypothesis
Once you have formulated the official research question, you may develop the formal hypothesis. When composing a hypothesis, it's essential to think carefully about the question you are trying to answer.
A solid hypothesis should be testable, meaning that it can be verified or disproved through research. It should also be specific and focused on one issue at a time. Here are some tips for crafting a well-crafted hypothesis:
- Consider the goal of your research: Think about what it is that you want to learn or determine from your experiment and make sure that your hypothesis reflects this goal.
- Create an educated guess as to why something is happening: Your hypothesis should explain why something is occurring based on what evidence you already have and direct further investigation into the matter. For example, if you hypothesize that increased carbon dioxide levels in the atmosphere will lead to global warming, your research should focus on examining this relationship further.
- Define any variables or parameters involved in the experiment: This includes things like temperature or chemical composition that could potentially affect the outcome of any experiments done in pursuit of testing your hypothesis.
- Use clear and precise language: Make sure your hypothesis is written with clear and precise language so that anyone reading it can understand exactly what you are attempting to investigate or explain. Avoid complex words and keep sentences short whenever possible.
Following these simple tips will help ensure that your hypothesis is well-crafted and ready for testing!
Examples of evidence that can support your hypothesis
When it comes to developing a hypothesis, supporting evidence is essential for making sure it holds up. This evidence helps strengthen the argument that is being driven by providing facts and logical reasoning that support the hypothesis.
Examples of evidence that can be used to back up a hypothesis include using data from experiments, case studies, and other research projects. Data from experiments can provide insight into how certain variables interact to form a particular outcome.
Case studies may offer greater depth in understanding a specific phenomenon's cause and effect; research projects may yield results that confirm or refute existing theories on a subject.
In addition to these traditional forms of evidence, personal experiences or observations can also help to support a hypothesis. For example, if someone's daily commute has been consistently faster since they changed routes, they could use their personal experience to argue that making this change resulted in shorter commutes.
Similarly, suppose someone has witnessed how two variables consistently coincide (i.e., when one goes up, another goes down). In that case, this could be used to support the notion that there is some correlation between these two aspects.
Overall, evidence to support your hypothesis is crucial for ensuring its validity and credibility. While conducting experiments or researching may seem like time-consuming processes, having solid supporting evidence will make it much easier to defend your ideas convincingly when challenged.
Therefore, it is crucial to take the time necessary to gather credible sources of information to provide the most substantial possible backing for your hypotheses.
Understanding the potential of hypotheses and how they can help your research project progress
The power of research lies in the ability to develop and test hypotheses. A hypothesis is a statement or an idea that can be tested to determine its validity.
Essentially, it is a form of educated guesswork that helps researchers form conclusions about their data. By developing a hypothesis for a research project, you are effectively setting up the framework for further exploration.
When developing a hypothesis, you must consider both the expected outcomes and possible alternative explanations. This will help you focus on testing the possible results without getting sidetracked by irrelevant information. Once you have established a concrete hypothesis, it can then be used as a basis for further research and experimentation.
The process of testing hypotheses is an integral part of the scientific method and can help researchers build confidence in their findings and conclusions. Through careful observation and experimentation, researchers can compare their results against what they initially hypothesized, allowing them to draw more accurate conclusions about their data. As such, hypotheses play an essential role in helping researchers connect the dots between different pieces of evidence and form meaningful conclusions.
Overall, understanding how hypotheses can be used in research projects can be immensely beneficial in helping progress towards reaching meaningful insights from their data. By setting up expectations ahead of time and then testing them against real-world conditions, researchers can gain valuable insights that could potentially change the way we understand our world – now that's something worth exploring!
Final thoughts
A hypothesis is a proposed explanation for an observable phenomenon. It's important to note that hypotheses are not the same thing as theories–a theory is a much broader and well-established frame of reference that explains multiple phenomena.
Generally, scientists form a research question and then narrow it down to a testable hypothesis. After making observations and conducting experiments to gather data, researchers can use evidence to support or reject the hypothesis.
By following these steps to formulate a solid hypothesis, you will be on your way to developing a successful research project. Happy researching!
Header image by Bnenin .
Related Posts
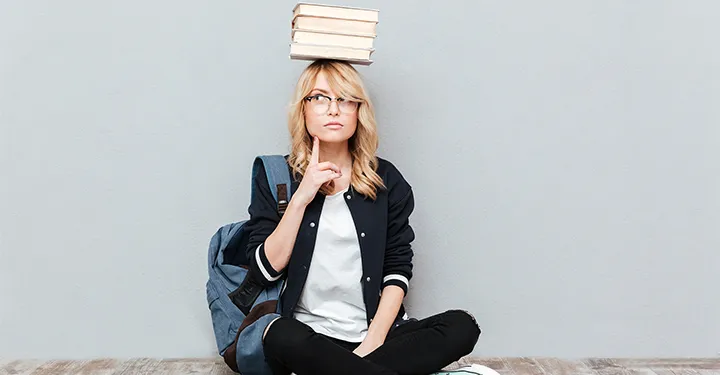
APA Heading Format: All 5 Levels Explained Plus Examples
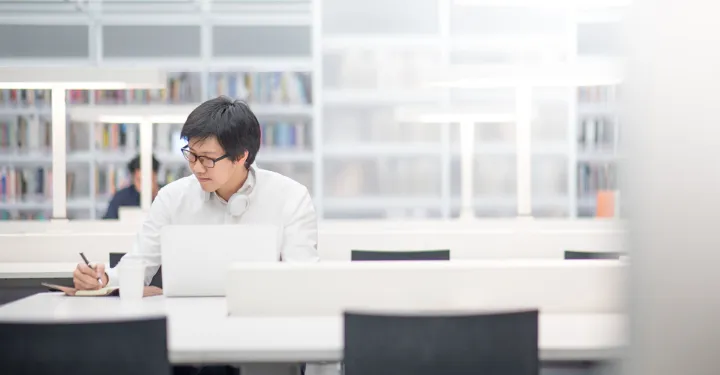
The Concluding Paragraph: How to End Your Essay
- Academic Writing Advice
- All Blog Posts
- Writing Advice
- Admissions Writing Advice
- Book Writing Advice
- Short Story Advice
- Employment Writing Advice
- Business Writing Advice
- Web Content Advice
- Article Writing Advice
- Magazine Writing Advice
- Grammar Advice
- Dialect Advice
- Editing Advice
- Freelance Advice
- Legal Writing Advice
- Poetry Advice
- Graphic Design Advice
- Logo Design Advice
- Translation Advice
- Blog Reviews
- Short Story Award Winners
- Scholarship Winners
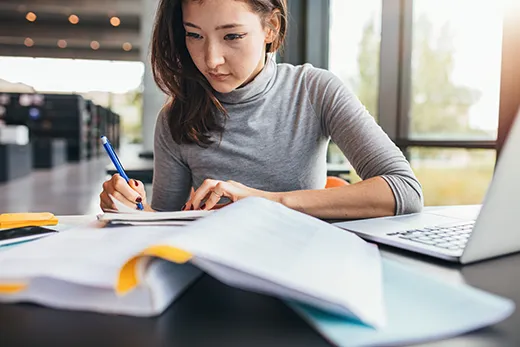
Need an academic editor before submitting your work?
- Bipolar Disorder
- Therapy Center
- When To See a Therapist
- Types of Therapy
- Best Online Therapy
- Best Couples Therapy
- Best Family Therapy
- Managing Stress
- Sleep and Dreaming
- Understanding Emotions
- Self-Improvement
- Healthy Relationships
- Student Resources
- Personality Types
- Guided Meditations
- Verywell Mind Insights
- 2024 Verywell Mind 25
- Mental Health in the Classroom
- Editorial Process
- Meet Our Review Board
- Crisis Support
How to Write a Great Hypothesis
Hypothesis Definition, Format, Examples, and Tips
Kendra Cherry, MS, is a psychosocial rehabilitation specialist, psychology educator, and author of the "Everything Psychology Book."
:max_bytes(150000):strip_icc():format(webp)/IMG_9791-89504ab694d54b66bbd72cb84ffb860e.jpg)
Amy Morin, LCSW, is a psychotherapist and international bestselling author. Her books, including "13 Things Mentally Strong People Don't Do," have been translated into more than 40 languages. Her TEDx talk, "The Secret of Becoming Mentally Strong," is one of the most viewed talks of all time.
:max_bytes(150000):strip_icc():format(webp)/VW-MIND-Amy-2b338105f1ee493f94d7e333e410fa76.jpg)
Verywell / Alex Dos Diaz
- The Scientific Method
Hypothesis Format
Falsifiability of a hypothesis.
- Operationalization
Hypothesis Types
Hypotheses examples.
- Collecting Data
A hypothesis is a tentative statement about the relationship between two or more variables. It is a specific, testable prediction about what you expect to happen in a study. It is a preliminary answer to your question that helps guide the research process.
Consider a study designed to examine the relationship between sleep deprivation and test performance. The hypothesis might be: "This study is designed to assess the hypothesis that sleep-deprived people will perform worse on a test than individuals who are not sleep-deprived."
At a Glance
A hypothesis is crucial to scientific research because it offers a clear direction for what the researchers are looking to find. This allows them to design experiments to test their predictions and add to our scientific knowledge about the world. This article explores how a hypothesis is used in psychology research, how to write a good hypothesis, and the different types of hypotheses you might use.
The Hypothesis in the Scientific Method
In the scientific method , whether it involves research in psychology, biology, or some other area, a hypothesis represents what the researchers think will happen in an experiment. The scientific method involves the following steps:
- Forming a question
- Performing background research
- Creating a hypothesis
- Designing an experiment
- Collecting data
- Analyzing the results
- Drawing conclusions
- Communicating the results
The hypothesis is a prediction, but it involves more than a guess. Most of the time, the hypothesis begins with a question which is then explored through background research. At this point, researchers then begin to develop a testable hypothesis.
Unless you are creating an exploratory study, your hypothesis should always explain what you expect to happen.
In a study exploring the effects of a particular drug, the hypothesis might be that researchers expect the drug to have some type of effect on the symptoms of a specific illness. In psychology, the hypothesis might focus on how a certain aspect of the environment might influence a particular behavior.
Remember, a hypothesis does not have to be correct. While the hypothesis predicts what the researchers expect to see, the goal of the research is to determine whether this guess is right or wrong. When conducting an experiment, researchers might explore numerous factors to determine which ones might contribute to the ultimate outcome.
In many cases, researchers may find that the results of an experiment do not support the original hypothesis. When writing up these results, the researchers might suggest other options that should be explored in future studies.
In many cases, researchers might draw a hypothesis from a specific theory or build on previous research. For example, prior research has shown that stress can impact the immune system. So a researcher might hypothesize: "People with high-stress levels will be more likely to contract a common cold after being exposed to the virus than people who have low-stress levels."
In other instances, researchers might look at commonly held beliefs or folk wisdom. "Birds of a feather flock together" is one example of folk adage that a psychologist might try to investigate. The researcher might pose a specific hypothesis that "People tend to select romantic partners who are similar to them in interests and educational level."
Elements of a Good Hypothesis
So how do you write a good hypothesis? When trying to come up with a hypothesis for your research or experiments, ask yourself the following questions:
- Is your hypothesis based on your research on a topic?
- Can your hypothesis be tested?
- Does your hypothesis include independent and dependent variables?
Before you come up with a specific hypothesis, spend some time doing background research. Once you have completed a literature review, start thinking about potential questions you still have. Pay attention to the discussion section in the journal articles you read . Many authors will suggest questions that still need to be explored.
How to Formulate a Good Hypothesis
To form a hypothesis, you should take these steps:
- Collect as many observations about a topic or problem as you can.
- Evaluate these observations and look for possible causes of the problem.
- Create a list of possible explanations that you might want to explore.
- After you have developed some possible hypotheses, think of ways that you could confirm or disprove each hypothesis through experimentation. This is known as falsifiability.
In the scientific method , falsifiability is an important part of any valid hypothesis. In order to test a claim scientifically, it must be possible that the claim could be proven false.
Students sometimes confuse the idea of falsifiability with the idea that it means that something is false, which is not the case. What falsifiability means is that if something was false, then it is possible to demonstrate that it is false.
One of the hallmarks of pseudoscience is that it makes claims that cannot be refuted or proven false.
The Importance of Operational Definitions
A variable is a factor or element that can be changed and manipulated in ways that are observable and measurable. However, the researcher must also define how the variable will be manipulated and measured in the study.
Operational definitions are specific definitions for all relevant factors in a study. This process helps make vague or ambiguous concepts detailed and measurable.
For example, a researcher might operationally define the variable " test anxiety " as the results of a self-report measure of anxiety experienced during an exam. A "study habits" variable might be defined by the amount of studying that actually occurs as measured by time.
These precise descriptions are important because many things can be measured in various ways. Clearly defining these variables and how they are measured helps ensure that other researchers can replicate your results.
Replicability
One of the basic principles of any type of scientific research is that the results must be replicable.
Replication means repeating an experiment in the same way to produce the same results. By clearly detailing the specifics of how the variables were measured and manipulated, other researchers can better understand the results and repeat the study if needed.
Some variables are more difficult than others to define. For example, how would you operationally define a variable such as aggression ? For obvious ethical reasons, researchers cannot create a situation in which a person behaves aggressively toward others.
To measure this variable, the researcher must devise a measurement that assesses aggressive behavior without harming others. The researcher might utilize a simulated task to measure aggressiveness in this situation.
Hypothesis Checklist
- Does your hypothesis focus on something that you can actually test?
- Does your hypothesis include both an independent and dependent variable?
- Can you manipulate the variables?
- Can your hypothesis be tested without violating ethical standards?
The hypothesis you use will depend on what you are investigating and hoping to find. Some of the main types of hypotheses that you might use include:
- Simple hypothesis : This type of hypothesis suggests there is a relationship between one independent variable and one dependent variable.
- Complex hypothesis : This type suggests a relationship between three or more variables, such as two independent and dependent variables.
- Null hypothesis : This hypothesis suggests no relationship exists between two or more variables.
- Alternative hypothesis : This hypothesis states the opposite of the null hypothesis.
- Statistical hypothesis : This hypothesis uses statistical analysis to evaluate a representative population sample and then generalizes the findings to the larger group.
- Logical hypothesis : This hypothesis assumes a relationship between variables without collecting data or evidence.
A hypothesis often follows a basic format of "If {this happens} then {this will happen}." One way to structure your hypothesis is to describe what will happen to the dependent variable if you change the independent variable .
The basic format might be: "If {these changes are made to a certain independent variable}, then we will observe {a change in a specific dependent variable}."
A few examples of simple hypotheses:
- "Students who eat breakfast will perform better on a math exam than students who do not eat breakfast."
- "Students who experience test anxiety before an English exam will get lower scores than students who do not experience test anxiety."
- "Motorists who talk on the phone while driving will be more likely to make errors on a driving course than those who do not talk on the phone."
- "Children who receive a new reading intervention will have higher reading scores than students who do not receive the intervention."
Examples of a complex hypothesis include:
- "People with high-sugar diets and sedentary activity levels are more likely to develop depression."
- "Younger people who are regularly exposed to green, outdoor areas have better subjective well-being than older adults who have limited exposure to green spaces."
Examples of a null hypothesis include:
- "There is no difference in anxiety levels between people who take St. John's wort supplements and those who do not."
- "There is no difference in scores on a memory recall task between children and adults."
- "There is no difference in aggression levels between children who play first-person shooter games and those who do not."
Examples of an alternative hypothesis:
- "People who take St. John's wort supplements will have less anxiety than those who do not."
- "Adults will perform better on a memory task than children."
- "Children who play first-person shooter games will show higher levels of aggression than children who do not."
Collecting Data on Your Hypothesis
Once a researcher has formed a testable hypothesis, the next step is to select a research design and start collecting data. The research method depends largely on exactly what they are studying. There are two basic types of research methods: descriptive research and experimental research.
Descriptive Research Methods
Descriptive research such as case studies , naturalistic observations , and surveys are often used when conducting an experiment is difficult or impossible. These methods are best used to describe different aspects of a behavior or psychological phenomenon.
Once a researcher has collected data using descriptive methods, a correlational study can examine how the variables are related. This research method might be used to investigate a hypothesis that is difficult to test experimentally.
Experimental Research Methods
Experimental methods are used to demonstrate causal relationships between variables. In an experiment, the researcher systematically manipulates a variable of interest (known as the independent variable) and measures the effect on another variable (known as the dependent variable).
Unlike correlational studies, which can only be used to determine if there is a relationship between two variables, experimental methods can be used to determine the actual nature of the relationship—whether changes in one variable actually cause another to change.
The hypothesis is a critical part of any scientific exploration. It represents what researchers expect to find in a study or experiment. In situations where the hypothesis is unsupported by the research, the research still has value. Such research helps us better understand how different aspects of the natural world relate to one another. It also helps us develop new hypotheses that can then be tested in the future.
Thompson WH, Skau S. On the scope of scientific hypotheses . R Soc Open Sci . 2023;10(8):230607. doi:10.1098/rsos.230607
Taran S, Adhikari NKJ, Fan E. Falsifiability in medicine: what clinicians can learn from Karl Popper [published correction appears in Intensive Care Med. 2021 Jun 17;:]. Intensive Care Med . 2021;47(9):1054-1056. doi:10.1007/s00134-021-06432-z
Eyler AA. Research Methods for Public Health . 1st ed. Springer Publishing Company; 2020. doi:10.1891/9780826182067.0004
Nosek BA, Errington TM. What is replication ? PLoS Biol . 2020;18(3):e3000691. doi:10.1371/journal.pbio.3000691
Aggarwal R, Ranganathan P. Study designs: Part 2 - Descriptive studies . Perspect Clin Res . 2019;10(1):34-36. doi:10.4103/picr.PICR_154_18
Nevid J. Psychology: Concepts and Applications. Wadworth, 2013.
By Kendra Cherry, MSEd Kendra Cherry, MS, is a psychosocial rehabilitation specialist, psychology educator, and author of the "Everything Psychology Book."
Research Hypothesis In Psychology: Types, & Examples
Saul Mcleod, PhD
Editor-in-Chief for Simply Psychology
BSc (Hons) Psychology, MRes, PhD, University of Manchester
Saul Mcleod, PhD., is a qualified psychology teacher with over 18 years of experience in further and higher education. He has been published in peer-reviewed journals, including the Journal of Clinical Psychology.
Learn about our Editorial Process
Olivia Guy-Evans, MSc
Associate Editor for Simply Psychology
BSc (Hons) Psychology, MSc Psychology of Education
Olivia Guy-Evans is a writer and associate editor for Simply Psychology. She has previously worked in healthcare and educational sectors.
On This Page:
A research hypothesis, in its plural form “hypotheses,” is a specific, testable prediction about the anticipated results of a study, established at its outset. It is a key component of the scientific method .
Hypotheses connect theory to data and guide the research process towards expanding scientific understanding
Some key points about hypotheses:
- A hypothesis expresses an expected pattern or relationship. It connects the variables under investigation.
- It is stated in clear, precise terms before any data collection or analysis occurs. This makes the hypothesis testable.
- A hypothesis must be falsifiable. It should be possible, even if unlikely in practice, to collect data that disconfirms rather than supports the hypothesis.
- Hypotheses guide research. Scientists design studies to explicitly evaluate hypotheses about how nature works.
- For a hypothesis to be valid, it must be testable against empirical evidence. The evidence can then confirm or disprove the testable predictions.
- Hypotheses are informed by background knowledge and observation, but go beyond what is already known to propose an explanation of how or why something occurs.
Predictions typically arise from a thorough knowledge of the research literature, curiosity about real-world problems or implications, and integrating this to advance theory. They build on existing literature while providing new insight.
Types of Research Hypotheses
Alternative hypothesis.
The research hypothesis is often called the alternative or experimental hypothesis in experimental research.
It typically suggests a potential relationship between two key variables: the independent variable, which the researcher manipulates, and the dependent variable, which is measured based on those changes.
The alternative hypothesis states a relationship exists between the two variables being studied (one variable affects the other).
A hypothesis is a testable statement or prediction about the relationship between two or more variables. It is a key component of the scientific method. Some key points about hypotheses:
- Important hypotheses lead to predictions that can be tested empirically. The evidence can then confirm or disprove the testable predictions.
In summary, a hypothesis is a precise, testable statement of what researchers expect to happen in a study and why. Hypotheses connect theory to data and guide the research process towards expanding scientific understanding.
An experimental hypothesis predicts what change(s) will occur in the dependent variable when the independent variable is manipulated.
It states that the results are not due to chance and are significant in supporting the theory being investigated.
The alternative hypothesis can be directional, indicating a specific direction of the effect, or non-directional, suggesting a difference without specifying its nature. It’s what researchers aim to support or demonstrate through their study.
Null Hypothesis
The null hypothesis states no relationship exists between the two variables being studied (one variable does not affect the other). There will be no changes in the dependent variable due to manipulating the independent variable.
It states results are due to chance and are not significant in supporting the idea being investigated.
The null hypothesis, positing no effect or relationship, is a foundational contrast to the research hypothesis in scientific inquiry. It establishes a baseline for statistical testing, promoting objectivity by initiating research from a neutral stance.
Many statistical methods are tailored to test the null hypothesis, determining the likelihood of observed results if no true effect exists.
This dual-hypothesis approach provides clarity, ensuring that research intentions are explicit, and fosters consistency across scientific studies, enhancing the standardization and interpretability of research outcomes.
Nondirectional Hypothesis
A non-directional hypothesis, also known as a two-tailed hypothesis, predicts that there is a difference or relationship between two variables but does not specify the direction of this relationship.
It merely indicates that a change or effect will occur without predicting which group will have higher or lower values.
For example, “There is a difference in performance between Group A and Group B” is a non-directional hypothesis.
Directional Hypothesis
A directional (one-tailed) hypothesis predicts the nature of the effect of the independent variable on the dependent variable. It predicts in which direction the change will take place. (i.e., greater, smaller, less, more)
It specifies whether one variable is greater, lesser, or different from another, rather than just indicating that there’s a difference without specifying its nature.
For example, “Exercise increases weight loss” is a directional hypothesis.
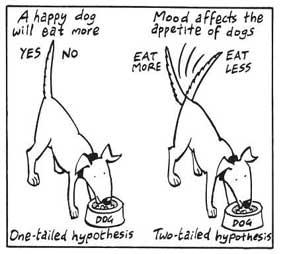
Falsifiability
The Falsification Principle, proposed by Karl Popper , is a way of demarcating science from non-science. It suggests that for a theory or hypothesis to be considered scientific, it must be testable and irrefutable.
Falsifiability emphasizes that scientific claims shouldn’t just be confirmable but should also have the potential to be proven wrong.
It means that there should exist some potential evidence or experiment that could prove the proposition false.
However many confirming instances exist for a theory, it only takes one counter observation to falsify it. For example, the hypothesis that “all swans are white,” can be falsified by observing a black swan.
For Popper, science should attempt to disprove a theory rather than attempt to continually provide evidence to support a research hypothesis.
Can a Hypothesis be Proven?
Hypotheses make probabilistic predictions. They state the expected outcome if a particular relationship exists. However, a study result supporting a hypothesis does not definitively prove it is true.
All studies have limitations. There may be unknown confounding factors or issues that limit the certainty of conclusions. Additional studies may yield different results.
In science, hypotheses can realistically only be supported with some degree of confidence, not proven. The process of science is to incrementally accumulate evidence for and against hypothesized relationships in an ongoing pursuit of better models and explanations that best fit the empirical data. But hypotheses remain open to revision and rejection if that is where the evidence leads.
- Disproving a hypothesis is definitive. Solid disconfirmatory evidence will falsify a hypothesis and require altering or discarding it based on the evidence.
- However, confirming evidence is always open to revision. Other explanations may account for the same results, and additional or contradictory evidence may emerge over time.
We can never 100% prove the alternative hypothesis. Instead, we see if we can disprove, or reject the null hypothesis.
If we reject the null hypothesis, this doesn’t mean that our alternative hypothesis is correct but does support the alternative/experimental hypothesis.
Upon analysis of the results, an alternative hypothesis can be rejected or supported, but it can never be proven to be correct. We must avoid any reference to results proving a theory as this implies 100% certainty, and there is always a chance that evidence may exist which could refute a theory.
How to Write a Hypothesis
- Identify variables . The researcher manipulates the independent variable and the dependent variable is the measured outcome.
- Operationalized the variables being investigated . Operationalization of a hypothesis refers to the process of making the variables physically measurable or testable, e.g. if you are about to study aggression, you might count the number of punches given by participants.
- Decide on a direction for your prediction . If there is evidence in the literature to support a specific effect of the independent variable on the dependent variable, write a directional (one-tailed) hypothesis. If there are limited or ambiguous findings in the literature regarding the effect of the independent variable on the dependent variable, write a non-directional (two-tailed) hypothesis.
- Make it Testable : Ensure your hypothesis can be tested through experimentation or observation. It should be possible to prove it false (principle of falsifiability).
- Clear & concise language . A strong hypothesis is concise (typically one to two sentences long), and formulated using clear and straightforward language, ensuring it’s easily understood and testable.
Consider a hypothesis many teachers might subscribe to: students work better on Monday morning than on Friday afternoon (IV=Day, DV= Standard of work).
Now, if we decide to study this by giving the same group of students a lesson on a Monday morning and a Friday afternoon and then measuring their immediate recall of the material covered in each session, we would end up with the following:
- The alternative hypothesis states that students will recall significantly more information on a Monday morning than on a Friday afternoon.
- The null hypothesis states that there will be no significant difference in the amount recalled on a Monday morning compared to a Friday afternoon. Any difference will be due to chance or confounding factors.
More Examples
- Memory : Participants exposed to classical music during study sessions will recall more items from a list than those who studied in silence.
- Social Psychology : Individuals who frequently engage in social media use will report higher levels of perceived social isolation compared to those who use it infrequently.
- Developmental Psychology : Children who engage in regular imaginative play have better problem-solving skills than those who don’t.
- Clinical Psychology : Cognitive-behavioral therapy will be more effective in reducing symptoms of anxiety over a 6-month period compared to traditional talk therapy.
- Cognitive Psychology : Individuals who multitask between various electronic devices will have shorter attention spans on focused tasks than those who single-task.
- Health Psychology : Patients who practice mindfulness meditation will experience lower levels of chronic pain compared to those who don’t meditate.
- Organizational Psychology : Employees in open-plan offices will report higher levels of stress than those in private offices.
- Behavioral Psychology : Rats rewarded with food after pressing a lever will press it more frequently than rats who receive no reward.

Related Articles
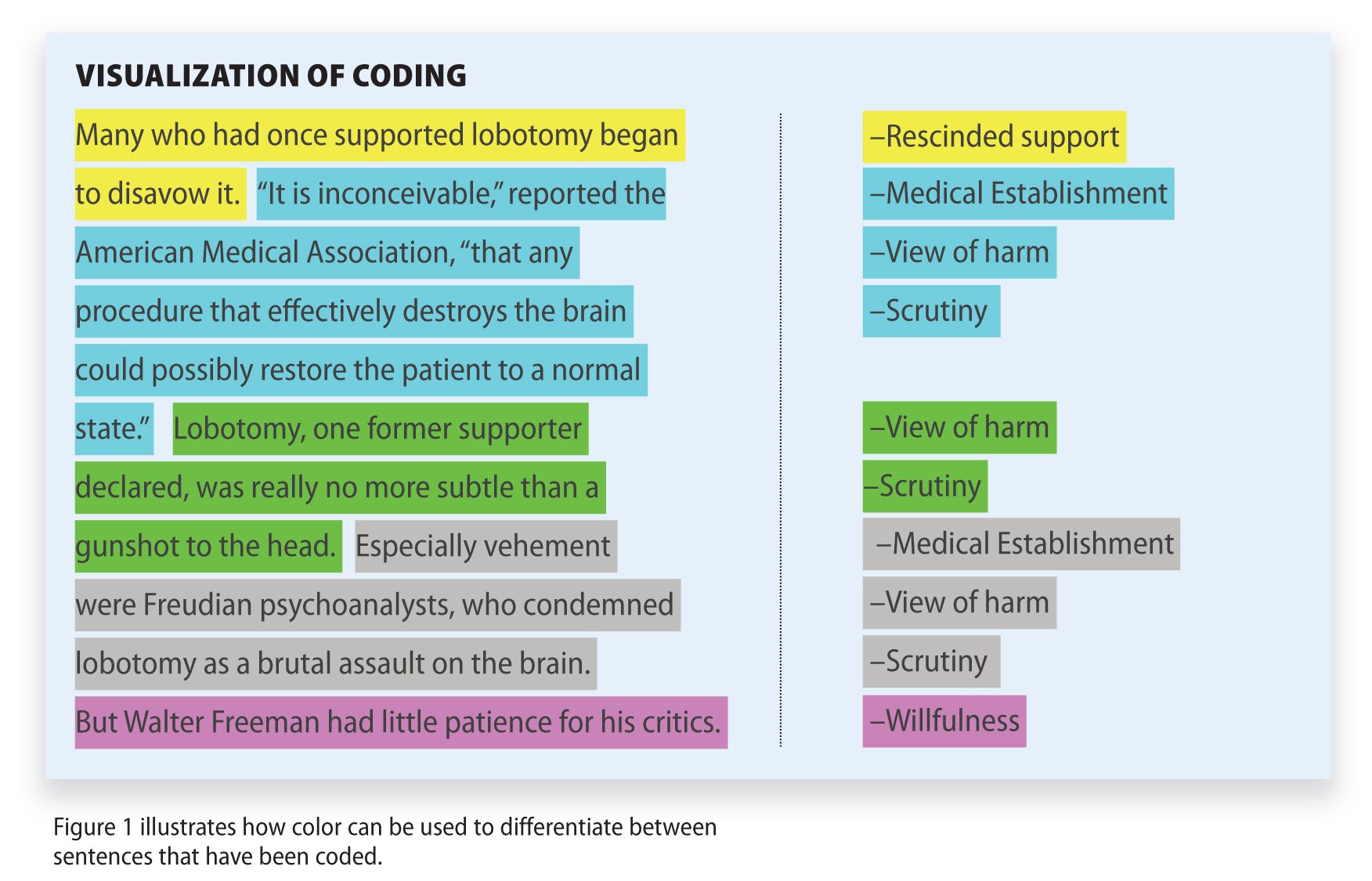
Research Methodology
Qualitative Data Coding
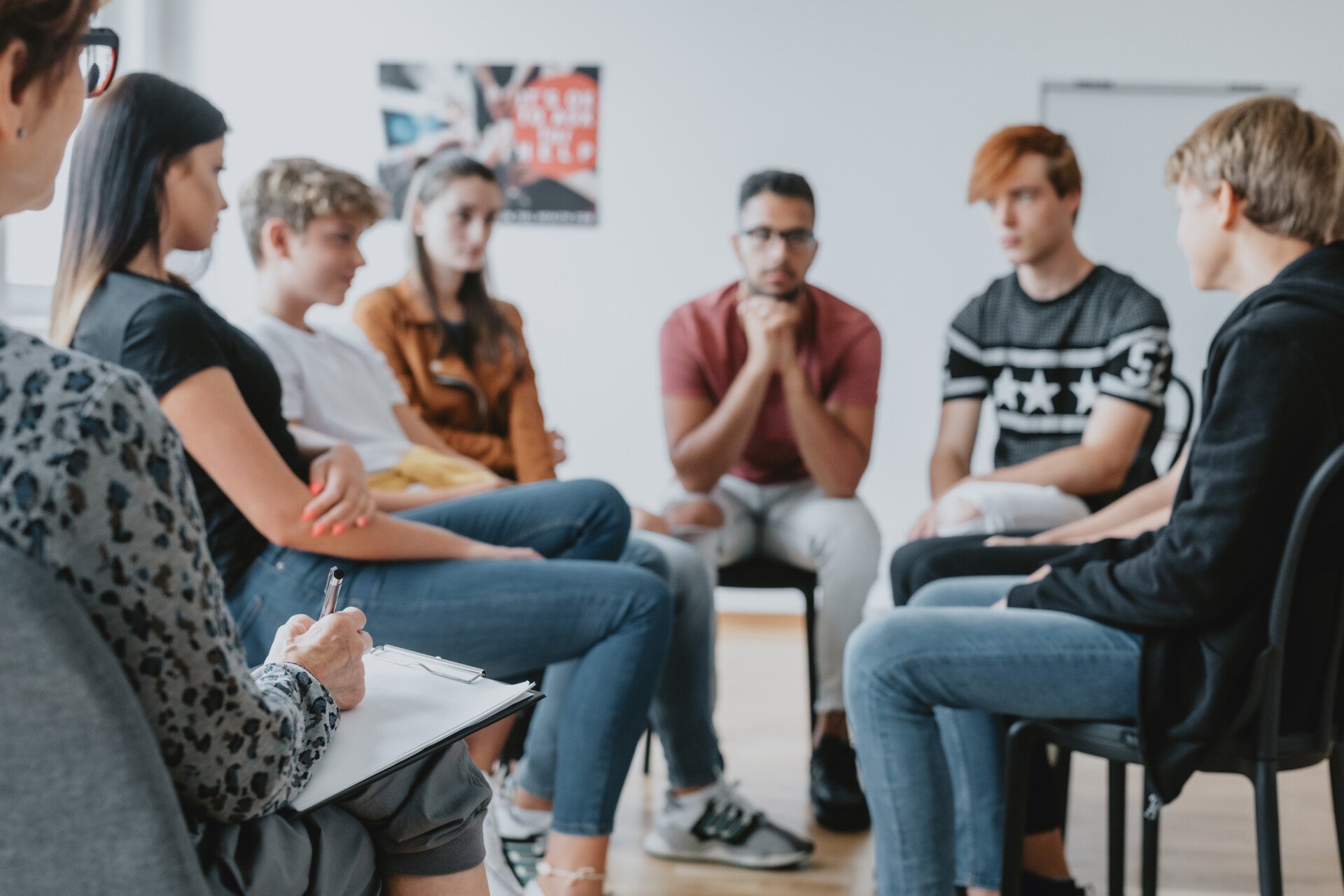
What Is a Focus Group?
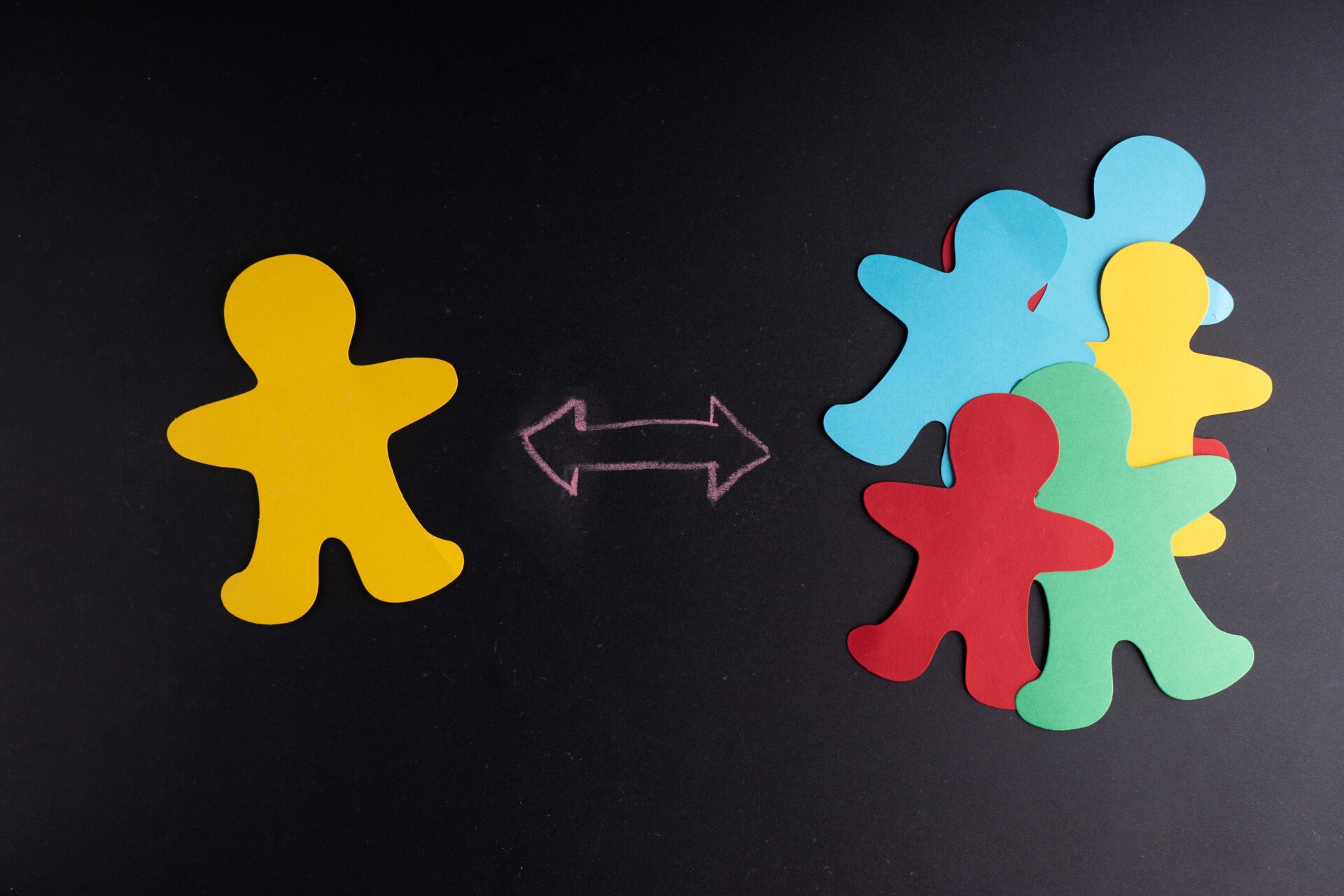
Cross-Cultural Research Methodology In Psychology
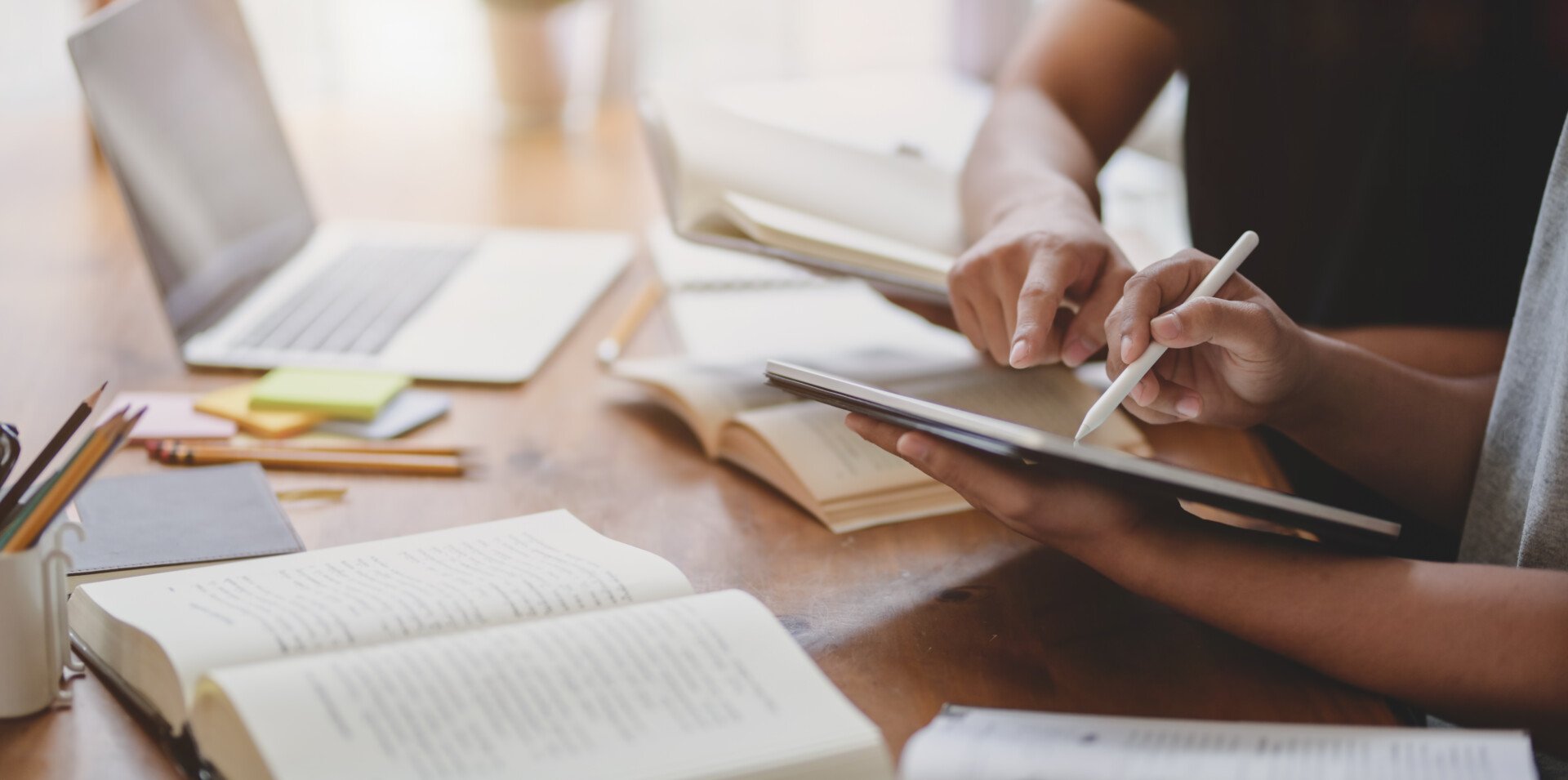
What Is Internal Validity In Research?
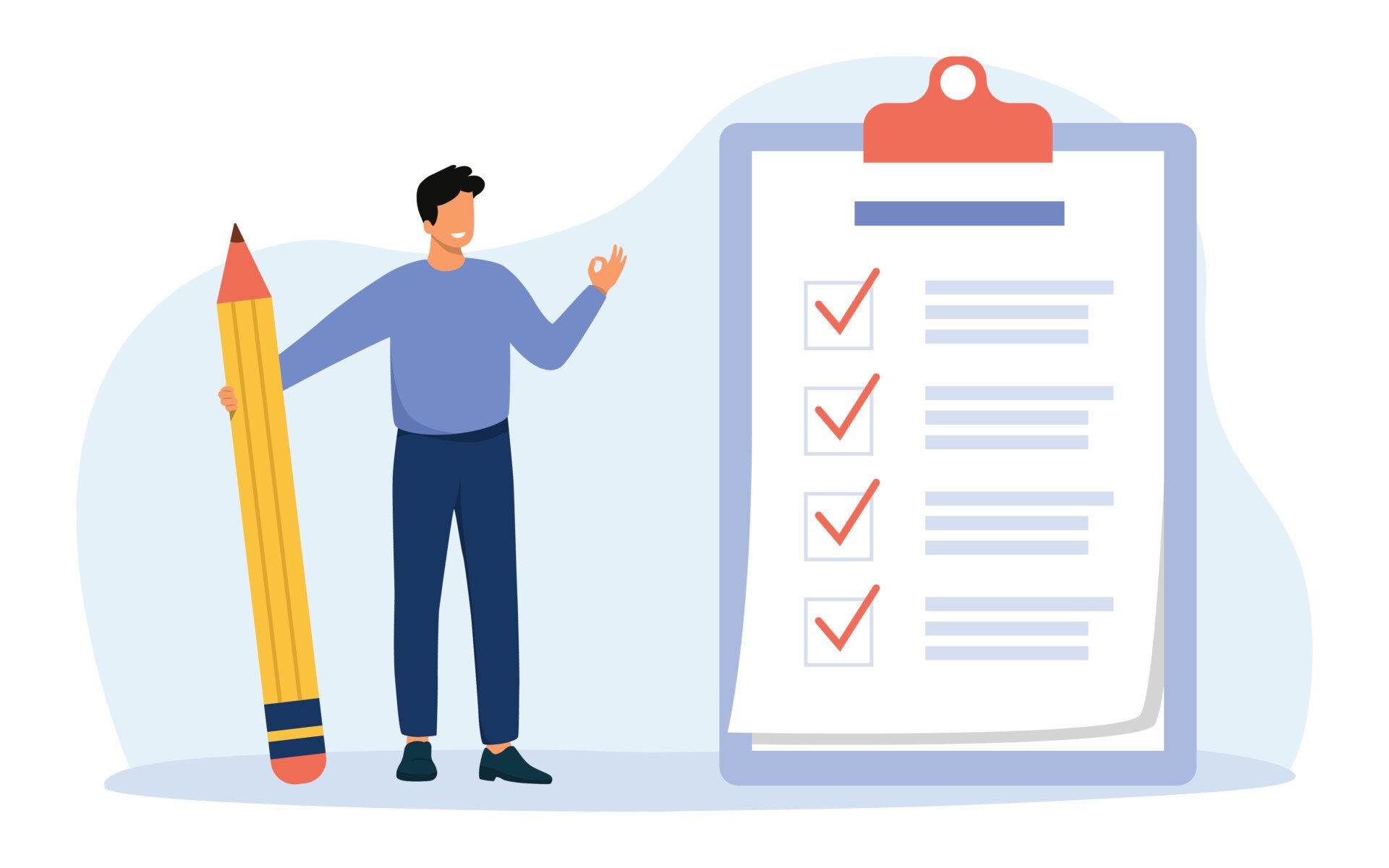
Research Methodology , Statistics
What Is Face Validity In Research? Importance & How To Measure
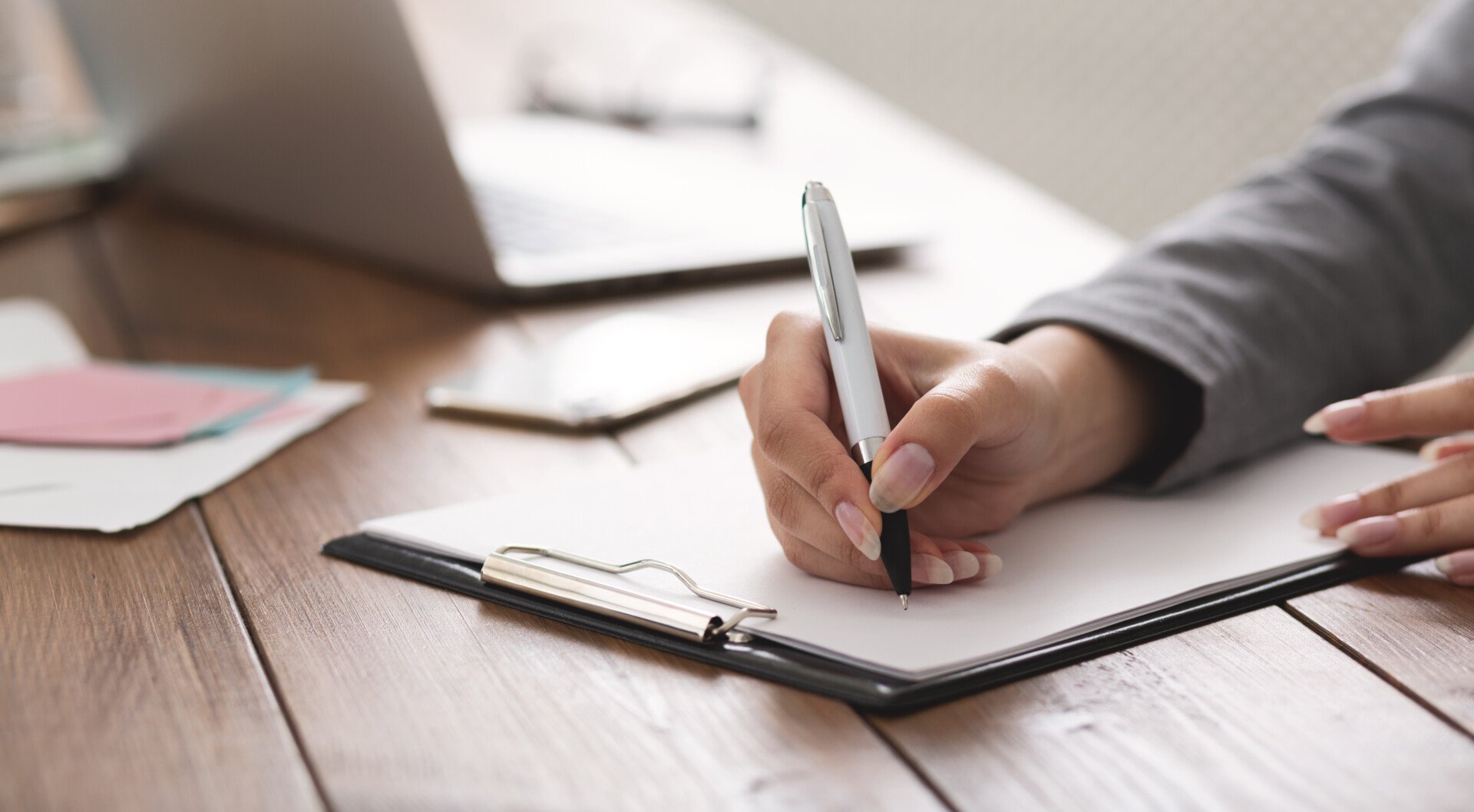
Criterion Validity: Definition & Examples

How to Develop a Good Research Hypothesis
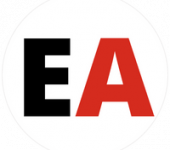
The story of a research study begins by asking a question. Researchers all around the globe are asking curious questions and formulating research hypothesis. However, whether the research study provides an effective conclusion depends on how well one develops a good research hypothesis. Research hypothesis examples could help researchers get an idea as to how to write a good research hypothesis.
This blog will help you understand what is a research hypothesis, its characteristics and, how to formulate a research hypothesis
Table of Contents
What is Hypothesis?
Hypothesis is an assumption or an idea proposed for the sake of argument so that it can be tested. It is a precise, testable statement of what the researchers predict will be outcome of the study. Hypothesis usually involves proposing a relationship between two variables: the independent variable (what the researchers change) and the dependent variable (what the research measures).
What is a Research Hypothesis?
Research hypothesis is a statement that introduces a research question and proposes an expected result. It is an integral part of the scientific method that forms the basis of scientific experiments. Therefore, you need to be careful and thorough when building your research hypothesis. A minor flaw in the construction of your hypothesis could have an adverse effect on your experiment. In research, there is a convention that the hypothesis is written in two forms, the null hypothesis, and the alternative hypothesis (called the experimental hypothesis when the method of investigation is an experiment).
Characteristics of a Good Research Hypothesis
As the hypothesis is specific, there is a testable prediction about what you expect to happen in a study. You may consider drawing hypothesis from previously published research based on the theory.
A good research hypothesis involves more effort than just a guess. In particular, your hypothesis may begin with a question that could be further explored through background research.
To help you formulate a promising research hypothesis, you should ask yourself the following questions:
- Is the language clear and focused?
- What is the relationship between your hypothesis and your research topic?
- Is your hypothesis testable? If yes, then how?
- What are the possible explanations that you might want to explore?
- Does your hypothesis include both an independent and dependent variable?
- Can you manipulate your variables without hampering the ethical standards?
- Does your research predict the relationship and outcome?
- Is your research simple and concise (avoids wordiness)?
- Is it clear with no ambiguity or assumptions about the readers’ knowledge
- Is your research observable and testable results?
- Is it relevant and specific to the research question or problem?
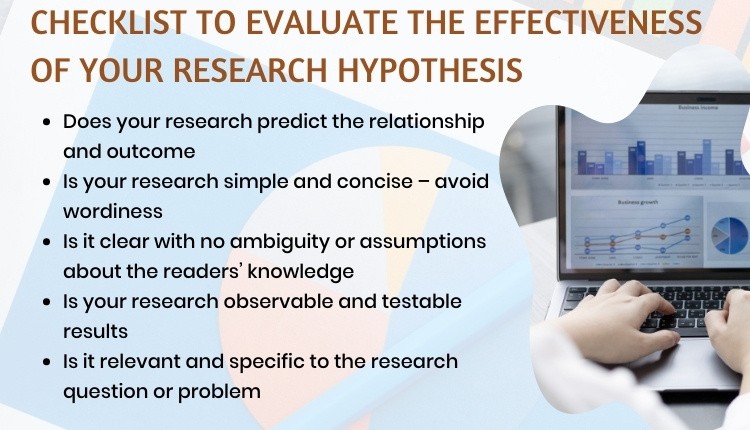
The questions listed above can be used as a checklist to make sure your hypothesis is based on a solid foundation. Furthermore, it can help you identify weaknesses in your hypothesis and revise it if necessary.
Source: Educational Hub
How to formulate a research hypothesis.
A testable hypothesis is not a simple statement. It is rather an intricate statement that needs to offer a clear introduction to a scientific experiment, its intentions, and the possible outcomes. However, there are some important things to consider when building a compelling hypothesis.
1. State the problem that you are trying to solve.
Make sure that the hypothesis clearly defines the topic and the focus of the experiment.
2. Try to write the hypothesis as an if-then statement.
Follow this template: If a specific action is taken, then a certain outcome is expected.
3. Define the variables
Independent variables are the ones that are manipulated, controlled, or changed. Independent variables are isolated from other factors of the study.
Dependent variables , as the name suggests are dependent on other factors of the study. They are influenced by the change in independent variable.
4. Scrutinize the hypothesis
Evaluate assumptions, predictions, and evidence rigorously to refine your understanding.
Types of Research Hypothesis
The types of research hypothesis are stated below:
1. Simple Hypothesis
It predicts the relationship between a single dependent variable and a single independent variable.
2. Complex Hypothesis
It predicts the relationship between two or more independent and dependent variables.
3. Directional Hypothesis
It specifies the expected direction to be followed to determine the relationship between variables and is derived from theory. Furthermore, it implies the researcher’s intellectual commitment to a particular outcome.
4. Non-directional Hypothesis
It does not predict the exact direction or nature of the relationship between the two variables. The non-directional hypothesis is used when there is no theory involved or when findings contradict previous research.
5. Associative and Causal Hypothesis
The associative hypothesis defines interdependency between variables. A change in one variable results in the change of the other variable. On the other hand, the causal hypothesis proposes an effect on the dependent due to manipulation of the independent variable.
6. Null Hypothesis
Null hypothesis states a negative statement to support the researcher’s findings that there is no relationship between two variables. There will be no changes in the dependent variable due the manipulation of the independent variable. Furthermore, it states results are due to chance and are not significant in terms of supporting the idea being investigated.
7. Alternative Hypothesis
It states that there is a relationship between the two variables of the study and that the results are significant to the research topic. An experimental hypothesis predicts what changes will take place in the dependent variable when the independent variable is manipulated. Also, it states that the results are not due to chance and that they are significant in terms of supporting the theory being investigated.
Research Hypothesis Examples of Independent and Dependent Variables
Research Hypothesis Example 1 The greater number of coal plants in a region (independent variable) increases water pollution (dependent variable). If you change the independent variable (building more coal factories), it will change the dependent variable (amount of water pollution).
Research Hypothesis Example 2 What is the effect of diet or regular soda (independent variable) on blood sugar levels (dependent variable)? If you change the independent variable (the type of soda you consume), it will change the dependent variable (blood sugar levels)
You should not ignore the importance of the above steps. The validity of your experiment and its results rely on a robust testable hypothesis. Developing a strong testable hypothesis has few advantages, it compels us to think intensely and specifically about the outcomes of a study. Consequently, it enables us to understand the implication of the question and the different variables involved in the study. Furthermore, it helps us to make precise predictions based on prior research. Hence, forming a hypothesis would be of great value to the research. Here are some good examples of testable hypotheses.
More importantly, you need to build a robust testable research hypothesis for your scientific experiments. A testable hypothesis is a hypothesis that can be proved or disproved as a result of experimentation.
Importance of a Testable Hypothesis
To devise and perform an experiment using scientific method, you need to make sure that your hypothesis is testable. To be considered testable, some essential criteria must be met:
- There must be a possibility to prove that the hypothesis is true.
- There must be a possibility to prove that the hypothesis is false.
- The results of the hypothesis must be reproducible.
Without these criteria, the hypothesis and the results will be vague. As a result, the experiment will not prove or disprove anything significant.
What are your experiences with building hypotheses for scientific experiments? What challenges did you face? How did you overcome these challenges? Please share your thoughts with us in the comments section.
Frequently Asked Questions
The steps to write a research hypothesis are: 1. Stating the problem: Ensure that the hypothesis defines the research problem 2. Writing a hypothesis as an 'if-then' statement: Include the action and the expected outcome of your study by following a ‘if-then’ structure. 3. Defining the variables: Define the variables as Dependent or Independent based on their dependency to other factors. 4. Scrutinizing the hypothesis: Identify the type of your hypothesis
Hypothesis testing is a statistical tool which is used to make inferences about a population data to draw conclusions for a particular hypothesis.
Hypothesis in statistics is a formal statement about the nature of a population within a structured framework of a statistical model. It is used to test an existing hypothesis by studying a population.
Research hypothesis is a statement that introduces a research question and proposes an expected result. It forms the basis of scientific experiments.
The different types of hypothesis in research are: • Null hypothesis: Null hypothesis is a negative statement to support the researcher’s findings that there is no relationship between two variables. • Alternate hypothesis: Alternate hypothesis predicts the relationship between the two variables of the study. • Directional hypothesis: Directional hypothesis specifies the expected direction to be followed to determine the relationship between variables. • Non-directional hypothesis: Non-directional hypothesis does not predict the exact direction or nature of the relationship between the two variables. • Simple hypothesis: Simple hypothesis predicts the relationship between a single dependent variable and a single independent variable. • Complex hypothesis: Complex hypothesis predicts the relationship between two or more independent and dependent variables. • Associative and casual hypothesis: Associative and casual hypothesis predicts the relationship between two or more independent and dependent variables. • Empirical hypothesis: Empirical hypothesis can be tested via experiments and observation. • Statistical hypothesis: A statistical hypothesis utilizes statistical models to draw conclusions about broader populations.

Wow! You really simplified your explanation that even dummies would find it easy to comprehend. Thank you so much.
Thanks a lot for your valuable guidance.
I enjoy reading the post. Hypotheses are actually an intrinsic part in a study. It bridges the research question and the methodology of the study.
Useful piece!
This is awesome.Wow.
It very interesting to read the topic, can you guide me any specific example of hypothesis process establish throw the Demand and supply of the specific product in market
Nicely explained
It is really a useful for me Kindly give some examples of hypothesis
It was a well explained content ,can you please give me an example with the null and alternative hypothesis illustrated
clear and concise. thanks.
So Good so Amazing
Good to learn
Thanks a lot for explaining to my level of understanding
Explained well and in simple terms. Quick read! Thank you
It awesome. It has really positioned me in my research project
Rate this article Cancel Reply
Your email address will not be published.
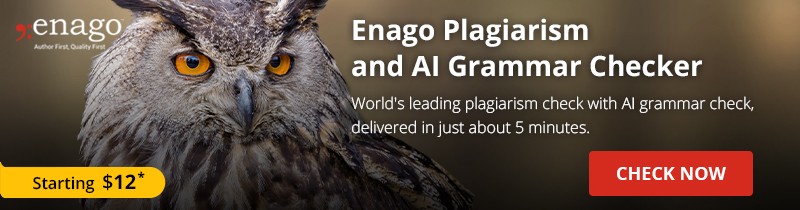
Enago Academy's Most Popular Articles
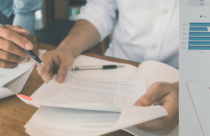
- Reporting Research
Choosing the Right Analytical Approach: Thematic analysis vs. content analysis for data interpretation
In research, choosing the right approach to understand data is crucial for deriving meaningful insights.…
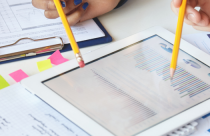
Comparing Cross Sectional and Longitudinal Studies: 5 steps for choosing the right approach
The process of choosing the right research design can put ourselves at the crossroads of…
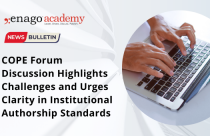
- Industry News
COPE Forum Discussion Highlights Challenges and Urges Clarity in Institutional Authorship Standards
The COPE forum discussion held in December 2023 initiated with a fundamental question — is…
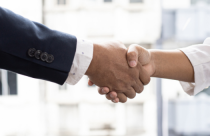
- Career Corner
Unlocking the Power of Networking in Academic Conferences
Embarking on your first academic conference experience? Fear not, we got you covered! Academic conferences…
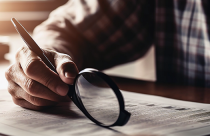
Research Recommendations – Guiding policy-makers for evidence-based decision making
Research recommendations play a crucial role in guiding scholars and researchers toward fruitful avenues of…
Choosing the Right Analytical Approach: Thematic analysis vs. content analysis for…
Comparing Cross Sectional and Longitudinal Studies: 5 steps for choosing the right…
How to Design Effective Research Questionnaires for Robust Findings

Sign-up to read more
Subscribe for free to get unrestricted access to all our resources on research writing and academic publishing including:
- 2000+ blog articles
- 50+ Webinars
- 10+ Expert podcasts
- 50+ Infographics
- 10+ Checklists
- Research Guides
We hate spam too. We promise to protect your privacy and never spam you.
I am looking for Editing/ Proofreading services for my manuscript Tentative date of next journal submission:
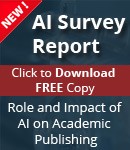
As a researcher, what do you consider most when choosing an image manipulation detector?
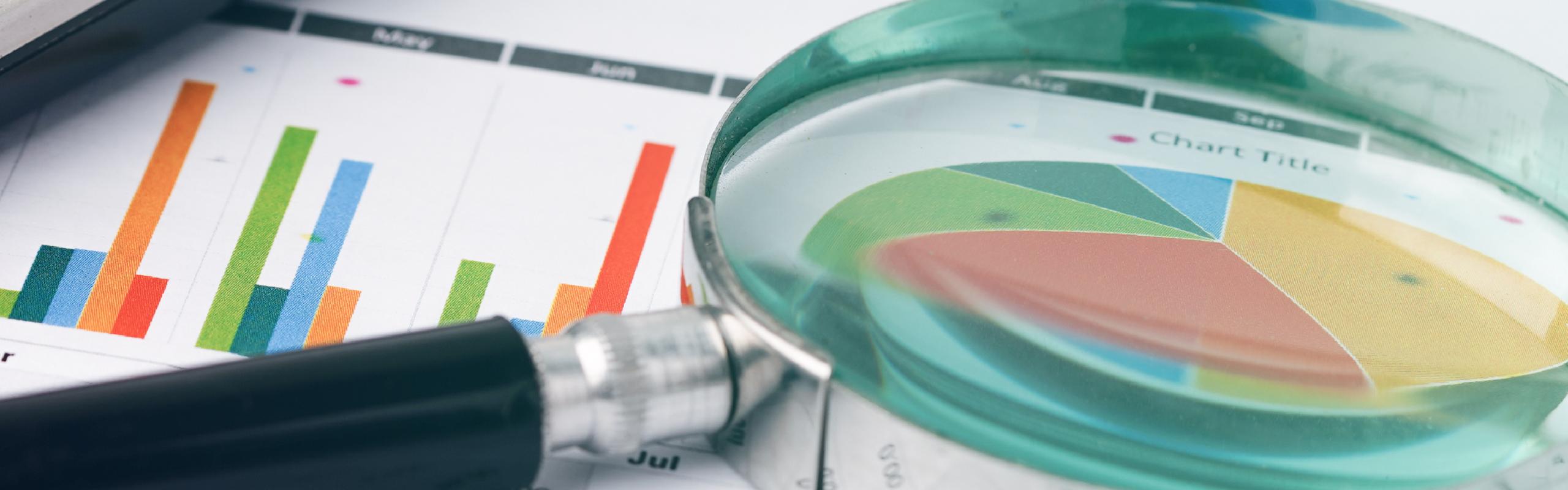
User Preferences
Content preview.
Arcu felis bibendum ut tristique et egestas quis:
- Ut enim ad minim veniam, quis nostrud exercitation ullamco laboris
- Duis aute irure dolor in reprehenderit in voluptate
- Excepteur sint occaecat cupidatat non proident
Keyboard Shortcuts
6a.2 - steps for hypothesis tests, the logic of hypothesis testing section .
A hypothesis, in statistics, is a statement about a population parameter, where this statement typically is represented by some specific numerical value. In testing a hypothesis, we use a method where we gather data in an effort to gather evidence about the hypothesis.
How do we decide whether to reject the null hypothesis?
- If the sample data are consistent with the null hypothesis, then we do not reject it.
- If the sample data are inconsistent with the null hypothesis, but consistent with the alternative, then we reject the null hypothesis and conclude that the alternative hypothesis is true.
Six Steps for Hypothesis Tests Section
In hypothesis testing, there are certain steps one must follow. Below these are summarized into six such steps to conducting a test of a hypothesis.
- Set up the hypotheses and check conditions : Each hypothesis test includes two hypotheses about the population. One is the null hypothesis, notated as \(H_0 \), which is a statement of a particular parameter value. This hypothesis is assumed to be true until there is evidence to suggest otherwise. The second hypothesis is called the alternative, or research hypothesis, notated as \(H_a \). The alternative hypothesis is a statement of a range of alternative values in which the parameter may fall. One must also check that any conditions (assumptions) needed to run the test have been satisfied e.g. normality of data, independence, and number of success and failure outcomes.
- Decide on the significance level, \(\alpha \): This value is used as a probability cutoff for making decisions about the null hypothesis. This alpha value represents the probability we are willing to place on our test for making an incorrect decision in regards to rejecting the null hypothesis. The most common \(\alpha \) value is 0.05 or 5%. Other popular choices are 0.01 (1%) and 0.1 (10%).
- Calculate the test statistic: Gather sample data and calculate a test statistic where the sample statistic is compared to the parameter value. The test statistic is calculated under the assumption the null hypothesis is true and incorporates a measure of standard error and assumptions (conditions) related to the sampling distribution.
- Calculate probability value (p-value), or find the rejection region: A p-value is found by using the test statistic to calculate the probability of the sample data producing such a test statistic or one more extreme. The rejection region is found by using alpha to find a critical value; the rejection region is the area that is more extreme than the critical value. We discuss the p-value and rejection region in more detail in the next section.
- Make a decision about the null hypothesis: In this step, we decide to either reject the null hypothesis or decide to fail to reject the null hypothesis. Notice we do not make a decision where we will accept the null hypothesis.
- State an overall conclusion : Once we have found the p-value or rejection region, and made a statistical decision about the null hypothesis (i.e. we will reject the null or fail to reject the null), we then want to summarize our results into an overall conclusion for our test.
We will follow these six steps for the remainder of this Lesson. In the future Lessons, the steps will be followed but may not be explained explicitly.
Step 1 is a very important step to set up correctly. If your hypotheses are incorrect, your conclusion will be incorrect. In this next section, we practice with Step 1 for the one sample situations.
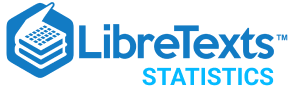
- school Campus Bookshelves
- menu_book Bookshelves
- perm_media Learning Objects
- login Login
- how_to_reg Request Instructor Account
- hub Instructor Commons
Margin Size
- Download Page (PDF)
- Download Full Book (PDF)
- Periodic Table
- Physics Constants
- Scientific Calculator
- Reference & Cite
- Tools expand_more
- Readability
selected template will load here
This action is not available.
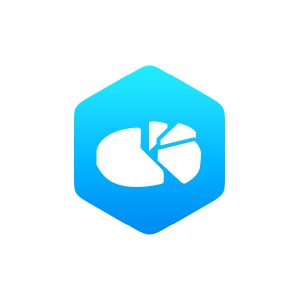
11.6: Reporting the Results of a Hypothesis Test
- Last updated
- Save as PDF
- Page ID 4012
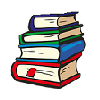
- Danielle Navarro
- University of New South Wales
\( \newcommand{\vecs}[1]{\overset { \scriptstyle \rightharpoonup} {\mathbf{#1}} } \)
\( \newcommand{\vecd}[1]{\overset{-\!-\!\rightharpoonup}{\vphantom{a}\smash {#1}}} \)
\( \newcommand{\id}{\mathrm{id}}\) \( \newcommand{\Span}{\mathrm{span}}\)
( \newcommand{\kernel}{\mathrm{null}\,}\) \( \newcommand{\range}{\mathrm{range}\,}\)
\( \newcommand{\RealPart}{\mathrm{Re}}\) \( \newcommand{\ImaginaryPart}{\mathrm{Im}}\)
\( \newcommand{\Argument}{\mathrm{Arg}}\) \( \newcommand{\norm}[1]{\| #1 \|}\)
\( \newcommand{\inner}[2]{\langle #1, #2 \rangle}\)
\( \newcommand{\Span}{\mathrm{span}}\)
\( \newcommand{\id}{\mathrm{id}}\)
\( \newcommand{\kernel}{\mathrm{null}\,}\)
\( \newcommand{\range}{\mathrm{range}\,}\)
\( \newcommand{\RealPart}{\mathrm{Re}}\)
\( \newcommand{\ImaginaryPart}{\mathrm{Im}}\)
\( \newcommand{\Argument}{\mathrm{Arg}}\)
\( \newcommand{\norm}[1]{\| #1 \|}\)
\( \newcommand{\Span}{\mathrm{span}}\) \( \newcommand{\AA}{\unicode[.8,0]{x212B}}\)
\( \newcommand{\vectorA}[1]{\vec{#1}} % arrow\)
\( \newcommand{\vectorAt}[1]{\vec{\text{#1}}} % arrow\)
\( \newcommand{\vectorB}[1]{\overset { \scriptstyle \rightharpoonup} {\mathbf{#1}} } \)
\( \newcommand{\vectorC}[1]{\textbf{#1}} \)
\( \newcommand{\vectorD}[1]{\overrightarrow{#1}} \)
\( \newcommand{\vectorDt}[1]{\overrightarrow{\text{#1}}} \)
\( \newcommand{\vectE}[1]{\overset{-\!-\!\rightharpoonup}{\vphantom{a}\smash{\mathbf {#1}}}} \)
When writing up the results of a hypothesis test, there’s usually several pieces of information that you need to report, but it varies a fair bit from test to test. Throughout the rest of the book I’ll spend a little time talking about how to report the results of different tests (see Section 12.1.9 for a particularly detailed example), so that you can get a feel for how it’s usually done. However, regardless of what test you’re doing, the one thing that you always have to do is say something about the p value, and whether or not the outcome was significant.
The fact that you have to do this is unsurprising; it’s the whole point of doing the test. What might be surprising is the fact that there is some contention over exactly how you’re supposed to do it. Leaving aside those people who completely disagree with the entire framework underpinning null hypothesis testing, there’s a certain amount of tension that exists regarding whether or not to report the exact p value that you obtained, or if you should state only that p<α for a significance level that you chose in advance (e.g., p<.05).
To see why this is an issue, the key thing to recognise is that p values are terribly convenient. In practice, the fact that we can compute a p value means that we don’t actually have to specify any α level at all in order to run the test. Instead, what you can do is calculate your p value and interpret it directly: if you get p=.062, then it means that you’d have to be willing to tolerate a Type I error rate of 6.2% to justify rejecting the null. If you personally find 6.2% intolerable, then you retain the null. Therefore, the argument goes, why don’t we just report the actual p value and let the reader make up their own minds about what an acceptable Type I error rate is? This approach has the big advantage of “softening” the decision making process – in fact, if you accept the Neyman definition of the p value, that’s the whole point of the p value. We no longer have a fixed significance level of α=.05 as a bright line separating “accept” from “reject” decisions; and this removes the rather pathological problem of being forced to treat p=.051 in a fundamentally different way to p=.049.
This flexibility is both the advantage and the disadvantage to the p value. The reason why a lot of people don’t like the idea of reporting an exact p value is that it gives the researcher a bit too much freedom. In particular, it lets you change your mind about what error tolerance you’re willing to put up with after you look at the data. For instance, consider my ESP experiment. Suppose I ran my test, and ended up with a p value of .09. Should I accept or reject? Now, to be honest, I haven’t yet bothered to think about what level of Type I error I’m “really” willing to accept. I don’t have an opinion on that topic. But I do have an opinion about whether or not ESP exists, and I definitely have an opinion about whether my research should be published in a reputable scientific journal. And amazingly, now that I’ve looked at the data I’m starting to think that a 9% error rate isn’t so bad, especially when compared to how annoying it would be to have to admit to the world that my experiment has failed. So, to avoid looking like I just made it up after the fact, I now say that my α is .1: a 10% type I error rate isn’t too bad, and at that level my test is significant! I win.
In other words, the worry here is that I might have the best of intentions, and be the most honest of people, but the temptation to just “shade” things a little bit here and there is really, really strong. As anyone who has ever run an experiment can attest, it’s a long and difficult process, and you often get very attached to your hypotheses. It’s hard to let go and admit the experiment didn’t find what you wanted it to find. And that’s the danger here. If we use the “raw” p-value, people will start interpreting the data in terms of what they want to believe, not what the data are actually saying… and if we allow that, well, why are we bothering to do science at all? Why not let everyone believe whatever they like about anything, regardless of what the facts are? Okay, that’s a bit extreme, but that’s where the worry comes from. According to this view, you really must specify your α value in advance, and then only report whether the test was significant or not. It’s the only way to keep ourselves honest.
proposed solutions
In practice, it’s pretty rare for a researcher to specify a single α level ahead of time. Instead, the convention is that scientists rely on three standard significance levels: .05, .01 and .001. When reporting your results, you indicate which (if any) of these significance levels allow you to reject the null hypothesis. This is summarised in Table 11.1. This allows us to soften the decision rule a little bit, since p<.01 implies that the data meet a stronger evidentiary standard than p<.05 would. Nevertheless, since these levels are fixed in advance by convention, it does prevent people choosing their α level after looking at the data.
Table 11.1: A commonly adopted convention for reporting p values: in many places it is conventional to report one of four different things (e.g., p<.05) as shown below. I’ve included the “significance stars” notation (i.e., a * indicates p<.05) because you sometimes see this notation produced by statistical software. It’s also worth noting that some people will write n.s. (not significant) rather than p>.05.
Nevertheless, quite a lot of people still prefer to report exact p values. To many people, the advantage of allowing the reader to make up their own mind about how to interpret p=.06 outweighs any disadvantages. In practice, however, even among those researchers who prefer exact p values it is quite common to just write p<.001 instead of reporting an exact value for small p. This is in part because a lot of software doesn’t actually print out the p value when it’s that small (e.g., SPSS just writes p=.000 whenever p<.001), and in part because a very small p value can be kind of misleading. The human mind sees a number like .0000000001 and it’s hard to suppress the gut feeling that the evidence in favour of the alternative hypothesis is a near certainty. In practice however, this is usually wrong. Life is a big, messy, complicated thing: and every statistical test ever invented relies on simplifications, approximations and assumptions. As a consequence, it’s probably not reasonable to walk away from any statistical analysis with a feeling of confidence stronger than p<.001 implies. In other words, p<.001 is really code for “as far as this test is concerned, the evidence is overwhelming.”
In light of all this, you might be wondering exactly what you should do. There’s a fair bit of contradictory advice on the topic, with some people arguing that you should report the exact p value, and other people arguing that you should use the tiered approach illustrated in Table 11.1. As a result, the best advice I can give is to suggest that you look at papers/reports written in your field and see what the convention seems to be. If there doesn’t seem to be any consistent pattern, then use whichever method you prefer.
What is a scientific hypothesis?
It's the initial building block in the scientific method.
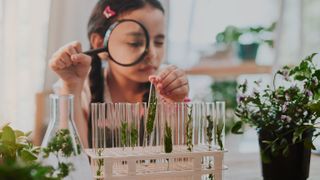
Hypothesis basics
What makes a hypothesis testable.
- Types of hypotheses
- Hypothesis versus theory
Additional resources
Bibliography.
A scientific hypothesis is a tentative, testable explanation for a phenomenon in the natural world. It's the initial building block in the scientific method . Many describe it as an "educated guess" based on prior knowledge and observation. While this is true, a hypothesis is more informed than a guess. While an "educated guess" suggests a random prediction based on a person's expertise, developing a hypothesis requires active observation and background research.
The basic idea of a hypothesis is that there is no predetermined outcome. For a solution to be termed a scientific hypothesis, it has to be an idea that can be supported or refuted through carefully crafted experimentation or observation. This concept, called falsifiability and testability, was advanced in the mid-20th century by Austrian-British philosopher Karl Popper in his famous book "The Logic of Scientific Discovery" (Routledge, 1959).
A key function of a hypothesis is to derive predictions about the results of future experiments and then perform those experiments to see whether they support the predictions.
A hypothesis is usually written in the form of an if-then statement, which gives a possibility (if) and explains what may happen because of the possibility (then). The statement could also include "may," according to California State University, Bakersfield .
Here are some examples of hypothesis statements:
- If garlic repels fleas, then a dog that is given garlic every day will not get fleas.
- If sugar causes cavities, then people who eat a lot of candy may be more prone to cavities.
- If ultraviolet light can damage the eyes, then maybe this light can cause blindness.
A useful hypothesis should be testable and falsifiable. That means that it should be possible to prove it wrong. A theory that can't be proved wrong is nonscientific, according to Karl Popper's 1963 book " Conjectures and Refutations ."
An example of an untestable statement is, "Dogs are better than cats." That's because the definition of "better" is vague and subjective. However, an untestable statement can be reworded to make it testable. For example, the previous statement could be changed to this: "Owning a dog is associated with higher levels of physical fitness than owning a cat." With this statement, the researcher can take measures of physical fitness from dog and cat owners and compare the two.
Types of scientific hypotheses
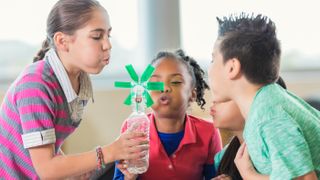
In an experiment, researchers generally state their hypotheses in two ways. The null hypothesis predicts that there will be no relationship between the variables tested, or no difference between the experimental groups. The alternative hypothesis predicts the opposite: that there will be a difference between the experimental groups. This is usually the hypothesis scientists are most interested in, according to the University of Miami .
For example, a null hypothesis might state, "There will be no difference in the rate of muscle growth between people who take a protein supplement and people who don't." The alternative hypothesis would state, "There will be a difference in the rate of muscle growth between people who take a protein supplement and people who don't."
If the results of the experiment show a relationship between the variables, then the null hypothesis has been rejected in favor of the alternative hypothesis, according to the book " Research Methods in Psychology " (BCcampus, 2015).
There are other ways to describe an alternative hypothesis. The alternative hypothesis above does not specify a direction of the effect, only that there will be a difference between the two groups. That type of prediction is called a two-tailed hypothesis. If a hypothesis specifies a certain direction — for example, that people who take a protein supplement will gain more muscle than people who don't — it is called a one-tailed hypothesis, according to William M. K. Trochim , a professor of Policy Analysis and Management at Cornell University.
Sometimes, errors take place during an experiment. These errors can happen in one of two ways. A type I error is when the null hypothesis is rejected when it is true. This is also known as a false positive. A type II error occurs when the null hypothesis is not rejected when it is false. This is also known as a false negative, according to the University of California, Berkeley .
A hypothesis can be rejected or modified, but it can never be proved correct 100% of the time. For example, a scientist can form a hypothesis stating that if a certain type of tomato has a gene for red pigment, that type of tomato will be red. During research, the scientist then finds that each tomato of this type is red. Though the findings confirm the hypothesis, there may be a tomato of that type somewhere in the world that isn't red. Thus, the hypothesis is true, but it may not be true 100% of the time.

Scientific theory vs. scientific hypothesis
The best hypotheses are simple. They deal with a relatively narrow set of phenomena. But theories are broader; they generally combine multiple hypotheses into a general explanation for a wide range of phenomena, according to the University of California, Berkeley . For example, a hypothesis might state, "If animals adapt to suit their environments, then birds that live on islands with lots of seeds to eat will have differently shaped beaks than birds that live on islands with lots of insects to eat." After testing many hypotheses like these, Charles Darwin formulated an overarching theory: the theory of evolution by natural selection.
"Theories are the ways that we make sense of what we observe in the natural world," Tanner said. "Theories are structures of ideas that explain and interpret facts."
- Read more about writing a hypothesis, from the American Medical Writers Association.
- Find out why a hypothesis isn't always necessary in science, from The American Biology Teacher.
- Learn about null and alternative hypotheses, from Prof. Essa on YouTube .
Encyclopedia Britannica. Scientific Hypothesis. Jan. 13, 2022. https://www.britannica.com/science/scientific-hypothesis
Karl Popper, "The Logic of Scientific Discovery," Routledge, 1959.
California State University, Bakersfield, "Formatting a testable hypothesis." https://www.csub.edu/~ddodenhoff/Bio100/Bio100sp04/formattingahypothesis.htm
Karl Popper, "Conjectures and Refutations," Routledge, 1963.
Price, P., Jhangiani, R., & Chiang, I., "Research Methods of Psychology — 2nd Canadian Edition," BCcampus, 2015.
University of Miami, "The Scientific Method" http://www.bio.miami.edu/dana/161/evolution/161app1_scimethod.pdf
William M.K. Trochim, "Research Methods Knowledge Base," https://conjointly.com/kb/hypotheses-explained/
University of California, Berkeley, "Multiple Hypothesis Testing and False Discovery Rate" https://www.stat.berkeley.edu/~hhuang/STAT141/Lecture-FDR.pdf
University of California, Berkeley, "Science at multiple levels" https://undsci.berkeley.edu/article/0_0_0/howscienceworks_19
Sign up for the Live Science daily newsletter now
Get the world’s most fascinating discoveries delivered straight to your inbox.
Iceland volcano eruption throws spectacular 160-foot-high wall of lava toward Grindavík
Earth from space: Ethereal algal vortex blooms at the heart of massive Baltic 'dead zone'
James Webb telescope discovers the 2 earliest galaxies in the known universe — and 1 is shockingly big
Most Popular
- 2 Alaska's rivers are turning bright orange and as acidic as vinegar as toxic metal escapes from melting permafrost
- 3 32 stunning photos of auroras seen from space
- 4 Things are finally looking up for the Voyager 1 interstellar spacecraft
- 5 Reaching absolute zero for quantum computing now much quicker thanks to breakthrough refrigerator design
- 2 32 optical illusions and why they trick your brain
- 3 Secrets of radioactive 'promethium' — a rare earth element with mysterious applications — uncovered after 80-year search
- 4 Auroras could paint Earth's skies again in early June. Here are the key nights to watch for.
- 5 Ramesses II's sarcophagus finally identified thanks to overlooked hieroglyphics
- Search Search Please fill out this field.
What Is Hypothesis Testing?
- How It Works
4 Step Process
The bottom line.
- Fundamental Analysis
Hypothesis Testing: 4 Steps and Example
:max_bytes(150000):strip_icc():format(webp)/ChristinaMajaski-5c9433ea46e0fb0001d880b1.jpeg)
Hypothesis testing, sometimes called significance testing, is an act in statistics whereby an analyst tests an assumption regarding a population parameter. The methodology employed by the analyst depends on the nature of the data used and the reason for the analysis.
Hypothesis testing is used to assess the plausibility of a hypothesis by using sample data. Such data may come from a larger population or a data-generating process. The word "population" will be used for both of these cases in the following descriptions.
Key Takeaways
- Hypothesis testing is used to assess the plausibility of a hypothesis by using sample data.
- The test provides evidence concerning the plausibility of the hypothesis, given the data.
- Statistical analysts test a hypothesis by measuring and examining a random sample of the population being analyzed.
- The four steps of hypothesis testing include stating the hypotheses, formulating an analysis plan, analyzing the sample data, and analyzing the result.
How Hypothesis Testing Works
In hypothesis testing, an analyst tests a statistical sample, intending to provide evidence on the plausibility of the null hypothesis. Statistical analysts measure and examine a random sample of the population being analyzed. All analysts use a random population sample to test two different hypotheses: the null hypothesis and the alternative hypothesis.
The null hypothesis is usually a hypothesis of equality between population parameters; e.g., a null hypothesis may state that the population mean return is equal to zero. The alternative hypothesis is effectively the opposite of a null hypothesis. Thus, they are mutually exclusive , and only one can be true. However, one of the two hypotheses will always be true.
The null hypothesis is a statement about a population parameter, such as the population mean, that is assumed to be true.
- State the hypotheses.
- Formulate an analysis plan, which outlines how the data will be evaluated.
- Carry out the plan and analyze the sample data.
- Analyze the results and either reject the null hypothesis, or state that the null hypothesis is plausible, given the data.
Example of Hypothesis Testing
If an individual wants to test that a penny has exactly a 50% chance of landing on heads, the null hypothesis would be that 50% is correct, and the alternative hypothesis would be that 50% is not correct. Mathematically, the null hypothesis is represented as Ho: P = 0.5. The alternative hypothesis is shown as "Ha" and is identical to the null hypothesis, except with the equal sign struck-through, meaning that it does not equal 50%.
A random sample of 100 coin flips is taken, and the null hypothesis is tested. If it is found that the 100 coin flips were distributed as 40 heads and 60 tails, the analyst would assume that a penny does not have a 50% chance of landing on heads and would reject the null hypothesis and accept the alternative hypothesis.
If there were 48 heads and 52 tails, then it is plausible that the coin could be fair and still produce such a result. In cases such as this where the null hypothesis is "accepted," the analyst states that the difference between the expected results (50 heads and 50 tails) and the observed results (48 heads and 52 tails) is "explainable by chance alone."
When Did Hypothesis Testing Begin?
Some statisticians attribute the first hypothesis tests to satirical writer John Arbuthnot in 1710, who studied male and female births in England after observing that in nearly every year, male births exceeded female births by a slight proportion. Arbuthnot calculated that the probability of this happening by chance was small, and therefore it was due to “divine providence.”
What are the Benefits of Hypothesis Testing?
Hypothesis testing helps assess the accuracy of new ideas or theories by testing them against data. This allows researchers to determine whether the evidence supports their hypothesis, helping to avoid false claims and conclusions. Hypothesis testing also provides a framework for decision-making based on data rather than personal opinions or biases. By relying on statistical analysis, hypothesis testing helps to reduce the effects of chance and confounding variables, providing a robust framework for making informed conclusions.
What are the Limitations of Hypothesis Testing?
Hypothesis testing relies exclusively on data and doesn’t provide a comprehensive understanding of the subject being studied. Additionally, the accuracy of the results depends on the quality of the available data and the statistical methods used. Inaccurate data or inappropriate hypothesis formulation may lead to incorrect conclusions or failed tests. Hypothesis testing can also lead to errors, such as analysts either accepting or rejecting a null hypothesis when they shouldn’t have. These errors may result in false conclusions or missed opportunities to identify significant patterns or relationships in the data.
Hypothesis testing refers to a statistical process that helps researchers determine the reliability of a study. By using a well-formulated hypothesis and set of statistical tests, individuals or businesses can make inferences about the population that they are studying and draw conclusions based on the data presented. All hypothesis testing methods have the same four-step process, which includes stating the hypotheses, formulating an analysis plan, analyzing the sample data, and analyzing the result.
Sage. " Introduction to Hypothesis Testing ," Page 4.
Elder Research. " Who Invented the Null Hypothesis? "
Formplus. " Hypothesis Testing: Definition, Uses, Limitations and Examples ."
:max_bytes(150000):strip_icc():format(webp)/shutterstock_67023106-5bfc2b9846e0fb005144dd87.jpg)
- Terms of Service
- Editorial Policy
- Privacy Policy
- Your Privacy Choices
New poll reveals how Trump trial verdict will impact 2024 election
A new national poll is the latest to indicate that regardless of whether former President Trump is found guilty or acquitted in his criminal trial, the verdict will not have a large impact on voters' perceptions in his 2024 election rematch with President Biden.
Two-thirds of registered voters nationwide questioned in a NPR/PBS NewsHour/Marist National Poll released on Thursday said a guilty verdict in the trial would make no difference to their vote in the presidential election. Seventeen percent said a conviction of Trump would make them less likely to vote for him and 15% said they'd be more inclined to support the former president at the ballot box.
If Trump is acquitted, three-quarters of those surveyed said it wouldn't impact their vote. Fourteen percent said a not guilty verdict would make them more likely to back Trump, and 9% said they'd be less likely to vote for the former president.
CLICK HERE FOR THE LATEST FOX NEWS DEVELOPMENTS IN THE TRUMP CRIMINAL TRIAL
Trump currently holds a slight edge over the president both in national polling and in public opinion surveys in most of the crucial battleground states that will likely decide their rematch.
But the pending verdict in the historic case – Trump is charged with 34 state felony charges in what is the first criminal trial in the nation's history for a former or current president – could impact what's shaping up to be a close contest with Biden.
READ ON THE FOX NEWS APP
Trump is charged with falsifying business records in relation to payments during the 2016 election that he made to Stormy Daniels to keep quiet about his alleged affair with the adult film actress. Trump’s former attorney, Michael Cohen, paid Daniels, whose real name is Stephanie Clifford, $130,000 in return for her silence about allegations of an affair with Trump in 2006. Prosecutors have argued that this amounted to illegally seeking to influence the 2016 election.
WARNING SIGNS FOR TRUMP AND BIDEN AS THEY CAREEN TOWARDS NEXT MONTH'S FIRST PRESIDENTIAL DEBATE
Both Cohen and Daniels testified for the prosecution and were grilled by Trump's attorneys during cross-examination in a case that has grabbed tons of attention on the cable news networks, online and on social media.
The former president has repeatedly denied falsifying business records as well as the alleged sexual encounter with Daniels, and he has repeatedly claimed, without providing evidence, that the case is a "SHAM TRIAL instigated and prosecuted directly from the inner halls of the White House and DOJ."
Trump has also been fined a couple of times and threatened with jail by the judge in the case for violating a gag order aimed at protecting witnesses and jurors from the former president's verbal attacks.
Veteran pollster Chris Anderson, a member of the Fox News Election Decision Team and the Democratic partner on the Fox News Poll, said he did not think "a guilty verdict would fundamentally change the landscape of the race."
Daron Shaw, a politics professor and chair at the University of Texas who also serves as a member of the Fox News Decision Team and the Republican partner on the Fox News Poll , noted that "prior to 2020, no one would have thought that a candidate could survive a criminal conviction."
"But times and circumstances have evolved. And while the specific findings of the jury could matter, I think there is a sense that a conviction in this case would not appreciably change the dynamics of the race," Shaw emphasized.
Both pointed to the fact that "attitudes are so set in concrete" regarding both the former Republican president and his Democratic successor in the White House.
The Marist Poll for NPR and PBS NewsHour was conducted May 21-23, with 1,122 registered voters nationwide. The survey's overall sampling error is plus or minus 3.7 percentage points.
Get the latest updates from the 2024 campaign trail, exclusive interviews and more at our Fox News Digital election hub.
Original article source: New poll reveals how Trump trial verdict will impact 2024 election

( Photo-illustration by Realtor.com; Source: Getty Images (3) )
Local Home Prices in Swing States Could Result in ‘Vote-Switching’ in 2024 Race
Home prices could play a subtle but important role in the 2024 presidential election, according to a recent first-of-its kind study.
The academic study, Housing Performance and the Electorate , analyzed home prices and election results at the county level for each of the six presidential elections from 2000 to 2020.
The authors found that local home price performance significantly affects voting in presidential elections at the county level. Counties with superior gains in home prices in the four years preceding an election were more likely to “vote-switch” to the incumbent party’s presidential candidate.
Conversely, counties with relatively inferior home price performance leading up to an election were more likely to flip their vote to support the candidate challenging the incumbent party.
In other words, quickly rising home prices tend to favor the incumbent president’s party, whether it be the Democrats or Republicans. The study found that the relationship is strongest in the years closest to an election, and that home prices were most influential in the small group of “swing counties” with a history of switching party preference.
University of Alabama Associate Professor of Finance Alan Tidwell , one of the study’s co-authors, explains that the logic driving this trend is simple: For most voters, their home represents their single largest asset.
“People feel more financially wealthy if they have a lot of housing equity, relative to lower housing equity,” he says. “How financially wealthy they feel really impacts their sense of financial and economic well-being.”
For the upcoming presidential election, the new finding suggests the outcome could be partly influenced by home prices in swing counties of the seven battleground states: Arizona, Georgia, Michigan, Nevada, North Carolina, Pennsylvania, and Wisconsin.
“In the swing counties, they care about this economic factor most, and real estate is one of the main drivers of household wealth,” says lead author Eren Cifci , an assistant professor of finance at Austin Peay State University in Tennessee. “So there may be many other factors that affect how people vote, but this definitely appears to be one of the factors influencing voters when they make their decisions.”
Explaining the 'homevoter hypothesis'
The study’s finding is an extension of the “homevoter hypothesis,” which holds that homeowners tend to vote in support of policies and candidates they believe will boost their home values.
While that phenomenon is well documented in local politics, where government policies have the clearest impact on home values, the new study is the first to show evidence of homevoter behavior in national elections.
The term “homevoter” was coined in 2001 by William A. Fischel , a now-retired economics professor at Dartmouth College and expert in local government and land use regulation.
Fischel conceived the homevoter hypothesis while serving on the local zoning board in Hannover, NH. Regularly, he would hear objections and concerns about zoning changes that seemed esoteric, and noticed that the complaints were always from homeowners.
Fischel says he came to realize that homeowners are essentially shareholders in their community, similar to owners of stock in a company—but that unlike corporate shareholders, they cannot easily diversify their portfolio or liquidate their holdings.
“It's people who are voting their homes, and that's actually an old concept in economics,” says Fischel. “But also, they're very risk-averse, because so much of their assets are stuck in one stock, in one place.”
Fischel says he was surprised by the recent study linking home prices and voting in national elections, since he had always viewed homevoting as primarily a local phenomenon.
“I can see, a little bit, what a presidential election might mean for home values. But it's so indirect, I was really quite surprised at the strength of the evidence,” he says. “How did they find such a strong mechanism? But I have no reason to doubt their evidence.”
For his part, Tidwell argues that the economy plays a major role in most presidential elections, and that rising home equity has a significant impact on how voters perceive the strength of the economy.
Even if local policies, such as zoning laws and public school funding, have a bigger direct impact on local home prices, national elections are where more voters take the opportunity to weigh in with their concern or satisfaction, he says.
“Local elections don't have big turnout, and they don't have big visibility, whereas the national election has a whole bunch more turnout and a whole lot more national media exposure, especially with talk of the economy,” says Tidwell.
Home prices play the biggest role in swing counties
To conduct their study, Cifci and Tidwell, with co-authors Sherwood Clements and Andres Jauregui , looked at the voting results for every county in the continental U.S. over the past six presidential elections.
Of those counties, 77% never changed their party preference, voting for either the Democrat or the Republican in every election since 2000, which was used as the base year for analyzing the 2004 election.
But 641 counties across the country–or 23%–switched their party vote at least once across the survey period, some as many as four times. In that subset of swing counties, home prices appeared to have the biggest impact on election results, according to the study.
In swing counties, for every 1% increase in home values over the four years preceding an election, the county was 0.36% more likely to vote for the incumbent party in the next election, the study found.
As well, the data showed that each 1% increase in home prices made the county 0.19% more likely to “flip” its vote to the incumbent party’s candidate. Those figures are after the study controlled for a variety of other factors that could sway elections, such as changes in demographics, the economy, and government benefits.
“The larger the return [on home values], the more likely you are to vote for the incumbent, or to flip for the incumbent,” explains Tidwell. “For every percent of positive return, there is a percentage increase in voting for the incumbent.”
What does it mean for 2024?
Home prices have risen rapidly across the country over the past four years, including in the seven swing states.
From March 2020 to March 2024, national home values rose 46.4%, according to the Freddie Mac Home Price Index. Of the swing states, North Carolina, Arizona, Georgia, and Wisconsin all outperformed the national average, with four-year price gains greater than 50%.
The study suggests that trend would tend broadly to favor the incumbent, President Joe Biden , as he seeks reelection, particularly in the areas that have seen the strongest home price gains. But the authors caution that their finding only demonstrates a statistical nudge in one direction or the other. They warn that there are many other variables at play in an election.
“It's just one of many factors,” Tidwell says of home price performance. “It's not really a forecast on its own.”
As well, voter turnout in counties that are reliably Democratic or Republican can be just as important to the state-level results in swing states as the marginal shifts in counties that flip from one party to another.
But in an election that is increasingly focused on the housing market, the new findings provide an interesting twist on the role of home prices in voter decision making.
Donald Trump , the presumptive Republican nominee, and his allies have recently levied attacks against Biden over rising home prices, pointing to the challenges raised for prospective first-time homebuyers.
“Under President Biden, home prices have risen almost 50%, making it nearly impossible for millennials to buy their first home and driving the American Dream further and further out of reach,” wrote Sen. Tim Scott , a South Carolina Republican and staunch Trump supporter, on the social media platform X.
On his own Truth Social platform, Trump himself recently wrote: “Crooked Joe has made it impossible for millions of Americans, especially YOUNG Americans, to buy a home.” (Conversely, Trump has also accused Biden of trying to “destroy your property values” by abolishing single-family zoning in the suburbs. The two arguments seem difficult to reconcile.)
It’s true that rising home prices, along with high mortgage rates, are key factors in a national housing crisis that has pushed ownership out of reach for many prospective homebuyers. But on the flip side, most voters are already homeowners. The U.S. homeownership rate is about 66%, and homeowners are significantly more likely to vote than renters.
For existing homeowners, rising home prices mean more equity and higher household net worth, the same as what rising stock prices mean for shareholders.
It suggests that for Republicans, attacking Biden over rising home prices might not carry the same weight with voters as criticism over inflation for goods such as gasoline and groceries.
“When you go to the grocery store or restaurant or the gas pump, I think maybe people feel a little bit different pain than if they own a house and they see their house price going up,” says Tidwell.
On the other hand, the study found evidence that, for swing counties, the economically rational choice might be to always flip to the non-incumbent party, which in 2024 would be the Republicans.
The study found that counties that flipped their vote to an incumbent party candidate were not rewarded with superior home price returns in the four years after the election.
However, counties that flipped to vote for the non-incumbent did experience “positive and significant post-election housing returns” if that candidate won. The authors speculate that this might be due to the winning party rewarding new supporters by increasing investment in those areas after regaining the White House.
“The counties that make the national results flip parties, they do well,” says Clements, a collegiate assistant professor of real estate at Virginia Tech. “Whatever counties voted for Biden last time and vote for Trump this time, if you believe our research, they’re going to have home prices rising if Trump wins.”
Editor's note: This article is part of a special Realtor.com series on the housing market and the swing states in the 2024 presidential election. For additional coverage in this series, click here .
Keith Griffith is a journalist at Realtor.com. He covers the housing market and real estate trends.
- Related Articles
Share this Article
Thank you for visiting nature.com. You are using a browser version with limited support for CSS. To obtain the best experience, we recommend you use a more up to date browser (or turn off compatibility mode in Internet Explorer). In the meantime, to ensure continued support, we are displaying the site without styles and JavaScript.
- View all journals
- My Account Login
- Explore content
- About the journal
- Publish with us
- Sign up for alerts
- Open access
- Published: 24 May 2024
A proteomics approach to isolating neuropilin-dependent α5 integrin trafficking pathways: neuropilin 1 and 2 co-traffic α5 integrin through endosomal p120RasGAP to promote polarised fibronectin fibrillogenesis in endothelial cells
- Christopher J. Benwell ORCID: orcid.org/0000-0003-0579-7920 1 , 2 ,
- Robert T. Johnson ORCID: orcid.org/0000-0003-3618-238X 1 , 2 nAff3 ,
- James A. G. E. Taylor 1 , 2 ,
- Jordi Lambert ORCID: orcid.org/0000-0003-4724-5200 1 , 2 nAff4 &
- Stephen D. Robinson ORCID: orcid.org/0000-0002-6606-7588 1 , 2
Communications Biology volume 7 , Article number: 629 ( 2024 ) Cite this article
318 Accesses
4 Altmetric
Metrics details
- Cell adhesion
- Cell migration
Integrin trafficking to and from membrane adhesions is a crucial mechanism that dictates many aspects of a cell’s behaviour, including motility, polarisation, and invasion. In endothelial cells (ECs), the intracellular traffic of α5 integrin is regulated by both neuropilin 1 (NRP1) and neuropilin 2 (NRP2), yet the redundancies in function between these co-receptors remain unclear. Moreover, the endocytic complexes that participate in NRP-directed traffic remain poorly annotated. Here we identify an important role for the GTPase-activating protein p120RasGAP in ECs, promoting the recycling of α5 integrin from early endosomes. Mechanistically, p120RasGAP enables transit of endocytosed α5 integrin-NRP1-NRP2 complexes to Rab11 + recycling endosomes, promoting cell polarisation and fibronectin (FN) fibrillogenesis. Silencing of both NRP receptors, or p120RasGAP, resulted in the accumulation of α5 integrin in early endosomes, a loss of α5 integrin from surface adhesions, and attenuated EC polarisation. Endothelial-specific deletion of both NRP1 and NRP2 in the postnatal retina recapitulated our in vitro findings, severely impairing FN fibrillogenesis and polarised sprouting. Our data assign an essential role for p120RasGAP during integrin traffic in ECs and support a hypothesis that NRP receptors co-traffic internalised cargoes. Importantly, we utilise comparative proteomics analyses to isolate a comprehensive map of NRP1-dependent and NRP2-dependent α5 integrin interactions in ECs.
Similar content being viewed by others
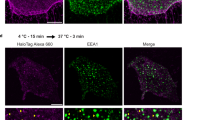
Neuropilin 1 and its inhibitory ligand mini-tryptophanyl-tRNA synthetase inversely regulate VE-cadherin turnover and vascular permeability
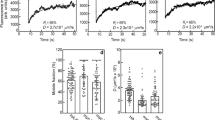
NRP1 interacts with endoglin and VEGFR2 to modulate VEGF signaling and endothelial cell sprouting
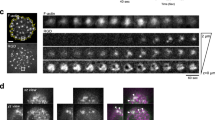
Podosome formation promotes plasma membrane invagination and integrin-β3 endocytosis on a viscous RGD-membrane
Introduction.
Dynamic adhesion to the extracellular matrix (ECM) is primarily mediated by integrin receptors. Blood vessel expansion during vascular development relies on the interaction between the ECM component fibronectin (FN) and its major integrin receptor α5β1 to establish, and subsequently balance the apico-basal polarity of migrating endothelial cells (ECs) 1 , 2 . During this process, the endocytosis and recycling of active α5β1 integrin receptors (those bound to an FN ligand), facilitates the turnover and replenishment of a newly synthesised matrix. Once the apico-basal axis has been defined, cycles of active α5β1 integrin traffic promote the assembly of a mechano-sensing fibrillar FN network, and therefore preserve a self-sustaining signalling cascade to maintain EC polarity 3 .
Depending on integrin heterodimer composition and ECM ligand attachment, integrin traffic involves the transit through specific RabGTPase + compartments. For example, whilst α5β1 integrin and αVβ3 integrin are endocytosed collectively via Rab5 or Rab21 GTPases, they are subsequently recycled via diverging Rab4-dependent (αVβ3 integrin) or Rab11-dependent (α5β1 integrin) routes 4 , 5 , 6 , 7 . The transmembrane glycoprotein neuropilin 1 (NRP1) was previously reported to selectively promote the endocytosis of FN-bound active α5β1 integrin from disassembling adhesions via its cytosolic interactor GAIP-interacting protein C terminus member 1 (Gipc1) and its associated motor protein myosin 6 in ECs. α5β1 integrin was subsequently shown to recycle in NRP1-associated complexes to stimulate FN fibrillogenesis 8 . We have since revealed that neuropilin 2 (NRP2), the ortholog of NRP1, also promotes Rab11-associated recycling of the α5 integrin subunit in ECs, and regulates the deposition of cell-secreted FN 9 , 10 . Since genetic ablation of either NRP1 or NRP2 has been shown to impair sprouting angiogenesis 9 , 11 , 12 , and a simultaneous co-deletion effectively inhibits pathological angiogenesis 13 , it has been proposed that a degree of synergy exists between the two receptors. It is unclear, however, which redundancies exist during NRP1-directed and NRP2-directed α5β1 integrin traffic, and which endocytic complexes participate.
p120RasGAP (alias: RASA1) is a ubiquitously expressed endosomal GTPase-activating protein (GAP) that contains canonical phospho-Tyrosine ( p Tyr)-interacting SH2 domains 14 , 15 . As an important negative regulator of the Ras signalling cascade, p120RasGAP global knockout (KO) mice exhibit major vascular defects that result in embryonic lethality 16 . Owing to its ability to interact with a wide array of SH2-binding proteins, p120RasGAP has, in recent years, also been shown to control the return of endocytosed integrin receptors back to the plasma membrane via interactions with the cytoplasmic tail of the integrin α-subunit. In MDA-MB-231 breast cancer cells, for example, p120RasGAP was demonstrated to competitively displace Rab21 from endocytosed β1 integrin in early endosomes, expediting its recycling via Rab11 + endosomes 17 . Indeed, integrin traffic is critically dependent upon the ability of Rab proteins to dynamically switch between GTP- and GDP-bound states: mutant GDP-locked or GTP-locked Rab21, Rab5, Rab4 and Rab11 all blocking intracellular transit 4 , 18 . Here, we show that p120RasGAP promotes ligand-bound α5 integrin subunit recycling via the Rab11 compartment in microvascular ECs. α5 integrin is first endocytosed to early endosomes in complex association with both NRP1 and NRP2 co-receptors, where p120RasGAP facilitates focal adhesion kinase (FAK) phosphorylation-dependent transit to Rab11 + recycling endosomes. siRNA silencing of both NRPs, p120RasGAP, Rab11 or inhibition of FAK phosphorylation results in the intracellular accumulation of α5 integrin. Utilising comparative proteomics analyses, we identify an upregulated escape mechanism to divert α5 integrin away from lysosomal degradation, preserving its total and surface-level expression. In addition, we isolate both NRP1-dependent and NRP2-dependent α5 integrin interactomes in ECs and where they intersect during intracellular traffic. Using inducible endothelial-specific knockout mouse models, we validate these findings by demonstrating a functional loss of FN in the postnatal retina upon co-deletion of both NRP1 and NRP2, which severely impairs polarised sprouting.
A proteome-scale map of the α5 integrin interactome in unstimulated microvascular ECs
Both NRP1 and NRP2 have been independently identified as key regulators of α5 integrin trafficking and fibronectin (FN) fibrillogenesis in ECs 8 , 9 , 10 . However, the redundancies that exist between NRP1- and NRP2-directed transport of α5 integrin, or indeed their ability to crosstalk during this process, remains unclear. Furthermore, the interacting complexes that participate in NRP-dependent α5 integrin traffic are poorly annotated.
To begin addressing this question, we employed label-free quantitative data-independent acquisition mass spectrometry ( LFQ -DIA-MS) to acquire an unbiased insight into the molecular binding partners of α5 integrin. To this aim, proteomic analysis of WT mouse-microvascular EC lysates immunoprecipitated with α5 integrin from a total of three independent experiments was performed, alongside Tat-Cre-deleted ECs lacking expression of α5 integrin (α5. EC KO ) to isolate non-specific partner binding. The Spectronaut label-free quantification (LFQ) algorithm was subsequently used for protein quantification with a p value cutoff of <0.01. A total of 3043 proteins were identified as putative interactors of α5 integrin, including fibronectin (Fn1), β1 integrin subunit (Itgb1), NRP1 (Nrp1) and NRP2 (Nrp2), indicating that our immunoprecipitation strategy had been successful (Fig. 1a–c ). Pathway enrichment analysis of these 3043 proteins revealed a high proportion to be involved in receptor endocytosis and trafficking, including 18 RabGTPase proteins, 18 myosin motor proteins, clathrin heavy chain-1 (Clt) (and its associated AP2 adaptor complex), caveolae-associated protein-1 (Cavn1), early endosome antigen-1 (EEA1) and Gipc1 (Fig. 1d and Suppl. Fig. 1A–D ).
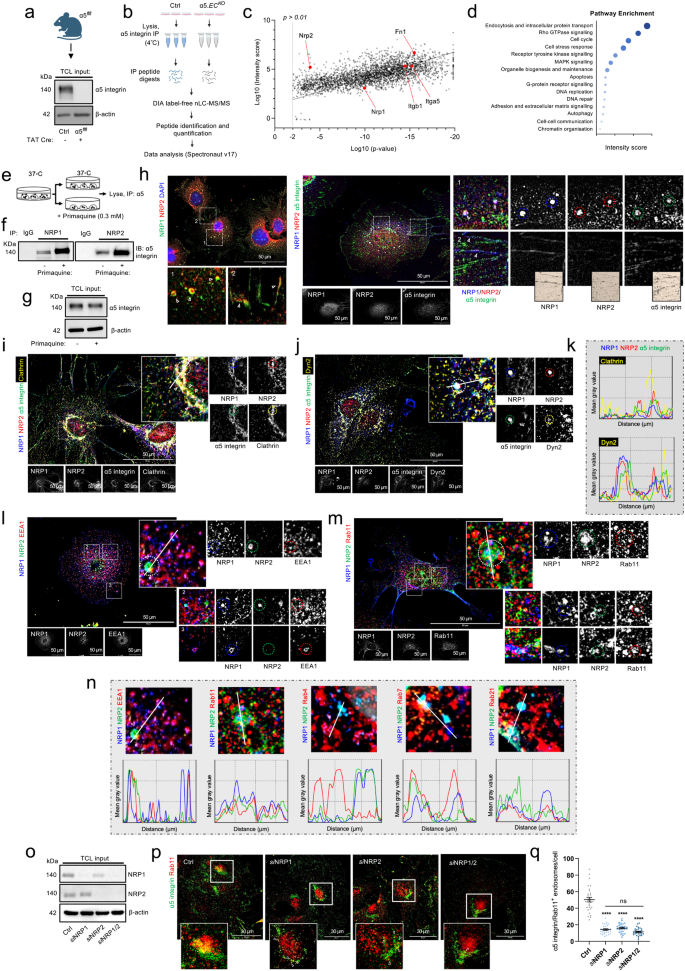
a Representative Western blot image showing confirmation of TAT-Cre-mediated deletion of α5 integrin from immortalised mouse-microvascular ECs isolated from α5 flfl mice. b Schematic representation of DIA nLC-MS/MS workflow from Ctrl and α5. EC KO EC lysates from three independent experiments. c Scatter plot showing the α5 integrin interactome in mouse-microvascular ECs ( p < 0.01 cutoff). Highlighted protein hits are shown in dark blue. d Pathway enrichment analysis showing the top 15 enriched pathways associated with the α5 integrin interactome. e Experim e ntal assay schematic: immunoprecipitation from EC lysates of NRP1 and NRP2 ± pre-treatment with primaquine (0.3 mM), followed by SDS-PAGE and Western blotting with anti-α5 integrin antibody. f Western blotting showing NRP interactions with α5 integrin ± pre-treatment with primaquine. g Total cell lysates showing α5 integrin expression in ECs ± pre-treatment with primaquine. h (Left panels): Representative confocal microscopy showing colocalisation between NRP1 and NRP2 within endosomal and adhesive structures. (Right panels): Representative confocal microscopy showing positive colocalisation between NRP1, NRP2 and α5 integrin . i – k Representative confocal microscopy showing positive colocalisation between NRP1, NRP2 α5 integrin and either clathrin heavy chain-1 ( i ) or dynam i n-2 ( j ). l , m Representative confocal microscopy showing positive colocalisation between NRP1, NRP2 and either EEA1 ( l ) or Rab11 ( m ). n Mean fluorescence intensity maps showing colocalisation between NRP1, NRP2 and EEA1, Rab11, Rab4, Rab7 or Rab21 respectively. o Total cell lysate input showing siRNA depletion of NRP1 and NRP2 by Western blotting. p Representative confocal microscopy images showing colocalisation between α5 integrin and Rab11 in Ctrl and siRNA-depleted ECs. q Quantification of α5 integrin + Rab11 endosomes/cell in Ctrl and siRNA-depleted ECs, ( n = 30 cells from three independent biological replicates), one-way ANOVA + post hoc multiple comparisons tests, **** p < 0.0001, ns non-significant. a , b , e created with BioRend e r.com.
Given the previously annotated roles of NRP1 and NRP2 during α5 integrin traffic 8 , 9 , 10 , we proceeded to examine their interaction more closely. To this end, we confirmed a robust biochemical interaction by co-immunoprecipitation and Western blotting. The stoichiometry of this interaction was also greatly increased following a 10-min pre-treatment with the recycling inhibitor primaquine (PMQ), which has been demonstrated to preserve endosomal-integrin contacts in the cell 8 , suggesting that NRP—α5 complexes exist more stably within endosomal punctae rather than at the plasma membrane (Fig. 1e–g ). Subsequent immunofluorescence confocal microscopy analysis to determine the subcellular localisation of both NRPs and α5 integrin revealed all three colocalise within endosomal structures, and albeit weakly, within proximity of fibrillar adhesions (Fig. 1h ), confirming our immunoprecipitation studies.
Next, we visualised the spatial segregation between endogenous NRP1 and NRP2 in endocytic compartments where α5 integrin has been reported to reside in, including EEA1 + /Rab21 + early endosomes 4 , 17 , Rab4 + /Rab11 + recycling endosomes 9 , 19 and Rab7 + late endosomes 19 . Importantly, confocal microscopy in fixed ECs revealed both NRPs to initially colocalise with α5 integrin in clathrin budding coats positive for both clathrin heavy chain-1 and dynamin-2 (Fig. 1i–k ). Furthermore, NRP1 and NRP2 were found to share a surprisingly high colocalisation fidelity with each other in EEA1 + , Rab21 + , Rab11 + and Rab7 + (but not in Rab4 + ) punctae (Fig. 1l–n ).
To corroborate our MS and confocal studies, we silenced expression of NRP1 and NRP2 individually ( si NRP1, si NRP2) or simultaneously ( si NRP1/2) by RNA interference (RNAi), transfecting mouse-microvascular ECs with either non-targeting (Ctrl) or NRP-specific siRNA oligonucleotides (Fig. 1o and Suppl. Fig. 1E ). After confirming the absence of any gene expression changes following siRNA targeting (Suppl. Fig. 1F ), we proceeded to examine α5 integrin colocalisation with endogenous Rab11. Depletion of either NRP1 or NRP2 significantly reduced the number of α5 integrin + Rab11 endosomes (Fig. 1p, q ), confirming previous studies, and indicating a reciprocal contribution in the Rab11 + long-loop recycling pathway.
Assembly of α5 integrin + adhesions and EDA-FN fibrillogenesis is impaired following NRP1/2 co-depletion in ECs
In ECs, both α5 and αV integrins are known to bind the extracellular matrix (ECM) ligand fibronectin (FN) 1 , 20 , 21 , 22 , 23 , 24 . To begin investigating the contributions of NRP1 and NRP2 during α5 integrin-dependent adhesion to FN, a process that relies on receptor trafficking 7 , we seeded Ctrl and siRNA-depleted ECs onto FN and assessed their ability to adhere over 3 h. siRNA-mediated depletion of either NRP1 or NRP2 significantly impaired adhesion to FN over this period as previously described 8 , 10 . Co-depleting both NRP1 and NRP2, however, was found to rescue EC adhesion at 90 min and significantly ameliorate EC adhesion at 180 min (Fig. 2a ). Confocal microscopy imaging to visualise endogenous α5 and αV integrin + adhesions, together with the focal adhesion (FA) adaptor protein paxillin in ECs fixed at 180 min ± VEGF stimulation subsequently revealed significant reductions in paxillin, α5 and αV integrin + adhesion area and number in si NRP1 and si NRP2 ECs, substantiating results by Ruhrberg et al., Alghamdi et al. and Benwell et al. and our adhesion studies 8 , 9 , 10 . In comparison, si NRP1/2 ECs assembled large punctate paxillin and αV integrin + adhesions, but very few α5 integrin + adhesions (Fig. 2b–d and Suppl. Fig. 2A ). High resolution XZ-ratio confocal imaging subsequently confirmed paxillin + adhesions to be largely absent of α5 integrin in ECs depleted for both NRP1 and NRP2 (Fig. 2e ). Photoactivation studies employing a tdTomato-expressing α5 integrin construct validated these findings (Fig. 2f ). Surprisingly, no gross changes in total endogenous expression of either integrin or paxillin between our siRNA-depleted groups were observed (Fig. 2g and Suppl. Fig. 2B ).
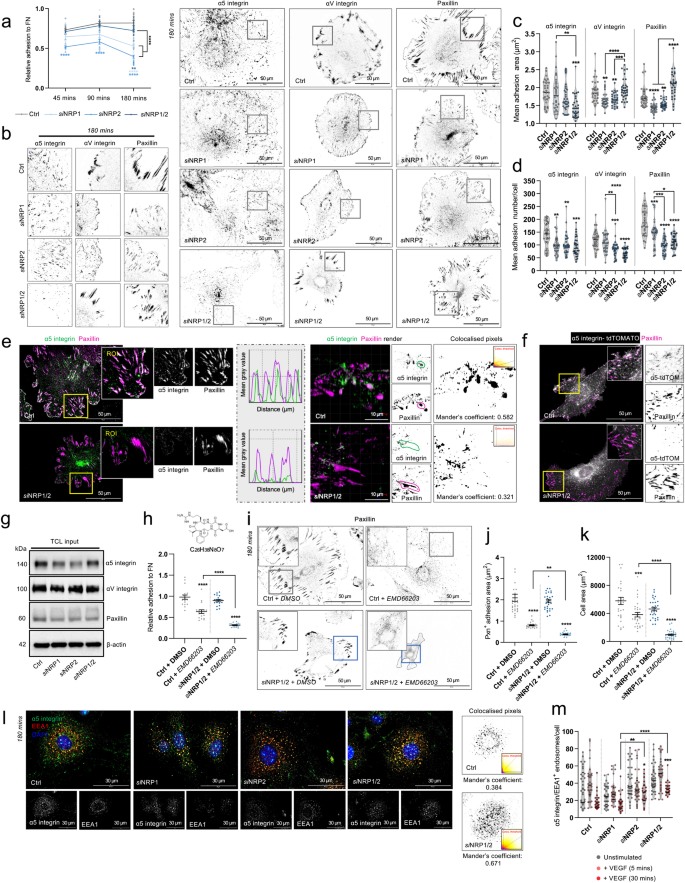
a Relative adhesion to FN by NRP1, NRP2 and NRP1/2 siRNA-treated ECs, ( N = 3 independent experiments), one-way ANOVA + post hoc multiple comparisons tests, ** p < 0.01, **** p < 0.0001. b Representative confocal microscopy images showing α5 integrin, αV integrin and paxillin + adhesions in Ctrl, si NRP1, si NRP2 and si NRP1/2 ECs following 180 mins adhesion to FN. c , d Analysis of α5 integrin, αV integrin and paxillin + adhesion size (µM 2 ) ( c ) and number ( d ) in unstimulated Ctrl, si NRP1, si NRP2 and si NRP1/2 ECs, ( n = 30 cells from three independent biological replicates), one-way ANOVA + post hoc multiple comparisons tests, * p < 0.05, ** p < 0.01, *** p < 0.001 and **** p < 0.0001. e Representative confocal microscopy images showing colocalisation between α5 integrin and paxillin in Ctrl and si NRP1/2 ECs in unstimulated conditions. Images are shown alongside mean fluorescence intensity maps. f Representative confocal microscopy images showing colocalisation between tdTOMATO expressing α5 integrin and paxillin in Ctrl and si NRP1/2 ECs. g Total cell lysate input showing expression levels of α5 integrin, αV integrin and paxillin by Western blotting. h Top panel: chemical structure of EMD66203 compound. Bottom panel: relative adhesion to FN by Ctrl and si NRP1/2 ECs ± 50 µM EMD66203, ( N = 2 independent experiments), one-way ANOVA + post hoc multiple comparisons tests, **** p < 0.0001. i Representative confocal microscopy images showing paxillin + adhesions presented by Ctrl and si NRP1/2 ECs ± 50 µM EMD66203 following 180 min adhesion to FN. J , k Quantification of paxillin + adhesion size (µM) ( j ) and cell area (µM 2 ) ( k ) of Ctrl and si NRP1/2 ECs ± 50 µ M EMD66203, ( n = 30 cells from three independent biological replicates), one-way ANOVA + post hoc multiple comparisons tests, ** p < 0.01, *** p < 0.001, **** p < 0.0001. l Representative confocal microscopy images showing α5 integrin + EEA1 early endosomes in Ctrl, si NRP1, si NRP2 and si NRP1/2 ECs following 180 min adhesion to FN. m Quantification of α5 integrin + EEA1 endosomes/cell ± 5 or 30 mins VEGF 165 stimulation, ( n = 30 cells from three independent biological replicates), one-way ANOVA + post hoc multiple comparisons tests, ** p < 0.01, *** p < 0.001, **** p < 0.0001.
Hypothesising that αV integrin likely compensates for the loss of α5 integrin from adhesions in si NRP1/2 ECs, as alluded to by Van der Flier et al. 23 , we next employed the αVβ3-specific Cilengitide mimetic EMD66203 25 to inhibit αVβ3-specific adhesion to FN. Ctrl siRNA ECs treated with EMD66203 exhibited partially reduced adhesion to FN at 180 min as expected and assembled smaller paxillin + adhesions. Simultaneous inhibition of αVβ3-specific adhesion via EMD662023, alongside co-depletion of NRP1 and NRP2, resulted in a near total loss of adhesion to FN; however, ECs exhibiting severe defects in cell spreading and adhesion assembly (Fig. 2h–k ). Taken together, we infer that additional loss of αVβ3-specific adhesion to FN sensitises ECs to the loss of α5 integrin-dependent adhesion exhibited following depletion of both NRP1 and NRP2.
Since si NRP1/2 ECs failed to assemble α5 integrin + adhesions, but displayed no reduced total expression, we proceeded to determine the subcellular localisation of α5 integrin following NRP depletion by immunofluorescence confocal microscopy. Compared to Ctrl ECs, where α5 integrin is distributed to both surface FAs and to perinuclear punctae, we observed α5 integrin to preferentially accumulate in EEA1 + early endosomes upon NRP co-depletion, particularly following VEGF stimulation (Fig. 2l–m ).
As stress-fibre-associated FAs become increasingly influenced by actomyosin tension, engaged α5 integrin is translocated centripetally with the actin-binding protein tensin-1 towards the cell body. As a consequence, following 24–48 h adhesion to FN, ECs exhibit a high density of hyperextended fibrillar adhesions enriched for both α5 integrin and tensin-1 26 , 27 , 28 . The actomyosin tension accrued by α5 integrin translocation into fibrillar structures is also fundamental for FN dimer unfolding and subsequent FN fibrillogenesis 3 , 29 . Since α5 integrin failed to translocate to adhesion punctae by 180 min, but instead preferentially distributed to intracellular sorting endosomes in si NRP1/2 ECs, we asked whether fibrillar adhesion assembly would also be affected. By 24 h, both si NRP1 and si NRP2 ECs displayed significantly smaller fibrillar adhesions than Ctrl ECs, whilst ECs co-depleted for both NRP1 and NRP2 failed to assemble α5 integrin + or tensin-1 + fibrillar adhesions entirely (Fig. 3a, b ). This was not found to result from changes in tensin-1 expression (Suppl. Fig. 3A, B ). Subsequent immuno-fluorescent visualisation of endogenous extra domain-A (EDA)- containing cellular FN (EDA-FN) (a spliced isoform of endothelial FN used to detect cell-secreted FN specifically 3 , 29 ) revealed a concomitant loss of fibrillar FN in si NRP1/2 ECs compared to Ctrl ECs or ECs individually depleted for either NRP1 or NRP2 as described previously 13 (Fig. 3c, d ). Furthermore, the compensatory actions of αVβ3 integrin observed at 180 minutes were insufficient to rescue cell adhesion (Fig. 3e ).
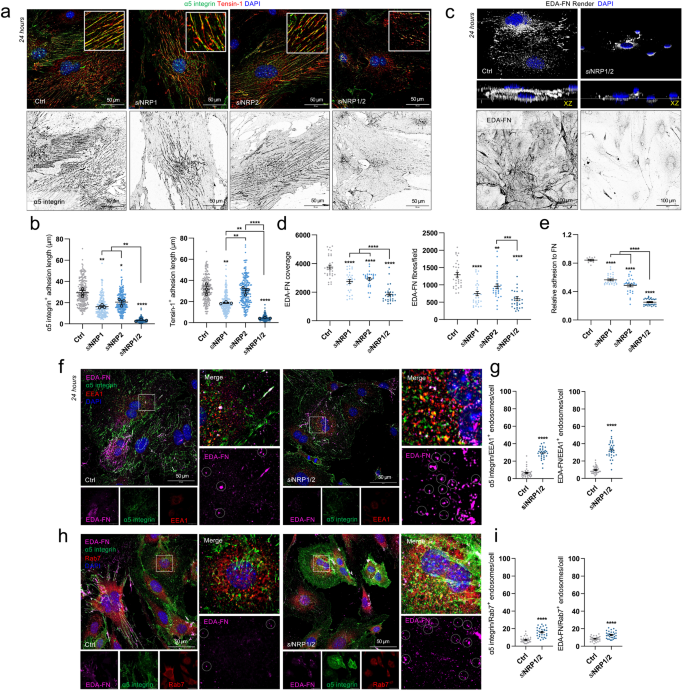
a Representative confocal microscopy images showing α5 integrin + and tensin-1 + fibrillar adhesions in Ctrl, si NRP1, si NRP2 and si NRP1/2 ECs following 24 h adhesion to FN. b Quantification of mean α5 integrin + and tensin-1 + adhesion length (µm), ( n = 30 cells from three independent biological replicates), one-way ANOVA + post hoc multiple comparisons tests, * p < 0.05, ** p < 0.01, **** p < 0.0001. c Representative confocal micros c opy images showing endogenous EDA-FN in Ctrl, si NRP1, si NRP2 and si NRP1/2 ECs following 24 h adhesion to FN. Middle panels show rendered EDA-FN in Ctrl and si NRP1/2 ECs with XZ-ratio. d Quantification of mean EDA-FN intensity and mean EDA-FN fibre density from confluent ECs, ( n = 30 ROIs from three independent biological replicates), one-way ANOVA + post hoc multiple comparisons tests, ** p < 0.01, **** p < 0.0001. e Relative adhesion to FN by NRP1, NRP2 and NRP1/2 siRNA-treated ECs at 24 h, ( N = 3 independent experiments), one-way ANOVA + post hoc multiple comparisons tests, **** p < 0.0001. f Representative confocal microscopy images showing colocalisation between EDA-FN, α5 integrin and EEA1 in Ctrl and si NRP1/2 ECs following 24 h adhesion to FN. g Quantification of α5 integrin + and EDA-FN + EEA1 early endosomes/cell, ( n = 30 cells from three independent biological replicates), Student’s t -test, **** p < 0.0001. h Representative confocal microscopy images showing colocalisation between EDA-FN, α5 integrin and Rab7 in Ctrl and si NRP1/2 ECs following 24 h adhesion to FN. i Quantification of α5 integrin + and EDA-FN + Rab7 late endosomes/cell, ( n = 30 cells from three independent biological replicates), Student’s t -test, **** p < 0.0001.
Rather than localise at or in close proximity to fibrillar structures, we observed both α5 integrin and EDA-FN to preferentially accumulate in EEA1 + (Fig. 3f, g ) and Rab7 + late endosomal punctae (Fig. 3h, i ). These data indicate that in the absence of NRP1 and NRP2, ligand-bound α5 integrin accumulates intracellularly after failing to recycle via Rab11 from early endosomes.
p120RasGAP regulates the plasticity of fibrillar adhesions and EDA-FN fibrillogenesis by mediating Rab11-dependent α5 integrin recycling in ECs
The ubiquitously expressed endosomal GTPase-activating protein p120RasGAP has previously been shown in breast cancer cells to control the return of endocytosed integrin to the plasma membrane. Following Rab21-mediated integrin endocytosis to the early endosome, p120RasGAP, via a direct mutually exclusive interaction with the cytoplasmic tail of the integrin α-subunit, competes for integrin binding and displaces Rab21. In the absence of p120RasGAP expression, β1 integrin was found to accumulate in EEA1 + endosomes, failing its exit to Rab11 + recycling endosomes 17 . It remains unclear however, whether p120RasGAP-dependent integrin recycling remains conserved in ECs.
Whilst our MS failed to detect p120RasGAP as directly associating with α5 integrin, a number of other GTPase interactors previously alluded to regulate endosomal transport were detected (RasGTPase-activating-like protein-1 (Iqgap1), RasGTPase-activating protein-binding protein-1 and -2 (G3bp1, G3bp2), Ras-interacting protein-1 (Rasip1), and RasGTPase-activating protein-like-2 (Rasal2) 30 , 31 , 32 , 33 , 34 . STRING analysis was subsequently employed to generate an interaction network of these proteins, which revealed all to have been previously reported as interactors of p120RasGAP (Suppl. Fig. 4A ). We postulate, therefore, that any interaction made with α5 integrin, as signposted by Mai et al. 17 exists weakly, and or very transiently via these adaptors.
To answer whether p120RasGAP promotes endosomal shuttling of α5 integrin in ECs, we first visualised the subcellular localisation of endogenous p120RasGAP in mouse-microvascular ECs by immunofluorescence confocal microscopy, and observed a strong colocalisation with α5 integrin, EEA1 + endosomes and Rab21 + endosomes (Fig. 4a, b ). Next, by means of surface biotinylation followed by biochemical immunoprecipitation (Suppl. Fig. 4B ), we assessed the impact of p120RasGAP siRNA-mediated depletion on total α5 integrin recycling. p120RasGAP silencing (Suppl. Fig. 4C ) was found to significantly impair the rate at which α5 integrin was recycled back to the plasma membrane compared to Ctrl ECs (Fig. 4c, d and Suppl. Fig. 4D ), concomitant with a significant reduction in the localisation of α5 integrin to Rab11 + punctae, recapitulating a co-depletion of NRP1 and NRP2 (Fig. 4e, f ).
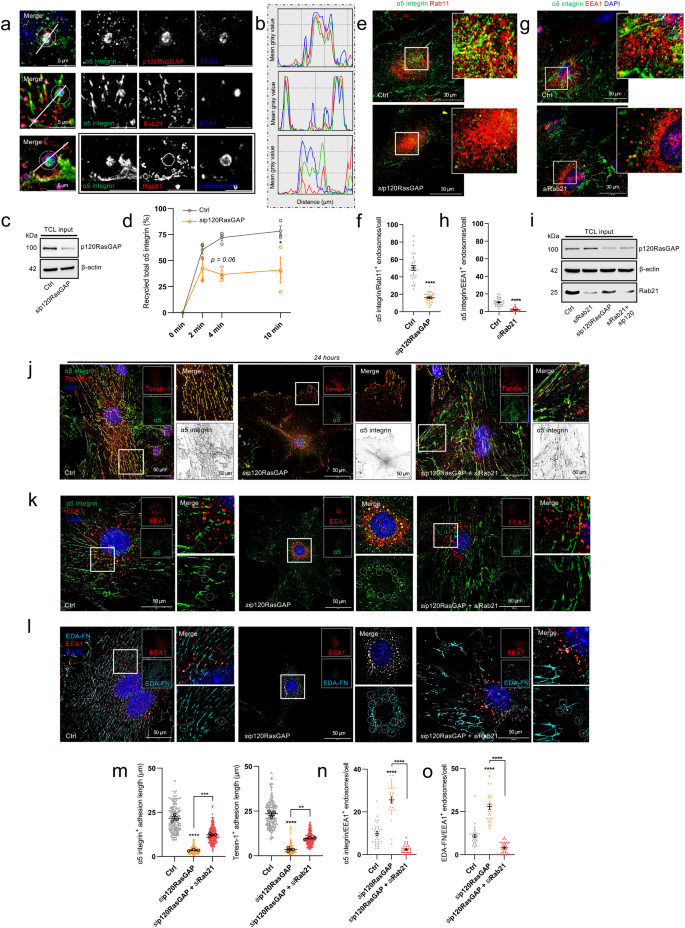
a Representative confocal microscopy images showing colocalisation between α5 integrin, p120RasGAP and EEA1 (top panels), α5 integrin, Rab21 and EEA1 (middle panels), and α5 integrin, Rab21 and p120RasGAP (bottom panels). b Mean fluorescence intensity maps showing respective colocalisation between α5 integrin, p120RasGAP, EEA1 and Rab21 as shown in ( a ). c Total cell lysates shown to confirm siRNA depletion of p120RasGAP. d Quantification of total α5 integrin recycling (%), ( N = 3 independent experiments), one-way ANOVA + post hoc multiple comparisons tests, * p < 0.05. e Representative confocal microscopy images showing colocalisation between α5 integrin and Rab11 in Ctrl and si p120RasGAP ECs. f Quantification of α5 integrin + Rab11 endosomes/cell in Ctrl and siRNA-depleted ECs, ( n = 30 cells from three independent biological replicates), Student’s t -test, **** p < 0.0001. g Representative confocal microscopy images showing colocalisation between α5 integrin and EEA1 in Ctrl and si Rab21 ECs. h Quantification of α5 integrin + EEA1 endosomes/cell in Ctrl and si Rab21 ECs, ( n = 30 cells from three independent biological replicates), Student’s t -test, **** p < 0.0001. i Total cell lysates confirming siRNA depletion of p120RasGAP and Rab21. j Representative confocal microscopy images showing α5 integrin and tensin-1 localisation to fibrillar adhesions at 24 h adhesion to FN in Ctrl, si p120RasGAP and si Rab21 ECs. k Representative confocal microscopy images showing α5 integrin colocalisation with EEA1 at 24 h adhesion to FN in Ctrl, si p120RasGAP and si Rab21 ECs. l Representative confocal microscopy images showing EDA-FN colocalisation with EEA1 at 24 h adhesion to FN in Ctrl, si p120RasGAP and si Rab21 ECs. m Quantification of α5 integrin and tensin-1 + adhesion length, ( n = 30 cells from three independent biological replicates), one-way ANOVA + post hoc multiple comparisons tests, ** p < 0.01, **** p < 0.0001. n Quantification of α5 integrin + EEA1 endosomes/cell, ( n = 30 cells from three independent biological replicates), one-way ANOVA + post hoc multiple comparisons tests, **** p < 0.0001. o Quantification of EDA-FN + EEA1 endosomes/cell, ( n = 30 cells from three independent biological replicates), one-way ANOVA + post hoc multiple comparisons tests, **** p < 0.0001.
To explore a functional association between Rab21 and p120RasGAP during α5 integrin trafficking, we then sought to impair the endocytosis of α5 integrin from fibrillar adhesions, alongside simultaneous loss of recycling to the membrane. First, we silenced Rab21 expression (to inhibit internalisation) (Suppl. Fig. 4E ) and confirmed the defective translocation of α5 integrin from fibrillar adhesions to EEA1 + early endosomes (Fig. 4g, h ). We subsequently co-silenced p120RasGAP (to inhibit recycling) and examined the localisation of α5 integrin and EDA-FN (Fig. 4i ). Compared to Ctrl ECs, where α5 integrin colocalised with tensin-1 + characteristic hyperextended structures, α5 integrin was lost completely from fibrillar adhesions in ECs depleted for p120RasGAP and accumulated with EDA-FN in EEA1 + early endosomes, recapitulating the loss of NRP1 and NRP2. Importantly, simultaneous depletion of both Rab21 and p120RasGAP preserved α5 integrin localisation to fibrillar adhesions and restored EDA-FN polymerisation into a fibrillar network (Fig. 4j–o ). Taken together, these data indicate that p120RasGAP promotes Rab11 + α5 integrin recycling from early endosomes, and that the plasticity of fibrillar adhesions and EDA-fibrillogenesis depends on a functional association between Rab21 and p120RasGAP to mediate α5 integrin endocytosis and recycling respectively.
FAK phosphorylation mediates p120RasGAP interactions with NRP - α5 integrin complexes
Concomitant to its interaction with integrin α-subunit tails, p120RasGAP has also been reported to interact with phosphorylated focal adhesion kinase ( p FAK), which is brought into close proximity following integrin complex endocytosis to the early endosome 35 .
With this in mind, we next determined whether NRP complexes interact with p120RasGAP in ECs during their transit to Rab11 + recycling endosomes, and whether this interaction is indeed dependent upon FAK activity. Lysates from ECs oligofected with either non-targeting Ctrl or NRP-specific siRNAs were immunoprecipitated with antibodies recognising either NRP1 or NRP2, before Western blotting for p120RasGAP. Both NRP1 and NRP2 co-immunoprecipitated with p120RasGAP, however the biochemical interaction between NRP2 and p120RasGAP was significantly increased following NRP1 silencing (Fig. 5a–d ), indicating a degree of compensation during complex assembly. To establish whether these interactions were mediated by FAK phosphorylation, we employed the well- characterised FAK-specific inhibitor PF562271, with which we initially confirmed a potent inhibition of FAK activation at both Tyr 397 and Tyr 407 phosphorylation sites at a concentration of >1 µM as previously described 36 , 37 , 38 (Fig. 5e ). PF562271 pre-treatment prior to lysis was found to ablate not only interactions with NRP1, but also α5 integrin and total FAK (Fig. 5f, g ), validating prior works describing a role for p FAK in mediating complex interactions with p120RasGAP 35 . Whilst NRP1–p120RasGAP complex association was found to be p FAK-dependent, PF562271 pre-treatment had no effect on the association between p120RasGAP and NRP2 however. Only when NRP1 expression was depleted, where previously we observed a significantly greater interaction, did their affinity become dependent upon FAK activity (Fig. 5h ). These data suggest that NRP1 and NRP2 competitively bind p120RasGAP during their transport of α5 integrin–FAK complexes, and that only in the absence or downregulated expression of NRP1 does NRP2 associate.
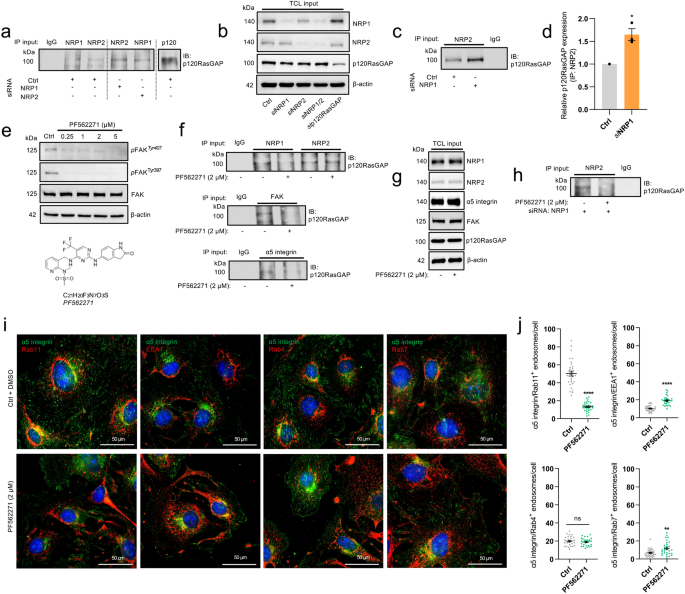
a Immunoprecipitation from Ctrl and siRNA-depleted EC lysates of NRP1, NRP2 and p120RasGAP followed by SDS-PAGE and Western blotting with anti-p120RasGAP antibody. b Total cell lysate input confirming siRNA depletion of NRP1, NRP2 and p120RasGAP by Western blotting. c Representative immunoprecipitation from Ctrl and si NRP1-depleted EC lysates of NRP2 followed by SDS-PAGE and Western blotting with anti-p120RasGAP antibody. d Quantification of relative p120RasGAP expression in Ctrl and si NRP1 lysates following immunoprecipitation of NRP2, ( N = 3 independent experiments) one-sample t -test, * p < 0.05. e Total c e ll lysate input confirming inhibition of FAK phosphorylation following pre-treatment with 2 µM PF562271. Below shows the chemical structure of PF562271 compound. f Immunoprecipitation of NRP1 and NRP2 from EC lysates followed by SDS-PAGE and Western blotting with anti-p120RasGAP antibody showing loss of association following pre-treatment with 2 µM PF562271. g Total cell lysate input showing NRP1, NRP2, α5 integrin, FAK and p120RasGAP expression by Western blotting following pre-treatment with 2 µM PF562271. h Immunoprecipitation of NRP2 from Ctrl and si NRP1 EC lysates followed by SDS-PAGE and Western blotting with anti-p120RasGAP antibody showing loss of association following pre-treatment with 2 µM PF562271. i Representative confocal microscopy images showing α5 integrin colocalisation with Rab11, EEA1, Rab4 or Rab7 in Ctrl ECs ± pre-treatment with PF562271. j Quantification of α5 integrin + Rab11, EEA1, Rab4 or Rab7 endosomes/cell in Ctrl and siRNA-depleted ECs, ±pre-treatment with PF562271 ( n = 30 cells from three independent biological replicates), Student’s t -test, **** p < 0.0001.
To corroborate our findings, we proceeded to examine the effect of PF562271 pre-treatment on α5 integrin subcellular localisation in Ctrl ECs. PF562271-elicited inhibition of FAK phosphorylation recapitulated the loss of α5 integrin colocalisation with Rab11 + endosomes and the concomitant accumulation in EEA1 + early endosomes observed following NRP co-depletion or silencing of p120RasGAP. Furthermore, whilst PF562271 incubation had no effect on α5 integrin - Rab4 colocalisation, significantly more α5 integrin was observed to reside in Rab7 + compartment punctae, suggesting that following α5 integrin accumulation in early endosomes, it is diverted for lysosomal processing (Fig. 5i, j ).
Retrograde transport via the Dynein–Dynactin complex saves α5 integrin from lysosomal degradation
Despite α5 integrin accumulating in EEA1 + early endosomes and Rab7 + late endosomes, both surface and total α5 integrin expression were observed to remain intact in si NRP1/2 and si p120RasGAP ECs (Fig. 6a–c ). This led us to consider whether, in the absence of recycling via the Rab11 compartment, α5 integrin utilises an alternative trafficking route to avoid lysosomal degradation. In an attempt to elucidate the novel α5 integrin interactors that participate in non-canonical trafficking pathways, we performed comparative proteomic analysis between our α5 integrin interactome dataset acquired from Ctrl EC lysates, and lysates collected from si NRP1, si NRP2, si NRP1/2 and s ip120RasGAP ECs from a total of three independent experiments in the same manner as described in Fig. 1a–c (Fig. 6d ).
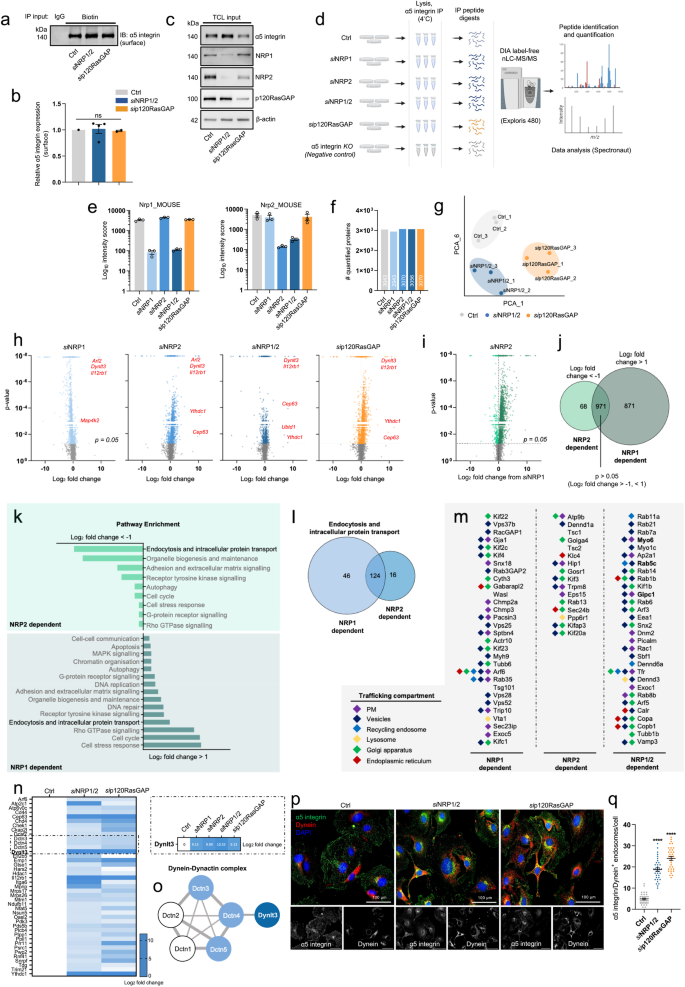
a Representative Western blotting showing surface α5 integrin expression from Ctrl, si NRP1/2 and si p120RasGAP lysates. b Densitometric quantification of surface α5 integrin expression, ( N ≥ 2 independent experiments), one-way ANOVA + post hoc multiple comparisons tests, ns non-significant. c Total cell lysate input showing NRP1, NRP2, α5 integrin and p120RasGAP expression by Western blotting. d Schematic representation of DIA nLC-MS/MS workflow from Ctrl and siRNA-depleted lysates. e Confirmation of NRP1/NRP2 depletion from MS output. f Total numbers of quantified proteins identified in Ctrl, si NRP1, si NRP2, si NRP1/2 and si p120RasGAP samples. g PCA plot showing two component separation between Ctrl, si NRP1/2 and si p120RasGAP samples. h Volcano plots showing the Log 2 fold-change ratios between si NRP1, si NRP2, si NRP1/2, si p120RasGAP samples and the Ctrl sample. Non-significant ( p < 0.05) interactions are coloured grey. Protein interactors with the highest positive fold-change are labelled. i Volcano plot showing the Log 2 fold-change ratio between siNRP2 and siNRP1 samples. Non-significant ( p < 0.05) fold-change differences are coloured grey. j Venn diagram associated with ( i ) showing the total number of protein interactors with a log 2 fold-change <−1 (NRP 2 -dependent interactions), the total number of protein interactors with a log 2 fold-change >1 (NRP1-dependent interactions), and the total number of protein interactions with a non-significant ( p < 0.05) fold-change difference. k Pathway enrichment analysis showing the top 15 enriched pathways associated with NRP1 and NRP2-specific interactions. l Venn diagram associated with ( i ) showing the number of trafficking-associated protein i nteractors (NRP2 dependent: 16, NRP1 dependent: 46, common: 124. m The top 30 protein interactors for each group are shown alongside their location. n Heatmap associated with ( h ) showing common interactors between siNRP1/2 and sip120RasGAP samples with a significant, positive log 2 fold-change (>1) away from the Ctrl sample. Highlighted box shows the comparison in log 2 fold-change between all siRNA-depleted groups. o STRING interaction network showing associations between Dynlt3 and Dctn1-5. p Representative confocal microscopy images showing α5 integrin colocalisation with dynein in Ctrl, si NRP1/2 and si p120RasGAP ECs. q Quantification of α5 integrin/dynein + endosomes/cell, ( n = 30 cells from three independent biological replicates), Student’s t -test, **** p < 0.0001. d Created with BioRender.com.
First, we established that the interaction between α5 integrin and both NRPs had been reduced to negligible levels, reaffirming the efficiency of our siRNA depletions (Fig. 6e ). Next, despite no gross changes in the number of quantified proteins (Fig. 6f ), we observed a clear segregation by principal component analysis (PCA) between our Ctrl datasets and those acquired from NRP1/NRP2 or p120RasGAP depleted lysates, indicating clear alterations to α5 integrin’s interactome (Fig. 6g ). These changes were subsequently visualised in each of our siRNA-depleted groups with volcano plots, identifying protein candidates with the greatest fold-change differences compared to our Ctrl dataset (with a p value cutoff >0.05) (Fig. 6h ).
Prior to evaluating any upregulated trafficking pathways unique to ECs depleted for both NRPs or p120RasGAP, we noted striking differences in the fold-change profiles acquired from our si NRP1 and si NRP2 EC datasets. In an attempt to isolate NRP1 and NRP2-dependent α5 integrin binding partners, we proceeded to visualise the protein fold-change ratios between si NRP2 and si NRP1 groups (Fig. 6i ). Of these α5 integrin interacting proteins, 68 were associated with α5 integrin at a log 2 fold-change <−1 (which we defined as NRP2-dependent interactions), 871 associated at a log 2 fold-change >1 (defined NRP1-dependent interactions), and 971 associated at an insignificant log 2 fold-change ( p > 0.05) (shared interactions) (Fig. 6j ). Comparative pathway enrichment analysis to evaluate these NRP-specific interactions revealed both to regulate unique intracellular trafficking pathways utilised by α5 integrin in ECs (46 NRP1-dependent, 16 NRP2-dependent and 122 shared interactions) (Fig. 6k–m ). These data support a paradigm whereby NRP1 and NRP2’s ability to co-traffic α5 integrin in ECs is context-dependent. Indeed, our findings suggest that both NRP1 and NRP2 independently regulate α5 integrin’s association with multiple trafficking proteins, in addition to mediating other interactions cooperatively. For example, and corroborating the work presented in this study, Rab11 and Rab21 were both detected as common denominators to both NRP1 and NRP2, alongside α5 integrin’s interactions with Myosin 6, Rab5 and Gipc1 as previously reported 8 (Fig. 6m ).
To elucidate non-canonical trafficking pathways upregulated in si NRP1/2 and si p120RasGAP ECs, we returned, however to our Ctrl comparisons shown in Fig. 6h . Of the 3056 proteins enriched from our si NRP1/2 sample, only 50 were identified as interacting with α5 integrin at a positive log 2 fold-change >1 compared to our Ctrl group, of which 41 shared a similar positive log 2 fold-change profile with our si p120RasGAP group. Of these 41 putative interacting proteins, four exhibited a positive log 2 fold-change >8 (fold-change >3): Centrosomal protein-63 (Cep63) 39 , Dynein light chain-3 (Dynlt3) 40 , Interleukin-12 receptor β1 (Il12rβ1) 41 and YTH N6-methyladenosine RNA binding protein C1 (Ythdc1) 42 . Upon inspection of our si NRP1 and si NRP2 datasets, Cep63, Dynlt3 and Il12rβ1 were all found to interact with α5 integrin at a similarly high magnitude, indicating their reciprocal contribution in the absence of the NRPs or p120RasGAP (Fig. 6n ).
Dynlt3 is known to homodimerize to assemble the light chain component of the cytoplasmic dynein motor protein complex, which with its cofactors Dynactin 1–5, promotes retrograde transport from Rab7a + late endosomes along the microtubule cytoskeleton to the trans-Golgi network (TGN) 43 , 44 , 45 . This non-canonical trafficking route has been elucidated to function as a defence mechanism against undesirable protein degradation, diverting integral receptors away from the lysosome and redirecting them back to the plasma membrane 46 , 47 . We hypothesise, therefore, that following the loss of Rab11-dependent recycling, elicited by the depletion of NRP1 and NRP2, or p120RasGAP, α5 integrin’s surface (and total) expression is maintained by retrograde transport via the TGN and the dynein–dynactin complex. In support of this hypothesis, α5 integrin’s interaction with Dynactin 3, 4 and 5 (Dctn3-5) was significantly elevated in si NRP1/2 and si p120RasGAP ECs compared to the Ctrl sample (Fig. 6o ). We subsequently validated our proteomics by immunofluorescence imaging, demonstrating that α5 integrin accumulated in dynein + punctae following co-depletion of NRP1 and NRP2, or by silencing p120RasGAP expression (Fig. 6p, q ). In sum, proteomics analyses has revealed that α5 integrin requires NRP1 and NRP2 to direct polarised transport through its canonical recycling pathway, and in their absence, ECs adopt a rescue mechanism to divert α5 integrin away from lysosomal degradation. In addition, we have identified a plethora of previously unknown, NRP-specific α5 integrin interactions. These putative associations encompass not only endosomal trafficking, as discussed here, but also cell cycle regulation, organelle biogenesis, cell-stress responses, and a range of canonical signalling pathways.
Endothelial NRPs and p120RasGAP promote cell polarity during directional cell migration
The SH2 domain-dependent p FAK association with p120RasGAP has been shown to promote cell polarisation in mouse embryonic fibroblasts, where silencing of p120RasGAP or inhibition of FAK phosphorylation results in disruptions to Golgi body orientation and subsequent impaired directional migration 14 , 35 . Long-loop recycling of α5 integrin from fibrillar adhesions and the accompanying exocytosis of fibrillar EDA-FN by Rab11 recycling endosomes has also been demonstrated to establish the apico-basal polarity axis in ECs, which is essential for vascular morphogenesis in vivo 3 .
We have demonstrated previously that NRP2 silencing in ECs moderately impairs the response of FAK phosphorylation to VEGF-stimulation 9 . We, therefore, hypothesised that attenuated FAK phosphorylation in ECs depleted for either NRP or p120RasGAP would also impair cell polarisation. To test this, we first assessed FAK phosphorylation at Tyr 397 or Tyr 407 residues, both reported to regulate EC adhesion and migration 9 , 48 , under basal conditions or following VEGF stimulation. When normalised against total FAK expression, silencing of either NRP1, NRP2, or p120RasGAP in confluent ECs stimulated with VEGF for 5 minutes significantly reduced FAK phosphorylation at Tyr 397 , and induced a robust reduction in phosphorylation at Tyr 407 (Fig. 7a, b and Suppl. Fig. 5A, B ). Expression of both p FAK Tyr397 and Tyr 407 under basal conditions was also found to be significantly attenuated following co-depletion of both NRP1 and NRP2 (Fig. 7c ). To corroborate our biochemical studies, we visualised endogenous p FAK Tyr407+ by immunofluorescence microscopy in ECs fixed 8 h-post initiation of a scratch-wound to assess their ability to assemble polarised adhesions (Fig. 7d ). Whilst Ctrl ECs assembled a higher proportion of leading edge p FAK Tyr407+ adhesions vs at their trailing edge, indicative of correctly establishing a polarity axis towards the direction of migration, ECs depleted for both NRP1 and NRP2, or p120RasGAP were observed to assemble equal numbers of both leading and trailing edge p FAK Tyr407+ adhesions (Fig. 7e, f ), suggesting an impaired ability to polarise.
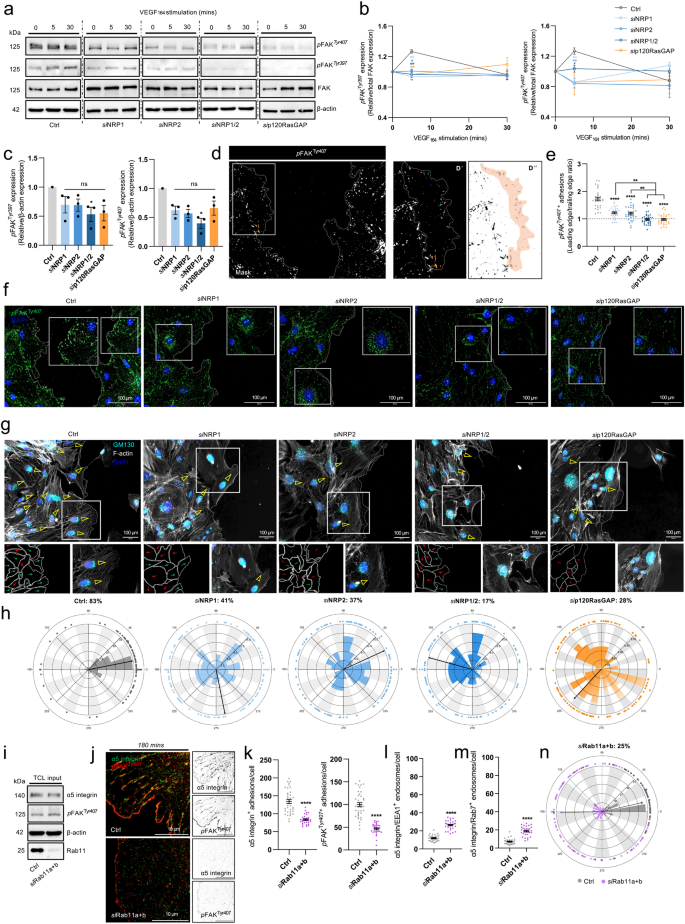
a Representative Western blotting of Ctrl and siRNA-depleted EC lysates ± 5 or 30 mins VEGF 165 stimulation, showing expression changes in total FAK, p FAK Tyr397 and p FAK Tyr407 . b Supporting densitometric timecourse analysis of p FAK Tyr397 and p FAK Tyr407 expression shown in ( a ), relative to total FAK expression, ( N ≥ 3 independent experiments), one-way ANOVA + post hoc multiple comparisons tests, * p < 0.05, ** p < 0.01. c Densitometric analysis of unstimulated p FAK Tyr397 and p FAK Tyr407 expression shown in ( a ), relative to β-actin loading control, ( N = 3 independent experiments), one-sample t -test, * p < 0.05. d Immunolabelling of endogenous p FAK Tyr407+ leading vs trailing edge focal adhesions the scratch-wound edge. D’ and D” panels show the process of skeletonising focal adhesions prior to analysis. e Ratio quantification of p FAK Tyr407+ leading vs trailing edge adhesions in Ctrl and siRNA-depleted ECs at the scratch-wound edge, ( n = 30). f Representative confocal microscopy images quantified in ( e ). g Representative confocal microscopy images showing GM130 + Golgi body positioning and F-actin protrusions in Ctrl and siRNA-depleted ECs at the scratch-wound edge fixed 8-h post scratch initiation. h Polar plots showing Golgi body orientation in Ctrl and siRNA-depleted ECs, ( n = 100). i Total cell lysate input confirm i ng siRNA depletion of Rab11 by Western blotting. j Representative confocal microscopy images showing α5 integrin colocalisation with p FAK Tyr407 in Ctrl and si Rab11a + b ECs following 180 min adhesion to FN. k Quantification of α5 integrin + and p FAK Tyr407+ adhesions/cell in Ctrl and si Rab11a + b ECs, ( n = 30 cells from three independent biological replicates), Student’s t -test, **** p < 0.0001. l Quantification of α5 integrin + EEA1 endosomes/cell, ( n = 30 cells from three independent biological replicates), Student’s t -test, **** p < 0.0001. m Quantification of α5 integrin + Rab7 endosomes/cell, ( n = 30 cells from three independent biological replicates), Student’s t -test, **** p < 0.0001. n Polar plot showing Golgi body orientation in Ctrl and si Rab11 ECs, ( n = 100).
To directly quantify the effect of NRP or p120RasGAP silencing on cell polarisation, we subsequently performed scratch-wound assays in confluent Ctrl and siRNA-depleted ECs before analysing Golgi body orientation. Compared to Ctrl ECs, where ~80% of Golgi bodies were orientated towards the direction of the scratch site, silencing of either NRP1 or NRP2 reduced correct Golgi polarisation by approximately half. This was further reduced to ~20 and ~30% following co-depletion of NRP1 and NRP2 or p120RasGAP, respectively. Alongside an impaired ability to polarise correctly, siRNA depletion also reduced the number of F-actin + lamellipodial protrusions directed into the avascular space (Fig. 7g–h ). Taken together, we postulate that NRP1 and NRP2 direct α5 integrin long-loop recycling via p120RasGAP in a p FAK-dependent manner to promote polarised EC migration over FN matrices.
Finally, to validate this hypothesis, we assessed the impact of Rab11 silencing on the assembly of α5 integrin and p FAK Tyr407+ adhesions in ECs. After confirming effective co-depletion of both Rab11a and Rab11b isoforms by Western blotting (Fig. 7i and Suppl. Fig. 5C ), we visualised both endogenous α5 integrin and p FAK Tyr407 in Ctrl and si Rab11 ECs fixed at 180 minutes adhesion to FN by immunofluorescence confocal microscopy. Rab11 depletion was found to significantly reduce the number of both α5 integrin and p FAK Tyr407+ adhesions (Fig. 7j, k ) and recapitulated the accumulation of α5 integrin in EEA1 + early endosomes and Rab7 + late endosomes (Fig. 7l, m and Suppl. Fig. 5D, E ) as observed in si NRP1/2 ECs, si p120RasGAP ECs, and ECs pre-treated with PF562271. Finally, si Rab11 ECs displayed significantly reduced Golgi body polarisation (Fig. 7n and Suppl. Fig. 5F ), further substantiating the plethora of studies describing the role of Rab11 in promoting cell polarisation via polarised transport of endocytosed cargoes 3 , 49 , 50 , 51 .
Endothelial NRPs are essential for polarised sprouting angiogenesis and FN fibrillogenesis
Polarised FN secretion and directional EC migration are required for establishing a functional vascular network 3 . To determine the role of NRP1 and NRP2 during FN fibrillogenesis in vivo, we utilised NRP1 flfl .Pdgfb-iCreER T2 (NRP1 flfl .EC KO ), NRP2 flfl .Pdgfb-iCreER T2 (NRP2 flfl .EC KO ), and NRP1 flfl NRP2 flfl .Pdgfb-iCreER T2 (NRP1 flfl NRP2 flfl .EC KO ) animals, generated as previously described 13 , and compared the effects of an acute endothelial-specific deletion of NRP1, NRP2, or NRP1 and NRP2 respectively during sprouting angiogenesis of the retina at postnatal day 6 (P6). Following P2 - P5 tamoxifen administrations (Fig. 8a ), we first confirmed successful depletion of both NRP1 and NRP2 expression from the superficial vascular plexus (Fig. 8b ). Loss of NRP1 expression was found to result in major sprouting defects, and a significant reduction in vascular outgrowth from the optic nerve head, as formerly reported 11 . Likewise, though less severe, deletion of endothelial NRP2 resulted in a mild reduction in vascular extension, and significant impediments to sprouting (as previously described) 9 . Unsurprisingly, endothelial knockout of both NRP1 and NRP2 elicited the most deleterious effects on vascular outgrowth, NRP1 flfl NRP2 flfl .EC KO animals exhibiting severe defects in vascular extension, branching, and total EC density via ERG1/2 staining, in addition to tip cell and filopodia number (Fig. 8c–g and Suppl. Fig. 6A, B ). A significant increase in vessel regression assessed by means of enumerating the frequency of collagen IV + empty sleeves was also observed (Suppl. Fig. 6C, D ). These results largely phenocopy those reported by Chen et al., when describing the impairments to retinal angiogenesis following endothelial knockout of p120RasGAP 16 . Despite severe defects to filopodia number in our NRP flfl .EC KO mice, no impairments to filopodial length or tortuosity were observed when compared against respective littermate controls (Suppl. Fig. 6E ).
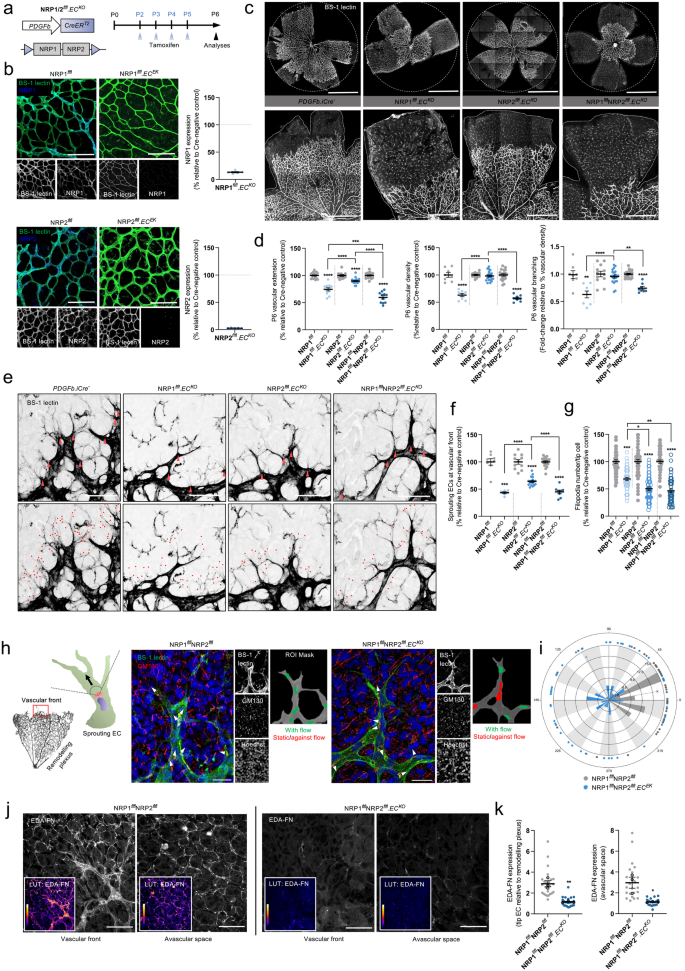
a Inducible, endothelial-specific deletion of NRP1, NRP2 or both NRP1 and NRP2 was achieved by crossing NRP flfl mice with Pdgfb-iCreER T2 mice. Activation of Pdgfb-iCreER T2 was achieved by subcutaneous injection of tamoxifen on P2 and P3, followed by intraperitoneal (IP) injections on P4 and P5. Retinas were then harvested at P6. b Tamoxifen-induced deletion of NRP1 and NRP2 was confirmed by immuno-colocalisation with BS-1 lectin. c Representative confocal microscopy images showing BS-1 lectin-labelled retinal vasculature of Pdgfb-iCreER T2 -negative, NRP1 flfl .EC KO , NRP2 flfl .EC KO and NRP1 flfl NRP2 flfl .EC KO animals. d Quantification of retinal vascular extension, vascular density and vascular branching (relative to vascular density), shown as relative percentages of respective littermate control animals, ( N ≥ 3 independent experiments ( n ≥ 6 retinas)), Student’s t -tests/one-way ANOVA + post hoc multiple comparisons tests, ** p < 0.01, *** p < 0.001, **** p < 0.0001. e Representative confocal microscopy images showing BS-1 lectin-labelled tip cells (red arrows) and filopodia (red dots) in Pdgfb-iCreER T2 -negative, NRP1 flfl .EC KO , NRP2 flfl .EC KO and NRP1 flfl NRP2 flfl .EC KO animals. f , g Quantification of the number of sprouting ECs, ( N ≥ 3 independent experiments ( n ≥ 6 retinas)), Student’s t -tests/one-way ANOVA + post hoc multiple comparisons tests, *** p < 0.001, **** p < 0.0001 ( f ), and filopodia number/ tip EC ( g ), ( n ≥ 50 ECs from five retinas), Student’s t -tests/one-way ANOVA + post hoc multiple comparisons tests, * p < 0.05, ** p < 0.01, *** p < 0.001, **** p < 0.0001, shown as relative percentages of respective littermate control animals. h (Left panel schematic): representation of a sprouting EC showing the correct orientation of the Golgi body (red) in relation to the nucleus (blue) and the direction of migration (arrow). (Right panels): Representative confocal microscopy images of the retinal vascular front immunolabelled with BS-1 lectin, GM130 and Hoechst in Pdgfb-iCreER T2 negative and NRP1 flfl NRP2 flfl .EC KO animals. White arrows indicate the direction of Golgi body orientation. i Polar plot showing Golgi body orientation in Pdgfb-iCreER T2 negative and NRP1 flfl NRP2 flfl .EC KO an i mals ( n = 50 ECs). j Representative confocal microscopy images showing endogenous EDA-FN at the vascular front and in the avascular space of Pdgfb-iCreER T2 negative and NRP1 flfl NRP2 flfl .EC KO animals. k (Left): Quantification of EDA-FN expression in sprouting tip cells relative to the remodelling plexus, ( n ≥ 24 sprouting tip cells from three retinas), (Right): Quantification of avascular EDA-FN expression directly ahead of the leading edge, ( n ≥ 24 ROIs from three retinas), Student’s t -tests, * p < 0.05, ** p < 0.01. h Created with BioRender.com.
Given our earlier findings, we proceeded to consider whether the combined loss of both NRP1 and NRP2 would also result in defects to tip cell polarisation during sprouting angiogenesis. To this end, we analysed front-rear polarity by GM130 labelling and immunofluorescence confocal microscopy in sprouting ECs. Compared to littermate control mice, whose sprouting ECs correctly oriented their Golgi bodies to align with the direction of flow, NRP1 flfl NRP2 flfl .EC KO animals exhibited a ~60% impaired front-rear Golgi body polarisation (Fig. 8h, i and Suppl. Fig. 6F ), validating our in vitro studies.
FN is highly expressed during the development of the retinal vascular plexi and provides an essential scaffold to facilitate polarised migration of sprouting ECs towards the retinal periphery 21 . In addition to being assembled ahead of the expanding vascular plexus by retinal astrocytes, EC-derived FN has more recently been shown to be expressed surrounding the vasculature itself 52 . To determine whether endothelial knockout of both NRP1 and NRP2 would impair EC-derived FN fibrillogenesis in vivo, we visualised the expression of EDA-FN in the superficial vascular plexus of P6 mice by immunofluorescence confocal microscopy. In control littermate animals, EDA-FN expression was highest at the vascular front, enriched at the apical ends of sprouting ECs and in the avascular space immediately ahead of the growing vascular bed. Retinal EDA-FN expression was found to be significantly reduced in NRP1 flfl NRP2 flfl .EC KO mice, however (Fig. 8j, k ), an impediment that we postulate would directly impair the ability of new sprouts to form stable cell-matrix interactions and subsequently polarise to promote directional EC migration (Fig. 9 ).
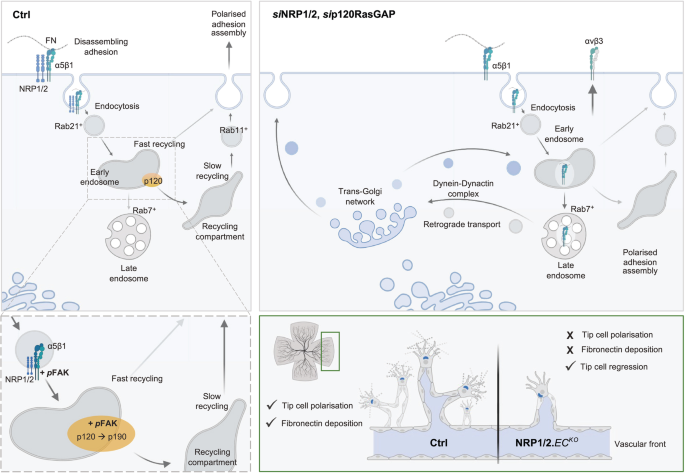
Internalised NRP-α5 integrin complexes undergo Rab11 + long-loop recycling via p120RasGAP, promoting polarised nascent adhesion assembly and directional migration in endothelial cells. In the absence of NRP1 and NRP2, α5 integrin arrests in early endosomes and Rab7 + late endosomes, before being recycled via retrograde transport and the dynein–dynactin complex. In vivo, endothelial deletion of NRP1 and NRP2 severely impairs polarised sprouting and fibronectin deposition. Created with BioRender.com.
In the present study, we show that α5 integrin is endocytosed as a complex with NRP1 and NRP2, before transiting to Rab11 + recycling endosomes via the GTPase-activating protein p120RasGAP. As a consequence of NRP or p120RasGAP silencing, α5 integrin is lost from surface focal and fibrillar adhesions, but rather accumulates with its FN ligand in early and late endosomes. Mechanistically, we show both NRPs biochemically interact with endosomal p120RasGAP, facilitating the displacement of Rab21 to promote traffic to Rab11 + recycling endosomes and subsequent cell polarisation. Comparative proteomics analysis subsequently identified a candidate escape pathway utilised by si NRP1/2 and si p120RasGAP ECs to divert α5 integrin away from lysosomal degradation. Importantly, our proteomics workflow enabled us to segregate a comprehensive set of NRP1-dependent and NRP2-dependent α5 integrin interactions, which we invite readers to explore as a resource for further insight.
Previous work has indeed annotated a role for both NRP1 and NRP2 in promoting α5 integrin traffic in ECs 8 , 9 , 10 , yet investigations have continued to pursue their roles in isolation rather than to consider their collective function. We observe their robust colocalisation in endocytic punctae at all stages of intracellular traffic, including clathrin-coated assemblies, early endosomes, Rab11 recycling endosomes and Rab7 late endosomes. Indeed, Rab21, EEA1, Rab11 and Rab7 were all identified as common to both NRP1 and NRP2-dependent interactors by our MS analysis. By extension, it is also possible that both NRP receptors are present during the turnover lifecycle of multiple other cell surface complexes, not only α5 integrin. For example, NRP1 has been shown to promote recycling of VEGFR-2 through Rab11 + vesicles, and colocalise with VEGFR-2 in Rab7 + vesicles 53 , 54 . Given NRP co-silencing impaired α5 integrin distribution to the Rab11 compartment to a greater extent than silencing either NRP receptor individually, we postulate that both share a mutual contribution in the ‘long-loop’ recycling pathway. For example, only in ECs depleted for both NRP1 and NRP2 did α5 integrin fail to assemble at adhesion sites entirely, and instead accumulate in early and late endosomes.
In addition to reaffirming their shared control of the Rab11 recycling pathway, our MS studies also provided great insight into the NRP’s diverging influence on non-canonical α5 integrin traffic in ECs. For example, α5 integrin’s interactions with 6 kinesin-family proteins (Kif22, Kif2c, Kif4, Kif23, Kifc1 and Kif2a) 55 , 56 , 57 , 58 , 59 , Sorting nexin 18 (Snx18) 60 , and Rab3GAP2, (which acts as a GAP for Rab3 61 and a guanine nucleotide exchange factor (GEF) for Rab18 62 ), were all found to be NRP1-specific. Conversely, α5 integrin’s interactions with Rab13 63 , Golgin A4 (Golga4) and Golgi SNAP receptor complex 1 (Gosr1) 64 were identified as NRP2 dependent. Whilst these NRP-specific interactions should be explored further, we also urge others to consider and investigate NRP-specific modulation of α5 integrin in other cellular pathways, such as the cell cycle and during cell-stress responses, both of which showed an enriched interaction score favouring regulation by NRP1. As such, we propose this α5 integrin interactome dataset as a comprehensive map of candidate associations in ECs.
Despite their reciprocal contribution during Rab11-mediated recycling, we do find it interesting that NRP2 only preferentially associated with p120RasGAP on early endosomes when NRP1 expression is silenced. Furthermore, this interaction only becomes dependent upon FAK phosphorylation in si NRP1 ECs. As p120RasGAP and Rab21 compete for a mutually exclusive interaction with α-integrin tails 17 , we hypothesise that NRP1 and NRP2 also competitively associate with p120RasGAP during their transit to Rab11 recycling endosomes. In fibroblasts, fibronectin-integrin-mediated FAK activation was shown to promote SH2-mediated binding of p120RasGAP to p190RhoGAP. In turn, p190RhoGAP phosphorylation stabilised their complex association at leading edge adhesions to regulate cell polarisation 35 . We believe it is likely that internalised integrin-NRP complexes deliver active FAK to p120RasGAP in ECs, thereby promoting polarised integrin recycling and directional migration by facilitating p120-p190 association. For example, si p120RasGAP and si NRP1/2 ECs failed to assemble polarised adhesions and exhibited impaired directional migratory capacity. As p120RasGAP recruitment to membranes has also been connected to a transient attenuation in RhoA signalling and the formation of cell protrusions via upregulated Rac activity in surface adhesions 17 , 65 , it follows that p120RasGAP should be considered as a key regulator of integrin traffic and signalling to promote cell polarity in ECs. As Rac1 activity was found to be attenuated in ECs depleted for either NRP1 or NRP2 10 , 66 , 67 , and our MS analyses identified RacGAP1 as an NRP1-specific interactor of α5 integrin, we recommend future studies to pursue this crosstalk further.
Consistent with these conclusions, temporal endothelial-specific deletion of p120RasGAP has been demonstrated to significantly reduce vessel sprouting and filopodia production in the postnatal retina of P6 mice 16 , largely phenocopying the defects observed in retinas harvested from NRP1 flfl NRP2 flfl .EC KO animals. In addition to a loss of tip cell polarisation, NRP1 flfl NRP2 flfl .EC KO retinas also exhibited significant reductions in EDA-FN matrix deposition, substantiating our in vitro findings and those reported by Valdembri et al., who described a significant loss of insoluble FN fibres upon NRP1 silencing in human umbilical vein ECs (HUVECs) 8 . Indeed, FN fibrillogenesis relies on the polarised recycling of active α5 integrin 3 . We hypothesise that without stable cell-matrix interactions, NRP1 flfl NRP2 flfl .EC KO vasculature undergoes profound vessel regression at both the vascular front and within the remodelling plexus, resulting in severe vascular sprouting and branching defects.
In summary, we describe a model in which α5 integrin is endocytosed to the early endosome by both NRP1 and NRP2 receptors. The integrin-associated FAK activity mediates the displacement of Rab21 and the shuttling of integrin-NRP complexes to Rab11 + recycling endosomes via a transient but direct interaction with p120RasGAP.
The foremost caveat to any conclusions drawn from these results remains the absence of an in vitro human EC model to consolidate our findings. Whilst beyond the range of this study, it is important that this limitation be considered. For example, whilst the fundamental mechanisms of α5 integrin trafficking are conserved between mouse and human ECs 7 , 8 , 9 , 10 , expression profiles of NRP1 and NRP2 are likely to differ between (and indeed within) mouse and human endothelial models. For example, even between HUVECs, human microvascular ECs (MECs) and human lymphatic ECs (LECs), the surface density of NRP1 was found to vary markedly and be subject to change dependent on states of VEGF stimulation 68 . Whilst predominantly expressed in the arterial endothelium, NRP2, by contrast, is preferentially expressed on and regulates responses in the lymphatic endothelium 69 . To inform future studies, it would be greatly beneficial to utilise a parameterised computational model similar to that described by Sarabipour et al. 70 (taking into account receptor surface density), to provide mechanistic insight into the differences in trafficking kinetics of such receptors between and within mouse and human endothelial cell lines.
Antibodies and reagents
The following primary antibodies were used for experimental analyses: α5 integrin (CST: 4705S, RRID: AB_2233962, 1:1000; Ab25251, RRID: AB_448738, 1:200), β-actin (CST: 8457S, RRID: AB_10950489, 1:2000), NRP1 (R&D: AF566, RRID: AB_355445, 1:100), NRP2 (SCB: sc-13117, RRID: AB_628044, 1:50; CST: 3366, RRID: AB_2155250, 1:1000), Clathrin heavy chain-1 (Ab21679, RRID: AB_2083165, 1:100), Dynamin-2 (Ab3457, RRID: AB_2093679, 1:100), EEA1 (Ab2900, RRID: AB_2262056, 1:100), Rab11 (Ab3612, RRID: AB_10861613, 1:2000), Rab4 (Ab13252, RRID: AB_2269374, 1:100), Rab7 (CST: 17286, 1:100), αV integrin (Inv: PA5-47096, RRID: AB_2609681, 1:200/1:1000), Paxillin (Ab32084, RRID: AB_779033, 1:100; CST: 2542, RRID: AB_10693603, 1:1000), Tensin-1 (NB: NBP1-84129, RRID: AB_11014791, 1:100), EDA-FN (Sigma: F6140, RRID: AB_476981, 1:400), p120RasGAP (Ab2922, RRID: AB_303418, 1:100/1:1000), Rab21 (SCB: sc-81917, RRID: AB_2253236, 1:100/1:1000), Dynein (Thermo: MA1-070, RRID: AB_2093668, 1:200), FAK (CST: 3285, RRID: AB_2269034, 1:1000), p FAK Tyr397 (CST: 8556, RRID: AB_10891442, 1:1000), p FAK Tyr407 (Inv: 44-650 G, RRID: AB_2533708, 1:1000/1:200), GM130 (NB: NBP2-53420, RRID: AB_2916095, 1:100), Collagen IV (Ab19808, RRID: AB_445160, 1:500).
CST: Cell Signalling Technologies; R&D: R&D Systems; SCB: Santa Cruz Biotechnology; Ab: Abcam; Inv: Invitrogen; NB: Novus Biologicals
tdTOMATO- expressing α5 integrin constructs were generated by standard PCR protocols using the following primer sequences: 5’-CTATGGGGAGCTGGACGC-3’, 5’-GCATCTGAGGTGGCTGGA-3’. The corresponding PCR product was subsequently cloned into linearised tdTOMATO-C1 plasmid (Addgene #54653), before transfected into ECs by electroporation.
Animal breeding and generation
All experiments were performed in accordance with UK home office regulations and the European Legal Framework for the Protection of Animals used for Scientific Purposes (European Directive 86/609/EEC). All experiments were approved by the Animal Welfare and Ethical Review Board (AWERB) committee at the University of East Anglia, UK. We have complied with all relevant ethical regulations for animal use. NRP1 floxed (NRP1 flfl ) 71 and NRP2 floxed (NRP2 flfl ) 72 mice generated on a C57/BL6 background were purchased from The Jackson Laboratory (Bar Harbour, Maine, USA). α5 integrin-floxed (α5 flfl ) mice (generated on a C57/BL6 background) were kindly provided by Richard Hynes (Massachusetts Institute of Technology, USA). Floxed mice were crossed with tamoxifen-inducible PDGFB.iCreER T2 mice, provided by Marcus Fruttiger (UCL, London, UK). Gene deletion was achieved either via tamoxifen administration in vivo or TAT-Cre recombinase nucleofection in vitro.
Cell isolation, immortalisation and cell culture
Primary mouse lung microvascular endothelial cells (mLMECs) were isolated from age-matched (3–6 weeks) wild-type (WT) or floxed C57/BL6 mice. Cellular digests were expelled through a 19 gauge-needle and filtered through a 70 µm sterile strainer (Fisher Scientific). Cell pellets were seeded onto plasticware coated with a solution of 0.1% gelatin containing 10 µg/ml human plasma fibronectin (FN) (Millipore). and collagen type 1. mLMECs were twice positively selected for using endomucin primary antibody and magnetic activated cell sorting (MACS) as previously described by Reynolds & Hodivala-Dilke 73 , prior to immortalisation using polyoma-middle-T-antigen (PyMT) as previously described by Robinson et al. 74 .
ECs were cultured in a 1:1 mix of Ham’s F-12:Dulbecco’s Modified Eagle Medium (DMEM) (low glucose) medium supplemented with 10% foetal bovine serum (FBS), 100 units/mL penicillin/streptomycin (P/S) and 50 μg/mL heparin (Sigma) at 37 °C in a humidified incubator (+5% CO 2 ) unless otherwise stated.
For experimental analyses, plasticware was coated using 10 µg/ml human plasma FN passaging of ECs did not exceed 20. VEGF-stimulation was achieved using 30 ng/ml VEGF-A 164 (VEGF-A) (murine equivalent to VEGF-A 165 ) post 3 h incubation in serum-free medium (OptiMEM®; Invitrogen). VEGF-A was made in-house, as previously described by Krilleke et al. 75 .
TAT-Cre recombinase/siRNA transfection
To excise α5 integrin from ECs isolated from α5 integrin-floxed mice, ECs were twice nucleofected with TAT-Cre recombinase (70 units, Sigma) in the same manner as siRNA oligofection, before being allowed to recover for 24 h.
Trypsinised ECs were subject to oligofection using either non-targeting control siRNA (Ctrl) or murine-specific siRNA duplexes suspended in nucleofection buffer (200 mM Hepes, 137 mM NaCl, 5 mM KCl, 6 mM d -glucose and 7 mM Na 2 HPO 4 in nuclease-free water). Oligofection was performed according to manufacturer’s instructions using the Amaxa 4D-nucleofector system (Lonza).
NRP1 siGENOME siRNA duplexes: D-040787- 03 , D-040787- 04 NRP2 siGENOME siRNA duplexes: D-040423- 03 , D-040423- 04
Western blotting
ECs were lysed in lysis buffer (Tris-HCL: 65 mM pH 7.4, sucrose: 60 mM, 3% SDS), homogenised and analysed for protein concentration using the bicinchoninic acid (BCA) assay (Pierce). Equivalent protein concentrations were run on 8% polyacrylamide gels before being subject to SDS-PAGE. Proteins were transferred to a 0.45 µm Amersham Protran® nitrocellulose membrane (GE Healthcare, Amersham) before being incubated in primary antibody resuspended in 5% milk-powder at 4 °C. Membranes were washed with 0.1% Tween-20 in PBS (PBST) and incubated in appropriate horseradish peroxidase (HRP)-conjugated secondary antibody (Dako) diluted 1:2000 for 2 h at RT. Bands were visualised by incubation with a 1:1 solution of Pierce ECL Western Blotting Substrate (Thermo). Chemiluminescence was detected on a ChemiDoc TM MP Imaging System (BioRad). Densitometric readings of band intensities were obtained using ImageJ TM .
Co-immunoprecipitation assays
ECs were placed on ice before being lysed at 4 °C in lysis buffer (25 mM Tris-HCl, pH 7.4, 100 mM NaCl, 2 mM MgCl 2 , 1 mM Na 3 VO 4 , 0.5 mM EGTA, 1% Triton X-100, 5% glycerol, supplemented with Halt TM protease inhibitors) and cleared by centrifugation at 12,000 × g for 20 min at 4 °C. Supernatant proteins were then quantified using the BCA assay and equivalent protein concentrations (800 µg) were immunoprecipitated with Dynabeads TM Protein G (Invitrogen) coupled to a primary antibody at 4 °C. Immunoprecipitated proteins, alongside a control IgG sample (Fisher Scientific: SA1-200, RRID: AB_325994), were separated by SDS-PAGE and subjected to Western blot analysis.
Mass spectrometry analysis
Mass spectrometry was carried out by the Fingerprints Proteomics Facility, Dundee University, UK, on EC samples immunoprecipitated against α5 integrin primary antibody as described in ‘co-immunoprecipitation assays’. Briefly, IP samples were resolved via 1D SDS-PAGE stained with Quick Coomassie Stain. Gel lanes were then excised and subjected to in-gel processing before overnight trypsin digestion. Digested peptides were run on a Q-ExactivePlus (Thermo) instrument coupled to a Dionex Ultimate 3000 HPLC system. Raw data was acquired in Data Independent Acquisition (DIA) mode. A scan cycle comprised a full MS scan with an m/z range of 345–1155, resolution of 70,000, Automatic Gain Control (AGC) target of 3 × 10 6 and a maximum injection time of 200 ms. MS scans were followed by DIA scans of dynamic window widths with an overlap of 0.5 Th. DIA spectra were recorded with a first fixed mass of 200 m/z, resolution of 17,500, AGC target 3 × 10 6 and a maximum IT of 55 ms. The normalised collision energy was set to 25% with a default charge state set at 3. Data for both MS scan and MS/MS DIA scan events were acquired in profile mode. Raw data was analysed, and significance was calculated using Spectronaut v17.
STRING interaction networks analysing identified proteins were defined based on default parameters, with the minimum required confidence score of 0.400. Connecting line thickness corresponds to confidence of interaction.
Pathway enrichment analysis was performed using the Reactome database and software 76 . ‘Gene expression and maintenance’ and Protein and RNA metabolism’ pathways are not shown.
Immunocytochemistry
ECs were seeded at low density onto pre-coated, acid-washed, sterilised glass coverslips for the indicated timepoints before fixation in 4% paraformaldehyde (PFA). Fixed ECs were incubated with 10% goat serum in PBS 0.3% triton X-100 prior to primary antibody overnight at 4 °C. Following primary antibody incubation, ECs were incubated with an appropriate Alexa fluor secondary antibody diluted 1:200 before mounting using flouromount-G with DAPI TM (Invitrogen). Images were captured using a Zeiss LSM880 Airyscan Confocal microscope at 63x magnification with an Axiocam 503 mono camera. Adhesion number and size were quantified using ImageJ TM software as previously described by Lambert et al. 77 , using an FA size lower detection limit of 0.8 microns. Scratch-wound immunofluorescence studies were performed by making a scratch through confluent ECs, then allowing ECs to polarise for 8 h prior to PFA fixation.
RNA extraction and RT-qPCR analysis
Total RNA was extracted using the Promega SV Total RNA Isolation System (#Z3100) from Ctrl and siRNA-depleted ECs. cDNA was prepared using the Promega GoScript TM Reverse Transcriptase Kit (#A5000). RT-qPCR analysis was performed using a Viia7 RT-qPCR System using Promega GoTaq® PCR mastermix (#A6102) following the manufacturer’s instructions. The following oligonucleotide primer/probe sets were used: mouse NRP1: Mm00435379_m1; mouse NRP2: Mm00803099_m1; mouse GAPDH: Mm99999915_g1 (Thermo).
Adhesion assays
ECs were seeded onto 96-well microtiter plates (Costar) pre-coated with FN overnight, then incubated in 5% bovine serum albumin (BSA). ECs were left to adhere for the indicated timepoints before fixation in 4% PFA. Plates were incubated with methylene blue solution, then washed in dH2O prior to de-stain solution (1:1 ethanol:0.1 M HCL). Absorbance was read at 630 nm and normalised to media-only control wells.
Recycling assays
ECs were placed on ice before being washed in Soerensen buffer (SBS) pH 7.8 (14.7 mM KH 2 PO 4 , 2 mM Na 2 HPO 4 and 120 mM Sorbitol pH 7.8) as previously described by Remacle et al. 78 . Cell surface proteins were subsequently labelled with 0.3 mg/ml EZ-Link™ Sulfo-NHS-SS-Biotin (Thermo Scientific) at 4 °C. Unreacted biotin was quenched with 100 mM glycine, before biotin-labelled surface proteins were allowed to internalise for 20 min at 37 °C. Following internalisation, ECs were washed with SBS buffer pH 8.2, and incubated with 100 mM sodium 2-mercaptoethane sulfonate (MESNA) at 4 °C. The internalised fraction was then allowed to recycle for the indicated timepoints at 37 °C. Following each timepoint, ECs were again subjected to MESNA incubation at 4 °C, alongside a MESNA-control sample. Excess MESNA was quenched with 100 mM iodoacetamide (Sigma) at 4 °C, prior to a further SBS pH 8.2 wash. ECs were then lysed at 4 °C in lysis buffer (25 mM Tris-HCl, pH 7.4, 100 mM NaCl, 2 mM MgCl 2 , 1 mM Na 3 VO 4 , 0.5 mM EGTA, 1% Triton X-100, 5% glycerol, supplemented with Halt TM protease inhibitors) and cleared by centrifugation at 12,000 × g for 20 min at 4 °C. Supernatant proteins were then quantified using the BCA assay and equivalent protein concentrations (800 µg) were immunoprecipitated with Dynabeads TM Protein G (Invitrogen) coupled to a mouse anti-biotin primary antibody at 4 °C. Immunoprecipitated biotin-labelled proteins were then separated by SDS-PAGE and subjected to Western blot analysis. The extent of recycled α5 integrin was determined by normalising MESNA-treated samples against their respective internal MESNA - control at each timepoint.
Retinal angiogenesis assays
Inducible, endothelial-specific deletion of NRP1 and/or NRP2 was achieved by subcutaneous tamoxifen injections (50 µl, 2 mg/ml stock) on postnatal (P) days 2–3, followed by intraperitoneal (IP) injections of the same dose on P4-P5. Mice were sacrificed on P6, and retinas were harvested as previously described. Dissected retinas were fixed in PFA for 30 min before permeabilised in PBS 0.25% triton X-100. Retinas were then incubated in Dako serum-free protein blocking solution for 1 h, before incubated in primary antibody. Following primary antibody incubation, retinas were washed in 0.1% triton X-100 and incubated in the appropriate Alexa fluor secondary antibody before being mounted with Flouromount-G. Images were captured using a Zeiss LSM880 Airyscan Confocal microscope with an Axiocam 503 mono camera. All analyses were performed using ImageJ TM software unless otherwise stated. AngioTool TM software was used to analyse mean vascular density and vessel branching from multiple ROIs per retinal leaflet. The mean number of EC sprouts/retina and filopodia number/sprouting EC were enumerated manually. EDA-FN expression was measured using relative corrected total cell fluorescence (CTCF) values (calculated according to the formula: IntDen - area * background IntDen).
Statistics and reproducibility
All graphic illustrations and analyses to determine statistical significance were generated using GraphPad Prism 9 software unless otherwise stated. Student’s t -tests were used to determine statistical significance between Ctrl and an individually shown siRNA group, or between an individual, paired Cre-negative to Cre-positive comparison. Statistical analysis between Ctrl and multiple siRNA groups, or between NRP1 flfl .EC KO , NRP2 flfl .EC KO , and NRP1 flfl NRP2 flfl .EC KO groups were performed using one-way ANOVA tests with post hoc multiple comparison tests. Bar charts show mean values with standard error of the mean (+SEM). Polarity histogram analyses were performed using NCSS software. Asterisks indicate the statistical significance of p values: p > 0.05 ns (not significant), * p < 0.05, ** p < 0.01, *** p < 0.001 and **** p < 0.0001.
Reporting summary
Further information on research design is available in the Nature Portfolio Reporting Summary linked to this article.
Data availability
The raw data supporting the conclusions of this article will be made available by the authors, without undue reservation, to any qualified researcher. The source data behind the graphs in the paper can be found in Supplementary Data 1 . Uncropped and unedited blot/gel images can be found in Supplementary Figs. 7 – 9 . The mass spectrometry proteomics data have been deposited to the ProteomeXchange Consortium via the PRIDE 79 partner repository with the dataset identifier PXD051778.
Hynes, R. O. Review integrins: bidirectional, allosteric signaling machines. Cell 110 , 673–687 (2002).
Article CAS PubMed Google Scholar
Zovein, A. C. et al. β1 integrin establishes endothelial cell polarity and arteriolar lumen formation via a Par3-dependent mechanism. Dev. Cell 18 , 39–51 (2010).
Article CAS PubMed PubMed Central Google Scholar
Mana, G. et al. PPFIA1 drives active α5β1 integrin recycling and controls fibronectin fibrillogenesis and vascular morphogenesis. Nat. Commun. 7 , 13546 (2016).
Pellinen, T. et al. Small GTPase Rab21 regulates cell adhesion and controls endosomal traffic of β1-integrins. J. Cell Biol. 173 , 767–780 (2006).
Subramani, D. & Alahari, S. K. Integrin-mediated function of Rab GTPases in cancer progression. Mol. Cancer 9 , 312 (2010).
Roberts, M., Barry, S., Woods, A., Van Der Sluijs, P. & Norman, J. PDGF-regulated Rab4-dependent recycling of v3 integrin from early endosomes is necessary for cell adhesion and spreading. Curr. Biol. 11 , 1392–1402 (2001).
Caswell, P. T. & Norman, J. C. Integrin trafficking and the control of cell migration. Traffic 7 , 14–21 (2006).
Valdembri, D. et al. Neuropilin-1/GIPC1 signaling regulates α5β1 integrin traffic and function in endothelial cells. PLoS Biol. 7 , e25 (2009).
Article PubMed Google Scholar
Benwell, C. J., Taylor, J. A. G. E. & Robinson, S. D. Endothelial neuropilin-2 influences angiogenesis by regulating actin pattern development and α5-integrin-p-FAK complex recruitment to assembling adhesion sites. FASEB J. 35 , e21679 (2021).
Alghamdi, A. A. A. et al. NRP2 as an emerging angiogenic player; promoting endothelial cell adhesion and migration by regulating recycling of α5 integrin. Front. Cell Dev. Biol. 8 , 395 (2020).
Article PubMed PubMed Central Google Scholar
Fantin, A. et al. NRP1 regulates CDC42 activation to promote filopodia formation in endothelial tip cells. Cell Rep. 11 , 1577–1590 (2015).
Fantin, A. et al. NRP1 acts cell autonomously in endothelium to promote tip cell function during sprouting angiogenesis. Blood 121 , 2352–2362 (2013).
Benwell, C. J., Johnson, R. T., Taylor, J. A. G. E., Price, C. A. & Robinson, S. D. Endothelial VEGFR coreceptors neuropilin-1 and neuropilin-2 are essential for tumor angiogenesis. Cancer Res. Commun. 2 , 1626–1640 (2022).
Chehayeb, R. J., Wang, J., Stiegler, A. L. & Boggon, T. J. The GTPase-activating protein p120RasGAP has an evolutionarily conserved ‘FLVR-unique’ SH2 domain. J. Biol. Chem. 295 , 10511–10521 (2020).
Article CAS Google Scholar
Vish, K. J., Stiegler, A. L. & Boggon, T. J. Diverse p120RasGAP interactions with doubly phosphorylated partners EphB4, p190RhoGAP, and Dok1. J. Biol. Chem. 299 , 105098 (2023).
Chen, D., Teng, J. M., North, P. E., Lapinski, P. E. & King, P. D. RASA1-dependent cellular export of collagen IV controls blood and lymphatic vascular development. J. Clin. Invest. 129 , 3545–3561 (2019).
Mai, A. et al. Competitive binding of Rab21 and p120RasGAP to integrins regulates receptor traffic and migration. J. Cell Biol. 194 , 291–306 (2011).
Eggers, C. T., Schafer, J. C., Goldenring, J. R. & Taylor, S. T. D-AKAP2 interacts with Rab4 and Rab11 throughi its RGS domains and regulates transferrin receptor recycling. J. Biol. Chem. 284 , 32869–32880 (2009).
Arjonen, A., Alanko, J., Veltel, S. & Ivaska, J. Distinct recycling of active and inactive β1 integrins. Traffic 13 , 610–625 (2012).
Koivunens, E., Gay, D. A. & Ruoslahti, E. Selection of peptides binding to the A5b1 integrin from phage display library. J. Biol. Chem. 268 , 20205–20210 (1993).
Article Google Scholar
Stenzel, D. et al. Integrin-dependent and -independent functions of astrocytic fibronectin in retinal angiogenesis. Development 138 , 4451–4463 (2011).
Zhang, Z. et al. The alpha V beta1 integrin functions as a fibronectin receptor but does not support fibronectin matrix assembly and cell migration on fibronectin. J. Cell Biol. 22, 235–242 (1993).
Van Der Flier, A. et al. Endothelial α5 and αv integrins cooperate in remodeling of the vasculature during development. Development 137 , 2439–2449 (2010).
Danen, E. H. J., Sonneveld, P., Brakebusch, C., Fässler, R. & Sonnenberg, A. The fibronectin-binding integrins α5β1 and αvβ3 differentially modulate RhoA-GTP loading, organization of cell matrix adhesions, and fibronectin fibrillogenesis. J. Cell Biol. 159 , 1071–1086 (2002).
Kau, D. K., Finnegan, E. & Barabino, G. A. Sickle red cell-endothelium interactions. Microcirculation 16 , 97–111 (2009).
Barber-Pérez, N. et al. Mechano-responsiveness of fibrillar adhesions on stiffness-gradient gels. J. Cell Sci. 133 , jcs242909 (2020).
Zamir, E. et al. Dynamics and segregation of cell-matrix adhesions in cultured fibroblasts. Nat. Cell Biol. 2 , 191–196 (2000).
Ajeian, J. N. et al. Proteomic analysis of integrin-associated complexes from mesenchymal stem cells. Proteomics Clin. Appl. 10 , 51–57 (2016).
Sundararaman, A., Fukushima, Y., Norman, J. C., Uemura, A. & Mellor, H. RhoJ regulates α5β1 integrin trafficking to control fibronectin remodeling during angiogenesis. Curr. Biol. 30 , 2146–2155.e5 (2020).
Conklin, M. W., Ada-Nguema, A., Parsons, M., Riching, K. M. & Keely, P. J. R-Ras regulates b 1-integrin trafficking via effects on membrane ruffling and endocytosis. BMC Cell Biol. 11 , 14 (2010).
Osman, M. A. An emerging role for IQGAP1 in regulating protein traffic. ScientificWorldJournal 10 , 944–953 (2010).
Kakuta, S. et al. Small GTPase Rab1B is associated with ATG9A vesicles and regulates autophagosome formation. FASEB J. 31 , 3757–3773 (2017).
Kawasaki, N. et al. TUFT1 interacts with RABGAP1 and regulates mTORC1 signaling. Cell Discov . 4 , 1 (2018).
Kimura, T. et al. Activated Cdc42-bound IQGAP1 determines the cellular endocytic site. Mol. Cell Biol. 33 , 4834–4843 (2013).
Tomar, A., Lim, S. T., Lim, Y. & Schlaepfer, D. D. A FAK-p120RasGAP-p190RhoGAP complex regulates polarity in migrating cells. J. Cell Sci. 122 , 3005 (2009).
Article CAS PubMed Central Google Scholar
Stokes, J. B. et al. Inhibition of focal adhesion kinase by PF-562,271 inhibits the growth and metastasis of pancreatic cancer concomitant with altering the tumor microenvironment. Mol. Cancer Ther. 10 , 2135–2145 (2011).
Lv, Z., Li, W. & Wei, X. S100a9 promotes prostate cancer cell invasion by activating tlr4/nf-κb/integrin β1/fak signaling. Onco Targets Ther. 13 , 6443–6452 (2020).
Crompton, B. D. et al. High-throughput tyrosine kinase activity profiling identifies FAK as a candidate therapeutic target in ewing sarcoma. Cancer Res. 73 , 2873–2883 (2013).
Marjanović, M. et al. CEP63 deficiency promotes p53-dependent microcephaly and reveals a role for the centrosome in meiotic recombination. Nat. Commun. 6 , 7676 (2015).
Lo, K. W. H., Kogoy, J. M. & Pfister, K. K. The DYNLT3 light chain directly links cytoplasmic dynein to a spindle checkpoint protein, Bub3. J. Biol. Chem. 282 , 11205–11212 (2007).
Robinson, R. T. IL12Rβ1: the cytokine receptor that we used to know. Cytokine 71 , 348–359 (2015).
Yan, H., Zhang, L., Cui, X., Zheng, S. & Li, R. Roles and mechanisms of the m6A reader YTHDC1 in biological processes and diseases. Cell Death Discov. 8 , 237 (2022).
Urnavicius, L. et al. The structure of the dynactin complex and its interaction with dynein. Science 347 , 1441–1446 (2015).
Jordens, I. et al. The Rab7 effector protein RILP controls lysosomal transport by inducing the recruitment of dynein-dynactin motors. Curr. Biol. 1 , 1680–5 (2001).
Cantalupo, G., Alifano, P., Roberti, V., Bruni, C. B. & Bucci, C. Rab-interacting lysosomal protein (RILP): the Rab7 effector required for transport to lysosomes. EMBO J. 20 , 683–693 (2001).
Cullen, P. J. & Steinberg, F. To degrade or not to degrade: mechanisms and significance of endocytic recycling. Nat. Rev. Mol. Cell Biol. 19 , 679–696 (2018).
Simonetti, B. et al. ESCPE-1 mediates retrograde endosomal sorting of the SARS-CoV-2 host factor Neuropilin-1. Proc. Natl Acad. Sci. USA 119 , e2201980119 (2022).
Li, S. et al. The role of the dynamics of focal adhesion kinase in the mechanotaxis of endothelial cells. Proc. Natl Acad. Sci. USA www.pnas.org (2001).
Jing, J. & Prekeris, R. Polarized endocytic transport: the roles of Rab11 and Rab11-FIPs in regulating cell polarity. Histol. Histopathol. 24 , 1171–1180 (2009).
CAS PubMed PubMed Central Google Scholar
Ossipova, O. et al. Role of Rab11 in planar cell polarity and apical constriction during vertebrate neural tube closure. Nat. Commun. 5 , 3734 (2014).
Prekeris, R., Klumperman, J. & Scheller, R. H. A Rab11/Rip11 protein complex regulates apical membrane trafficking via recycling endosomes. Mol. Cell 6 , 1437–1448 (2000).
Turner, C. J., Badu-Nkansah, K. & Hynes, R. O. Endothelium-derived fibronectin regulates neonatal vascular morphogenesis in an autocrine fashion. Angiogenesis 20 , 519–531 (2017).
Seerapu, H. R. et al. The cytoplasmic domain of neuropilin-1 regulates focal adhesion turnover. FEBS Lett. 587 , 3392–3399 (2013).
Ballmer-Hofer, K., Andersson, A. E., Ratcliffe, L. E. & Berger, P. Neuropilin-1 promotes VEGFR-2 trafficking through Rab11 vesicles thereby specifying signal output. Blood 118 , 816–826 (2011).
Zheng, R. et al. KIF2C regulates synaptic plasticity and cognition in mice through dynamic microtubule depolymerization. Elife 11 , e72483 (2022).
Yu, Y. et al. Inhibition of KIF22 suppresses cancer cell proliferation by delaying mitotic exit through upregulating CDC25C expression. Carcinogenesis 35 , 1416–1425 (2014).
Joseph, N. F., Swarnkar, S. & Puthanveettil, S. V. Double duty: mitotic kinesins and their post-mitotic functions in neurons. Cells 10 , 1–11 (2021).
Yang, W. X. & Sperry, A. O. C-Terminal kinesin motor KIFC1 participates in acrosome biogenesis and vesicle transport. Biol. Reprod. 69 , 1719–1729 (2003).
Sheng, L., Hao, S. L., Yang, W. X. & Sun, Y. The multiple functions of kinesin-4 family motor protein KIF4 and its clinical potential. Gene 678 , 90–99 (2018).
Søreng, K. et al. SNX 18 regulates ATG 9A trafficking from recycling endosomes by recruiting Dynamin‐2. EMBO Rep. 19 , e44837 (2018).
Nagano, F. et al. Molecular cloning and characterization of the noncatalytic subunit of the Rab3 subfamily-specific GTPase-activating protein. J. Biol. Chem. 273 , 24781–24785 (1998).
Gerondopoulos, A. et al. Rab18 and a Rab18 GEF complex are required for normal ER structure. J. Cell Biol. 205 , 707–720 (2014).
Nokes, R. L., Fields, I. C., Collins, R. N. & Fölsch, H. Rab13 regulates membrane trafficking between TGN and recycling endosomes in polarized epithelial cells. J. Cell Biol. 182 , 845–853 (2008).
Wong, M. & Munro, S. The specificity of vesicle traffic to the Golgi is encoded in the golgin coiled-coil proteins. Science 346 , 1256898 (2014).
Tomar, A. & Schlaepfer, D. D. Focal adhesion kinase: switching between GAPs and GEFs in the regulation of cell motility. Curr. Opin. Cell Biol. 21 , 676–683 (2009).
Cao, S. et al. Neuropilin-1 promotes cirrhosis of the rodent and human liver by enhancing PDGF/TGF-β signaling in hepatic stellate cells. J. Clin. Invest. 120 , 2379–2394 (2010).
Riese, A. et al. Epidermal expression of neuropilin 1 protects murine keratinocytes from UVB-induced apoptosis. PLoS ONE 7 , e50944 (2012).
Imoukhuede, P. I. & Popel, A. S. Quantification and cell-to-cell variation of vascular endothelial growth factor receptors. Exp. Cell Res. 317 , 955–965 (2011).
Bouvrée, K. et al. Semaphorin3A, neuropilin-1, and plexinA1 are required for lymphatic valve formation. Circ. Res. 111 , 437–45 (2012).
Sarabipour, S. et al. Trafficking dynamics of VEGFR1, VEGFR2, and NRP1 in human endothelial cells. PLoS Comput. Biol. 20 , e1011798 (2024).
Gu, C. et al. Neuropilin-1 conveys semaphorin and VEGF signaling during neural and cardiovascular development. Dev. Cell 5 , 45–57 (2003).
Walz, A., Rodriguez, I. & Mombaerts, P. Aberrant sensory innervation of the olfactory bulb in neuropilin-2 mutant mice. J. Neurosci. 22 , 4025-4035 (2002).
Reynolds, L. E. & Hodivala-Dilke, K. M. in Breast Cancer Research Protocols Ch. 35 (Humana Press, 2006).
Robinson, S. D. et al. αvβ3 integrin limits the contribution of neuropilin-1 to vascular endothelial growth factor-induced angiogenesis. J. Biol. Chem. 284 , 33966–33981 (2009).
Krilleke, D. et al. Molecular mapping and functional characterization of the VEGF164 heparin-binding domain. J. Biol. Chem. 282 , 28045–28056 (2007).
Fabregat, A. et al. Reactome diagram viewer: data structures and strategies to boost performance. Bioinformatics 34 , 1208–1214 (2018).
Lambert, J. et al. ADAMTS-1 and syndecan-4 intersect in the regulation of cell migration and angiogenesis. J. Cell Sci . 133 , jcs235762 (2020).
Remacle, A., Murphy, G. & Roghi, C. Membrane type I-matrix metalloproteinase (MT1-MMP) is internalised by two different pathways and is recycled to the cell surface. J. Cell Sci. 116 , 3905–3916 (2003).
Perez-Riverol, Y. et al. The PRIDE database resources in 2022: A Hub for mass spectrometry-based proteomics evidences. Nucleic Acids Res. 50 , D543–D552 (2022).
Download references
Acknowledgements
This work was supported by funding from BHF (grant number PG/22/11033); S.D.R. gratefully acknowledges the support of the Biotechnology and Biological Sciences Research Council (BBSRC); this research was partially funded by the BBSRC Institute Strategic Programme Food Microbiome and Health BB/X011054/1 and its constituent project BBS/E/F/000PR13632.
Author information
Robert T. Johnson
Present address: Department of Biomedicine, Aarhus University, Aarhus, Denmark
Jordi Lambert
Present address: Section of Cardiorespiratory Medicine, University of Cambridge, VPD Heart & Lung Research Institute, Papworth Road, Cambridge Biomedical Campus, Cambridge, UK
Authors and Affiliations
Food Microbiome and Health Programme, Quadram Institute Bioscience, Norwich Research Park, Norwich, UK
Christopher J. Benwell, Robert T. Johnson, James A. G. E. Taylor, Jordi Lambert & Stephen D. Robinson
School of Biological Sciences, University of East Anglia, Norwich Research Park, Norwich, UK
You can also search for this author in PubMed Google Scholar
Contributions
Conceptualisation: C.J.B. and S.D.R.; Formal analyses and investigation: C.J.B., R.T.J. and J.A.G.E.T.; Resources, supervision and funding acquisition: S.D.R.; Review and editing: C.J.B., R.T.J., J.A.G.E.T., J.R.L. and S.D.R.; Visualisation: C.J.B., R.T.J. and J.A.G.E.T.
Corresponding authors
Correspondence to Christopher J. Benwell or Stephen D. Robinson .
Ethics declarations
Competing interests.
The authors declare no competing interests.
Peer review
Peer review information.
Communications Biology thanks the anonymous reviewers for their contribution to the peer review of this work. Primary Handling Editor: Manuel Breuer. A peer review file is available.
Additional information
Publisher’s note Springer Nature remains neutral with regard to jurisdictional claims in published maps and institutional affiliations.
Supplementary information
Peer review file, supplementary figs., description of additional supplementary files, reporting summary, rights and permissions.
Open Access This article is licensed under a Creative Commons Attribution 4.0 International License, which permits use, sharing, adaptation, distribution and reproduction in any medium or format, as long as you give appropriate credit to the original author(s) and the source, provide a link to the Creative Commons licence, and indicate if changes were made. The images or other third party material in this article are included in the article’s Creative Commons licence, unless indicated otherwise in a credit line to the material. If material is not included in the article’s Creative Commons licence and your intended use is not permitted by statutory regulation or exceeds the permitted use, you will need to obtain permission directly from the copyright holder. To view a copy of this licence, visit http://creativecommons.org/licenses/by/4.0/ .
Reprints and permissions
About this article
Cite this article.
Benwell, C.J., Johnson, R.T., Taylor, J.A.G.E. et al. A proteomics approach to isolating neuropilin-dependent α5 integrin trafficking pathways: neuropilin 1 and 2 co-traffic α5 integrin through endosomal p120RasGAP to promote polarised fibronectin fibrillogenesis in endothelial cells. Commun Biol 7 , 629 (2024). https://doi.org/10.1038/s42003-024-06320-4
Download citation
Received : 24 December 2023
Accepted : 09 May 2024
Published : 24 May 2024
DOI : https://doi.org/10.1038/s42003-024-06320-4
Share this article
Anyone you share the following link with will be able to read this content:
Sorry, a shareable link is not currently available for this article.
Provided by the Springer Nature SharedIt content-sharing initiative
By submitting a comment you agree to abide by our Terms and Community Guidelines . If you find something abusive or that does not comply with our terms or guidelines please flag it as inappropriate.
Quick links
- Explore articles by subject
- Guide to authors
- Editorial policies
Sign up for the Nature Briefing newsletter — what matters in science, free to your inbox daily.

- Open access
- Published: 28 May 2024
The effect of information provision on consumers’ risk perceptions of, support for a ban, and behavioral intention towards the preventive use of antibiotics in food animals
- Yingnan Zhou 1 , 2 ,
- Airong Zhang 1 ,
- Rieks Dekker van Klinken 1 &
- Junxiu Wang 3 , 4
BMC Public Health volume 24 , Article number: 1428 ( 2024 ) Cite this article
63 Accesses
Metrics details
Antibiotics have been widely used in feed and drinking water for food animals to prevent them from getting sick. Such preventive use of antibiotics has become a contributor to increasing antibiotic resistance and thus poses threats to human health. However, consumers have little knowledge about this practice and the associated health risks of increasing transmission of antibiotic residues and antibiotic resistant bacteria. This study aimed to examine the effect of information provision on consumers’ risk perceptions, support for a ban, and behavioral intention regarding the preventive use of antibiotics in food animals. Especially, the study sought to test two competing hypotheses which were informed by two theoretical perspectives of fear appeal theory — the linear model and the plateau effect model. The former suggested that providing information on the health risks of both antibiotic residues and antibiotic resistant bacteria would have a stronger effect compared to providing information on only one of them, while the latter posited that providing information on both risks might not have additional influence, as the effect of information on either risk could reach the plateau.
An experimental study with four conditions was conducted where participants read different information on the health risks associated with the preventive use first and then answered questions regarding consumers’ risk perceptions, support for a ban, and behavioral intention regarding the preventive use. Condition 1 was the control condition, where basic information about antibiotics, antibiotic resistance, and the preventive use was provided. Condition 2 and Condition 3 further added information on the health risk of antibiotic residues (Condition 2) and antibiotic resistant bacteria (Condition 3) due to the preventive use, respectively. Condition 4 provided all information contained in the first three conditions.
The results showed that compared to participants in the control condition, participants in Conditions 2-4 reported higher risk perceptions, stronger support for a ban on the preventive use, and a higher intention to buy meat produced without the preventive use of antibiotics. However, there were no significant differences in these factors between Conditions 2-4, indicating that providing information on the health risk of either antibiotic residues, or antibiotic resistant bacteria, or both, has similar effect on these variables. That is, the hypothesis based on the plateau effect model was supported.
Conclusions
The findings suggested that informing the public with the health risk of either antibiotic residues or antibiotic resistant bacteria associated with the preventive use is effective enough to reach plateau effect in increasing risk perceptions, support for a ban, and behavioral intention, which has important implications for policymakers and livestock industries to develop effective communication strategies to promote responsible antibiotic use in food animals.
Peer Review reports
Antibiotic resistance has posed a serious health threat worldwide. New antibiotic resistant bacteria are emerging and spreading globally, leading to increased mortality and higher health care burden [ 1 ]. It was estimated that antibiotic resistant infections were responsible for 1.27 million deaths in 2019 [ 2 ]. Without urgent action, the figure is projected to reach 10 million deaths every year by 2050 [ 1 ]. Moreover, antibiotic resistant bacteria are spreading within and between different ecosystems, thus has become a global ecological problem affecting the health of humans, animals, and the environment [ 3 , 4 ]. Antibiotic resistance is largely accelerated by the inappropriate use and overuse of antibiotics in multiple sectors, especially in humans and food animals [ 5 ]. The use of antibiotics and the action taken to control antibiotic resistance in one sector affects the others [ 5 , 6 ]. Therefore, to address the health threat posed by increasing antibiotic resistance, it’s necessary to take a One Health approach [ 3 , 7 , 8 ]. One Health is an integrated and unifying approach that mobilizes collaborative effort of multiple sectors, disciplines, and communities to sustainably balance and optimize the health of humans, animals, and the environment [ 9 ]. From One Health perspective, it’s important to reduce the inappropriate use of antibiotics in both humans and food animals. Although a growing body of evidence has demonstrated the link between antibiotic use in food animals and increasing antibiotic resistance [ 10 , 11 , 12 , 13 ], compared to antibiotic misuse and antibiotic resistance in humans, inappropriate use of antibiotics and antibiotic resistance in food animals has drawn less attention [ 14 ].
Antibiotics administered to food animals include therapeutic use and subtherapeutic use. Therapeutic use refers to using antibiotics to treat infectious diseases in sick animals [ 15 ]. The therapeutic use of antibiotics is essential for treating bacterial infections and protecting animal welfare. Subtherapeutic use refers to administering antibiotics to animals with doses lower than therapeutic use for a longer period to prevent healthy animals from getting sick or to promote growth [ 16 , 17 , 18 ]. In this case, antibiotics are usually added to feed or water [ 19 , 20 ]. Recently, many countries (e.g., the EU, the US, and China) have banned antibiotics from being used as growth promoters [ 21 , 22 , 23 ].
The preventive use of antibiotics was, however, only banned in the EU countries since 2022 and is still allowed in most countries globally [ 19 , 24 ]. Farmers routinely added antibiotics in feed or water to prevent diseases in groups of animals in various countries such as Brazil [ 25 ], Cambodia [ 26 ], Thailand [ 27 ], Vietnam [ 28 ], and China [ 29 ]. In Thailand, an average of 303 mg of antibiotics including tilmicosin, doxycycline, amoxicillin, colistin, and oxytetracycline were given to each chicken for disease prevention during the 41 days of raising period [ 27 ]. In Brazil, antibiotics were widely used for disease prevention among sows, newborn piglets, and weaning pigs [ 25 ]. Farmers alternately used antibiotics of different classes (e.g., aminopenicillin, pleuromutilin, amphenicol, polymyxin, tetracycline, quinolone, and macrolide) to prevent diseases in piglets. As a result, a piglet could be exposed to more than five antibiotic classes between 28 and 70 days of life [ 25 ]. Such preventative use of antibiotics in healthy animals may have serious consequences for human health [ 5 , 30 ]. Therefore, the present study focused on the preventive use of antibiotics in food animals.
The health risk associated with antibiotic residues due to the preventive use of antibiotics in food animals
The preventive use of antibiotics in food animals may result in antibiotic residues presenting in food [ 16 , 31 , 32 ]. Antibiotic residues have been found in various animal-derived food, such as meat, fish, milk, and eggs in many countries and regions globally (e.g., the US, Brazil, Cameroon, Egypt, Ghana, Greece, Nigeria, Bangladesh, Zambia, Iran, Kenya, China, India, and South Africa) [ 32 , 33 ]. High concentration of antibiotic residues in food can have direct toxic effects on human beings [ 16 , 31 , 32 ]. Among those, allergic reactions against β -lactam antibiotic (e.g., cephalosporin and penicillin) residues in meat or milk are most common [ 6 ]. The symptoms may include acute interstitial nephritis, vasculitis, skin rashes, bronchospasm, acute interstitial nephritis, vasculitis, serum sickness, erythema multiforme, toxic epidermal necrolysis, hemolytic anemia, anaphylaxis thrombocytopenia, angioedema, and Stevens–Johnson syndrome [ 6 , 34 ]. Antibiotic residues in food may also damage the immune system, organs (i.e., liver, kidneys, and reproductive organs), and bone marrow, as well as increase the chance of mutations and carcinoma [ 31 , 35 , 36 ].
The health risk associated with antibiotic resistant bacteria due to the preventive use of antibiotics in food animals
The preventive use of antibiotics in food animals has become a great contributor to the increase of antibiotic resistant bacteria both in animals and in the environment, thus increases the risk of humans getting infected with antibiotic resistant bacteria [ 37 , 38 , 39 , 40 ]. Studies have found a strong association between the prevalence of antibiotic resistant bacteria in food animals and in human beings [ 41 , 42 ]. The most common antibiotic resistant foodborne bacteria affecting human health are antibiotic resistant E. coli, salmonella, campylobacters, and enterococci [ 16 , 43 ]. A growing body of evidence has shown an increasing prevalence of these antibiotic resistant bacteria in animals. For instance, Roth et al. investigated the prevalence of antibiotic resistant bacteria in poultry in the US, China, Brazil, and the EU. They found the average proportion of antibiotic resistant E. coli isolated from chickens was over 40% [ 44 ]. Van Boeckel et al. revealed that the prevalence of antibiotic resistant bacteria (i.e., E. coli, campylobacters, salmonella, and staphylococcus aureus) in chickens, pigs, and cattle has all largely increased from 2000 to 2018 in low- and middle-income countries [ 14 ]. Furthermore, animals cannot fully metabolize the administered antibiotics. Consequently, antibiotic residues and antibiotic resistant bacteria are discharged into the environment (i.e., water and soils) through manure [ 20 , 45 , 46 ]. High level of antibiotic resistant bacteria has been found in almost all parts of the environment, including soil [ 47 , 48 ], freshwater aquaculture ponds [ 49 ], rivers [ 50 ], groundwater [ 51 ], sediments and sea water [ 52 ]. Consequently, humans may get infected with antibiotic resistant bacteria when they have direct contact with animals, handle contaminated food, consume undercooked food, consume contaminated water and vegetables, or have contact with antibiotic resistant bacteria in the environment [ 4 , 53 ]. If humans are infected with antibiotic resistant bacteria, it would be more difficult, or even impossible to treat as existing antibiotics have become ineffective in treating these bacterial infections [ 1 ]. For instance, antibiotic resistant foodborne bacteria E. coli and Staphylococcus aureus infections were responsible for over 500, 000 deaths in 2019 [ 2 ].
Consumers’ knowledge about, perceptions of, and attitudes towards the preventive use of antibiotics in food animals
Research so far has mainly focused on investigating consumers’ knowledge about, perceptions of, and attitudes towards the overall use of antibiotics in food animals [ 54 , 55 , 56 , 57 , 58 , 59 , 60 , 61 , 62 , 63 , 64 , 65 ]. The results of these studies suggested that, although having little knowledge about antibiotic use in food animals and its contribution to antibiotic resistance, consumers somehow believed antibiotics are widely used in livestock industries and concerned about it [ 54 , 55 , 56 , 57 , 58 , 59 , 60 , 62 , 63 ]. Moreover, the public concern about overall antibiotic use in food animals has facilitated the purchase demand and higher willingness to pay for animal-derived food produced without any use of antibiotics [ 66 , 67 ]. In response to this consumer demand, “antibiotic-free” or “raised without antibiotics” labelled food products have emerged in many countries (e.g., the US, Germany, the UK, Italy, and Australia) [ 55 , 56 , 68 , 69 ]. However, removing therapeutic use of antibiotics in livestock is detrimental to both animal welfare and food safety [ 55 , 70 ]. Therefore, from the perspective of One Health, it’s of extreme importance to promote responsible antibiotic use in food animals rather than eliminating antibiotics altogether, as it can balance and optimize the health of humans, animals, and the environment. The purchase demand for antibiotic-free animal-derived food might be due to concern about food safety and preference for less chemicals and additives in food [ 64 , 71 , 72 ], the lack of knowledge about the difference between therapeutic and subtherapeutic use of antibiotics, and the misunderstanding that all antibiotics used in food animals are harmful [ 73 ].
Hence, it’s necessary to differentiate the preventative use from therapeutic use of antibiotics, and to investigate consumers’ knowledge about, perceptions of, and attitudes towards the preventive use of antibiotics separately. However, only limited studies have shed some light on this area. Research in Ireland found most consumers were unfamiliar with the preventive use of antibiotics in food animals and were surprised when being informed about it, because they thought that antibiotics can only be used for treatments [ 62 ]. On the other hand, some consumers considered the preventive use of antibiotics as a normative and standard practice in farming [ 62 ]. Research in the US revealed that only about one third of consumers were very concerned about the preventive use of antibiotics in food animals and even less of them considered the preventive use as unacceptable [ 61 ]. These findings suggested that consumers have little knowledge about the preventive use of antibiotics in food animals and limited understanding of the health risks associated with it.
Emerging experimental studies suggested that receiving information on the health risk of antibiotic resistance associated with antibiotic use in food animals could significantly increase consumers’ knowledge and risk perception regarding antibiotic use and antibiotic resistance in food animals [ 60 , 74 ] as well as willingness to pay for antibiotic-free animal-derived food [ 66 , 67 ]. However, some of these studies focused on the hazard of using antibiotics for promoting growth [ 66 ]. While others focused on the health risk in relation to the overall use of antibiotics in food animals without differentiating the preventative use and therapeutic use [ 60 , 67 , 74 ].
The present study
Given that consumers have little knowledge about the preventive use of antibiotics in food animals (hereafter referred to as “the preventive use”), this study sought to improve consumers’ knowledge through providing information on the health risks associated with the preventive use, and investigating its effect on risk perceptions, support for a ban, and behavioral intention. The current research applied quasi-experimental methodology [ 75 , 76 ] to systematically present information on the health risks associated with the preventive use. This allowed us to explore if variations in information can affect consumers’ risk perceptions, support for a ban, and behavioral intention regarding the preventive use.
We anticipated that increased knowledge about the health risks of antibiotic residues and antibiotic resistant bacteria associated with the preventive use would influence risk perceptions, support for a ban, and behavioral intention towards the preventive use. We could not assume whether there would be significant differences in the effects of increased knowledge on antibiotic residues only versus knowledge on antibiotic resistant bacteria only, as there is no existing literature allowing us to make any assumptions.
Further, we hypothesized that, compared to providing knowledge on either antibiotic residues only or antibiotic resistant bacteria only, providing knowledge on both antibiotic residues and antibiotic resistant bacteria associated with the preventive use would either lead to a stronger effect on changes in risk perceptions, support for a ban, and behavioral intention, or result in no further increase. These competing hypotheses were informed by research based on fear appeal theory. Fear appeal is a persuasive communication strategy aiming at promoting attitude and behavioral changes by arousing fear via emphasizing the potential risk [ 77 , 78 ]. Research has suggested two theoretical perspectives of fear appear — the linear model and the plateau effect model. The linear model posits a positive linear relationship between depicted fear and persuasion, such that the more fear depicts, the more effective the information is in affecting risk perceptions, attitudes, and behavioral intentions [ 79 , 80 , 81 , 82 , 83 ]. From the perspective of the linear model, providing information about the health risks of both antibiotic residues and antibiotic resistant bacteria would have a stronger effect compared to providing information on only one of them. On the other hand, the plateau effect model suggests that the effect of depicted fear will reach a plateau at certain point, beyond which depicting additional fear has no additional influence on risk perceptions, attitudes, intentions, and behaviors [ 78 , 84 ]. From this perspective, providing information on both antibiotic residues and antibiotic resistant bacteria may not have additional influence, as it is likely that the effect of increased knowledge on either antibiotic residues only or antibiotic resistant bacteria only would reach its plateau.
Taken together, we hypothesized that providing information on the health risk of either antibiotic residues, or antibiotic resistant bacteria, or both caused by the preventive use would significantly increase consumers’ risk perceptions, support for a ban, and behavioral intention regarding the preventive use. We further hypothesized that providing information on the health risks of both antibiotic residues and antibiotic resistant bacteria would have an either stronger or similar effect on these variables in comparison with providing information on only one of them.
Research design
An experimental study with four conditions was employed via an online survey in China. Condition 1 was the control condition, where participants read background information (i.e., definitions of antibiotics, antibiotic resistance, and the preventive use of antibiotics in food animals). Condition 2 further provided information on the effect of the preventive use on human health via increasing antibiotic residues in food, in addition to the information provided in Condition 1. Condition 3 provided information on the effect of the preventive use on human health via increasing the risk of getting infected with antibiotic resistant bacteria, in addition to the information provided in Condition 1. Condition 4 provided all information contained in the first three conditions. The provided information was developed based on findings in previous studies [ 1 , 4 , 6 , 10 , 11 , 15 , 19 , 32 , 44 , 53 , 85 ]. Figure 1 outlines the information provided for each condition and the underlying rationale. The experimental material is presented in Table 1 .
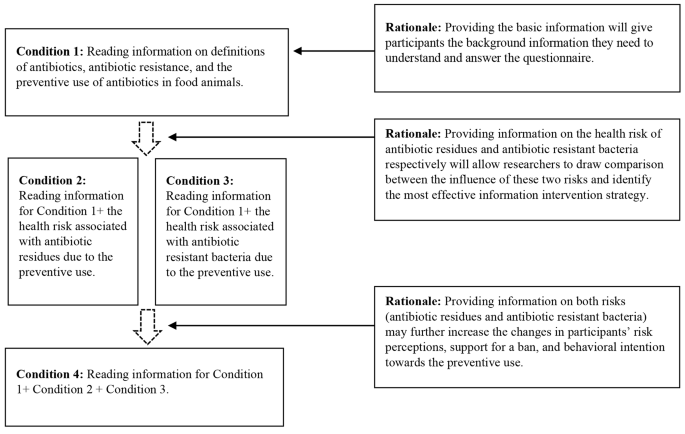
Experimental design diagram and rationale
Participants were randomly assigned to one of the four conditions and were then asked to rate on a number of questions regarding risk perceptions (i.e., concern about antibiotic residues and antibiotic resistant bacteria, fear towards use of antibiotics as a preventative in food animals), support for a ban, and behavioral intention towards the preventive use (i.e., intention to buy meat produced without the preventive use of antibiotics).
Procedure and participants
A professional online research platform (Credamo) was used to collect data. The survey link was sent to the participants panel of the research platform. Participants read the information and consent sheet first, which included a brief introduction to the study, information regarding participation and withdrawal (i.e., the participation is voluntary and participants can withdraw at any time), risks and benefits (i.e., no foreseeable risks), confidentiality (i.e., no personally identifiable information will be collected and all collected information will be treated confidentially), and contacts. Participants were asked to click ‘Next page’ button if they consent to take part in the survey. After answering the questions on demographics (i.e., gender, age group, and education), participants were randomly assigned to one of the four conditions, and then answered the questions on the dependent variables (i.e., risk perceptions, support for a ban, and behavioral intention regarding the preventive use). To ensure the participants would read the provided information carefully, timers were included. For participants in Conditions 1-4, the information was displayed on the page for 45, 60, 90, and 100 s respectively before being able to move on. The timers increased as the length of information increased, which was informed by the pretesting within the research team. A small fee was paid to participants who completed the survey.
A total of 2533 participants across China completed the survey. The majority of them were female (61.4%), at the age of 18 to 44 years (80.6%), and had completed at least a bachelor’s degree (80.6%). Table 2 presents participants’ demographic information for each condition.
A 5-point Likert scale (1 = Strongly disagree, 5 = Strongly agree; unless stated otherwise) was provided for all responses. Cronbach’s alpha was calculated for multi-item measurements to examine the reliability of these measurements. An α value of 0.70 and above was considered acceptable [ 86 , 87 ]. The average scores of items for multi-item measurements were used in data analysis.
Three aspects were measured to examine participants’ risk perceptions: concern about antibiotic residues, concern about antibiotic resistant bacteria, and fear towards use of antibiotics as a preventative in food animals. Concern about antibiotic residues was measured by asking participants to indicate their degree of agreement with four statements adapted from Michaelidou and Hassan [ 88 ] : “I think most meat contain antibiotic residues,” “I’m concerned about the amount of antibiotic residues in meat,” “Antibiotic residues are widespread in the environment,” “I’m concerned about the amount of antibiotic residues in the environment,” ( α = 0.76). Concern about antibiotic resistant bacteria was measured by asking participants to indicate their degree of agreement with four statements adapted from Michaelidou and Hassan [ 88 ] : “I think most meat contain antibiotic resistant bacteria,” “I’m concerned about the amount of antibiotic resistant bacteria in meat,” “Antibiotic resistant bacteria are widespread in the environment,” “I’m concerned about the amount of antibiotic resistant bacteria in the environment,” ( α = 0.77). Fear towards use of antibiotics as a preventative in food animals was measured by asking participants to express their feelings of fear (frightened, anxious, and worried) towards use of antibiotics as a preventative in food animals (1 = Not at all, 5 = Very much; α = 0.83) (Adapted from Milne et al. [ 89 ]).
Supporting a ban for the preventive use was measured with “Please indicate to what extent do you support a ban for the preventive use of antibiotics in food animals?” (1 = I don’t support at all, 5 = I totally support) (Adapted from Lusk et al. [ 90 ]).
Intention to buy meat produced without the preventive use of antibiotics was measured by asking participants to indicate their degree of agreement with two statements adapted from Bradford et al. [ 54 ]: “I intend to buy meat produced without the preventive use of antibiotics” and “I will look for meat produced without the preventive use of antibiotics” ( α = 0.75).
Data analysis
Data analysis was conducted by using SPSS version 22.0. One-way analysis of variance (ANOVA) was utilized to test the differences in demographics and the dependent variables between the four conditions. For variables where significant differences were found between the four conditions, Tukey (when equal variance assumption was satisfied) and Games-Howell (when equal variance assumption was not satisfied) post-hoc comparisons with bias-corrected and accelerated bootstrap estimation (1,000 samples) were carried out. A 95% confidence interval (CI) of the difference between means was used to determine whether the difference was significant. A 95% CI without zero indicates that the difference is statistically significant.
A series of one-way between-subjects ANOVA analyses were first conducted to examine the differences in demographics (i.e., gender, age, and education). The results suggested that there were no significant differences in these demographic variables among the four conditions: gender, F (3, 2529) = 0.63, p = 0.598; age, F (3, 2529) = 0.93, p = 0.428; education, F (3, 2529) = 0.95, p = 0.417. These results indicated that any differences in the dependent variables between the four conditions were very likely due to the differences in information provision.
Another series of one-way ANOVA were carried out to further examine the differences in dependent variables (i.e., risk perceptions, support for a ban, and behavioral intention regarding the preventive use) across the four conditions. The results revealed significant differences among the four conditions in concern about antibiotic residues, F (3, 2529) = 11.11, p < 0.001, η p 2 = 0.013, concern about antibiotic resistant bacteria, F (3, 2529) = 7.38, p < 0.001, η p 2 = 0.009, fear towards use of antibiotics as a preventative in food animals, F (3, 2529) = 21.48, p < 0.001, η p 2 = 0.025, supporting a ban for the preventive use, F (3, 2529) = 12.47, p < 0.001, η p 2 = 0.015, and intention to buy meat produced without the preventive use of antibiotics, F (3, 2529) = 7.10, p < 0.001, η p 2 = 0.008. Post-hoc comparisons indicated that all these variables were significantly lower in Condition 1 than in all other conditions (Fig. 2 ), all ps < 0.05, all 95% CIs of the differences between means did not include 0. However, there were no significant differences in these variables between Conditions 2-4 (all ps > 0.05, all 95% CIs of the differences between means included 0). The descriptive statistics of dependent variables, the correlations between dependent variables and with demographics, and the 95% CIs of the differences between means of dependent variables in the four conditions are presented in Appendices A , B , and C , respectively.
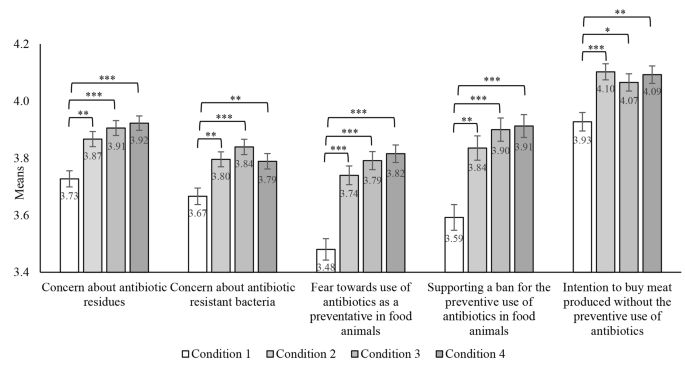
Means of the dependant variables with error bar. Note * p < 0.05, ** p < 0.01, *** p < 0.001. Concern about antibiotic residues, concern about antibiotic resistant bacteria, and intention to buy meat produced without the preventive use of antibiotics were measured on a 5-point scale (1 = Strongly disagree, 5 = Strongly agree). Fear towards use of antibiotics as a preventative in food animals was measured on a 5-point scale (1 = Not at all, 5 = Very much). Supporting a ban for the preventive use of antibiotics in food animals was measured on a 5-point scale (1 = I don’t support at all, 5 = I totally support)
The preventive use of antibiotics in food animals poses serious threats to human health globally. Previous research, however, suggested consumers have little knowledge about this practice. This study sought to examine the effect of information provision on the risk perceptions of, support for a ban, and behavioral intention towards the preventive use through an experimental study.
The results suggested that providing information on the health risks caused by the preventive use has significant influence on consumers’ risk perceptions, support for a ban, and behavioral intention regarding the preventive use. Compared to participants in control condition, where no health risk information was provided (Condition 1), participants who received information on the health risk of antibiotic residues (Condition 2), antibiotic resistant bacteria (Condition 3), and both antibiotic residues and antibiotic resistant bacteria (Condition 4) associated with the preventive use reported significantly higher level of risk perceptions of the preventive use (i.e., concern about antibiotic residues and antibiotic resistant bacteria, fear towards use of antibiotics as a preventative in food animals), stronger support for a ban on the preventive use, and a higher intention to buy meat produced without the preventive use. These findings demonstrated that increasing knowledge about the health risks of the preventive use was influential in increasing risk perceptions, support for a ban, and behavioral intention regarding the preventive use.
While there is no pre-existing literature allowing us to make assumptions about what differences it would make by providing participants with information on antibiotic residues or antibiotic resistant bacteria, this study revealed that information on the health risk of either antibiotic residues or antibiotic resistant bacteria led to similar levels of changes in risk perceptions, support for a ban, and behavioral intention in comparison to no health risk information being provided. That is, providing information on the health risk associated with either antibiotic residues or antibiotic resistant bacteria is equally effective in affecting these variables. Interestingly, when only information on the risk of antibiotic residues was provided, consumers’ concern about antibiotic resistant bacteria was also significantly increased, and vice versa. A possible explanation is that information on either of them could increase consumers’ overall risk perceptions of the preventive use. Therefore, providing information on the health risk of either antibiotic residues or antibiotic resistant bacteria enhanced the risk perceptions for both of them, despite that antibiotic residues and antibiotic resistant bacteria represented two different pathways in affecting human health. Besides, due to the abstract nature of antibiotic resistance, the understanding of this health threat was limited, resulting in misconceptions and low risk perceptions among the public [ 91 , 92 , 93 ]. Hence, it is likely that providing information about the transmission risks of either antibiotic residues or antibiotic resistant bacteria from animals to humans helped to make the issue of antibiotic resistance less abstract to the participants, thus increased the risk perception for both.
Furthermore, our findings suggested that providing information on both health risks in antibiotic residues and antibiotic resistant bacteria has a similar effect on consumers’ risk perceptions, support for a ban, and behavioral intention as providing information on only one of them. Although the pathways of how antibiotic residues and antibiotic resistant bacteria affect human health differ, the results demonstrated that providing information on both did not have an additive effect. This finding supported the hypothesis based on the plateau effect model rather than the linear model [ 78 , 84 ]. That is, providing information on the health risk of either antibiotic residues or antibiotic resistant bacteria is effective enough to reach the plateau effect. Consequently, there is no significant additional effect by depicting both risks. Thus, consumers’ exposure to either information led to the greatest changes in risk perceptions, support for a ban, and behavioral intention.
The findings of the current study have important implications for livestock industries and policymakers. First, the findings provide insights for developing effective risk communication strategies to increase public risk perceptions and promote attitude and behavioral intention changes regarding the preventive use. Future risk communication can convey simple messages about the health risk of either antibiotic residues or antibiotic resistant bacteria associated with the preventive use, as exposure to information on either of them is influential. Noticeably, though the results of the present study indicated a “plateau effect” of information provision, it needs to be cautious when applying the findings to campaigns in the real world. Future research needs to further validate the results and explore if the “plateau effect” holds true in other scenarios. For instance, the information we provided on the health risks of both antibiotic residues and antibiotic resistant bacteria might be too long for the participants to fully process in the survey setting. Future research can provide information on both via shorter message and examine if there is additional effect. In addition, the effect of using video instead of text to convey the information should be examined, as video might be more influential than text [ 73 ]. Moreover, to make the health risks of antibiotic residues and antibiotic resistant bacteria more realistic and relevant to the public, future research can include storytelling from people who have been affected by antibiotic residues or antibiotic resistant infections [ 94 ]. Further, future studies can also explore the moderating variables between the relationship of information provision and consumers’ risk perception, support for a ban, and behavioral intention. For example, animal-derived food products produced without the preventive use of antibiotics might be more expensive than conventional products. Therefore, participants’ income level might be a moderator. Health literacy is also a potential moderator. It is the capacity to access, understand, evaluate, and use information to maintain or improve health and quality of life [ 95 ]. Low health literacy is related to less healthy choices and riskier behaviors [ 95 ]. It’s possible that information provision is more effective among people who have higher level of health literacy as they might be able to understand the provided information better and make a healthier purchase decision. Second, this study has important implications for antibiotic stewardship in food animals. The findings indicated that once the public is aware of the health risks posed by the preventive use, they express a stronger demand to ban the practice, which challenges the industries’ social license to operate. Such public demand will help facilitate the implementation of a ban on this practice. Further, this research highlights a potential market for animal-derived food produced without the preventive use of antibiotics among informed consumers, which could incentivize livestock industries to use antibiotics responsibly. This consumer preference is of great value for promoting responsible antibiotic use in food animals from One Health perspective, especially considering that the increasing concern about antibiotic overuse in food animals might lead to consumer demand for eliminating antibiotics in livestock industries [ 55 , 56 , 69 ]. Given the severe health risks posed by the inappropriate use and overuse of antibiotics in food animals, the public needs to be made more aware of the issue. However, people shouldn’t be alarmist and overzealously seek to boycott necessary use of antibiotics, as it is harmful to both human health and animal welfare.
While the current research shed lights on how building consumer knowledge can enhance consumers’ risk perceptions, support for a ban, and behavioral intention regarding the preventive use, there are some limitations. For instance, there might be some vegetarians among the participants. We did not filter out and exclude them because the proportion of vegetarians in Chinese population is relatively small (about 4-5%) [ 96 ] and the number of them should be reasonably balanced across the four conditions via random assignment. Future research should consider excluding vegetarians from analysis.
To our best knowledge, this was the first study exploring the effect of information provision on risk perceptions, support for a ban, and behavioral intention regarding the preventive use of antibiotics in food animals. The findings demonstrated that providing information on the health risk of antibiotic residues, or antibiotic resistant bacteria, or both in relation to the preventive use is similarly effective in increasing consumers’ risk perceptions, support for a ban, and behavioral intention regarding this practice. These results suggested that increasing consumers’ knowledge about the health risk of either antibiotic residues or antibiotic resistant bacteria can lead to the greatest changes in these variables. The findings of the research can provide important insights to inform policymakers and livestock industries to develop effective communication strategies and public policies to promote responsible antibiotic use in food animals.
Descriptive statistics of dependent variables ( M±SD )
- Note C1, Condition 1, C2, Condition 2, C3, Condition 3, C4, Condition 4. Concern about antibiotic residues, concern about antibiotic resistant bacteria, and intention to buy meat produced without the preventive use of antibiotics were measured on a 5-point scale (1 = Strongly disagree, 5 = Strongly agree). Fear towards use of antibiotics as a preventative in food animals was measured on a 5-point scale (1 = Not at all, 5 = Very much). Supporting a ban for the preventive use of antibiotics in food animals was measured on a 5-point scale (1 = I don’t support at all, 5 = I totally support)
Pearson correlations between dependent variables and with demographics
- Note C1, Condition 1, C2, Condition 2, C3, Condition 3, C4, Condition 4. * p < 0.05, ** p < 0.01, *** p < 0.001. Gender, 1 = Male, 2 = Female. Age, 1 = 18-24 years, 2 = 25-34 years, 3 = 35-44 years, 4 = 45-54 years, 5 =55+ years. Education, 1 = Senior high school and below (year 12), 2 = College certificate, 3 = Bachelor’s degree, 4 = Postgraduate
Differences in means of dependent variables between the four conditions
- Note C1, Condition 1, C2, Condition 2, C3, Condition 3, C4, Condition 4. M diff , Differences in mean. 95% CI, 95% confidence interval. * p < 0.05, ** p < 0.01, *** p < 0.001
Data availability
The datasets used in the current study are available from the corresponding author on reasonable request.
WHO. Who fact sheets: Antibiotic resistance. 2020. https://www.who.int/news-room/fact-sheets/detail/antibiotic-resistance . Accessed 10 May 2023.
Antimicrobial Resistance Collaborators. Global burden of bacterial antimicrobial resistance in 2019: a systematic analysis. Lancet. 2022;399(10325):629–55.
Article Google Scholar
McEwen SA, Collignon PJ. Antimicrobial resistance: a One Health perspective. Antimicrob Resist bacteria Livest Companion Anim. 2018;1:521–47.
Sharma C, Rokana N, Chandra M, Singh BP, Gulhane RD, Gill JPS, et al. Antimicrobial resistance: its surveillance, impact, and alternative management strategies in dairy animals. Front Vet Sci. 2017;4:237.
Article PubMed Google Scholar
O’Neill J. Antimicrobials in agriculture and the environment: Reducing unnecessary use and waste. The review on antimicrobial resistance. 2015:1-41.
Kumar A, Patyal A, Panda AK. Sub-therapeutic use of antibiotics in animal feed and their potential impact on environmental and human health: a comprehensive review. J Anim Feed Sci Technol. 2018;6:15–25.
Google Scholar
Mouiche MMM, Moffo F, Akoachere JTK, Okah-Nnane NH, Mapiefou NP, Ndze VN, et al. Antimicrobial resistance from a one health perspective in Cameroon: a systematic review and meta-analysis. BMC Public Health. 2019;19(1):1135.
Article PubMed PubMed Central Google Scholar
Hernando-Amado S, Coque TM, Baquero F, Martinez JL. Defining and combating antibiotic resistance from one health and Global Health perspectives. Nat Microbiol. 2019;4(9):1432–42.
Article CAS PubMed Google Scholar
One Health Commission. What is One Health? https://www.onehealthcommission.org/en/why_one_health/what_is_one_health? Accessed 12 March 2024.
Hassan MM, El Zowalaty ME, Lundkvist A, Jarhult JD, Khan Nayem MR, Tanzin AZ, et al. Residual antimicrobial agents in food originating from animals. Trends Food Sci Technol. 2021;111:141–50.
Article CAS PubMed PubMed Central Google Scholar
Shao Y, Wang Y, Yuan Y, Xie Y. A systematic review on antibiotics misuse in livestock and aquaculture and regulation implications in China. Sci Total Environ. 2021;798:149205.
Waters WF, Baca M, Graham JP, Butzin-Dozier Z, Vinueza L. Antibiotic use by backyard food animal producers in Ecuador: a qualitative study. BMC Public Health. 2022;22(1):685.
You Y, Silbergeld EK. Learning from agriculture: understanding low-dose antimicrobials as drivers of resistome expansion. Front Microbiol. 2014;5:284.
PubMed PubMed Central Google Scholar
Van Boeckel TP, Pires J, Silvester R, Zhao C, Song J, Criscuolo NG, et al. Global trends in antimicrobial resistance in animals in low- and middle-income countries. Science. 2019;365:eaaw1944.
WHO. WHO guidelines on use of medically important antimicrobials in food producing animals. 2017. https://iris.who.int/bitstream/handle/10665/258970/9789241550130-eng.pdf?sequence=1 . Accessed 20 November 2023.
Bacanli M, Basaran N. Importance of antibiotic residues in animal food. Food Chem Toxicol. 2019;125:462–6.
Chee-Sanford JC, Mackie RI, Koike S, Krapac IG, Lin YF, Yannarell AC, et al. Fate and transport of antibiotic residues and antibiotic resistance genes following land application of manure waste. J Environ Qual. 2009;38(3):1086–108.
Van Boeckel TP, Brower C, Gilbert M, Grenfell BT, Levin SA, Robinson TP, et al. Global trends in antimicrobial use in food animals. Proc Natl Acad Sci. 2015;112(18):5649–54.
Patel SJ, Wellington M, Shah RM, Ferreira MJ. Antibiotic stewardship in food-producing animals: challenges, progress, and opportunities. Clin Ther. 2020;42(9):1649–58.
Quaik S, Embrandiri A, Ravindran B, Hossain K, Al-Dhabi NA, Arasu MV, et al. Veterinary antibiotics in animal manure and manure laden soil: scenario and challenges in Asian countries. J King Saud Univ - Sci. 2020;32(2):1300–5.
Centres for Disease Control and Prevention. Where resistance spreads: Food supply. 2020. https://www.cdc.gov/drugresistance/food.html . Accessed 17 November 2023.
European Commission. Ban on antibiotics as growth promoters in animal feed enters into effect. 2005. https://ec.europa.eu/commission/presscorner/detail/en/IP_05_1687 . Accessed 17 November 2023.
Ministry of Agriculture and Rural Affairs of People’s Republic of China. Official veterinary bulletin. 2019. http://www.moa.gov.cn/gk/sygb/201911/P020191108590803635472.pdf . Accessed 20 November 2023.
More SJ. European perspectives on efforts to reduce antimicrobial usage in food animal production. Ir Vet J. 2020;73:2.
Albernaz-Goncalves R, Olmos G, Hotzel MJ. Exploring farmers’ reasons for antibiotic use and misuse in pig farms in Brazil. Antibiotics. 2021;10:331.
Om C, McLaws ML, Antibiotics. Practice and opinions of Cambodian commercial farmers, animal feed retailers and veterinarians. Antimicrob Resist Infect Control. 2016;5:42.
Wongsuvan G, Wuthiekanun V, Hinjoy S, Day NP, Limmathurotsakul D. Antibiotic use in poultry: a survey of eight farms in Thailand. Bull World Health Organ. 2018;96(2):94–100.
Carrique-Mas JJ, Trung NV, Hoa NT, Mai HH, Thanh TH, Campbell JI, et al. Antimicrobial usage in chicken production in the Mekong Delta of Vietnam. Zoonoses Public Health. 2015;62(Suppl 1):70–8.
Xu J, Sangthong R, McNeil E, Tang R, Chongsuvivatwong V. Antibiotic use in chicken farms in Northwestern China. Antimicrob Resist Infect Control. 2020;9(1):10.
Pokharel S, Shrestha P, Adhikari B. Antimicrobial use in food animals and human health: time to implement ‘one health’ approach. Antimicrob Resist Infect Control. 2020;9:181.
Kyuchukova R. Antibiotic residues and human health hazard-review. Bulgarian J Agricultural Sci. 2020;26(3):664–8.
Arsene MMJ, Davares AKL, Viktorovna PI, Andreevna SL, Sarra S, Khelifi I, et al. The public health issue of antibiotic residues in food and feed: causes, consequences, and potential solutions. Vet World. 2022;15(3):662–71.
Ben Y, Fu C, Hu M, Liu L, Wong MH, Zheng C. Human health risk assessment of antibiotic resistance associated with antibiotic residues in the environment: a review. Environ Res. 2019;169:483–93.
Granowitz EV, Brown RB. Antibiotic adverse reactions and drug interactions. Crit Care Clin. 2008;24(2):421–42.
Beyene T. Veterinary drug residues in food-animal products: its risk factors and potential effects on public health. J Veterinary Sci Technol. 2016;7:1.
Treiber FM, Beranek-Knauer H. Antimicrobial residues in food from animal origin-a review of the literature focusing on products collected in stores and markets worldwide. Antibiotics. 2021;10:534.
Cabello FC. Heavy use of prophylactic antibiotics in aquaculture: a growing problem for human and animal health and for the environment. Environ Microbiol. 2006;8(7):1137–44.
Docic M, Bilkei G. Differences in antibiotic resistance in Escherichia coli, isolated from East-European swine herds with or without prophylactic use of antibiotics. J Vet Med B. 2003;50(1):27–30.
Article CAS Google Scholar
Done HY, Venkatesan AK, Halden RU. Does the recent growth of aquaculture create antibiotic resistance threats different from those associated with land animal production in agriculture? AAPS J. 2015;17(3):513–24.
Zalewska M, Blazejewska A, Czapko A, Popowska M. Antibiotics and antibiotic resistance genes in animal manure - consequences of its application in agriculture. Front Microbiol. 2021;12:610656.
De Vries SP, Vurayai M, Holmes M, Gupta S, Bateman M, Goldfarb D, et al. Phylogenetic analyses and antimicrobial resistance profiles of Campylobacter Spp. From diarrhoeal patients and chickens in Botswana. PLoS ONE. 2018;13(3):e0194481.
Ward M, Gibbons C, McAdam P, Van Bunnik B, Girvan E, Edwards G, et al. Time-scaled evolutionary analysis of the transmission and antibiotic resistance dynamics of Staphylococcus aureus clonal complex 398. Appl Environ Microbiol. 2014;80(23):7275–82.
Sweileh WM. Global research activity on antimicrobial resistance in food-producing animals. Arch Public Health. 2021;79(1):49.
Roth N, Kasbohrer A, Mayrhofer S, Zitz U, Hofacre C, Domig KJ. The application of antibiotics in broiler production and the resulting antibiotic resistance in Escherichia coli: a global overview. Poult Sci. 2019;98(4):1791–804.
Cycon M, Mrozik A, Piotrowska-Seget Z. Antibiotics in the soil environment-degradation and their impact on microbial activity and diversity. Front Microbiol. 2019;10:338.
Manyi-Loh C, Mamphweli S, Meyer E, Okoh A. Antibiotic use in agriculture and its consequential resistance in environmental sources: potential public health implications. Molecules. 2018;23(4).
Xiao K-Q, Li B, Ma L, Bao P, Zhou X, Zhang T, et al. Metagenomic profiles of antibiotic resistance genes in paddy soils from South China. FEMS Microbiol Ecol. 2016;92:fiw023.
Zhu YG, Johnson TA, Su JQ, Qiao M, Guo GX, Stedtfeld RD, et al. Diverse and abundant antibiotic resistance genes in Chinese swine farms. Proc Natl Acad Sci U S A. 2013;110(9):3435–40.
Wang C, Liu X, Yang Y, Wang Z. Antibiotic and antibiotic resistance genes in freshwater aquaculture ponds in China: a meta-analysis and assessment. J Clean Prod. 2021;329:129719.
Singh R, Singh AP, Kumar S, Giri BS, Kim K-H. Antibiotic resistance in major rivers in the world: a systematic review on occurrence, emergence, and management strategies. J Clean Prod. 2019;234:1484–505.
Wahome CN. Contamination levels of groundwater, antimicrobial resistance patterns, plasmid profiles and chlorination efficacy in Ongata Rongai. Kajiado North County, Kenya: Kenyatta University Nairobi City, Kenya; 2013.
Chen CQ, Zheng L, Zhou JL, Zhao H. Persistence and risk of antibiotic residues and antibiotic resistance genes in major mariculture sites in Southeast China. Sci Total Environ. 2017;580:1175–84.
Chen J, Ying GG, Deng WJ. Antibiotic residues in food: extraction, analysis, and human health concerns. J Agric Food Chem. 2019;67(27):7569–86.
Bradford H, McKernan C, Elliott C, Dean M. Consumer purchase intention towards a quick response (QR) code for antibiotic information: an exploratory study. Npj Sci Food. 2022;6:23.
Bradford H, McKernan C, Elliott C, Dean M. Consumers’ perceptions and willingness to purchase pork labelled ‘Raised without antibiotics’. Appetite. 2022;171:105900.
Busch G, Kassas B, Palma MA, Risius A. Perceptions of antibiotic use in livestock farming in Germany, Italy and the United States. Livest Sci. 2020;241:104251.
Denver S, Jensen JD, Christensen T. Consumer preferences for reduced antibiotic use in Danish pig production. Prev Vet Med. 2021;189:105310.
Ding Q, Gao J, Ding X, Huang D, Zhao Y, Yang M. Consumers’ knowledge, attitude, and behavior towards antimicrobial resistance and antimicrobial use in food production in China. Front Public Health. 2022;10:1015950.
Ishak MA, Ali HM, Kheder SI. Prevalence and knowledge of antibiotics misuse and resistance in poultry and livestock in Khartoum State–Sudan. Sudan Med Monit. 2018;12:45–50.
Meerza SIA, Brooks KR, Gustafson CR, Yiannaka A. Information avoidance behavior: does ignorance keep us uninformed about antimicrobial resistance? Food Policy. 2021;102:102067.
Meerza SIA, Gulab S, Brooks KR, Gustafson CR. Us consumer attitudes toward antibiotic use in livestock production. Sustainability. 2022;14:7035.
Regan Á, Sweeney S, McKernan C, Benson T, Dean M. Consumer perception and understanding of the risks of antibiotic use and antimicrobial resistance in farming. Agric Hum Values. 2023(40):989–1001.
Shan H, Maqbool S, Hassan U, Noor A. Public awareness and understanding about spread of antimicrobial resistance linked with food chain. Isra Med J. 2021;13(3):6.
Smith RA, Zhu X, Shartle K, Glick L. Understanding the public’s intentions to purchase and to persuade others to purchase antibiotic-free meat. Health Commun. 2017;32(8):9.
Wemette M, Greiner Safi A, Wolverton AK, Beauvais W, Shapiro M, Moroni P, et al. Public perceptions of antibiotic use on dairy farms in the United States. J Dairy Sci. 2021;104(3):2807–21.
Paudel B, Kolady D, Grebitus C, Roy A, Ishaq M. Consumers’ willingness to pay for pork produced with different levels of antibiotics. Q Open. 2022;2:1–17.
Schell RC, Bulut E, Padda H, Safi AG, Moroni P. Responsible antibiotic use labeling and consumers’ willingness to buy and pay for fluid milk. J Dairy Sci. 2022;106:1.
Malek L, Umberger WJ, Goddard E. Committed vs. uncommitted meat eaters: understanding willingness to change protein consumption. Appetite. 2019;138:115–26.
Steede GM, Meyers C, Li N, Irlbeck E, Gearhart S. The influence of framing effects on public opinion of antibiotic use in livestock. J Appl Commun. 2020;104(2):3.
Tang KL, Caffrey NP, Nobrega DB, Cork SC, Ronksley PE, Barkema HW, et al. Restricting the use of antibiotics in food-producing animals and its associations with antibiotic resistance in food-producing animals and human beings: a systematic review and meta-analysis. Lancet Planet Health. 2017;1(8):e316–27.
Dickson-Spillmann M, Siegrist M, Keller C. Attitudes toward chemicals are associated with preference for natural food. Food Qual Prefer. 2011;22(1):149–56.
Ha TM, Shakur S, Pham Do KH. Consumer concern about food safety in Hanoi, Vietnam. Food Control. 2019;98:238–44.
Redding LE, Parsons B, Bender JS. Educational interventions to address misconceptions about antibiotic residues in milk can alter consumer perceptions and may affect purchasing habits. J Dairy Sci. 2021;104(11):11474–85.
Freivogel C, Visschers VHM. Antimicrobial-resistant bacteria in food: which behaviour change strategies increase consumers’ risk awareness and preventive food-handling behaviour? Health Psychol Behav Med. 2021;9(1):350–79.
Maciejewski ML. Quasi-experimental design. Biostatistics Epidemiol. 2020;4(1):38–47.
Zhang A, Measham TG, Moffat K. Preconditions for social licence: the importance of information in initial engagement. J Clean Prod. 2018;172:1559–66.
Dillard JP, Plotnick CA, Godbold LC, Freimuth VS, Edgar T. The multiple affective outcomes of AIDS PSAs: fear appeals do more than scare people. Communication Res. 1996;23(1):44–72.
Tannenbaum MB, Hepler J, Zimmerman RS, Saul L, Jacobs S, Wilson K, et al. Appealing to fear: a meta-analysis of fear appeal effectiveness and theories. Psychol Bull. 2015;141(6):1178–204.
Bigsby E, Albarracín D. Self- and response efficacy information in fear appeals: a meta-analysis. J Communication. 2022;72(2):241–63.
Dillard JP, Li SS. How scary are threat appeals? Evaluating the iintensity of fear in experimental research. Hum Commun Res. 2020;46(1):1–24.
Ruiter RAC, Abraham C, Kok G. Scary warnings and rational precautions: a review of the psychology of fear appeals. Psychol Health. 2001;16(6):613–30.
Shen L. Putting the fear back again (and within individuals): revisiting the role of fear in persuasion. Health Commun. 2017;32(11):1331–41.
Witte K, Allen M. A meta-analysis of fear appeals: implications for effective public health campaigns. Health Educ Behav. 2000;27(5):591–615.
Skurka C, Myrick JG, Yang Y. Fanning the flames or burning out? Testing competing hypotheses about repeated exposure to threatening climate change messages. Clim Change. 2023;176:52.
Van Boeckel TP, Glennon EE, Chen D, Gilbert M, Robinson TP, Grenfell BT, et al. Reducing antimicrobial use in food animals. Science. 2017;357(6358):1350–2.
Taber KS. The use of Cronbach’s alpha when developing and reporting research instruments in science education. Res Sci Educ. 2017;48(6):1273–96.
Tavakol M, Dennick R. Making sense of Cronbach’s alpha. Int J Med Educ. 2011;2:53–5.
Michaelidou N, Hassan LM. The role of health consciousness, food safety concern and ethical identity on attitudes and intentions towards organic food. Int J Consumer Stud. 2008;32(2):163–70.
Milne S, Orbell S, Sheeran P. Combining motivational and volitional interventions to promote exercise participation: Protection motivation theory and implementation intentions. Br J Health Psychol. 2002;7(Pt 2):163–84.
Lusk JL, Norwood FB, Pruitt JR. Consumer demand for a ban on antibiotic drug use in pork production. Am J Agric Econ. 2006;88(4):1015–33.
Carter RR, Sun J, Jump RL. A survey and analysis of the American public’s perceptions and knowledge about antibiotic resistance. Open Forum Infect Dis. 2016;3(3):ofw112.
David JC, Piednoir E, Delouvee S. Knowledge and perceptions of antibiotic resistance in the French population. Infect Dis Now. 2022;52(5):306–10.
Hawkings NJ, Wood F, Butler CC. Public attitudes towards bacterial resistance: a qualitative study. J Antimicrob Chemother. 2007;59(6):1155–60.
McCall B, Shallcross L, Wilson M, Hayward A. Making microbes matter: storytelling’s potential to make antibiotic resistance real and relevant to the public. Npj Antimicrobials Resist. 2023;1:10.
Kickbusch I, Pelikan JM, Apfel F, Tsouros AD. Health literacy: The solid facts (WHO Regional Office for Europe). World Health Organization, Europe. 2013. https://iris.who.int/bitstream/handle/10665/326432/9789289000154-eng.pdf?sequence=1 Accessed 13 March 2024.
The Times of India. China’s vegetarian population touches 50 million: Report. 1 February 2014. https://timesofindia.indiatimes.com/world/china/chinas-vegetarian-population-touches-50-million-report/articleshow/29725767.cms Accessed 13 March 2024.
Download references
Acknowledgements
The authors would like to thank Chris Angwin for proofreading this article.
This work was supported by Key Projects of Philosophy and Social Sciences Research, Ministry of Education of the People’s Republic of China (Award number: 21JZD038), the Trusted Agrifood Exports Mission program of Commonwealth Scientific and Industrial Research Organisation (CSIRO), and China Scholarship Council (CSC Award Number: 202004920045).
Author information
Authors and affiliations.
Health and Biosecurity, Commonwealth Scientific and Industrial Research Organisation (CSIRO), Brisbane, QLD, 4102, Australia
Yingnan Zhou, Airong Zhang & Rieks Dekker van Klinken
School of Sociology and Ethnology, University of Chinese Academy of Social Sciences, Beijing, 102488, China
Yingnan Zhou
School of Mental Health, Wenzhou Medical University, Wenzhou, Zhejiang, 325035, China
Junxiu Wang
The Affiliated Kangning Hospital of Wenzhou Medical University, Wenzhou, Zhejiang, 325007, China
You can also search for this author in PubMed Google Scholar
Contributions
YZ, AZ, RDvK, and JW conceived and designed the study. YZ conducted data collection and data analysis. YZ and AZ wrote the first draft of the manuscript. RDvK and JW provided critical feedback for revisions. All authors reviewed and approved the final version of the manuscript.
Corresponding author
Correspondence to Junxiu Wang .
Ethics declarations
Ethics approval and consent to participate.
This study has been approved by CSIRO’s Social Science Human Research Ethics Committee, within the guidelines of the Australian National Statement on Ethical Conduct in Human Research (2007, updated 2018). Informed consent was obtained from all participants in the study.
Consent for publication
Not applicable.
Competing interests
The authors declare that they have no competing interests.
Additional information
Publisher’s note.
Springer Nature remains neutral with regard to jurisdictional claims in published maps and institutional affiliations.
Rights and permissions
Open Access This article is licensed under a Creative Commons Attribution 4.0 International License, which permits use, sharing, adaptation, distribution and reproduction in any medium or format, as long as you give appropriate credit to the original author(s) and the source, provide a link to the Creative Commons licence, and indicate if changes were made. The images or other third party material in this article are included in the article’s Creative Commons licence, unless indicated otherwise in a credit line to the material. If material is not included in the article’s Creative Commons licence and your intended use is not permitted by statutory regulation or exceeds the permitted use, you will need to obtain permission directly from the copyright holder. To view a copy of this licence, visit http://creativecommons.org/licenses/by/4.0/ . The Creative Commons Public Domain Dedication waiver ( http://creativecommons.org/publicdomain/zero/1.0/ ) applies to the data made available in this article, unless otherwise stated in a credit line to the data.
Reprints and permissions
About this article
Cite this article.
Zhou, Y., Zhang, A., van Klinken, R.D. et al. The effect of information provision on consumers’ risk perceptions of, support for a ban, and behavioral intention towards the preventive use of antibiotics in food animals. BMC Public Health 24 , 1428 (2024). https://doi.org/10.1186/s12889-024-18859-2
Download citation
Received : 19 January 2024
Accepted : 15 May 2024
Published : 28 May 2024
DOI : https://doi.org/10.1186/s12889-024-18859-2
Share this article
Anyone you share the following link with will be able to read this content:
Sorry, a shareable link is not currently available for this article.
Provided by the Springer Nature SharedIt content-sharing initiative
- Information provision
- Risk perception
- Support for a ban
- Behavioral intention
- The preventive use of antibiotics
- Food animals
BMC Public Health
ISSN: 1471-2458
- General enquiries: [email protected]
We've detected unusual activity from your computer network
To continue, please click the box below to let us know you're not a robot.
Why did this happen?
Please make sure your browser supports JavaScript and cookies and that you are not blocking them from loading. For more information you can review our Terms of Service and Cookie Policy .
For inquiries related to this message please contact our support team and provide the reference ID below.
- Environment
A Republican pollster asked Florida voters about energy. The results surprised him
- Emily L. Mahoney Times staff
Floridians of both political parties are overwhelmingly concerned about the cost of energy in the state and Florida’s heavy reliance on natural gas as a source of electricity, according to a poll by a Republican strategy firm. It also found that environmental issues will factor into voters’ decisions in the 2024 election.
The poll was commissioned by the Environmental Defense Fund, which advocates for environmental causes including renewable energy. To conduct the poll, the group hired Spry Strategies, a Tennessee firm whose clients have included Gov. Ron DeSantis, U.S. Rep. Anna Paulina Luna and the Republican Party of Florida, according to the company’s website.
The April poll of 463 likely general election voters in Florida found that 84% of respondents, including 83% of Republicans, are concerned about Florida’s cost of energy. Twice as many people said their electric bill is higher than last year compared to the portion who said it wasn’t, across all political parties.
When pollsters informed participants that two-thirds of Florida’s electricity comes from burning natural gas that’s largely piped in from out-of-state, 76% of those surveyed, including 67% of Republicans, said that was concerning. A vast majority said they would support further diversification of energy sources.
Ryan Burrell, president of Spry Strategies, said he was “pretty taken aback” by the findings.
“As a red state, Florida’s Republican base is more environmentally conscious than arguably any other red state in the country,” he wrote in a summary report, attributing that to the state’s abundant natural resources and reliance on tourism. “The survey results surprised me as a Republican pollster and conservative because of Floridians’ broad consciousness on issues outside of the scope of marine, water, and wildlife protection.”
One of the more unexpected answers came from a question about hurricane severity, Burrell said. The question was: “Are you concerned about the cost of increasing storm severity due to climate change, including higher property taxes and flood insurance rates?”
A majority of Republicans said “yes.” Typically when polling in red states, less than half of Republicans believe increased storm severity is caused by climate change, Burrell said.
The latest research suggests hurricanes are growing stronger in a warming world, and rapid intensification — when top wind speeds increase by 35 mph in a single day — is projected to happen more frequently with climate change. But nationwide, a minority of Republicans — 36% — say climate change is affecting their own community, according to a survey by Pew conducted last year.
“One thing it makes you want to do as a pollster is poll deeper into the issue,” Burrell said in an interview. “There’s no question this is a big enough surprise that it’s worth a deeper understanding.”
Keep up with Tampa Bay’s top headlines
Subscribe to our free DayStarter newsletter
You’re all signed up!
Want more of our free, weekly newsletters in your inbox? Let’s get started.
Dawn Shirreffs, the Florida Director of the Environmental Defense Fund, said her group hired a Republican firm because of the GOP’s supermajorities in the state Legislature.
“We really wanted to make sure we were providing information that would be viewed as credible to policymakers,” she said. Her group plans to disseminate the results of the survey to both lawmakers and state utility regulators, an effort that she said is particularly important on the heels of a new law, House Bill 1645.
Recently signed by Gov. Ron DeSantis, the law, which deleted the majority of mentions of climate change in state law and outlawed offshore wind turbines in state waters , also relaxed some regulations for natural gas pipelines and storage facilities. Florida’s reliance on this fuel source led to skyrocketing electric bills in recent years, as residents acutely felt the impact of historic spikes in the price of natural gas.
Shirreffs said she’s noticed an uptick in “misinformation” from the fossil fuel industry, including op-eds about that law, that Shirreffs said are aimed “to convince folks that fossil fuels should have continued dominance in Florida.”
National polling has found that while most Americans support expanding solar and wind energy, Republican support has dropped in recent years as partisan gaps are starting to form .
“I think there’s been a broad effort to politicize climate and the need for energy policy change,” Shirreffs said. Meanwhile, she’s heard from Floridians who took less than their prescribed amount of medications to save money when their electric bills were high. “We’re helping people understand this is not a theoretical issue. This is affecting their quality of life.”
Dale Calhoun, executive director of the Florida Natural Gas Association, said in an email that it’s other states whose energy policy have become politicized, causing “high prices and rolling blackouts.”
“Here in Florida, our diverse energy portfolio enables families and business to access safe, reliable and affordable power, and natural gas is an important part of that,” he said. “Especially as we head into hurricane season, Floridians turn to natural gas a reliable fuel source to support emergency response efforts, power generators, keep hospitals open and maintain A/C at nursing homes.”
As for the poll results, Shirreffs believes that Florida Republicans stand out because they’ve felt the effects of climate change firsthand, like hotter summers and more severe hurricanes.
“I think there’s a moment where people’s lived experience becomes more important than partisan talking points,” she said. “That moment seems to have arrived.”
Emily L. Mahoney is the energy reporter. Reach her at [email protected].
MORE FOR YOU
- Advertisement
ONLY AVAILABLE FOR SUBSCRIBERS
The Tampa Bay Times e-Newspaper is a digital replica of the printed paper seven days a week that is available to read on desktop, mobile, and our app for subscribers only. To enjoy the e-Newspaper every day, please subscribe.

IMAGES
VIDEO
COMMENTS
Developing a hypothesis (with example) Step 1. Ask a question. Writing a hypothesis begins with a research question that you want to answer. The question should be focused, specific, and researchable within the constraints of your project. Example: Research question.
The results of a test may either support or contradict—oppose—a hypothesis. Results that support a hypothesis can't conclusively prove that it's correct, but they do mean it's likely to be correct. On the other hand, if results contradict a hypothesis, that hypothesis is probably not correct. Unless there was a flaw in the test—a ...
H0: μafter = μbefore (the mean number of defective widgets is the same before and after using the new method) HA: μafter ≠ μbefore (the mean number of defective widgets produced is different before and after using the new method) Suppose the p-value of the test turns out to be 0.27. Here is how he would report the results of the ...
Hypothesis testing is a crucial procedure to perform when you want to make inferences about a population using a random sample. These inferences include estimating population properties such as the mean, differences between means, proportions, and the relationships between variables. This post provides an overview of statistical hypothesis testing.
The results of hypothesis testing will be presented in the results and discussion sections of your research paper, dissertation or thesis. ... and state whether the result of our test did or did not support the alternate hypothesis. If your null hypothesis was rejected, this result is interpreted as "supported the alternate hypothesis." ...
It is often used as a starting point for testing the research hypothesis, and if the results of the study reject the null hypothesis, it suggests that there is a significant difference or relationship between variables. ... This means that it must be possible to collect data that will either support or refute the hypothesis. Falsifiable: A ...
Depending on the results of scientific evaluation, a hypothesis typically is either rejected as false or accepted as true. However, because a hypothesis inherently is falsifiable, even hypotheses supported by scientific evidence and accepted as true are susceptible to rejection later, when new evidence has become available. In some instances ...
Step 5: Phrase your hypothesis in three ways. To identify the variables, you can write a simple prediction in if … then form. The first part of the sentence states the independent variable and the second part states the dependent variable. If a first-year student starts attending more lectures, then their exam scores will improve.
By developing a hypothesis for a research project, you are effectively setting up the framework for further exploration. When developing a hypothesis, you must consider both the expected outcomes and possible alternative explanations. This will help you focus on testing the possible results without getting sidetracked by irrelevant information.
S.3 Hypothesis Testing. In reviewing hypothesis tests, we start first with the general idea. Then, we keep returning to the basic procedures of hypothesis testing, each time adding a little more detail. The general idea of hypothesis testing involves: Making an initial assumption. Collecting evidence (data).
A hypothesis is a tentative statement about the relationship between two or more variables. Explore examples and learn how to format your research hypothesis. ... In many cases, researchers may find that the results of an experiment do not support the original hypothesis. When writing up these results, the researchers might suggest other ...
The above image shows a table with some of the most common test statistics and their corresponding tests or models.. A statistical hypothesis test is a method of statistical inference used to decide whether the data sufficiently support a particular hypothesis. A statistical hypothesis test typically involves a calculation of a test statistic.Then a decision is made, either by comparing the ...
Using the test statistic or the p-value, determine if you can reject or fail to reject the null hypothesis based on the significance level. The p-value tells us the strength of evidence in support of a null hypothesis. If the p-value is less than the significance level, we reject the null hypothesis. 5. Interpret the results.
Every hypothesis has some assumptions that be met to make sure that the results of the hypothesis are valid. The assumptions are different for each test. This test has the following assumptions. ... We also acknowledge previous National Science Foundation support under grant numbers 1246120, 1525057, and 1413739. Legal.
A research hypothesis, in its plural form "hypotheses," is a specific, testable prediction about the anticipated results of a study, established at its outset. It is a key component of the scientific method. Hypotheses connect theory to data and guide the research process towards expanding scientific understanding.
The results of the hypothesis must be reproducible. Without these criteria, the hypothesis and the results will be vague. As a result, the experiment will not prove or disprove anything significant. ... • Null hypothesis: Null hypothesis is a negative statement to support the researcher's findings that there is no relationship between two ...
Below these are summarized into six such steps to conducting a test of a hypothesis. Set up the hypotheses and check conditions: Each hypothesis test includes two hypotheses about the population. One is the null hypothesis, notated as H 0, which is a statement of a particular parameter value. This hypothesis is assumed to be true until there is ...
When reporting your results, you indicate which (if any) of these significance levels allow you to reject the null hypothesis. This is summarised in Table 11.1. This allows us to soften the decision rule a little bit, since p<.01 implies that the data meet a stronger evidentiary standard than p<.05 would. Nevertheless, since these levels are ...
Bibliography. A scientific hypothesis is a tentative, testable explanation for a phenomenon in the natural world. It's the initial building block in the scientific method. Many describe it as an ...
A two-sample t test was used to test the hypothesis that higher social distance from environmental problems would reduce the intent to donate to environmental organizations, with donation intention (recorded as a score from 1 to 10) as the outcome variable and social distance (categorized as either a low or high level of social distance) as the predictor variable.Social distance was found to ...
Hypothesis testing is an act in statistics whereby an analyst tests an assumption regarding a population parameter. The methodology employed by the analyst depends on the nature of the data used ...
Here are some good research hypothesis examples: "The use of a specific type of therapy will lead to a reduction in symptoms of depression in individuals with a history of major depressive disorder.". "Providing educational interventions on healthy eating habits will result in weight loss in overweight individuals.".
5. Collect Data. 6. Analyze Results. Be the first to add your personal experience. 7. Here's what else to consider. Be the first to add your personal experience. Developing a statistical ...
A new national poll indicates that if former President Trump is found guilty in his criminal trial, the verdict will have little impact his 2024 election rematch with President Biden.
Explaining the 'homevoter hypothesis' The study's finding is an extension of the "homevoter hypothesis," which holds that homeowners tend to vote in support of policies and candidates they ...
In support of this hypothesis, α5 integrin's interaction with Dynactin 3, 4 and 5 (Dctn3-5) was significantly elevated in siNRP1/2 and sip120RasGAP ECs compared to the Ctrl sample (Fig. 6o).
The flexibility of the support produced high variability in the experimental results, making it difficult to objectively calibrate the model. Therefore, for this work, values that could be considered typical of a quasi-brittle material were adopted: E = 17 G Pa , G f = 70 N / m , and σ t = 10 M Pa .
Participants were randomly assigned to one of the four conditions and were then asked to rate on a number of questions regarding risk perceptions (i.e., concern about antibiotic residues and antibiotic resistant bacteria, fear towards use of antibiotics as a preventative in food animals), support for a ban, and behavioral intention towards the preventive use (i.e., intention to buy meat ...
May 30, 2024 at 11:33 AM PDT. Listen. 5:06. These are the latest verified results from South Africa's national election, released by the Electoral Commission of South Africa. The tables show ...
The April poll of 463 likely general election voters in Florida found that 84% of respondents, including 83% of Republicans, are concerned about Florida's cost of energy. Twice as many people ...