MIT Libraries home DSpace@MIT
- DSpace@MIT Home
- MIT Libraries
- Graduate Theses

A continuous silent speech recognition system for AlterEgo, a silent speech interface
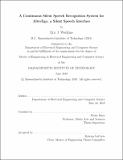
Other Contributors
Terms of use, description, date issued, collections.
A Computational Model for the Automatic Recognition of Affect in Speech
Feb. 1, 2004
- Raul Fernandez Former Research Assistant
- Rosalind W. Picard Professor of Media Arts and Sciences
Share this publication
Fernandez, R. "A Computational Model for the Automatic Recognition of Affect in Speech"
Spoken language, in addition to serving as a primary vehicle for externalizing linguistic structures and meaning, acts as a carrier of carious sources of information, including background, age, gender, membership in social structures, as well as physiological, pathological and emotional states. These sources of information are more than just ancillary to the main purpose of linguistic communication: Humans react to the various non-linguistic factors encoded in the speech signal, shaping and adjusting their interactions to satisfy interpersonal and social protocols.
Computer science, artificial intelligence and computational linguistics have devoted much active research to systems that aim to model the production and recovery of linguistic lexico-semantic structures from speech. However, less attention has been devoted to systems that model and understand the paralinguistic and extralinguistic information in the signal. As the breadth and nature of human-computer interaction escalates to levels previously reserved for human-to-human communication, there is a growing need to endow computational systems with human-like abilities which facilitate the interaction and make it more natural. OF paramount importance amongst these is the human ability to make inferences regarding the affective content of our exchanges.
This thesis proposes a framework for the recognition of affective qualifiers from prosodic-acoustic parameters extracted form spoken language. It is argued that modeling the affective prosodic variation of speech can be approached by integrating acoustic parameters from various prosodic time scales, summarizing information from more localized (e.g., syllable level) to more global prosodic phenomena (e.g., utterance level). In this framework speech is structurally modeled as a dynamically evolving hierarchical model in which levels of the hierarchy are determined by prosodic constituency and contain parameters that evolve according to dynamical systems. The acoustic parameters have been chosen to reflect four main components of speech thought to reflect paralinguistic and affective-specific information: intonation, loudness, rhythm and voice quality. The thesis addresses the contribution of each of these components separately, and evaluates the full model by testing it on datasets of acted and of spontaneous speech perceptually annotated with effective labels, and by comparing it against human performance benchmarks.
Signal Processing for Recognition of Human Frustration
Raul Fernandez, Rosalind W. Picard
Expression Glasses: A Wearable Device for Facial Expression Recognition
J. Scheirer, Raul Fernandez, Rosalind W. Picard
Modeling Drivers' Speech Under Stress
Frustrating the user on purpose: a step toward building an affective computer.
J. Scheirer, Raul Fernandez, J. Klein, Rosalind W. Picard

Speech Communication Group

Speech Communication Group at MIT
Speech Research
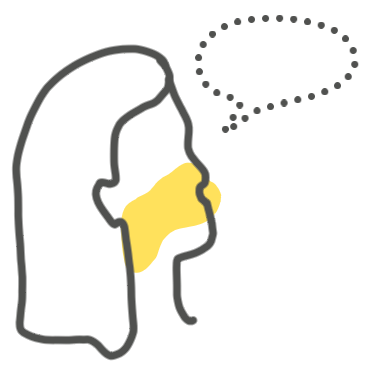
Gesture Research
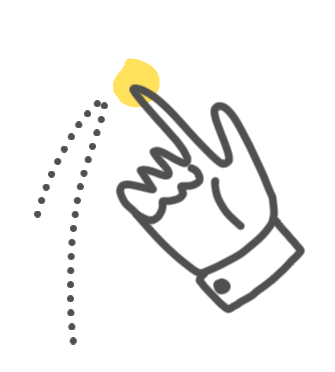
We are a research group of speech and gesture scientists under MIT's Research Laboratory of Electronics ( rle.mit.edu ).
In Memory of Ken Stevens
On the day Kenneth Noble Stevens was first appointed at MIT as an Assistant Professor in 1954, no one could have predicted the number of scientific careers he would launch, the way he would transform the thinking of his students and colleagues, and the breadth of the influence he would have on acoustic phonetics and beyond. He was a member of the MIT faculty for more than half a century and supervised at least 50 Ph.D. dissertations, as well as an untold number of Masters students, undergraduates, postdoctoral fellows, and visiting scientists. His first Ph.D. student was James Flanagan (1955), and his last one was Youngsook Jung (2009).
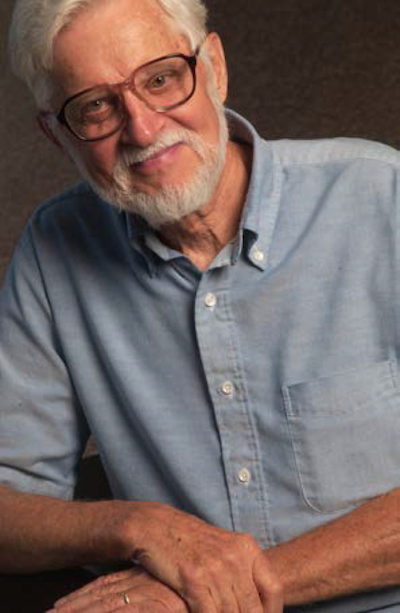
Being in Ken’s Speech Communication Laboratory was an extraordinary experience. The laboratory was full of energy and brought together researchers from many disciplines related to speech (linguistics, psychology, acoustics, computer science, physiology). This was at a time when multidisciplinary research was not the norm in either academia or industry. Ken was also unique, for his time, in that he supervised a substantial number of female graduate students. In fact, of the first 20 women who received PhD’s in Electrical Engineering and Computer Science at MIT, four were Ken’s students, and of the doctoral students he supervised, 23 were women.
Ken was a gentle and rigorous mentor, and met regularly with his students and postdocs. As Haruko Kawasaki, a former postdoc with Ken, says, “What struck me most about Ken at these meetings was his being an exceptionally good listener.” He had a low-key manner, yet he would let his students know when something was not right, sometimes by simply raising one eyebrow! Patti Price, another former postdoc of Ken, recalls him telling her, “Well, when you measure productivity and a student is involved, you have to count both products: the progress of the research and the progress of the student.” His legendary support of students is reflected in his reluctance to put his name on their papers, even though he would spend uncounted hours discussing the work and editing successive drafts.
The depth of his understanding of science formed an indelible imprint on his students and colleagues that became a model for those who pursued scientific careers, so that Ken's influence reached far beyond any particular time and place. He was creative yet meticulous in his attention to understanding and explaining every detail of a model or of a physical mechanism. He also used words with great precision. It is no surprise, then, that his 1998 *Acoustic Phonetics* book, which became a classic overnight, took more than 20 years to complete. Among his seminal intellectual contributions is the quantal theory of speech production relating the underlying sound categories of language to the acoustics, physiology, and physics of the vocal tract. Another is his 2002 model of speech perception based on feature cues such as landmarks. His long-term collaborations with colleagues such as Gunnar Fant, Morris Halle, Sheila Blumstein, Dennis Klatt, and Jay Keyser led to important papers and lively debates on issues such as Analysis-by-Synthesis, Invariance, Distinctive Features, Lexical Access, and Feature Enhancement. He also treasured his friendship and collaboration with Amar Bose, which led to a co-authored book on introductory network theory in 1965. In addition to developing theories of speech production and perception, Ken also helped develop several systems, including the Klatttalk (with Dennis Klatt) and HLSYN speech synthesizers, articulatory synthesis, automatic speech recognition (especially using acoustic landmarks), and speech training methods for deaf children.
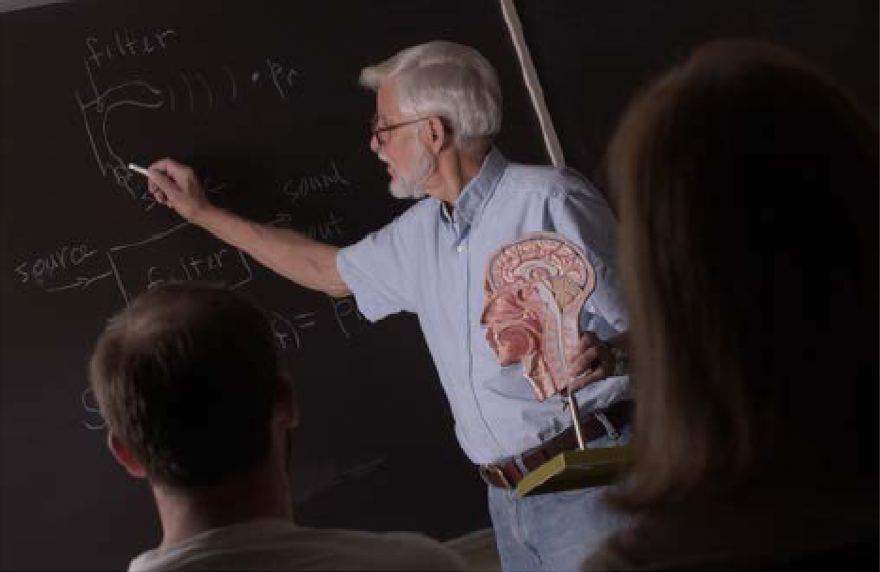
Ken supported the Acoustical Society of America (ASA) and believed it was the appropriate venue for promoting, discussing, and publishing all things related to speech acoustics. He served as a member of the ASA executive council, and as the Vice President and then President of the organization. In recognition of his scientific contributions and influence, the ASA awarded Ken its Silver Medal in 1983 and its Gold Medal in 1995. In 1999, President Clinton awarded Ken the National Medal of Science “For his leadership and pioneering contributions to the theory of acoustics of speech production and perception, development of mathematical methods of analysis and modeling to study the acoustics of speech production, and establishing the contemporary foundations of speech science."
Ken was devoted to his family and six children. He was an avid fan of the outdoors and took great pleasure in biking, hiking, skiing, and working on his house in Maine with family and friends. He loved classical music, ice cream, and fish chowder, and was a well-tested cook of lasagna and chili.
Kenneth N. Stevens was born on March 24, 1924, in Toronto, Canada. He received his B.A.Sc. and M.A.Sc. in engineering physics from the University of Toronto in 1945 and 1948 respectively, and his Sc.D. in electrical engineering from MIT in 1952. His dissertation was entitled “Perception of Sounds Shaped by Resonance Circuits”. His advisor was Leo Beranek; other thesis committee advisors included J.C. Licklider and Walter Rosenblith. Ken died in Clackamas, Oregon on August 19th, 2013 of complications due to Alzheimer’s. For those of us who knew him, he will always be present in our thoughts, our work, our lives, and our teaching to our own students.
- Abeer Alwan, UCLA, Los Angeles, CA
- Stefanie Shattuck-Hufnagel, RLE, MIT, Cambridge, MA
- Maria-Gabriella DiBenedetto, Sapienza University of Rome, Italy
Name Institution
Thesis Defense: On Internal Language Representations in Deep Learning: An Analysis of Machine Translation and Speech Recognition
April 26 2018, organizer & contact.
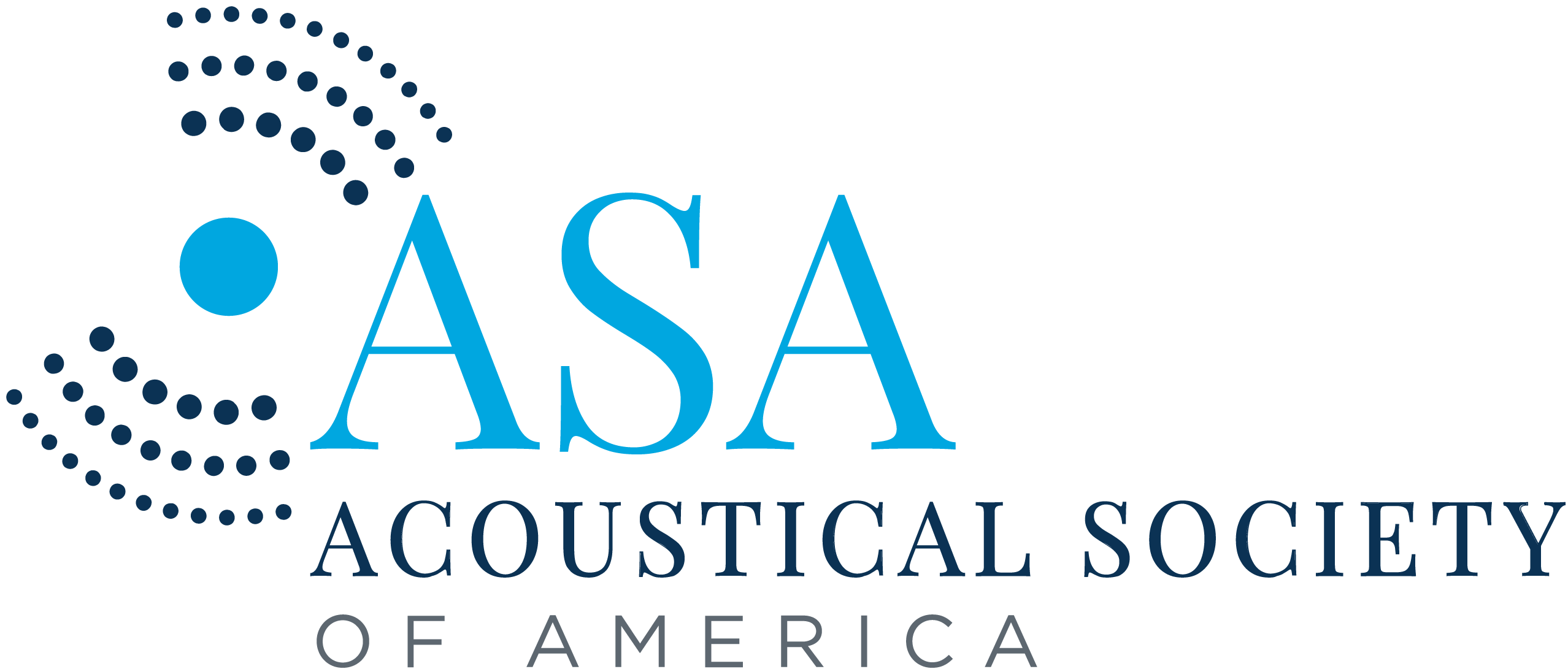
- Previous Article
- Next Article
Speech production knowledge in automatic speech recognition
Electronic mail: [email protected]
- Article contents
- Figures & tables
- Supplementary Data
- Peer Review
- Reprints and Permissions
- Cite Icon Cite
- Search Site
Simon King , Joe Frankel , Karen Livescu , Erik McDermott , Korin Richmond , Mirjam Wester; Speech production knowledge in automatic speech recognition. J. Acoust. Soc. Am. 1 February 2007; 121 (2): 723–742. https://doi.org/10.1121/1.2404622
Download citation file:
- Ris (Zotero)
- Reference Manager
Although much is known about how speech is produced, and research into speech production has resulted in measured articulatory data, feature systems of different kinds, and numerous models, speech production knowledge is almost totally ignored in current mainstream approaches to automatic speech recognition. Representations of speech production allow simple explanations for many phenomena observed in speech which cannot be easily analyzed from either acoustic signal or phonetic transcription alone. In this article, a survey of a growing body of work in which such representations are used to improve automatic speech recognition is provided.
Sign in via your Institution
Citing articles via.
- Online ISSN 1520-8524
- Print ISSN 0001-4966
- For Researchers
- For Librarians
- For Advertisers
- Our Publishing Partners
- Physics Today
- Conference Proceedings
- Special Topics
pubs.aip.org
- Privacy Policy
- Terms of Use
Connect with AIP Publishing
This feature is available to subscribers only.
Sign In or Create an Account
- Bibliography
- More Referencing guides Blog Automated transliteration Relevant bibliographies by topics
- Automated transliteration
- Relevant bibliographies by topics
- Referencing guides

IMAGES
VIDEO
COMMENTS
In this thesis, I present my work on a continuous silent speech recognition system for AlterEgo, a silent speech interface. By transcribing residual neurological signals sent from the brain to speech articulators during internal articulation, the system allows one to communicate without the need to speak or perform any visible movements or ...
Automatic speech recognition (ASR) decodes speech signals into text. While ASR can pro-duce accurate word recognition in clean environments, system performance can degrade dramatically when noise and reverberation are present. In this thesis, speech denoising and model adaptation for robust speech recognition were studied, and four novel meth-
Thesis: Ph. D., Massachusetts Institute of Technology, Department of Electrical Engineering and Computer Science, 2018. Cataloged from PDF version of thesis. ... The analyses illuminate the inner workings of end-to-end machine translation and speech recognition systems, explain how they capture different language properties, and suggest ...
A. R. Titus, A Study of Adaptive Enhancement Methods for Improved Distant Speech Recognition, M.Eng. Thesis, MIT Department of Electrical Engineering and Computer Science, June 2018. 2016 J. Drexler, Deep Unsupervised Learning from Speech, S. M. Thesis, MIT Department of Electrical Engineering and Computer Science, June 2016.
Deep Unsupervised Learning from Speech by Jennifer Fox Drexler B.S.E., Princeton University (2009) Submitted to the Department of Electrical Engineering and Computer Science in partial ful llment of the requirements for the degree of Master of Science in Electrical Engineering and Computer Science at the MASSACHUSETTS INSTITUTE OF TECHNOLOGY ...
Thesis (M. Eng.)--Massachusetts Institute of Technology, Dept. of Electrical Engineering and Computer Science, 2012. This electronic version was submitted by the student author. The certified thesis is available in the Institute Archives and Special Collections. ... Improving speech recognition accuracy for clinical conversations. Author(s ...
Automatic speech recognition (ASR) has been a grand challenge machine learning problem for decades. Our ongoing research in this area examines the use of deep learning models for distant and noisy recording conditions, multilingual, and low-resource scenarios. Unlike humans, automatic speech recognizers are not particularly sensitive to ...
thesis itself. My group taught me a lot over the years about various topics in speech and language and were excellent for bouncing around ideas. T. J. Hazen taught me a lot about the speech recognizer and Hung-An Chang helped me develop and understand various ideas for acoustic modeling. To all these people and many others
Multimodal Speech Recognition with Ultrasonic Sensors by Bo Zhu S.B., Massachusetts Institute of Technology (2007) Submitted to the Department of Electrical Engineering and Computer Science in partial fulfillment of the requirements for the degree of Master of Engineering in Electrical Engineering and Computer Science at the
In this thesis, I present my work on a continuous silent speech recognition system for AlterEgo, a silent speech interface. By transcribing residual neurological signals sent from the brain to speech articulators during internal articulation, the system allows one to communicate without the need to speak or perform any visible movements or gestures.
This thesis proposes a framework for the recognition of affective qualifiers from prosodic-acoustic parameters extracted form spoken language. It is argued that modeling the affective prosodic variation of speech can be approached by integrating acoustic parameters from various prosodic time scales, summarizing information from more localized ...
about 100 languages with Automatic Speech Recognition (ASR) capability. This is due to the fact that a vast amount of resources is required to build a speech recog-nizer. This often includes thousands of hours of transcribed speech data, a phonetic pronunciation dictionary or lexicon which spans all words in the language, and a text
Automatic speech recognition (ASR) is a key component of these interfaces that is computationally intensive. This thesis shows how we designed special-purpose integrated circuits to bring local ASR capabilities to electronic devices with a small size and power footprint. This thesis adopts a holistic, system-driven approach to ASR hardware design.
For a second correction pass and a conservative estimate of only. 30% corrections, the total time for our method becomes 1.2s + (1.35s + 1.5s + 1.5s * .3) * m =. 1.2s + 3.3s * m, making it the more effective method if 60% or more errors can be corrected in. both passes, or if the user is a particularly slow typer.
for Automatic Speech Recognition by Timothy J. Hazen S.M., Massachusetts Institute of Technology, 1993 S.B., Massachusetts Institute of Technology, 1991 Submitted to the Department of Electrical Engineering and Computer Science in Partial Ful llment of the Requirements for the Degree of Doctor of Philosophy at the Massachusetts Institute of ...
Kenneth N. Stevens was born on March 24, 1924, in Toronto, Canada. He received his B.A.Sc. and M.A.Sc. in engineering physics from the University of Toronto in 1945 and 1948 respectively, and his Sc.D. in electrical engineering from MIT in 1952. His dissertation was entitled "Perception of Sounds Shaped by Resonance Circuits".
Add to Calendar 2018-04-26 10:30:00 2018-04-26 11:30:00 America/New_York Thesis Defense: On Internal Language Representations in Deep Learning: An Analysis of Machine Translation and Speech Recognition Abstract:Language technology has become pervasive in everyday life, powering applications like Apple's Siri or Google's Assistant. Neural networks are a key component in these systems thanks ...
Representations of speech production allow simple explanations for many phenomena observed in speech which cannot be easily analyzed from either acoustic signal or phonetic transcription alone. In this article, a survey of a growing body of work in which such representations are used to improve automatic speech recognition is provided.
Thesis (M.Eng.)--Massachusetts Institute of Technology, Dept. of Electrical Engineering and Computer Science, February 2003. Includes bibliographical references (leaf 63). This thesis describes a speech recognition system that was built to support spontaneous speech understanding.
Scientists using AI have found sperm whales can vary the tempo, rhythm and length of their click sequences, creating a richer communication system than realized.