- Resources Home 🏠
- Try SciSpace Copilot
- Search research papers
- Add Copilot Extension
- Try AI Detector
- Try Paraphraser
- Try Citation Generator
- April Papers
- June Papers
- July Papers


The Craft of Writing a Strong Hypothesis

Table of Contents
Writing a hypothesis is one of the essential elements of a scientific research paper. It needs to be to the point, clearly communicating what your research is trying to accomplish. A blurry, drawn-out, or complexly-structured hypothesis can confuse your readers. Or worse, the editor and peer reviewers.
A captivating hypothesis is not too intricate. This blog will take you through the process so that, by the end of it, you have a better idea of how to convey your research paper's intent in just one sentence.
What is a Hypothesis?
The first step in your scientific endeavor, a hypothesis, is a strong, concise statement that forms the basis of your research. It is not the same as a thesis statement , which is a brief summary of your research paper .
The sole purpose of a hypothesis is to predict your paper's findings, data, and conclusion. It comes from a place of curiosity and intuition . When you write a hypothesis, you're essentially making an educated guess based on scientific prejudices and evidence, which is further proven or disproven through the scientific method.
The reason for undertaking research is to observe a specific phenomenon. A hypothesis, therefore, lays out what the said phenomenon is. And it does so through two variables, an independent and dependent variable.
The independent variable is the cause behind the observation, while the dependent variable is the effect of the cause. A good example of this is “mixing red and blue forms purple.” In this hypothesis, mixing red and blue is the independent variable as you're combining the two colors at your own will. The formation of purple is the dependent variable as, in this case, it is conditional to the independent variable.
Different Types of Hypotheses

Types of hypotheses
Some would stand by the notion that there are only two types of hypotheses: a Null hypothesis and an Alternative hypothesis. While that may have some truth to it, it would be better to fully distinguish the most common forms as these terms come up so often, which might leave you out of context.
Apart from Null and Alternative, there are Complex, Simple, Directional, Non-Directional, Statistical, and Associative and casual hypotheses. They don't necessarily have to be exclusive, as one hypothesis can tick many boxes, but knowing the distinctions between them will make it easier for you to construct your own.
1. Null hypothesis
A null hypothesis proposes no relationship between two variables. Denoted by H 0 , it is a negative statement like “Attending physiotherapy sessions does not affect athletes' on-field performance.” Here, the author claims physiotherapy sessions have no effect on on-field performances. Even if there is, it's only a coincidence.
2. Alternative hypothesis
Considered to be the opposite of a null hypothesis, an alternative hypothesis is donated as H1 or Ha. It explicitly states that the dependent variable affects the independent variable. A good alternative hypothesis example is “Attending physiotherapy sessions improves athletes' on-field performance.” or “Water evaporates at 100 °C. ” The alternative hypothesis further branches into directional and non-directional.
- Directional hypothesis: A hypothesis that states the result would be either positive or negative is called directional hypothesis. It accompanies H1 with either the ‘<' or ‘>' sign.
- Non-directional hypothesis: A non-directional hypothesis only claims an effect on the dependent variable. It does not clarify whether the result would be positive or negative. The sign for a non-directional hypothesis is ‘≠.'
3. Simple hypothesis
A simple hypothesis is a statement made to reflect the relation between exactly two variables. One independent and one dependent. Consider the example, “Smoking is a prominent cause of lung cancer." The dependent variable, lung cancer, is dependent on the independent variable, smoking.
4. Complex hypothesis
In contrast to a simple hypothesis, a complex hypothesis implies the relationship between multiple independent and dependent variables. For instance, “Individuals who eat more fruits tend to have higher immunity, lesser cholesterol, and high metabolism.” The independent variable is eating more fruits, while the dependent variables are higher immunity, lesser cholesterol, and high metabolism.
5. Associative and casual hypothesis
Associative and casual hypotheses don't exhibit how many variables there will be. They define the relationship between the variables. In an associative hypothesis, changing any one variable, dependent or independent, affects others. In a casual hypothesis, the independent variable directly affects the dependent.
6. Empirical hypothesis
Also referred to as the working hypothesis, an empirical hypothesis claims a theory's validation via experiments and observation. This way, the statement appears justifiable and different from a wild guess.
Say, the hypothesis is “Women who take iron tablets face a lesser risk of anemia than those who take vitamin B12.” This is an example of an empirical hypothesis where the researcher the statement after assessing a group of women who take iron tablets and charting the findings.
7. Statistical hypothesis
The point of a statistical hypothesis is to test an already existing hypothesis by studying a population sample. Hypothesis like “44% of the Indian population belong in the age group of 22-27.” leverage evidence to prove or disprove a particular statement.
Characteristics of a Good Hypothesis
Writing a hypothesis is essential as it can make or break your research for you. That includes your chances of getting published in a journal. So when you're designing one, keep an eye out for these pointers:
- A research hypothesis has to be simple yet clear to look justifiable enough.
- It has to be testable — your research would be rendered pointless if too far-fetched into reality or limited by technology.
- It has to be precise about the results —what you are trying to do and achieve through it should come out in your hypothesis.
- A research hypothesis should be self-explanatory, leaving no doubt in the reader's mind.
- If you are developing a relational hypothesis, you need to include the variables and establish an appropriate relationship among them.
- A hypothesis must keep and reflect the scope for further investigations and experiments.
Separating a Hypothesis from a Prediction
Outside of academia, hypothesis and prediction are often used interchangeably. In research writing, this is not only confusing but also incorrect. And although a hypothesis and prediction are guesses at their core, there are many differences between them.
A hypothesis is an educated guess or even a testable prediction validated through research. It aims to analyze the gathered evidence and facts to define a relationship between variables and put forth a logical explanation behind the nature of events.
Predictions are assumptions or expected outcomes made without any backing evidence. They are more fictionally inclined regardless of where they originate from.
For this reason, a hypothesis holds much more weight than a prediction. It sticks to the scientific method rather than pure guesswork. "Planets revolve around the Sun." is an example of a hypothesis as it is previous knowledge and observed trends. Additionally, we can test it through the scientific method.
Whereas "COVID-19 will be eradicated by 2030." is a prediction. Even though it results from past trends, we can't prove or disprove it. So, the only way this gets validated is to wait and watch if COVID-19 cases end by 2030.
Finally, How to Write a Hypothesis
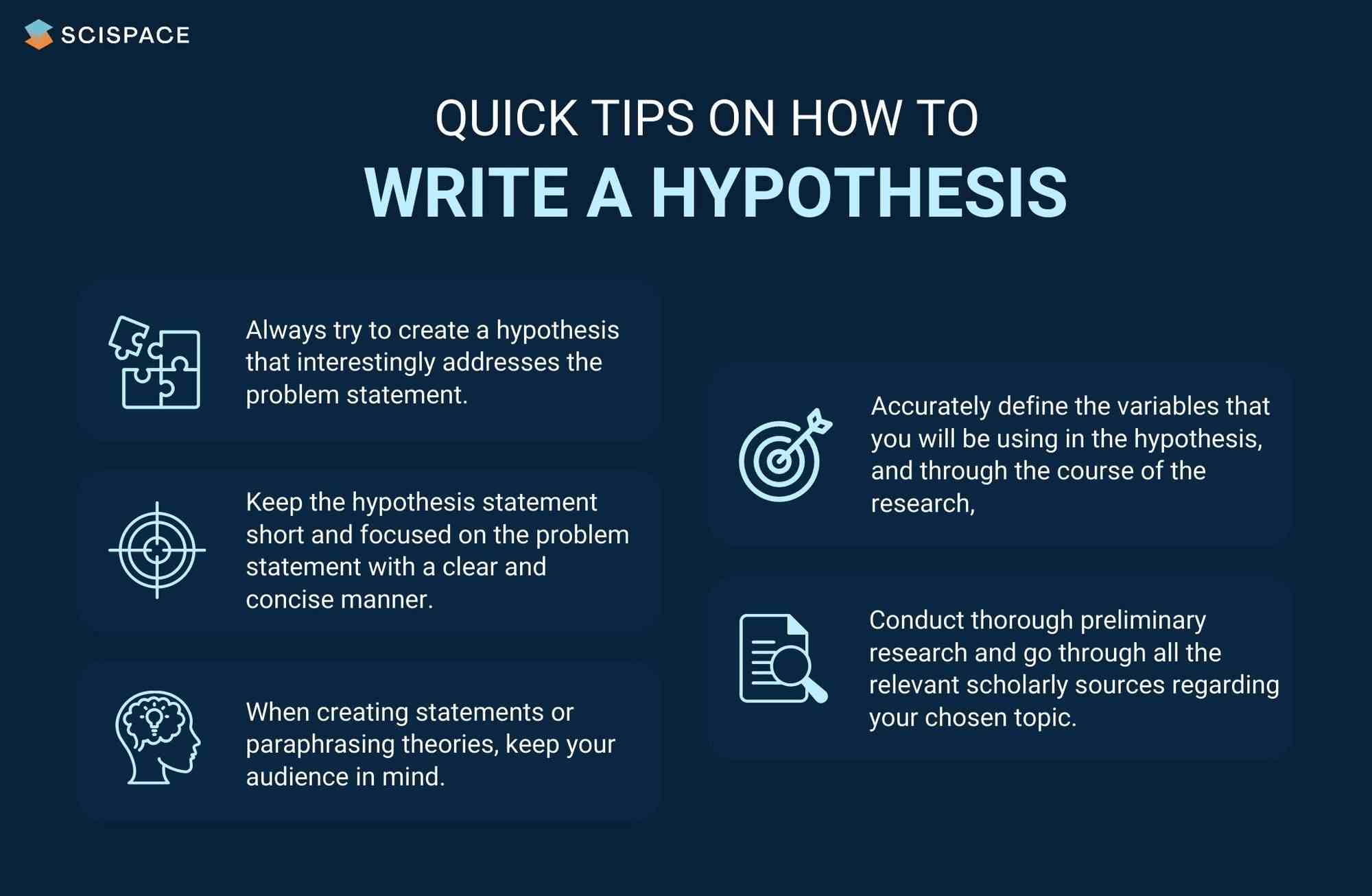
Quick tips on writing a hypothesis
1. Be clear about your research question
A hypothesis should instantly address the research question or the problem statement. To do so, you need to ask a question. Understand the constraints of your undertaken research topic and then formulate a simple and topic-centric problem. Only after that can you develop a hypothesis and further test for evidence.
2. Carry out a recce
Once you have your research's foundation laid out, it would be best to conduct preliminary research. Go through previous theories, academic papers, data, and experiments before you start curating your research hypothesis. It will give you an idea of your hypothesis's viability or originality.
Making use of references from relevant research papers helps draft a good research hypothesis. SciSpace Discover offers a repository of over 270 million research papers to browse through and gain a deeper understanding of related studies on a particular topic. Additionally, you can use SciSpace Copilot , your AI research assistant, for reading any lengthy research paper and getting a more summarized context of it. A hypothesis can be formed after evaluating many such summarized research papers. Copilot also offers explanations for theories and equations, explains paper in simplified version, allows you to highlight any text in the paper or clip math equations and tables and provides a deeper, clear understanding of what is being said. This can improve the hypothesis by helping you identify potential research gaps.
3. Create a 3-dimensional hypothesis
Variables are an essential part of any reasonable hypothesis. So, identify your independent and dependent variable(s) and form a correlation between them. The ideal way to do this is to write the hypothetical assumption in the ‘if-then' form. If you use this form, make sure that you state the predefined relationship between the variables.
In another way, you can choose to present your hypothesis as a comparison between two variables. Here, you must specify the difference you expect to observe in the results.
4. Write the first draft
Now that everything is in place, it's time to write your hypothesis. For starters, create the first draft. In this version, write what you expect to find from your research.
Clearly separate your independent and dependent variables and the link between them. Don't fixate on syntax at this stage. The goal is to ensure your hypothesis addresses the issue.
5. Proof your hypothesis
After preparing the first draft of your hypothesis, you need to inspect it thoroughly. It should tick all the boxes, like being concise, straightforward, relevant, and accurate. Your final hypothesis has to be well-structured as well.
Research projects are an exciting and crucial part of being a scholar. And once you have your research question, you need a great hypothesis to begin conducting research. Thus, knowing how to write a hypothesis is very important.
Now that you have a firmer grasp on what a good hypothesis constitutes, the different kinds there are, and what process to follow, you will find it much easier to write your hypothesis, which ultimately helps your research.
Now it's easier than ever to streamline your research workflow with SciSpace Discover . Its integrated, comprehensive end-to-end platform for research allows scholars to easily discover, write and publish their research and fosters collaboration.
It includes everything you need, including a repository of over 270 million research papers across disciplines, SEO-optimized summaries and public profiles to show your expertise and experience.
If you found these tips on writing a research hypothesis useful, head over to our blog on Statistical Hypothesis Testing to learn about the top researchers, papers, and institutions in this domain.
Frequently Asked Questions (FAQs)
1. what is the definition of hypothesis.
According to the Oxford dictionary, a hypothesis is defined as “An idea or explanation of something that is based on a few known facts, but that has not yet been proved to be true or correct”.
2. What is an example of hypothesis?
The hypothesis is a statement that proposes a relationship between two or more variables. An example: "If we increase the number of new users who join our platform by 25%, then we will see an increase in revenue."
3. What is an example of null hypothesis?
A null hypothesis is a statement that there is no relationship between two variables. The null hypothesis is written as H0. The null hypothesis states that there is no effect. For example, if you're studying whether or not a particular type of exercise increases strength, your null hypothesis will be "there is no difference in strength between people who exercise and people who don't."
4. What are the types of research?
• Fundamental research
• Applied research
• Qualitative research
• Quantitative research
• Mixed research
• Exploratory research
• Longitudinal research
• Cross-sectional research
• Field research
• Laboratory research
• Fixed research
• Flexible research
• Action research
• Policy research
• Classification research
• Comparative research
• Causal research
• Inductive research
• Deductive research
5. How to write a hypothesis?
• Your hypothesis should be able to predict the relationship and outcome.
• Avoid wordiness by keeping it simple and brief.
• Your hypothesis should contain observable and testable outcomes.
• Your hypothesis should be relevant to the research question.
6. What are the 2 types of hypothesis?
• Null hypotheses are used to test the claim that "there is no difference between two groups of data".
• Alternative hypotheses test the claim that "there is a difference between two data groups".
7. Difference between research question and research hypothesis?
A research question is a broad, open-ended question you will try to answer through your research. A hypothesis is a statement based on prior research or theory that you expect to be true due to your study. Example - Research question: What are the factors that influence the adoption of the new technology? Research hypothesis: There is a positive relationship between age, education and income level with the adoption of the new technology.
8. What is plural for hypothesis?
The plural of hypothesis is hypotheses. Here's an example of how it would be used in a statement, "Numerous well-considered hypotheses are presented in this part, and they are supported by tables and figures that are well-illustrated."
9. What is the red queen hypothesis?
The red queen hypothesis in evolutionary biology states that species must constantly evolve to avoid extinction because if they don't, they will be outcompeted by other species that are evolving. Leigh Van Valen first proposed it in 1973; since then, it has been tested and substantiated many times.
10. Who is known as the father of null hypothesis?
The father of the null hypothesis is Sir Ronald Fisher. He published a paper in 1925 that introduced the concept of null hypothesis testing, and he was also the first to use the term itself.
11. When to reject null hypothesis?
You need to find a significant difference between your two populations to reject the null hypothesis. You can determine that by running statistical tests such as an independent sample t-test or a dependent sample t-test. You should reject the null hypothesis if the p-value is less than 0.05.

You might also like

Consensus GPT vs. SciSpace GPT: Choose the Best GPT for Research
Literature Review and Theoretical Framework: Understanding the Differences

Types of Essays in Academic Writing - Quick Guide (2024)
Educational resources and simple solutions for your research journey
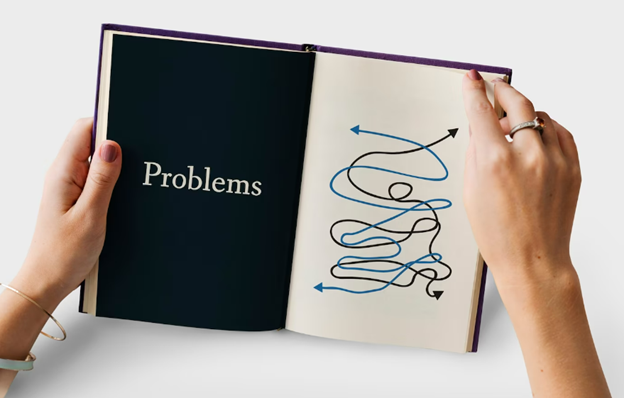
What is a Research Hypothesis: How to Write it, Types, and Examples
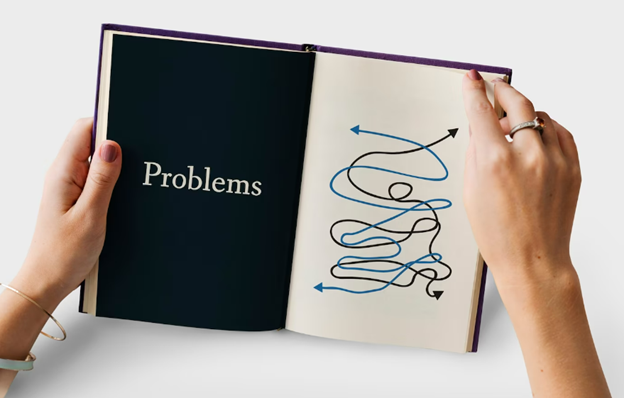
Any research begins with a research question and a research hypothesis . A research question alone may not suffice to design the experiment(s) needed to answer it. A hypothesis is central to the scientific method. But what is a hypothesis ? A hypothesis is a testable statement that proposes a possible explanation to a phenomenon, and it may include a prediction. Next, you may ask what is a research hypothesis ? Simply put, a research hypothesis is a prediction or educated guess about the relationship between the variables that you want to investigate.
It is important to be thorough when developing your research hypothesis. Shortcomings in the framing of a hypothesis can affect the study design and the results. A better understanding of the research hypothesis definition and characteristics of a good hypothesis will make it easier for you to develop your own hypothesis for your research. Let’s dive in to know more about the types of research hypothesis , how to write a research hypothesis , and some research hypothesis examples .
Table of Contents
What is a hypothesis ?
A hypothesis is based on the existing body of knowledge in a study area. Framed before the data are collected, a hypothesis states the tentative relationship between independent and dependent variables, along with a prediction of the outcome.
What is a research hypothesis ?
Young researchers starting out their journey are usually brimming with questions like “ What is a hypothesis ?” “ What is a research hypothesis ?” “How can I write a good research hypothesis ?”
A research hypothesis is a statement that proposes a possible explanation for an observable phenomenon or pattern. It guides the direction of a study and predicts the outcome of the investigation. A research hypothesis is testable, i.e., it can be supported or disproven through experimentation or observation.
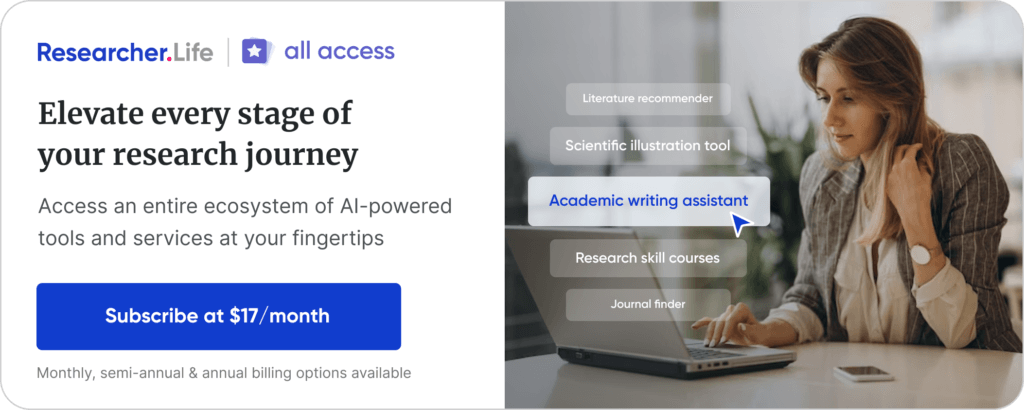
Characteristics of a good hypothesis
Here are the characteristics of a good hypothesis :
- Clearly formulated and free of language errors and ambiguity
- Concise and not unnecessarily verbose
- Has clearly defined variables
- Testable and stated in a way that allows for it to be disproven
- Can be tested using a research design that is feasible, ethical, and practical
- Specific and relevant to the research problem
- Rooted in a thorough literature search
- Can generate new knowledge or understanding.
How to create an effective research hypothesis
A study begins with the formulation of a research question. A researcher then performs background research. This background information forms the basis for building a good research hypothesis . The researcher then performs experiments, collects, and analyzes the data, interprets the findings, and ultimately, determines if the findings support or negate the original hypothesis.
Let’s look at each step for creating an effective, testable, and good research hypothesis :
- Identify a research problem or question: Start by identifying a specific research problem.
- Review the literature: Conduct an in-depth review of the existing literature related to the research problem to grasp the current knowledge and gaps in the field.
- Formulate a clear and testable hypothesis : Based on the research question, use existing knowledge to form a clear and testable hypothesis . The hypothesis should state a predicted relationship between two or more variables that can be measured and manipulated. Improve the original draft till it is clear and meaningful.
- State the null hypothesis: The null hypothesis is a statement that there is no relationship between the variables you are studying.
- Define the population and sample: Clearly define the population you are studying and the sample you will be using for your research.
- Select appropriate methods for testing the hypothesis: Select appropriate research methods, such as experiments, surveys, or observational studies, which will allow you to test your research hypothesis .
Remember that creating a research hypothesis is an iterative process, i.e., you might have to revise it based on the data you collect. You may need to test and reject several hypotheses before answering the research problem.
How to write a research hypothesis
When you start writing a research hypothesis , you use an “if–then” statement format, which states the predicted relationship between two or more variables. Clearly identify the independent variables (the variables being changed) and the dependent variables (the variables being measured), as well as the population you are studying. Review and revise your hypothesis as needed.
An example of a research hypothesis in this format is as follows:
“ If [athletes] follow [cold water showers daily], then their [endurance] increases.”
Population: athletes
Independent variable: daily cold water showers
Dependent variable: endurance
You may have understood the characteristics of a good hypothesis . But note that a research hypothesis is not always confirmed; a researcher should be prepared to accept or reject the hypothesis based on the study findings.
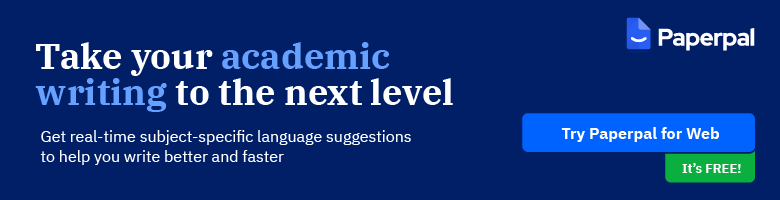
Research hypothesis checklist
Following from above, here is a 10-point checklist for a good research hypothesis :
- Testable: A research hypothesis should be able to be tested via experimentation or observation.
- Specific: A research hypothesis should clearly state the relationship between the variables being studied.
- Based on prior research: A research hypothesis should be based on existing knowledge and previous research in the field.
- Falsifiable: A research hypothesis should be able to be disproven through testing.
- Clear and concise: A research hypothesis should be stated in a clear and concise manner.
- Logical: A research hypothesis should be logical and consistent with current understanding of the subject.
- Relevant: A research hypothesis should be relevant to the research question and objectives.
- Feasible: A research hypothesis should be feasible to test within the scope of the study.
- Reflects the population: A research hypothesis should consider the population or sample being studied.
- Uncomplicated: A good research hypothesis is written in a way that is easy for the target audience to understand.
By following this research hypothesis checklist , you will be able to create a research hypothesis that is strong, well-constructed, and more likely to yield meaningful results.
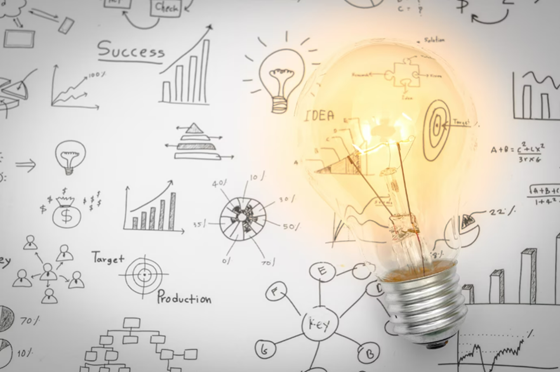
Types of research hypothesis
Different types of research hypothesis are used in scientific research:
1. Null hypothesis:
A null hypothesis states that there is no change in the dependent variable due to changes to the independent variable. This means that the results are due to chance and are not significant. A null hypothesis is denoted as H0 and is stated as the opposite of what the alternative hypothesis states.
Example: “ The newly identified virus is not zoonotic .”
2. Alternative hypothesis:
This states that there is a significant difference or relationship between the variables being studied. It is denoted as H1 or Ha and is usually accepted or rejected in favor of the null hypothesis.
Example: “ The newly identified virus is zoonotic .”
3. Directional hypothesis :
This specifies the direction of the relationship or difference between variables; therefore, it tends to use terms like increase, decrease, positive, negative, more, or less.
Example: “ The inclusion of intervention X decreases infant mortality compared to the original treatment .”
4. Non-directional hypothesis:
While it does not predict the exact direction or nature of the relationship between the two variables, a non-directional hypothesis states the existence of a relationship or difference between variables but not the direction, nature, or magnitude of the relationship. A non-directional hypothesis may be used when there is no underlying theory or when findings contradict previous research.
Example, “ Cats and dogs differ in the amount of affection they express .”
5. Simple hypothesis :
A simple hypothesis only predicts the relationship between one independent and another independent variable.
Example: “ Applying sunscreen every day slows skin aging .”
6 . Complex hypothesis :
A complex hypothesis states the relationship or difference between two or more independent and dependent variables.
Example: “ Applying sunscreen every day slows skin aging, reduces sun burn, and reduces the chances of skin cancer .” (Here, the three dependent variables are slowing skin aging, reducing sun burn, and reducing the chances of skin cancer.)
7. Associative hypothesis:
An associative hypothesis states that a change in one variable results in the change of the other variable. The associative hypothesis defines interdependency between variables.
Example: “ There is a positive association between physical activity levels and overall health .”
8 . Causal hypothesis:
A causal hypothesis proposes a cause-and-effect interaction between variables.
Example: “ Long-term alcohol use causes liver damage .”
Note that some of the types of research hypothesis mentioned above might overlap. The types of hypothesis chosen will depend on the research question and the objective of the study.
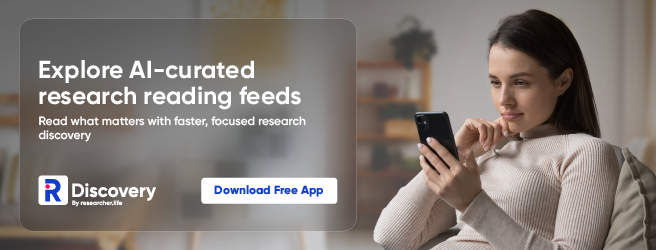
Research hypothesis examples
Here are some good research hypothesis examples :
“The use of a specific type of therapy will lead to a reduction in symptoms of depression in individuals with a history of major depressive disorder.”
“Providing educational interventions on healthy eating habits will result in weight loss in overweight individuals.”
“Plants that are exposed to certain types of music will grow taller than those that are not exposed to music.”
“The use of the plant growth regulator X will lead to an increase in the number of flowers produced by plants.”
Characteristics that make a research hypothesis weak are unclear variables, unoriginality, being too general or too vague, and being untestable. A weak hypothesis leads to weak research and improper methods.
Some bad research hypothesis examples (and the reasons why they are “bad”) are as follows:
“This study will show that treatment X is better than any other treatment . ” (This statement is not testable, too broad, and does not consider other treatments that may be effective.)
“This study will prove that this type of therapy is effective for all mental disorders . ” (This statement is too broad and not testable as mental disorders are complex and different disorders may respond differently to different types of therapy.)
“Plants can communicate with each other through telepathy . ” (This statement is not testable and lacks a scientific basis.)
Importance of testable hypothesis
If a research hypothesis is not testable, the results will not prove or disprove anything meaningful. The conclusions will be vague at best. A testable hypothesis helps a researcher focus on the study outcome and understand the implication of the question and the different variables involved. A testable hypothesis helps a researcher make precise predictions based on prior research.
To be considered testable, there must be a way to prove that the hypothesis is true or false; further, the results of the hypothesis must be reproducible.
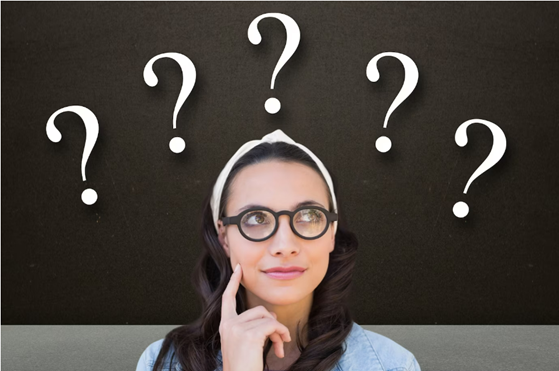
Frequently Asked Questions (FAQs) on research hypothesis
1. What is the difference between research question and research hypothesis ?
A research question defines the problem and helps outline the study objective(s). It is an open-ended statement that is exploratory or probing in nature. Therefore, it does not make predictions or assumptions. It helps a researcher identify what information to collect. A research hypothesis , however, is a specific, testable prediction about the relationship between variables. Accordingly, it guides the study design and data analysis approach.
2. When to reject null hypothesis ?
A null hypothesis should be rejected when the evidence from a statistical test shows that it is unlikely to be true. This happens when the test statistic (e.g., p -value) is less than the defined significance level (e.g., 0.05). Rejecting the null hypothesis does not necessarily mean that the alternative hypothesis is true; it simply means that the evidence found is not compatible with the null hypothesis.
3. How can I be sure my hypothesis is testable?
A testable hypothesis should be specific and measurable, and it should state a clear relationship between variables that can be tested with data. To ensure that your hypothesis is testable, consider the following:
- Clearly define the key variables in your hypothesis. You should be able to measure and manipulate these variables in a way that allows you to test the hypothesis.
- The hypothesis should predict a specific outcome or relationship between variables that can be measured or quantified.
- You should be able to collect the necessary data within the constraints of your study.
- It should be possible for other researchers to replicate your study, using the same methods and variables.
- Your hypothesis should be testable by using appropriate statistical analysis techniques, so you can draw conclusions, and make inferences about the population from the sample data.
- The hypothesis should be able to be disproven or rejected through the collection of data.
4. How do I revise my research hypothesis if my data does not support it?
If your data does not support your research hypothesis , you will need to revise it or develop a new one. You should examine your data carefully and identify any patterns or anomalies, re-examine your research question, and/or revisit your theory to look for any alternative explanations for your results. Based on your review of the data, literature, and theories, modify your research hypothesis to better align it with the results you obtained. Use your revised hypothesis to guide your research design and data collection. It is important to remain objective throughout the process.
5. I am performing exploratory research. Do I need to formulate a research hypothesis?
As opposed to “confirmatory” research, where a researcher has some idea about the relationship between the variables under investigation, exploratory research (or hypothesis-generating research) looks into a completely new topic about which limited information is available. Therefore, the researcher will not have any prior hypotheses. In such cases, a researcher will need to develop a post-hoc hypothesis. A post-hoc research hypothesis is generated after these results are known.
6. How is a research hypothesis different from a research question?
A research question is an inquiry about a specific topic or phenomenon, typically expressed as a question. It seeks to explore and understand a particular aspect of the research subject. In contrast, a research hypothesis is a specific statement or prediction that suggests an expected relationship between variables. It is formulated based on existing knowledge or theories and guides the research design and data analysis.
7. Can a research hypothesis change during the research process?
Yes, research hypotheses can change during the research process. As researchers collect and analyze data, new insights and information may emerge that require modification or refinement of the initial hypotheses. This can be due to unexpected findings, limitations in the original hypotheses, or the need to explore additional dimensions of the research topic. Flexibility is crucial in research, allowing for adaptation and adjustment of hypotheses to align with the evolving understanding of the subject matter.
8. How many hypotheses should be included in a research study?
The number of research hypotheses in a research study varies depending on the nature and scope of the research. It is not necessary to have multiple hypotheses in every study. Some studies may have only one primary hypothesis, while others may have several related hypotheses. The number of hypotheses should be determined based on the research objectives, research questions, and the complexity of the research topic. It is important to ensure that the hypotheses are focused, testable, and directly related to the research aims.
9. Can research hypotheses be used in qualitative research?
Yes, research hypotheses can be used in qualitative research, although they are more commonly associated with quantitative research. In qualitative research, hypotheses may be formulated as tentative or exploratory statements that guide the investigation. Instead of testing hypotheses through statistical analysis, qualitative researchers may use the hypotheses to guide data collection and analysis, seeking to uncover patterns, themes, or relationships within the qualitative data. The emphasis in qualitative research is often on generating insights and understanding rather than confirming or rejecting specific research hypotheses through statistical testing.
Researcher.Life is a subscription-based platform that unifies the best AI tools and services designed to speed up, simplify, and streamline every step of a researcher’s journey. The Researcher.Life All Access Pack is a one-of-a-kind subscription that unlocks full access to an AI writing assistant, literature recommender, journal finder, scientific illustration tool, and exclusive discounts on professional publication services from Editage.
Based on 21+ years of experience in academia, Researcher.Life All Access empowers researchers to put their best research forward and move closer to success. Explore our top AI Tools pack, AI Tools + Publication Services pack, or Build Your Own Plan. Find everything a researcher needs to succeed, all in one place – Get All Access now starting at just $17 a month !
Related Posts

Language and Cultural Barriers in Research: How to Bridge the Gap

Tips on Writing the Acknowledgments Section
- Bipolar Disorder
- Therapy Center
- When To See a Therapist
- Types of Therapy
- Best Online Therapy
- Best Couples Therapy
- Best Family Therapy
- Managing Stress
- Sleep and Dreaming
- Understanding Emotions
- Self-Improvement
- Healthy Relationships
- Student Resources
- Personality Types
- Guided Meditations
- Verywell Mind Insights
- 2024 Verywell Mind 25
- Mental Health in the Classroom
- Editorial Process
- Meet Our Review Board
- Crisis Support
How to Write a Great Hypothesis
Hypothesis Definition, Format, Examples, and Tips
Kendra Cherry, MS, is a psychosocial rehabilitation specialist, psychology educator, and author of the "Everything Psychology Book."
:max_bytes(150000):strip_icc():format(webp)/IMG_9791-89504ab694d54b66bbd72cb84ffb860e.jpg)
Amy Morin, LCSW, is a psychotherapist and international bestselling author. Her books, including "13 Things Mentally Strong People Don't Do," have been translated into more than 40 languages. Her TEDx talk, "The Secret of Becoming Mentally Strong," is one of the most viewed talks of all time.
:max_bytes(150000):strip_icc():format(webp)/VW-MIND-Amy-2b338105f1ee493f94d7e333e410fa76.jpg)
Verywell / Alex Dos Diaz
- The Scientific Method
Hypothesis Format
Falsifiability of a hypothesis.
- Operationalization
Hypothesis Types
Hypotheses examples.
- Collecting Data
A hypothesis is a tentative statement about the relationship between two or more variables. It is a specific, testable prediction about what you expect to happen in a study. It is a preliminary answer to your question that helps guide the research process.
Consider a study designed to examine the relationship between sleep deprivation and test performance. The hypothesis might be: "This study is designed to assess the hypothesis that sleep-deprived people will perform worse on a test than individuals who are not sleep-deprived."
At a Glance
A hypothesis is crucial to scientific research because it offers a clear direction for what the researchers are looking to find. This allows them to design experiments to test their predictions and add to our scientific knowledge about the world. This article explores how a hypothesis is used in psychology research, how to write a good hypothesis, and the different types of hypotheses you might use.
The Hypothesis in the Scientific Method
In the scientific method , whether it involves research in psychology, biology, or some other area, a hypothesis represents what the researchers think will happen in an experiment. The scientific method involves the following steps:
- Forming a question
- Performing background research
- Creating a hypothesis
- Designing an experiment
- Collecting data
- Analyzing the results
- Drawing conclusions
- Communicating the results
The hypothesis is a prediction, but it involves more than a guess. Most of the time, the hypothesis begins with a question which is then explored through background research. At this point, researchers then begin to develop a testable hypothesis.
Unless you are creating an exploratory study, your hypothesis should always explain what you expect to happen.
In a study exploring the effects of a particular drug, the hypothesis might be that researchers expect the drug to have some type of effect on the symptoms of a specific illness. In psychology, the hypothesis might focus on how a certain aspect of the environment might influence a particular behavior.
Remember, a hypothesis does not have to be correct. While the hypothesis predicts what the researchers expect to see, the goal of the research is to determine whether this guess is right or wrong. When conducting an experiment, researchers might explore numerous factors to determine which ones might contribute to the ultimate outcome.
In many cases, researchers may find that the results of an experiment do not support the original hypothesis. When writing up these results, the researchers might suggest other options that should be explored in future studies.
In many cases, researchers might draw a hypothesis from a specific theory or build on previous research. For example, prior research has shown that stress can impact the immune system. So a researcher might hypothesize: "People with high-stress levels will be more likely to contract a common cold after being exposed to the virus than people who have low-stress levels."
In other instances, researchers might look at commonly held beliefs or folk wisdom. "Birds of a feather flock together" is one example of folk adage that a psychologist might try to investigate. The researcher might pose a specific hypothesis that "People tend to select romantic partners who are similar to them in interests and educational level."
Elements of a Good Hypothesis
So how do you write a good hypothesis? When trying to come up with a hypothesis for your research or experiments, ask yourself the following questions:
- Is your hypothesis based on your research on a topic?
- Can your hypothesis be tested?
- Does your hypothesis include independent and dependent variables?
Before you come up with a specific hypothesis, spend some time doing background research. Once you have completed a literature review, start thinking about potential questions you still have. Pay attention to the discussion section in the journal articles you read . Many authors will suggest questions that still need to be explored.
How to Formulate a Good Hypothesis
To form a hypothesis, you should take these steps:
- Collect as many observations about a topic or problem as you can.
- Evaluate these observations and look for possible causes of the problem.
- Create a list of possible explanations that you might want to explore.
- After you have developed some possible hypotheses, think of ways that you could confirm or disprove each hypothesis through experimentation. This is known as falsifiability.
In the scientific method , falsifiability is an important part of any valid hypothesis. In order to test a claim scientifically, it must be possible that the claim could be proven false.
Students sometimes confuse the idea of falsifiability with the idea that it means that something is false, which is not the case. What falsifiability means is that if something was false, then it is possible to demonstrate that it is false.
One of the hallmarks of pseudoscience is that it makes claims that cannot be refuted or proven false.
The Importance of Operational Definitions
A variable is a factor or element that can be changed and manipulated in ways that are observable and measurable. However, the researcher must also define how the variable will be manipulated and measured in the study.
Operational definitions are specific definitions for all relevant factors in a study. This process helps make vague or ambiguous concepts detailed and measurable.
For example, a researcher might operationally define the variable " test anxiety " as the results of a self-report measure of anxiety experienced during an exam. A "study habits" variable might be defined by the amount of studying that actually occurs as measured by time.
These precise descriptions are important because many things can be measured in various ways. Clearly defining these variables and how they are measured helps ensure that other researchers can replicate your results.
Replicability
One of the basic principles of any type of scientific research is that the results must be replicable.
Replication means repeating an experiment in the same way to produce the same results. By clearly detailing the specifics of how the variables were measured and manipulated, other researchers can better understand the results and repeat the study if needed.
Some variables are more difficult than others to define. For example, how would you operationally define a variable such as aggression ? For obvious ethical reasons, researchers cannot create a situation in which a person behaves aggressively toward others.
To measure this variable, the researcher must devise a measurement that assesses aggressive behavior without harming others. The researcher might utilize a simulated task to measure aggressiveness in this situation.
Hypothesis Checklist
- Does your hypothesis focus on something that you can actually test?
- Does your hypothesis include both an independent and dependent variable?
- Can you manipulate the variables?
- Can your hypothesis be tested without violating ethical standards?
The hypothesis you use will depend on what you are investigating and hoping to find. Some of the main types of hypotheses that you might use include:
- Simple hypothesis : This type of hypothesis suggests there is a relationship between one independent variable and one dependent variable.
- Complex hypothesis : This type suggests a relationship between three or more variables, such as two independent and dependent variables.
- Null hypothesis : This hypothesis suggests no relationship exists between two or more variables.
- Alternative hypothesis : This hypothesis states the opposite of the null hypothesis.
- Statistical hypothesis : This hypothesis uses statistical analysis to evaluate a representative population sample and then generalizes the findings to the larger group.
- Logical hypothesis : This hypothesis assumes a relationship between variables without collecting data or evidence.
A hypothesis often follows a basic format of "If {this happens} then {this will happen}." One way to structure your hypothesis is to describe what will happen to the dependent variable if you change the independent variable .
The basic format might be: "If {these changes are made to a certain independent variable}, then we will observe {a change in a specific dependent variable}."
A few examples of simple hypotheses:
- "Students who eat breakfast will perform better on a math exam than students who do not eat breakfast."
- "Students who experience test anxiety before an English exam will get lower scores than students who do not experience test anxiety."
- "Motorists who talk on the phone while driving will be more likely to make errors on a driving course than those who do not talk on the phone."
- "Children who receive a new reading intervention will have higher reading scores than students who do not receive the intervention."
Examples of a complex hypothesis include:
- "People with high-sugar diets and sedentary activity levels are more likely to develop depression."
- "Younger people who are regularly exposed to green, outdoor areas have better subjective well-being than older adults who have limited exposure to green spaces."
Examples of a null hypothesis include:
- "There is no difference in anxiety levels between people who take St. John's wort supplements and those who do not."
- "There is no difference in scores on a memory recall task between children and adults."
- "There is no difference in aggression levels between children who play first-person shooter games and those who do not."
Examples of an alternative hypothesis:
- "People who take St. John's wort supplements will have less anxiety than those who do not."
- "Adults will perform better on a memory task than children."
- "Children who play first-person shooter games will show higher levels of aggression than children who do not."
Collecting Data on Your Hypothesis
Once a researcher has formed a testable hypothesis, the next step is to select a research design and start collecting data. The research method depends largely on exactly what they are studying. There are two basic types of research methods: descriptive research and experimental research.
Descriptive Research Methods
Descriptive research such as case studies , naturalistic observations , and surveys are often used when conducting an experiment is difficult or impossible. These methods are best used to describe different aspects of a behavior or psychological phenomenon.
Once a researcher has collected data using descriptive methods, a correlational study can examine how the variables are related. This research method might be used to investigate a hypothesis that is difficult to test experimentally.
Experimental Research Methods
Experimental methods are used to demonstrate causal relationships between variables. In an experiment, the researcher systematically manipulates a variable of interest (known as the independent variable) and measures the effect on another variable (known as the dependent variable).
Unlike correlational studies, which can only be used to determine if there is a relationship between two variables, experimental methods can be used to determine the actual nature of the relationship—whether changes in one variable actually cause another to change.
The hypothesis is a critical part of any scientific exploration. It represents what researchers expect to find in a study or experiment. In situations where the hypothesis is unsupported by the research, the research still has value. Such research helps us better understand how different aspects of the natural world relate to one another. It also helps us develop new hypotheses that can then be tested in the future.
Thompson WH, Skau S. On the scope of scientific hypotheses . R Soc Open Sci . 2023;10(8):230607. doi:10.1098/rsos.230607
Taran S, Adhikari NKJ, Fan E. Falsifiability in medicine: what clinicians can learn from Karl Popper [published correction appears in Intensive Care Med. 2021 Jun 17;:]. Intensive Care Med . 2021;47(9):1054-1056. doi:10.1007/s00134-021-06432-z
Eyler AA. Research Methods for Public Health . 1st ed. Springer Publishing Company; 2020. doi:10.1891/9780826182067.0004
Nosek BA, Errington TM. What is replication ? PLoS Biol . 2020;18(3):e3000691. doi:10.1371/journal.pbio.3000691
Aggarwal R, Ranganathan P. Study designs: Part 2 - Descriptive studies . Perspect Clin Res . 2019;10(1):34-36. doi:10.4103/picr.PICR_154_18
Nevid J. Psychology: Concepts and Applications. Wadworth, 2013.
By Kendra Cherry, MSEd Kendra Cherry, MS, is a psychosocial rehabilitation specialist, psychology educator, and author of the "Everything Psychology Book."
- Privacy Policy

Home » What is a Hypothesis – Types, Examples and Writing Guide
What is a Hypothesis – Types, Examples and Writing Guide
Table of Contents

Definition:
Hypothesis is an educated guess or proposed explanation for a phenomenon, based on some initial observations or data. It is a tentative statement that can be tested and potentially proven or disproven through further investigation and experimentation.
Hypothesis is often used in scientific research to guide the design of experiments and the collection and analysis of data. It is an essential element of the scientific method, as it allows researchers to make predictions about the outcome of their experiments and to test those predictions to determine their accuracy.
Types of Hypothesis
Types of Hypothesis are as follows:
Research Hypothesis
A research hypothesis is a statement that predicts a relationship between variables. It is usually formulated as a specific statement that can be tested through research, and it is often used in scientific research to guide the design of experiments.
Null Hypothesis
The null hypothesis is a statement that assumes there is no significant difference or relationship between variables. It is often used as a starting point for testing the research hypothesis, and if the results of the study reject the null hypothesis, it suggests that there is a significant difference or relationship between variables.
Alternative Hypothesis
An alternative hypothesis is a statement that assumes there is a significant difference or relationship between variables. It is often used as an alternative to the null hypothesis and is tested against the null hypothesis to determine which statement is more accurate.
Directional Hypothesis
A directional hypothesis is a statement that predicts the direction of the relationship between variables. For example, a researcher might predict that increasing the amount of exercise will result in a decrease in body weight.
Non-directional Hypothesis
A non-directional hypothesis is a statement that predicts the relationship between variables but does not specify the direction. For example, a researcher might predict that there is a relationship between the amount of exercise and body weight, but they do not specify whether increasing or decreasing exercise will affect body weight.
Statistical Hypothesis
A statistical hypothesis is a statement that assumes a particular statistical model or distribution for the data. It is often used in statistical analysis to test the significance of a particular result.
Composite Hypothesis
A composite hypothesis is a statement that assumes more than one condition or outcome. It can be divided into several sub-hypotheses, each of which represents a different possible outcome.
Empirical Hypothesis
An empirical hypothesis is a statement that is based on observed phenomena or data. It is often used in scientific research to develop theories or models that explain the observed phenomena.
Simple Hypothesis
A simple hypothesis is a statement that assumes only one outcome or condition. It is often used in scientific research to test a single variable or factor.
Complex Hypothesis
A complex hypothesis is a statement that assumes multiple outcomes or conditions. It is often used in scientific research to test the effects of multiple variables or factors on a particular outcome.
Applications of Hypothesis
Hypotheses are used in various fields to guide research and make predictions about the outcomes of experiments or observations. Here are some examples of how hypotheses are applied in different fields:
- Science : In scientific research, hypotheses are used to test the validity of theories and models that explain natural phenomena. For example, a hypothesis might be formulated to test the effects of a particular variable on a natural system, such as the effects of climate change on an ecosystem.
- Medicine : In medical research, hypotheses are used to test the effectiveness of treatments and therapies for specific conditions. For example, a hypothesis might be formulated to test the effects of a new drug on a particular disease.
- Psychology : In psychology, hypotheses are used to test theories and models of human behavior and cognition. For example, a hypothesis might be formulated to test the effects of a particular stimulus on the brain or behavior.
- Sociology : In sociology, hypotheses are used to test theories and models of social phenomena, such as the effects of social structures or institutions on human behavior. For example, a hypothesis might be formulated to test the effects of income inequality on crime rates.
- Business : In business research, hypotheses are used to test the validity of theories and models that explain business phenomena, such as consumer behavior or market trends. For example, a hypothesis might be formulated to test the effects of a new marketing campaign on consumer buying behavior.
- Engineering : In engineering, hypotheses are used to test the effectiveness of new technologies or designs. For example, a hypothesis might be formulated to test the efficiency of a new solar panel design.
How to write a Hypothesis
Here are the steps to follow when writing a hypothesis:
Identify the Research Question
The first step is to identify the research question that you want to answer through your study. This question should be clear, specific, and focused. It should be something that can be investigated empirically and that has some relevance or significance in the field.
Conduct a Literature Review
Before writing your hypothesis, it’s essential to conduct a thorough literature review to understand what is already known about the topic. This will help you to identify the research gap and formulate a hypothesis that builds on existing knowledge.
Determine the Variables
The next step is to identify the variables involved in the research question. A variable is any characteristic or factor that can vary or change. There are two types of variables: independent and dependent. The independent variable is the one that is manipulated or changed by the researcher, while the dependent variable is the one that is measured or observed as a result of the independent variable.
Formulate the Hypothesis
Based on the research question and the variables involved, you can now formulate your hypothesis. A hypothesis should be a clear and concise statement that predicts the relationship between the variables. It should be testable through empirical research and based on existing theory or evidence.
Write the Null Hypothesis
The null hypothesis is the opposite of the alternative hypothesis, which is the hypothesis that you are testing. The null hypothesis states that there is no significant difference or relationship between the variables. It is important to write the null hypothesis because it allows you to compare your results with what would be expected by chance.
Refine the Hypothesis
After formulating the hypothesis, it’s important to refine it and make it more precise. This may involve clarifying the variables, specifying the direction of the relationship, or making the hypothesis more testable.
Examples of Hypothesis
Here are a few examples of hypotheses in different fields:
- Psychology : “Increased exposure to violent video games leads to increased aggressive behavior in adolescents.”
- Biology : “Higher levels of carbon dioxide in the atmosphere will lead to increased plant growth.”
- Sociology : “Individuals who grow up in households with higher socioeconomic status will have higher levels of education and income as adults.”
- Education : “Implementing a new teaching method will result in higher student achievement scores.”
- Marketing : “Customers who receive a personalized email will be more likely to make a purchase than those who receive a generic email.”
- Physics : “An increase in temperature will cause an increase in the volume of a gas, assuming all other variables remain constant.”
- Medicine : “Consuming a diet high in saturated fats will increase the risk of developing heart disease.”
Purpose of Hypothesis
The purpose of a hypothesis is to provide a testable explanation for an observed phenomenon or a prediction of a future outcome based on existing knowledge or theories. A hypothesis is an essential part of the scientific method and helps to guide the research process by providing a clear focus for investigation. It enables scientists to design experiments or studies to gather evidence and data that can support or refute the proposed explanation or prediction.
The formulation of a hypothesis is based on existing knowledge, observations, and theories, and it should be specific, testable, and falsifiable. A specific hypothesis helps to define the research question, which is important in the research process as it guides the selection of an appropriate research design and methodology. Testability of the hypothesis means that it can be proven or disproven through empirical data collection and analysis. Falsifiability means that the hypothesis should be formulated in such a way that it can be proven wrong if it is incorrect.
In addition to guiding the research process, the testing of hypotheses can lead to new discoveries and advancements in scientific knowledge. When a hypothesis is supported by the data, it can be used to develop new theories or models to explain the observed phenomenon. When a hypothesis is not supported by the data, it can help to refine existing theories or prompt the development of new hypotheses to explain the phenomenon.
When to use Hypothesis
Here are some common situations in which hypotheses are used:
- In scientific research , hypotheses are used to guide the design of experiments and to help researchers make predictions about the outcomes of those experiments.
- In social science research , hypotheses are used to test theories about human behavior, social relationships, and other phenomena.
- I n business , hypotheses can be used to guide decisions about marketing, product development, and other areas. For example, a hypothesis might be that a new product will sell well in a particular market, and this hypothesis can be tested through market research.
Characteristics of Hypothesis
Here are some common characteristics of a hypothesis:
- Testable : A hypothesis must be able to be tested through observation or experimentation. This means that it must be possible to collect data that will either support or refute the hypothesis.
- Falsifiable : A hypothesis must be able to be proven false if it is not supported by the data. If a hypothesis cannot be falsified, then it is not a scientific hypothesis.
- Clear and concise : A hypothesis should be stated in a clear and concise manner so that it can be easily understood and tested.
- Based on existing knowledge : A hypothesis should be based on existing knowledge and research in the field. It should not be based on personal beliefs or opinions.
- Specific : A hypothesis should be specific in terms of the variables being tested and the predicted outcome. This will help to ensure that the research is focused and well-designed.
- Tentative: A hypothesis is a tentative statement or assumption that requires further testing and evidence to be confirmed or refuted. It is not a final conclusion or assertion.
- Relevant : A hypothesis should be relevant to the research question or problem being studied. It should address a gap in knowledge or provide a new perspective on the issue.
Advantages of Hypothesis
Hypotheses have several advantages in scientific research and experimentation:
- Guides research: A hypothesis provides a clear and specific direction for research. It helps to focus the research question, select appropriate methods and variables, and interpret the results.
- Predictive powe r: A hypothesis makes predictions about the outcome of research, which can be tested through experimentation. This allows researchers to evaluate the validity of the hypothesis and make new discoveries.
- Facilitates communication: A hypothesis provides a common language and framework for scientists to communicate with one another about their research. This helps to facilitate the exchange of ideas and promotes collaboration.
- Efficient use of resources: A hypothesis helps researchers to use their time, resources, and funding efficiently by directing them towards specific research questions and methods that are most likely to yield results.
- Provides a basis for further research: A hypothesis that is supported by data provides a basis for further research and exploration. It can lead to new hypotheses, theories, and discoveries.
- Increases objectivity: A hypothesis can help to increase objectivity in research by providing a clear and specific framework for testing and interpreting results. This can reduce bias and increase the reliability of research findings.
Limitations of Hypothesis
Some Limitations of the Hypothesis are as follows:
- Limited to observable phenomena: Hypotheses are limited to observable phenomena and cannot account for unobservable or intangible factors. This means that some research questions may not be amenable to hypothesis testing.
- May be inaccurate or incomplete: Hypotheses are based on existing knowledge and research, which may be incomplete or inaccurate. This can lead to flawed hypotheses and erroneous conclusions.
- May be biased: Hypotheses may be biased by the researcher’s own beliefs, values, or assumptions. This can lead to selective interpretation of data and a lack of objectivity in research.
- Cannot prove causation: A hypothesis can only show a correlation between variables, but it cannot prove causation. This requires further experimentation and analysis.
- Limited to specific contexts: Hypotheses are limited to specific contexts and may not be generalizable to other situations or populations. This means that results may not be applicable in other contexts or may require further testing.
- May be affected by chance : Hypotheses may be affected by chance or random variation, which can obscure or distort the true relationship between variables.
About the author
Muhammad Hassan
Researcher, Academic Writer, Web developer
You may also like

Research Process – Steps, Examples and Tips

Significance of the Study – Examples and Writing...

Research Report – Example, Writing Guide and...

Dissertation – Format, Example and Template

Research Problem – Examples, Types and Guide

Limitations in Research – Types, Examples and...

How to Develop a Good Research Hypothesis
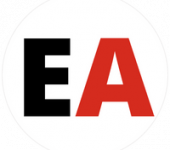
The story of a research study begins by asking a question. Researchers all around the globe are asking curious questions and formulating research hypothesis. However, whether the research study provides an effective conclusion depends on how well one develops a good research hypothesis. Research hypothesis examples could help researchers get an idea as to how to write a good research hypothesis.
This blog will help you understand what is a research hypothesis, its characteristics and, how to formulate a research hypothesis
Table of Contents
What is Hypothesis?
Hypothesis is an assumption or an idea proposed for the sake of argument so that it can be tested. It is a precise, testable statement of what the researchers predict will be outcome of the study. Hypothesis usually involves proposing a relationship between two variables: the independent variable (what the researchers change) and the dependent variable (what the research measures).
What is a Research Hypothesis?
Research hypothesis is a statement that introduces a research question and proposes an expected result. It is an integral part of the scientific method that forms the basis of scientific experiments. Therefore, you need to be careful and thorough when building your research hypothesis. A minor flaw in the construction of your hypothesis could have an adverse effect on your experiment. In research, there is a convention that the hypothesis is written in two forms, the null hypothesis, and the alternative hypothesis (called the experimental hypothesis when the method of investigation is an experiment).
Characteristics of a Good Research Hypothesis
As the hypothesis is specific, there is a testable prediction about what you expect to happen in a study. You may consider drawing hypothesis from previously published research based on the theory.
A good research hypothesis involves more effort than just a guess. In particular, your hypothesis may begin with a question that could be further explored through background research.
To help you formulate a promising research hypothesis, you should ask yourself the following questions:
- Is the language clear and focused?
- What is the relationship between your hypothesis and your research topic?
- Is your hypothesis testable? If yes, then how?
- What are the possible explanations that you might want to explore?
- Does your hypothesis include both an independent and dependent variable?
- Can you manipulate your variables without hampering the ethical standards?
- Does your research predict the relationship and outcome?
- Is your research simple and concise (avoids wordiness)?
- Is it clear with no ambiguity or assumptions about the readers’ knowledge
- Is your research observable and testable results?
- Is it relevant and specific to the research question or problem?
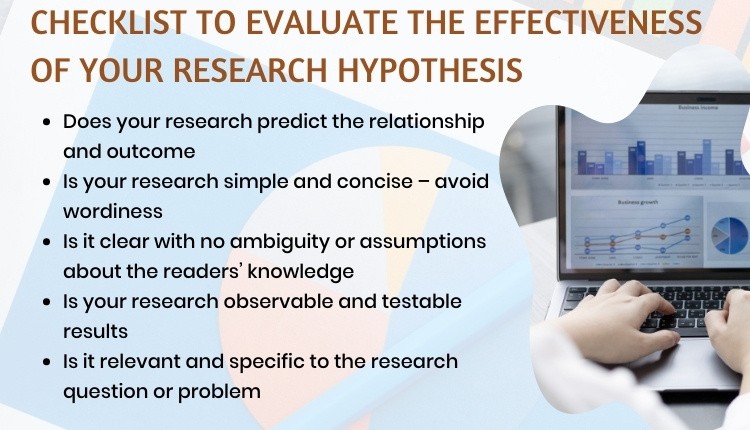
The questions listed above can be used as a checklist to make sure your hypothesis is based on a solid foundation. Furthermore, it can help you identify weaknesses in your hypothesis and revise it if necessary.
Source: Educational Hub
How to formulate a research hypothesis.
A testable hypothesis is not a simple statement. It is rather an intricate statement that needs to offer a clear introduction to a scientific experiment, its intentions, and the possible outcomes. However, there are some important things to consider when building a compelling hypothesis.
1. State the problem that you are trying to solve.
Make sure that the hypothesis clearly defines the topic and the focus of the experiment.
2. Try to write the hypothesis as an if-then statement.
Follow this template: If a specific action is taken, then a certain outcome is expected.
3. Define the variables
Independent variables are the ones that are manipulated, controlled, or changed. Independent variables are isolated from other factors of the study.
Dependent variables , as the name suggests are dependent on other factors of the study. They are influenced by the change in independent variable.
4. Scrutinize the hypothesis
Evaluate assumptions, predictions, and evidence rigorously to refine your understanding.
Types of Research Hypothesis
The types of research hypothesis are stated below:
1. Simple Hypothesis
It predicts the relationship between a single dependent variable and a single independent variable.
2. Complex Hypothesis
It predicts the relationship between two or more independent and dependent variables.
3. Directional Hypothesis
It specifies the expected direction to be followed to determine the relationship between variables and is derived from theory. Furthermore, it implies the researcher’s intellectual commitment to a particular outcome.
4. Non-directional Hypothesis
It does not predict the exact direction or nature of the relationship between the two variables. The non-directional hypothesis is used when there is no theory involved or when findings contradict previous research.
5. Associative and Causal Hypothesis
The associative hypothesis defines interdependency between variables. A change in one variable results in the change of the other variable. On the other hand, the causal hypothesis proposes an effect on the dependent due to manipulation of the independent variable.
6. Null Hypothesis
Null hypothesis states a negative statement to support the researcher’s findings that there is no relationship between two variables. There will be no changes in the dependent variable due the manipulation of the independent variable. Furthermore, it states results are due to chance and are not significant in terms of supporting the idea being investigated.
7. Alternative Hypothesis
It states that there is a relationship between the two variables of the study and that the results are significant to the research topic. An experimental hypothesis predicts what changes will take place in the dependent variable when the independent variable is manipulated. Also, it states that the results are not due to chance and that they are significant in terms of supporting the theory being investigated.
Research Hypothesis Examples of Independent and Dependent Variables
Research Hypothesis Example 1 The greater number of coal plants in a region (independent variable) increases water pollution (dependent variable). If you change the independent variable (building more coal factories), it will change the dependent variable (amount of water pollution).
Research Hypothesis Example 2 What is the effect of diet or regular soda (independent variable) on blood sugar levels (dependent variable)? If you change the independent variable (the type of soda you consume), it will change the dependent variable (blood sugar levels)
You should not ignore the importance of the above steps. The validity of your experiment and its results rely on a robust testable hypothesis. Developing a strong testable hypothesis has few advantages, it compels us to think intensely and specifically about the outcomes of a study. Consequently, it enables us to understand the implication of the question and the different variables involved in the study. Furthermore, it helps us to make precise predictions based on prior research. Hence, forming a hypothesis would be of great value to the research. Here are some good examples of testable hypotheses.
More importantly, you need to build a robust testable research hypothesis for your scientific experiments. A testable hypothesis is a hypothesis that can be proved or disproved as a result of experimentation.
Importance of a Testable Hypothesis
To devise and perform an experiment using scientific method, you need to make sure that your hypothesis is testable. To be considered testable, some essential criteria must be met:
- There must be a possibility to prove that the hypothesis is true.
- There must be a possibility to prove that the hypothesis is false.
- The results of the hypothesis must be reproducible.
Without these criteria, the hypothesis and the results will be vague. As a result, the experiment will not prove or disprove anything significant.
What are your experiences with building hypotheses for scientific experiments? What challenges did you face? How did you overcome these challenges? Please share your thoughts with us in the comments section.
Frequently Asked Questions
The steps to write a research hypothesis are: 1. Stating the problem: Ensure that the hypothesis defines the research problem 2. Writing a hypothesis as an 'if-then' statement: Include the action and the expected outcome of your study by following a ‘if-then’ structure. 3. Defining the variables: Define the variables as Dependent or Independent based on their dependency to other factors. 4. Scrutinizing the hypothesis: Identify the type of your hypothesis
Hypothesis testing is a statistical tool which is used to make inferences about a population data to draw conclusions for a particular hypothesis.
Hypothesis in statistics is a formal statement about the nature of a population within a structured framework of a statistical model. It is used to test an existing hypothesis by studying a population.
Research hypothesis is a statement that introduces a research question and proposes an expected result. It forms the basis of scientific experiments.
The different types of hypothesis in research are: • Null hypothesis: Null hypothesis is a negative statement to support the researcher’s findings that there is no relationship between two variables. • Alternate hypothesis: Alternate hypothesis predicts the relationship between the two variables of the study. • Directional hypothesis: Directional hypothesis specifies the expected direction to be followed to determine the relationship between variables. • Non-directional hypothesis: Non-directional hypothesis does not predict the exact direction or nature of the relationship between the two variables. • Simple hypothesis: Simple hypothesis predicts the relationship between a single dependent variable and a single independent variable. • Complex hypothesis: Complex hypothesis predicts the relationship between two or more independent and dependent variables. • Associative and casual hypothesis: Associative and casual hypothesis predicts the relationship between two or more independent and dependent variables. • Empirical hypothesis: Empirical hypothesis can be tested via experiments and observation. • Statistical hypothesis: A statistical hypothesis utilizes statistical models to draw conclusions about broader populations.

Wow! You really simplified your explanation that even dummies would find it easy to comprehend. Thank you so much.
Thanks a lot for your valuable guidance.
I enjoy reading the post. Hypotheses are actually an intrinsic part in a study. It bridges the research question and the methodology of the study.
Useful piece!
This is awesome.Wow.
It very interesting to read the topic, can you guide me any specific example of hypothesis process establish throw the Demand and supply of the specific product in market
Nicely explained
It is really a useful for me Kindly give some examples of hypothesis
It was a well explained content ,can you please give me an example with the null and alternative hypothesis illustrated
clear and concise. thanks.
So Good so Amazing
Good to learn
Thanks a lot for explaining to my level of understanding
Explained well and in simple terms. Quick read! Thank you
It awesome. It has really positioned me in my research project
Rate this article Cancel Reply
Your email address will not be published.
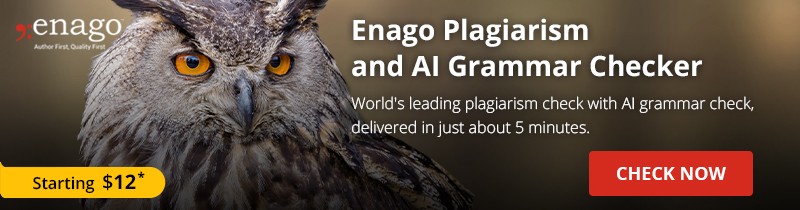
Enago Academy's Most Popular Articles
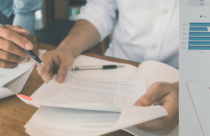
- Reporting Research
Choosing the Right Analytical Approach: Thematic analysis vs. content analysis for data interpretation
In research, choosing the right approach to understand data is crucial for deriving meaningful insights.…
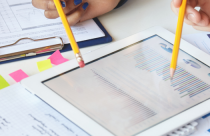
Comparing Cross Sectional and Longitudinal Studies: 5 steps for choosing the right approach
The process of choosing the right research design can put ourselves at the crossroads of…
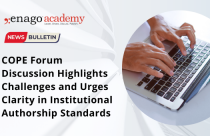
- Industry News
COPE Forum Discussion Highlights Challenges and Urges Clarity in Institutional Authorship Standards
The COPE forum discussion held in December 2023 initiated with a fundamental question — is…
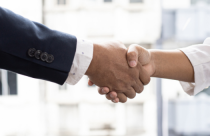
- Career Corner
Unlocking the Power of Networking in Academic Conferences
Embarking on your first academic conference experience? Fear not, we got you covered! Academic conferences…
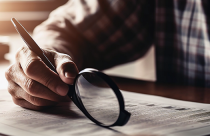
Research Recommendations – Guiding policy-makers for evidence-based decision making
Research recommendations play a crucial role in guiding scholars and researchers toward fruitful avenues of…
Choosing the Right Analytical Approach: Thematic analysis vs. content analysis for…
Comparing Cross Sectional and Longitudinal Studies: 5 steps for choosing the right…
How to Design Effective Research Questionnaires for Robust Findings

Sign-up to read more
Subscribe for free to get unrestricted access to all our resources on research writing and academic publishing including:
- 2000+ blog articles
- 50+ Webinars
- 10+ Expert podcasts
- 50+ Infographics
- 10+ Checklists
- Research Guides
We hate spam too. We promise to protect your privacy and never spam you.
I am looking for Editing/ Proofreading services for my manuscript Tentative date of next journal submission:
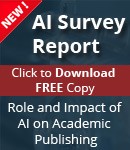
As a researcher, what do you consider most when choosing an image manipulation detector?
Have a language expert improve your writing
Run a free plagiarism check in 10 minutes, automatically generate references for free.
- Knowledge Base
- Methodology
- How to Write a Strong Hypothesis | Guide & Examples
How to Write a Strong Hypothesis | Guide & Examples
Published on 6 May 2022 by Shona McCombes .
A hypothesis is a statement that can be tested by scientific research. If you want to test a relationship between two or more variables, you need to write hypotheses before you start your experiment or data collection.
Table of contents
What is a hypothesis, developing a hypothesis (with example), hypothesis examples, frequently asked questions about writing hypotheses.
A hypothesis states your predictions about what your research will find. It is a tentative answer to your research question that has not yet been tested. For some research projects, you might have to write several hypotheses that address different aspects of your research question.
A hypothesis is not just a guess – it should be based on existing theories and knowledge. It also has to be testable, which means you can support or refute it through scientific research methods (such as experiments, observations, and statistical analysis of data).
Variables in hypotheses
Hypotheses propose a relationship between two or more variables . An independent variable is something the researcher changes or controls. A dependent variable is something the researcher observes and measures.
In this example, the independent variable is exposure to the sun – the assumed cause . The dependent variable is the level of happiness – the assumed effect .
Prevent plagiarism, run a free check.
Step 1: ask a question.
Writing a hypothesis begins with a research question that you want to answer. The question should be focused, specific, and researchable within the constraints of your project.
Step 2: Do some preliminary research
Your initial answer to the question should be based on what is already known about the topic. Look for theories and previous studies to help you form educated assumptions about what your research will find.
At this stage, you might construct a conceptual framework to identify which variables you will study and what you think the relationships are between them. Sometimes, you’ll have to operationalise more complex constructs.
Step 3: Formulate your hypothesis
Now you should have some idea of what you expect to find. Write your initial answer to the question in a clear, concise sentence.
Step 4: Refine your hypothesis
You need to make sure your hypothesis is specific and testable. There are various ways of phrasing a hypothesis, but all the terms you use should have clear definitions, and the hypothesis should contain:
- The relevant variables
- The specific group being studied
- The predicted outcome of the experiment or analysis
Step 5: Phrase your hypothesis in three ways
To identify the variables, you can write a simple prediction in if … then form. The first part of the sentence states the independent variable and the second part states the dependent variable.
In academic research, hypotheses are more commonly phrased in terms of correlations or effects, where you directly state the predicted relationship between variables.
If you are comparing two groups, the hypothesis can state what difference you expect to find between them.
Step 6. Write a null hypothesis
If your research involves statistical hypothesis testing , you will also have to write a null hypothesis. The null hypothesis is the default position that there is no association between the variables. The null hypothesis is written as H 0 , while the alternative hypothesis is H 1 or H a .
Research question | Hypothesis | Null hypothesis |
---|---|---|
What are the health benefits of eating an apple a day? | Increasing apple consumption in over-60s will result in decreasing frequency of doctor’s visits. | Increasing apple consumption in over-60s will have no effect on frequency of doctor’s visits. |
Which airlines have the most delays? | Low-cost airlines are more likely to have delays than premium airlines. | Low-cost and premium airlines are equally likely to have delays. |
Can flexible work arrangements improve job satisfaction? | Employees who have flexible working hours will report greater job satisfaction than employees who work fixed hours. | There is no relationship between working hour flexibility and job satisfaction. |
How effective is secondary school sex education at reducing teen pregnancies? | Teenagers who received sex education lessons throughout secondary school will have lower rates of unplanned pregnancy than teenagers who did not receive any sex education. | Secondary school sex education has no effect on teen pregnancy rates. |
What effect does daily use of social media have on the attention span of under-16s? | There is a negative correlation between time spent on social media and attention span in under-16s. | There is no relationship between social media use and attention span in under-16s. |
Hypothesis testing is a formal procedure for investigating our ideas about the world using statistics. It is used by scientists to test specific predictions, called hypotheses , by calculating how likely it is that a pattern or relationship between variables could have arisen by chance.
A hypothesis is not just a guess. It should be based on existing theories and knowledge. It also has to be testable, which means you can support or refute it through scientific research methods (such as experiments, observations, and statistical analysis of data).
A research hypothesis is your proposed answer to your research question. The research hypothesis usually includes an explanation (‘ x affects y because …’).
A statistical hypothesis, on the other hand, is a mathematical statement about a population parameter. Statistical hypotheses always come in pairs: the null and alternative hypotheses. In a well-designed study , the statistical hypotheses correspond logically to the research hypothesis.
Cite this Scribbr article
If you want to cite this source, you can copy and paste the citation or click the ‘Cite this Scribbr article’ button to automatically add the citation to our free Reference Generator.
McCombes, S. (2022, May 06). How to Write a Strong Hypothesis | Guide & Examples. Scribbr. Retrieved 11 June 2024, from https://www.scribbr.co.uk/research-methods/hypothesis-writing/
Is this article helpful?
Shona McCombes
Other students also liked, operationalisation | a guide with examples, pros & cons, what is a conceptual framework | tips & examples, a quick guide to experimental design | 5 steps & examples.
- How it works
Published by Nicolas at January 16th, 2024 , Revised On January 23, 2024
How To Write A Hypotheses – Guide For Students
The word “hypothesis” might conjure up images of scientists in white coats, but crafting a solid hypothesis is a crucial skill for students in any field. Whether you are analyzing Shakespeare’s sonnets or conducting a science experiment, a well-defined research hypothesis sets the stage for your dissertation or thesis and fuels your investigation.
Table of Contents
Writing a hypothesis is a crucial step in the research process. A hypothesis serves as the foundation of your research paper because it guides the direction of your study and provides a clear framework for investigation. But how to write a hypothesis? This blog will help you craft one. Let’s get started.
What Is A Hypothesis
A hypothesis is a clear and testable thesis statement or prediction that serves as the foundation of a research study. It is formulated based on existing knowledge, observations, and theoretical frameworks.
A hypothesis articulates the researcher’s expectations regarding the relationship between variables in a study.
Hypothesis Example
Students exposed to multimedia-enhanced teaching methods will demonstrate higher retention of information compared to those taught using traditional methods.
The formulation of a hypothesis is crucial for guiding the research process and providing a clear direction for data collection and analysis. A well-crafted research hypothesis not only makes the research purpose explicit but also sets the stage for drawing meaningful conclusions from the study’s findings.
What Is A Null Hypothesis And Alternative Hypothesis
There are two main types of hypotheses: the null hypothesis (H0) and the alternative hypothesis (H1 or Ha).
The null hypothesis posits that there is no significant effect or relationship, while the alternative hypothesis suggests the presence of a significant effect or relationship.
For example, in a study investigating the effect of a new drug on blood pressure, the null hypothesis might state that there is no difference in blood pressure between the control group (not receiving the drug) and the experimental group (receiving the drug). The alternative hypothesis, on the other hand, would propose that there is a significant difference in blood pressure between the two groups.
The literature review we write have:
- Precision and Clarity
- Zero Plagiarism
- High-level Encryption
- Authentic Sources
How To Write A Good Research Hypothesis
Writing a hypothesis involves a systematic process that guides your research and provides a clear and testable statement about the expected relationship between variables. Go through the MLA vs. APA guidelines before writing. Here are the steps to help you how to write a hypothesis:
Step 1: Identify The Research Topic
Clearly define the research topic or question that you want to investigate. Ensure that your research question is specific and focused, providing a clear direction for your study.
Step 2: Conduct A Literature Review
Review existing literature related to your research topic. A thorough literature review helps you understand what is already known in the field, identify gaps, and build a foundation for formulating your hypothesis.
Step 3: Define Variables
Identify the variables involved in your study. The independent variable is the factor you manipulate, and the dependent variable is the one you measure. Clearly define the characteristics or conditions you are studying.
Step 4: Establish The Relationship
Determine the expected relationship between the independent and dependent variables. Will a change in the independent variable lead to a change in the dependent variable? Specify whether you anticipate a positive, negative, or no relationship.
Step 5: Formulate The Null Hypothesis (H0)
The null hypothesis represents the default position, suggesting that there is no significant effect or relationship between the variables you are studying. It serves as the baseline to be tested against. The null hypothesis is often denoted as H0.
Step 6: Formulate The Alternative Hypothesis (H1 or Ha)
The alternative hypothesis articulates the researcher’s expectation about the existence of a significant effect or relationship. It is what you aim to support with your research paper . The alternative hypothesis is denoted as H1 or Ha.
For example, if your research topic is about the effect of a new fertilizer on plant growth:
- Null Hypothesis (H0): There is no significant difference in plant growth between plants treated with the traditional fertilizer and those treated with the new fertilizer.
- Alternative Hypothesis (H1): There is a significant difference in plant growth between plants treated with the traditional fertilizer and those treated with the new fertilizer.
Step 7: Ensure Testability And Specificity
Confirm that your research hypothesis is testable and can be empirically investigated. Ensure that it is specific, providing a clear and measurable statement that can be validated or refuted through data collection and analysis.
Hypothesis Examples
Does caffeine consumption affect reaction time? | There is a significant difference in reaction time between individuals who consume caffeine and those who do not. | There is no significant difference in reaction time between individuals who consume caffeine and those who do not. | There is a significant difference in reaction time between individuals who consume caffeine and those who do not. |
What is the impact of exercise on weight loss? | Increased exercise leads to a greater amount of weight loss. | Increased exercise has no impact on the amount of weight loss. | Increased exercise does not lead to a greater amount of weight loss. |
Is there a correlation between study hours and exam scores? | There is a positive correlation between the number of study hours and exam scores. | There is no correlation between the number of study hours and exam scores. | There is a negative correlation between the number of study hours and exam scores. |
How does temperature affect plant growth? – | Plants grow better in higher temperatures. | There is no effect of temperature on plant growth. | Plants grow better in lower temperatures. |
Can music improve concentration during work? | Listening to music enhances concentration and productivity. | Listening to music has no effect on concentration and productivity. | Listening to music impairs concentration and productivity. |
What Makes A Good Hypothesis
- Clear Statement: A hypothesis should be stated clearly and precisely. It should be easily understandable and convey the expected relationship between variables.
- Testability: A hypothesis must be testable through empirical observation or experimentation. This means that there should be a feasible way to collect data and assess whether the expected relationship holds true.
- Specificity: The research hypothesis should be specific in terms of the variables involved and the nature of the expected relationship. Vague or ambiguous hypotheses can lead to unclear research outcomes.
- Measurability: Variables in a hypothesis should be measurable, meaning they can be quantified or observed objectively. This ensures that the research can be conducted with precision.
- Falsifiability: A good research hypothesis should be falsifiable, meaning there should be a possibility of proving it wrong. This concept is fundamental to the scientific method, as hypotheses that cannot be tested or disproven lack scientific validity.
Frequently Asked Questions
How to write a hypothesis.
- Clearly state the research question.
- Identify the variables involved.
- Formulate a clear and testable prediction.
- Use specific and measurable terms.
- Align the hypothesis with the research question.
- Distinguish between the null hypothesis (no effect) and alternative hypothesis (expected effect).
- Ensure the hypothesis is falsifiable and subject to empirical testing.
How to write a hypothesis for a lab?
- Identify the purpose of the lab.
- Clearly state the relationship between variables.
- Use concise language and specific terms.
- Make the hypothesis testable through experimentation.
- Align with the lab’s objectives.
- Include an if-then statement to express the expected outcome.
- Ensure clarity and relevance to the experimental setup.
What Is A Null Hypothesis?
A null hypothesis is a statement suggesting no effect or relationship between variables in a research study. It serves as the default assumption, stating that any observed differences or effects are due to chance. Researchers aim to reject the null hypothesis based on statistical evidence to support their alternative hypothesis.
How to write a null hypothesis?
- State there is no effect, difference, or relationship between variables.
- Use clear and specific language.
- Frame it in a testable manner.
- Align with the research question.
- Specify parameters for statistical testing.
- Consider it as the default assumption to be tested and potentially rejected in favour of the alternative hypothesis.
What is the p-value of a hypothesis test?
The p-value in a hypothesis test represents the probability of obtaining observed results, or more extreme ones, if the null hypothesis is true. A lower p-value suggests stronger evidence against the null hypothesis, often leading to its rejection. Common significance thresholds include 0.05 or 0.01.
How to write a hypothesis in science?
- Clearly state the research question
- Identify the variables and their relationship.
- Formulate a testable and falsifiable prediction.
- Use specific, measurable terms.
- Distinguish between the null and alternative hypotheses.
- Ensure clarity and relevance to the scientific investigation.
How to write a hypothesis for a research proposal?
- Clearly define the research question.
- Identify variables and their expected relationship.
- Formulate a specific, testable hypothesis.
- Align the hypothesis with the proposal’s objectives.
- Clearly articulate the null hypothesis.
- Use concise language and measurable terms.
- Ensure the hypothesis aligns with the proposed research methodology.
How to write a good hypothesis psychology?
- Formulate a specific and testable prediction.
- Use precise and measurable terms.
- Align the hypothesis with psychological theories.
- Articulate the null hypothesis.
- Ensure the hypothesis guides empirical testing in psychological research.
You May Also Like
To cite a TED Talk in APA style, include speaker’s name, publication year, talk title, “TED Conferences,” and URL for clarity and accuracy.
Common topics in Botany papers include taxonomy, plant physiology, ecology and biodiversity, plant pathology, and genetics.
Introduction establishes context, states research question, and justifies study. The abstract is a concise summary of key paper elements.
Ready to place an order?
USEFUL LINKS
Learning resources, company details.
- How It Works
Automated page speed optimizations for fast site performance
Have a language expert improve your writing
Run a free plagiarism check in 10 minutes, generate accurate citations for free.
- Knowledge Base
- Null and Alternative Hypotheses | Definitions & Examples
Null & Alternative Hypotheses | Definitions, Templates & Examples
Published on May 6, 2022 by Shaun Turney . Revised on June 22, 2023.
The null and alternative hypotheses are two competing claims that researchers weigh evidence for and against using a statistical test :
- Null hypothesis ( H 0 ): There’s no effect in the population .
- Alternative hypothesis ( H a or H 1 ) : There’s an effect in the population.
Table of contents
Answering your research question with hypotheses, what is a null hypothesis, what is an alternative hypothesis, similarities and differences between null and alternative hypotheses, how to write null and alternative hypotheses, other interesting articles, frequently asked questions.
The null and alternative hypotheses offer competing answers to your research question . When the research question asks “Does the independent variable affect the dependent variable?”:
- The null hypothesis ( H 0 ) answers “No, there’s no effect in the population.”
- The alternative hypothesis ( H a ) answers “Yes, there is an effect in the population.”
The null and alternative are always claims about the population. That’s because the goal of hypothesis testing is to make inferences about a population based on a sample . Often, we infer whether there’s an effect in the population by looking at differences between groups or relationships between variables in the sample. It’s critical for your research to write strong hypotheses .
You can use a statistical test to decide whether the evidence favors the null or alternative hypothesis. Each type of statistical test comes with a specific way of phrasing the null and alternative hypothesis. However, the hypotheses can also be phrased in a general way that applies to any test.
Prevent plagiarism. Run a free check.
The null hypothesis is the claim that there’s no effect in the population.
If the sample provides enough evidence against the claim that there’s no effect in the population ( p ≤ α), then we can reject the null hypothesis . Otherwise, we fail to reject the null hypothesis.
Although “fail to reject” may sound awkward, it’s the only wording that statisticians accept . Be careful not to say you “prove” or “accept” the null hypothesis.
Null hypotheses often include phrases such as “no effect,” “no difference,” or “no relationship.” When written in mathematical terms, they always include an equality (usually =, but sometimes ≥ or ≤).
You can never know with complete certainty whether there is an effect in the population. Some percentage of the time, your inference about the population will be incorrect. When you incorrectly reject the null hypothesis, it’s called a type I error . When you incorrectly fail to reject it, it’s a type II error.
Examples of null hypotheses
The table below gives examples of research questions and null hypotheses. There’s always more than one way to answer a research question, but these null hypotheses can help you get started.
( ) | ||
Does tooth flossing affect the number of cavities? | Tooth flossing has on the number of cavities. | test: The mean number of cavities per person does not differ between the flossing group (µ ) and the non-flossing group (µ ) in the population; µ = µ . |
Does the amount of text highlighted in the textbook affect exam scores? | The amount of text highlighted in the textbook has on exam scores. | : There is no relationship between the amount of text highlighted and exam scores in the population; β = 0. |
Does daily meditation decrease the incidence of depression? | Daily meditation the incidence of depression.* | test: The proportion of people with depression in the daily-meditation group ( ) is greater than or equal to the no-meditation group ( ) in the population; ≥ . |
*Note that some researchers prefer to always write the null hypothesis in terms of “no effect” and “=”. It would be fine to say that daily meditation has no effect on the incidence of depression and p 1 = p 2 .
The alternative hypothesis ( H a ) is the other answer to your research question . It claims that there’s an effect in the population.
Often, your alternative hypothesis is the same as your research hypothesis. In other words, it’s the claim that you expect or hope will be true.
The alternative hypothesis is the complement to the null hypothesis. Null and alternative hypotheses are exhaustive, meaning that together they cover every possible outcome. They are also mutually exclusive, meaning that only one can be true at a time.
Alternative hypotheses often include phrases such as “an effect,” “a difference,” or “a relationship.” When alternative hypotheses are written in mathematical terms, they always include an inequality (usually ≠, but sometimes < or >). As with null hypotheses, there are many acceptable ways to phrase an alternative hypothesis.
Examples of alternative hypotheses
The table below gives examples of research questions and alternative hypotheses to help you get started with formulating your own.
Does tooth flossing affect the number of cavities? | Tooth flossing has an on the number of cavities. | test: The mean number of cavities per person differs between the flossing group (µ ) and the non-flossing group (µ ) in the population; µ ≠ µ . |
Does the amount of text highlighted in a textbook affect exam scores? | The amount of text highlighted in the textbook has an on exam scores. | : There is a relationship between the amount of text highlighted and exam scores in the population; β ≠ 0. |
Does daily meditation decrease the incidence of depression? | Daily meditation the incidence of depression. | test: The proportion of people with depression in the daily-meditation group ( ) is less than the no-meditation group ( ) in the population; < . |
Null and alternative hypotheses are similar in some ways:
- They’re both answers to the research question.
- They both make claims about the population.
- They’re both evaluated by statistical tests.
However, there are important differences between the two types of hypotheses, summarized in the following table.
A claim that there is in the population. | A claim that there is in the population. | |
| ||
Equality symbol (=, ≥, or ≤) | Inequality symbol (≠, <, or >) | |
Rejected | Supported | |
Failed to reject | Not supported |
To help you write your hypotheses, you can use the template sentences below. If you know which statistical test you’re going to use, you can use the test-specific template sentences. Otherwise, you can use the general template sentences.
General template sentences
The only thing you need to know to use these general template sentences are your dependent and independent variables. To write your research question, null hypothesis, and alternative hypothesis, fill in the following sentences with your variables:
Does independent variable affect dependent variable ?
- Null hypothesis ( H 0 ): Independent variable does not affect dependent variable.
- Alternative hypothesis ( H a ): Independent variable affects dependent variable.
Test-specific template sentences
Once you know the statistical test you’ll be using, you can write your hypotheses in a more precise and mathematical way specific to the test you chose. The table below provides template sentences for common statistical tests.
( ) | ||
test
with two groups | The mean dependent variable does not differ between group 1 (µ ) and group 2 (µ ) in the population; µ = µ . | The mean dependent variable differs between group 1 (µ ) and group 2 (µ ) in the population; µ ≠ µ . |
with three groups | The mean dependent variable does not differ between group 1 (µ ), group 2 (µ ), and group 3 (µ ) in the population; µ = µ = µ . | The mean dependent variable of group 1 (µ ), group 2 (µ ), and group 3 (µ ) are not all equal in the population. |
There is no correlation between independent variable and dependent variable in the population; ρ = 0. | There is a correlation between independent variable and dependent variable in the population; ρ ≠ 0. | |
There is no relationship between independent variable and dependent variable in the population; β = 0. | There is a relationship between independent variable and dependent variable in the population; β ≠ 0. | |
Two-proportions test | The dependent variable expressed as a proportion does not differ between group 1 ( ) and group 2 ( ) in the population; = . | The dependent variable expressed as a proportion differs between group 1 ( ) and group 2 ( ) in the population; ≠ . |
Note: The template sentences above assume that you’re performing one-tailed tests . One-tailed tests are appropriate for most studies.
If you want to know more about statistics , methodology , or research bias , make sure to check out some of our other articles with explanations and examples.
- Normal distribution
- Descriptive statistics
- Measures of central tendency
- Correlation coefficient
Methodology
- Cluster sampling
- Stratified sampling
- Types of interviews
- Cohort study
- Thematic analysis
Research bias
- Implicit bias
- Cognitive bias
- Survivorship bias
- Availability heuristic
- Nonresponse bias
- Regression to the mean
Hypothesis testing is a formal procedure for investigating our ideas about the world using statistics. It is used by scientists to test specific predictions, called hypotheses , by calculating how likely it is that a pattern or relationship between variables could have arisen by chance.
Null and alternative hypotheses are used in statistical hypothesis testing . The null hypothesis of a test always predicts no effect or no relationship between variables, while the alternative hypothesis states your research prediction of an effect or relationship.
The null hypothesis is often abbreviated as H 0 . When the null hypothesis is written using mathematical symbols, it always includes an equality symbol (usually =, but sometimes ≥ or ≤).
The alternative hypothesis is often abbreviated as H a or H 1 . When the alternative hypothesis is written using mathematical symbols, it always includes an inequality symbol (usually ≠, but sometimes < or >).
A research hypothesis is your proposed answer to your research question. The research hypothesis usually includes an explanation (“ x affects y because …”).
A statistical hypothesis, on the other hand, is a mathematical statement about a population parameter. Statistical hypotheses always come in pairs: the null and alternative hypotheses . In a well-designed study , the statistical hypotheses correspond logically to the research hypothesis.
Cite this Scribbr article
If you want to cite this source, you can copy and paste the citation or click the “Cite this Scribbr article” button to automatically add the citation to our free Citation Generator.
Turney, S. (2023, June 22). Null & Alternative Hypotheses | Definitions, Templates & Examples. Scribbr. Retrieved June 11, 2024, from https://www.scribbr.com/statistics/null-and-alternative-hypotheses/
Is this article helpful?
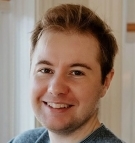
Shaun Turney
Other students also liked, inferential statistics | an easy introduction & examples, hypothesis testing | a step-by-step guide with easy examples, type i & type ii errors | differences, examples, visualizations, what is your plagiarism score.
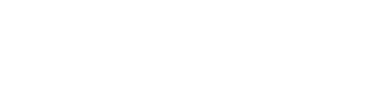
Verify originality of an essay
Get ideas for your paper
Find top study documents
How to Write a Hypothesis for a Research Paper: Best Hacks and Examples
Updated 11 Mar 2024
The narrative of a research study commences with the formulation of a question. Inquisitive researchers worldwide are constantly posing questions and crafting research hypotheses. The effectiveness of a paper’s conclusion hinges on the quality of every research element. From this guide, you’ll learn how to write a hypothesis for a research paper and find examples that can assist you in grasping the process of crafting a strong text. We aim to clarify the definition and characteristics of a research hypothesis and guide researchers in formulating one effectively.
What is a research hypothesis?
It is a tentative answer to a research question that has not been tested yet. It should be based on established theories and knowledge and be testable through scientific methods like experiments and data analysis.
To understand a hypothesis definition and its purpose, one must analyze a scientist's steps when doing research. To address a particular issue, the initial step involves identifying the research question, conducting a preliminary study, and then proceeding to answer the question by conducting experiments and analyzing the observed outcomes. Still, before embarking on the experimental phase, it’s essential to determine the expected results. At this stage, researchers make an informed estimation and formulate a supposition that they aim to confirm or disprove throughout their study.
The essential characteristics of a hypothesis
Now that you have a brief understanding of what a hypothesis in a research paper is, let’s examine its key defining characteristics that contribute to its effectiveness:
- Clear and specific: A good hypothesis is clear, concise, and specific in its formulation. It precisely states the relationship or expected outcome being investigated.
- Testable: It is testable, meaning it can be empirically examined through observations, experiments, or data analysis. Gathering evidence to support or refute the researcher’s guess should be possible.
- Grounded in existing knowledge: A good hypothesis in a research paper is based on existing theories, concepts, or empirical evidence. It demonstrates a solid understanding of the relevant literature and builds upon prior knowledge in the field.
- Falsifiable: It can be potentially proven false. This characteristic allows obtaining data that contradicts the primary assumption, enabling meaningful scientific inquiry.
- Logical and plausible: A supposition in research is logically reasoned and plausible. It should align with known facts and be supported by sound reasoning and evidence.
- Relevant and significant: It addresses a meaningful research question and has implications for the field. It should contribute to the existing knowledge base and have practical or theoretical significance.
- Limited in scope: It is focused and limited in scope. It should address a specific aspect or relationship rather than attempting to explain or predict everything in a broad context.
By embodying these characteristics, a good hypothesis provides a solid foundation for research, guiding the study’s design, data collection, and analysis, ultimately contributing to the generation of valuable scientific knowledge.
What are the sources for building a hypothesis?
There are several potential sources for developing a good research paper hypothesis. Let’s consider their details and examples:
- Scientific theories
Hypotheses can stem from existing scientific theories. Suppose we have an established theory in psychology that suggests a positive correlation between sleep quality and cognitive performance. Based on this theory, we can create a statement:
“If individuals experience better sleep quality, then their cognitive performance will improve compared to those with poorer sleep quality.”
- Previous studies and experiences
Observations from past studies and current experiences can contribute to formulating suppositions. Let’s say previous studies have shown that a particular herb has anti-inflammatory properties. Building upon this finding, we can formulate the following:
“If individuals consume the herb extract, then their inflammation levels will decrease compared to a control group.”
- Similarities among phenomena
Resemblances between different phenomena can inspire hypotheses. Consider a study investigating the effects of exercise on mood. Drawing an analogy from previous research showing that outdoor nature exposure improves mood, a scientist can formulate a guess:
“If individuals engage in outdoor exercise, then their mood will improve compared to those engaging in indoor exercise.”
- Empirical observations
Direct observations of phenomena or patterns in the real world can spark the development of ideas. Suppose a researcher observes that learners who study in a quiet environment tend to perform better on exams. This observation can lead to the next statement:
“If learners study in a quiet environment, then their exam scores will be higher compared to those who study in a noisy environment.”
Transform AI drafts with human editing!
Bring a human touch to your AI-generated drafts. Our expert editors refine your content for just $7/page.
Types of research hypotheses
They can be classified into one or more of the seven primary categories, depending on the nature of your investigation, a chosen research methodology , and anticipated findings. These categories are not mutually exclusive, meaning a single supposition can belong to multiple types.
- A simple hypothesis is based on the relationship between two variables: one independent and one dependent. Let’s see a hypothesis example:
“Increased study time leads to improved test scores.”
- A complex approach involves the relationship between numerous variables (more than two), e.g., two dependent variables and one independent, or vice versa.
“Both exercise frequency and diet quality have a combined effect on weight loss.”
- A null hypothesis suggests no relationship between variables.
“There is no significant difference in anxiety levels between Group A and Group B.”
- An alternative hypothesis is used alongside a null one, stating the opposite and asserting that only one of the two ideas can be true.
“The new drug treatment reduces symptoms of depression more effectively than the current standard treatment.”
- A logical approach relies on a relationship between variables based on reasoning or deduction, lacking actual data or evidence.
“If students receive regular feedback on their assignments, their academic performance will improve.”
- An empirical (“working”) hypothesis is currently being tested and relies on concrete data.
“Increasing the temperature will accelerate the rate of the chemical reaction.”
- A statistical approach involves testing a population sample and using statistical evidence to conclude about the whole population. This method tests only a portion of the population and generalizes based on existing data.
“Based on the sample data, there is a significant correlation between sleep duration and memory retention in the population.”
How to write a hypothesis for a research paper step-by-step
- Search for answers to your questions. Start by questioning the world around you, exploring why things are the way they are and what causes the phenomena you observe. Follow your curiosity and choose a research topic that genuinely interests you.
- Do preliminary research. Gather background information for your outline, depending on the scope of your research. This may involve reading books or performing quick web searches. Focus on gathering the necessary information to prove or disprove your idea.
- Determine variables. Define the independent and dependent variables for your research. Consider the factors you have control over and ensure they align with your experiment’s limitations.
- Formulate an if-then statement. Create your guess using an if-then format, illustrating the cause-and-effect relationship you intend to test. For example, “If we do morning exercise, then we’ll be healthier.”
- Gather supportive data. Conduct experiments to gather data that maintains your idea. Remember, even if your research disproves your supposition, it contributes to the scientific process.
- Write confidently. Finally, document your findings in your work for others to access. Writing a thesis requires distinct skills separate from conducting experiments.
Tips on creating a flawless research paper hypothesis
- Be realistic and feasible: Consider the practicality and limitations of your study. Ensure that your hypothesis is realistic and can be tested within the constraints of your available resources, time, and ethical considerations.
- Avoid value judgments: Be neutral and objective. Avoid including personal beliefs, value judgments, or subjective opinions. Stick to empirical statements based on evidence.
- Be concise: Aim for a concise and focused hypothesis. Avoid unnecessary complexity or unnecessary elaboration. Ensure it is succinctly stated in a single or a few sentences.
- Revise and refine: Continuously revise and refine your content as you gather more information and insights throughout your research process. Be open to modifying or adjusting your hypothesis based on new evidence or unexpected findings.

Some examples to inspire you
By following our guide and tips, you can easily create well-formed hypotheses. To help you get started, we have curated a list of research questions and relevant hypothesis examples.
Research question: Does regular exercise improve cognitive function in older adults?
Hypothesis: If older adults exercise regularly, their cognitive function will improve compared to sedentary ones.
Null hypothesis : No significant difference in cognitive function exists between older adults who exercise regularly and those who lead a sedentary lifestyle.
Research question: Does caffeine consumption affect sleep quality?
Hypothesis: If individuals consume high amounts of caffeine before bedtime, their sleep quality will be negatively impacted compared to those who consume low or no caffeine.
Null hypothesis : There is no significant difference in sleep quality between individuals who consume high amounts of caffeine before bedtime and those who consume low or no caffeine.
Was this helpful?
Thanks for your feedback.

Written by Steven Robinson
Steven Robinson is an academic writing expert with a degree in English literature. His expertise, patient approach, and support empower students to express ideas clearly. On EduBirdie's blog, he provides valuable writing guides on essays, research papers, and other intriguing topics. Enjoys chess in free time.
Related Blog Posts
Learn how to write an introduction for a research paper.
Though introduction to any writing is frequently associated with beginning, it's not that simple for an introduction to a research paper. Here you ...
How to Write a Research Question: Common Types and Winning Examples
The starting point for any investigation is a research question. Still, formulating valid and relevant questions can be challenging for many writer...
How to Structure a Research Paper: Meaningful Step-by-step Guide
Are you struggling to write paper that effectively contributes to your field? You’re at the right place! A properly structured research paper lets ...
Join our 150K of happy users
- Get original papers written according to your instructions
- Save time for what matters most
- Research article
- Open access
- Published: 25 June 2018
Identification of research hypotheses and new knowledge from scientific literature
- Matthew Shardlow 1 ,
- Riza Batista-Navarro 1 ,
- Paul Thompson 1 ,
- Raheel Nawaz 1 ,
- John McNaught 1 &
- Sophia Ananiadou ORCID: orcid.org/0000-0002-4097-9191 1
BMC Medical Informatics and Decision Making volume 18 , Article number: 46 ( 2018 ) Cite this article
10k Accesses
47 Citations
5 Altmetric
Metrics details
Text mining (TM) methods have been used extensively to extract relations and events from the literature. In addition, TM techniques have been used to extract various types or dimensions of interpretative information, known as Meta-Knowledge (MK), from the context of relations and events, e.g. negation, speculation, certainty and knowledge type. However, most existing methods have focussed on the extraction of individual dimensions of MK, without investigating how they can be combined to obtain even richer contextual information. In this paper, we describe a novel, supervised method to extract new MK dimensions that encode Research Hypotheses (an author’s intended knowledge gain) and New Knowledge (an author’s findings). The method incorporates various features, including a combination of simple MK dimensions.
We identify previously explored dimensions and then use a random forest to combine these with linguistic features into a classification model. To facilitate evaluation of the model, we have enriched two existing corpora annotated with relations and events, i.e., a subset of the GENIA-MK corpus and the EU-ADR corpus, by adding attributes to encode whether each relation or event corresponds to Research Hypothesis or New Knowledge. In the GENIA-MK corpus, these new attributes complement simpler MK dimensions that had previously been annotated.
We show that our approach is able to assign different types of MK dimensions to relations and events with a high degree of accuracy. Firstly, our method is able to improve upon the previously reported state of the art performance for an existing dimension, i.e., Knowledge Type. Secondly, we also demonstrate high F1-score in predicting the new dimensions of Research Hypothesis (GENIA: 0.914, EU-ADR 0.802) and New Knowledge (GENIA: 0.829, EU-ADR 0.836).
We have presented a novel approach for predicting New Knowledge and Research Hypothesis, which combines simple MK dimensions to achieve high F1-scores. The extraction of such information is valuable for a number of practical TM applications.
Peer Review reports
The goal of information extraction (IE) is to automatically distil and structure associations from unstructured text, with the aim of making it easier to locate information of interest in huge volumes of text. Within biomedical research articles, the textual context of a particular piece of knowledge often provides clues as to its current status along the ‘research journey’ timeline. Sentences (1)–(3) below exemplify a number of different points along the research timeline regarding the establishment of an association between Interleukin-17 (IL-17) and psoriasis . The association is firstly introduced in (1) as a hypothesis to be investigated. In (2), which is taken from the same paper [ 1 ], the putative association is backed up by initial experimental evidence. Sentence (3) comes from a paper published 10 years later [ 2 ], by which time the association is presented as widely accepted knowledge, presumably on the basis of many further positive experimental results.
(1) ‘To investigate the role of Interleukin-17 (IL-17) in the pathogenesis of psoriasis...’ (2) ‘These findings indicate that up-regulated expression of IL-17 might be involved in the pathogenesis of psoriasis.’ (3) ‘IL-17 is a critical factor in the pathogenesis of psoriasis and other inflammatory diseases.’
There is a strong need to identify different types of emerging knowledge, such as those shown in sentences (1–2), in a number of different scenarios. It has been shown elsewhere that incorporating this type of information improves the automated curation of biomedical networks and models [ 3 ].
In processing sentences (1)–(3) above, a typical IE system would firstly detect that Interleukin-17 and IL-17 are phrases that describe the same gene concept and that psoriasis represents a disease concept. Subsequently, the system would recognise that a specific association exists between these concepts. These associations may be binary relations between concepts, which encode that a specific type of association exists, or they may be events , which encode complex n -ary relations between a trigger word and multiple concepts or other events. Figure 1 shows the specific characteristics of both a relation and an event using the visualisation of the brat rapid annotation tool [ 4 ]. The output of the IE system would allow the location of all sentences within a large document collection, regardless of their varied phrasing, that explicitly mention the same association, or those mentioning other related types of associations, e.g., to find different genes that have an association with psoriasis. The structured associations that are extracted may subsequently be used as input to further stages of reasoning or data mining. Many IE systems would consider that sentences (1)–(3) each conveys exactly the same information, since most such systems only take into account the key information and not the wider context. Recently, however, there has been a trend towards detecting various aspects of contextual/interpretative information (such as negation or speculation) automatically [ 5 – 8 ].

An example of two sentences, one containing events and the other containing one relation. The first sentence shows two events. The first event in the sentence concerns the term ‘activation’ which is a type of positive regulation. The theme of this event is ‘NF-kappaB’, indicating that this protein is being activated. The next event in the sentence is centered around ‘dependent’ which is a type of positive regulation. This event has the cause ‘oxidative stress’ and its theme is the first event in the sentence. The example of a relation between two entities is, in contrast to the event, clearly much more simple. The relation indicates that NPTN is related to Schizophrenia in a relation that can be categorised as ‘Target-Disorder’
In this work, we focus on the automatic assignment of two interpretative dimensions to relations and events extracted by text mining tools. Specifically, we aim to determine whether or not each relation and event corresponds to a Research Hypothesis , as in sentence (1), or to New Knowledge , as in sentence (2). To the best of our knowledge, this work represents the first effort to apply a supervised approach to detect this type of information at such a fine-grained level.
We envisage that the recognition of these two interpretative dimensions is valuable in tasks where the discovery of emerging knowledge is important. To demonstrate the utility and portability of our method, we show that it can be used to enrich instances of both events and relations.
Related work
The task of automatically classifying knowledge contained within scientific literature according to its intended interpretation has long been recognised as an important step towards helping researchers to make sense of the information reported, and to allow important details to be located in an efficient manner. Previous work, focussing either on general scientific text or biomedical text, has aimed to assign interpretative information to continuous textual units, varying in granularity from segments of sentences to complete paragraphs, but most frequently concerning complete sentences. Specific aspects of interpretation addressed have included negation [ 5 ], speculation [ 6 – 8 ], general information content/rhetorical intent, e.g., background, methods, results, insights, etc. [ 9 – 12 ] and the distinction between novel information and background knowledge [ 13 , 14 ].
Despite the demonstrated utility of approaches such as the above, performing such classifications at the level of continuous text spans is not straightforward. For example, a single sentence or clause can introduce multiple types of information (e.g., several interactions or associations), each of which may have a different interpretation, in terms of speculation, negation, research novelty, etc. As can be seen from Fig. 1 , events and relations can structure and categorise the potentially complex information that is described in a continuous text span. Following on from the successful development of IE systems that are able to extract both gene-disease relations [ 15 – 17 ] and biomolecular events [ 18 , 19 ], there has been a growing interest in the task of assigning interpretative information to relations and events. However, given that a single sentence may contain mutiple events or relations, the challenge is to determine whether and how the interpretation of each of these structures is affected by the presence of particular words or phrases in the sentence that denote negation or speculation, etc.
IE systems are typically developed by applying supervised or semi-supervised methods to annotated corpora marked up with relations and events. There have been several efforts to manually enrich corpora with interpretative information, such that it is possible to train models to determine automatically how particular types of contxtual information in a sentence affect the interpretation of different events and relations. Most work on enriching relations and events has been focussed on one or two specific aspects of interpretation (e.g., negation [ 20 , 21 ] and/or speculation [ 22 , 23 ]). Subsequent work has shown that these types of information can be detected automatically [ 24 , 25 ].
In contrast, work on Meta-Knowledge (MK) captures a wider range of contextual information, integrating and building upon various aspects of the above-mentioned schemes to create a number of separate ‘dimensions’ of information, which are aimed at capturing subtle differences in the interpretation of relations and events. Domain-specific versions of the MK scheme have been created to enrich complex event structures in two different domain corpora, i.e., the ACE-MK corpus [ 26 ], which enriches the general domain news-related events of the ACE2005 corpus [ 27 ], and the GENIA-MK corpus [ 28 ], which adds MK to the biomolecular interactions captured as events in the GENIA event corpus [ 22 ]. Recent work has focussed on the detection of uncertainty around events in the GENIA-MK Corpus. Uncertainty was detected using a hybrid approach of rules and machine learning. The authors were able to show that incorporating uncertainty into a pathway modelling task led to an improvement in curator performance [ 3 ].
The GENIA-MK annotation scheme defines five distinct core dimensions of MK for events, each of which has a number of possible values, as shown in Fig. 2 :
Knowledge Type , which categorises the knowledge that the author wishes to express into one of: Observation, Investigation, Analysis, Method, Fact or Other.
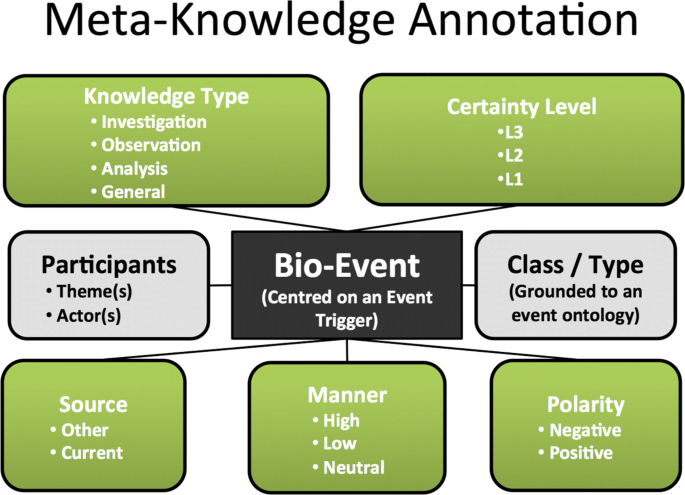
The GENIA-MK annotation scheme. There are five Meta-Knowledge dimensions introduced by Thompson et al. as well as two further hyperdimensions
Knowledge Source , which encodes whether the author presents the knowledge as part of their own work (Current), or whether it is referring to previous work (Other).
Polarity , which is set to Positive if the event took place, and to Negative if it is negated, i.e., it did not take place.
Manner , which denotes the event’s intensity, i.e., High, Low or Neutral.
Certainty Level or Uncertainty , which indicates how certain an event is. It may be certain (L3), probable (L2) or possible (L1).
These five dimensions are considered to be independent of one another, in that the value of one dimension does not affect the value of any other dimension. There may, however, be emergent correlations between the dimensions (i.e., an event with the MK value ’Knowledge Source=Other’ is more frequently negated), which occur due to the characteristics of the events. Previous work using the GENIA-MK corpus has demonstrated the feasibility of automatically recognising one or more of the MK dimensions [ 29 – 31 ]. In addition to the five core dimensions, Thompson et al. [ 28 ] introduced the notion of hyperdimensions , (i.e., New Knowledge and Hypothesis) which represent higher level dimensions of information whose values are determined according to specific combinations of values that are assigned to different core MK dimensions. These hyperdimensions are also represented in Fig. 2 . We build upon these approaches in our own work to develop novel techniques for the recognition of New Knowledge and Hypothesis, which take into account several of the core MK dimensions described above, as well as other features pertaining to the structure of the event and sentence.
Our work took as its starting point the MK hyperdimensions defined by Thompson et al. [ 28 ], since we are also interested in idenfifying relations and events that describe hypotheses or new knowledge. However, we found a number of issues with the original work on these hyperdimensions. Firstly, Thompson et al. [ 28 ] did not provide clear definitions for of ‘Hypothesis‘ and ‘New Knowledge’. In response, we have formulated concise definitions for each of them, as shown below. Secondly, by performing an analysis of events that takes into account these definitions, we found that it was not possible to reliably and consistently identify events that describe new knowledge or hypotheses based only on the values of the core MK dimensions. As such, we decided to carry out a new annotation effort to mark up both ‘Research Hypothesis’ and ‘New Knowledge’ as independent MK dimensions (i.e., their values do not necessarily have any dependence on the values of other core MK dimensons), and to explore supervised, rather than rule-based methods, to facilitate their automated recognition.
Annotation guidelines
The starting point for our novel annotation effort was our tightened definitions of Research Hypothesis and New Knowledge ; our initial definitions were refined throughout the process of annotation. As the definitions and guidelines evolved, we asked the annotators to revisit previously annotated documents in each new round. Our final definitions are presented below:
Research Hypothesis: A relation or event is considered as a Research Hypothesis if it encompasses a statement of the authors’ anticipated knowledge gain. This is shown in examples (1) and (2) in Table 1 . Table 1 Examples of sentences containing research hypotheses and new knowledge Full size table
New Knowledge: A relation or event is considered as New Knowledge if it corresponds to a novel research outcome resulting from the work the author is describing, as per examples (3) and (4) in Table 1 .
Whereas the value assigned to each of the core MK dimensions of Thompson et al. is completely independent of the values assigned to the other core dimensions, our newly introduced dimensions do not maintain this independence. Rather, Research Hypothesis and New Knowledge possess the property of mutual exclusivity, as an event or relation cannnot be simultaneously both a Research Hypothesis and New Knowledge. We chose to enrich two different corpora with attributes encoding Research Hypothesis and New Knowledge, i.e., a subset of the biomolecular interactions annotated as events in the GENIA-MK corpus [ 28 ], and the biomarker-relevant relations involving genes, diseases and treatments in the EU-ADR corpus [ 23 ]. Leveraging the previously-added core MK annotations in the GENIA-MK corpus, we explored how these can contribute to the accurate recognition of New Knowledge and Research Hypothesis. Specifically, we have introduced new approaches for predicting the values of the core Knowledge Type and Knowledge Source dimensions, demonstrating an improvement over the former state of the art for Knowledge Type. We subsequently use supervised methods to automatically detect New Knowledge and Research Hypothesis, incorporating the values of Knowledge Type, Knowledge Source and Uncertainty as features into the trained models.
The GENIA-MK corpus consists of one thousand MEDLINE abstracts on the subject of transcription factors in human blood cells, which have been annotated with a range of entities and events that provide detailed, structured information about various types of biomolecular interactions that are described in text. In the GENIA-MK corpus, values for all five core MK dimensions are already manually annotated for all of the 36,000 events. The MK annotation effort also involved the identification of ‘clue words’, i.e., words or phrases that provide evidence for the assignment of values for particular MK dimensions. For example, the word ‘suggest’ would be annotated as a clue both for Uncertainty and Knowledge Type, as it indicates that the information encoded in the event is stated based on a speculative analysis of results.
The EU-ADR corpus consists of three sets of 100 MEDLINE abstracts, each obtained using different PubMed queries aimed at retrieving abstracts that are likely to contain three specific types of relations (i.e., gene-disease, gene-drug and drug-disease), the former two of which can be important in discovering how different types of genetic information influence disease susceptibility and treatment response. The original annotation task involved identifying three types of entities, i.e., targets (proteins, genes and variants), diseases and drugs, together with relationships between these entity types, where these are present. In contrast to the richness of the event representations in the GENIA-MK corpus, each relation annotation in the EU-ADR corpus consists only of links between entities of two specific types. Relations were annotated in 159 of the 300 abstracts selected for inclusion in the corpus.
Annotation of new knowledge and research hypothesis
As an initial step of our work, subsets of GENIA-MK and EU-ADR were manually enriched with additional annotations, which identify those events or relations corresponding to Research Hypotheses or New Knowledge. Since high quality annotations are key to ensuring that accurate supervised models can be trained, we engaged with a number of experts and carried out an exploratory annotation exercise prior to the the final annotation effort, in order to ensure the highest possible inter-annotator agreement (IAA).
Initially, we worked with two domain experts, a text mining researcher and a medical professional. They added the novel MK annotations to events that had been automatically detected in sentences from full-text papers. We found, however, that there were some issues with this annotation set-up. Firstly, we found that events denoting Research Hypotheses and New Knowledge were very sparse in full papers. Secondly, we found that isolated sentences often provided insufficient context for annotators to determine accurately whether or not the event described new knowledge or a hypothesis. Finally, we found that errors in the automatically detected events were detracting the annotators’ attention from the task at hand. Based on these findings, we decided not to pursue this apporach, and instead focussed our anotation efforts on annotating Research Hypotheses and New Knowledge in abstracts containing gold-standard, expert-annotated events and relations, whose quality had previously been verified. Since abstracts also generally contain denser and more consolidated statements of New Knowledge and Research Hypotheses than full papers [ 32 ], we also expected that this approach would produce more useful training data.
We then employed two PhD students (both working in disciplines related to biological sciences) to carry out the next round of annotation work. We held regular meetings to discuss new annotations and provided feedback as necessary. A subset of the abstracts was doubly annotated by both annotators, allowing us to evaluate the annotation quality by calculating IAA using Cohen’s Kappa [ 33 ].
Table 2 , which shows IAA at three different points during the annotation process, illustrates a steady increase in IAA as time progressed and as more discussions were held, demonstrating a convergence towards a common understanding of the guidelines by the two annotators. We get a final agreement of above 0.8 on most dimensions, indicating a strong level of agreement [ 34 ]. Annotation of Research Hypothesis in the EU-ADR corpus achieved slightly lower agreement of 0.761, indicating moderate agreement between the annotators [ 34 ]. At the end of the annotation process, the annotators were asked to revisit their earlier annotations to make revisions based on their enhanced understanding of the guidelines. Remaining discrepancies were resolved by the lead author after consultation with both annotators.
Each annotator marked up 112 abstracts from the EU-ADR corpus (70 of which were doubly annotated), and 100 abstracts from the GENIA-MK corpus (50 of which were doubly annotated). This resulted in a total of 150 GENIA-MK abstracts and 159 EU-ADR abstracts annotated with New Knowledge and Research Hypothesis. Statistics on the final corpus are shown in Table 3 .
Baseline method for new knowledge and research hypothesis
Thompson et al. [ 28 ] suggest a method for detecting new knowledge and hypothesis based on automatic inferences from core MK values. Their inferences state that an event will be an instance of new knowledge if the Knowledge Source dimension is equal to ‘Current’ , the Uncertainty dimension is equal to ‘L3’ (equivalent to ‘Certain’ in our work, see below) and the Knowledge Type dimension is equal to either ‘Observation’ or ‘Analysis’ . Similarly, according to their inferences, an event will be an instance of Hypothesis if the Knowledge Type dimension is equal to ‘Analysis’ and Uncertainty is equal to either ‘L2’ or ‘L1’ (which are both equivalent to ‘Uncertain’ in our work, see below).
We use these automated inferences as a baseline for our techniques. To best reflect the work of Thompson et al. [ 28 ], we use their manually annotated values of Knowledge Type, Uncertainty and Knowledge Source for the GENIA-MK corpus. This allows us to compare our own work with previous efforts, as well as providing a lower bound for the performance of a rule based system, which we contrast with our supervised learning system, as introduced in the next section.
A supervised method for extracting new knowledge and research hypothesis
We took a supervised approach to annotating events with instances of our target dimensions of New Knowledge and Research Hypothesis. According to the previously mentioned intrinsic links to the core MK dimensions of Knowledge Source, Knowledge Type and Uncertainty, we incorporated the values of these dimensions as features that are used by our classifiers.
Uncertainty
For the Uncertainty dimension, we used an existing system [ 3 ]. Adopting their treatment of Uncertainty, we differ from Thompson et al. [ 28 ] as we use only have 2 levels (certain and uncertain), as opposed to their three levels (L3 = certain, L2 = probable and L1 = possible). Since our development of the original MK scheme, we have experimented and discussed different levels of granularity for this dimension with domain experts, and have concluded that the differences between the two different levels of uncertainty in our original scheme (i.e., L1 and L2) are often too subtle to be of benefit in practical scenarios. Therefore, it was decided to focus instead on the binary distinction between certainty and uncertainty.
Knowledge source
The Knowledge Source dimension distinguishes events that encode information originating from an author’s own work (Knowledge Source = Current), from those describing work from an alternative source (Knowledge Source = Other). Such information is relevant to the identification of New Knowledge, as a relation or event that corresponds to information reported in background literature definitely cannot be classed as New Knowledge. Attribution by citation is a well-established practice in the scientific literature. Citations can be expressed heterogeneously between documents, but are typically expressed homogeneously within a single document, or a collection of similarly-sourced documents. We used regular expressions to identify citations following the work of Miwa et al. [ 35 ], in conjunction with a set of clue expressions that aim to detect background knowledge in cases where no citation is given. These include statements such as ‘we previously showed…’ or ‘as seen in our former work’. Whereas Miwa et al. use a supervised learning method to detect Knowledge Source, we found that supervised learning approaches overfitted to the overwhelming majority class (Source =Current) in the GENIA-MK dataset. This meant that we suffered poor performance on unseen data, such as the EU-ADR corpus. To alleviate this, we simply used the regular expression feature as described above as an indicator of Knowledge Source being ‘Other’. A list of our regular expressions and clue expressions is made available as part of the Additional files .
Knowledge type
For Knowledge Type, we used an implementation of the random forest algorithm [ 36 ] from the WEKA library [ 37 ]. We used the standard parameters of the random forest in the WEKA implementation. We used ten-fold cross validation for all experiments, and results are reported as the macro-average across the ten folds. We treat the identification of Knowledge Type as a multi-class classification problem and we took a supervised approach to categorising relations and events in the two corpora according to the values of the Knowledge Type dimension. To facilitate this, we used the following seven types of features to generate information about each event from GENIA-MK and relation from EU-ADR:
Sentence features describing the sentence containing the relation or event.
Structural features, inspired by the structural differences of events.
Participant features, representing the participants in the relation or event.
Lexical features, capturing the presence of clue words.
Constituency features, corresponding to relationships between a clue and the relation or event, based on the output of a parser.
Dependency features, which capture relationships between a clue and the relation or event based on the dependency parse tree.
Parse tree features, which pertain to the structure of the dependency parse tree.
These features are further described in Table 4 . To generate these features, we made use of the GENIA Tagger [ 38 ] to obtain part-of-speech (POS) tags, and the Enju parser [ 39 ] to compute syntactic parse trees.
Research hypotheses and new knowledge
We followed a similar approach to predicting Research Hypothesis and New Knowledge values to that described above for the recognition of Knowledge Type. We used the same features and also a random forest classifier. We incorporated additional features encoding the Knowledge Source, Knowledge Type and Uncertainty of each relation and event.
Clue lists, developed by the authors, were used for the detection of Knowledge Type, Knowledge Source and Uncertainty. For the detection of New Knowledge and Hypothesis, a combination of clues for Knowledge Type, Knowledge Source and Uncertainty was used. The exact clue lists are available in the Additional files .
In this section, we present our experiments to detect the core Knowledge Type dimension, in which we determine the most appropriate feature subset to use, and also compare our approach to previous work. We then extend this approach to recognise New Knowledge and Research Hypothesis, and to evaluate our results in terms of precision Footnote 1 , recall , Footnote 2 and F1-score . Footnote 3
Our experiments to predict the correct values for the Knowledge Type dimension were carried out only using the events in the GENIA-MK corpus, given that Knowledge Type is only annotated in this corpus and not in EU-ADR. We performed an analysis of each feature subset to assess its impact on classifier performance, as shown in Table 5 . It was established that removing each of the participant, dependency and parse tree features individually leads to a small increase in F1-score. However, in subsequent experiments, we found that removing all three features does not lead to an additional increase in performance. We therefore used all feature subsets except for the participant features in subsequent experiments, as this gave us the best overall score. By observing the isolated performance of each feature subset, we also determined that the lexical and structural features are both significant individual contributors to the final classification score. In Table 6 , we compare the performance of our classifier in predicting each Knowledge Type value with the results obtained by the state-of-the-art method developed by Miwa et al. [ 31 ]. The results reveal that our approach achieves an increase in F1-score over Miwa et al. [ 31 ] by a minimum of 0.063 for the Other value, and a maximum of 0.113 for Method. We also see corresponding performance boosts in terms of precision and recall. Although we observe a small drop in recall for Fact and Method, this is offset by an increase in precision of 0.210 and 0.299, respectively.
To further investigate our improvement over Miwa et al., we swapped our classifier for an SVM, but used all the same features. The results of this are shown in Table 6 . This experiment allowed us to compare the performance of our features with the same classification algorithm (SVM), as used by Miwa et al. We note that using the SVM with our features leads to a similar, but slightly worse performance in terms of F1 score than Miwa et al. on all categories except for Analysis. However we do note an increase in Precision for certain categories (Method, Investigation, Analysis) and Recall for others (Observation, Analysis). As our features are tuned for performance with a Random Forest, this experiment demonstrates that different types of classifiers may require different feature sets to achieve optimal performance.
To further understand the impact of our feature categories, we analysed the correlation of each feature with each Knowledge Type value. This allowed us to determine the most informative features for each Knowlegde Type value, as displayed in Table 7 . In addition to this, we calculated the average rank of each feature across all Knowledge Type values. This measure shows us the most globally useful features. The top features according to average rank are displayed in Table 8 .
For the identification of New Knowledge and Research Hypothesis, we firstly performed 10-fold cross validation on each corpus (GENIA-MK and EU-ADR) and for each dimension of interest, yielding the results in Table 9 . In our presentation of results, we term the negative class for New Knowledge as “Other Knowledge”, as it covers a number of categories that we wish to exclude (e.g., background knowledge, irrelevant knowledge, supporting knowledge, etc.). We were able to classify Knowledge Type for relations in the EU-ADR corpus by setting the event and participant features to sensible static values — e.g., the number of participants in a relation is always 2.
In Table 5 , we observed the effects of each feature subset on the overall classification score for Knowledge Type. We found that the structural, lexical and sentence features had particularly strong contributions. The structural features encoded information about the structure of the event and were particularly useful for identifying events that participate in other events. The lexical features depended on the identification of clue words that appeared in the context of relations and events, which provided important evidence to determine the most appropriate MK values to assign. However, the usefulness of this feature is directly tied to the comprehensiveness of the list of clues associated with each MK value.
In addition to the feature analysis in Table 5 , we also provided additional analysis of each specific feature in Tables 7 and 8 . In line with the results from Table 5 , these tables demonstrate that the structural features were particularly informative for most classes, as well as the lexical, dependency and constituency features. It is interesting to note from Table 7 that no individual feature is particularly strongly correlated with each class label. This supports our ensemble approach and indicates that multiple feature sources are needed to attain a high classification accuracy. In addition, we can see that the correlations drop fairly quickly for all classes - indicating that not all features are used for every class. Finally, we can see that different features occur in each column (with some repetition), indicating that certain features were more useful for specific classes.
For the classification of New Knowledge and Hypothesis, we incorporated features denoting the existing meta-knowledge values of the event for Knowledge Source, Knowledge Type and Uncertainty. Knowledge Source indicates whether an event is current to the research in question, or whether it describes background work. This may be especially helpful for the detection of new knowledge, since it is clear that any background work cannot be classified as new knowledge. Knowledge Type classifies events as falling into one of six categories, i.e., Fact, Method, Analysis, Investigation, Observation or Other. The Investigation category may have contributed to the classification of Hypothetical events, whereas Observation and Analysis may have helped to contribute to the detection of New Knowledge events. The Fact, Method and Other categories could have helped the system to determine that events did not convey either hyperdimension. Finally, Uncertainty describes whether an author presented their results with confidence in their accuracy, or with some hedging (e.g., use of the words may, possibly, perhaps , etc.). This dimension could have helped to contribute to the classification of hypotheses (where an author states that an event may occur) and new knowledge, where we expect an author to be certain about their results.
We compared our results to those of Miwa et al. (2012) in Table 6 , where we showed a consistent improvement of precision, recall and F1-score across all categories. Their system used support vector machines (SVMs) for classification, with a set of features similar to our lexical and structural features. However, our work used an enhanced set of features as well as a random forest classifier, which is typically robust in high dimensional classification problems [ 36 ]. These two factors contributed to our system’s improved performance. Our system yielded an average increase in precision of 0.156, but only yielded an average increase in recall of 0.04. This implies that the use of a random forest and additional features mainly helped to ensure that the system returned results which are consistently correct. For both the ‘Fact’ and ‘Method’ Knowledge Type values, our system yielded a slight dip in recall compared to previous work. However, this was coupled with an increase in precision of 0.210 and 0.298, respectively.
To understand the relative contributions made by our switches in both feature set and type of classifier, compared to previous work, we analysed the performance of our system when using an SVM with our features instead of a Random Forest. We attained a similar performance to Miwa et al. using our feature set and SVM, although some values were lower than those reported by Miwa et al. This implies that our decision to use a different type of classifier to Miwa et al. (i.e., Random Forest instead of SVM) was the main reason behind our improved performance. Different feature sets are better suited to different types of classifiers, and our feature set was carefully selected (as documented in Table 5 ) to be performant with a Random Forest. Miwa et al.’s features were equally selected to perform well with an SVM. We have shown similar results in prior work for a task on detecting metaknowledge for negated bio-events [ 29 ], where we showed that tree-based methods, including the Random Forest, outperformed other techniques such as the SVM for detecting the negation dimension of metaknowledge.
We illustrated our results for the identification of the novel dimensions New Knowledge and Research Hypothesis in Table 9 . These showed strong performance across both corpora and association types (events and relations). The results for the GENIA-MK corpus (events) outperformed those for the EU-ADR corpus (relations). This was most likely due to the difference in size between the corpora. There are over ten times more annotated events in the subset of GENIA-MK that we annotated than relations in the subset of EU-ADR (6899 events vs. 622 relations). The fact that we annotated all of the 159 abstracts available in the EU-ADR corpus and only 150 abstracts from GENIA-MK indicates that event structures are more densely packed in GENIA-MK than relations in EU-ADR.
In particular, the EU-ADR corpus yielded a poor recall value for Research Hypotheses. There were only 38 examples of relations annotated as Research Hypothesis in the EU-ADR corpus. Our annotators reported that several relations occuring in hypothetical contexts appeared to have been missed by the original annotators of the EU-ADR corpus, which may be the cause of this sparsity. However, adding additional relations to the corpus was beyond the scope of the current work. The precision for the prediction of Research Hypothesis in the EU-ADR corpus was 1.00, indicating that of those relations automatically classified as Research Hypothesis, all were indeed Research Hypotheses (i.e., there were no false positives). It is usually the case in minority class situations that a classifier will tend towards classifying instances as the majority class (i.e., favouring false negatives over false positives), so this result is expected. We chose not to perform subsampling of the majority class, as the density of Research Hypotheses or New Knowledge in our training data is reflective of the density we would expect in other biomedical abstracts.
Our corpus has focussed on identifying Research Hypotheses and New Knowledge in biomedical abstracts. However, it has been shown elsewhere that full texts contain more information than abstracts alone [ 40 ]. Whilst our future goal is to additionally facilitate the recognition of New Knowledge and Research Hypothesis in full papers, our decision to focus initially on abstracts was motivated by the findings of our earlier rounds of annotation. These initial annotation efforts revealed that the density of the types of MK that form the focus of the current paper are very low in full papers and are consequently difficult for annotators to reliably identify. Therefore we chose to use abstracts, where the density was higher, since the availability of as many examples as possible of relevant MK was important for the development of our methods. We noted that abstracts fairly consistently mention the main Research Hypotheses and New Knowledge outcomes from a paper. However, further information may be available in the full paper that has not been mentioned in the abstract. To access this information we will need to further adapt our techniques and develop annotated corpora of full papers — this is left for future work.
Error analysis
Finally, we present an analysis of some common errors that our system makes and strategies for overcoming these in future work. In the following sentence, the event centred on “regulation” was marked as Non-Hypothetical by the annotators, but our system recognised it as a Hypothetical event.
To continue our investigation of the cellular events that occur following human CMV (HCMV) infection, we focused on the regulation of cellular activation following viral binding to human monocytes.
| E1 |
| regulation |
| activation following viral binding |
| N/A |
| focused |
It is likely that this event was marked as a hypothesis by the system because of the words ‘investigation’ and ‘focused’ that occur before it. However in this case, the main hypothesis that the annotators have marked is on the event centred on ‘occur’ preceding the event centred around ‘focused’. To overcome this in future work, we could implement a classification strategy that takes into account MK information that has already been assigned to other events that occur in the context of the focussed event. A conditional random field or deep learning model could be used for sequence labelling to accomplish this.
The second error, which concerns the event centred on “effects” in the following sentence, was marked as Hypothetical by our annotators, but was classified as Non-Hypothetical by our system.
MATERIAL AND METHODS: In the present study, we analyzed the effects of CyA, aspirin, and indomethacin \(\dots \)
| E2 |
| effects |
| Cya, aspirin, and indomethacin |
| N/A |
| present study |
This event is clearly stating the subject of the authors’ investigation, and so should be marked as hypothesis. It is likely that our system was confused by the preceding section heading, which led it to believe that this was part of the background or methods, and not a statement of the authors’ intended research goals. To overcome this, we could identify these section headings automatically and either exclude them from the text to be analysed, or use them as extra features in our classification scheme.
In our third example error, the event in the sentence below is centred on the phrase “result in decreased”. The event was marked as new knowledge by the annotators, but the system was not able to recognise it as such.
Down-regulation of MCP-1 expression by aspirin may result in decreased recruitment of monocytes into the arterial intima beneath stressed EC.
| E3 |
| result in decreased |
| recruitment of monocytes |
| Down-regulation of MCP-1 expression |
by aspirin | |
| N/A |
We believe that the cause of this classification errors is the unusual event trigger - the majority of events only have a single verb as their trigger. To help the system to better determine cases in which such events denote new knowledge, it would be necessary to further increase our corpus size, such that the training set includes a wider variety of trigger types. A further factor affecting the inability of the system to determine the new knowledge classification may have been be the lack of an appropriate new knowledge clue. In this case, the annotators most likely determined this as an example of new knowledge due to information from the wider context of the discourse. We could improve our classifier by looking for clues in a wider window, or by looking for discourse clues that might indicate that the author is drawing their conclusions.
The final example below concerns an event (centred on the verb “enhanced”), which was marked as ‘other knowledge’ by the annotators, but which the system determined to be an example of new knowledge.
Taken together, these data indicate that the unexpected expression of megakaryocytic genes is a specific property of immortalized cells that cannot be explained only by enhanced expression of Spi-1 and/or Fli-1 genes
| E4 |
| expression |
| megakaryotic genes |
| N/A |
| indicate |
| E5 |
| enhanced |
| expression of Spi-1 and… |
| E4 |
| N/A |
In this example, the event is somewhat problematic as regards the assignment of MK. Although it is clear both that the sentence is a concluding statement, and that there is some new knowledge contained within it, the annotators chose not to mark the event with the trigger “enhanced” as new knowledge, indicating that they did not consider it to convey the main aspect of new knowledge in this sentence. Interestingly, however, both annotators agreed with the system that the event centred on the first instance of “expression” should be marked as an instance of new knowledge. The presence of the clue ‘indicate’ may be affecting the system’s classification decision in both cases. A human annotator can distinguish that indicate is most relevant to ‘expression’, rather than ‘enhanced’, whereas our system was unable to make this distinction.
Conclusions
We have presented a novel application of text mining techniques for the discovery of Research Hypotheses and New Knowledge at the level of events and relations. This constitutes the first study into the application of supervised methods to assign these interpretative aspects at such a fine-grained level. We firstly showed that by applying a Random Forest classifier using a new feature set, we were able to achieve a better performance than previous efforts in detecting Knowledge Type. We subsequently showed that the core MK dimensions of Knowledge Type, Knowledge Source and Uncertainty could feed into the training of classifiers that can predict whether events and relations represent Research Hypotheses and New Knowledge, with a high degree of accuracy. Our techniques can be incorporated into a system that allows researchers to quickly filter information contained within the abstracts of research articles, as shown in previous literature [ 3 ]. Our methods generally favour precision on the positive class (i.e., Research Hypothesis or New Knowledge). Specifically, we attain a precision of between 0.863 and 1.00 on all of the corpus experiments. This demonstrates that our approach is successful in avoiding the identification of false positives, thus allowing researchers to be confident that instances of Research Hypothesis or New Knowledge identified by our method will usually be correct.
the proportion of results returned by the system which are correct.
the proportion of correct results returned by the system as a fraction of all the correct results that should have been found.
the balanced harmonic mean between precision and recall, providing a single overall measure of performance.
Abbreviations
Adverse Drug Reaction
F1 Score (The harmonic mean between Precision and Recall)
Information Extraction
Inter-Annotator Agreement
Meta-Knowledge
Support Vector Machine
Text Mining
Jiawen L, Dongsheng L, Zhijian T. The expression of interleukin-17, interferon-gamma, and macrophage inflammatory protein-3 alpha mRNA in patients with psoriasis vulgaris. J Huazhong University Sci Technol [Med Sci]. 2004; 24(3):294–6. https://doi.org/10.1007/BF02832018 .
Article Google Scholar
Scharffetter-Kochanek K, Singh K, Tasdogan A, Wlaschek M, Gatzka M, Hainzl A, Peters T. Reduction of CD18 promotes expansion of inflammatory gd T cells collaborating with CD4 T cells in chronic murine psoriasiform dermatitis. J Immunol. 2013; 191:5477–88. https://doi.org/10.4049/jimmunol.1300976 .
Article PubMed CAS Google Scholar
Zerva C, Batista-Navarro R, Day P, Ananiadou S. Using uncertainty to link and rank evidence from biomedical literature for model curation. Bioinformatics. btx466. https://doi.org/10.1093/bioinformatics/btx466 .
Stenetorp P, Pyysalo S, Topić G, Ohta T, Ananiadou S, Tsujii J. BRAT: a web-based tool for NLP-assisted text annotation. In: Proceedings of the Demonstrations at the 13th Conference of the European Chapter of the Association for Computational Linguistics. Association for Computational Linguistics: 2012. p. 102–107.
Agarwal S, Yu H, Kohane I. BioNØT: A searchable database of biomedical negated sentences. BMC Bioinformatics. 2011; 12(1):420. https://doi.org/10.1186/1471-2105-12-420 .
Article PubMed PubMed Central Google Scholar
Medlock B, Briscoe T. Weakly supervised learning for hedge classification in scientific literature. In: Proceedings of the 45th Annual Meeting of the Association of Computational Linguistics. Prague, Czech Republic: Association for Computational Linguistics: 2007. p. 992–9. http://www.aclweb.org/anthology/P07-1125 .
Google Scholar
Vincze V, Szarvas G, Farkas R, Móra G, Csirik J. The BioScope corpus: biomedical texts annotated for uncertainty, negation and their scopes. BMC Bioinformatics. 2008; 9(11):1–9.
Malhotra A, Younesi E, Gurulingappa H, Hofmann-Apitius M. ‘HypothesisFinder:’ a strategy for the detection of speculative statements in scientific text. PLOS Comput Biol. 2013; 9(7):1–10. https://doi.org/10.1371/journal.pcbi.1003117 .
Article CAS Google Scholar
Ruch P, Boyer C, Chichester C, Tbahriti I, Geissbühler A, Fabry P, Gobeill J, Pillet V, Rebholz-Schuhmann D, Lovis C, et al. Using argumentation to extract key sentences from biomedical abstracts. Int J Med Inform. 2007; 76(2):195–200.
Article PubMed Google Scholar
Teufel S, Carletta J, Moens M. An annotation scheme for discourse-level argumentation in research articles. In: Proceedings of the Ninth Conference on European Chapter of the Association for Computational Linguistics. EACL ’99. Stroudsburg: Association for Computational Linguistics: 1999. p. 110–7. https://doi.org/10.3115/977035.977051 .
Mizuta Y, Collier N. Zone identification in biology articles as a basis for information extraction. In: Proceedings of the International Joint Workshop on Natural Language Processing in Biomedicine and Its Applications. JNLPBA ’04. Stroudsburg: Association for Computational Linguistics: 2004. p. 29–35. http://dl.acm.org/citation.cfm?id=1567594.1567600 .
Burns G, Dasigi P, de Waard A, Hovy EH. Automated detection of discourse segment and experimental types from the text of cancer pathway results sections. Database. 2016; 2016:122. https://doi.org/10.1093/database/baw122 .
Liakata M, Saha S, Dobnik S, Batchelor C, Rebholz-Schuhmann D. Automatic recognition of conceptualization zones in scientific articles and two life science applications. Bioinformatics. 2012; 28(7):991. https://doi.org/10.1093/bioinformatics/bts071 .
Article PubMed PubMed Central CAS Google Scholar
Simsek D, Buckingham Shum S, Sandor A, De Liddo A, Ferguson R. Xip dashboard: visual analytics from automated rhetorical parsing of scientific metadiscourse. In: 1st International Workshop on Discourse-Centric Learning Analytics. Leuven: 2013.
Bundschus M, Dejori M, Stetter M, Tresp V, Kriegel HP. Extraction of semantic biomedical relations from text using conditional random fields. BMC Bioinformatics. 2008; 9(1):207.
Bravo A, Piñero J, Queralt-Rosinach N, Rautschka LIM. Furlong: Extraction of relations between genes and diseases from text and large-scale data analysis: implications for translational research. BMC Bioinformatics. 2015; 16(1):55.
Verspoor KM, Heo EG, Kang KY, Song M. Establishing a baseline for literature mining human genetic variants and their relationships to disease cohorts. BMC Med Inf Decis Mak. 2016; 16(1):68.
Nedellec C. Learning language in logic-genic interaction extraction challenge. In: Proceedings of the ICML-2005 Workshop on Learning Language in Logic (LLL05): 2005. p. 31–7.
Kim JD, Pyysalo S, Ohta T, Bossy R, Nguyen N, Tsujii J. Overview of BioNLP shared task 2011. In: Proceedings of the BioNLP Shared Task 2011 Workshop. Portland: Association for Computational Linguistics: 2011. p. 1–6.
Pyysalo S, Ginter F, Heimonen J, Björne F, Boberg F, Järvinen F, Salakoski T. BioInfer: a corpus for information extraction in the biomedical domain. BMC Bioinformatics. 2007; 8(1):50.
Sanchez-Graillet O, Poesio M. Negation of protein—protein interactions: analysis and extraction. Bioinformatics. 2007; 23(13):424. https://doi.org/10.1093/bioinformatics/btm184 .
Kim JD, Ohta T, Tsujii J. Corpus annotation for mining biomedical events from literature. BMC Bioinformatics. 2008; 9(1):1–25.
Van Mulligen EM, Fourrier-Reglat A, Gurwitz D, Molokhia M, Nieto A, Trifiro G, Kors JA, Furlong LI. The EU-ADR corpus: annotated drugs, diseases, targets, and their relationships. J Biomed Inform. 2012; 45(5):879–84.
Björne J, Ginter F, Salakoski T. University of Turku in the BioNLP’11 shared task. BMC Bioinformatics. 2012; 13(11):4.
Kilicoglu H, Bergler S. Biological event composition. BMC Bioinformatics. 2012; 13(11):7.
Thompson P, Nawaz R, McNaught J, Ananiadou S. Enriching news events with meta-knowledge information. Lang Resour Eval. 2016:1–30. https://doi.org/10.1007/s10579-016-9344-9 .
Walker C, Strassel S, Medero J, Maeda K. ACE 2005 multilingual training corpus. Philadelphia: Linguistic Data Consortium; 2006.
Thompson P, Nawaz R, McNaught J, Ananiadou S. Enriching a biomedical event corpus with meta-knowledge annotation. BMC Bioinformatics. 2011; 12(1):1–18.
Nawaz R, Thompson P, Ananiadou S. Negated BioEvents: Analysis and identification. BMC Bioinformatics. 2013; 14(1):14. https://doi.org/10.1186/1471-2105-14-14 .
Nawaz R, Thompson P, Ananiadou S. Something old, something new: identifying knowledge source in bio-events. Int J Comput Linguist Appl. 2013; 4(1):129–44.
Miwa M, Thompson P, McNaught J, Kell DB, Ananiadou S. Extracting semantically enriched events from biomedical literature. BMC Bioinformatics. 2012; 13:108. https://doi.org/10.1186/1471-2105-13-108 . Highly Accessed.
Nawaz R, Thompson P, Ananiadou S. Meta-knowledge annotation at the event level: Comparison between abstracts and full papers. In: Proceedings of the Third Workshop on Building and Evaluating Resources for Biomedical Text Mining (BioTxtM 2012): 2012. p. 24–31.
Cohen J. A coefficient of agreement for nominal scales. Educ Psychol Meas. 1960; 20(1):37–46. https://doi.org/10.1177/001316446002000104 .
McHugh ML. Interrater reliability: the kappa statistic. Biochemia medica. 2012; 22(3):276–82.
Miwa M, Sætre R, Kim JD, Tsujii J. Event extraction with complex event classification using rich features. J Bioinforma Comput Biol. 2010; 8(01):131–46.
Breiman L. Random forests. Machine Learning. 2001; 45(1):5–32.
Hall M, Frank E, Holmes G, Pfahringer B, Reutemann P, Witten IH. The WEKA data mining software: An update. SIGKDD Explor Newsl. 2009; 11(1):10–18. https://doi.org/10.1145/1656274.1656278 .
Tsuruoka Y, Tateishi Y, Kim JD, Ohta T, McNaught J, Ananiadou S, Tsujii J. Developing a robust part-of-speech tagger for biomedical text. Berlin, Heidelberg: Springer; 2005, pp. 382–92. Advances in Informatics: 10th Panhellenic Conference on Informatics, PCI 2005, Volas, Greece, November 11-13, 2005.
Book Google Scholar
Miyao Y, Tsujii J. Feature forest models for probabilistic HPSG parsing. Comput Linguist. 2008; 34(1):35–80. https://doi.org/10.1162/coli.2008.34.1.35 .
Schuemie MJ, Weeber M, Schijvenaars BJA, van Mulligen EM, van der Eijk CC, Jelier R, Mons B, Kors JA. Distribution of information in biomedical abstracts and full-text publications. Bioinformatics. 2004; 20(16):2597–604. https://doi.org/10.1093/bioinformatics/bth291 .
Download references
Acknowledgements
The authors wish to thank the annotators involved in creating the dataset for this paper, without whom this research would not have been possible. Out thanks also go to the reviewers for their considered feedback on our research.
The authors of this work were funded by the European Commission (an Open Mining Infrastructure for Text and Data. OpenMinTeD. Grant: 654021), the Medical Research Council (Manchester Molecular Pathology Innovation Centre. MMPathIC Grant: MR/N00583X/1) and the Biotechnology and Biological Sciences Research Council (Enriching Metabolic PATHwaY models with evidence from the literature. EMPATHY. Grant: BB/M006891/1). The funders played no part in either the design of the study or the collection, analysis, and interpretation of data, or in writing the manuscript.
Availability of data and materials
The datasets generated and analysed during the current study are available as Additional files to this paper.
Author information
Authors and affiliations.
National Centre for Text Mining, University of Manchester, Manchester, UK
Matthew Shardlow, Riza Batista-Navarro, Paul Thompson, Raheel Nawaz, John McNaught & Sophia Ananiadou
You can also search for this author in PubMed Google Scholar
Contributions
MS ran the principal experiments, performed the analysis of the results and participated in authoring the paper. RB helped with the design of the experiments and authoring the paper. PT contributed work on the preparation of the EU-ADR corpus as well as participating in the authorship of the paper. RN contributed to the experimental design, guidelines for the annotators and participated in the authorship of the paper. JM and SA jointly supervised the research and participated in authoring the paper. All authors read and approved the final version of this manuscript prior to publication.
Corresponding author
Correspondence to Sophia Ananiadou .
Ethics declarations
Ethics approval and consent to participate.
No ethics approval was required for any element of this study.
Consent for publication
Not Applicable.
Competing interests
The authors declare that they have no competing interests.
Publisher’s Note
Springer Nature remains neutral with regard to jurisdictional claims in published maps and institutional affiliations.
Additional files
Additional file 1.
The annotation guidelines that were given to annotators for reference. (PDF 830 kb)
Additional file 2
A table providing an in depth description of each feature. (PDF 32 kb)
Additional file 3
Read me documentation explaining the structure of the clue files. (TXT 4 kb)
Additional file 4
The clues used to detect the Analysis component of the Knowledge Type meta-knowledge dimension. (FILE 3 kb)
Additional file 5
The clues used to detect the Fact component of the Knowledge Type meta-knowledge dimension. (FILE 4 kb)
Additional file 6
The clues used to detect the Investigation component of the Knowledge Type meta-knowledge dimension. (FILE 2 kb)
Additional file 7
The clues used to detect the Method component of the Knowledge Type meta-knowledge dimension. (FILE 4 kb)
Additional file 8
The clues used to detect the Observation component of the Knowledge Type meta-knowledge dimension. (FILE 4 kb)
Additional file 9
The clues used to detect the Other component of the Knowledge Source meta-knowledge dimension. (FILE 1 kb)
Additional file 10
The clues used to detect the Uncertain component of the Certainty Level meta-knowledge dimension. (FILE 4 kb)
Rights and permissions
Open Access This article is distributed under the terms of the Creative Commons Attribution 4.0 International License ( http://creativecommons.org/licenses/by/4.0/ ), which permits unrestricted use, distribution, and reproduction in any medium, provided you give appropriate credit to the original author(s) and the source, provide a link to the Creative Commons license, and indicate if changes were made. The Creative Commons Public Domain Dedication waiver ( http://creativecommons.org/publicdomain/zero/1.0/ ) applies to the data made available in this article, unless otherwise stated.
Reprints and permissions
About this article
Cite this article.
Shardlow, M., Batista-Navarro, R., Thompson, P. et al. Identification of research hypotheses and new knowledge from scientific literature. BMC Med Inform Decis Mak 18 , 46 (2018). https://doi.org/10.1186/s12911-018-0639-1
Download citation
Received : 10 August 2017
Accepted : 11 June 2018
Published : 25 June 2018
DOI : https://doi.org/10.1186/s12911-018-0639-1
Share this article
Anyone you share the following link with will be able to read this content:
Sorry, a shareable link is not currently available for this article.
Provided by the Springer Nature SharedIt content-sharing initiative
- Text mining
- Meta-knowledge
- New knowledge
BMC Medical Informatics and Decision Making
ISSN: 1472-6947
- General enquiries: [email protected]
- USC Libraries
- Research Guides
Organizing Your Social Sciences Research Paper
- The Research Problem/Question
- Purpose of Guide
- Design Flaws to Avoid
- Independent and Dependent Variables
- Glossary of Research Terms
- Reading Research Effectively
- Narrowing a Topic Idea
- Broadening a Topic Idea
- Extending the Timeliness of a Topic Idea
- Academic Writing Style
- Applying Critical Thinking
- Choosing a Title
- Making an Outline
- Paragraph Development
- Research Process Video Series
- Executive Summary
- The C.A.R.S. Model
- Background Information
- Theoretical Framework
- Citation Tracking
- Content Alert Services
- Evaluating Sources
- Primary Sources
- Secondary Sources
- Tiertiary Sources
- Scholarly vs. Popular Publications
- Qualitative Methods
- Quantitative Methods
- Insiderness
- Using Non-Textual Elements
- Limitations of the Study
- Common Grammar Mistakes
- Writing Concisely
- Avoiding Plagiarism
- Footnotes or Endnotes?
- Further Readings
- Generative AI and Writing
- USC Libraries Tutorials and Other Guides
- Bibliography
A research problem is a definite or clear expression [statement] about an area of concern, a condition to be improved upon, a difficulty to be eliminated, or a troubling question that exists in scholarly literature, in theory, or within existing practice that points to a need for meaningful understanding and deliberate investigation. A research problem does not state how to do something, offer a vague or broad proposition, or present a value question. In the social and behavioral sciences, studies are most often framed around examining a problem that needs to be understood and resolved in order to improve society and the human condition.
Bryman, Alan. “The Research Question in Social Research: What is its Role?” International Journal of Social Research Methodology 10 (2007): 5-20; Guba, Egon G., and Yvonna S. Lincoln. “Competing Paradigms in Qualitative Research.” In Handbook of Qualitative Research . Norman K. Denzin and Yvonna S. Lincoln, editors. (Thousand Oaks, CA: Sage, 1994), pp. 105-117; Pardede, Parlindungan. “Identifying and Formulating the Research Problem." Research in ELT: Module 4 (October 2018): 1-13; Li, Yanmei, and Sumei Zhang. "Identifying the Research Problem." In Applied Research Methods in Urban and Regional Planning . (Cham, Switzerland: Springer International Publishing, 2022), pp. 13-21.
Importance of...
The purpose of a problem statement is to:
- Introduce the reader to the importance of the topic being studied . The reader is oriented to the significance of the study.
- Anchors the research questions, hypotheses, or assumptions to follow . It offers a concise statement about the purpose of your paper.
- Place the topic into a particular context that defines the parameters of what is to be investigated.
- Provide the framework for reporting the results and indicates what is probably necessary to conduct the study and explain how the findings will present this information.
In the social sciences, the research problem establishes the means by which you must answer the "So What?" question. This declarative question refers to a research problem surviving the relevancy test [the quality of a measurement procedure that provides repeatability and accuracy]. Note that answering the "So What?" question requires a commitment on your part to not only show that you have reviewed the literature, but that you have thoroughly considered the significance of the research problem and its implications applied to creating new knowledge and understanding or informing practice.
To survive the "So What" question, problem statements should possess the following attributes:
- Clarity and precision [a well-written statement does not make sweeping generalizations and irresponsible pronouncements; it also does include unspecific determinates like "very" or "giant"],
- Demonstrate a researchable topic or issue [i.e., feasibility of conducting the study is based upon access to information that can be effectively acquired, gathered, interpreted, synthesized, and understood],
- Identification of what would be studied, while avoiding the use of value-laden words and terms,
- Identification of an overarching question or small set of questions accompanied by key factors or variables,
- Identification of key concepts and terms,
- Articulation of the study's conceptual boundaries or parameters or limitations,
- Some generalizability in regards to applicability and bringing results into general use,
- Conveyance of the study's importance, benefits, and justification [i.e., regardless of the type of research, it is important to demonstrate that the research is not trivial],
- Does not have unnecessary jargon or overly complex sentence constructions; and,
- Conveyance of more than the mere gathering of descriptive data providing only a snapshot of the issue or phenomenon under investigation.
Bryman, Alan. “The Research Question in Social Research: What is its Role?” International Journal of Social Research Methodology 10 (2007): 5-20; Brown, Perry J., Allen Dyer, and Ross S. Whaley. "Recreation Research—So What?" Journal of Leisure Research 5 (1973): 16-24; Castellanos, Susie. Critical Writing and Thinking. The Writing Center. Dean of the College. Brown University; Ellis, Timothy J. and Yair Levy Nova. "Framework of Problem-Based Research: A Guide for Novice Researchers on the Development of a Research-Worthy Problem." Informing Science: the International Journal of an Emerging Transdiscipline 11 (2008); Thesis and Purpose Statements. The Writer’s Handbook. Writing Center. University of Wisconsin, Madison; Thesis Statements. The Writing Center. University of North Carolina; Tips and Examples for Writing Thesis Statements. The Writing Lab and The OWL. Purdue University; Selwyn, Neil. "‘So What?’…A Question that Every Journal Article Needs to Answer." Learning, Media, and Technology 39 (2014): 1-5; Shoket, Mohd. "Research Problem: Identification and Formulation." International Journal of Research 1 (May 2014): 512-518.
Structure and Writing Style
I. Types and Content
There are four general conceptualizations of a research problem in the social sciences:
- Casuist Research Problem -- this type of problem relates to the determination of right and wrong in questions of conduct or conscience by analyzing moral dilemmas through the application of general rules and the careful distinction of special cases.
- Difference Research Problem -- typically asks the question, “Is there a difference between two or more groups or treatments?” This type of problem statement is used when the researcher compares or contrasts two or more phenomena. This a common approach to defining a problem in the clinical social sciences or behavioral sciences.
- Descriptive Research Problem -- typically asks the question, "what is...?" with the underlying purpose to describe the significance of a situation, state, or existence of a specific phenomenon. This problem is often associated with revealing hidden or understudied issues.
- Relational Research Problem -- suggests a relationship of some sort between two or more variables to be investigated. The underlying purpose is to investigate specific qualities or characteristics that may be connected in some way.
A problem statement in the social sciences should contain :
- A lead-in that helps ensure the reader will maintain interest over the study,
- A declaration of originality [e.g., mentioning a knowledge void or a lack of clarity about a topic that will be revealed in the literature review of prior research],
- An indication of the central focus of the study [establishing the boundaries of analysis], and
- An explanation of the study's significance or the benefits to be derived from investigating the research problem.
NOTE: A statement describing the research problem of your paper should not be viewed as a thesis statement that you may be familiar with from high school. Given the content listed above, a description of the research problem is usually a short paragraph in length.
II. Sources of Problems for Investigation
The identification of a problem to study can be challenging, not because there's a lack of issues that could be investigated, but due to the challenge of formulating an academically relevant and researchable problem which is unique and does not simply duplicate the work of others. To facilitate how you might select a problem from which to build a research study, consider these sources of inspiration:
Deductions from Theory This relates to deductions made from social philosophy or generalizations embodied in life and in society that the researcher is familiar with. These deductions from human behavior are then placed within an empirical frame of reference through research. From a theory, the researcher can formulate a research problem or hypothesis stating the expected findings in certain empirical situations. The research asks the question: “What relationship between variables will be observed if theory aptly summarizes the state of affairs?” One can then design and carry out a systematic investigation to assess whether empirical data confirm or reject the hypothesis, and hence, the theory.
Interdisciplinary Perspectives Identifying a problem that forms the basis for a research study can come from academic movements and scholarship originating in disciplines outside of your primary area of study. This can be an intellectually stimulating exercise. A review of pertinent literature should include examining research from related disciplines that can reveal new avenues of exploration and analysis. An interdisciplinary approach to selecting a research problem offers an opportunity to construct a more comprehensive understanding of a very complex issue that any single discipline may be able to provide.
Interviewing Practitioners The identification of research problems about particular topics can arise from formal interviews or informal discussions with practitioners who provide insight into new directions for future research and how to make research findings more relevant to practice. Discussions with experts in the field, such as, teachers, social workers, health care providers, lawyers, business leaders, etc., offers the chance to identify practical, “real world” problems that may be understudied or ignored within academic circles. This approach also provides some practical knowledge which may help in the process of designing and conducting your study.
Personal Experience Don't undervalue your everyday experiences or encounters as worthwhile problems for investigation. Think critically about your own experiences and/or frustrations with an issue facing society or related to your community, your neighborhood, your family, or your personal life. This can be derived, for example, from deliberate observations of certain relationships for which there is no clear explanation or witnessing an event that appears harmful to a person or group or that is out of the ordinary.
Relevant Literature The selection of a research problem can be derived from a thorough review of pertinent research associated with your overall area of interest. This may reveal where gaps exist in understanding a topic or where an issue has been understudied. Research may be conducted to: 1) fill such gaps in knowledge; 2) evaluate if the methodologies employed in prior studies can be adapted to solve other problems; or, 3) determine if a similar study could be conducted in a different subject area or applied in a different context or to different study sample [i.e., different setting or different group of people]. Also, authors frequently conclude their studies by noting implications for further research; read the conclusion of pertinent studies because statements about further research can be a valuable source for identifying new problems to investigate. The fact that a researcher has identified a topic worthy of further exploration validates the fact it is worth pursuing.
III. What Makes a Good Research Statement?
A good problem statement begins by introducing the broad area in which your research is centered, gradually leading the reader to the more specific issues you are investigating. The statement need not be lengthy, but a good research problem should incorporate the following features:
1. Compelling Topic The problem chosen should be one that motivates you to address it but simple curiosity is not a good enough reason to pursue a research study because this does not indicate significance. The problem that you choose to explore must be important to you, but it must also be viewed as important by your readers and to a the larger academic and/or social community that could be impacted by the results of your study. 2. Supports Multiple Perspectives The problem must be phrased in a way that avoids dichotomies and instead supports the generation and exploration of multiple perspectives. A general rule of thumb in the social sciences is that a good research problem is one that would generate a variety of viewpoints from a composite audience made up of reasonable people. 3. Researchability This isn't a real word but it represents an important aspect of creating a good research statement. It seems a bit obvious, but you don't want to find yourself in the midst of investigating a complex research project and realize that you don't have enough prior research to draw from for your analysis. There's nothing inherently wrong with original research, but you must choose research problems that can be supported, in some way, by the resources available to you. If you are not sure if something is researchable, don't assume that it isn't if you don't find information right away--seek help from a librarian !
NOTE: Do not confuse a research problem with a research topic. A topic is something to read and obtain information about, whereas a problem is something to be solved or framed as a question raised for inquiry, consideration, or solution, or explained as a source of perplexity, distress, or vexation. In short, a research topic is something to be understood; a research problem is something that needs to be investigated.
IV. Asking Analytical Questions about the Research Problem
Research problems in the social and behavioral sciences are often analyzed around critical questions that must be investigated. These questions can be explicitly listed in the introduction [i.e., "This study addresses three research questions about women's psychological recovery from domestic abuse in multi-generational home settings..."], or, the questions are implied in the text as specific areas of study related to the research problem. Explicitly listing your research questions at the end of your introduction can help in designing a clear roadmap of what you plan to address in your study, whereas, implicitly integrating them into the text of the introduction allows you to create a more compelling narrative around the key issues under investigation. Either approach is appropriate.
The number of questions you attempt to address should be based on the complexity of the problem you are investigating and what areas of inquiry you find most critical to study. Practical considerations, such as, the length of the paper you are writing or the availability of resources to analyze the issue can also factor in how many questions to ask. In general, however, there should be no more than four research questions underpinning a single research problem.
Given this, well-developed analytical questions can focus on any of the following:
- Highlights a genuine dilemma, area of ambiguity, or point of confusion about a topic open to interpretation by your readers;
- Yields an answer that is unexpected and not obvious rather than inevitable and self-evident;
- Provokes meaningful thought or discussion;
- Raises the visibility of the key ideas or concepts that may be understudied or hidden;
- Suggests the need for complex analysis or argument rather than a basic description or summary; and,
- Offers a specific path of inquiry that avoids eliciting generalizations about the problem.
NOTE: Questions of how and why concerning a research problem often require more analysis than questions about who, what, where, and when. You should still ask yourself these latter questions, however. Thinking introspectively about the who, what, where, and when of a research problem can help ensure that you have thoroughly considered all aspects of the problem under investigation and helps define the scope of the study in relation to the problem.
V. Mistakes to Avoid
Beware of circular reasoning! Do not state the research problem as simply the absence of the thing you are suggesting. For example, if you propose the following, "The problem in this community is that there is no hospital," this only leads to a research problem where:
- The need is for a hospital
- The objective is to create a hospital
- The method is to plan for building a hospital, and
- The evaluation is to measure if there is a hospital or not.
This is an example of a research problem that fails the "So What?" test . In this example, the problem does not reveal the relevance of why you are investigating the fact there is no hospital in the community [e.g., perhaps there's a hospital in the community ten miles away]; it does not elucidate the significance of why one should study the fact there is no hospital in the community [e.g., that hospital in the community ten miles away has no emergency room]; the research problem does not offer an intellectual pathway towards adding new knowledge or clarifying prior knowledge [e.g., the county in which there is no hospital already conducted a study about the need for a hospital, but it was conducted ten years ago]; and, the problem does not offer meaningful outcomes that lead to recommendations that can be generalized for other situations or that could suggest areas for further research [e.g., the challenges of building a new hospital serves as a case study for other communities].
Alvesson, Mats and Jörgen Sandberg. “Generating Research Questions Through Problematization.” Academy of Management Review 36 (April 2011): 247-271 ; Choosing and Refining Topics. Writing@CSU. Colorado State University; D'Souza, Victor S. "Use of Induction and Deduction in Research in Social Sciences: An Illustration." Journal of the Indian Law Institute 24 (1982): 655-661; Ellis, Timothy J. and Yair Levy Nova. "Framework of Problem-Based Research: A Guide for Novice Researchers on the Development of a Research-Worthy Problem." Informing Science: the International Journal of an Emerging Transdiscipline 11 (2008); How to Write a Research Question. The Writing Center. George Mason University; Invention: Developing a Thesis Statement. The Reading/Writing Center. Hunter College; Problem Statements PowerPoint Presentation. The Writing Lab and The OWL. Purdue University; Procter, Margaret. Using Thesis Statements. University College Writing Centre. University of Toronto; Shoket, Mohd. "Research Problem: Identification and Formulation." International Journal of Research 1 (May 2014): 512-518; Trochim, William M.K. Problem Formulation. Research Methods Knowledge Base. 2006; Thesis and Purpose Statements. The Writer’s Handbook. Writing Center. University of Wisconsin, Madison; Thesis Statements. The Writing Center. University of North Carolina; Tips and Examples for Writing Thesis Statements. The Writing Lab and The OWL. Purdue University; Pardede, Parlindungan. “Identifying and Formulating the Research Problem." Research in ELT: Module 4 (October 2018): 1-13; Walk, Kerry. Asking an Analytical Question. [Class handout or worksheet]. Princeton University; White, Patrick. Developing Research Questions: A Guide for Social Scientists . New York: Palgrave McMillan, 2009; Li, Yanmei, and Sumei Zhang. "Identifying the Research Problem." In Applied Research Methods in Urban and Regional Planning . (Cham, Switzerland: Springer International Publishing, 2022), pp. 13-21.
- << Previous: Background Information
- Next: Theoretical Framework >>
- Last Updated: Jun 12, 2024 8:53 AM
- URL: https://libguides.usc.edu/writingguide
How to Identify a Hypothesis
Pharaba witt.
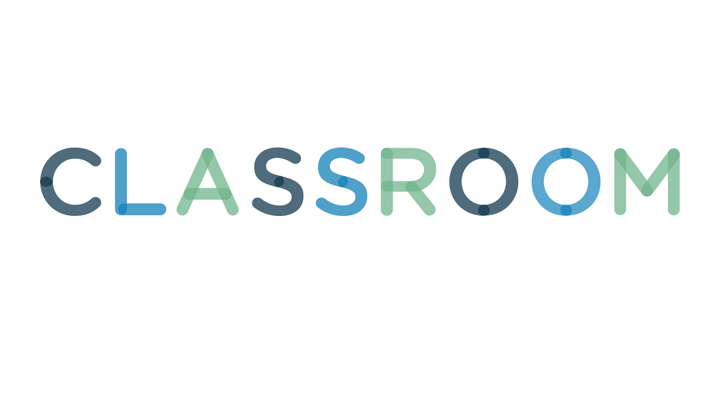
Identifying a hypothesis allows students to know what is being proven by a particular experiment or paper. Being able to determine the overall point not only makes you a more effective reader but also better at formulating your own theories when writing your own paper. By asking a few simple questions while you read, you should be able to pick out the intent of the author and identify the hypothesis.
Explore this article
- Read over the beginning of the material
- Look for if-then statements
- Ask if the if-then statement
- Read through the rest of the paper
1 Read over the beginning of the material
Read over the beginning of the material while asking what the purpose of the introduction is.
2 Look for if-then statements
Look for if-then statements. This type of wording is usually the hypothesis. It lays out a position for the overall paper or project.
3 Ask if the if-then statement
Ask if the if-then statement is testable or provable. Is this the type of statement you could supply evidence for in order to prove? Decide if you agree with the hypothesis. This puts you in a position to be convinced as you read the paper or follow the experiment.
4 Read through the rest of the paper
Read through the rest of the paper to determine if it is going in the direction you suspect. If you get to a point where the words seem to be proving something entirely different, revisit the first paragraph to see if there is another if-then statement.
- Try not to jump to conclusions. Read the paragraph thoroughly through a few times to be certain you have not missed any other potential hypothesis.
- When presented with the information, ask yourself what you would aim to prove. Oftentimes you will formulate a similar question. While your expectations might be different, picking out the hypothesis can be easier.
- Not every hypothesis is accurate. Part of testing a theory is determining if the expectation is accurate. By the end of the paper the writer might draw a new conclusion. The author could even take that space to formulate an entirely new hypothesis.
- Practice writing if-then statements. The more familiar you are with formulating hypothesis statements the better you will be at identifying the hypothesis.
- 1 SlideShare: Hypothesis Conclusion (Geometry)
- 2 Cornell University: Null Hypothesis vs. Alternative Hypothesis
About the Author
Pharaba Witt has worked as a writer in Los Angeles for more than 10 years. She has written for websites such as USA Today, Red Beacon, LIVESTRONG, WiseGeek, Web Series Network, Nursing Daily and major film studios. When not traveling she enjoys outdoor activities such as backpacking, snowboarding, ice climbing and scuba diving. She is constantly researching equipment and seeking new challenges.
Related Articles
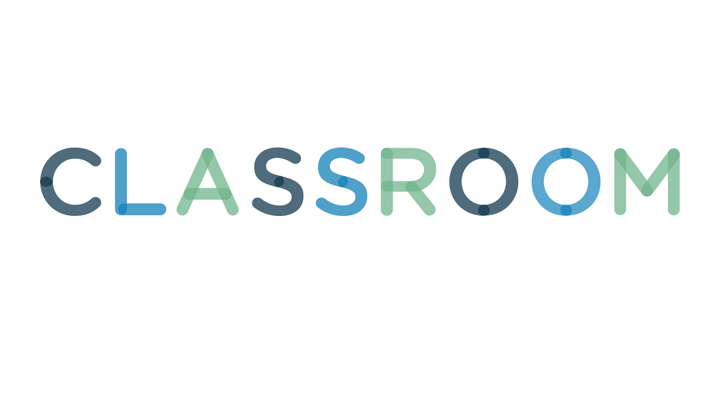
How to Write a Rationale
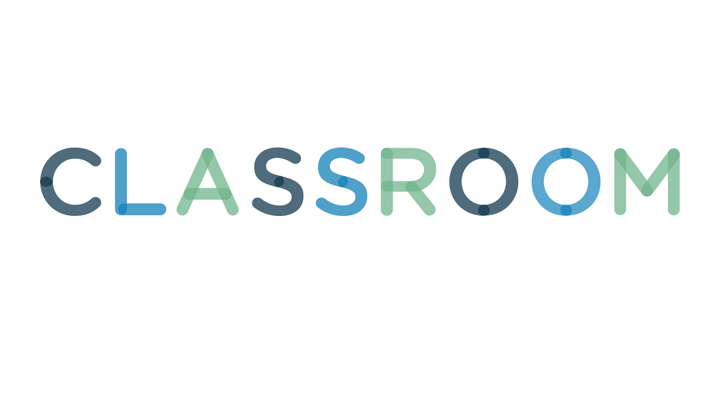
How to Start a Thesis Statement
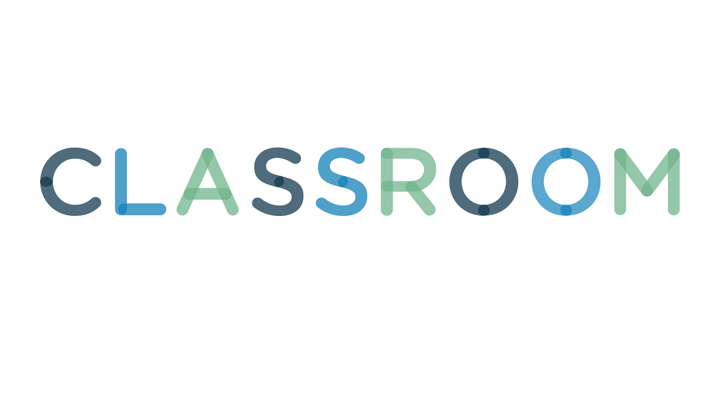
How to Find a Thesis in an Essay
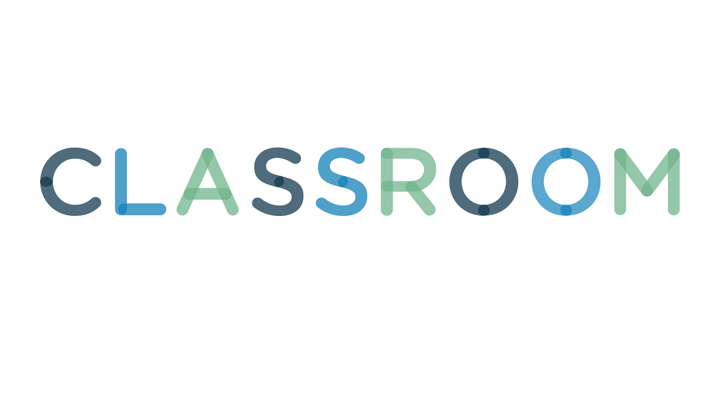
How to Write a Thesis Statement in High School Essays
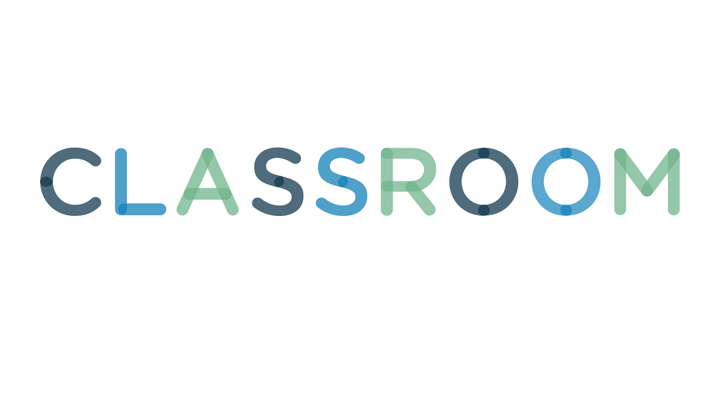
Research Paper Thesis Topics
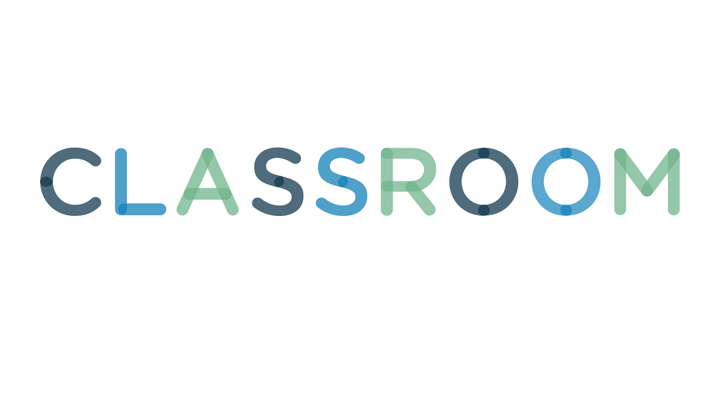
What Is a Lead-in Statement?
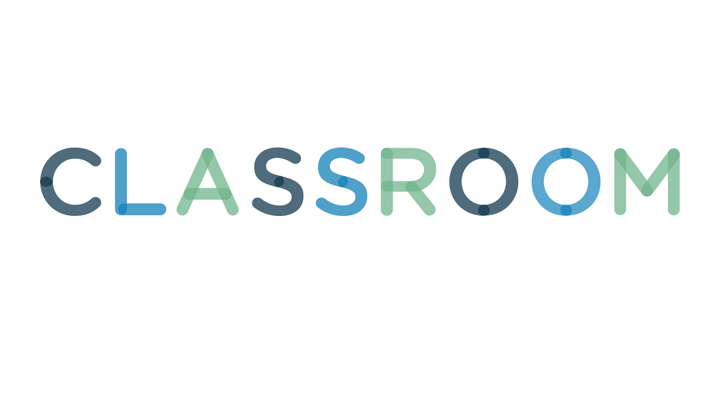
Comprehension Skills That Require Critical Thinking
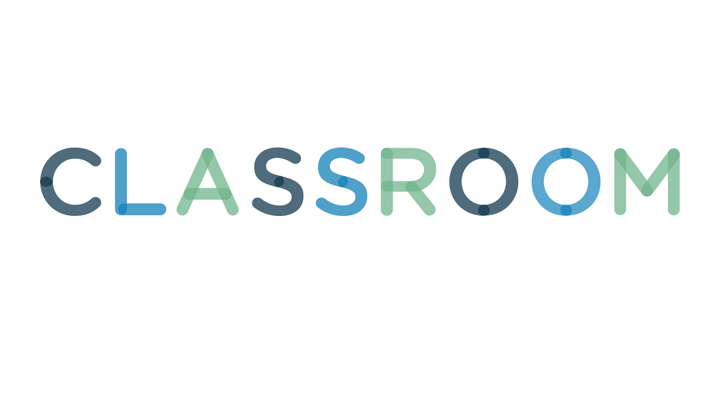
How to Improve Adult Reading Comprehension
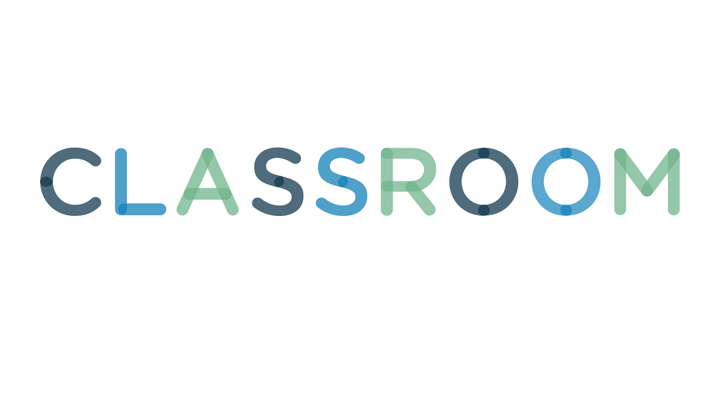
Steps in Writing a Report
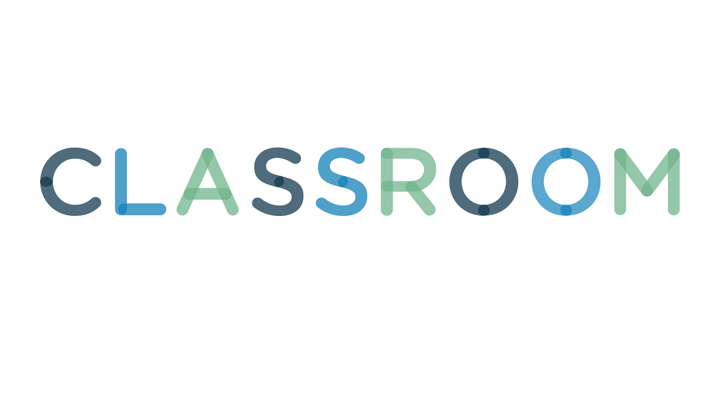
How to Answer Open-Ended Essay Questions
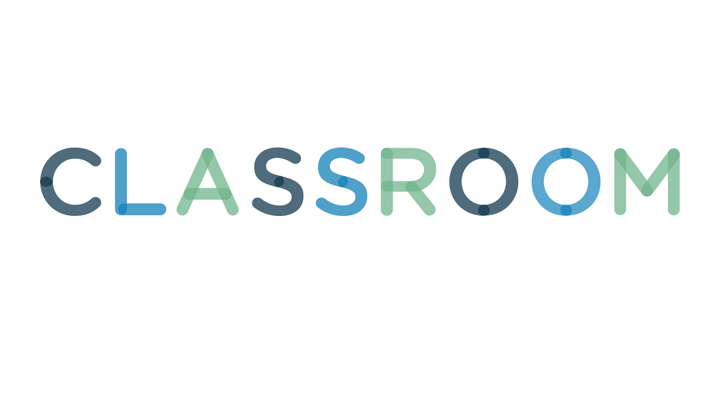
How to Write a DBQ Essay
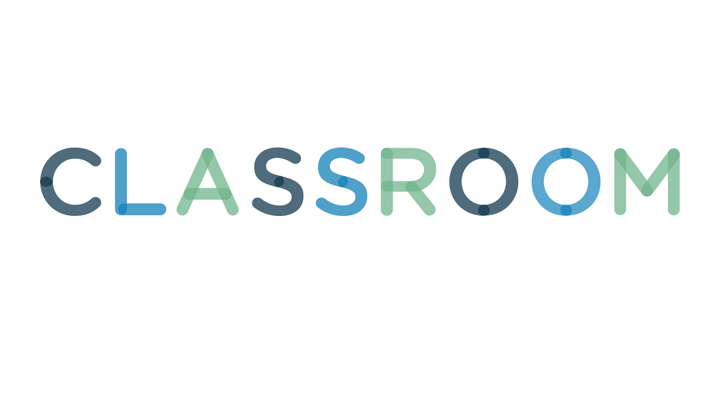
How to Write a Paper: Title, Introduction, Body & Conclusion
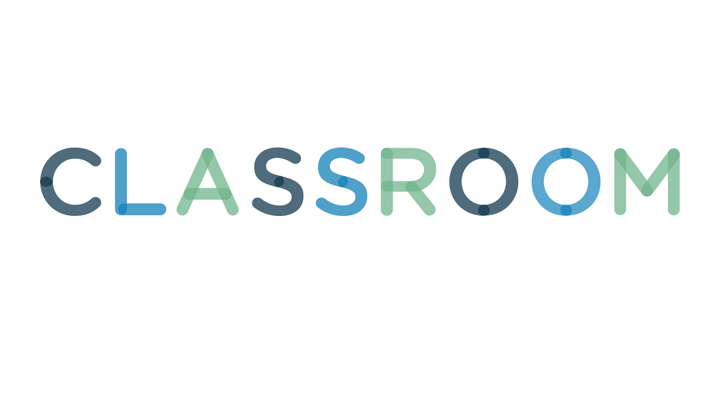
How to Write a Thesis Statement for an Article Critique
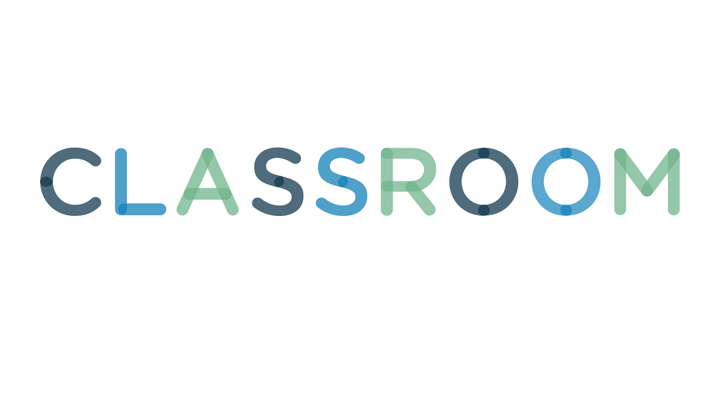
How to Write an Analytical Book Report
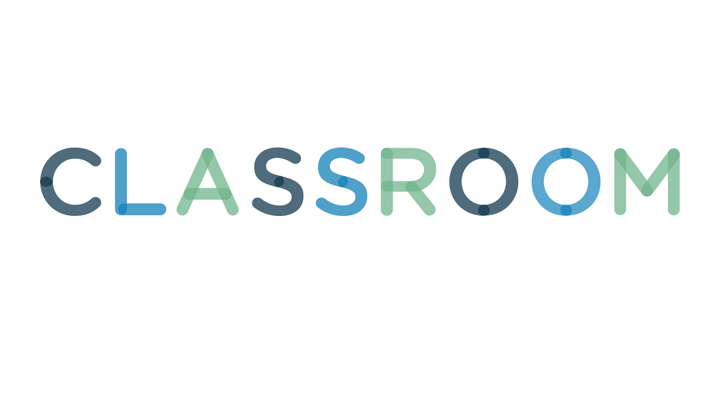
How to Write a Hypothesis to an Analytical Essay
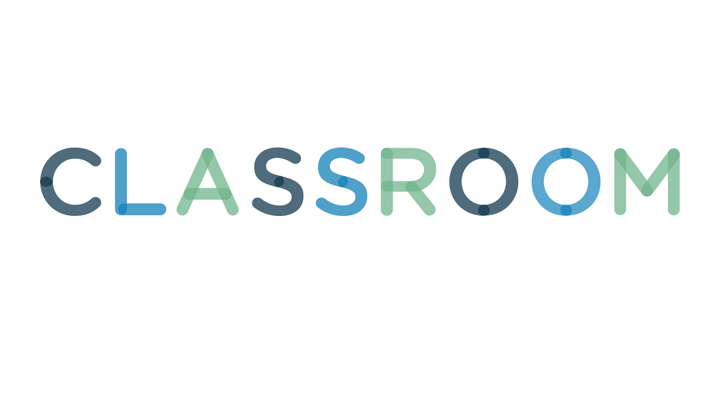
How to Write an Introduction Paragraph With Thesis...
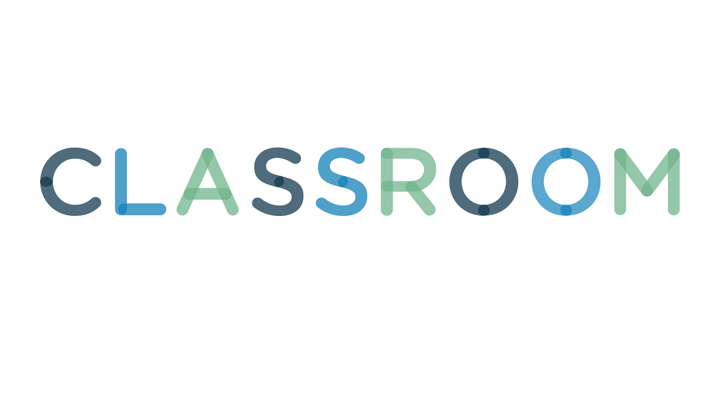
How to Write a Good High School English Essay
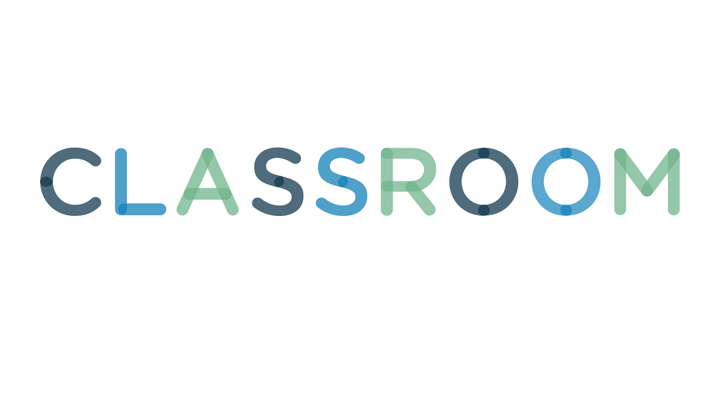
How to Make an Introduction to an Informative Essay
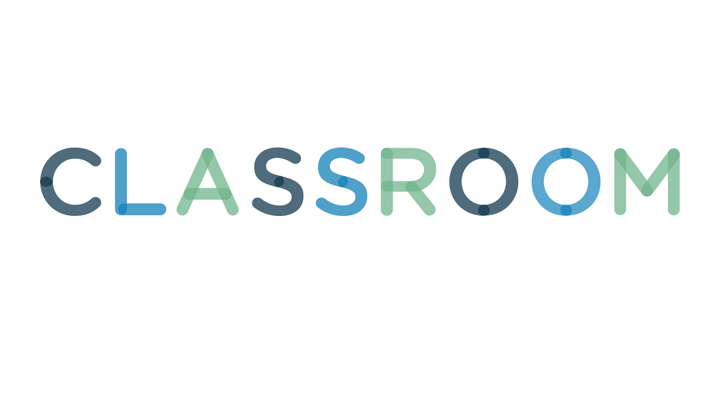
What Are the Differences Between Bias & Fallacy?
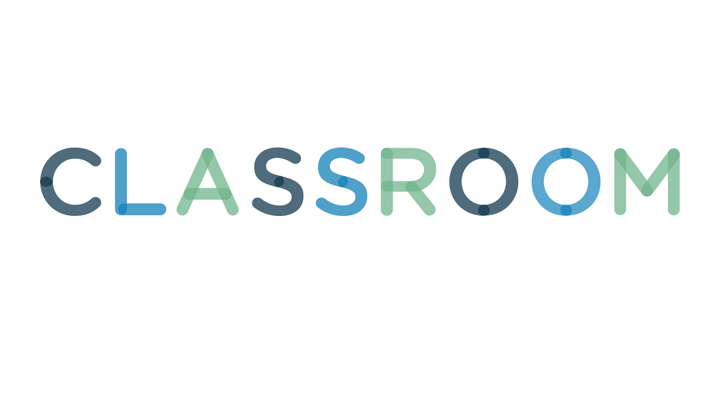
How to Write a Persuasive Essay
Regardless of how old we are, we never stop learning. Classroom is the educational resource for people of all ages. Whether you’re studying times tables or applying to college, Classroom has the answers.
- Accessibility
- Terms of Use
- Privacy Policy
- Copyright Policy
- Manage Preferences
© 2020 Leaf Group Ltd. / Leaf Group Media, All Rights Reserved. Based on the Word Net lexical database for the English Language. See disclaimer .

An official website of the United States government
The .gov means it’s official. Federal government websites often end in .gov or .mil. Before sharing sensitive information, make sure you’re on a federal government site.
The site is secure. The https:// ensures that you are connecting to the official website and that any information you provide is encrypted and transmitted securely.
- Publications
- Account settings
Preview improvements coming to the PMC website in October 2024. Learn More or Try it out now .
- Advanced Search
- Journal List
- J Korean Med Sci
- v.37(16); 2022 Apr 25

A Practical Guide to Writing Quantitative and Qualitative Research Questions and Hypotheses in Scholarly Articles
Edward barroga.
1 Department of General Education, Graduate School of Nursing Science, St. Luke’s International University, Tokyo, Japan.
Glafera Janet Matanguihan
2 Department of Biological Sciences, Messiah University, Mechanicsburg, PA, USA.
The development of research questions and the subsequent hypotheses are prerequisites to defining the main research purpose and specific objectives of a study. Consequently, these objectives determine the study design and research outcome. The development of research questions is a process based on knowledge of current trends, cutting-edge studies, and technological advances in the research field. Excellent research questions are focused and require a comprehensive literature search and in-depth understanding of the problem being investigated. Initially, research questions may be written as descriptive questions which could be developed into inferential questions. These questions must be specific and concise to provide a clear foundation for developing hypotheses. Hypotheses are more formal predictions about the research outcomes. These specify the possible results that may or may not be expected regarding the relationship between groups. Thus, research questions and hypotheses clarify the main purpose and specific objectives of the study, which in turn dictate the design of the study, its direction, and outcome. Studies developed from good research questions and hypotheses will have trustworthy outcomes with wide-ranging social and health implications.
INTRODUCTION
Scientific research is usually initiated by posing evidenced-based research questions which are then explicitly restated as hypotheses. 1 , 2 The hypotheses provide directions to guide the study, solutions, explanations, and expected results. 3 , 4 Both research questions and hypotheses are essentially formulated based on conventional theories and real-world processes, which allow the inception of novel studies and the ethical testing of ideas. 5 , 6
It is crucial to have knowledge of both quantitative and qualitative research 2 as both types of research involve writing research questions and hypotheses. 7 However, these crucial elements of research are sometimes overlooked; if not overlooked, then framed without the forethought and meticulous attention it needs. Planning and careful consideration are needed when developing quantitative or qualitative research, particularly when conceptualizing research questions and hypotheses. 4
There is a continuing need to support researchers in the creation of innovative research questions and hypotheses, as well as for journal articles that carefully review these elements. 1 When research questions and hypotheses are not carefully thought of, unethical studies and poor outcomes usually ensue. Carefully formulated research questions and hypotheses define well-founded objectives, which in turn determine the appropriate design, course, and outcome of the study. This article then aims to discuss in detail the various aspects of crafting research questions and hypotheses, with the goal of guiding researchers as they develop their own. Examples from the authors and peer-reviewed scientific articles in the healthcare field are provided to illustrate key points.
DEFINITIONS AND RELATIONSHIP OF RESEARCH QUESTIONS AND HYPOTHESES
A research question is what a study aims to answer after data analysis and interpretation. The answer is written in length in the discussion section of the paper. Thus, the research question gives a preview of the different parts and variables of the study meant to address the problem posed in the research question. 1 An excellent research question clarifies the research writing while facilitating understanding of the research topic, objective, scope, and limitations of the study. 5
On the other hand, a research hypothesis is an educated statement of an expected outcome. This statement is based on background research and current knowledge. 8 , 9 The research hypothesis makes a specific prediction about a new phenomenon 10 or a formal statement on the expected relationship between an independent variable and a dependent variable. 3 , 11 It provides a tentative answer to the research question to be tested or explored. 4
Hypotheses employ reasoning to predict a theory-based outcome. 10 These can also be developed from theories by focusing on components of theories that have not yet been observed. 10 The validity of hypotheses is often based on the testability of the prediction made in a reproducible experiment. 8
Conversely, hypotheses can also be rephrased as research questions. Several hypotheses based on existing theories and knowledge may be needed to answer a research question. Developing ethical research questions and hypotheses creates a research design that has logical relationships among variables. These relationships serve as a solid foundation for the conduct of the study. 4 , 11 Haphazardly constructed research questions can result in poorly formulated hypotheses and improper study designs, leading to unreliable results. Thus, the formulations of relevant research questions and verifiable hypotheses are crucial when beginning research. 12
CHARACTERISTICS OF GOOD RESEARCH QUESTIONS AND HYPOTHESES
Excellent research questions are specific and focused. These integrate collective data and observations to confirm or refute the subsequent hypotheses. Well-constructed hypotheses are based on previous reports and verify the research context. These are realistic, in-depth, sufficiently complex, and reproducible. More importantly, these hypotheses can be addressed and tested. 13
There are several characteristics of well-developed hypotheses. Good hypotheses are 1) empirically testable 7 , 10 , 11 , 13 ; 2) backed by preliminary evidence 9 ; 3) testable by ethical research 7 , 9 ; 4) based on original ideas 9 ; 5) have evidenced-based logical reasoning 10 ; and 6) can be predicted. 11 Good hypotheses can infer ethical and positive implications, indicating the presence of a relationship or effect relevant to the research theme. 7 , 11 These are initially developed from a general theory and branch into specific hypotheses by deductive reasoning. In the absence of a theory to base the hypotheses, inductive reasoning based on specific observations or findings form more general hypotheses. 10
TYPES OF RESEARCH QUESTIONS AND HYPOTHESES
Research questions and hypotheses are developed according to the type of research, which can be broadly classified into quantitative and qualitative research. We provide a summary of the types of research questions and hypotheses under quantitative and qualitative research categories in Table 1 .
Quantitative research questions | Quantitative research hypotheses |
---|---|
Descriptive research questions | Simple hypothesis |
Comparative research questions | Complex hypothesis |
Relationship research questions | Directional hypothesis |
Non-directional hypothesis | |
Associative hypothesis | |
Causal hypothesis | |
Null hypothesis | |
Alternative hypothesis | |
Working hypothesis | |
Statistical hypothesis | |
Logical hypothesis | |
Hypothesis-testing | |
Qualitative research questions | Qualitative research hypotheses |
Contextual research questions | Hypothesis-generating |
Descriptive research questions | |
Evaluation research questions | |
Explanatory research questions | |
Exploratory research questions | |
Generative research questions | |
Ideological research questions | |
Ethnographic research questions | |
Phenomenological research questions | |
Grounded theory questions | |
Qualitative case study questions |
Research questions in quantitative research
In quantitative research, research questions inquire about the relationships among variables being investigated and are usually framed at the start of the study. These are precise and typically linked to the subject population, dependent and independent variables, and research design. 1 Research questions may also attempt to describe the behavior of a population in relation to one or more variables, or describe the characteristics of variables to be measured ( descriptive research questions ). 1 , 5 , 14 These questions may also aim to discover differences between groups within the context of an outcome variable ( comparative research questions ), 1 , 5 , 14 or elucidate trends and interactions among variables ( relationship research questions ). 1 , 5 We provide examples of descriptive, comparative, and relationship research questions in quantitative research in Table 2 .
Quantitative research questions | |
---|---|
Descriptive research question | |
- Measures responses of subjects to variables | |
- Presents variables to measure, analyze, or assess | |
What is the proportion of resident doctors in the hospital who have mastered ultrasonography (response of subjects to a variable) as a diagnostic technique in their clinical training? | |
Comparative research question | |
- Clarifies difference between one group with outcome variable and another group without outcome variable | |
Is there a difference in the reduction of lung metastasis in osteosarcoma patients who received the vitamin D adjunctive therapy (group with outcome variable) compared with osteosarcoma patients who did not receive the vitamin D adjunctive therapy (group without outcome variable)? | |
- Compares the effects of variables | |
How does the vitamin D analogue 22-Oxacalcitriol (variable 1) mimic the antiproliferative activity of 1,25-Dihydroxyvitamin D (variable 2) in osteosarcoma cells? | |
Relationship research question | |
- Defines trends, association, relationships, or interactions between dependent variable and independent variable | |
Is there a relationship between the number of medical student suicide (dependent variable) and the level of medical student stress (independent variable) in Japan during the first wave of the COVID-19 pandemic? |
Hypotheses in quantitative research
In quantitative research, hypotheses predict the expected relationships among variables. 15 Relationships among variables that can be predicted include 1) between a single dependent variable and a single independent variable ( simple hypothesis ) or 2) between two or more independent and dependent variables ( complex hypothesis ). 4 , 11 Hypotheses may also specify the expected direction to be followed and imply an intellectual commitment to a particular outcome ( directional hypothesis ) 4 . On the other hand, hypotheses may not predict the exact direction and are used in the absence of a theory, or when findings contradict previous studies ( non-directional hypothesis ). 4 In addition, hypotheses can 1) define interdependency between variables ( associative hypothesis ), 4 2) propose an effect on the dependent variable from manipulation of the independent variable ( causal hypothesis ), 4 3) state a negative relationship between two variables ( null hypothesis ), 4 , 11 , 15 4) replace the working hypothesis if rejected ( alternative hypothesis ), 15 explain the relationship of phenomena to possibly generate a theory ( working hypothesis ), 11 5) involve quantifiable variables that can be tested statistically ( statistical hypothesis ), 11 6) or express a relationship whose interlinks can be verified logically ( logical hypothesis ). 11 We provide examples of simple, complex, directional, non-directional, associative, causal, null, alternative, working, statistical, and logical hypotheses in quantitative research, as well as the definition of quantitative hypothesis-testing research in Table 3 .
Quantitative research hypotheses | |
---|---|
Simple hypothesis | |
- Predicts relationship between single dependent variable and single independent variable | |
If the dose of the new medication (single independent variable) is high, blood pressure (single dependent variable) is lowered. | |
Complex hypothesis | |
- Foretells relationship between two or more independent and dependent variables | |
The higher the use of anticancer drugs, radiation therapy, and adjunctive agents (3 independent variables), the higher would be the survival rate (1 dependent variable). | |
Directional hypothesis | |
- Identifies study direction based on theory towards particular outcome to clarify relationship between variables | |
Privately funded research projects will have a larger international scope (study direction) than publicly funded research projects. | |
Non-directional hypothesis | |
- Nature of relationship between two variables or exact study direction is not identified | |
- Does not involve a theory | |
Women and men are different in terms of helpfulness. (Exact study direction is not identified) | |
Associative hypothesis | |
- Describes variable interdependency | |
- Change in one variable causes change in another variable | |
A larger number of people vaccinated against COVID-19 in the region (change in independent variable) will reduce the region’s incidence of COVID-19 infection (change in dependent variable). | |
Causal hypothesis | |
- An effect on dependent variable is predicted from manipulation of independent variable | |
A change into a high-fiber diet (independent variable) will reduce the blood sugar level (dependent variable) of the patient. | |
Null hypothesis | |
- A negative statement indicating no relationship or difference between 2 variables | |
There is no significant difference in the severity of pulmonary metastases between the new drug (variable 1) and the current drug (variable 2). | |
Alternative hypothesis | |
- Following a null hypothesis, an alternative hypothesis predicts a relationship between 2 study variables | |
The new drug (variable 1) is better on average in reducing the level of pain from pulmonary metastasis than the current drug (variable 2). | |
Working hypothesis | |
- A hypothesis that is initially accepted for further research to produce a feasible theory | |
Dairy cows fed with concentrates of different formulations will produce different amounts of milk. | |
Statistical hypothesis | |
- Assumption about the value of population parameter or relationship among several population characteristics | |
- Validity tested by a statistical experiment or analysis | |
The mean recovery rate from COVID-19 infection (value of population parameter) is not significantly different between population 1 and population 2. | |
There is a positive correlation between the level of stress at the workplace and the number of suicides (population characteristics) among working people in Japan. | |
Logical hypothesis | |
- Offers or proposes an explanation with limited or no extensive evidence | |
If healthcare workers provide more educational programs about contraception methods, the number of adolescent pregnancies will be less. | |
Hypothesis-testing (Quantitative hypothesis-testing research) | |
- Quantitative research uses deductive reasoning. | |
- This involves the formation of a hypothesis, collection of data in the investigation of the problem, analysis and use of the data from the investigation, and drawing of conclusions to validate or nullify the hypotheses. |
Research questions in qualitative research
Unlike research questions in quantitative research, research questions in qualitative research are usually continuously reviewed and reformulated. The central question and associated subquestions are stated more than the hypotheses. 15 The central question broadly explores a complex set of factors surrounding the central phenomenon, aiming to present the varied perspectives of participants. 15
There are varied goals for which qualitative research questions are developed. These questions can function in several ways, such as to 1) identify and describe existing conditions ( contextual research question s); 2) describe a phenomenon ( descriptive research questions ); 3) assess the effectiveness of existing methods, protocols, theories, or procedures ( evaluation research questions ); 4) examine a phenomenon or analyze the reasons or relationships between subjects or phenomena ( explanatory research questions ); or 5) focus on unknown aspects of a particular topic ( exploratory research questions ). 5 In addition, some qualitative research questions provide new ideas for the development of theories and actions ( generative research questions ) or advance specific ideologies of a position ( ideological research questions ). 1 Other qualitative research questions may build on a body of existing literature and become working guidelines ( ethnographic research questions ). Research questions may also be broadly stated without specific reference to the existing literature or a typology of questions ( phenomenological research questions ), may be directed towards generating a theory of some process ( grounded theory questions ), or may address a description of the case and the emerging themes ( qualitative case study questions ). 15 We provide examples of contextual, descriptive, evaluation, explanatory, exploratory, generative, ideological, ethnographic, phenomenological, grounded theory, and qualitative case study research questions in qualitative research in Table 4 , and the definition of qualitative hypothesis-generating research in Table 5 .
Qualitative research questions | |
---|---|
Contextual research question | |
- Ask the nature of what already exists | |
- Individuals or groups function to further clarify and understand the natural context of real-world problems | |
What are the experiences of nurses working night shifts in healthcare during the COVID-19 pandemic? (natural context of real-world problems) | |
Descriptive research question | |
- Aims to describe a phenomenon | |
What are the different forms of disrespect and abuse (phenomenon) experienced by Tanzanian women when giving birth in healthcare facilities? | |
Evaluation research question | |
- Examines the effectiveness of existing practice or accepted frameworks | |
How effective are decision aids (effectiveness of existing practice) in helping decide whether to give birth at home or in a healthcare facility? | |
Explanatory research question | |
- Clarifies a previously studied phenomenon and explains why it occurs | |
Why is there an increase in teenage pregnancy (phenomenon) in Tanzania? | |
Exploratory research question | |
- Explores areas that have not been fully investigated to have a deeper understanding of the research problem | |
What factors affect the mental health of medical students (areas that have not yet been fully investigated) during the COVID-19 pandemic? | |
Generative research question | |
- Develops an in-depth understanding of people’s behavior by asking ‘how would’ or ‘what if’ to identify problems and find solutions | |
How would the extensive research experience of the behavior of new staff impact the success of the novel drug initiative? | |
Ideological research question | |
- Aims to advance specific ideas or ideologies of a position | |
Are Japanese nurses who volunteer in remote African hospitals able to promote humanized care of patients (specific ideas or ideologies) in the areas of safe patient environment, respect of patient privacy, and provision of accurate information related to health and care? | |
Ethnographic research question | |
- Clarifies peoples’ nature, activities, their interactions, and the outcomes of their actions in specific settings | |
What are the demographic characteristics, rehabilitative treatments, community interactions, and disease outcomes (nature, activities, their interactions, and the outcomes) of people in China who are suffering from pneumoconiosis? | |
Phenomenological research question | |
- Knows more about the phenomena that have impacted an individual | |
What are the lived experiences of parents who have been living with and caring for children with a diagnosis of autism? (phenomena that have impacted an individual) | |
Grounded theory question | |
- Focuses on social processes asking about what happens and how people interact, or uncovering social relationships and behaviors of groups | |
What are the problems that pregnant adolescents face in terms of social and cultural norms (social processes), and how can these be addressed? | |
Qualitative case study question | |
- Assesses a phenomenon using different sources of data to answer “why” and “how” questions | |
- Considers how the phenomenon is influenced by its contextual situation. | |
How does quitting work and assuming the role of a full-time mother (phenomenon assessed) change the lives of women in Japan? |
Qualitative research hypotheses | |
---|---|
Hypothesis-generating (Qualitative hypothesis-generating research) | |
- Qualitative research uses inductive reasoning. | |
- This involves data collection from study participants or the literature regarding a phenomenon of interest, using the collected data to develop a formal hypothesis, and using the formal hypothesis as a framework for testing the hypothesis. | |
- Qualitative exploratory studies explore areas deeper, clarifying subjective experience and allowing formulation of a formal hypothesis potentially testable in a future quantitative approach. |
Qualitative studies usually pose at least one central research question and several subquestions starting with How or What . These research questions use exploratory verbs such as explore or describe . These also focus on one central phenomenon of interest, and may mention the participants and research site. 15
Hypotheses in qualitative research
Hypotheses in qualitative research are stated in the form of a clear statement concerning the problem to be investigated. Unlike in quantitative research where hypotheses are usually developed to be tested, qualitative research can lead to both hypothesis-testing and hypothesis-generating outcomes. 2 When studies require both quantitative and qualitative research questions, this suggests an integrative process between both research methods wherein a single mixed-methods research question can be developed. 1
FRAMEWORKS FOR DEVELOPING RESEARCH QUESTIONS AND HYPOTHESES
Research questions followed by hypotheses should be developed before the start of the study. 1 , 12 , 14 It is crucial to develop feasible research questions on a topic that is interesting to both the researcher and the scientific community. This can be achieved by a meticulous review of previous and current studies to establish a novel topic. Specific areas are subsequently focused on to generate ethical research questions. The relevance of the research questions is evaluated in terms of clarity of the resulting data, specificity of the methodology, objectivity of the outcome, depth of the research, and impact of the study. 1 , 5 These aspects constitute the FINER criteria (i.e., Feasible, Interesting, Novel, Ethical, and Relevant). 1 Clarity and effectiveness are achieved if research questions meet the FINER criteria. In addition to the FINER criteria, Ratan et al. described focus, complexity, novelty, feasibility, and measurability for evaluating the effectiveness of research questions. 14
The PICOT and PEO frameworks are also used when developing research questions. 1 The following elements are addressed in these frameworks, PICOT: P-population/patients/problem, I-intervention or indicator being studied, C-comparison group, O-outcome of interest, and T-timeframe of the study; PEO: P-population being studied, E-exposure to preexisting conditions, and O-outcome of interest. 1 Research questions are also considered good if these meet the “FINERMAPS” framework: Feasible, Interesting, Novel, Ethical, Relevant, Manageable, Appropriate, Potential value/publishable, and Systematic. 14
As we indicated earlier, research questions and hypotheses that are not carefully formulated result in unethical studies or poor outcomes. To illustrate this, we provide some examples of ambiguous research question and hypotheses that result in unclear and weak research objectives in quantitative research ( Table 6 ) 16 and qualitative research ( Table 7 ) 17 , and how to transform these ambiguous research question(s) and hypothesis(es) into clear and good statements.
Variables | Unclear and weak statement (Statement 1) | Clear and good statement (Statement 2) | Points to avoid |
---|---|---|---|
Research question | Which is more effective between smoke moxibustion and smokeless moxibustion? | “Moreover, regarding smoke moxibustion versus smokeless moxibustion, it remains unclear which is more effective, safe, and acceptable to pregnant women, and whether there is any difference in the amount of heat generated.” | 1) Vague and unfocused questions |
2) Closed questions simply answerable by yes or no | |||
3) Questions requiring a simple choice | |||
Hypothesis | The smoke moxibustion group will have higher cephalic presentation. | “Hypothesis 1. The smoke moxibustion stick group (SM group) and smokeless moxibustion stick group (-SLM group) will have higher rates of cephalic presentation after treatment than the control group. | 1) Unverifiable hypotheses |
Hypothesis 2. The SM group and SLM group will have higher rates of cephalic presentation at birth than the control group. | 2) Incompletely stated groups of comparison | ||
Hypothesis 3. There will be no significant differences in the well-being of the mother and child among the three groups in terms of the following outcomes: premature birth, premature rupture of membranes (PROM) at < 37 weeks, Apgar score < 7 at 5 min, umbilical cord blood pH < 7.1, admission to neonatal intensive care unit (NICU), and intrauterine fetal death.” | 3) Insufficiently described variables or outcomes | ||
Research objective | To determine which is more effective between smoke moxibustion and smokeless moxibustion. | “The specific aims of this pilot study were (a) to compare the effects of smoke moxibustion and smokeless moxibustion treatments with the control group as a possible supplement to ECV for converting breech presentation to cephalic presentation and increasing adherence to the newly obtained cephalic position, and (b) to assess the effects of these treatments on the well-being of the mother and child.” | 1) Poor understanding of the research question and hypotheses |
2) Insufficient description of population, variables, or study outcomes |
a These statements were composed for comparison and illustrative purposes only.
b These statements are direct quotes from Higashihara and Horiuchi. 16
Variables | Unclear and weak statement (Statement 1) | Clear and good statement (Statement 2) | Points to avoid |
---|---|---|---|
Research question | Does disrespect and abuse (D&A) occur in childbirth in Tanzania? | How does disrespect and abuse (D&A) occur and what are the types of physical and psychological abuses observed in midwives’ actual care during facility-based childbirth in urban Tanzania? | 1) Ambiguous or oversimplistic questions |
2) Questions unverifiable by data collection and analysis | |||
Hypothesis | Disrespect and abuse (D&A) occur in childbirth in Tanzania. | Hypothesis 1: Several types of physical and psychological abuse by midwives in actual care occur during facility-based childbirth in urban Tanzania. | 1) Statements simply expressing facts |
Hypothesis 2: Weak nursing and midwifery management contribute to the D&A of women during facility-based childbirth in urban Tanzania. | 2) Insufficiently described concepts or variables | ||
Research objective | To describe disrespect and abuse (D&A) in childbirth in Tanzania. | “This study aimed to describe from actual observations the respectful and disrespectful care received by women from midwives during their labor period in two hospitals in urban Tanzania.” | 1) Statements unrelated to the research question and hypotheses |
2) Unattainable or unexplorable objectives |
a This statement is a direct quote from Shimoda et al. 17
The other statements were composed for comparison and illustrative purposes only.
CONSTRUCTING RESEARCH QUESTIONS AND HYPOTHESES
To construct effective research questions and hypotheses, it is very important to 1) clarify the background and 2) identify the research problem at the outset of the research, within a specific timeframe. 9 Then, 3) review or conduct preliminary research to collect all available knowledge about the possible research questions by studying theories and previous studies. 18 Afterwards, 4) construct research questions to investigate the research problem. Identify variables to be accessed from the research questions 4 and make operational definitions of constructs from the research problem and questions. Thereafter, 5) construct specific deductive or inductive predictions in the form of hypotheses. 4 Finally, 6) state the study aims . This general flow for constructing effective research questions and hypotheses prior to conducting research is shown in Fig. 1 .

Research questions are used more frequently in qualitative research than objectives or hypotheses. 3 These questions seek to discover, understand, explore or describe experiences by asking “What” or “How.” The questions are open-ended to elicit a description rather than to relate variables or compare groups. The questions are continually reviewed, reformulated, and changed during the qualitative study. 3 Research questions are also used more frequently in survey projects than hypotheses in experiments in quantitative research to compare variables and their relationships.
Hypotheses are constructed based on the variables identified and as an if-then statement, following the template, ‘If a specific action is taken, then a certain outcome is expected.’ At this stage, some ideas regarding expectations from the research to be conducted must be drawn. 18 Then, the variables to be manipulated (independent) and influenced (dependent) are defined. 4 Thereafter, the hypothesis is stated and refined, and reproducible data tailored to the hypothesis are identified, collected, and analyzed. 4 The hypotheses must be testable and specific, 18 and should describe the variables and their relationships, the specific group being studied, and the predicted research outcome. 18 Hypotheses construction involves a testable proposition to be deduced from theory, and independent and dependent variables to be separated and measured separately. 3 Therefore, good hypotheses must be based on good research questions constructed at the start of a study or trial. 12
In summary, research questions are constructed after establishing the background of the study. Hypotheses are then developed based on the research questions. Thus, it is crucial to have excellent research questions to generate superior hypotheses. In turn, these would determine the research objectives and the design of the study, and ultimately, the outcome of the research. 12 Algorithms for building research questions and hypotheses are shown in Fig. 2 for quantitative research and in Fig. 3 for qualitative research.

EXAMPLES OF RESEARCH QUESTIONS FROM PUBLISHED ARTICLES
- EXAMPLE 1. Descriptive research question (quantitative research)
- - Presents research variables to be assessed (distinct phenotypes and subphenotypes)
- “BACKGROUND: Since COVID-19 was identified, its clinical and biological heterogeneity has been recognized. Identifying COVID-19 phenotypes might help guide basic, clinical, and translational research efforts.
- RESEARCH QUESTION: Does the clinical spectrum of patients with COVID-19 contain distinct phenotypes and subphenotypes? ” 19
- EXAMPLE 2. Relationship research question (quantitative research)
- - Shows interactions between dependent variable (static postural control) and independent variable (peripheral visual field loss)
- “Background: Integration of visual, vestibular, and proprioceptive sensations contributes to postural control. People with peripheral visual field loss have serious postural instability. However, the directional specificity of postural stability and sensory reweighting caused by gradual peripheral visual field loss remain unclear.
- Research question: What are the effects of peripheral visual field loss on static postural control ?” 20
- EXAMPLE 3. Comparative research question (quantitative research)
- - Clarifies the difference among groups with an outcome variable (patients enrolled in COMPERA with moderate PH or severe PH in COPD) and another group without the outcome variable (patients with idiopathic pulmonary arterial hypertension (IPAH))
- “BACKGROUND: Pulmonary hypertension (PH) in COPD is a poorly investigated clinical condition.
- RESEARCH QUESTION: Which factors determine the outcome of PH in COPD?
- STUDY DESIGN AND METHODS: We analyzed the characteristics and outcome of patients enrolled in the Comparative, Prospective Registry of Newly Initiated Therapies for Pulmonary Hypertension (COMPERA) with moderate or severe PH in COPD as defined during the 6th PH World Symposium who received medical therapy for PH and compared them with patients with idiopathic pulmonary arterial hypertension (IPAH) .” 21
- EXAMPLE 4. Exploratory research question (qualitative research)
- - Explores areas that have not been fully investigated (perspectives of families and children who receive care in clinic-based child obesity treatment) to have a deeper understanding of the research problem
- “Problem: Interventions for children with obesity lead to only modest improvements in BMI and long-term outcomes, and data are limited on the perspectives of families of children with obesity in clinic-based treatment. This scoping review seeks to answer the question: What is known about the perspectives of families and children who receive care in clinic-based child obesity treatment? This review aims to explore the scope of perspectives reported by families of children with obesity who have received individualized outpatient clinic-based obesity treatment.” 22
- EXAMPLE 5. Relationship research question (quantitative research)
- - Defines interactions between dependent variable (use of ankle strategies) and independent variable (changes in muscle tone)
- “Background: To maintain an upright standing posture against external disturbances, the human body mainly employs two types of postural control strategies: “ankle strategy” and “hip strategy.” While it has been reported that the magnitude of the disturbance alters the use of postural control strategies, it has not been elucidated how the level of muscle tone, one of the crucial parameters of bodily function, determines the use of each strategy. We have previously confirmed using forward dynamics simulations of human musculoskeletal models that an increased muscle tone promotes the use of ankle strategies. The objective of the present study was to experimentally evaluate a hypothesis: an increased muscle tone promotes the use of ankle strategies. Research question: Do changes in the muscle tone affect the use of ankle strategies ?” 23
EXAMPLES OF HYPOTHESES IN PUBLISHED ARTICLES
- EXAMPLE 1. Working hypothesis (quantitative research)
- - A hypothesis that is initially accepted for further research to produce a feasible theory
- “As fever may have benefit in shortening the duration of viral illness, it is plausible to hypothesize that the antipyretic efficacy of ibuprofen may be hindering the benefits of a fever response when taken during the early stages of COVID-19 illness .” 24
- “In conclusion, it is plausible to hypothesize that the antipyretic efficacy of ibuprofen may be hindering the benefits of a fever response . The difference in perceived safety of these agents in COVID-19 illness could be related to the more potent efficacy to reduce fever with ibuprofen compared to acetaminophen. Compelling data on the benefit of fever warrant further research and review to determine when to treat or withhold ibuprofen for early stage fever for COVID-19 and other related viral illnesses .” 24
- EXAMPLE 2. Exploratory hypothesis (qualitative research)
- - Explores particular areas deeper to clarify subjective experience and develop a formal hypothesis potentially testable in a future quantitative approach
- “We hypothesized that when thinking about a past experience of help-seeking, a self distancing prompt would cause increased help-seeking intentions and more favorable help-seeking outcome expectations .” 25
- “Conclusion
- Although a priori hypotheses were not supported, further research is warranted as results indicate the potential for using self-distancing approaches to increasing help-seeking among some people with depressive symptomatology.” 25
- EXAMPLE 3. Hypothesis-generating research to establish a framework for hypothesis testing (qualitative research)
- “We hypothesize that compassionate care is beneficial for patients (better outcomes), healthcare systems and payers (lower costs), and healthcare providers (lower burnout). ” 26
- Compassionomics is the branch of knowledge and scientific study of the effects of compassionate healthcare. Our main hypotheses are that compassionate healthcare is beneficial for (1) patients, by improving clinical outcomes, (2) healthcare systems and payers, by supporting financial sustainability, and (3) HCPs, by lowering burnout and promoting resilience and well-being. The purpose of this paper is to establish a scientific framework for testing the hypotheses above . If these hypotheses are confirmed through rigorous research, compassionomics will belong in the science of evidence-based medicine, with major implications for all healthcare domains.” 26
- EXAMPLE 4. Statistical hypothesis (quantitative research)
- - An assumption is made about the relationship among several population characteristics ( gender differences in sociodemographic and clinical characteristics of adults with ADHD ). Validity is tested by statistical experiment or analysis ( chi-square test, Students t-test, and logistic regression analysis)
- “Our research investigated gender differences in sociodemographic and clinical characteristics of adults with ADHD in a Japanese clinical sample. Due to unique Japanese cultural ideals and expectations of women's behavior that are in opposition to ADHD symptoms, we hypothesized that women with ADHD experience more difficulties and present more dysfunctions than men . We tested the following hypotheses: first, women with ADHD have more comorbidities than men with ADHD; second, women with ADHD experience more social hardships than men, such as having less full-time employment and being more likely to be divorced.” 27
- “Statistical Analysis
- ( text omitted ) Between-gender comparisons were made using the chi-squared test for categorical variables and Students t-test for continuous variables…( text omitted ). A logistic regression analysis was performed for employment status, marital status, and comorbidity to evaluate the independent effects of gender on these dependent variables.” 27
EXAMPLES OF HYPOTHESIS AS WRITTEN IN PUBLISHED ARTICLES IN RELATION TO OTHER PARTS
- EXAMPLE 1. Background, hypotheses, and aims are provided
- “Pregnant women need skilled care during pregnancy and childbirth, but that skilled care is often delayed in some countries …( text omitted ). The focused antenatal care (FANC) model of WHO recommends that nurses provide information or counseling to all pregnant women …( text omitted ). Job aids are visual support materials that provide the right kind of information using graphics and words in a simple and yet effective manner. When nurses are not highly trained or have many work details to attend to, these job aids can serve as a content reminder for the nurses and can be used for educating their patients (Jennings, Yebadokpo, Affo, & Agbogbe, 2010) ( text omitted ). Importantly, additional evidence is needed to confirm how job aids can further improve the quality of ANC counseling by health workers in maternal care …( text omitted )” 28
- “ This has led us to hypothesize that the quality of ANC counseling would be better if supported by job aids. Consequently, a better quality of ANC counseling is expected to produce higher levels of awareness concerning the danger signs of pregnancy and a more favorable impression of the caring behavior of nurses .” 28
- “This study aimed to examine the differences in the responses of pregnant women to a job aid-supported intervention during ANC visit in terms of 1) their understanding of the danger signs of pregnancy and 2) their impression of the caring behaviors of nurses to pregnant women in rural Tanzania.” 28
- EXAMPLE 2. Background, hypotheses, and aims are provided
- “We conducted a two-arm randomized controlled trial (RCT) to evaluate and compare changes in salivary cortisol and oxytocin levels of first-time pregnant women between experimental and control groups. The women in the experimental group touched and held an infant for 30 min (experimental intervention protocol), whereas those in the control group watched a DVD movie of an infant (control intervention protocol). The primary outcome was salivary cortisol level and the secondary outcome was salivary oxytocin level.” 29
- “ We hypothesize that at 30 min after touching and holding an infant, the salivary cortisol level will significantly decrease and the salivary oxytocin level will increase in the experimental group compared with the control group .” 29
- EXAMPLE 3. Background, aim, and hypothesis are provided
- “In countries where the maternal mortality ratio remains high, antenatal education to increase Birth Preparedness and Complication Readiness (BPCR) is considered one of the top priorities [1]. BPCR includes birth plans during the antenatal period, such as the birthplace, birth attendant, transportation, health facility for complications, expenses, and birth materials, as well as family coordination to achieve such birth plans. In Tanzania, although increasing, only about half of all pregnant women attend an antenatal clinic more than four times [4]. Moreover, the information provided during antenatal care (ANC) is insufficient. In the resource-poor settings, antenatal group education is a potential approach because of the limited time for individual counseling at antenatal clinics.” 30
- “This study aimed to evaluate an antenatal group education program among pregnant women and their families with respect to birth-preparedness and maternal and infant outcomes in rural villages of Tanzania.” 30
- “ The study hypothesis was if Tanzanian pregnant women and their families received a family-oriented antenatal group education, they would (1) have a higher level of BPCR, (2) attend antenatal clinic four or more times, (3) give birth in a health facility, (4) have less complications of women at birth, and (5) have less complications and deaths of infants than those who did not receive the education .” 30
Research questions and hypotheses are crucial components to any type of research, whether quantitative or qualitative. These questions should be developed at the very beginning of the study. Excellent research questions lead to superior hypotheses, which, like a compass, set the direction of research, and can often determine the successful conduct of the study. Many research studies have floundered because the development of research questions and subsequent hypotheses was not given the thought and meticulous attention needed. The development of research questions and hypotheses is an iterative process based on extensive knowledge of the literature and insightful grasp of the knowledge gap. Focused, concise, and specific research questions provide a strong foundation for constructing hypotheses which serve as formal predictions about the research outcomes. Research questions and hypotheses are crucial elements of research that should not be overlooked. They should be carefully thought of and constructed when planning research. This avoids unethical studies and poor outcomes by defining well-founded objectives that determine the design, course, and outcome of the study.
Disclosure: The authors have no potential conflicts of interest to disclose.
Author Contributions:
- Conceptualization: Barroga E, Matanguihan GJ.
- Methodology: Barroga E, Matanguihan GJ.
- Writing - original draft: Barroga E, Matanguihan GJ.
- Writing - review & editing: Barroga E, Matanguihan GJ.
Science and the scientific method: Definitions and examples
Here's a look at the foundation of doing science — the scientific method.
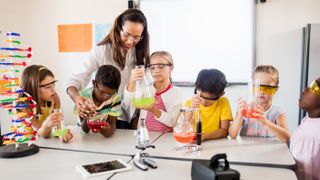
The scientific method
Hypothesis, theory and law, a brief history of science, additional resources, bibliography.
Science is a systematic and logical approach to discovering how things in the universe work. It is also the body of knowledge accumulated through the discoveries about all the things in the universe.
The word "science" is derived from the Latin word "scientia," which means knowledge based on demonstrable and reproducible data, according to the Merriam-Webster dictionary . True to this definition, science aims for measurable results through testing and analysis, a process known as the scientific method. Science is based on fact, not opinion or preferences. The process of science is designed to challenge ideas through research. One important aspect of the scientific process is that it focuses only on the natural world, according to the University of California, Berkeley . Anything that is considered supernatural, or beyond physical reality, does not fit into the definition of science.
When conducting research, scientists use the scientific method to collect measurable, empirical evidence in an experiment related to a hypothesis (often in the form of an if/then statement) that is designed to support or contradict a scientific theory .
"As a field biologist, my favorite part of the scientific method is being in the field collecting the data," Jaime Tanner, a professor of biology at Marlboro College, told Live Science. "But what really makes that fun is knowing that you are trying to answer an interesting question. So the first step in identifying questions and generating possible answers (hypotheses) is also very important and is a creative process. Then once you collect the data you analyze it to see if your hypothesis is supported or not."
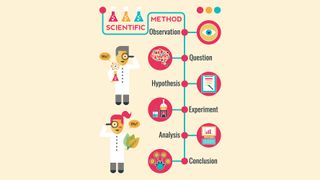
The steps of the scientific method go something like this, according to Highline College :
- Make an observation or observations.
- Form a hypothesis — a tentative description of what's been observed, and make predictions based on that hypothesis.
- Test the hypothesis and predictions in an experiment that can be reproduced.
- Analyze the data and draw conclusions; accept or reject the hypothesis or modify the hypothesis if necessary.
- Reproduce the experiment until there are no discrepancies between observations and theory. "Replication of methods and results is my favorite step in the scientific method," Moshe Pritsker, a former post-doctoral researcher at Harvard Medical School and CEO of JoVE, told Live Science. "The reproducibility of published experiments is the foundation of science. No reproducibility — no science."
Some key underpinnings to the scientific method:
- The hypothesis must be testable and falsifiable, according to North Carolina State University . Falsifiable means that there must be a possible negative answer to the hypothesis.
- Research must involve deductive reasoning and inductive reasoning . Deductive reasoning is the process of using true premises to reach a logical true conclusion while inductive reasoning uses observations to infer an explanation for those observations.
- An experiment should include a dependent variable (which does not change) and an independent variable (which does change), according to the University of California, Santa Barbara .
- An experiment should include an experimental group and a control group. The control group is what the experimental group is compared against, according to Britannica .
The process of generating and testing a hypothesis forms the backbone of the scientific method. When an idea has been confirmed over many experiments, it can be called a scientific theory. While a theory provides an explanation for a phenomenon, a scientific law provides a description of a phenomenon, according to The University of Waikato . One example would be the law of conservation of energy, which is the first law of thermodynamics that says that energy can neither be created nor destroyed.
A law describes an observed phenomenon, but it doesn't explain why the phenomenon exists or what causes it. "In science, laws are a starting place," said Peter Coppinger, an associate professor of biology and biomedical engineering at the Rose-Hulman Institute of Technology. "From there, scientists can then ask the questions, 'Why and how?'"
Laws are generally considered to be without exception, though some laws have been modified over time after further testing found discrepancies. For instance, Newton's laws of motion describe everything we've observed in the macroscopic world, but they break down at the subatomic level.
This does not mean theories are not meaningful. For a hypothesis to become a theory, scientists must conduct rigorous testing, typically across multiple disciplines by separate groups of scientists. Saying something is "just a theory" confuses the scientific definition of "theory" with the layperson's definition. To most people a theory is a hunch. In science, a theory is the framework for observations and facts, Tanner told Live Science.
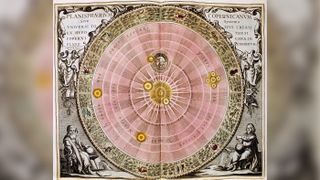
The earliest evidence of science can be found as far back as records exist. Early tablets contain numerals and information about the solar system , which were derived by using careful observation, prediction and testing of those predictions. Science became decidedly more "scientific" over time, however.
1200s: Robert Grosseteste developed the framework for the proper methods of modern scientific experimentation, according to the Stanford Encyclopedia of Philosophy. His works included the principle that an inquiry must be based on measurable evidence that is confirmed through testing.
1400s: Leonardo da Vinci began his notebooks in pursuit of evidence that the human body is microcosmic. The artist, scientist and mathematician also gathered information about optics and hydrodynamics.
1500s: Nicolaus Copernicus advanced the understanding of the solar system with his discovery of heliocentrism. This is a model in which Earth and the other planets revolve around the sun, which is the center of the solar system.
1600s: Johannes Kepler built upon those observations with his laws of planetary motion. Galileo Galilei improved on a new invention, the telescope, and used it to study the sun and planets. The 1600s also saw advancements in the study of physics as Isaac Newton developed his laws of motion.
1700s: Benjamin Franklin discovered that lightning is electrical. He also contributed to the study of oceanography and meteorology. The understanding of chemistry also evolved during this century as Antoine Lavoisier, dubbed the father of modern chemistry , developed the law of conservation of mass.
1800s: Milestones included Alessandro Volta's discoveries regarding electrochemical series, which led to the invention of the battery. John Dalton also introduced atomic theory, which stated that all matter is composed of atoms that combine to form molecules. The basis of modern study of genetics advanced as Gregor Mendel unveiled his laws of inheritance. Later in the century, Wilhelm Conrad Röntgen discovered X-rays , while George Ohm's law provided the basis for understanding how to harness electrical charges.
1900s: The discoveries of Albert Einstein , who is best known for his theory of relativity, dominated the beginning of the 20th century. Einstein's theory of relativity is actually two separate theories. His special theory of relativity, which he outlined in a 1905 paper, " The Electrodynamics of Moving Bodies ," concluded that time must change according to the speed of a moving object relative to the frame of reference of an observer. His second theory of general relativity, which he published as " The Foundation of the General Theory of Relativity ," advanced the idea that matter causes space to curve.
In 1952, Jonas Salk developed the polio vaccine , which reduced the incidence of polio in the United States by nearly 90%, according to Britannica . The following year, James D. Watson and Francis Crick discovered the structure of DNA , which is a double helix formed by base pairs attached to a sugar-phosphate backbone, according to the National Human Genome Research Institute .
2000s: The 21st century saw the first draft of the human genome completed, leading to a greater understanding of DNA. This advanced the study of genetics, its role in human biology and its use as a predictor of diseases and other disorders, according to the National Human Genome Research Institute .
- This video from City University of New York delves into the basics of what defines science.
- Learn about what makes science science in this book excerpt from Washington State University .
- This resource from the University of Michigan — Flint explains how to design your own scientific study.
Merriam-Webster Dictionary, Scientia. 2022. https://www.merriam-webster.com/dictionary/scientia
University of California, Berkeley, "Understanding Science: An Overview." 2022. https://undsci.berkeley.edu/article/0_0_0/intro_01
Highline College, "Scientific method." July 12, 2015. https://people.highline.edu/iglozman/classes/astronotes/scimeth.htm
North Carolina State University, "Science Scripts." https://projects.ncsu.edu/project/bio183de/Black/science/science_scripts.html
University of California, Santa Barbara. "What is an Independent variable?" October 31,2017. http://scienceline.ucsb.edu/getkey.php?key=6045
Encyclopedia Britannica, "Control group." May 14, 2020. https://www.britannica.com/science/control-group
The University of Waikato, "Scientific Hypothesis, Theories and Laws." https://sci.waikato.ac.nz/evolution/Theories.shtml
Stanford Encyclopedia of Philosophy, Robert Grosseteste. May 3, 2019. https://plato.stanford.edu/entries/grosseteste/
Encyclopedia Britannica, "Jonas Salk." October 21, 2021. https://www.britannica.com/ biography /Jonas-Salk
National Human Genome Research Institute, "Phosphate Backbone." https://www.genome.gov/genetics-glossary/Phosphate-Backbone
National Human Genome Research Institute, "What is the Human Genome Project?" https://www.genome.gov/human-genome-project/What
Live Science contributor Ashley Hamer updated this article on Jan. 16, 2022.
Sign up for the Live Science daily newsletter now
Get the world’s most fascinating discoveries delivered straight to your inbox.
What's the difference between a rock and a mineral?
Earth from space: Mysterious, slow-spinning cloud 'cyclone' hugs the Iberian coast
4,000-year-old 'Seahenge' in UK was built to 'extend summer,' archaeologist suggests
Most Popular
- 2 Space photo of the week: James Webb and Chandra telescopes spot a 'lighthouse' pointed at Earth
- 3 1st Neuralink user describes highs and lows of living with Elon Musk's brain chip
- 4 James Webb telescope finds carbon at the dawn of the universe, challenging our understanding of when life could have emerged
- 5 Neanderthals and humans interbred 47,000 years ago for nearly 7,000 years, research suggests
- 2 Evidence of more than 200 survivors of Mount Vesuvius eruption discovered in ancient Roman records
- 3 7 potential 'alien megastructures' spotted in our galaxy are not what they seem
- 4 Hundreds of centuries-old coins unearthed in Germany likely belonged to wealthy 17th-century mayor
- 5 'Physics itself disappears': How theoretical physicist Thomas Hertog helped Stephen Hawking produce his final, most radical theory of everything

Where to Find The Hypothesis in a Research Article
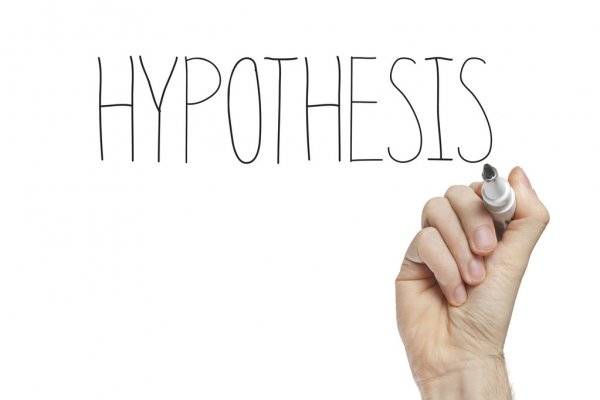
The question of “Where to Find The Hypothesis in a Research Article” can only be answered by exploring how research articles represent scientific methods.
Table of Contents
Introduction
A research article represents a compilation of information by a scientist concerning an original research idea. It is characterized by a wide range of information including, the purpose of the study, the thesis statement, hypothesis, literature review, methodology, results and conclusion.
The examination of a research article is an important process, and the ability to identify crucial elements of research is paramount for the effective analysis of a research article.
Research articles are usually arranged in specific ways. A hypothesis in a research article is usually located in a specific position in an article. The ability to quickly pinpoint where the hypothesis is located is crucial in becoming an expert in exploring research articles as well as formulating them.
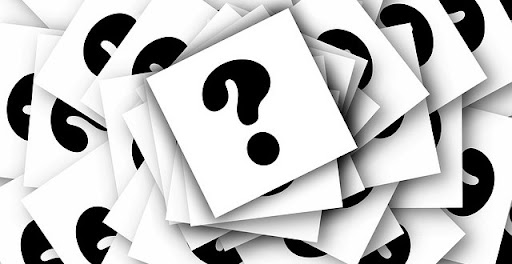
What is a hypothesis
A hypothesis represents a scientific guess that is stated in research. It is a speculative statement concerning the relationship between two or more variables in research.
Therefore, a good hypothesis is a prediction that is testable, specific, and explores what a researcher expects to find in the study.
Formulating a Hypothesis
The creation of a hypothesis represents a critical part of the scientific method. Formulating a hypothesis is important, especially when testing a theory. Most scientific research involves testing theories. Theories, in this case, refer to ideas about the way things relate to one another. For one to formulate a hypothesis to be used in research, they have to be to predict the outcome of the research.
If one cannot predict the outcome, then the research does not need the formulating of a hypothesis because it is either exploratory or descriptive. These forms of research cannot have a hypothesis, and the reason is that there is a limited base of knowledge concerning the subject matter for the prediction of the outcome to be possible.
A good Hypothesis
A good hypothesis has to have two or more variables. These variables have to be measurable or have the potential to be measured. The hypothesis also has to specify how the variables are related to one another.
Where to Find the Hypothesis in a Research Article
The scientific method is characterized by several steps. They include:
- Coming up with a question or the problem that needs to be solved.
- Conducting background research on the problem
- Formulating a hypothesis
- Establishing how the research will be conducted using a research design
- Collecting data
- Analyzing the data and coming up with results
- Provide conclusions
- Presenting the information through a research article.
Based on the above structure, it is evident that the hypothesis is located in the introduction section of a research article. One should look out for “if-then” statements. However, for such statements to be hypotheses, they need to:
- Demonstrates the relationship between variables,
- The relationship needs to be testable and
- The prediction needs to be measurable
A hypothesis is not always clearly labeled. This means that the statement can appear in different forms apart from when formulated using the “if-then” statements. One should, therefore, look out for a statement that offers a prediction of what readers need to expect from the research.
The ability to identify where to find a hypothesis is located in a research article is very important in several ways:
- One can quickly know what the researcher wants to prove using the research.
- It makes individuals effective in reading research articles.
- It enhances an individual’s ability to formulate their own hypothesis when conducting research
Entrust your assignments to our expert writers for excellent results!
Eagle Academics prides itself in providing quality custom papers for many students around the world. We guarantee exceptional standards, and this is based on the selection of highly professional and experienced tutors and writers in different fields. Our experts can handle any work regardless of the deadline.
You must choose what you do with your time. Let our experts help you with your schoolwork while you focus on other essential things in your life. Talk to us, and we will give you the help that you need.
Calculate the price of your paper
Free features.
- Formatting (APA, MLA, Harvard, Chicago/Turabian)
- Bibliography
- Upload custom grading criteria
Additional services
- Part-by-part payment
- Links to used sources
- Review your writer’s samples
- Charts and PowerPoint slides
Don’t look far for academic help. We have you covered
Money back guarantee.
Our goal is to give the best services to our clients. However, if for one reason or the other a client is not satisfied, we will refund your money. So the funds paid to us are safe.
Confidentiality
We value our clients’ privacy. No single information of our clients can be shared by third parties.
Our service is legit
Our services follow the laid down academic guidelines. We cannot blackmail our clients, but only strive to offer them the best academic assistance they need.
Get a plagiarism-free paper
Our writers work hand in hand with the quality assurance department to ensure whatever paper you get is totally plagiarism-free.
We can help with urgent tasks
We have standby writers who can handle papers even within 6 hours. Just get in touch and let us know.
Pay a fair price
We have competitive prices that are pocket friendly. In addition, we have plenty of discounts to offer.
Need a better grade? We've got you covered.
May 30, 2024
A general passion
Image credit: pgen.1011291
research article
Conserved signalling functions for Mps1, Mad1 and Mad2 in the Cryptococcus neoformans spindle checkpoint
Mps1-dependent phosphorylation of C-terminal Mad1 residues is a critical step in Cryptococcus spindle checkpoint signalling.
Image credit: pgen.1011302
Recently Published Articles
- Growth deficiency in a mouse model of Kabuki syndrome 2 bears mechanistic similarities to Kabuki syndrome 1
- Streptococcus mitis and Streptococcus pneumoniae leads to higher genetic diversity within rather than between human populations">Long-term evolution of Streptococcus mitis and Streptococcus pneumoniae leads to higher genetic diversity within rather than between human populations
- LINC03045 regulating glioblastoma invasion">CRISPRi screen of long non-coding RNAs identifies LINC03045 regulating glioblastoma invasion
Current Issue May 2024
Adaptations to nitrogen availability drive ecological divergence of chemosynthetic symbionts
The importance of nitrogen availability in driving the ecological diversification of chemosynthetic symbiont species and the role that bacterial symbionts may play in the adaptation of marine organisms to changing environmental conditions.
Image credit: pgen.1011295
Paramutation at the maize pl1 locus is associated with RdDM activity at distal tandem repeats
pl1 paramutation depends on trans-chromosomal RNA-directed DNA methylation operating at a discrete cis-linked and copy-number-dependent transcriptional regulatory element.
Image credit: pgen.1011296
Research Article
Genomic analyses of Symbiomonas scintillans show no evidence for endosymbiotic bacteria but does reveal the presence of giant viruses
A multi-gene tree showed the three SsV genome types branched within highly supported clades with each of BpV2, OlVs, and MpVs, respectively.
Image credit: pgen.1011218
A natural bacterial pathogen of C . elegans uses a small RNA to induce transgenerational inheritance of learned avoidance
A mechanism of learning and remembering pathogen avoidance likely happens in the wild.
Image credit: pgen.1011178
Spoink , a LTR retrotransposon, invaded D. melanogaster populations in the 1990s
Evidence of Spoink retrotransposon's horizontal transfer into D. melanogaster populations post-1993, suggesting its origin from D.willistoni .
Image credit: pgen.1011201
Comparison of clinical geneticist and computer visual attention in assessing genetic conditions
Understanding AI, specifically Deep Learning, in facial diagnostics for genetic conditions can enhance the design and utilization of AI tools.
Image credit: pgen.1011168
Maintenance of proteostasis by Drosophila Rer1 is essential for competitive cell survival and Myc-driven overgrowth
Loss of Rer1 induces proteotoxic stress, leading to cell competition and elimination ...
Image credit: pgen.1011171
Anthracyclines induce cardiotoxicity through a shared gene expression response signature
TOP2i induce thousands of shared gene expression changes in cardiomyocytes.
Image credit: pgen.1011164
New PLOS journals accepting submissions
Five new journals unified in addressing global health and environmental challenges are now ready to receive submissions: PLOS Climate , PLOS Sustainability and Transformation , PLOS Water , PLOS Digital Health , and PLOS Global Public Health
COVID-19 Collection
The COVID-19 Collection highlights all content published across the PLOS journals relating to the COVID-19 pandemic.
Submit your Lab and Study Protocols to PLOS ONE !
PLOS ONE is now accepting submissions of Lab Protocols, a peer-reviewed article collaboration with protocols.io, and Study Protocols, an article that credits the work done prior to producing and publishing results.
PLOS Reviewer Center
A collection of free training and resources for peer reviewers of PLOS journals—and for the peer review community more broadly—drawn from research and interviews with staff editors, editorial board members, and experienced reviewers.
Ten Simple Rules
PLOS Computational Biology 's "Ten Simple Rules" articles provide quick, concentrated guides for mastering some of the professional challenges research scientists face in their careers.
Welcome New Associate Editors!
PLOS Genetics welcomes several new Associate Editors to our board: Nicolas Bierne, Julie Simpson, Yun Li, Hongbin Ji, Hongbing Zhang, Bertrand Servin, & Benjamin Schwessinger
Expanding human variation at PLOS Genetics
The former Natural Variation section at PLOS Genetics relaunches as Human Genetic Variation and Disease. Read the editors' reasoning behind this change.
PLOS Genetics welcomes new Section Editors
Quanjiang Ji (ShanghaiTech University) joined the editorial board and Xiaofeng Zhu (Case Western Reserve University) was promoted as new Section Editors for the PLOS Genetics Methods section.
PLOS Genetics editors elected to National Academy of Sciences
Congratulations to Associate Editor Michael Lichten and Consulting Editor Nicole King, who are newly elected members of the National Academy of Sciences.
Harmit Malik receives Novitski Prize
Congratulations to Associate Editor Harmit Malik, who was awarded the Edward Novitski Prize by the Genetics Society of America for his work on genetic conflict. Harmit has also been elected as a new member of the American Academy of Arts & Sciences.
Publish with PLOS
- Submission Instructions
- Submit Your Manuscript
Connect with Us
- PLOS Genetics on Twitter
- PLOS on Facebook
Get new content from PLOS Genetics in your inbox
Thank you you have successfully subscribed to the plos genetics newsletter., sorry, an error occurred while sending your subscription. please try again later..
- Open access
- Published: 13 June 2024
Dyport: dynamic importance-based biomedical hypothesis generation benchmarking technique
- Ilya Tyagin 1 &
- Ilya Safro 2
BMC Bioinformatics volume 25 , Article number: 213 ( 2024 ) Cite this article
Metrics details
Automated hypothesis generation (HG) focuses on uncovering hidden connections within the extensive information that is publicly available. This domain has become increasingly popular, thanks to modern machine learning algorithms. However, the automated evaluation of HG systems is still an open problem, especially on a larger scale.
This paper presents a novel benchmarking framework Dyport for evaluating biomedical hypothesis generation systems. Utilizing curated datasets, our approach tests these systems under realistic conditions, enhancing the relevance of our evaluations. We integrate knowledge from the curated databases into a dynamic graph, accompanied by a method to quantify discovery importance. This not only assesses hypotheses accuracy but also their potential impact in biomedical research which significantly extends traditional link prediction benchmarks. Applicability of our benchmarking process is demonstrated on several link prediction systems applied on biomedical semantic knowledge graphs. Being flexible, our benchmarking system is designed for broad application in hypothesis generation quality verification, aiming to expand the scope of scientific discovery within the biomedical research community.
Conclusions
Dyport is an open-source benchmarking framework designed for biomedical hypothesis generation systems evaluation, which takes into account knowledge dynamics, semantics and impact. All code and datasets are available at: https://github.com/IlyaTyagin/Dyport .
Peer Review reports
Introduction
Automated hypothesis generation (HG, also known as Literature Based Discovery, LBD) has gone a long way since its establishment in 1986, when Swanson introduced the concept of “Undiscovered Public Knowledge” [ 1 ]. It pertains to the idea that within the public domain, there is a significant abundance of information, allowing for the uncovering of implicit connections among various pieces of information. There are many systems developed throughout the years, which incorporate different reasoning methods: from concept co-occurrence in scientific literature [ 2 , 3 ] to the advanced deep learning-based algorithms and generative models (such as BioGPT [ 4 ] and CBAG [ 5 ]). Examples include but are not limited to probabilistic topic modeling over relevant papers [ 6 ], semantic inference [ 7 ], association rule discovery [ 8 ], latent semantic indexing [ 9 ], semantic knowledge network completion [ 10 ] or human-aware artificial intelligence [ 11 ] to mention just a few. The common thread running through these lines of research is that they are all meant to fill in the gaps between pieces of existing knowledge.
The evaluation of HG is still one of the major problems of these systems, especially when it comes to fully automated large-scale general purpose systems (such as IBM Watson Drug Discovery [ 12 ], AGATHA [ 10 ] or BioGPT [ 4 ]). For these, a massive assessment (that is normal in the machine learning and general AI domains) performed manually by the domain experts is usually not feasible and other methods are required.
One traditional evaluation approach is to make a system “rediscover” some of the landmark findings, similar to what was done in numerous works replicating well-known connections, such as: Fish Oil \(\leftrightarrow\) Raynaud’s Syndrome [ 13 ], Migraine \(\leftrightarrow\) Magnesium [ 13 ] or Alzheimer \(\leftrightarrow\) Estrogen [ 14 ]. This technique is frequently used even in a majority of the recently published papers, despite of its obvious drawbacks, such as very limited number of validation samples and their general obsolesce (some of these connections are over 30 years old). Furthermore, in some of these works, the training set is not carefully chosen to include only the information published prior the discovery of interest which turns the HG goal into the information retrieval task.
Another commonly used technique is based on the time-slicing [ 10 , 15 ], when a system is trained on a subset of data prior to a specified cut-off date and then evaluated on the data from the future. This method addresses the weaknesses of previous approach and can be automated, but it does not immediately answer the question of how significant or impactful the connections are. The lack of this information may lead to deceiving results: many connections, even recently published, are trivial (especially if they are found by the text mining methods) and do not advance the scientific field in a meaningful way.
A related area that faces similar evaluation challenges is Information Extraction (IE), a field crucial to enabling effective HG by identifying and categorizing relevant information in publicly available data sources. Within the realm of biomedical and life sciences IE, there are more targeted, small-scale evaluation protocols such as the BioCreative competitions [ 16 ], where the domain experts provide curated training and test datasets, which allows participants to refine and assess their systems within a controlled environment. While such targeted evaluations as conducted in BioCreative are both crucial and insightful, they inherently lack the scope and scale needed for the evaluation of expansive HG systems.
The aforementioned issues emphasize the critical need for research into effective, scalable evaluation methods in automated hypothesis generation. Our primary interest is in establishing an effective and sustainable benchmark for large-scale, general-purpose automated hypothesis generation systems within the biomedical domain. We seek to identify substantial, non-trivial insights, prioritizing them over mere data volume and ensuring scalability with respect to ever-expanding biocurated knowledge databases. We emphasize the significance of implementing sustainable evaluation strategies, relying on constantly updated datasets reflecting the latest research. Lastly, our efforts are targeted towards distinguishing between hypotheses with significant impact and those with lesser relevance, thus moving beyond trivial generation of hypotheses to ensuring their meaningful contribution to scientific discovery.
Our contribution
We propose a high quality benchmark dataset Dyport for hypothesis prediction systems evaluation. It incorporates information extracted from a number of biocurated databases. We normalize all concepts to the unified format for seamless integration and each connection is supplied with rich metadata, including timestamp information to enable time-slicing.
We introduce an evaluation method for the impact of connections in time-slicing paradigm. It will allow to benchmark HG systems more thoroughly and extensively by assigning an importance weight to every connection over the time. This weight represents the overall impact a connection makes on future discoveries.
We demonstrate the computational results of several prediction algorithms using the proposed benchmark and discuss their performance and quality.
We propose to use our benchmark to evaluate the quality of HG systems. The benchmark is designed to be updated on a yearly basis. Its structure facilitates relatively effortless expansion and reconfiguration by users and developers.
Background and related work
Unfortunately, the evaluation in the hypothesis generation field is often coupled with the systems to evaluate and currently not universally standardized. If one would like to compare the performance of two or more systems, they need to understand their training protocol to instantiate models from scratch and then test them on the same data they used in their experiment.
This problem is well known and there are attempts to provide a universal way to evaluate such systems. For example, OpenBioLink [ 17 ] is designed as a software package for evaluation of link prediction models. It supports time-slicing and contains millions of edges with different quality settings. The authors describe it as “highly challenging” dataset that does not include “trivially predictable” connections, but they do not provide a quantification of difficulty nor range the edges accordingly.
Another attempt to set up a large-scale validation of HG systems was performed in our earlier work [ 18 ]. The proposed methodology is based on the semantic triples extracted from SemMedDB [ 19 ] database and setting up a cut date for training and testing. Triples are converted to pairs by removing the “verb” part from each (subject-verb-object) triple. For the test data, a list of “highly cited” pairs is identified, which is based on the citation counts from SemMedDB, MEDLINE and Semantic Scholar. Only connections occurring in papers published after the cut date and cited over 100 times are considered. It is worth mentioning that this approach is prone to noise (due to SemMedDB text mining methods) and also skewed towards the discoveries published closer to the cut-date, since the citations accumulate over time.
One more aspect of the proposed approach relates to the quantification and detection of scientific novelty. Research efforts range from protein design domain studies [ 20 ] to analyzing scientific publications through their titles [ 21 ] or using manual curation in combination with machine learning [ 22 ]. However, none of these techniques were integrated into a general purpose biomedical evaluation framework, where the novelty would be taken into account.
Currently, Knowledge Graph Embeddings (KGE) are becoming increasingly popular and the hypothesis generation problem can be formulated in terms of link prediction in knowledge graphs. Knowledge Graphs often evaluate the likelihood of a particular connection with the scoring function of choice. For example, TransE [ 23 ] evaluates each sample with the following equation:
where h is the embedding vector of a head entity, r is the embedding vector of relation, t is the embedding vector of a tail entity and \(||\cdot ||\) denotes the L1 or L2 norm.
These days KGE-based models are of interest to the broad scientific community, including researchers in the drug discovery field. Recently they carefully investigated the factors affecting the performance of KGE models [ 24 ] and reviewed biomedical databases related to drug discovery [ 25 ]. These publications, however, do not focus on any temporal information nor attempt to describe the extracted concept associations quantitatively. We also aim to fill in this currently existing gap in our current work.
\(c_i\) —concept in some arbitrary vocabulary;
\(m(\cdot )\) —function that maps a concept \(c_i\) to the subset of corresponding UMLS CUI. The result is denoted by \(m_i =m(c_i)\) . The \(m_i\) is not necessarily a singleton. We will somewhat abuse the notation by denoting \(m_i\) a single or any of the UMLS terms obtained by mapping \(c_i\) to UMLS.
\(m(\cdot ,\cdot )\) —function that maps pairs of \(c_i\) and \(c_j\) into the corresponding set of all possible UMLS pairs \(m_i\) and \(m_j\) . Recall that the mapping of \(c_i\) to UMLS may not be unique. In this case \(|m(c_i,c_j)| = |m(c_i)|\cdot |m(c_j)|\) .
\((m_i, m_j)\) —a pair of UMLS CUIs, which is extracted as a co-occurrence from MEDLINE records. It also represents an edge in network G and is cross-referenced with biocurated databases;
D —set of pairs \((m_i, m_j)\) extracted from biocurated databases;
P —set of pairs \((m_i, m_j)\) extracted from MEDLINE abstracts;
E —set of cross-referenced pairs \((m_i, m_j)\) , such that \(E = D \cap P\) ;
G —dynamic network, containing temporal snapshots \(G_t\) , where t —timestamp (year);
\(\hat{G}_t\) —snapshot of network G for a timestamp t only containing nodes from \(G_{t-1}\) .
The main unit of analysis in HG is a connection between two biomedical concepts, which we also refer to as “pair”, “pairwise interaction” or “edge” (in network science context when we will be discussing semantic networks). These connections can be obtained from two main sources: biomedical databases and scientific texts. Extracting pairs from biomedical databases is done with respect to the nature and content of the database: some of them already contain pairwise interactions, whereas others focus on more complex structures such as pathways which may contain multiple pairwise interactions or motifs (e.g., KEGG [ 26 ]). Extracting pairs from textual data is done via information retrieval methods, such as relation extraction or co-occurrence mining. In this work, we use the abstract-based co-occurrence approach, which is explained later in the paper.
Method in summary
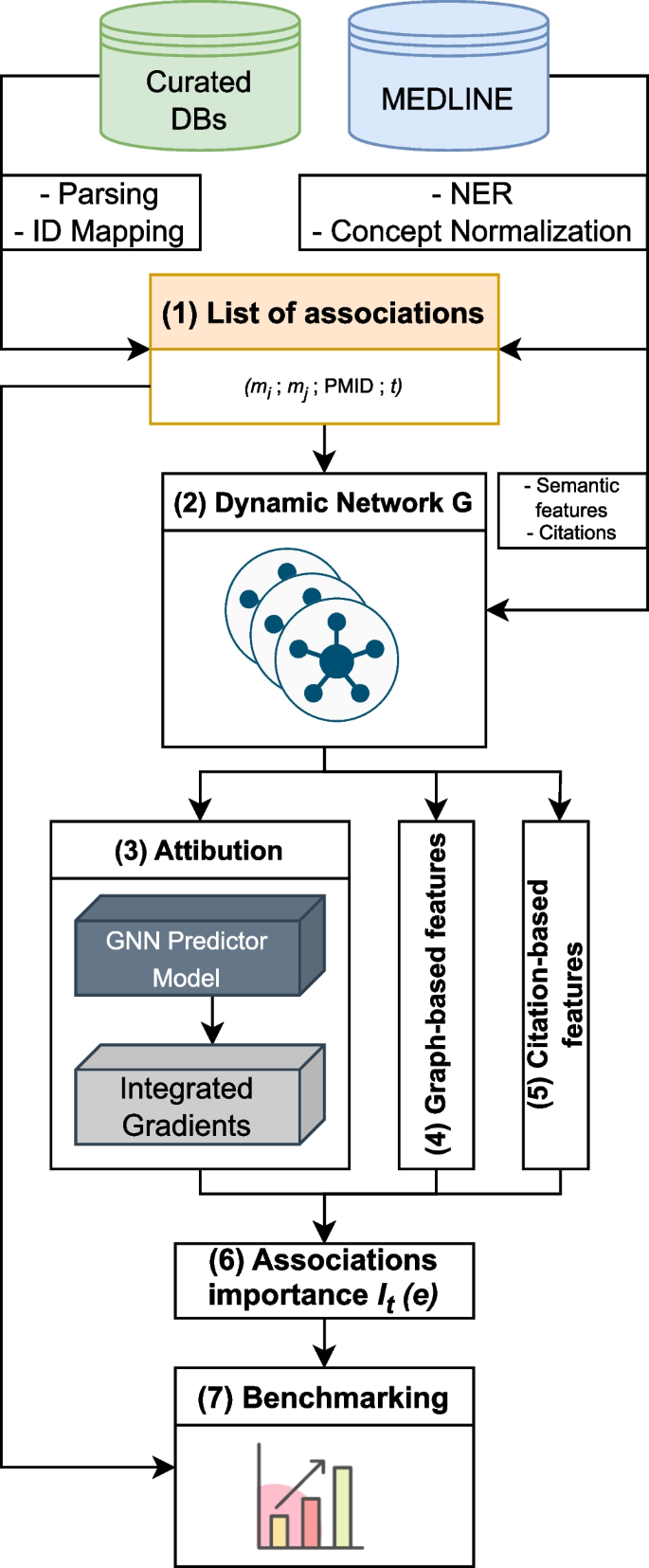
Summary of the HG benchmarking approach. We start with collecting data from Curated DBs and Medline, then process it: records from Curated DBs go through parsing, cleaning and ID mapping, MEDLINE records are fed into SemRep system, which performs NER and concept normalization. After that we obtain a list of UMLS CUI associations with attached PMIDs and timestamps (TS). This data is then used to construct a dynamic network G , which is used to calculate the importance measure I for edges in the network. At the end, edges \(e \in G\) with their corresponding importance scores \(I_t(e)\) are added to the benchmark dataset
The HG benchmarking pipeline is presented in Fig. 1 . The end goal of the pipeline is to provide a way to evaluate any end-to-end hypothesis generation system trained to predict potential pairwise associations between biomedical instances or concepts.
We start with collecting pairwise entity associations from a list of biocurated databases, which we then normalize and represent as pairs of UMLS [ 27 ] terms \((m_i, m_j)\) . The set of these associations is then cross-referenced with scientific abstracts extracted from MEDLINE database, such that for each pair \((m_i, m_j)\) we keep all PubMed identifiers (PMID) that correspond to the paper abstracts in which \(m_i\) and \(m_j\) co-occured. As a result, there is a list of tuples (step 1, Fig. 1 ) \((m_i, m_j, \text {PMID}, t)\) , where t is a timestamp for a given PMID extracted from its metadata. We then split this list into a sequence \(\{E_t\}\) according to the timestamp t . In this work t is taken with a yearly resolution.
Each individual \(E_t\) can be treated as an edgelist, which yields an edge-induced network \(G_t\) constructed from edges \((m_i, m_j) \in E_t\) . It gives us a sequence of networks \(G = \{G_t\}\) (step 2, Fig. 1 ), which is then used to compute the importance of individual associations in \(E_t\) with different methods.
The main goal of importance is to describe each edge from \(E_t\) using additional information. The majority of it comes from the future network snapshot \(G_{t+1}\) , which allows us to track the impact that a particular edge had on the network in the future. The predictive impact is calculated with an attribution technique called Integrated Gradients (IG) (step 3, Fig. 1 ). Structural impact is calculated with graph-based measures (such as centrality) (step 4, Fig. 1 ) and citation impact is calculated with respect to how frequently edges are referenced in the literature after their initial discovery (step 5, Fig. 1 ).
All the obtained scores are then merged together to obtain a ranking \(I_t(e)\) (step 6, Fig. 1 ), where \(e \in E_t\) for all edges from a snapshot \(G_t\) . Finally, this ranking is used to perform stratified evaluation of how well hypothesis generation systems perform in discovering connections with different importance values (step 7, Fig. 1 ).
Databases processing and normalization
We begin by gathering the links and relationships from publicly available databases, curated by domain experts. We ensure that all pairwise concept associations we utilize are from curated sources. For databases like STRING, which compile associations from various channels with differing levels of confidence, we exclusively select associations derived from curated sources.
Ensuring correct correspondence of the same concepts from diverse databases is highly crucial. Therefore, we also conduct mapping of all concepts to UMLS CUI (Concept Unique Identifier). Concepts, which identifiers cannot be mapped to UMLS CUI, are dropped. In our process, we sometimes encounter situations where a concept \(c_{i}\) , may have multiple mappings to UMLS CUIs, i.e., \(|m_i|=k>1\) for \(m_i = m(c_i)\) . To capture these diverse mappings, we use the Cartesian product rule. In this approach, we take the mapping sets for both concepts \(c_{i}\) and \(c_{j}\) , denoted as \(m(c_{i})\) and \(m(c_{j})\) , and generate a new set of pairs encapsulating all possible combinations of these mappings. Essentially, for each original pair \((c_{i}, c_{j})\) , we produce a set of pairs \(m(c_{i}, c_{j})\) such that the cardinality of this new set equals the product of the cardinalities of the individual mappings. Let us say that \(c_i\) has k different UMLS mappings and \(c_j\) has s , then \(|m(c_{1},c_{2})| = |m(c_{1})| \cdot |m(c_{2})| = k\cdot s\) .
In other words, we ensure that every possible mapping of the original pair is accounted for, enabling our system to consider all potential pairwise interactions across all UMLS mappings. To this end, we have collected all pairs of UMLS CUI that are present in different datasets, forming a set D .
Processing MEDLINE records
To match pairwise interactions extracted from biocurated databases to literature, we use records from MEDLINE database with their PubMed identifiers. These records, primarily composed of the titles and abstracts of scientific papers, are each assigned a unique PubMed reference number (PMID). They are also supplemented with rich metadata, which includes information about authors, full-text links (when applicable), and date of publication timestamps indicating when the record became publicly available. We process records with an NLM-developed natural language processing tool SemRep [ 28 ] to perform named entity recognition, concept mapping and normalization. To this end, we obtain a list of UMLS CUI for each MEDLINE record.
Connecting database records with literature
The next step is to form connections between biocurated records and their corresponding mentions in the literature. With UMLS CUIs identified in the previous step, we track the instances where these CUIs are mentioned together within the same scientific abstract. Our method considers the simultaneous appearance of a pair of concepts, denoted as \(m_i\) and \(m_j\) , within a single abstract to represent a co-occurrence. This co-occurrence may indicate a potential relationship between the two concepts within the context of the abstract. All the co-occurring pairs \((m_i, m_j)\) , extracted from MEDLINE abstracts, form the set P .
No specific “significance” score is assigned to these co-occurrences at this point beyond their presence in the same abstract. Subsequently, these pairs are cross-referenced with pairs in biocurated databases. More specifically, for each co-occurrence \((m_i, m_j) \in P\) we check its presence in set D . Pairs not present in both sets D and P are discarded. This forms the set E :
This step validates each co-occurring pair, effectively reducing noise and confirming that each pair holds biological significance. Conversely, E can be described as a set of biologically relevant associations, with each element enriched by contextual information extracted from scientific literature. The procedure is described in [ 29 ] as distant supervised annotation .
Constructing time-sliced graphs
After we find the set of co-occurrences in abstracts extracted from MEDLINE and cross-referenced with pairs in biocurated databases (set E ), we split it based on the timestamps extracted from the abstracts metadata. The timestamps t are assigned to each PMID and are used to determine when they became publicly available. We use these timestamps to track how often was a pair of UMLS CUIs \((m_i, m_j)\) appearing in the biomedical literature over time. As a result, we have a list of biologically relevant cross-referenced UMLS CUI co-occurrences, each connected to all PMIDs containing them.
This list is then split into edge lists \(E_t\) , such that each edge list contains pairs \((m_i, m_j)\) added in or before year t . These edge lists are then transformed to dynamic network G with T snapshots:
where \(N_t\) and \(E_t\) represent the set of unique UMLS CUIs (nodes) and their cross-referenced abstract co-occurrences (edges), respectively, and t is the annual timestamp (time resolution can be changed as needed), such that \(G_{t}\) is constructed from all MEDLINE records published before t (e.g., \(t=2011\) ). All networks \(G_{t}\) are simple and undirected.
For each timestamp t , \(G_{t}\) represents a cumulative network, including all the information from \(G_{t-1}\) and new information added in year t .
Tracking the edge importance of time-sliced graphs
We enrich the proposed benchmarking strategy with the information about associations importance at each time step t . In the context of scientific discovery, the importance may be considered from several different perspectives, e.g., as an the influence of an individual finding on future discoveries. In this section we take three different perspectives into account and then combine them together to obtain a final importance score, which we later use to evaluate different hypothesis generation systems with respect to their ability to predict the important associations.
Integrated gradients pipeline
In this step we obtain the information about how edges from graph \(G_t\) influence the appearance of new edges in \(G_{t+1}\) . For that we train a machine learning model, which is able to perform link predictions and then we use an attribution method called Integrated Gradients (IG).
In general, IG is used to understand input features importance with respect to the output a given predictor model produces. In case of link prediction problem, a model outputs likelihood of two nodes \(m_i\) and \(m_j\) being connected for a given network \(G_t\) . The input features for a link prediction model will include the adjacency matrix of \(G_t\) , \(A_t\) , and the predictions themselves can be drawn from a list of edges appearing in the next timestamp \(t + 1\) . If IG is applied to this particular problem, it will provide attribution values for each element of \(A_t\) , which can be reformulated as the importance of edges existing at the timestamp t with respect to their contribution to predicting the edges added at the next timestamp \(t+1\) . This could be interpreted as the influence of current dynamic network structural elements on the information that will be added in future.
Link prediction problem In our setting, the link prediction problem is formulated as following:
We note that predictions of edges \(\hat{E}_{t+1}\) are performed only for nodes \(N_t\) from the graph \(G_t\) at year t .
Adding Node and Edge Features : To enrich the dynamic network G with non-redundant information extracted from text, we add node features and edge weights. Node features are required for Graph Neural Network-based predictor training, which we use in the proposed pipeline.
Node features : Node features are added to each \(G_t\) by applying word2vec algorithm [ 30 ] to the corresponding snapshot of MEDLINE dataset obtained for a timestamp t . In order to perform cleaning and normalization, we replace all tokens in the input texts by their corresponding UMLS CUIs obtained at the NER stage. It significantly reduces the vocabulary size, automatically removing stop-words and enabling vocabulary-guided phrase mining [ 31 ]. It is important to note that each node m has a different vector representation for each time stamp t , which we can refer to as n 2 v ( m , t ).
Edge features (weights) : For simplicity, edge weights are constructed by counting the number of MEDLINE records mentioning a pair of concepts \(e \in E_{t}\) . In other words, for each pair \(e = (m_i, m_j)\) we assign a weight representing the total number of mentions for a pair e in year t .
GNN training
We use a graph neural network-based encoder-decoder architecture. Its encoder consists of two graph convolutional layers [ 32 ] and produces an embedding for each graph node. Decoder takes the obtained node embeddings and outputs the sum of element-wise multiplication of encoded node representations as a characteristic of each pair of nodes.
Attribution
To obtain a connection between newly introduced edges \(\hat{E}_{t+1}\) and existing edges \(E_t\) , we use an attribution method Integrated Gradients (IG) [ 33 ]. It is based on two key assumptions:
Sensitivity: any change in input that affects the output gets a non-zero attribution;
Implementation Invariance: attribution is consistent with the model’s output, regardless of the model’s architecture.
The IG can be applied to a wide variety of ML models as it calculates the attribution scores with respect to input features and not the model weights/activations, which is important, because we focus on relationships between the data points and not the model internal structure.
The integrated gradient (IG) score along \(i^{th}\) dimension for an input x and baseline \(x'\) is defined as:
where \(\frac{\partial F(x)}{\partial x_i}\) is the gradient of F ( x ) along \(i^{th}\) dimension. In our case, input x is the adjacency matrix of \(G_t\) filled with 1 s as default values (we provide all edges \(E_t \in G_t\) ) and baseline \(x'\) is the matrix of zeroes. As a result, we obtain an adjacency matrix \(A(G_t)\) filled with attribution values for each edge \(E_t\) .
Graph-based measures
Betweenness Centrality In order to estimate the structural importance of selected edges, we calculate their betweenness centrality [ 34 ]. This importance measure shows the amount of information passing through the edges, therefore indicating their influence over the information flow in the network. It is defined as
where \(\sigma _{st}\) —the number of shortest paths between nodes s and t ; \(\sigma _{st}(e)\) —the number of shortest paths between nodes s and t passing through edge e .
To calculate the betweenness centrality with respect to the future connections, we restrict the set of vertices V to only those, that are involved in future connections we would like to use for explanation.
Eigenvector Centrality Another graph-based structural importance metric we use is the eigenvector centrality. The intuition behind it is that a node of the network is considered important if it is close to other important nodes. It can be found as a solution of the eigenvalue problem equation:
where A is the network weighted adjacency matrix. Finding the eigenvector corresponding to the largest eigenvalue gives us a list of centrality values \(C_E(v)\) for each vertex \(v \in V\) .
However, we are interested in edge-based metric, which we obtain by taking an absolute difference between the adjacent vertex centralities:
where \(e=(u,v)\) . The last step is to connect this importance measure to time snapshot, which we do by taking a time-base difference between edge-based eigenvector centralities
This metric gives us the eigenvector centrality change with respect to future state of the dynamic graph ( \(t+1\) ).
Second Order Jaccard Similarity One more indicator of how important a particular newly discovered network connection is related to its adjacent nodes neighborhood similarity. The intuition is that more similar their neighborhood is, more trivial the connection is, therefore, it is less important.
We consider a second-order Jaccard similarity index for a given pair of nodes \(m_i\) and \(m_j\) :
Second-order neighborhood of a node u is defined by:
where w iterates over all neighbors of u and N ( w ) returns the neighbors of w .
The second order gives a much better “resolution” or granularity for different connections compared to first-order neighborhood. We also note that it is calculated for a graph \(G_{t-1}\) for all edges \(\hat{E}_{t}\) (before these edges were discovered).
Literature-based measures
Cumulative citation counts Another measure of a connection importance is related to bibliometrics. At each moment in time for each targeted edge we can obtain a list of papers mentioning this edge.
We also have access to a directed citation network, where nodes represent documents and edges represent citations: edges connect one paper to all the papers that it cites. Therefore, the number of citations of a specific paper would equal to in-degree of a corresponding node in a citation network.
To connect paper citations to concepts connections, we compute the sum of citation counts of all papers mentioning a specific connection. Usually, the citation counts follow heavy-tailed distributions (e.g., power law) and counting them at the logarithmic scale is a better practice. However, in our case the citation counts are taken “as-is” to emphasize the difference between the number of citations and the number of mentions. This measure shows the overall citation-based impact of a specific edge over time. The citation information comes from the citation graph, which is consistent with the proposed dynamic network in terms of time slicing methodology.
Combined importance measure for ranking connections
To connect all the components of the importance measure I for edge e , we use the mean percentile rank (PCTRank) of each individual component:
where \(C_i\) is the importance component (one of the described earlier, C —set of all importance components). The importance measure is calculated for each individual edge in graph for each moment in time t with respect to its future (or previous) state \(t+1\) (or \(t-1\) ). Using the mean percentile rank guarantees that the component will stay within a unit interval. The measure I is used to implement an importance-based stratification strategy for benchmarking, as it is discussed in Results section.
In this section we describe the experimental setup and propose a methodology based on different stratification methods. This methodology is unique for the proposed benchmark, because each record is supplied with additional information giving a user more flexible evaluation protocol.
Data collection and processing
Dynamic graph construction.
The numbers of concepts and their associations successfully mapped to UMLS CUI \((m_i, m_j)\) from each dataset are summarized in Table 1 . The number of associations with respect to time is shown in Fig. 2 . It can be seen that the number of concept associations steadily and consistently grows for every subsequent year.
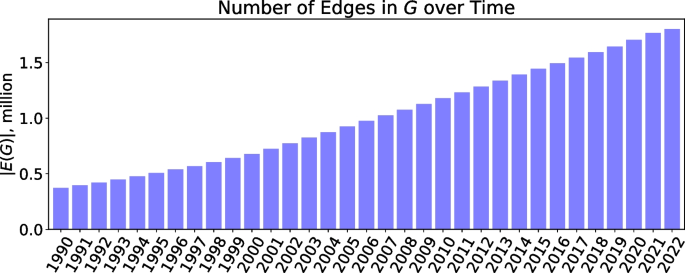
Number of edges in the network G over time. The numbers are reported in millions. Each edge represents a pair of cross-referenced UMLS CUI concepts \((m_i, m_j)\)
Data collection and aggregation is performed in the following pipeline:
All databases are downloaded in their corresponding formats such as comma-separated or Excel spreadsheets, SQL databases or Docker images.
All pairwise interactions in each database are identified.
From all these interactions we create a set of unique concepts, which we then map to UMLS CUIs. Concepts that do not have UMLS representations are dropped.
All original pairwise interactions are mapped with respect to the UMLS codes, as discussed in Databases Processing and Normalization section.
A set of all pairwise interactions is created by merging the mapped interactions from all databases.
This set is then used to find pairwise occurrences in MEDLINE.
Pairwise occurrences found in step 6 are used to construct the main dynamic network G . As it was mentioned earlier, G is undirected and non-attributed (we do not provide types of edges as they are much harder to collect reliably on large scale), which allows us to cover a broader range of pairwise interactions and LBD systems to test. Other pairwise interactions, which are successfully mapped to UMLS CUI, but are not found in the literature, can still be used. They do not have easily identifiable connections to scientific literature and do not contain temporal information, which make them a more difficult target to predict (will be discussed later).
Compound importance calculation
Once the dynamic graph G is constructed, we calculate the importance measure. For that we need to decide on three different timestamps:
Training timestamp: when the predictor models of interest are trained;
Testing timestamp: what moment in time to use to accumulate recently (with respect to step 1) discovered concept associations for models testing;
Importance timestamp: what moment in time to use to calculate the importance measure for concept associations from step 2.
To demonstrate our benchmark, we experiment with different predictive models. In our experimental setup, all models are trained on the data published prior to 2016, tested on associations discovered in 2016 and the importance measure I is calculated based on the most recent fully available timestamp (2022, at the time of writing) with respect to the PubMed annual baseline release. We note that, depending on the evaluation goals, other temporal splits can be used as well. For example, one can decide to evaluate the predictive performance of selected models on more recently discovered connections. For that, they may use the following temporal split: training timestamp—2020, testing timestamp—2021, importance timestamp—2022.
The importance measure I has multiple components, which are described in Methods section. To investigate their relationships and how they are connected to each other, we plot a Spearman correlation matrix showed in Table 2 . Spearman correlation is used because only component’s rank matters in the proposed measure as all components are initially scaled differently.
Evaluation protocol
In our experiments, we demonstrate a scenario for benchmarking hypothesis generation systems. All of the systems are treated as predictors capable of ranking true positive samples (which come from the dynamic network G ) higher than the synthetically generated negatives. The hypothesis generation problem is formulated as binary classification with significant class imbalance.
Evaluation metric
The evaluation metric of choice for our benchmarking is Receiver Operating Characteristic (ROC) curve and its associated Area Under the Curve (AUC), which is calulated as:
where \({\textbf {1}}\) is the indicator function that equals 1 if the score of a negative example \(t_0\) is less than the score of a positive example \(t_1\) ; \(D^0\) , \(D^1\) are the sets of negative and positive examples, respectively. The ROC AUC score quantifies the model’s ability to rank a random positive higher than a random negative.
We note than the scores do not have to be within a specific range, the only requirement is that they can be compared with each other. In fact, using this metric allows us to compare purely classification-based models (such as Node2Vec logistic regression pipeline) and ranking models (like TransE or DistMult), even though the scores of these models may have arbitrary values.
Negative sampling
Our original evaluation protocol can be found in [ 10 ], which is called subdomain recommendation . It is inspired by how biomedical experts perform large-scale experiments to identify the biological instances of interest from a large pool of candidates [ 35 ]. To summarize:
We collect all positive samples after a pre-defined cut date. The data before this cut date is used for prediction system training.
For each positive sample (subject-object pair) we generate N negative pairs, such that the subject is the same and the object in every newly generated pair has the same UMLS semantic type as the object in positive pair;
We evaluate a selected performance measure (ROC AUC) with respect to pairs of semantic types (for example, gene-gene or drug-disease) to better understand domain specific differences.
For this experiment we set \(N=10\) as a trade-off between the evaluation quality and runtime. It can be set higher if more thorough evaluation is needed.
Baseline models description
To demonstrate how the proposed benchmark can be used to evaluate and compare different hypothesis generation system, we use a set of existing models. To make the comparison more fair, all of them are trained on the same snapshots of MEDLINE dataset.
The AGATHA is a general purpose HG system [ 10 , 36 ] incorporates a multi-step pipeline, which processes the entire MEDLINE database of scientific abstracts, constructs a semantic graph from it and trains a predictor model based on transformer encoder architecture. Besides the algorithmic pipeline, the key difference between AGATHA and other link prediction systems is that AGATHA is an end-to-end hypothesis generation framework, where the link prediction is only one of its components.
Node2Vec-based predictor is trained as suggested in the original publication [ 37 ]. We use a network purely constructed with text-mining-based methods.
Knowledge graph embeddings-based models
Knowledge Graph Embeddings (KGE) models are becoming increasingly popular these days, therefore we include them into our comparison. We use Ampligraph [ 38 ] library to train and query a list of KGE models: TransE, HolE, ComplEx and DistMult.
Evaluation with different stratification
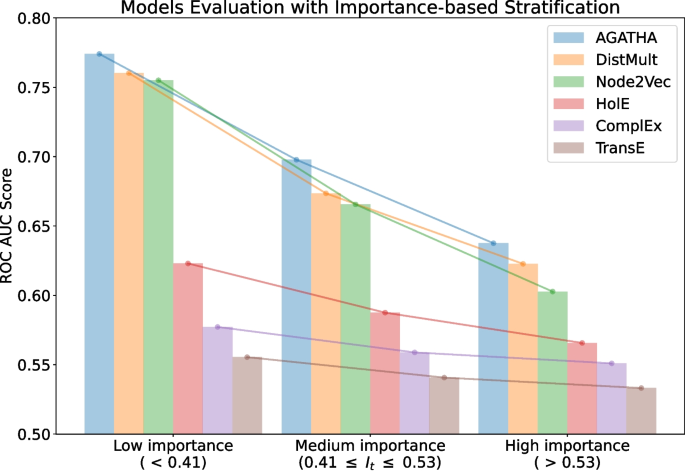
ROC AUC scores for different models trained on the same PubMed snapshot from 2015 and tested on semantic predicates added in 2016 binned with respect to their importance scores
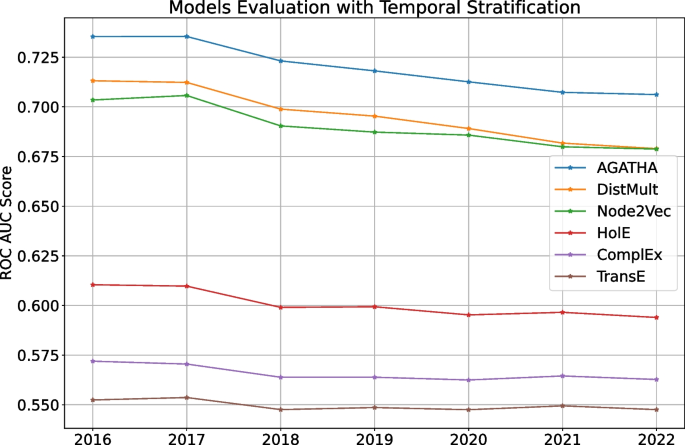
ROC AUC scores for different models trained on the same PubMed snapshot from 2015 and tested on semantic predicates added over time
The proposed benchmarking pipeline enables us to perform different kinds of systems evaluation and comparison with flexibility usually unavailable to other methods. Incorporating both temporal and importance information is helpful to identify trends in models behavior and extend the variety of criteria for domain experts when they decide on a best model suitable for their needs.
Below we present three distinct stratification methods and show how predictor models perform under different evaluation protocols. Even though we use the same performance metric (ROC AUC) across the board, the results differ substantially, suggesting that evaluation strategy plays a significant role in the experimental design.
Semantic stratification
Semantic stratification strategy is the natural way to benchmark hypothesis generation systems, when the goal is to evaluate performance in specific semantic categories. It is especially relevant to the subdomain recommendation problem, which defines our negative sampling procedure. For that we take the testing set of subject-object pairs and group them according to their semantic types and evaluate each group separately (Table 3 ).
Importance-based stratification
The next strategy is based on the proposed importance measure I . This measure ranks all the positive subject-object pairs from the test set and, therefore, can be used to split them into equally-sized bins, according to their importance score. In our experiment, we split the records into three bins, representing low, medium and high importance values. Negative samples are split accordingly. Then each group is evaluated separately. The results of this evaluation are presented in Fig. 3 .
The results indicate that the importance score I could also reflect the difficulty of making a prediction. Specifically, pairs that receive higher importance scores tend to be more challenging for the systems to be identified correctly. In models that generally exhibit high performance (e.g., DistMult), the gap in ROC AUC scores between pairs with low importance scores and those with high importance scores is especially pronounced. The best model in this list is AGATHA as it utilizes the most nuanced hypothesis representation, namely, its transformer architecture is trained to leverage not only node embeddings but also to account for the non-overlapping neighborhoods of concepts.
Temporal stratification
The last strategy shows how different models trained once perform over time . For that we fix the training timestamp on 2015 and evaluate each models on testing timestamps from 2016 to 2022. For clarity, we do not use importance values for this experiment and only focus on how the models perform over time on average . The results are shown in Fig. 4 .
Figure 4 highlights how predictive performance gradually decays over time for every model in the list. This behavior can be expected: the gap between training and testing data increases over time, which makes it more difficult for models to perform well as time goes by. Therefore, it is a good idea to keep the predictor models up-to-date, which we additionally discuss in the next section.
We divide the discussion into separate parts: topics related to evaluation challenges and topics related to different predictor model features. We also describe the challenges and scope for the future work at the end of the section.
Evaluation-based topics
Data collection and processing challenges.
The main challenge of this work comes from the diverse nature of biomedical data. This data may be described in many different ways and natural language may not be the most commonly used. Our results indicate that a very significant part of biocurated connections “flies under the radar” of text-mining systems and pipelines due to several reasons:
Imperfections of text-mining methods;
Multiple standards to describe biomedical concepts;
The diversity of scientific language: many biomedical associations (e.g. gene-gene interactions may be primarily described in terms of co-expression);
Abstracts are not enough for text mining [ 39 ].
The proposed methodology for the most part takes the lowest common denominator approach: we discard concepts not having UMLS representations and associations not appearing in PubMed abstracts. However, our approach still allows us to extract a significant number of concept associations and to use them for quantitative analysis. We should also admit that the aforementioned phenomenon of biomedical data discrepancy leads us to some interesting results, which we discuss below.
Different nature of biomedical DBs and literature-extracted data
The experiment clearly indicates significant differences between different kinds of associations with respect their corresponding data sources in models performance comparison. For this experiment we take one of the evaluated earlier systems (AGATHA 2015) and run the semantically-stratified version of benchmark collected from three different data sources:
Proposed benchmark dataset: concept associations extracted from biocurated databases with cross-referenced literature data;
Concept associations extracted from biocurated databases, but which we could not cross-reference with literature data;
Dataset composed of associations extracted with a text mining framework (SemRep).
Datasets (1) and (3) were constructed from associations found in MEDLINE snapshot from 2020. For dataset (2) it was impossible to identify the time connections were added, therefore the cut date approach was not used. All three datasets were downsampled with respect to the proposed benchmark (1), such that the number of associations is the same across all of them.
The results of this experiment are shown in Table 4 . It is evident that associations extracted from biocurated databases (1) and (2) propose a more significant challenge for a text-mining-based system. Cross-referencing from literature makes sure that similar associations can be at least discovered by these systems at the training time, therefore, the AGATHA performance on dataset (1) is higher compared to dataset (2). These results may indicate that biocurated associations, which cannot be cross-referenced, belong to a different data distribution, and, therefore, purely text mining-based systems fall short due to the limitations of the underlying information extraction algorithms.
Models-related topics
Text mining data characteristics.

Degree distributions and nodes with highest degrees for two networks: the one used for training of text-mining-based predictor models (red, top) and the network G from the proposed benchmark dataset (blue, bottom)
In order to demonstrate the differences between biologically curated and text mining-based knowledge, we can consider their network representations.
The network-based models we show in this work are trained on text-mining-based networks, which are built on top of semantic predicates extracted from a NLP tool SemRep. This tool takes biomedical text as input and extracts triples (subject-verb-object) from the text and performs a number of additional tasks, such as:
Named Entity Recognition
Concept Normalization
Co-reference Resolution
and some others. This tool operates on UMLS Metathesaurus, one of the largest and most diverse biomedical thesaurus, including many different vocabularies.
The main problem of text-mining tools like SemRep is that they tend to produce noisy (and often not quite meaningful from the biomedical prospective) data. As a result, the underlying data that is used to build and validate literature-based discovery systems may not represent the results that domain experts expect to see.
However, these systems are automated and, therefore, are widely used as a tool to extract information from literature in uninterrupted manner. Then this information is used for training different kinds of predictors (either rule-based, statistical or deep learning).
To demonstrate this phenomenon, we compare two networks, where nodes are biomedical terms and edges are associations between them. The difference between them lies in their original data source, which is either:
PubMed abstracts processed with SemRep tool;
Biocurated databases, which connections are mapped to pairs of UMLS CUI terms and cross-referenced with MEDLINE records.
Connections from the network (2) are used in the main proposed benchmarking framework (network G ). The comparison is shown in Fig. 5 as a degree distribution of both networks. We can see that network (1) has a small number of very high-degree nodes. These nodes may affect negatively to the overall predictive power of any model using networks like (1) as a training set, because they introduce a large number of “shortcuts” to the network, which do not have any significant biological value. We also show the top most high-degree nodes for both networks. For the network (1), all of them appear to be very general and most of them (e.g. “Patients” or “Pharmaceutical Preparations”) can be described as noise. Network (2), in comparison, contain real biomedical entities, which carry domain-specific meaning.
Training data threshold influence
As the Temporal Stratification experiment in the Results section suggests, the gap between training and testing timestamps plays a noticeable role in models predictive performance.
To demonstrate this phenomena from a different perspective, we now fix the testing timestamp and vary the training timestamp. We use two identical AGATHA instances, but trained on different MEDLINE snapshots: 2015 and 2020. The testing timestamp for this experiment is 2021, such that none of the models has access to the test data.
The results shown in Table 5 illustrate that having more recent training data does not significantly increase model’s predictive power for the proposed benchmark. This result may be surprising, but there is a possible explanation: a model learns the patterns from the training data distribution and that data distribution stays consistent for both training cut dates (2015 and 2020). However, that does not mean that the data distribution in the benchmark behaves the same way. In fact, it changes with respect to both data sources: textual and DB-related.
Semantic types role in predictive performance
Another aspect affecting models predictive performance is having access to domain information. Since we formulate the problem as subdomain recommendation, knowing concept-domain relationships may be particularly valuable. We test this idea by injecting semantic types information into the edge type for tested earlier Knowledge Graph Embedding models. As opposed to classic link prediction methods (such as node2vec), Knowledge Graph modeling was designed around typed edges and allows this extension naturally.
Results in Table 6 show that semantic type information provides a very significant improvement for models predictive performance.
Large language models for scientific discovery

Confusion matrix obtained by the BioGPT-QA model. Only confident answers (Yes/No) were taken into account
Recent advances in language model development raised a logical question about usefulness of these models in scientific discovery, especially in biomedical area [ 40 ]. Problems like drug discovery, drug repurposing, clinical trial optimization and many others may benefit significantly from systems trained on a large amount of scientific biomedical data.
Therefore, we decide to test how one of these systems would perform in our benchmark. We take one of the recently released generative pre-trained transformer models BioGPT [ 4 ] and run a set of test queries.
BioGPT model was chosen due to the following reasons:
It is recently released (2022);
It includes fine-tuned models, which show good performance on downstream tasks;
It is open source and easily accessible.
We use a BioGPT-QA model to perform the benchmarking, because it was fine-tuned on PubMedQA [ 41 ] dataset and outputs the answer as yes/maybe/no, which is easy to parse and represent as a (binary) classifier output.
The question prompt was formulated as the following: “Is there a relationship between <term 1> and <term 2>?”. PubMedQA format also requires a context from a PubMed abstract, which does not exist in our case, because it is a discovery problem. However, we supply an abstract-like context, which is constructed by concatenating term definitions extracted from UMLS Metathesaurus for both source and target terms.
A sample prompt looks like this: “Is there a relationship between F1-ATPase and pyridoxal phosphate? context: F1-ATPase—The catalytic sector of proton-translocating ATPase complexes. It contains five subunits named alpha, beta, gamma, delta and eta. pyridoxal phosphate—This is the active form of VITAMIN B6 serving as a coenzyme for synthesis of amino acids, neurotransmitters (serotonin, norepinephrine), sphingolipids, aminolevulinic acid...”
When we ran the experiment, we noticed two things:
BioGPT is often not confident in its responses, which means that it outputs “maybe” or two answers (both “yes” and “no”) for about 40% of the provided queries;
The overwhelming majority of provided queries are answered positively when the answer is confident.
Figure 6 shows a confusion matrix for queries with confident answer. We generate the queries set with 1:1 positive to negative ratio. Most of the answers BioGPT-QA provides are positive, which means that the system produces too many false positives and is not usable in the discovery setting.
Challenges in benchmarking for hypothesis generation
Binary interactions. Not every discovery can be represented as a pair of terms, but this is something that most of biomedical graph-based knowledge discovery systems work with. It is a significant limitation of the current approach and a motif discovery is a valid potential direction for future work. Moreover, many databases represent their records as binary interactions [ 42 , 43 , 44 , 45 , 46 ], which can be easily integrated into a link prediction problem.
Directionality. Currently, our choice for pairwise interactions is to omit the directionality information to allow more systems to be evaluated with our framework and cover more pairwise interactions. Directionality is an important component of pairwise interactions, especially when they have types and are formulated in a predication form as a triple: (subject-predicate-object) . Currently, we omit the predicate part and only keep pairs of terms for easier generalization. In many cases, a uni-directional edge \(i\rightarrow j\) does not imply non-existence of \(i\leftarrow j\) . Moreover, in the low-dimensional graph representation construction it is clearly preferable to use undirected edges in our context due to the scarcity of biomedical information. Another caveat is that the tools that detect the logical direction of the predicate in the texts are not perfect [ 47 ]. The information about each particular direction can still be recovered from the underlying cross-referencing citations.
Concept normalization . UMLS is a powerful system combining many biomedical vocabularies together. However, it has certain limitations, such as relatively small number of proteins and chemical compounds. We also observe that many UMLS terms are never covered in the scientific abstracts, even though they exist in the Metathesaurus. This limits the number of obtainable interactions significantly. However, UMLS covers many areas of biomedicine, such as genes, diseases, proteins, chemicals and many others and also provides rich metadata. In addition, NLM provides software for information extraction. There are other vocabularies, which have greater coverage in certain areas (e.g., UniProt ID for proteins or PubChem ID for chemicals), but their seamless integration into a heterogeneous network with literature poses additional challenges that will be gradually addressed in the future work.
We have developed and implemented a comprehensive benchmarking system Dyport for evaluating biomedical hypothesis generation systems. This benchmarking system is advancing the field by providing a structured and systematic approach to assess the efficacy of various hypothesis generation methodologies.
In our pipeline we utilized several curated datasets, which provide a basis in testing the hypothesis generation systems under realistic conditions. The informative discoveries have been integrated into the dynamic graph on top of which we introduced the quantification of discovery importance. This approach allowed us to add a new dimension to the benchmarking process, enabling us to not only assess the accuracy of the hypotheses generated but also their relevance and potential impact in the field of biomedical research. This quantification of discovery importance is a critical step forward, as it aligns the benchmarking process more closely with the practical and applied goals of biomedical research.
We have demonstrated the use case of several graph-based link prediction systems’ verification and concluded that such testing is way more productive than traditional link prediction benchmarks. However, the utility of our benchmarking system extends beyond these examples. We advocate for its widespread adoption to validate the quality of hypothesis generation, aiming to broaden the range of scientific discoveries accessible to the wider research community. Our system is designed to be inclusive, welcoming the addition of more diverse cases.
Future work includes integration of the benchmarking process in the hypothesis system visualization [ 48 ], spreading to other than biomedical areas [ 49 ], integration of novel importance measures, and healthcare benchmarking cases.
Swanson DR. Undiscovered public knowledge. Libr Q. 1986;56(2):103–18.
Article Google Scholar
Swanson DR, Smalheiser NR, Torvik VI. Ranking indirect connections in literature-based discovery: the role of medical subject headings. J Am Soc Inform Sci Technol. 2006;57(11):1427–39.
Article CAS Google Scholar
Peng Y, Bonifield G, Smalheiser N. Gaps within the biomedical literature: Initial characterization and assessment of strategies for discovery. Front Res Metrics Anal. 2017;2:3.
Luo R, Sun L, Xia Y, Qin T, Zhang S, Poon H, Liu T-Y. Biogpt: generative pre-trained transformer for biomedical text generation and mining. Brief Bioinform. 2022;23(6):409.
Sybrandt J, Safro I. Cbag: conditional biomedical abstract generation. PLoS ONE. 2021;16(7):0253905.
Sybrandt J, Shtutman M, Safro I. Moliere: automatic biomedical hypothesis generation system. In: Proceedings of the 23rd ACM SIGKDD. KDD ’17, 2017. pp. 1633–1642. ACM, New York, NY, USA. https://doi.org/10.1145/3097983.3098057 .
Sedler AR, Mitchell CS. Semnet: using local features to navigate the biomedical concept graph. Front Bioeng Biotechnol. 2019;7:156.
Article PubMed PubMed Central Google Scholar
Hristovski D, Peterlin B, Mitchell JA, Humphrey SM. Using literature-based discovery to identify disease candidate genes. Int J Med Inform. 2005;74(2):289–98.
Article PubMed Google Scholar
Gordon MD, Dumais S. Using latent semantic indexing for literature based discovery. J Am Soc Inf Sci. 1998;49(8):674–85.
Sybrandt J, Tyagin I, Shtutman M, Safro I. AGATHA: automatic graph mining and transformer based hypothesis generation approach. In: Proceedings of the 29th ACM international conference on information and knowledge management, 2020;2757–64.
Sourati J, Evans J. Accelerating science with human-aware artificial intelligence. Nat Hum Behav. 2023;7:1682–96.
Chen Y, Argentinis JE, Weber G. IBM Watson: how cognitive computing can be applied to big data challenges in life sciences research. Clin Ther. 2016;38(4):688–701.
Xun G, Jha K, Gopalakrishnan V, Li Y, Zhang A. Generating medical hypotheses based on evolutionary medical concepts. In: 2017 IEEE International conference on data mining (ICDM), pp. 535–44 (2017). https://doi.org/10.1109/ICDM.2017.63 .
Cameron D, Kavuluru R, Rindflesch TC, Sheth AP, Thirunarayan K, Bodenreider O. Context-driven automatic subgraph creation for literature-based discovery. J Biomed Inform. 2015;54:141–57. https://doi.org/10.1016/j.jbi.2015.01.014 .
Sebastian Y, Siew E-G, Orimaye SO. Learning the heterogeneous bibliographic information network for literature-based discovery. Knowl-Based Syst. 2017;115:66–79.
Miranda A, Mehryary F, Luoma J, Pyysalo S, Valencia A, Krallinger M. Overview of drugprot biocreative vii track: quality evaluation and large scale text mining of drug-gene/protein relations. In: Proceedings of the seventh biocreative challenge evaluation workshop, 2021;11–21.
Breit A, Ott S, Agibetov A, Samwald M. OpenBioLink: a benchmarking framework for large-scale biomedical link prediction. Bioinformatics. 2020;36(13):4097–8. https://doi.org/10.1093/bioinformatics/btaa274 .
Article CAS PubMed Google Scholar
Sybrandt J, Shtutman M, Safro I. Large-scale validation of hypothesis generation systems via candidate ranking. In: 2018 IEEE international conference on big data, 2018; 1494–1503. https://doi.org/10.1109/bigdata.2018.8622637 .
Kilicoglu H, Shin D, Fiszman M, Rosemblat G, Rindflesch TC. Semmeddb: a pubmed-scale repository of biomedical semantic predications. Bioinformatics. 2012;28(23):3158–60.
Article CAS PubMed PubMed Central Google Scholar
Fannjiang C, Listgarten J. Is novelty predictable? Cold Spring Harb Perspect Biol. 2024;16: a041469.
Jeon D, Lee J, Ahn J, Lee C. Measuring the novelty of scientific publications: a fastText and local outlier factor approach. J Inform. 2023;17: 101450.
Small H, Tseng H, Patek M. Discovering discoveries: Identifying biomedical discoveries using citation contexts. J Inform. 2017;11:46–62.
Bordes A, Usunier N, Garcia-Duran A, Weston J, Yakhnenko O. Translating embeddings for modeling multi-relational data. In: Advances in neural information processing systems, 2013; 2787–2795.
Bonner S, Barrett IP, Ye C, Swiers R, Engkvist O, Hoyt CT, Hamilton WL. Understanding the performance of knowledge graph embeddings in drug discovery. Artif Intell Life Sci. 2022;2: 100036.
Google Scholar
Bonner S, Barrett IP, Ye C, Swiers R, Engkvist O, Bender A, Hoyt CT, Hamilton WL. A review of biomedical datasets relating to drug discovery: a knowledge graph perspective. Brief Bioinform. 2022;23(6):404.
Kanehisa M, Sato Y, Kawashima M, Furumichi M, Tanabe M. KEGG as a reference resource for gene and protein annotation. Nucleic Acids Res. 2015;44(D1):457–62. https://doi.org/10.1093/nar/gkv1070 .
Bodenreider O. The unified medical language system (umls): integrating biomedical terminology. Nucleic Acids Res. 2004;32(suppl_1):267–70.
Rindflesch TC, Fiszman M. The interaction of domain knowledge and linguistic structure in natural language processing: interpreting hypernymic propositions in biomedical text. J Biomed Inform. 2003;36(6):462–77. https://doi.org/10.1016/j.jbi.2003.11.003 .
Xing R, Luo J, Song T. Biorel: towards large-scale biomedical relation extraction. BMC Bioinform. 2020;21(16):1–13.
Mikolov T, Sutskever I, Chen K, Corrado GS, Dean J. Distributed representations of words and phrases and their compositionality. Adv Neural Inf Process Syst 2013;26.
Aronson AR. Effective mapping of biomedical text to the umls metathesaurus: the metamap program. In: Proceedings of the AMIA symposium, 2001;p. 17.
Welling M, Kipf TN. Semi-supervised classification with graph convolutional networks. In: Journal of international conference on learning representations (ICLR 2017), 2016.
Sundararajan M, Taly A, Yan Q. Axiomatic attribution for deep networks. In: International conference on machine learning, pp. 3319–3328, 2017.
Brandes U. A faster algorithm for betweenness centrality. J Math Sociol. 2001;25(2):163–77.
Aksenova M, Sybrandt J, Cui B, Sikirzhytski V, Ji H, Odhiambo D, Lucius MD, Turner JR, Broude E, Peña E, et al. Inhibition of the dead box rna helicase 3 prevents hiv-1 tat and cocaine-induced neurotoxicity by targeting microglia activation. J Neuroimmune Pharmacol. 2019;1–15.
Tyagin I, Kulshrestha A, Sybrandt J, Matta K, Shtutman M, Safro I. Accelerating covid-19 research with graph mining and transformer-based learning. In: Proceedings of the AAAI conference on artificial intelligence, 2022;36:12673–9.
Grover A, Leskovec J. Node2vec: Scalable feature learning for networks. In: Proceedings of the 22nd ACM SIGKDD international conference on knowledge discovery and data mining. KDD ’16, 2016, pp. 855–864. Association for Computing Machinery, New York. https://doi.org/10.1145/2939672.2939754 .
Costabello L, Bernardi A, Janik A, Pai S, Van CL, McGrath R, McCarthy N, Tabacof P. AmpliGraph: a library for representation learning on knowledge graphs, 2019. https://doi.org/10.5281/zenodo.2595043 .
Sybrandt J, Carrabba A, Herzog A, Safro I. Are abstracts enough for hypothesis generation? In: 2018 IEEE international conference on big data, 2018;1504–1513. https://doi.org/10.1109/bigdata.2018.8621974 .
Liu Z, Roberts RA, Lal-Nag M, Chen X, Huang R, Tong W. Ai-based language models powering drug discovery and development. Drug Discovery Today. 2021;26(11):2593–607.
Jin Q, Dhingra B, Liu Z, Cohen WW, Lu X. Pubmedqa: a dataset for biomedical research question answering, 2019; arXiv preprint arXiv:1909.06146 .
Davis AP, Wiegers TC, Johnson RJ, Sciaky D, Wiegers J, Mattingly CJ. Comparative toxicogenomics database (ctd): update 2023. Nucleic Acids Res. 2022. https://doi.org/10.1093/nar/gkac833 .
Piñero J, Ramírez-Anguita JM, Saüch-Pitarch J, Ronzano F, Centeno E, Sanz F. Furlong LI The DisGeNET knowledge platform for disease genomics: 2019 update. Nucleic Acids Res. 2019;48(D1):845–55. https://doi.org/10.1093/nar/gkz1021 .
Ursu O, Holmes J, Knockel J, Bologa CG, Yang JJ, Mathias SL, Nelson SJ, Oprea TI. DrugCentral: online drug compendium. Nucleic Acids Research. 2016;45(D1):932–9. https://doi.org/10.1093/nar/gkw993 .
Calderone A, Castagnoli L, Cesareni G. Mentha: a resource for browsing integrated protein-interaction networks. Nat Methods. 2013;10(8):690–1.
Zeng K, Bodenreider O, Kilbourne J, Nelson SJ. Rxnav: a web service for standard drug information. In: AMIA annual symposium proceedings, 2006; vol. 2006, p. 1156.
Kilicoglu H, Rosemblat G, Fiszman M, Shin D. Broad-coverage biomedical relation extraction with SemRep. BMC Bioinform. 2020;21:1–28.
Tyagin I, Safro I. Interpretable visualization of scientific hypotheses in literature-based discovery. BioCretive Workshop VII; 2021. https://www.biorxiv.org/content/10.1101/2021.10.29.466471v1 .
Marasco D, Tyagin I, Sybrandt J, Spencer JH, Safro I. Literature-based discovery for landscape planning, 2023. arXiv preprint arXiv:2306.02588 .
Rehurek R, Sojka P. Gensim-python framework for vector space modelling. NLP Centre, Faculty of Informatics, Masaryk University, Brno, Czech Republic 2011;3(2).
Fey M, Lenssen JE. Fast graph representation learning with PyTorch Geometric. In: ICLR workshop on representation learning on graphs and manifolds, 2019.
Kokhlikyan, N., Miglani, V., Martin, M., Wang, E., Alsallakh, B., Reynolds, J., Melnikov, A., Kliushkina, N., Araya, C., Yan, S., Reblitz-Richardson, O. Captum: a unified and generic model interpretability library for PyTorch, 2020.
Sollis E, Mosaku A, Abid A, Buniello A, Cerezo M, Gil L, Groza T, Güneş O, Hall P, Hayhurst J, Ibrahim A, Ji Y, John S, Lewis E, MacArthur JL, McMahon A, Osumi-Sutherland D, Panoutsopoulou K, Pendlington Z, Ramachandran S, Stefancsik R, Stewart J, Whetzel P, Wilson R, Hindorff L, Cunningham F, Lambert S, Inouye M, Parkinson H, Harris L. The NHGRI-EBI GWAS catalog: knowledgebase and deposition resource. Nucleic Acids Res. 2022;51(D1):977–85. https://doi.org/10.1093/nar/gkac1010 .
Szklarczyk D, Gable AL, Nastou KC, Lyon D, Kirsch R, Pyysalo S, Doncheva NT, Legeay M, Fang T, Bork P, Jensen LJ, von Mering C. The STRING database in 2021: customizable protein-protein networks, and functional characterization of user-uploaded gene/measurement sets. Nucleic Acids Research. 2020;49(D1):605–12. https://doi.org/10.1093/nar/gkaa1074 .
Fricke S. Semantic scholar. J Med Lib Assoc: JMLA. 2018;106(1):145.
Download references
Acknowledgements
We would like to thank two anonymous referees whose thoughtful comments helped to improve the paper significantly. This research was supported by NIH award #R01DA054992. The computational experiments were supported in part through the use of DARWIN computing system: DARWIN—A Resource for Computational and Data-intensive Research at the University of Delaware and in the Delaware Region, which is supported by NSF Grant #1919839.
This research was supported by NIH award #R01DA054992. The computational experiments were supported in part through the use of DARWIN computing system: DARWIN—A Resource for Computational and Data-intensive Research at the University of Delaware and in the Delaware Region, which is supported by NSF Grant #1919839.
Author information
Authors and affiliations.
Center for Bioinformatics and Computational Biology, University of Delaware, Newark, DE, 19713, USA
Ilya Tyagin
Department of Computer and Information Sciences, University of Delaware, Newark, DE, 19716, USA
You can also search for this author in PubMed Google Scholar
Contributions
IT processed and analyzed the textual and database data, trained models and implemented the computational pipeline. IS formulated the main idea, supervised the project and provided feedback. Both authors contributed to writing, read and approved the final manuscript.
Corresponding authors
Correspondence to Ilya Tyagin or Ilya Safro .
Ethics declarations
Competing interests.
I declare that the authors have no Conflict of interest as defined by BMC, or other interests that might be perceived to influence the results and/or discussion reported in this paper.
Availability of data and materials
The dataset(s), materials and code supporting the conclusions of this article is(are) available in the GitHub repository: https://github.com/IlyaTyagin/Dyport .
Additional information
Publisher's note.
Springer Nature remains neutral with regard to jurisdictional claims in published maps and institutional affiliations.
Appendix A: Incorporated technologies
To construct the benchmark, we propose a multi-step pipeline, which requires several key technologies to be used. For the text mining part, we use SemRep [ 28 ] and gensim [ 50 ] implementation of word2vec algorithm. For further stages involving graph learning, we utilize Pytorch Geometric framework and Captum explainability library.
UMLS (Unified Medical Language System) [ 27 ] is one of the fundamental technologies provided by NLM, which consolidates and disseminates essential terminology, taxonomies, and coding norms, along with related materials, such as definitions and semantic types. UMLS is used in the proposed work as a system of concept unique identifiers (CUI) bringing together terms from different vocabularies.
SemRep [ 47 ] is an NLM-developed software, performing extraction of semantic predicates from biomedical texts. It also has the named entity recognition (NER) capabilities (based on MetaMap [ 31 ] backend) and automatically performs entity normalization based on the context.
Word2Vec [ 30 ] is an approach for creating efficient word embeddings. It was proposed in 2013 and is proven to be an excellent technique for generating static (context-independent) latent word representations. The implementation used in this work is based on gensim [ 50 ] library.
Pytorch geometric (PyG) [ 51 ] library is built on top of Pytorch framework focusing on graph geometric learning. It implements a variety of algorithms from published research papers, supports arbitrary-scaled graphs and is well integrated into Pytorch ecosystem. We use PyG to train a graph neural network (GNN) for link prediction problem, which we explain in more detail in methods section.
Captum [ 52 ] package is an extension of Pytorch enabling the explainability of many ML models. It contains attribution methods, such as saliency maps, integrated gradients, Shapley value sampling and others. Captum is supported by PyG library and used in this work to calculate attributions of the proposed GNN.
Appendix B: Incorporated data sources
We review and include a variety of biomedical databases, containing curated connections between different kinds of entities.
KEGG (Kyoto Encyclopedia of Genes and Genomes) [ 26 ] is a collection of resources for understanding principles of work of biological systems (such as cells, organisms or ecosystems) and offering a wide variety of entry points. One of the main components of KEGG is a set of pathway maps, representing molecular interactions as network diagrams.
CTD (The Comparative Toxicogenomics Database) [ 42 ] is a publicly available database focused on collecting the information about environmental exposures effects on human health.
DisGenNET [ 43 ] is a discovery platform covering genes and variants and their connections to human diseases. It integrates data from a list of publicly available databases and repositories and scientific literature.
GWAS (Genome-Wide Association Studies) [ 53 ] is a catalog of human genome-wide association studies, developed by EMBL-EBI and NHGRI. Its aim is to identify and systematize associations of genotypes with phenotypes across human genome.
STRING [ 54 ] is a database aiming to integrate known and predicted protein associations, both physical and functional. It utilizes a network-centric approach and assigns a confidence score for all interactions in the network based on the evidence coming from different sources: text mining, computational predictions and biocurated databases.
DrugCentral [ 44 ] is an online drug information resource aggregating information about active ingredients, indications, pharmacologic action and other related data with respect to FDA, EMA and PMDA-approved drugs.
Mentha [ 45 ] is an evidence-based protein interaction browser (and corresponding database), which takes advantage of International Molecular Exchange (IMEx) consortium. The interactions are curated by experts in compliance with IMEx policies enabling regular weekly updates. Compared to STRING, Mentha is focused on precision over comprehensiveness and excludes any computationally predicted records.
RxNav [ 46 ] is a web-service providing an integrated view on drug information. It contains the information from NLM drug terminology RxNorm, drug classes RxClass and drug-drug interactions collected from ONCHigh and DrugBank sources.
Semantic scholar [ 55 ] is a search engine and research tool for scientific papers developed by the Allen Institute for Artificial Intelligence (AI2). It provides rich metadata about publications which enables us to use Semantic Scholar data for network-based citation analysis.
Rights and permissions
Open Access This article is licensed under a Creative Commons Attribution 4.0 International License, which permits use, sharing, adaptation, distribution and reproduction in any medium or format, as long as you give appropriate credit to the original author(s) and the source, provide a link to the Creative Commons licence, and indicate if changes were made. The images or other third party material in this article are included in the article's Creative Commons licence, unless indicated otherwise in a credit line to the material. If material is not included in the article's Creative Commons licence and your intended use is not permitted by statutory regulation or exceeds the permitted use, you will need to obtain permission directly from the copyright holder. To view a copy of this licence, visit http://creativecommons.org/licenses/by/4.0/ . The Creative Commons Public Domain Dedication waiver ( http://creativecommons.org/publicdomain/zero/1.0/ ) applies to the data made available in this article, unless otherwise stated in a credit line to the data.
Reprints and permissions
About this article
Cite this article.
Tyagin, I., Safro, I. Dyport: dynamic importance-based biomedical hypothesis generation benchmarking technique. BMC Bioinformatics 25 , 213 (2024). https://doi.org/10.1186/s12859-024-05812-8
Download citation
Received : 31 January 2024
Accepted : 16 May 2024
Published : 13 June 2024
DOI : https://doi.org/10.1186/s12859-024-05812-8
Share this article
Anyone you share the following link with will be able to read this content:
Sorry, a shareable link is not currently available for this article.
Provided by the Springer Nature SharedIt content-sharing initiative
- Hypothesis Generation
- Literature-based Discovery
- Link Prediction
- Benchmarking
- Natural Language Processing
BMC Bioinformatics
ISSN: 1471-2105
- General enquiries: [email protected]
Information
- Author Services
Initiatives
You are accessing a machine-readable page. In order to be human-readable, please install an RSS reader.
All articles published by MDPI are made immediately available worldwide under an open access license. No special permission is required to reuse all or part of the article published by MDPI, including figures and tables. For articles published under an open access Creative Common CC BY license, any part of the article may be reused without permission provided that the original article is clearly cited. For more information, please refer to https://www.mdpi.com/openaccess .
Feature papers represent the most advanced research with significant potential for high impact in the field. A Feature Paper should be a substantial original Article that involves several techniques or approaches, provides an outlook for future research directions and describes possible research applications.
Feature papers are submitted upon individual invitation or recommendation by the scientific editors and must receive positive feedback from the reviewers.
Editor’s Choice articles are based on recommendations by the scientific editors of MDPI journals from around the world. Editors select a small number of articles recently published in the journal that they believe will be particularly interesting to readers, or important in the respective research area. The aim is to provide a snapshot of some of the most exciting work published in the various research areas of the journal.
Original Submission Date Received: .
- Active Journals
- Find a Journal
- Proceedings Series
- For Authors
- For Reviewers
- For Editors
- For Librarians
- For Publishers
- For Societies
- For Conference Organizers
- Open Access Policy
- Institutional Open Access Program
- Special Issues Guidelines
- Editorial Process
- Research and Publication Ethics
- Article Processing Charges
- Testimonials
- Preprints.org
- SciProfiles
- Encyclopedia
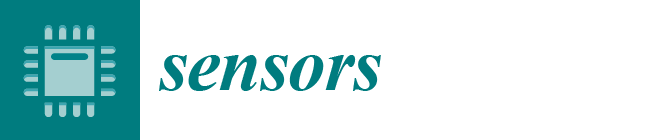
Article Menu
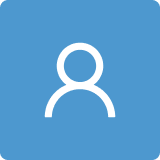
- Subscribe SciFeed
- Recommended Articles
- Google Scholar
- on Google Scholar
- Table of Contents
Find support for a specific problem in the support section of our website.
Please let us know what you think of our products and services.
Visit our dedicated information section to learn more about MDPI.
JSmol Viewer
Design of evaluation classification algorithm for identifying conveyor belt mistracking in a continuous transport system’s digital twin.
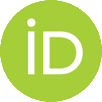
1. Introduction
2. materials and methods, digital twin, 3. undesirable operating conditions of continuous transport systems, 3.1. identification of an undesirable operating condition, causes of belt mistracking, 4. identification of belt mistracking by an evaluation algorithm.
- To select the optimal position(s) from the various positions where contact force was measured, such that the asymmetry of tensioning could be estimated using a regression model;
- To establish the best method for handling the variable “asymmetry of conveyor belt tensioning”, including determining how to construct this variable (whether as a ratio or a difference) and, consequently, how to quantify the asymmetry.
4.1. Experimental Settings
4.2. processing of measured data, 4.2.1. step 1—force ratios of cf during asymmetric tensioning, 4.2.2. step 2—method of defining asymmetric tensioning, 4.2.3. statistical verification of the hypothesis, 4.2.4. step 3—classification and verification.
- In the first step of classification and verification, the parameters of the regression model (3) were estimated using the method of least squares, which calculated the theoretical values of the variable AsymRatioZerofit (ARZf) for the known values of CF19 and CF20, corresponding to the variable AsymRatioZero. Then, the regression model (3) was verified using statistical indicators, comparing theoretical and empirical values and independent test data.
5. Conclusions
Author contributions, institutional review board statement, informed consent statement, data availability statement, conflicts of interest.
- He, D.; Liu, X.; Zhong, B. Sustainable belt conveyor operation by active speed control. Measurement 2020 , 154 , 107458. [ Google Scholar ] [ CrossRef ]
- Hu, X.; Zong, M. Fault Prediction Method of Belt Conveyor Based on Grey Least Square Support Vector Machine. In Proceedings of the International Conference On Measuring Technology And Mechatronics Automation (ICMTMA 2021), Beihai, China, 16–17 January 2021; pp. 55–58. [ Google Scholar ]
- Bortnowski, P.; Kawalec, W.; Krol, R.; Ozdoba, M. Types and causes of damage to the conveyor belt—Review, classification, and mutual relations. Eng. Fail. Anal. 2022 , 140 , 106520. [ Google Scholar ] [ CrossRef ]
- Parmar, N.J.; James, A.T. Development of a framework for safety performance measurement of belt conveyor systems. Int. J. Product. Perform. Manag. 2023 , 72 , 1001–1024. [ Google Scholar ] [ CrossRef ]
- Wang, S.; Guo, W.; Wen, W.; Chen, R.; Li, T.; Fang, F. Research on belt conveyor monitoring and control system. In Proceedings of the Communications in Computer and Information Science, Ustron, Poland, 15–19 June 2010; Volume 105, pp. 334–339. [ Google Scholar ]
- Li, M.; Sun, Y.; Luo, C. Reliability Analysis of Belt Conveyor Based on Fault Data. In Proceedings of the 5th International Conference On Mechanical Engineering And Automation Science (ICMEAS 2019), Wuhan, China, 10–12 October 2019; Volume 692. [ Google Scholar ]
- Gao, Y.; Qiao, T.; Zhang, H.; Yang, Y.; Pang, Y.; Wei, H. A contactless measuring speed belt conveyor system based on machine vision and machine learning. Measurement 2019 , 139 , 127–133. [ Google Scholar ] [ CrossRef ]
- Wang, Y.; Miao, C.; Liu, Y.; Meng, D. Research on a sound-based method for belt conveyor longitudinal tear detection. Measurement 2022 , 190 , 110787. [ Google Scholar ] [ CrossRef ]
- Zeng, F.; Wu, Q.; Chu, X.; Yue, Z. Measurement of bulk material flow based on laser scanning technology for the energy efficiency improvement of belt conveyors. Meas. J. Int. Meas. Confed. 2015 , 75 , 230–243. [ Google Scholar ] [ CrossRef ]
- Liu, Y.; Miao, C.; Li, X.; Ji, J.; Meng, D. Research on the fault analysis method of belt conveyor idlers based on sound and thermal infrared image features. Measurement 2021 , 186 , 110177. [ Google Scholar ] [ CrossRef ]
- Gladysiewicz, L.; Krol, R.; Kisielewski, W. Measurements of loads on belt conveyor idlers operated in actual conditions. Measurement 2019 , 134 , 336–344. [ Google Scholar ] [ CrossRef ]
- Zhang, M.; Jiang, K.; Cao, Y.; Li, M.; Wang, Q.; Li, D.; Zhang, Y. A new paradigm for intelligent status detection of belt conveyors based on deep learning. Measurement 2023 , 213 , 112735. [ Google Scholar ] [ CrossRef ]
- Zhang, M.; Shi, H.; Zhang, Y.; Yu, Y.; Zhou, M. Deep learning-based damage detection of mining conveyor belt. Measurement 2021 , 175 , 109130. [ Google Scholar ] [ CrossRef ]
- Rumin, P.; Kotowicz, J.; Hogg, D.; Zastawna-Rumin, A. Utilization of measurements, machine learning, and analytical calculation for preventing belt flip over on conveyor belts. Measurement 2023 , 218 , 113157. [ Google Scholar ] [ CrossRef ]
- Zhang, M.; Cao, Y.; Jiang, K.; Li, M.; Liu, L.; Yu, Y.; Zhou, M.; Zhang, Y. Proactive measures to prevent conveyor belt Failures: Deep Learning-based faster foreign object detection. Eng. Fail. Anal. 2022 , 141 , 106653. [ Google Scholar ] [ CrossRef ]
- Kirjanow-Blazej, A.; Jurdziak, L.; Burduk, R.; Blazej, R. Forecast of the remaining lifetime of steel cord conveyor belts based on regression methods in damage analysis identified by subsequent DiagBelt scans. Eng. Fail. Anal. 2019 , 100 , 119–126. [ Google Scholar ] [ CrossRef ]
- Fedorko, G.; Molnar, V.; Marasova, D.; Grincova, A.; Dovica, M.; Zivcak, J.; Toth, T.; Husakova, N. Failure analysis of belt conveyor damage caused by the falling material. Part I: Experimental measurements and regression models. Eng. Fail. Anal. 2014 , 36 , 30–38. [ Google Scholar ] [ CrossRef ]
- Fedorko, G.; Molnar, V.; Marasova, D.; Grincova, A.; Dovica, M.; Zivcak, J.; Toth, T.; Husakova, N. Failure analysis of belt conveyor damage caused by the falling material. Part II: Application of computer metrotomography. Eng. Fail. Anal. 2013 , 34 , 431–442. [ Google Scholar ] [ CrossRef ]
- Homisin, J.; Grega, R.; Kassay, P.; Fedorko, G.; Molnar, V. Removal of a systematic failure of belt conveyor drive by reducing vibrations. Eng. Fail. Anal. 2019 , 99 , 192–202. [ Google Scholar ] [ CrossRef ]
- Vasic, M.; Stojanovic, B.; Blagojevic, M. Failure analysis of idler roller bearings in belt conveyors. Eng. Fail. Anal. 2020 , 117 , 104898. [ Google Scholar ] [ CrossRef ]
- ContiTech Deutschland GmbH Causes of Belt Mistracking. Available online: https://www.continental-industry.com/belt-mistracking (accessed on 10 April 2024).
- Kobayashi, Y.; Toya, K. Effect of belt transport speed and other factors on belt mistracking. Microsyst. Technol. 2007 , 13 , 1325–1330. [ Google Scholar ] [ CrossRef ]
- Zamiralova, M.E.; Lodewijks, G. Shape stability of pipe belt conveyors: From throughability to pipe-ability. FME Trans. 2016 , 44 , 263–271. [ Google Scholar ] [ CrossRef ]
- Otto, H.; Katterfeld, A. Belt Mistracking—Simulation and Measurements of Belt Sideways Dynamics. In Proceedings of the 13th International Conference on Bulk Materials Storage, Handling and Transportation, Gold Coast, Australia, 9–11 July 2019. [ Google Scholar ]
- Grevenstuk, R. Getting Back on Track. Available online: https://www.mhea.co.uk/wp-content/uploads/2015/06/FlexcoFNL.pdf (accessed on 10 April 2024).
- Otto, H.; Katterfeld, A. Prediction and simulation of mistracking of conveyors belts. In Proceedings of the 8th International Conference on Conveying and Handling of Particulate Solids (CHOPS 2015), Tel Aviv, Israel, 3–7 May 2015; pp. 1–11. [ Google Scholar ]
- Dabek, P.; Wroblewski, A.; Wodecki, J.; Bortnowski, P.; Ozdoba, M.; Krol, R.; Zimroz, R. Application of the Methods of Monitoring and Detecting the Belt Mistracking in Laboratory Conditions. Appl. Sci. 2023 , 13 , 2111. [ Google Scholar ] [ CrossRef ]
- Wu, J.; Yang, Y.; Cheng, X.; Zuo, H.; Cheng, Z. The Development of Digital Twin Technology Review. In Proceedings of the 2020 Chinese Automation Congress (CAC 2020), Shanghai, China, 6–8 November 2020; pp. 4901–4906. [ Google Scholar ]
- Bondoc, A.E.; Tayefeh, M.; Barari, A. LIVE Digital Twin: Developing a Sensor Network to Monitor the Health of Belt Conveyor System. IFAC Pap. 2022 , 55 , 49–54. [ Google Scholar ] [ CrossRef ]
- R Core Team. R: A Language and Environment for Statistical Computing. Available online: https://www.r-project.org/ (accessed on 10 April 2024).
- Fisher, R.A. Statistical Methods for Research Workers ; Cosmo Study Guides; Cosmo Publications: Delhi, India, 2006; ISBN 9788130701332. [ Google Scholar ]
- Keynes, J.M. A Treatise on Probability ; Macmillan: London, UK, 1921; ISBN 0486159647. [ Google Scholar ]
- Fedorko, G.; Molnar, V.; Vasil, M.; Salai, R. Proposal of a digital twin for testing and measuring transport belts for pipe conveyors within the concept Industry 4.0. Measurement 2021 , 174 , 108978. [ Google Scholar ] [ CrossRef ]
- Zidek, K.; Pitel, J.; Adamek, M.; Lazorik, P.; Hosovsky, A. Digital Twin of Experimental Smart Manufacturing Assembly System for Industry 4.0 Concept. Sustainability 2020 , 12 , 3658. [ Google Scholar ] [ CrossRef ]
- Tukey, J.W. Comparing individual means in the analysis of variance. Biometrics 1949 , 5 , 99–114. [ Google Scholar ] [ CrossRef ] [ PubMed ]
- Hartley, H.O. The maximum F-ratio as a short-cut test for variance heterogeneity. Biometrika 1950 , 37 , 308–312. [ Google Scholar ] [ CrossRef ]
- Shapiro, S.S.; Wilk, M.B. An analysis of variance test for normality (complete samples). Biometrika 1965 , 52 , 591–611. [ Google Scholar ] [ CrossRef ]
Set Tension Force [N] | Set Asymmetry Level (Difference TF23–TF24 [N] in ID23, ID24) |
---|---|
36,000 | asym_0 (0 N) |
40,000 | asym_1 (1000 N) |
44,000 | asym_-1 (−1000 N) |
asym_2 (2000 N) | |
asym_-2 (−2000 N) |
Position | Asymmetry Type | Statistical Indicators | ||||||||
---|---|---|---|---|---|---|---|---|---|---|
asym_0 | asym_1 | asym_-1 | asym_2 | asym_-2 | Min | Max | Range | Average | Ratio | |
ID1 | 108 | 107 | 106 | 109 | 106 | 106 | 109 | 3 | 107 | 3% |
ID2 | 39 | 39 | 39 | 41 | 39 | 39 | 41 | 2 | 40 | 5% |
ID3 | 110 | 105 | 107 | 111 | 109 | 105 | 111 | 5 | 108 | 5% |
ID4 | 332 | 334 | 334 | 338 | 334 | 332 | 338 | 6 | 334 | 2% |
ID5 | 311 | 318 | 319 | 323 | 318 | 311 | 323 | 12 | 318 | 4% |
ID6 | 354 | 356 | 356 | 359 | 356 | 354 | 359 | 5 | 356 | 1% |
ID7 | 30 | 28 | 29 | 29 | 31 | 28 | 31 | 3 | 30 | 9% |
ID8 | 23 | 23 | 24 | 24 | 24 | 23 | 24 | 1 | 24 | 4% |
ID9 | 33 | 34 | 34 | 35 | 33 | 33 | 35 | 2 | 34 | 6% |
ID10 | 110 | 112 | 112 | 113 | 111 | 110 | 113 | 4 | 112 | 3% |
ID11 | 73 | 74 | 75 | 77 | 76 | 73 | 77 | 4 | 75 | 5% |
ID12 | 112 | 111 | 112 | 113 | 113 | 111 | 113 | 2 | 112 | 2% |
ID13 | 58 | 56 | 56 | 56 | 56 | 56 | 58 | 2 | 56 | 4% |
ID14 | 39 | 39 | 39 | 39 | 38 | 38 | 39 | 1 | 39 | 2% |
ID14 | 39 | 39 | 39 | 39 | 38 | 38 | 39 | 1 | 39 | 2% |
ID15 | 90 | 93 | 93 | 93 | 93 | 90 | 93 | 3 | 92 | 3% |
ID16 | 116 | 118 | 118 | 118 | 117 | 116 | 118 | 2 | 117 | 2% |
ID17 | 61 | 60 | 60 | 60 | 59 | 59 | 61 | 2 | 60 | 4% |
ID18 | 94 | 89 | 88 | 88 | 87 | 87 | 94 | 7 | 89 | 8% |
ID19 | 18 | 22 | 12 | 23 | 8 | 8 | 23 | 14 | 17 | 85% |
ID20 | 12 | 7 | 16 | 8 | 19 | 7 | 19 | 12 | 13 | 95% |
ID23 | 19,846 | 20,218 | 19,383 | 20,713 | 18,813 | 18,813 | 20,713 | 1899 | 19,795 | 10% |
ID24 | 19,757 | 19,270 | 20,358 | 19,006 | 20,879 | 19,006 | 20,879 | 1873 | 19,854 | 9% |
Asymmetry | p adj |
---|---|
asym_1–asym_0 | 1 × 10 |
asym_-1–asym_0 | 1 × 10 |
asym_2–asym_0 | 0 |
asym_-2–asym_0 | 0 |
asym_-1–asym_1 | 0 |
asym_2–asym_1 | 0 |
asym_-2–asym_1 | 0 |
asym_2–asym_-1 | 0 |
asym_-2–asym_-1 | 4 × 10 |
asym_-2–asym_2 | 0 |
Parameter | Estimate | Std. Error | t-Value | Pr(>|t|) |
---|---|---|---|---|
a | –0.068193 | 0.009131 | –7.468 | 6.27 × 10 |
a | 0.088633 | 0.021157 | 4.189 | 0.000285 |
a | 0.027980 | 0.005911 | 4.733 | 7.9 × 10 |
TRUE | ||
Classified | 0 | 1 |
0 | 27 | 3 |
1 | 0 | 64 |
The statements, opinions and data contained in all publications are solely those of the individual author(s) and contributor(s) and not of MDPI and/or the editor(s). MDPI and/or the editor(s) disclaim responsibility for any injury to people or property resulting from any ideas, methods, instructions or products referred to in the content. |
Share and Cite
Fedorko, G.; Molnar, V.; Stehlikova, B.; Michalik, P.; Saliga, J. Design of Evaluation Classification Algorithm for Identifying Conveyor Belt Mistracking in a Continuous Transport System’s Digital Twin. Sensors 2024 , 24 , 3810. https://doi.org/10.3390/s24123810
Fedorko G, Molnar V, Stehlikova B, Michalik P, Saliga J. Design of Evaluation Classification Algorithm for Identifying Conveyor Belt Mistracking in a Continuous Transport System’s Digital Twin. Sensors . 2024; 24(12):3810. https://doi.org/10.3390/s24123810
Fedorko, Gabriel, Vieroslav Molnar, Beata Stehlikova, Peter Michalik, and Jan Saliga. 2024. "Design of Evaluation Classification Algorithm for Identifying Conveyor Belt Mistracking in a Continuous Transport System’s Digital Twin" Sensors 24, no. 12: 3810. https://doi.org/10.3390/s24123810
Article Metrics
Further information, mdpi initiatives, follow mdpi.
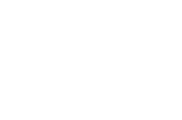
Subscribe to receive issue release notifications and newsletters from MDPI journals

IMAGES
VIDEO
COMMENTS
6. Write a null hypothesis. If your research involves statistical hypothesis testing, you will also have to write a null hypothesis. The null hypothesis is the default position that there is no association between the variables. The null hypothesis is written as H 0, while the alternative hypothesis is H 1 or H a.
3. Simple hypothesis. A simple hypothesis is a statement made to reflect the relation between exactly two variables. One independent and one dependent. Consider the example, "Smoking is a prominent cause of lung cancer." The dependent variable, lung cancer, is dependent on the independent variable, smoking. 4.
It seeks to explore and understand a particular aspect of the research subject. In contrast, a research hypothesis is a specific statement or prediction that suggests an expected relationship between variables. It is formulated based on existing knowledge or theories and guides the research design and data analysis. 7.
A hypothesis is a tentative statement about the relationship between two or more variables. It is a specific, testable prediction about what you expect to happen in a study. It is a preliminary answer to your question that helps guide the research process. Consider a study designed to examine the relationship between sleep deprivation and test ...
Definition: Hypothesis is an educated guess or proposed explanation for a phenomenon, based on some initial observations or data. It is a tentative statement that can be tested and potentially proven or disproven through further investigation and experimentation. Hypothesis is often used in scientific research to guide the design of experiments ...
The steps to write a research hypothesis are: 1. Stating the problem: Ensure that the hypothesis defines the research problem. 2. Writing a hypothesis as an 'if-then' statement: Include the action and the expected outcome of your study by following a 'if-then' structure. 3.
Research question. Interest in a particular topic usually begins the research process, but it is the familiarity with the subject that helps define an appropriate research question for a study. 1 Questions then arise out of a perceived knowledge deficit within a subject area or field of study. 2 Indeed, Haynes suggests that it is important to know "where the boundary between current ...
Step 5: Phrase your hypothesis in three ways. To identify the variables, you can write a simple prediction in if … then form. The first part of the sentence states the independent variable and the second part states the dependent variable. If a first-year student starts attending more lectures, then their exam scores will improve.
HYPOTHESIS TESTING. A clinical trial begins with an assumption or belief, and then proceeds to either prove or disprove this assumption. In statistical terms, this belief or assumption is known as a hypothesis. Counterintuitively, what the researcher believes in (or is trying to prove) is called the "alternate" hypothesis, and the opposite ...
If your null hypothesis was rejected, this result is interpreted as "supported the alternate hypothesis." Stating results in a research paper We found a difference in average height between men and women of 14.3cm, with a p-value of 0.002, consistent with our hypothesis that there is a difference in height between men and women.
Identify the variables involved. Formulate a clear and testable prediction. Use specific and measurable terms. Align the hypothesis with the research question. Distinguish between the null hypothesis (no effect) and alternative hypothesis (expected effect). Ensure the hypothesis is falsifiable and subject to empirical testing.
Step 3: Formulate a Clear Statement. Precision is the key to shaping a concise and strong hypothesis. To create a well-structured hypothesis, condense your thoughts into a single, easy-to-follow sentence. Also, do not forget to clearly express the expected connection between your independent and dependent variables.
Your hypothesis is what you propose to "prove" by your research. As a result of your research, you will arrive at a conclusion, a theory, or understanding that will be useful or applicable beyond the research itself. 3. Avoid judgmental words in your hypothesis. Value judgments are subjective and are not appropriate for a hypothesis.
A snapshot analysis of citation activity of hypothesis articles may reveal interest of the global scientific community towards their implications across various disciplines and countries. As a prime example, Strachan's hygiene hypothesis, published in 1989,10 is still attracting numerous citations on Scopus, the largest bibliographic database ...
A research hypothesis is your proposed answer to your research question. The research hypothesis usually includes an explanation ("x affects y because …"). A statistical hypothesis, on the other hand, is a mathematical statement about a population parameter. Statistical hypotheses always come in pairs: the null and alternative hypotheses.
Ensure that your hypothesis is realistic and can be tested within the constraints of your available resources, time, and ethical considerations. Avoid value judgments: Be neutral and objective. Avoid including personal beliefs, value judgments, or subjective opinions. Stick to empirical statements based on evidence.
A scientific hypothesis is a tentative, testable explanation for a phenomenon in the natural world. It's the initial building block in the scientific method.Many describe it as an "educated guess ...
Research problems and hypotheses are important means for attaining valuable knowledge. They are pointers or guides to such knowledge, or as formulated by Kerlinger ( 1986, p. 19): " … they direct investigation.". There are many kinds of problems and hypotheses, and they may play various roles in knowledge construction.
Our corpus has focussed on identifying Research Hypotheses and New Knowledge in biomedical abstracts. However, it has been shown elsewhere that full texts contain more information than abstracts alone . Whilst our future goal is to additionally facilitate the recognition of New Knowledge and Research Hypothesis in full papers, our decision to ...
Offers detailed guidance on how to develop, organize, and write a college-level research paper in the social and behavioral sciences. ... (October 2018): 1-13; Li, Yanmei, and Sumei Zhang. "Identifying the Research Problem." In Applied Research Methods in Urban and ... the researcher can formulate a research problem or hypothesis stating the ...
Identifying a hypothesis allows students to know what is being proven by a particular experiment or paper. Being able to determine the overall point not only makes you a more effective reader but also better at formulating your own theories when writing your own paper. By asking a few simple questions while you read, ...
INTRODUCTION. Scientific research is usually initiated by posing evidenced-based research questions which are then explicitly restated as hypotheses.1,2 The hypotheses provide directions to guide the study, solutions, explanations, and expected results.3,4 Both research questions and hypotheses are essentially formulated based on conventional theories and real-world processes, which allow the ...
When conducting research, scientists use the scientific method to collect measurable, empirical evidence in an experiment related to a hypothesis (often in the form of an if/then statement) that ...
The examination of a research article is an important process, and the ability to identify crucial elements of research is paramount for the effective analysis of a research article. Research articles are usually arranged in specific ways. A hypothesis in a research article is usually located in a specific position in an article.
Research Article. Genomic analyses of Symbiomonas scintillans show no evidence for endosymbiotic bacteria but does reveal the presence of giant viruses. A multi-gene tree showed the three SsV genome types branched within highly supported clades with each of BpV2, OlVs, and MpVs, respectively.
Automated hypothesis generation (HG) focuses on uncovering hidden connections within the extensive information that is publicly available. This domain has become increasingly popular, thanks to modern machine learning algorithms. However, the automated evaluation of HG systems is still an open problem, especially on a larger scale. This paper presents a novel benchmarking framework Dyport for ...
In the paper, the scientists explained the hypothesis for aliens on Earth. The researchers said that they believe that "remnant forms," which are an ancient, highly advanced human civilization ...
Within this paper, research will be presented aiming to verify the hypothesis that, based on a measurement of selected parameters, it is possible to identify belt mistracking in a continuous transport system. ... To research the possibility of identifying and quantifying the asymmetry of the belt's tensioning, repeated experimental ...