- DSpace@MIT Home
- MIT Libraries
This collection of MIT Theses in DSpace contains selected theses and dissertations from all MIT departments. Please note that this is NOT a complete collection of MIT theses. To search all MIT theses, use MIT Libraries' catalog .
MIT's DSpace contains more than 58,000 theses completed at MIT dating as far back as the mid 1800's. Theses in this collection have been scanned by the MIT Libraries or submitted in electronic format by thesis authors. Since 2004 all new Masters and Ph.D. theses are scanned and added to this collection after degrees are awarded.
MIT Theses are openly available to all readers. Please share how this access affects or benefits you. Your story matters.
If you have questions about MIT theses in DSpace, [email protected] . See also Access & Availability Questions or About MIT Theses in DSpace .
If you are a recent MIT graduate, your thesis will be added to DSpace within 3-6 months after your graduation date. Please email [email protected] with any questions.

Permissions
MIT Theses may be protected by copyright. Please refer to the MIT Libraries Permissions Policy for permission information. Note that the copyright holder for most MIT theses is identified on the title page of the thesis.
Theses by Department
- Comparative Media Studies
- Computation for Design and Optimization
- Computational and Systems Biology
- Department of Aeronautics and Astronautics
- Department of Architecture
- Department of Biological Engineering
- Department of Biology
- Department of Brain and Cognitive Sciences
- Department of Chemical Engineering
- Department of Chemistry
- Department of Civil and Environmental Engineering
- Department of Earth, Atmospheric, and Planetary Sciences
- Department of Economics
- Department of Electrical Engineering and Computer Sciences
- Department of Humanities
- Department of Linguistics and Philosophy
- Department of Materials Science and Engineering
- Department of Mathematics
- Department of Mechanical Engineering
- Department of Nuclear Science and Engineering
- Department of Ocean Engineering
- Department of Physics
- Department of Political Science
- Department of Urban Studies and Planning
- Engineering Systems Division
- Harvard-MIT Program of Health Sciences and Technology
- Institute for Data, Systems, and Society
- Media Arts & Sciences
- Operations Research Center
- Program in Real Estate Development
- Program in Writing and Humanistic Studies
- Science, Technology & Society
- Science Writing
- Sloan School of Management
- Supply Chain Management
- System Design & Management
- Technology and Policy Program
Collections in this community
Doctoral theses, graduate theses, undergraduate theses, recent submissions.
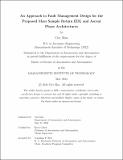
An Approach to Fault Management Design for the Proposed Mars Sample Return EDL and Ascent Phase Architectures
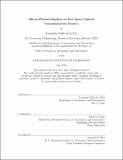
Silicon Photomultipliers as Free Space Optical Communication Sensors

Study of Cavity Geometry to Improve Optical Quality of Windows in Hypersonic Flow
Show Statistical Information


Member-only story
Five Tips For Writing A Great Data Science Thesis
Write for your reader, not for yourself.

Wouter van Heeswijk, PhD
Towards Data Science
In this article, I will share some tips on how to improve your Data Science thesis . Over the years, I have supervised my share of Data Science thesis projects, ranging from Big Four firms to local SMEs and from multinational banks to software consultancies. The academic program I am active typically involves…
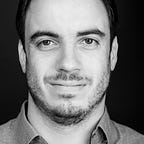
Written by Wouter van Heeswijk, PhD
Assistant professor in Financial Engineering and Operations Research. Writing about reinforcement learning, optimization problems, and data science.
Text to speech

Undergraduate Research
Main navigation, stanford data science undergraduate research pathways (dsurp).
The Stanford Data Science Undergraduate Research Pathways program is an 8-week full-time research experience designed to provide students at institutions without access to research opportunities the chance to conduct a research project under the supervision of both a mentor and faculty member. This is an in-person experience held at Stanford from June 24 to August 16, 2024.
- The program is held during the Stanford summer quarter from June 24–August 16 (8 weeks).
- Participants will receive a stipend of $6000, but the program is not otherwise able to provide housing support.
- Available slots are limited and selection is competitive. Priority is given first to students from non-R1 universities, and also to those from backgrounds underrepresented in data science research.
- The program is not open to Stanford students.
How to Apply
Applicants will need to provide the following by 11:59 pm PST on March 3, 2024:
- Personal and demographic information.
- Resume and unofficial transcript.
- Demonstration of data science reasoning ability.
Applications will open in the next academic year (2024-2025)*
Any questions should be directed by email to Daniel LeJeune .
* The Stanford Data Science Undergraduate Pathways (DSURP) program recognizes that the Supreme Court issued a ruling in June 2023 about the consideration of certain types of demographic information as part of an admission review. All applications submitted during upcoming application cycles will be reviewed in conformance with that decision.
- Search This Site All UCSD Sites Faculty/Staff Search Term
- Courses/Curricula/Faculty
- About UC San Diego
- Academic Integrity
- Regulations & Policies
- Additional Resources
- Undergraduate Education Overview
- Degrees Offered
- Degree Requirements
- Registration
- Graduation Requirements
- Graduate Financial
- General Requirements for Higher Degrees
- Graduate Education Overview
- Graduate Admission
- Graduate & Professional Schools
Data Science
[ undergraduate program | courses | faculty ]
All courses, faculty listings, and curricular and degree requirements described herein are subject to change or deletion without notice.
The field of data science spans mathematical models, computational methods, and analysis tools for navigating and understanding data and applying these skills to a broad and emerging range of application domains. A whole range of industries—from drug discovery to healthcare management, from manufacturing to enterprise business processes as well as government organizations—are creating demand for data scientists with a skill set that enables them to create mathematical models of data, identify trends and patterns using suitable algorithms, and present the results in effective manners. The target systems can be, for example, biological (e.g., clinical data from cancer patients), physical (e.g., transportation networks), social (e.g., social networks), or cyber-physical (e.g., smart grids). In all these cases, there is a combination of core knowledge in information processing coupled with the skills to abstract, build, and test predictive and descriptive models that must be taught and learned in the context of an application domain. These application areas are in many domains served by engineering, physical sciences, social sciences, health and life sciences, and arts and humanities.
The Halıcıoğlu Data Science Institute’s (HDSI) data science programs are structured to provide access to education in data science for students drawn from diverse backgrounds. As a fundamentally quantitative discipline, an undergraduate education in quantitative disciplines is assumed. These include bachelor’s and/or master’s degrees in a quantitative field such as engineering, computer science, mathematics, statistics, cognitive science, disciplines in physical or life sciences, as well as quantitative social sciences such as econometrics, economics, or computational social sciences. Other degree options are acceptable with demonstrated course work or experience in programming, calculus, probability, and statistics.
For a listing of current participating faculty, please visit: https://datascience.ucsd.edu/about/faculty/
Overview of Graduate Degree Programs in Data Science
The Halıcıoğlu Data Science Institute (HDSI) offers the following three graduate degree programs in the data science area:
- A residential degree program in master of science in data science, MS/DS
- A residential degree program in doctor of philosophy in data science, PhD/DS
- An online degree program in master of data science (online), MDS
The online and residential degree programs have different admission requirements and processes that are described separately. Admission into one degree program does not automatically imply admission into any other degree program. For students enrolled in the residential MS/DS program, an independent application is needed for admission into the PhD/DS degree program. For students in the PhD/DS program, a residential MS/DS degree can be earned by following prescribed review and approval processes.
Residential Graduate Degree Programs
Admission to the residential degree programs in data science is done through the Graduate Division at UC San Diego. The application deadline is December for admissions effective the following fall quarter. For admission deadline and requirements, please refer to the departmental web page: http://datascience.ucsd.edu .
Admission decisions for the MS and PhD programs are made separately. A current MS student who wishes to enter the PhD program must submit a petition, including a new statement of purpose and three new letters of recommendation, to the HDSI graduate admissions committee.
Online Graduate Degree Program
The online master of data science (MDS) is the first degree program of its kind at the University of California, San Diego. The program is designed with the express goal of broadening participation into the growing field of data science by attracting talent from very different fields that are likely to benefit from advances in data science. The MDS program is also designed for working professionals who are able to pace their learning in view of their work-life balance. The fully online program is offered with a mostly asynchronous course delivery in order to accommodate students in different time zones and with varying work schedules. The curriculum combines theoretical foundations with practical application by combining concepts from statistics, computer science, and applications where data is forefront and center. This includes processes and their models that generate data; methods and tools that enable us to store, analyze, understand, and visualize data; the interaction of data systems with humans and physical systems; and societal impact. The online program has a separate admissions cycle from the residential graduate programs. See the program website for more information.
Doctor of Philosophy (PhD) in Data Science
The goal of the doctoral program is to create leaders in the field of data science who will lay the foundation and expand the boundaries of knowledge in the field. The doctoral program aims to provide a research-oriented education to students, teaching them the knowledge, skills, and awareness required to perform data driven research, and enabling them to, using this shared background, carry out research that expands the boundaries of knowledge in data science. The doctoral program spans from foundational aspects, including computational methods, machine learning, mathematical models, and statistical analysis, to applications in data science.
Admission into the Program
A PhD degree in data science is an advanced degree that prepares students for leadership in data science research in academia, industry, and civic organizations. To be successful in this program, the students must have a background in quantitative analysis typically seen in degree programs with substantial mathematical preparation and programming skills. Admissions requirements for the PhD program are:
- Bachelor’s and/or master’s degree in a quantitative field such as engineering, computer science, mathematics, statistics, cognitive science, scientific disciplines, or quantitative social sciences such as economics or computational social science. Other degree options are acceptable with demonstrated course work or experience in programming, calculus, probability, and statistics.
- Undergraduate GPA of at least 3.0 on a 4.0 scale.
- College transcripts.
- Three letters of recommendation.
- Optional GRE requirements as per the latest guidance from the Graduate Division at UC San Diego.
- A statement of purpose that clearly outlines the motivation, background preparation, any relevant work experience in data science related areas, and topical interests for a degree in data science. Prospective students would be asked to identify any faculty members that they would like to seek as a research adviser.
- The Test of English as a Foreign Language (TOEFL): The minimum TOEFL score for admission is 85 for the internet-based test and 64 for the paper-based test. Please note the paper-based test does not have a speaking component.
- The International English Language Testing System (IELTS) Academic Training exam: The minimum IELTS score is band 7.0.
- The Pearson Test of English Academic (PTE Academic). The minimum PTE academic score required for graduate admission is an overall score of 65.
Academic Preparation of Students Entering the PhD Program
Given the novelty of the degree programs in data science at the undergraduate level, we anticipate entering students to the graduate program with undergraduate training in areas outside of data science. In fact, the graduate program is designed to enable maximum participation of interested students from diverse educational backgrounds. However, ensuring a successful and timely completion of the graduate degree program requires academic preparation in five key areas of data science at the undergraduate level: algorithmic and programming skills, data organization methods and skills, numerical linear algebra, multivariate calculus, probability, and statistics.
While students with an undergraduate degree from a data science major or data science minor would have taken courses in all the five areas mentioned, we expect that students graduating from other quantitative undergraduate programs would have knowledge in the majority of the five areas mentioned above. There will be incoming students who would be lacking requisite knowledge and skills in some of these areas. To fill this gap, the program offers a set of foundational courses described in the next section.
These courses are designed to serve the needs of three classes of incoming students: (a) students with preparation in computing and/or information sciences at a level to master algorithmic programming and cloud computing skills; (b) students with preparation in mathematical subjects at a level to master statistical analysis and probability necessary for meaningful data analysis; (c) students who enter the program from other areas of science that rely upon collecting and analyzing observational or experimental data in order to advance scientific understanding. These are students with a degree in natural sciences such as physics, chemistry, biology, environmental sciences, etc., or coming from a social science background such as economics, political science, psychology, etc. Application examples may be causal inference in economics, assessing statistical significance of a pharmaceutical experiment or psychological treatment, the study of social networks in political science, etc.
We note that these are broad and overlapping categories. Even when students come prepared in both advanced computing and mathematics/statistics, data science education challenges them to apply these skills meaningfully in diverse applications, as well as improve their visualization/presentation skills. To do this successfully, students may need a working knowledge of the topics they may have already studied. As a result, Group A courses normalize background preparation of all our students with options that enable them to skip courses as appropriate but under careful supervision and advising discussed next.
Our graduate admissions process uses text analysis methods to automatically sort and bin admitted students into three pools as above, and thus drive the subsequent advising process; this will also include prior communication with the students regarding their preparation options using online and other offers by UC San Diego and other organizations.
Within the first week of arrival, each student will be scheduled for a one-on-one meeting with a faculty adviser and/or graduate program academic coordinator. After meeting with their faculty adviser, newly admitted students may be directed to take specific upper-division undergraduate courses from different areas, in order to solidify their backgrounds when or if there is some perceived weakness; up to two such courses may count towards their PhD degree units. The faculty adviser will also determine if an incoming student has strength in a particular area, and can thus avoid taking the area-associated course(s) among the five foundational courses of Group A.
The institute also offers preterm summer boot camp programs to help entering students with background preparation.
Course Requirements
There are foundation, core, and elective and research requirements for the graduate program. These course requirements are intended to ensure that students are exposed to (1) fundamental concepts and tools (foundation), (2) advanced, up-to-date views in topics central to data science for all students (core requirement), and (3) a deep, current view of their research or application (elective requirement). Courses may not fulfill more than one requirement.
The doctoral program is structured as a total of fifty-two units in courses grouped into foundational, core, professional preparation, and research experience areas as described below. Successful completion of the program requires successful and timely completion of three examinations and completion of a doctoral dissertation . Out of the fifty-two units, forty-eight units (or twelve courses) must be taken for letter grade and at least forty units must be using graduate-level courses.
The remaining four (= 52–48) units are for professional preparation , consisting of one unit of faculty research seminar, two units of TA/tutor training, and one unit of a survival skills course taken for a passing (satisfactory) grade. Finally, as mentioned earlier, out of the twelve regular courses, at least ten must be graduate-level courses; at most two can be upper-division undergraduate courses. Thirty-six units or nine courses must be completed within six quarters from the start of the degree program.
Group A, Group B, and Group C. Group A courses are introductory-level graduate courses in the foundational areas of data science. Group B are core graduate-level courses with prerequisites from Group A courses. Group C are advanced, specialized, and free-standing courses, often part of the required courses in the data science specialization of the graduate program in other departments. In all three groups, required courses are indicated as such; they cannot be substituted by other courses without exception approval from the graduate program committee.
Group A: Preparatory Courses
Group A courses are foundational courses for data science. All students are required to understand the material in the Group A courses. Students must either take a Group A course or successfully petition a previously taken undergraduate upper-division course equivalent to waive the requirement. All petitions must be processed prior to the start of fall quarter.
There are five important knowledge and skills areas necessary for understanding (and advancing) core data science. It is, therefore, important that all our entering students either have background preparation or courses available in the program to ensure a successful completion of the stipulated doctoral degree program. A student can receive credit towards the PhD degree for a maximum of three courses from the list of courses below:
- DSC 200. Data Science Programming
- DSC 202. Data Management for Data Science
- DSC 210. Numerical Linear Algebra
- DSC 211. Introduction to Optimization
- DSC 212. Probability and Statistics for Data Science
Group B: Core Courses
Four core courses are required for all PhD students, including those with a bachelor’s degree in data science. The four required courses are:
- DSC 240. Machine Learning
- DSC 260. Data Ethics and Fairness
- (*)DSC 241. Statistical Models (or MATH 282B)
- (*)DSC 204A. Scalable Data Systems (or CSE 202 or DSC 206 )
(*) Depending on academic preparation, a PhD student can take an advanced course on applied statistics, such as MATH 282B instead of DSC 241. Similarly, instead of DSC 204A, a student can take a course on algorithms, such as CSE 202, Design and Analysis of Algorithms, or DSC 206, Algorithms for Data Science .
In addition, a doctoral student must select at least two out of the following eight core courses:
- DSC 203. Data Visualization and Scalable Visual Analytics
- DSC 204B. Big Data Analytics and Applications
- DSC 242. High-Dimensional Probability and Statistics
- DSC 243. Advanced Optimization
- DSC 244. Large-Scale Statistical Analysis
- DSC 245. Introduction to Causal Inference
- DSC 250. Advanced Data Mining
- DSC 261. Responsible Data Science
Thus, doctoral students are required to take a minimum of six courses for letter-grade credit from Group B courses. Students can take more than six courses from this group to satisfy letter-grade course requirements except (satisfactory completion of professional preparation) teaching, survival skills, and research seminar courses. Students who satisfy all letter-grade course requirements are expected to enroll in individual research (DSC 298) in a section offered by the faculty adviser to meet residency requirements and maintain graduate student standing during the period of dissertation research.
Group C: Professional Preparation and Elective Courses
Group C courses aim to provide either practical experiences in chosen specialization areas or advanced training for students preparing for doctoral programs. The courses include required professional preparation courses: two-unit TA/tutor training (DSC 599), one unit of academic survival skills (DSC 295), and one-unit faculty research seminar (DSC 293), all of which must be completed with a Satisfactory (S) grade using the S/U option.
Professional Preparation Courses
- DSC 599. TA/Tutor Training
- DSC 293. Faculty Research Seminar
- DSC 294. Research Rotation
- DSC 295. Academia Survival Skills
General Elective and Specialization Courses
Courses here aim to provide advanced training for students in the doctoral programs, or practical experiences in chosen specialization areas. These specializations are internal and do not show in students’ transcripts. However, students are welcome to cite their specializations in their curriculum vitae. Elective courses will be offered based on faculty interest and availability.
Research Rotation Program
Research rotations provide the opportunity for first-year PhD students to obtain research experience under the guidance of HDSI faculty members. Through the rotations, students can identify a faculty member under whose supervision their dissertation research will be completed.
A research rotation is a guided research experience lasting one quarter (ten weeks) obtained by registering for DSC 294 with an instructor. All PhD students will participate in a minimum of two research rotations during their first year , and with a minimum of two different faculty members and as many as four rotations including summer quarter. A student may rotate twice under the same faculty member as long as they rotate with at least two different faculty members. The goal is to help the student identify and develop their research interests and to expose students to new methodological approaches or domain knowledge that may be outside the scope of their eventual thesis.
Research rotations must be completed before the end of the second year with a signed commitment form from a faculty adviser. Those who fail to identify a research adviser may be advised to leave the doctoral program with an optional assessment for completion of a terminal MS/DS degree.
Preliminary Assessment Examination
The preliminary assessment is an advisory examination. It consists of an oral examination in an area selected by the student with the goal to assess the student’s preparation for the proposed area, including several relevant topics, and identify any courses that are required or recommended for the candidate based on knowledge shown and critical missing background revealed.
The preliminary examination must be completed before the end of the second year in the doctoral degree program. The examination dates are announced no later than the start of the winter quarter along with the logistical details of the preliminary examination conducted by the graduate committee of HDSI. A failing grade in the preliminary examination would include a recommendation for the opportunity to receive a terminal MS/DS degree, provided the student can meet the degree requirements in no more than one extra quarter over the standard time for the MS program. Students who fail the preliminary examination may file a petition to retake it; if the petition is approved, they will be allowed to retake it one (and only one) more time.
After a student successfully completes the preliminary assessment examination, in the next annual review of the student (conducted in the fall quarter), the departmental committee on graduate affairs of the HDSI faculty council assigns the academic adviser to provide necessary updates to the departmental committee on graduate affairs and to help the student select the doctoral dissertation committee and schedule when the research qualifying examination and dissertation defense will occur .
Research Qualifying Examination (UQE)
A research qualifying examination (UQE) is conducted by the dissertation committee. One senate faculty member must have a primary appointment in the department outside of HDSI. Faculty with 25 percent or less partial appointment in HDSI may be considered for meeting this requirement on an exceptional basis upon approval from the Graduate Division.
The goal of UQE is to assess the ability of the candidate to perform independent critical research as evidenced by a presentation and writing a technical report at the level of a peer-reviewed journal or conference publication. The examination is taken after the student and his or her adviser have identified a topic for the dissertation and an initial demonstration of feasible progress has been made. The candidate is expected to describe his or her accomplishments to date as well as future work. The research qualifying examination must be completed no later than fourth year or twelve quarters from the start of the degree program; the UQE is tantamount to advancement to the PhD candidacy exam.
A petition to the graduate committee is required for students who take UQE after the required twelve quarters deadline. Students who fail the research qualifying examination may file a petition to retake it; if the petition is approved, they will be allowed to retake it one (and only one) more time. Students who fail UQE may also petition to transition to a MS/DS track.
Dissertation Defense Examination and Thesis Requirements
Students must successfully complete a final dissertation defense presentation and examination to the doctoral committee. One senate faculty member must have a primary appointment in the department outside of HDSI. As explained earlier, partially appointed faculty in HDSI (at 25 percent or less) are acceptable in meeting this outside department requirement as long as their main (lead) department is not HDSI.
A dissertation in the scope of data science is required of every candidate for the PhD degree. HDSI PhD program thesis requirements must meet Regulation 715 requirements. The final form of the dissertation document must comply with published guidelines by the Graduate Division.
Special Requirements: Professional Training and Communications
All graduate students in the doctoral program are required to complete at least one quarter of experience in the classroom as teaching assistants regardless of their eventual career goals. Effective communications and ability to explain deep technical subjects is considered a key measure of a well-rounded doctoral education. Thus, PhD students are also required to take a one-unit DSC 295 (Academia Survival Skills) course for a Satisfactory grade.
Special Requirements: Generalization, Reproducibility, and Responsibility (GRR)
A candidate for the doctoral degree in data science is expected to demonstrate evidence of generalization skills and reproducibility in research results, as well as the ability to responsibly conduct and use data science in light of potential ethical and societal implications of the research results.
Evidence of generalization skills may be in the form of—but not limited to—the generalization of results arrived at across domains or across applications within a domain, the generalization of applicability of method(s) proposed, or the generalization of thesis conclusions rooted in formal or mathematical proof or quantitative reasoning supported by robust statistical measures. Reproducibility requirements may be satisfied by supplying additional supplementary material consisting of code, data repository along with evidence of independent external use, or adoption.
Evidence of the responsible use of data science includes the ability to collaboratively identify and respond to ethical and societal opportunities and risks and adhering to “best practices” in terms of ethical consequences (for example, obtaining appropriate consent for data collection about humans, documenting design, and modeling choices, etc.).
The GRR requirements will necessarily require a PhD student to be exposed to one or more application domains since understanding data upon which method advances are tried must be understood well by the researchers so that the objects of generalization, reproducibility, and responsible use are indeed supported by the experimental data. Normally this would be through an adviser or co-adviser who works in an application domain area, or through the rotation program. The institute provides software and services to help graduate students discover and meet relevant domain and method experts.
Relation to the Master of Science in Data Science (MS/DS) Degree Program
While the master’s and PhD programs are two independent programs, the PhD program provides students the ability to fulfill all requirements for the MS degree on their way to completion of the PhD program. This enables a doctoral student to apply for and receive a master’s degree in data science before the conferral of the PhD degree.
Student with Disabilities
In order for the program to respond, a student requiring accommodation for disability may make a request for accommodation upon submission of the student’s intent to apply to the graduate program. Declaration of any disability information is not part of the admissions review process and will not be a factor in admissions. Information concerning accommodation requests is available at: https://disabilities.ucsd.edu/ . Distance learning sites must confirm their ability to support students with disabilities.
Master of Science (MS) in Data Science
The goal of the master’s program is to teach students knowledge and skills required to be successful at performing data driven tasks, and lay the foundation for future researchers who can expand the boundaries of knowledge in data science itself. To meet its goals, the master of science in data science (MS/DS) program consists of two components: formal courses, as well as a terminating thesis or a course-directed comprehensive examination.
Admissions requirements for the MS/DS program are:
- Bachelor’s degree in a quantitative field such as engineering, computer science, mathematics, statistics, cognitive science, scientific disciplines, or quantitative social sciences such as economics or computational social science. Other degree options are acceptable with demonstrated course work or experience in programming, calculus, probability, and statistics.
- Undergraduate GPA of at least 3.0 on a 4.0 scale
- College transcripts
- Three letters of recommendation
- Optional GRE requirements as per the latest guidance from the Graduate Division at UC San Diego
Academic Preparation and Course Planning for Students Entering the MS/DS Program
While students with an undergraduate degree from a data science major or data science minor would have taken courses in all five areas mentioned, we expect that students graduating from other quantitative undergraduate programs may be lacking requisite knowledge and skills in some of these areas. To fill this gap, the program offers a set of foundational courses described in the next section.
In case a student has to take all five foundational courses in Group A, the student should be prepared to spend one extra quarter in the degree program. It is possible, however, for the students who are trained in an application area of data science to save some time from elective courses and devise a graduation schedule within six quarters by exercising the thesis option that enables them to apply data science techniques to the applied field of their original expertise, thus reducing the course load in the elective series.
There are introductory, core, and elective and research requirements (Group A, B, and C courses below) for the master’s program. These course requirements are intended to ensure that students are exposed to (1) fundamental concepts and tools (foundation), (2) advanced, up-to-date views in topics central to data science for all students (core requirement), and (3) a deep, current view of their research or application (elective requirement). Courses may not fulfill more than one requirement.
The master of science in the data science (MS/DS) program is structured as a total of twelve (12) four-unit courses grouped into foundational, core, and specialization areas as described below. Successful completion of the program requires completion of a thesis or a course-based comprehensive examination that tests integrative knowledge across multiple courses. Out of the forty-eight units, at least forty units must be using graduate-level courses. In addition, two out of ten graduate courses can be in areas not directly related to data science but a domain specialization such as economics, biology, medicine, etc., upon approval of the student’s faculty adviser.
Group A: Introductory Courses: Maximum of Four Course Credit
These courses seek to provide five critical foundational knowledge and skills areas that each student graduating from the master’s program is expected to receive at a graduate level: programming skills, data organization and methods skills, numerical linear algebra, multivariate calculus, probability, and statistics.
The program is designed so that students lacking in any (and all) of these foundational knowledge and skills can take credit for a maximum of four courses from the following five courses: DSC 200, DSC 202, DSC 210, DSC 211, and DSC 212.
Group B: Core Courses: Three Required Courses, Minimum of Six Courses
These courses build upon foundational courses. All students must take three required core courses: DSC 240, DSC 241 (*), and DSC 260. In addition, students can select at least three out of the following core courses: DSC 203, DSC 204A (*), DSC 204B, DSC 206, DSC 215, DSC 242, DSC 243, DSC 244, DSC 245, DSC 250, DSC 261.
(*) Depending on academic preparation, a master’s student can take an advanced course on applied statistics, such as MATH 282B instead of DSC 241. Similarly, instead of DSC 204A, a student can take a course on algorithms, such as CSE 202, Design and Analysis of Algorithms, or DSC 206, Algorithms for Data Science .
Group C: Elective and Specialization Courses: Remaining Course Credit Requirements
The MS/DS students can take advantage of electives to complete their course of study. These courses can be advanced courses in core data science subjects , special topics (DSC 291) courses, or , subject to approval by the student’s HDSI faculty adviser, they can be data science upper-division courses or graduate (or upper-division undergraduate) courses in other departments. Upon prior approval from a graduate adviser, students can sign up for an available specialization area. A specialization requires a minimum of three courses in one of the following specialization areas:
Specialization: Bioengineering
Specialization: Business
Specialization: Machine Vision and Interaction Design
Specialization: Computational Neuroscience
Specialization: Networks
Availability of all specializations is not guaranteed. Additional specialization areas may be added by student petition. These specializations are internal and do not show in students’ transcripts. However, students are welcome to cite their specializations in their curriculum vitae .
Thesis or Comprehensive Exam Requirements
The MS/DS degree can be pursued under either the thesis option (Plan I) or the comprehensive examination option (Plan II). The comprehensive examination option follows a course-based comprehensive examination plan under the supervision of a comprehensive examination committee. For full-time students, all the requirements can be completed within one to two years. Students must register for a minimum of three quarters for residency requirements. To maintain good academic standing, students must be making timely and satisfactory progress toward completion of degree requirements and must maintain a minimum overall GPA of 3.0 at UC San Diego.
Approved Elective Courses and Research Credits
The number of elective and research units required varies by degree (see below). Electives are chosen from graduate courses in DSC, CSE, cognitive science, ECE, mathematics, or from other departments as approved. Please refer to the HDSI website for a list of approved electives. Courses must be completed for a letter grade, except for research units that are taken on a Satisfactory/Unsatisfactory basis. Seminar and teaching units may not count toward the electives and research requirement, although both are encouraged.
● Plan I: Thesis Option
The student must sign up for a minimum of eight and maximum of twelve units of DSC 299 (Independent Research) as a part of Group C courses. All courses must be completed for a letter grade, except the DSC 299 units which are taken only on a Satisfactory/Unsatisfactory basis. The student will perform thesis research under the guidance of a thesis adviser and a thesis committee consisting of at least three members. It is required that at least two members of the committee are members of the HDSI faculty council and one of the three committee members can be an industry fellow with an adjunct appointment or a faculty member drawn from another department or division. The chair of the committee shall be approved by the MS program committee. Alternatively, an HDSI industry fellow may be requested to serve as the fourth member of the committee. The committee must be approved by the Graduate Division by the end of the third quarter in the MS program. Students opting for Plan I are required to file an approved thesis to satisfy requirements for completion of the program.
● Plan II: Course-Based Comprehensive Examination Option
Under this plan, the student must complete a practical course-based comprehensive examination designed to evaluate the student’s ability to integrate knowledge and understanding. In this format of the comprehensive examination, the students must answer comprehensive questions in their chosen domain in each of the three selected courses . The comprehensive examination is integrated into the host courses, and in most cases, the associated work serves dual purposes, contributing independently to the student’s course grade and comprehensive examination score.
The comprehensive examination typically consists of a specific class assignment or examination, or a portion thereof, that has been explicitly approved by the MS program committee. Determination of the outcome on the comprehensive exam is separate from the grade in the host course. The students are required to successfully pass the comprehensive examination in three courses drawn from each of the three areas: computing , math/statistics , systems .
Students are permitted up to five attempts, that is, five different courses. No more than three course-hosted comprehensive examinations can be taken in a single quarter, and no comprehensive examination can be repeated in a single quarter. The courses marked for comprehensive examination can be taken only for a letter grade. Course-hosted examinations are registered at the beginning of each quarter and students must register in advance by the specified deadline for the examination. The examination is supervised by a faculty committee responsible for the content, evaluation, and administration of the examination which is separate from the course instructor who is responsible for the course grade but not success in the comprehensive examination.
For more and the latest information regarding the comprehensive examination, please check the HDSI website under graduate programs .
In order for the program to respond, a student requiring accommodation for a disability must make a request for accommodation upon submission of the student’s intent to apply to the graduate program.
Information concerning accommodation requests is available at: http://disabilities.ucsd.edu/ . Distance learning sites must confirm their ability to support students with disabilities.
Master of Data Science Online (MDS)
The Halıcıoğlu Data Science Institute (HDSI), in cooperation with the Department of Computer Science and Engineering (CSE), offers a master’s degree in data science to working professionals who are seeking to expand their skill set in data science. MDS is a formally recognized degree by the University of California that is delivered in a fully online learning format.
The MDS program combines concepts from statistics, computer science, and applications where data is at the forefront. The goal of the MDS program is to teach students the skills required to be successful at performing data-driven tasks. This includes the ability to: (1) collect raw data from various sources and convert this raw data into a curated form amenable to algorithmic analysis, (2) understand machine learning algorithms and how to run them on large data sets, (3) interpret the results of these algorithms, iteratively drill down into the data, and perform more analysis to answer questions about the data.
Admissions prerequisites are as follows:
- Bachelor’s degree with an undergraduate GPA of at least 3.0 on a 4.0 scale; preferably in a field of study that provides a strong mathematical background, such as: computer science, mathematics, engineering, quantitative social sciences, computational life sciences, and computational health sciences.
- Students whose undergraduate degree is in a nontechnical or nonquantitative field must still satisfy minimum program course prerequisites of an introductory programming course, calculus, and linear algebra.
- Two (2) years prior work experience or current employment in a data science related role is preferred.
Application requirements:
- University transcript.
- Statement of Purpose.
- Three (3) letters of recommendation, one (1) of which is recommended to be from the applicant’s current employer.
- English proficiency exam such as TOEFL or IELTS (international applicants only). Note: Students in the fully online program are not eligible for F-1 visa status for study in the United States.
Course requirements are broken down into three categories: foundation, core, and elective. The program also includes a capstone requirement. The course requirements are intended to ensure that students are exposed to (1) fundamental concepts and tools (foundation), (2) advanced, up-to-date views in topics central to data science (core), and (3) a deep, current view of areas for the application of data science tools and techniques (elective). Courses may not fulfill more than one requirement.
The master of data science program requires forty units of study structured as a ten four-unit courses inclusive of the final capstone project course.
Candidates for the m aster of d ata s cience (online) degree must maintain a 3.0 grade point average in all course work undertaken as a graduate student at the University of California.
Foundations (take all three courses, twelve units total)
The foundation courses provide critical foundational knowledge and skills needed in the remainder of the program.
- DSC 207R. Python for Data Science
- DSC 215R. Foundations of Probability and Statistics in Data Science
- DSC 255R. Machine Learning Fundamentals
Core (take all three courses, twelve units total)
The core courses build upon foundational courses and cover the central topics of the program.
- DSC 208R. Data Management for Analytics
- DSC 232R. Big Data Analytics Using Spark
- DSC 256R. Data Mining on the Web
Electives (choose any three courses, twelve units total)
Students will be able to customize their experience in the program by taking three elective courses.
- DSC 209R. Data Visualization
- DSC 257R. Unsupervised Learning
- DSC 258R. Natural Language Processing
- DSC 259R. Practice and Applications
- DSC 266R. Human-Centered AI
- DSC 267R. Data Fairness and Ethics
Capstone (one course, four units)
DSC 288R. Graduate Capstone in Data Science . This course consists of a quarter-long project which requires application of the data science knowledge and skills acquired through the MDS curriculum. Students will pick one project out of several available options, each project from a different application domain. Projects are individually completed and graded based on a ten-step process translated into executable notebook-based reports throughout.
As a fully online program, collaboration with the instructional designers in the Teaching and Learning Commons ensures that online courses in the MDS program meet the electronic accessibility standards established by the UC Office of the President. Such considerations include:
- All videos will have captions.
- All videos will be accessible for screen readers for students who are visually impaired.
- For students who need additional accommodation, voice navigation and voice dictation will be available upon request.
- Care has been taken to avoid using colors to signify or promote particular actions, in order to accommodate students with color blindness.
- All online materials will have the ability to have the font sizes increased.
- Course text (pdfs, other documents) will also be accessible.
In order for the program to respond, a student requiring accommodation for disability must make a request for accommodation upon submission of the student’s intent to apply to the graduate program.
Information concerning accommodation requests is available at: http://disabilities.ucsd.edu/ .
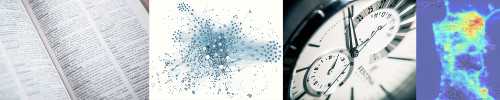
BSc/MSc Thesis
Our research group offers various interesting topics for a BSc or MSc thesis, the latter both in Computer Science and Scientific Computing . These topics are typically closely related to ongoing research projects (see our Research Page and Publications ). Below, we outline the basic procedure you should follow when planning to do a thesis in our group. Please read the following carefully! You also might want to take a quick look at past topics students covered in their theses. Please also note that we currently cannot accommodate all requests for advising a thesis as in current semester as well as in the upcoming summer semester 2024 we are already advising numerous MSc and BSc theses.
Requirements
A key requirement is that you have taken some advanced courses offered by our group. This includes Data Science for Text Analytics or Complex Network Analysis (ICNA) and the more recent master level class on Natural Language Processing with Transformers (INLPT). Student should also have some background in machine learning, ideally in combination with NLP. We also strongly recommend that prior to starting a thesis (especially a BSc thesis) in our group, you do an advanced software practical to become familiar with the data and tools we use in many of our projects. Most students typically do this in the semester before they officially start their thesis. Further requirements include
- very good programming experience with Python (strongly preferred, including framework like pandas and numpy)
- solid background in statistics and linear algebra
- (optionally) experience with the machine learning frameworks such as PyTorch
- (optionally) experience with NLP frameworks such as spaCy, gensim, LangChain
- (optionally) experience with Opensearch or Elasticsearch
- knowledge using tools such as Github and Docker
It is also advantageous if you have taken some graduate courses in the areas of efficient algorithms (e.g., IEA1 ) and in particular machine learning (e.g., IML , IFML or IAI ). Being familiar with frameworks like scikit-learn , Keras or PyTorch is advantageous.
If you have only taken the undergraduate course introduction to databases (IDB) and none of the other above courses, it is unlikely that we can accommodate your request.
Make also sure that you are familiar with the examination regulations ("Prüfungsordnung") that apply to your program of study.
Getting in Contact
Prior to getting in contact with us you should, of course, read this page in its entirety. If you think your interests and expertise are a good fit for our group and research activities, send an email to Prof. Michael Gertz with the subject "Anfrage BSc Arbeit" or "Anfrage MSc Arbeit" and include the following information:
- your current transcript (as PDF). You can download this from the LSF .
- information about your field of application ("Anwendungsfach"), in particular the courses you have taken
- your programming experience and projects you worked on
- areas of interest based on the research conducted in our group
- any other information you think might strengthen your request
We will then review this information and get back to you with the scheduling of an appointment in person to discuss further details.
Thesis Expose
Once we agree on a topic for your thesis, before you officially register for a thesis, we would like to get an idea of how you approach scientific research and whether you are able to do scientific writing. For this, we require that you write an expose of your planned thesis research (see, e.g., here or here ) . This document is about 4-6 pages and has to include a description of
- the context of your project and research
- problem statement(s)
- objectives and planned approaches
- related work
- milestones towards a timely completion of the thesis
Especially for the related work, it is important that you get a good overview early on in your thesis project; of course, your advisor will give you some starting points. Most of the time, such an expose becomes an integral part of the introductory chapter of your thesis, so there is no time and effort wasted. The expose needs to be submitted to your advisor on schedule (which you arrange with your advisor), who will then discuss the expose with you and coordinate the next steps. Occasionally we also have students give a 10-15 minute presentation of their research plan in front of the members of our group in order to get further ideas, comments, suggestions, and pointers on their thesis.
Official Registration
In agreement with your advisor, after you have submitted an expose of good quality, you plan for an official start date of the thesis. For this, please fill out the form suitable for your program of study:
- Für Anmeldung einer Bachelorarbeit, siehe hier .
- For officially registering your master's thesis, see here .
- Registration form for a MSc thesis in Scientific Computing (please see Mrs. Kiesel to obtain a form).
Hand in this form to Prof. Michael Gertz who will then turn in the signed form.
Thesis Research and Advising
- Here are some hints on grammar and style we maintain locally.
- Some easy, purely syntactic hints on writing good research papers (from Prof. Felix Naumann )
- Dos and don'ts, Universität Heidelberg, Prof. Dr. Anette Frank
- Leitfaden zur Abfassung wissenschaftlicher Arbeiten, Ruhr-Universität Bochum, Katarina Klein
- Leitfaden zur Abfassung wissenschaftlicher Arbeiten, TU Dresden, Maria Lieber
In addition, you can find a detailed description how to write a seminar paper using our template for seminar papers. The hints in this template might also be crucial when you are writing a thesis: [ seminar template .zip ] [ report sample pdf ] [ slides english pdf ] [ slides german pdf ]
Feel also free to ask us for copies of BSc/MSc thesis students did in the past in our group.
Thesis Template
- Thesis template [.zip] ; see a sample PDF here .
Thesis Presentation
- English LaTeX-Beamer template for the presentation: template [.zip] , sample PDF
- German LaTeX-Beamer template for the presentation: template [.zip] , sample PDF

Research Topics & Ideas: Data Science
50 Topic Ideas To Kickstart Your Research Project
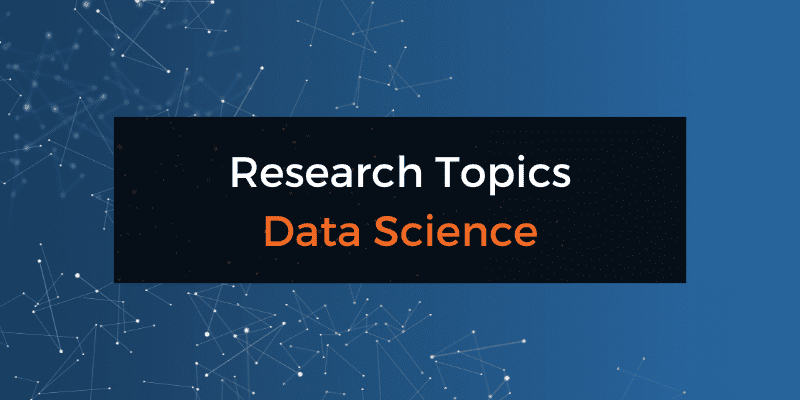
If you’re just starting out exploring data science-related topics for your dissertation, thesis or research project, you’ve come to the right place. In this post, we’ll help kickstart your research by providing a hearty list of data science and analytics-related research ideas , including examples from recent studies.
PS – This is just the start…
We know it’s exciting to run through a list of research topics, but please keep in mind that this list is just a starting point . These topic ideas provided here are intentionally broad and generic , so keep in mind that you will need to develop them further. Nevertheless, they should inspire some ideas for your project.
To develop a suitable research topic, you’ll need to identify a clear and convincing research gap , and a viable plan to fill that gap. If this sounds foreign to you, check out our free research topic webinar that explores how to find and refine a high-quality research topic, from scratch. Alternatively, consider our 1-on-1 coaching service .
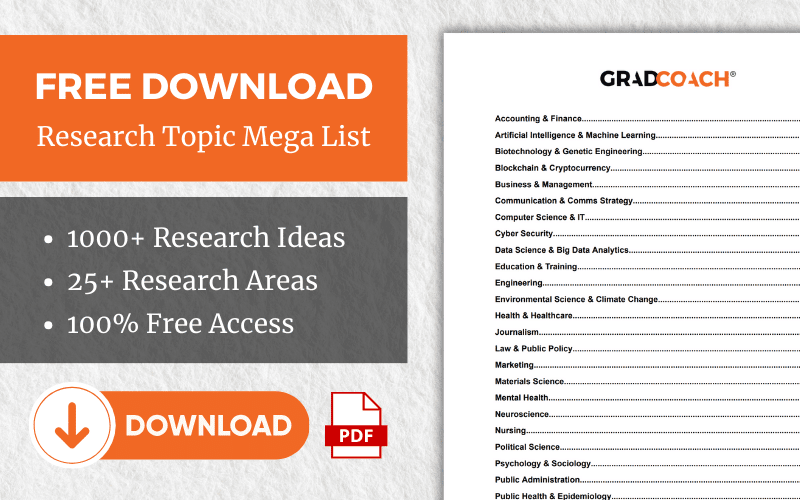
Data Science-Related Research Topics
- Developing machine learning models for real-time fraud detection in online transactions.
- The use of big data analytics in predicting and managing urban traffic flow.
- Investigating the effectiveness of data mining techniques in identifying early signs of mental health issues from social media usage.
- The application of predictive analytics in personalizing cancer treatment plans.
- Analyzing consumer behavior through big data to enhance retail marketing strategies.
- The role of data science in optimizing renewable energy generation from wind farms.
- Developing natural language processing algorithms for real-time news aggregation and summarization.
- The application of big data in monitoring and predicting epidemic outbreaks.
- Investigating the use of machine learning in automating credit scoring for microfinance.
- The role of data analytics in improving patient care in telemedicine.
- Developing AI-driven models for predictive maintenance in the manufacturing industry.
- The use of big data analytics in enhancing cybersecurity threat intelligence.
- Investigating the impact of sentiment analysis on brand reputation management.
- The application of data science in optimizing logistics and supply chain operations.
- Developing deep learning techniques for image recognition in medical diagnostics.
- The role of big data in analyzing climate change impacts on agricultural productivity.
- Investigating the use of data analytics in optimizing energy consumption in smart buildings.
- The application of machine learning in detecting plagiarism in academic works.
- Analyzing social media data for trends in political opinion and electoral predictions.
- The role of big data in enhancing sports performance analytics.
- Developing data-driven strategies for effective water resource management.
- The use of big data in improving customer experience in the banking sector.
- Investigating the application of data science in fraud detection in insurance claims.
- The role of predictive analytics in financial market risk assessment.
- Developing AI models for early detection of network vulnerabilities.
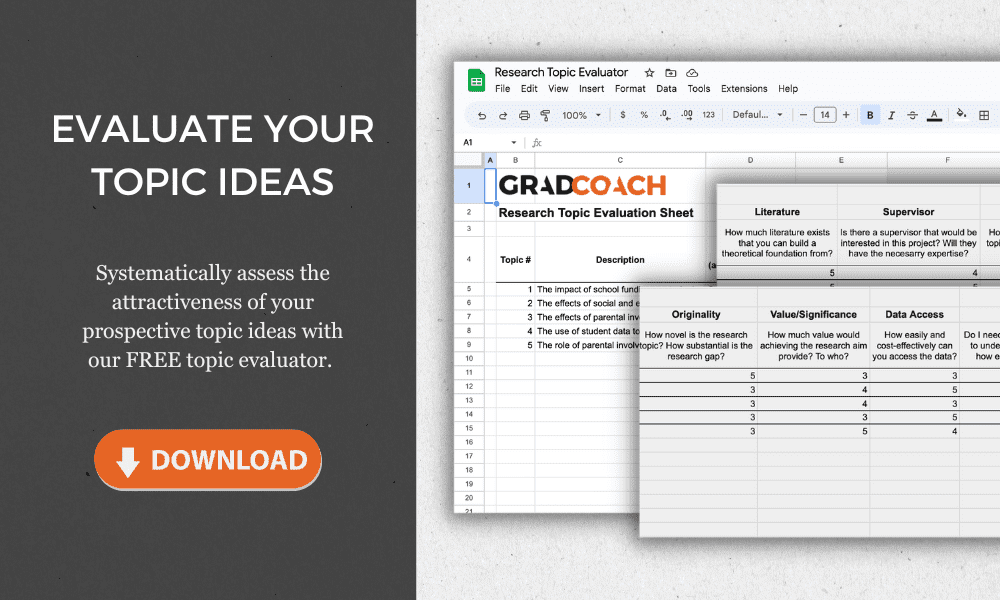
Data Science Research Ideas (Continued)
- The application of big data in public transportation systems for route optimization.
- Investigating the impact of big data analytics on e-commerce recommendation systems.
- The use of data mining techniques in understanding consumer preferences in the entertainment industry.
- Developing predictive models for real estate pricing and market trends.
- The role of big data in tracking and managing environmental pollution.
- Investigating the use of data analytics in improving airline operational efficiency.
- The application of machine learning in optimizing pharmaceutical drug discovery.
- Analyzing online customer reviews to inform product development in the tech industry.
- The role of data science in crime prediction and prevention strategies.
- Developing models for analyzing financial time series data for investment strategies.
- The use of big data in assessing the impact of educational policies on student performance.
- Investigating the effectiveness of data visualization techniques in business reporting.
- The application of data analytics in human resource management and talent acquisition.
- Developing algorithms for anomaly detection in network traffic data.
- The role of machine learning in enhancing personalized online learning experiences.
- Investigating the use of big data in urban planning and smart city development.
- The application of predictive analytics in weather forecasting and disaster management.
- Analyzing consumer data to drive innovations in the automotive industry.
- The role of data science in optimizing content delivery networks for streaming services.
- Developing machine learning models for automated text classification in legal documents.
- The use of big data in tracking global supply chain disruptions.
- Investigating the application of data analytics in personalized nutrition and fitness.
- The role of big data in enhancing the accuracy of geological surveying for natural resource exploration.
- Developing predictive models for customer churn in the telecommunications industry.
- The application of data science in optimizing advertisement placement and reach.
Recent Data Science-Related Studies
While the ideas we’ve presented above are a decent starting point for finding a research topic, they are fairly generic and non-specific. So, it helps to look at actual studies in the data science and analytics space to see how this all comes together in practice.
Below, we’ve included a selection of recent studies to help refine your thinking. These are actual studies, so they can provide some useful insight as to what a research topic looks like in practice.
- Data Science in Healthcare: COVID-19 and Beyond (Hulsen, 2022)
- Auto-ML Web-application for Automated Machine Learning Algorithm Training and evaluation (Mukherjee & Rao, 2022)
- Survey on Statistics and ML in Data Science and Effect in Businesses (Reddy et al., 2022)
- Visualization in Data Science VDS @ KDD 2022 (Plant et al., 2022)
- An Essay on How Data Science Can Strengthen Business (Santos, 2023)
- A Deep study of Data science related problems, application and machine learning algorithms utilized in Data science (Ranjani et al., 2022)
- You Teach WHAT in Your Data Science Course?!? (Posner & Kerby-Helm, 2022)
- Statistical Analysis for the Traffic Police Activity: Nashville, Tennessee, USA (Tufail & Gul, 2022)
- Data Management and Visual Information Processing in Financial Organization using Machine Learning (Balamurugan et al., 2022)
- A Proposal of an Interactive Web Application Tool QuickViz: To Automate Exploratory Data Analysis (Pitroda, 2022)
- Applications of Data Science in Respective Engineering Domains (Rasool & Chaudhary, 2022)
- Jupyter Notebooks for Introducing Data Science to Novice Users (Fruchart et al., 2022)
- Towards a Systematic Review of Data Science Programs: Themes, Courses, and Ethics (Nellore & Zimmer, 2022)
- Application of data science and bioinformatics in healthcare technologies (Veeranki & Varshney, 2022)
- TAPS Responsibility Matrix: A tool for responsible data science by design (Urovi et al., 2023)
- Data Detectives: A Data Science Program for Middle Grade Learners (Thompson & Irgens, 2022)
- MACHINE LEARNING FOR NON-MAJORS: A WHITE BOX APPROACH (Mike & Hazzan, 2022)
- COMPONENTS OF DATA SCIENCE AND ITS APPLICATIONS (Paul et al., 2022)
- Analysis on the Application of Data Science in Business Analytics (Wang, 2022)
As you can see, these research topics are a lot more focused than the generic topic ideas we presented earlier. So, for you to develop a high-quality research topic, you’ll need to get specific and laser-focused on a specific context with specific variables of interest. In the video below, we explore some other important things you’ll need to consider when crafting your research topic.
Get 1-On-1 Help
If you’re still unsure about how to find a quality research topic, check out our Research Topic Kickstarter service, which is the perfect starting point for developing a unique, well-justified research topic.
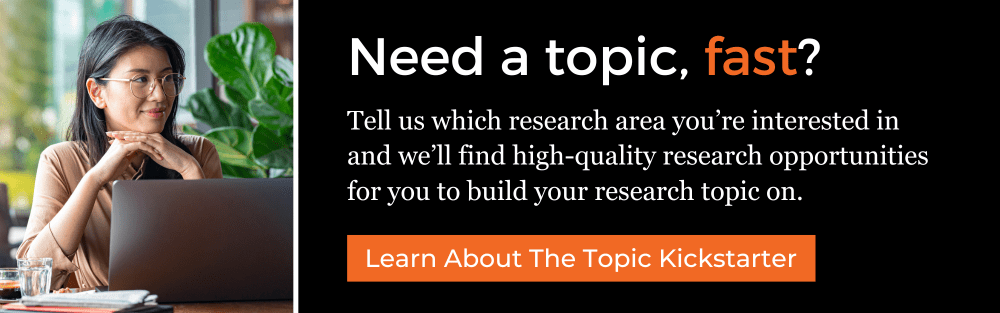
You Might Also Like:
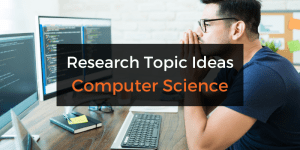
Submit a Comment Cancel reply
Your email address will not be published. Required fields are marked *
Save my name, email, and website in this browser for the next time I comment.
- Print Friendly
- Departments and Units
- Majors and Minors
- LSA Course Guide
- LSA Gateway
Search: {{$root.lsaSearchQuery.q}}, Page {{$root.page}}
{{item.snippet}} |
- News and Events
- Computing Resources
- Diversity, Equity, and Inclusion
- Provide Climate Feedback
- Undergraduate Students
- Ph.D. Students
- Master's Students
- Alumni and Friends

- Undergraduate FAQs
- Statistics Grad Student Tutors
- Transfer Credit
- Undergraduate Programs
- Michigan Undergraduate Students of Statistics (MUgSS)
- Undergraduate Courses
- Undergraduate Research
- Statistics Ph.D. Student Council
- FAQs for Current Students
- Ph.D. Program
- Graduate Courses
- Prospective Ph.D. Students
- Graduate Resources
- Master's Degree Programs
- Prospective Master's Students - Admissions
- Frequently Asked Questions (FAQs)
- Alumni Spotlight
- Statistics Alumni
- Giving Opportunities
- Statistics PhD Alumni
- Statistics Career Placements
Undergraduate research in statistics provides opportunities for gaining experience in data analysis, reading and writing about statistics, and collaboration with Statistics faculty mentors and their research teams. By doing an undergraduate research project, you will develop a deeper understanding of statistics, whether as a first/second year student considering a statistics major, or as a junior/senior considering graduate school and other career options. It is recommended that students considering graduate school participate in research during the course of their academic studies.
The two largest programs for Undergraduate research in data science and statistics are the Undergraduate Research Program in Statistics and the honors thesis. There are also other faculty research projects that include undergraduates that are not in either of these programs. Other statistics research-related activities involving undergraduates include the following:
- The Undergraduate Research Opportunity Program (UROP) provides research opportunities for first and second year students.
- The annual Michigan Student Symposium for Interdisciplinary Statistical Sciences (MSSISS) . MSSISS provides a forum for presenting completed research projects, and an opportunity to see the range and scope of statistical activity across the University of Michigan. Most of the research projects are carried out by graduate students, but undergraduates are welcome to participate and many have!
- The Statistics department occasionally runs a data mining competition.
- A relevant national forum is the free Electronic Undergraduate Statistics Research Conference , and the associated Undergraduate Statistics Project Competition .
- The Center for Statistics, Computing, and Analytics Research (CSCAR) sometimes employs undergraduates. Email [email protected] if you are interested in learning more about opportunities for involvement with CSCAR.
Undergraduate Research Program in Statistics (URPS)
URPS is a competitive program where Statistics faculty offer undergraduate reserach projects in the winter semester.
2025 Projects will be posted, including meeting information and an application link, in November 2024.
Past Projects
Writing an Honors Thesis
An honors thesis provides an opportunity for eligible students to carry out faculty-supervised research in their senior year. The application process and requirements for the Statistics , Data Science , and Informatics honors programs are described on the department website. Students are encouraged to contribute their thesis to the archive of honors theses at the University of Michigan Library.
Past Honors Theses
- Qi Chen, Statistics - Conditional Clustering Method on KNN for Big Data
- Yize Hao, Data Science - PAL versus SMC: Two Approaches in Compartmental Modeling
- Zhongming Jiang, Statistics - Large N, Small T, Multiple P. A Causal Matrix Completion Method for CRM Panel Data
- Xinpei Shen, Data Science - A Dimension Reduction Approach to Multivariate Mediation Analysis
- Weizhe Sun, Statistics - Model Based Inference of Stochastic Volatility via Iterated Filtering
- Jiayi Xu, Data Science - Investigating Measles Dynamics in the Pre-Vaccination Era: A POMP Model Approach
- Ziyu Zhou, Statistics - Kernel Dimension Reduction with Missing Data
- Zuyuan Han, Data Science - Signature Methods in Variance Swap Pricing
- Yiwen (Oliver) Wu, Data Science - Assessment of Privacy in Synthetic Data
- Chen Shang, Statistics and Mathematics - Mat ́ern Models for Graphs: Definition and Inference
- Mingxuan Ge, Statistics and Mathematics - Redistribution of Equity Returns After The Minimum Wage Policy
- Will Schmutz, Data Science - Statistically Ranking Teams in the English Premier League
- Xinyi Xie, Statistics and Mathematics - Logistic Regression With Log-Contrast Transformation
- Yiling Huang, Statistics and Mathematics - Balance Assessment of Matched Data with Multiple Treatment Levels
- Chenxi Fan, Statistics - An evaluation of information criteria for model selection in quasi-likelihood regression, with application to modeling COVID mortality and case incidence in the United States
- Siqi Li, Statistics - Local False Discovery Rates in the Multi-Parameter Case, with Application to Epigenetics of Human Growth
- Wanqi Liang, Data Science - An Applet and Tutorial for Calculating the Sample Size (and Power) for a Clustered Sequential, Multiple Assignment Randomized Trial
- Juejue Wang, Statistics - Comparison of Document Co-clustering aslgorithms and Application of Single-cell RNA-seq Data Clustering to Twitter Data
- Chao Peter Yang, Data Science - The Classical-Romantic Dichotomy: A Machine Learning Approach
- Ziyang Shao, Statistics - College Ranking Based on Pairwise Preferences
- Haoyu Chen, Statistics - Kernel Methods for Activation Energy Prediction
- We Han, Statistics - Argo Data Mean Field Modeling
- Jiahui Ji, Statistics - NYC Optimal Transport and Ridesharing
- Xiaotong Yang, Statistics - Fitting mechanistic models to Daphnia panel data within a panelPOMP framework
- Shuaiji Li, Statistics - Auto Sales Prediction with attention to the Parable of the boiled frog: Functional Data Analysis and Time Series Forecasting
- Zifan Li, Statistics - Perturbation Algorithms for Adversarial Online Learning
- Tianwen Ma, Statistics - A Functional Data Analysis Approach to Looking at Handwriting Data
- Xige Zhang, Statistics - Robustness of the Contextual Bandit Algorithm to A Physical Activity Motivation Effect
- Rong Zhou, Statistics - The Comparison of ACI and MCB Methods for Choosing a Set that Contains the Optimal Dynamic Treatment Regime
- Xinyan Han, Statistics - An Empirical Comparison of Various Online Binary Classification Algorithms
- Hwanwoo Kim, Statistics - A Sample Size Calculator for SMART Pilot Studies
- Yuchen Lin, Statistics - Auto Car Sales Prediction: A Statistical Study Using Functional Data Analysis and Time Series
- Kelsey Pakkala, Statistics - A Functional Data Analysis Approach to Women’s Health Screening Adherence for Breast Cancer and Cervical Cancer
- Emily Slade, Statistics - Functional Data Analysis in Cephalometric Tracing and Mandibular Examination
- Ben Charoenwong, Statistics - An Exploration of Simple Optimized Technical Trading Strategies
- Matthew Lomont, Statistics - Detecting Active Pathways in Gene Sets
- Xuanzhong Wang, Statistics - An Exploration of Influential Observations in the Panel Study of Income Dynamics - An Exploration of Gender Gap in Labor Market; Money Resource Allocation to Children in PSID
- Christopher Worsham, Statistics - A Stochastic Model of Retinal Development in Zebrafish
Faculty Supervising Undergraduate Research
• Danny Almiral l supervises undergraduate researchers with an interest in applied issues in causal inference, dynamic treatment regimens and sequential multiple assignment randomized trials (SMART). Projects include: o Topics in design and analysis of clinical trials for adaptive treatment plans, by Hwanwoo Kim. Co-advised with Ed Ionides. 2nd prize winner in the national Undergraduate Statistics Project Competition. o Adaptive intervention designs in substance use prevention. o An Investigation of Predictor for Tailoring Ecological momentary Assessment and Contextual Recall. o Introduction to Sequential Multiple Assignment Randomized Trials (SMARTs) with Zero Inflated Count Outcomes for the Development of Dynamic Treatment Regimens (DTRs): with application to substance use research.
If you are interested in working with Dr. Almirall, please visit his web page first to see if he is currently accepting new students: http://www-personal.umich.edu/~dalmiral/ .
• Moulinath Banerjee has supervised undergraduate projects including: o Detecting Active Pathways in Gene Sets.
• Ben Hansen has supervised undergraduate projects including: o Proposals for Generating and Utilizing Well Informed Initialization Values to Improve the Computational Efficiency of Optmatch.
• Al Hero has supervised undergraduate projects including: o Dynamic distributed multidimensional scaling (MDS) for data visualization. o Spatio-temporal network anomaly detection in Abilene data streams. o Canonical correlation analysis for sunspot and coronal mass ejection image representation.
• Tailen Hsing has supervised undergraduate projects including: o Analyzing Argo Data Co-advised with Stilian Stoev o Argo Data Mean Field Modeling Co-advised with Stilian Stoev
• Ed Ionides has supervised undergraduate projects including: o Topics in design and analysis of clinical trials for adaptive treatment plans. Co-advised with Danny Almirall. 2nd prize winner in the national Undergraduate Statistics Project Competition. o Modeling cholera as a stochastic process. o Building POMP objects in R for a dynamic general stochastic equilibrium model.. o Investigating sequential Monte Carlo methods for time series analysis. o Identification of insurance companies at risk of insolvency. Co-advised with Kristen Moore.
• Long Nguyen has supervised undergraduate projects including: o Traffic Flow and Density Analysis of NYC TLC Taxi Data. o NYC Optimal Transport and Ridesharing.
• Kerby Shedden supervises undergraduate research with an emphasis on bioinformatics. Examples include: o Statistical analysis of high frequency motion capture and muscle activity data: applications to assessing development of trunk postural control. o Sparsity in the distribution of correlation coefficients in molecular screening data. Co-advised with Ji Zhu. o Individual-specific and disease-specific factors in acquired copy number variations in cancer. o Detection of DNA lesions in acute myelogenous leukemia. o Two-tiered false discovery rates. o Selective targeting of stem-cell-like cancer cell lines. Co-advised with Gus Rosania.
• Ambuj Tewari has supervised undergraduate research projects and an honors theses. Former projects include: o Development of an Android app for mobile health. o Simulations comparing bandit algorithms. o Development of HeartSteps, an Android app for encouraging physical activity. Co-advised with Predrag Klasnja o Empirical evaluation of online learning algorithms (honors thesis). o Numerical experiments with Lasso in high dimensional VAR models.
• Ji Zhu has supervised undergraduate research projects and honors theses. Projects include: o Forecasting Stock Returns in the Chinese Market with Convolutional Neural Networks. o Medical Image Classification Building Upon Pre-trained Neural Networks: An Application on Diabetic Retinopathy Detection.
Undergraduate Research Opportunity Program (UROP)
UROP is a great way to get an introduction to research during the first two years at University of Michigan. See the UROP website for more information. For the most part, Statistics and Data Science research projects require foundational preparation in statistics, mathematics and computer programming. Sometimes, first year students have sufficient preparation through AP courses and other experiences. Otherwise, it may be appropriate to take introductory statistics, computer programming and calculus courses in the first year to be ready for a second year UROP project.
Other Opportunities for Undergraduate Research
It is possible to conduct undergraduate research that does not fall into either the honors program or UROP. If you find yourself interested in the research agenda of a Statistics faculty member, you can email to enquire about available options. This research can be carried out as part of Stats 489 [Independent Study in Statistics], as a paid position if one is available, or as an informal arrangement for neither course credit nor payment. Arrangements must be made on a case-by-case basis with the potential faculty superviser.

- Information For
- Prospective Students
- Current Students
- Faculty and Staff
- More about LSA
- How Do I Apply?
- LSA Magazine
- Student Resources
- Academic Advising
- Global Studies
- LSA Opportunity Hub
- Social Media
- Update Contact Info
- Privacy Statement
- Report Feedback
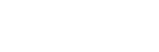
Statistical Science
- Undergraduate theses
- Finding information @ Duke
- Data sets & collections
- Data & visualization services This link opens in a new window
- Statistics consulting This link opens in a new window
- Citing sources
- Excel This link opens in a new window
- Bayesian statistics
- Actuarial science
- Sports analytics
Librarian for the Nicholas School of the Environment

Ask a Librarian
Submit thesis to dukespace.
If you are an undergraduate honors student interested in submitting your thesis to DukeSpace , Duke University's online repository for publications and other archival materials in digital format, please contact Joan Durso to get this process started.
DukeSpace Electronic Theses and Dissertations (ETD) Submission Tutorial
- DukeSpace Electronic Theses and Dissertation Self-Submission Guide
Need help submitting your thesis? Contact [email protected] .
- << Previous: Sports analytics
- Last Updated: May 22, 2024 11:27 AM
- URL: https://guides.library.duke.edu/stats

Services for...
- Faculty & Instructors
- Graduate Students
- Undergraduate Students
- International Students
- Patrons with Disabilities

- Harmful Language Statement
- Re-use & Attribution / Privacy
- Support the Libraries

- Latest News
.png)
- Cryptocurrencies
- White Papers
10 Best Research and Thesis Topic Ideas for Data Science in 2022
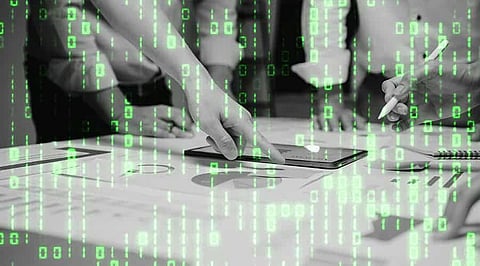
These research and thesis topics for data science will ensure more knowledge and skills for both students and scholars
As businesses seek to employ data to boost digital and industrial transformation, companies across the globe are looking for skilled and talented data professionals who can leverage the meaningful insights extracted from the data to enhance business productivity and help reach company objectives successfully. Recently, data science has turned into a lucrative career option. Nowadays, universities and institutes are offering various data science and big data courses to prepare students to achieve success in the tech industry. The best course of action to amplify the robustness of a resume is to participate or take up different data science projects. In this article, we have listed 10 such research and thesis topic ideas to take up as data science projects in 2022.
- Handling practical video analytics in a distributed cloud: With increased dependency on the internet, sharing videos has become a mode of data and information exchange. The role of the implementation of the Internet of Things (IoT), telecom infrastructure, and operators is huge in generating insights from video analytics. In this perspective, several questions need to be answered, like the efficiency of the existing analytics systems, the changes about to take place if real-time analytics are integrated, and others.
- Smart healthcare systems using big data analytics: Big data analytics plays a significant role in making healthcare more efficient, accessible, and cost-effective. Big data analytics enhances the operational efficiency of smart healthcare providers by providing real-time analytics. It enhances the capabilities of the intelligent systems by using short-span data-driven insights, but there are still distinct challenges that are yet to be addressed in this field.
- Identifying fake news using real-time analytics: The circulation of fake news has become a pressing issue in the modern era. The data gathered from social media networks might seem legit, but sometimes they are not. The sources that provide the data are unauthenticated most of the time, which makes it a crucial issue to be addressed.
- TOP 10 DATA SCIENCE JOB SKILLS THAT WILL BE ON HIGH DEMAND IN 2022
- TOP 10 DATA SCIENCE UNDERGRADUATE COURSES IN INDIA FOR 2022
- TOP DATA SCIENCE PROJECTS TO DO DURING YOUR OMICRON QUARANTINE
- Secure federated learning with real-world applications : Federated learning is a technique that trains an algorithm across multiple decentralized edge devices and servers. This technique can be adopted to build models locally, but if this technique can be deployed at scale or not, across multiple platforms with high-level security is still obscure.
- Big data analytics and its impact on marketing strategy : The advent of data science and big data analytics has entirely redefined the marketing industry. It has helped enterprises by offering valuable insights into their existing and future customers. But several issues like the existence of surplus data, integrating complex data into customers' journeys, and complete data privacy are some of the branches that are still untrodden and need immediate attention.
- Impact of big data on business decision-making: Present studies signify that big data has transformed the way managers and business leaders make critical decisions concerning the growth and development of the business. It allows them to access objective data and analyse the market environments, enabling companies to adapt rapidly and make decisions faster. Working on this topic will help students understand the present market and business conditions and help them analyse new solutions.
- Implementing big data to understand consumer behaviour : In understanding consumer behaviour, big data is used to analyse the data points depicting a consumer's journey after buying a product. Data gives a clearer picture in understanding specific scenarios. This topic will help understand the problems that businesses face in utilizing the insights and develop new strategies in the future to generate more ROI.
- Applications of big data to predict future demand and forecasting : Predictive analytics in data science has emerged as an integral part of decision-making and demand forecasting. Working on this topic will enable the students to determine the significance of the high-quality historical data analysis and the factors that drive higher demand in consumers.
- The importance of data exploration over data analysis : Exploration enables a deeper understanding of the dataset, making it easier to navigate and use the data later. Intelligent analysts must understand and explore the differences between data exploration and analysis and use them according to specific needs to fulfill organizational requirements.
- Data science and software engineering : Software engineering and development are a major part of data science. Skilled data professionals should learn and explore the possibilities of the various technical and software skills for performing critical AI and big data tasks.
Disclaimer: Analytics Insight does not provide financial advice or guidance. Also note that the cryptocurrencies mentioned/listed on the website could potentially be scams, i.e. designed to induce you to invest financial resources that may be lost forever and not be recoverable once investments are made. You are responsible for conducting your own research (DYOR) before making any investments. Read more here.
Related Stories


Academic Catalog 2023-2024
- Data Science
The Bachelor of Science in Data Science studies the collection, manipulation, storage, retrieval, and computational analysis of data in its various forms, including numeric, textual, image, and video data from small to large volumes. The program combines computer science, information science, mathematics, statistics, and probability theory into an integrated curriculum that is designed to prepare students for careers or graduate studies in Big Data analysis, data science, and data analytics. The coursework covers exploratory data analysis, data manipulation in a variety of programming languages, large-scale data storage, predictive analytics, machine learning, data mining, and information visualization and presentation. Data science has emerged as a discipline due to the confluence of two major events:
- The ability to collect, store, prune, process, and transmit large amounts of data in the cloud
- The convergence of programming, statistics, artificial intelligence, and visualization as complementary tools for the analysis and understanding of data
Bachelor of Science (BS)
DS 1990. Elective. (1-4 Hours)
Offers elective credit for courses taken at other academic institutions. May be repeated without limit.
DS 2000. Programming with Data. (2 Hours)
Introduces programming for data and information science through case studies in business, sports, education, social science, economics, and the natural world. Presents key concepts in programming, data structures, and data analysis through Python and Excel. Integrates the use of data analytics libraries and tools. Surveys techniques for acquiring and programmatically integrating data from different sources. Explains the data analytics pipeline and how to apply programming at each stage. Discusses the programmatic retrieval of data from application programming interfaces (APIs) and from databases. Introduces predictive analytics for forecasting and classification. Demonstrates the limitations of statistical techniques.
Corequisite(s): DS 2001
Attribute(s): NUpath Analyzing/Using Data
DS 2001. Data Science Programming Practicum. (2 Hours)
Applies data science principles in interdisciplinary contexts, with each section focusing on applications to a different discipline. Involves new experiments and readings in multiple disciplines (both computer science and the discipline focus of the particular section). Requires multiple projects combining interdisciplinary subjects.
Corequisite(s): DS 2000
DS 2500. Intermediate Programming with Data. (4 Hours)
Offers intermediate to advanced Python programming for data science. Covers object-oriented design patterns using Python, including encapsulation, composition, and inheritance. Advanced programming skills cover software architecture, recursion, profiling, unit testing and debugging, lineage and data provenance, using advanced integrated development environments, and software control systems. Uses case studies to survey key concepts in data science with an emphasis on machine-learning (classification, clustering, deep learning); data visualization; and natural language processing. Additional assigned readings survey topics in ethics, model bias, and data privacy pertinent to today's big data world. Offers students an opportunity to prepare for more advanced courses in data science and to enable practical contributions to software development and data science projects in a commercial setting.
Prerequisite(s): DS 2000 with a minimum grade of D-
Corequisite(s): DS 2501
DS 2501. Lab for DS 2500. (1 Hour)
Practices the programming techniques discussed in DS 2500 through hands-on experimentation.
Corequisite(s): DS 2500
DS 2990. Elective. (1-4 Hours)
DS 2991. Research in Data Science. (1-4 Hours)
Offers an opportunity to conduct introductory-level research or creative endeavors under faculty supervision.
DS 3000. Foundations of Data Science. (4 Hours)
Introduces core modern data science technologies and methods that provide a foundation for subsequent Data Science classes. Covers: working with tensors and applied linear algebra in standard numerical computing libraries (e.g., NumPy); processing and integrating data from a variety of structured and unstructured sources; introductory concepts in probability, statistics, and machine learning; basic data visualization techniques; and now standard data science tools such as Jupyter notebooks.
Prerequisite(s): CS 2510 with a minimum grade of D- or DS 2500 with a minimum grade of D-
Attribute(s): NUpath Analyzing/Using Data, NUpath Natural/Designed World
DS 3500. Advanced Programming with Data. (4 Hours)
Offers a deep dive into the design and implementation of enterprise-grade software systems with an emphasis on software architectures for more complex data-driven applications. Covers extensible architectures that support testing, data provenance, reuse, maintainability, scalability, and robustness and building software APIs and libraries for wide-scale adoption and ease of use. Students design, implement, and test complex loosely coupled service-oriented architectures using distributed processing, stream-based data processing, and interprocess communication via message passing. Explores the features, capabilities, and underlying design of popular data analysis and visualization frameworks.
Prerequisite(s): DS 2500 with a minimum grade of D-
DS 3990. Elective. (1-4 Hours)
DS 4200. Information Presentation and Visualization. (4 Hours)
Introduces foundational principles, methods, and techniques of visualization to enable creation of effective information representations suitable for exploration and discovery. Covers the design and evaluation process of visualization creation, visual representations of data, relevant principles of human vision and perception, and basic interactivity principles. Studies data types and a wide range of visual data encodings and representations. Draws examples from physics, biology, health science, social science, geography, business, and economics. Emphasizes good programming practices for both static and interactive visualizations. Creates visualizations in Excel and Tableau as well as R, Python, and open web-based authoring libraries. Requires programming in Python, JavaScript, HTML, and CSS. Requires extensive writing including documentation, explanations, and discussions of the findings from the data analyses and the visualizations.
Attribute(s): NUpath Analyzing/Using Data, NUpath Writing Intensive
DS 4300. Large-Scale Information Storage and Retrieval. (4 Hours)
Introduces data and information storage approaches for structured and unstructured data. Covers how to build large-scale information storage structures using distributed storage facilities. Explores data quality assurance, storage reliability, and challenges of working with very large data volumes. Studies how to model multidimensional data. Implements distributed databases. Considers multitier storage design, storage area networks, and distributed data stores. Applies algorithms, including graph traversal, hashing, and sorting, to complex data storage systems. Considers complexity theory and hardness of large-scale data storage and retrieval. Requires use of nonrelational, document, key-column, key-value, and graph databases and programming in R, Python, and C++.
Prerequisite(s): CS 3200 with a minimum grade of D- ; (DS 4100 with a minimum grade of D- or DS 3000 with a minimum grade of D- )
DS 4400. Machine Learning and Data Mining 1. (4 Hours)
Introduces supervised and unsupervised predictive modeling, data mining, and machine-learning concepts. Uses tools and libraries to analyze data sets, build predictive models, and evaluate the fit of the models. Covers common learning algorithms, including dimensionality reduction, classification, principal-component analysis, k-NN, k-means clustering, gradient descent, regression, logistic regression, regularization, multiclass data and algorithms, boosting, and decision trees. Studies computational aspects of probability, statistics, and linear algebra that support algorithms, including sampling theory and computational learning. Requires programming in R and Python. Applies concepts to common problem domains, including recommendation systems, fraud detection, or advertising.
Prerequisite(s): ((DS 4100 with a minimum grade of D- or DS 3000 with a minimum grade of D- ); ( CS 2810 with a minimum grade of D- or ECON 2350 with a minimum grade of D- or ENVR 2500 with a minimum grade of D- or MATH 3081 with a minimum grade of D- or MGSC 2301 with a minimum grade of D- or PHTH 2210 with a minimum grade of D- or PSYC 2320 with a minimum grade of D- )) or ( CS 2810 with a minimum grade of D- ; CS 3500 with a minimum grade of D- )
Attribute(s): NUpath Analyzing/Using Data, NUpath Capstone Experience, NUpath Writing Intensive
DS 4420. Machine Learning and Data Mining 2. (4 Hours)
Continues with supervised and unsupervised predictive modeling, data mining, and machine-learning concepts. Covers mathematical and computational aspects of learning algorithms, including kernels, time-series data, collaborative filtering, support vector machines, neural networks, Bayesian learning and Monte Carlo methods, multiple regression, and optimization. Uses mathematical proofs and empirical analysis to assess validity and performance of algorithms. Studies additional computational aspects of probability, statistics, and linear algebra that support algorithms. Requires programming in R and Python. Applies concepts to common problem domains, including spam filtering.
Prerequisite(s): DS 4400 with a minimum grade of D-
DS 4440. Practical Neural Networks. (4 Hours)
Offers a hands-on introduction to modern neural network ("deep learning") methods and tools. Covers fundamentals of neural networks and introduces standard and new architectures from simple feedforward networks to recurrent and “transformer” architectures. Also covers stochastic gradient descent and backpropagation, along with related parameter estimation techniques. Emphasizes using these technologies in practice, via modern toolkits. Reviews applications of these models to various types of data, including images and text.
Prerequisite(s): DS 4400 (may be taken concurrently) with a minimum grade of D-
DS 4970. Junior/Senior Honors Project 1. (4 Hours)
Focuses on in-depth project in which a student conducts research or produces a product related to the student’s major field. Combined with Junior/Senior Project 2 or college-defined equivalent for 8 credit honors in the discipline project.
DS 4971. Junior/Senior Honors Project 2. (4 Hours)
Focuses on second semester of in-depth project in which a student conducts research or produces a product related to the student’s major field.
Prerequisite(s): DS 4970 with a minimum grade of D-
DS 4973. Topics in Data Science. (4 Hours)
Offers a lecture course in data science on a topic not regularly taught in a formal course. Topics may vary from offering to offering. May be repeated up to four times.
Prerequisite(s): CS 3000 with a minimum grade of D- ; ( CS 3500 with a minimum grade of D- or DS 3500 with a minimum grade of D- )
DS 4990. Elective. (1-4 Hours)
DS 4991. Research. (4 Hours)
Offers an opportunity to conduct research under faculty supervision.
Attribute(s): NUpath Integration Experience
DS 4992. Directed Study. (1-4 Hours)
Offers independent work under the direction of members of the department on a chosen topic. May be repeated without limit.
DS 4996. Experiential Education Directed Study. (1-4 Hours)
Draws upon the student’s approved experiential activity and integrates it with study in the academic major. Restricted to those students who are using it to fulfill their experiential education requirement. May be repeated without limit.
DS 4997. Data Science Thesis. (4 Hours)
Offers students an opportunity to prepare an undergraduate thesis under faculty supervision.
DS 4998. Data Science Thesis Continuation. (4 Hours)
Focuses on student continuing to prepare an undergraduate thesis under faculty supervision.
DS 5010. Introduction to Programming for Data Science. (4 Hours)
Offers an introductory course on fundamentals of programming and data structures. Covers lists, arrays, trees, hash tables, etc.; program design, programming practices, testing, debugging, maintainability, data collection techniques, and data cleaning and preprocessing. Includes a class project, where students use the concepts covered to collect data from the web, clean and preprocess the data, and make it ready for analysis.
DS 5020. Introduction to Linear Algebra and Probability for Data Science. (4 Hours)
Offers an introductory course on the basics of statistics, probability, and linear algebra. Covers random variables, frequency distributions, measures of central tendency, measures of dispersion, moments of a distribution, discrete and continuous probability distributions, chain rule, Bayes’ rule, correlation theory, basic sampling, matrix operations, trace of a matrix, norms, linear independence and ranks, inverse of a matrix, orthogonal matrices, range and null-space of a matrix, the determinant of a matrix, positive semidefinite matrices, eigenvalues, and eigenvectors.
DS 5110. Introduction to Data Management and Processing. (4 Hours)
Introduces students to the core tasks in data science, including data collection, storage, tidying, transformation, processing, management, and modeling for the purpose of extracting knowledge from raw observations. Programming is a cross-cutting aspect of the course. Offers students an opportunity to gain experience with data science tasks and tools through short assignments. Includes a term project based on real-world data.
DS 5220. Supervised Machine Learning and Learning Theory. (4 Hours)
Introduces supervised machine learning, which is the study and design of algorithms that enable computers/machines to learn from experience or data, given examples of data with a known outcome of interest. Offers a broad view of models and algorithms for supervised decision making. Discusses the methodological foundations behind the models and the algorithms, as well as issues of practical implementation and use, and techniques for assessing the performance. Includes a term project involving programming and/or work with real-world data sets. Requires proficiency in a programming language such as Python, R, or MATLAB.
Attribute(s): NUpath Capstone Experience, NUpath Writing Intensive
DS 5230. Unsupervised Machine Learning and Data Mining. (4 Hours)
Introduces unsupervised machine learning and data mining, which is the process of discovering and summarizing patterns from large amounts of data, without examples of data with a known outcome of interest. Offers a broad view of models and algorithms for unsupervised data exploration. Discusses the methodological foundations behind the models and the algorithms, as well as issues of practical implementation and use, and techniques for assessing the performance. Includes a term project involving programming and/or work with real-life data sets. Requires proficiency in a programming language such as Python, R, or MATLAB.
DS 5500. Data Science Capstone. (4 Hours)
Offers students a capstone opportunity to practice data science skills learned in previous courses and to build a portfolio. Students practice visualization, data wrangling, and machine learning skills by applying them to semester-long term projects on real-world data. Students may either propose their own projects or choose from a selection of industry options. Emphasizes the overall data science process, including identification of the scientific problem, selection of appropriate machine learning methods, and visualization and communication of results. Lectures may include additional topics, including visualization, communication, and data science ethics.
Prerequisite(s): ( CS 5800 with a minimum grade of C- or EECE 7205 with a minimum grade of C- ); DS 5110 with a minimum grade of C- ; DS 5220 with a minimum grade of C- ; DS 5230 with a minimum grade of C-
Print Options
Send Page to Printer
Print this page.
Download Page (PDF)
The PDF will include all information unique to this page.
2023-24 Undergraduate Day PDF
2023-24 CPS Undergraduate PDF
2023-24 Graduate/Law PDF
2023-24 Course Descriptions PDF
Master's in Data Science
Master’s in data science program overview.
The Data Science master's program, jointly led by the Computer Science and Statistics faculties, trains students in the rapidly growing field of data science.
Data Science lies at the intersection of statistical methodology, computational science, and a wide range of application domains. The program offers strong preparation in statistical modeling, machine learning, optimization, management and analysis of massive data sets, and data acquisition. The program focuses on topics such as reproducible data analysis, collaborative problem solving, visualization and communication, and security and ethical issues that arise in data science.
To earn the Master of Science in Data Science, students must complete 12 courses. This requires students to be on campus for at least 3 semesters (one and a half academic years). Some students will choose to extend their studies for a fourth semester to take additional courses or complete a master’s thesis research project.
SEAS will be hosting virtual information sessions this Fall for students interested in the Data Science program. Registration for these sessions is available on the Admissions Events page for prospective graduate students .
Why pursue a master’s degree in Data Science?
With companies and organizations better able to capture data in a multitude of ways, data-driven decision making is changing the way businesses operate. Powerful analytics tools can model and predict how consumers will behave or markets will respond. Consequently, an understanding of data science is a 21st century job skill that can be beneficial in many different careers.
Data Science Degree Career Paths
Data Science career paths are flexible. There are different pathways to use data science skills.
- Data science professional - data analyst, database developer, or data scientist.
- Analytics-enabled jobs - functional business analyst or data-driven manager.
Data science professionals like data analysts can become qualified for a data science or data system developer role depending on where they deepen their expertise. By expanding knowledge in Artificial Intelligence, statistics, data management, and big data analytics, a data analyst can transition into a data scientist role. By building on existing technical skills in Python, relational databases, and machine learning, a data analyst can become a data system developer.
Requirements
There are no formal prerequisites for applicants to this master’s program. However, successful applicants do need to have sufficient background knowledge of calculus, linear algebra and differential equations; familiarity with probability and statistical inference; fluency in at least one programming language such as python or R, and an understanding of basic computer science concepts. As Data Science is an interdisciplinary field, SEAS welcomes applicants with undergraduate training in a wide range of academic disciplines.
- How to Apply
Learn more about how to apply to the Data Science degree program or apply now .
What should a graduate of the Data Science program be able to do?
The design of the program is based on eleven learning outcomes developed through discussions between the computer science and statistics faculty:
Build statistical models and understand their power and limitations
Design an experiment
Use machine learning and optimization to make decisions
Acquire, clean, and manage data
Visualize data for exploration, analysis, and communication
Collaborate within teams
Deliver reproducible data analysis
Manage and analyze massive data sets
Assemble computational pipelines to support data science from widely available tools
Conduct data science activities aware of and according to policy, privacy, security and ethical considerations
Apply problem-solving strategies to open-ended questions
Financing Your Degree
Students typically finance their master’s degree program with a combination of loans, savings, family support, grants (from governments, foundations and companies), fellowships and scholarships. We recommend you visit the Harvard Kenneth C. Griffin Graduate School of Arts and Sciences (Harvard Griffin GSAS) Funding and Financial Aid website prior to your application to learn more about your options.
Teaching Fellowships
Approximately 15% of our students are paid Teaching Fellows, usually in the second year. TFing in the first semester is highly unusual. Teaching compensation is paid out at Harvard graduate student rates.
Master's in Data Science Leadership
In master's in data science.
- Degree Requirements
- Secondary Field in Data Science
- Alumni News
- Info for Current Students
Featured Stories
Master's student capstone spotlight: AI-Enabled Information Extraction for Investment Management
Extracting complicated data from long documents
Academics , AI / Machine Learning , Applied Computation , Computer Science , Industry
Master's student capstone spotlight: AI-Assisted Frontline Negotiation
Speeding up document analysis ahead of negotiations
Academics , AI / Machine Learning , Applied Computation , Computer Science
Master's student capstone spotlight: A Remote Sensing Framework for Rail Incident Situational Awareness Drones
Using drones to rapidly assess disaster sites
- Zur Metanavigation
- Zur Hauptnavigation
- Zur Subnavigation
- Zum Seitenfuss
Photo: Sarah Buth
Bachelor and Master Thesis
We offer a variety of cutting-edge and exciting research topics for Bachelor's and Master's theses. We cover a wide range of topics from Data Science, Natural Language Processing, Argument Mining, the Use of AI in Business, Ethics in AI and Multimodal AI. We are always open to suggestions for your own topics, so please feel free to contact us. We supervise students from all disciplines of business administration, business informatics, computer science and industrial engineering.
Thesis Topics
Example topics could be:
- Conversational Artificial Intelligence in Insurance and Finance
- Natural Language Processing for Understanding Financial Narratives: An Overview
- Ethics at the Intersection of Finance and AI: A Comprehensive Literature Review
- Explainable Natural Language Processing for Credit Risk Assessment Models: A Literature Review
Thesis Template
- Latex Template for bachelor and master theses
- How to use the latex template
Q1: How many pages do I need to write?
A: In general, the number of pages is only a poor indicator of the quality of a thesis. However, as a rule of thumb, bachelor theses should have around 30 pages, while master theses should be around 60 pages of main content (that is, without the appendix and lists of tables, symbols, figures, references etc.).
Q2: How often should I meet with my supervisor?
A: Your supervisors are typically very busy people. However, don't hesitate to ask in case you have questions. For instance, if you are unsure of some requirements, or in case you have methodological problems, it is absolutely necessary to talk to your supervisor. As a rule of thumb, you should meet at least three times (once in the beginning, once in the middle, and once before the submission).
Q3: Am I allowed to use any AI models in the process of writing my thesis?
A: In general, we neither forbid nor recommend the use of AI for writing support. However, if you use AI, please inform your supervisor. Also, you need to adhere to the recommendations on the use of AI writing assistants given by the faculty.
Q4: How much time do I have?
A: The exact timing is dependent on your study program! Thus, please check the examination requirements before the official start of your thesis -- you are responsible for sticking to the rules.
Chair of Data Science
Thesis guide.
This short guide is intended to give you a brief guideline on how to organise and conduct your thesis. This guide covers both, a master and a bachelor thesis.
Managing your Thesis
A thesis is a complex task, similar to a software project has to be managed properly. It can be seen to have four stages, which are iterated several time.
Define the goal of your thesis together with your supervisor. Write them down as bullet point list. This list should have 3-5. If there are more points, you have to aggregate them and if you can not aggregated them, then you have set yourself too many goals. Note that goals are not tasks. Goals determine what you want to find out with your thesis and form the basis of the research questions. Most likely you will refine your goals throughout the thesis. That is OK because your knowledge on the domain gets better and therewith you are able to write down more accurate questions.
It is often underestimated, but very important to search what others did. Most likely others worked on similar topics and so you have to set out searching for what they did. Use search engines like Goolge Scholar or grab a recent Book on the topic of your thesis and start your literature research from there. A in depth research allows you to avoid the unpleasant suprise that after 6 months of work one identifies the same solution made by somebody others. Moreover, it builds up your background knowledge in the domain. Only with sufficient background knowledge you are able to take the correct decisions.
Good implementation starts with a workplan that has tasks, milestone (i.e. what to achieve when) and thoughts on how to get there. You do not have to create a full fledged Gantt and Pert Chart, a simple task list might be sufficient to structure your work. Use a issue tracking system or something similar, because it also helps you to keep track of things your already tried (and it gives a good feeling when closing open issues).
This is the most important part, which is often overlooked. You have invested a most time in implementing/realising your goals, and so it seems that evaluation is annoying and time consuming. However, it is the evaluation of your system that answers your research questions or let you judge whether you achieved your goals or not. So plan your evaluation before the implementation by writing down a coarse-grained evaluation plan, and, reserve enough time for it.
Note that this is not a linear, but a iterative process. In your evaluation you discover the something does not work out and so you have to adapt your evaluation or even your goals. But that is pretty fine since you learn as you go. If you already would know it from the beginning, there is no need for research.
Templates and Further Ressources
- Structure+Hints for Seminar, Bachelor, Master and PhD Talks
- Latex Template for MA/BA Thesis
- Scientific Writing
- Hints on Scientific Presentations - although focused on Theoretical Computer Science, most parts are also relevant for Computer Science in general (where proofs are not given in a formal way but by implementation and/or empirical analysis)
How to conduct a good scientific thesis
Regardless whether you are a Bachelor, Master or PhD Student (or later on a researcher), there is always the central question on how to conduct a good thesis. Sadly, there are no strict “rules” for doing so and it requires a lot of expertise. Fortunately, some simple tips allow you to bootstrap the quality of writings, presentations and your thesis in general and enable you to develop a critical thinking, but open mind – the most important tool for any future career. In this article i want to give you some tips on how to bootstrapp your scientific skills. So what are scientific skills? Science is about discovering stuff nobody has known before and to explain your discovery to other people. Explaining your discovery is important, since it
- allows others to validate your work
- helps yourself in gaining a deeper understanding
- enable future discovery based on what you have found out.
That is not only true for research, but for nearly any industry jobs for academically educated presons. How to convince your boss that your solution for a particular project is the best one? How to argue that the current roadmap does not make sense? How to convince your team members to invest time in a particular functionality? How to judge the validity of your own decisions? Conducting a good scientific thesis requires you to do a decent job and communicate the results. Hence it involves
- Reading Skills
- Writing Skill
- Presentation Skills
- Discussions
- Critical Thinking and self-reflexion
I will cover all three points below and give tips on how to bootstrap the particular skills. In my seminars and when i have to judge thesis, i apply those principles as criterions for the grade you obtain. So at least my students should take the advices seriously ;) Disclaimer: it is by no means exhaustive and only my personal opinion/experience. The most important thing to do is to find your own way, while giving credit to all the people that walked the way before and trying to learn from their experience. An attitude not only important in science and research.
One of the most important skills is motivation. Once upon a time a student asked the teacher how to learn uninteresting stuff efficiently. The short answer is you can’t, at least not for beyond the exam. So the key insight to motivation is in choosing stuff you really like to do. Often student make the error in going the easy way, taking courses where exams are “easy going”. That is a waste of time since it is by far harder to learn boring stuff, the really interesting stuff. Think of it when you have watched a really entertaining movie. You can remember nearly all details and you had a good feeling after watching it. How do you feel after a bad movie?
So when you are choosing a topic for a scientific thesis, take one that makes you feel every day like you have watched the best movie ever. Most supervisors give you the freedom to adjust a particular topic of a thesis so that it fits the motivation of the student better. So engage a critical discussion with your supervisor and what you want to do and what not. Of course this requires you to go deeper on potential topics and to explore what best fits your interest. But especially when you are studying computer science, you should have a natural habit on being attracted by strange, nerdy stuff. Key lesson: Learn to motivate yourself and take topics that you are excited about it.
- Read with a purpose (start with questions to the article. the more specific, the better)
- Discuss what you read with others
- Write a short summary of your key findings. either do it graphically (Mindmaps, Rhino Maps) or textually.
Write often; write concise. Writing requires you in expressing your (parallel, mostly non-linear) thoughts on a linear medium. And this is very difficult. But the medium forces you to express your thoughts in a clear manner and connecting each thought with each other (the so called flow or read thread in German). That is difficult and learning good writing techniques covers whole courses and books. However, for getting started there are five simple rules:
- Write down the 3-6 most important questions you want to answer. Two sentences per question maximum
- Write down the motivation, why those questions are important
- Give an example for every question
- Write down how you will answer the three questions.
- Write down the answer to the questions
If 2. and 3. do have nothing in common, you have put the wrong questions together in the same box.
Presentations follow the same rules as writing, with the exception that oral presentations does not allow you to present all your details. So avoid explaining every little detail, because you will loose the audience. In general your talk should be structured similar to your written thesis
- Motivation: Why is your talk relevant. Give an example.
- State-of-the-Art: What did others do in the context of your talk?
- Questions: What questions will you answer in this talk?
- Methodology: How will you answer the questions?
- Experiments: How did you implement the methodology?
- Evaluation: What is the answer to every question? What did you learn?
- Future Work: What are the loose ends of your work (a work without loose ends is most often not very helpful)
Keep that simple structure and use the question to guide the audience through your thoughts and findings. Stick to the KISS principle (Keep it short and simple). Your audience will appreciate it.
Beim Anzeigen des Videos wird Ihre IP-Adresse an einen externen Server (Vimeo.com) gesendet.
Center for Statistics and Machine Learning

SML 310 / 312 — Research Projects in Data Science

A project-based seminar course in which students work individually or in small teams to tackle data science and machine learning problems, working with real-world datasets. The course emphasizes critical thinking about experiments and large dataset analysis and the ability to clearly communicate one's research. This course is intended to support students in developing the analytical skills necessary for quantitative independent work. Students are not required to bring in their own project proposal and dataset for this course; however, if they do, students should consult with their home department about how this course could appropriately complement, but not replace, their independent work requirements. (Note: SML 312 is an alternative version of SML 310; the two courses are equivalent.)
Can I use my work in SML 310 as part of my undergraduate thesis? With permission from their thesis advisor and/or their undergraduate Department, students can incorporate work they did in SML 310 into their thesis. Students should indicate in their thesis which parts of the work were completed as part of SML 310.
Can I use my work in SML 310 to fulfill the CSML Certificate’s Independent Work requirement? That is in principle possible if the scope of your SML 310 project is sufficiently large and the write-up is sufficiently comprehensive. However, many SML 310 students would need to expand their SML 310 project in order to fulfill the IW requirement.
Can I use work that I did for an earlier project (e.g. for my JP) as my project in SML 310? You cannot resubmit work that you already have completed elsewhere. You can build on work that you had done previously. Your write-up must clearly indicate what part of the work was done as part of SML 310, and what part of the work was done earlier.
Boston University Academics
Boston University
- Campus Life
- Schools & Colleges
- Degree Programs
- Search Academics
- Data Science
The listing of a course description here does not guarantee a course’s being offered in a particular term. Please refer to the published schedule of classes on the MyBU Student Portal for confirmation a class is actually being taught and for specific course meeting dates and times.
- New Explore BU’s free online courses on edX
View courses in
- All Departments
- Bioinformatics
- CDS DS 592: Special Topics in Mathematical and Computational Sciences Undergraduate Prerequisites: CASMA581 or CDSDS122 AND CDSDS320 Spring 2024 - Randomized Algorithms. A little randomness is a surprisingly powerful ingredient in algorithm design. Sometimes this amounts to producing approximate solutions to a problem where exact global solutions are forbiddingly hard to find, and other times the randomness itself--as a probability distribution--is the goal. This course will present some classic and some cutting-edge randomized algorithms, with an emphasis on discrete and graph-based problems.
- CDS DS 594: Spark! Data Visualization X-Lab Practicum Undergraduate Prerequisites: CDS DS 310 ; CDS DS 122 ; CDS DS 121. Spring 2024: The Data Visualization X-Lab Practicum offers students an opportunity to learn data visualization skills through course and project-based work. Projects will be completed on a schedule that aligns with topics being covered in class and assignments. This course provides an accurate experience of solving real-world problems with data visualization, and the various tradeoffs that need to be considered. Whether it's how to efficiently use color and space, effectively understand the profile of a dataset or cautiously avoid bias, this course will provide students with a solid understanding of applicable data visualization practices.
- CDS DS 595: Special Topics in Physical and Engineering Sciences Coverage of a specific topic in relation to physical and engineering sciences in data science. Topics vary semester to semester.
- CDS DS 596: Special Topics in Natural, Biological and Medical Sciences Undergraduate Prerequisites: CDS DS 110 ; CDS DS 120 ; CDS DS 121 ; CDS DS 122; or equivalent courses For Spring 2024 - Foundations of Biological Data Science. This course establishes a foundation in applied statistics and data science in biology for those interested in pursuing data-driven research. Students will develop fundamental and transferable computational and statistical skills for critically thinking about and using data in biology. The course will develop the foundations of and illustrate major methods applied in modern biological problems and data sets. Data science topics may include data wrangling, exploration and visualization, statistical programming, likelihood based inference, bootstrap, regularization, statistical modeling, principal components analysis, multiple hypothesis testing, network modeling, and causality. The course will explore application of these methods in the context of gene regulatory networks, genotype to phenotype mapping, chromatin structure analysis, single-cell biology, and quantitative biological imaging. The python programming language is extensively used to explore methods and analyze data.
- CDS DS 597: Special Topics in Social and Behavioral Sciences Coverage of a specific topic in relation to social and behavioral sciences in data science. Topics vary semester to semester.
- CDS DS 598: Special Topics in Machine Learning Undergraduate Prerequisites: DS210 or equivalent, DS320 or equivalent, DS 340 or equivalent - Spring 2024: A Section - This course introduces students to the field of Reinforcement Learning (RL). We will cover (1) the basic concepts in r. and (3) modern challenges (exploration, partial observability, multi-agent RL). B Section - In this course students will gain an understanding of the fundamentals in Deep Learning and then apply those concepts in exercises and applications in python. You'll learn the foundations of training and inference, then become familiar with canonical network architectures including convolutional neural networks and transformers. We'll look at generative pre- trained Transformer models and pros and cons of prompt engineering versus finetuning. Finally, students will be able to apply many of the techniques they learned in a final class project.
- CDS DS 599: CDS Research Development Seminar The first--year doctoral seminar is a required two--semester cohort--based course (4 credits) that must be taken during the first full academic year that a student enrolls in the PhD program in CDS. It is divided into two parts, each providing 2 credits. "CDS Research Initiation Seminar" is offered in the fall semester, and "CDS Research Development Seminar" is offered in the spring semester. The seminar serves three key purposes: 1. It introduces students to the scholarship of (and the rich set of research projects pursued by) the CDS faculty and their guests through colloquia pitched to a multidisciplinary audience. 2. It guides students through the challenging transition into the graduate program in CDS by introducing them to the variety of skills and capacities that are needed to succeed as a scholar. 3. It engenders a sense of community amongst the group of students entering the program as a cohort. 4 cr. Either sem.
- CDS DS 644: Machine Learning for Business Analytics The internet has become a ubiquitous channel for reaching consumers and gathering massive amounts of business-intelligence data. This course will teach students how to perform hands-on analytics on such datasets using modern machine learning techniques through series a lectures and in- class team exercises. Students will analyze data using the R programming language, derive actionable insights from the data, and present their findings. The goal of the course is to create an understanding of modern analytics methods, and the types of problems they can be applied to. The course is open to students with or without a technical background who are interested in analytics. While no prior programming experience is required, students will learn the fundamentals of the R programming language to build and evaluate predictive models.
- CDS DS 657: Law for Algorithms Algorithms - those information-processing machines designed by humans - reach ever more deeply into our lives, creating alternate and sometimes enhanced manifestations of social and biological processes. In doing so, algorithms yield powerful levers for good and ill amidst a sea of unforeseen consequences. This crosscutting and interdisciplinary course investigates several aspects of algorithms and their impact on society and law. Specifically, the course connects concepts of proof, verifiability, privacy, security, trust, and randomness in computer science with legal concepts of autonomy, consent, governance, and liability, and examines interests at the evolving intersection of technology and the law. Grades will be based on a combination of short weekly reflection papers and a final project, to be completed collaboratively in mixed teams of law and computer and data science students. This course will include attendees from the computer science faculty, students and scholars based at Boston University and UC Berkeley.
- CDS DS 680: AI Ethics This course develops students' ability to critically examine and question the interplay between data science and computational technologies on the one hand, and society and public policy on the other. Students will complete exercises to demonstrate their facility with key ethics tools and techniques, and analyze a series of real-world case studies presented alongside ethical tools and analyses that are useful both for staying alert to emerging ethical challenges and responding to them as they arise in both employment settings and everyday life.
- CDS DS 682: Responsible AI, Law, Ethics & Society Undergraduate Prerequisites: CDSDS100/CDSDS110 (Intro to data science OR equivalent) and CDSDS340 (intro to ML and AI OR equivalent) This course addresses the deployment of Artificial Intelligence systems across various societal domains, raising fundamental challenges and concerns such as accountability, liability, fairness, transparency, and privacy. Tackling these challenges necessitates an interdisciplinary approach, integrating principles and practices from data science, ethics, and law. This unique course will bring together students from computing and data science disciplines as well as law and public policy disciplines from multiple institutions. Permission is required to register for this course. Course page: https://learn.responsibly.ai. Please fill out an application form here: https://forms.gle/bMRECdYcMUwHj7xG8. Instructor: [email protected].
- CDS DS 690: Directed Study in Computing & Data Sciences Directed study in Computing & Data Sciences provides students the opportunity to complete directed research in a selected topic not covered in a regularly scheduled course under the supervision of a faculty member. Student and supervising faculty member arrange and document expectations and requirements. Examples include in-depth study of a special topic or independent research project.
- CDS DS 699: Advanced Topics in Data Science Various advanced topics in data science that vary semester to semester. Please contact CDS for detailed descriptions.
- CDS DS 701: Tools for Data Science This is a new course to be designed specifically for the MS DS program. Students will take this course in their first semester. The goal of the course is to give students exposure to, and practical experience in, formulating data science questions -- particularly learning how to ask good questions in a specific domain. The course will also cover methods of obtaining data and common methods of processing data from a practical standpoint. It will be organized around a semester-long group project in which students are organized into teams and engage with "clients" who bring data science questions from a particular domain. The course will include a formal presentation of results at the end of the semester.
- CDS DS 719: Data Science Product Management 1
- CDS DS 729: Data Science Product Management 2
- CDS DS 990: Computing & Data Sciences Lab Rotation Experience with translational or applied research pursued in an industrial or laboratory setting is important for in-the-field graduate training. To provide this training, graduate students may complete a lab rotation that provides them with the opportunity to (1) explore lab settings and industrial collaborations that may be relevant to their thesis work; (2) experience the diversity of applied and in-the-field research styles in computing and data sciences; and (3) expand their external network of potential research collaborators. Before beginning an external lab rotation, students are expected to develop a plan for the scope and expected outcomes of the work to be pursued under the supervision of a named lab rotation advisor. This plan must be pre-approved by the student's academic, research, or thesis advisor in CDS. While desirable, it is neither required nor expected that the rotation will result in completion of a substantial body of work. Lab rotation credits will be noted on the student transcript as a 4-credit pass/fail independent study course numbered as DS-990.
- CDS DS 992: Computing & Data Sciences Research Rotation Experience with diverse research group projects and styles are an essential part of graduate training. To provide this training, graduate students are expected to complete a series of research rotations that provide them with the opportunity to (1) explore research groups where they may pursue future thesis work; (2) experience the diversity of sub-disciplines and research styles in computing and data sciences; and (3) expand their network of potential research collaborators. Before beginning a rotation, students are expected to discuss their plans with the faculty leadership of the BU research group they would like to join, leading to a clear definition of the scope and expected outcomes of the work pursued under the supervision of a named rotation advisor. While desirable, it is neither required nor expected that the rotation will result in completion of a substantial body of work. Research rotation credits will be noted on the student transcript as a 4-credit independent study course numbered as DS-991 (for Fall semester rotations) or DS-992 (for Spring semester rotations).
Related Bulletin Pages
- Abbreviations and Symbols
Beyond the Bulletin
- Faculty of Computing & Data Sciences
- Data Science for Good
- Impact Labs & Co-Labs
Terms of Use
Note that this information may change at any time. Read the full terms of use .
Accreditation
Boston University is accredited by the New England Commission of Higher Education (NECHE).
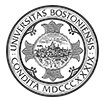
- © Copyright
- Mobile Version
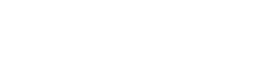
Recent Dissertation Topics
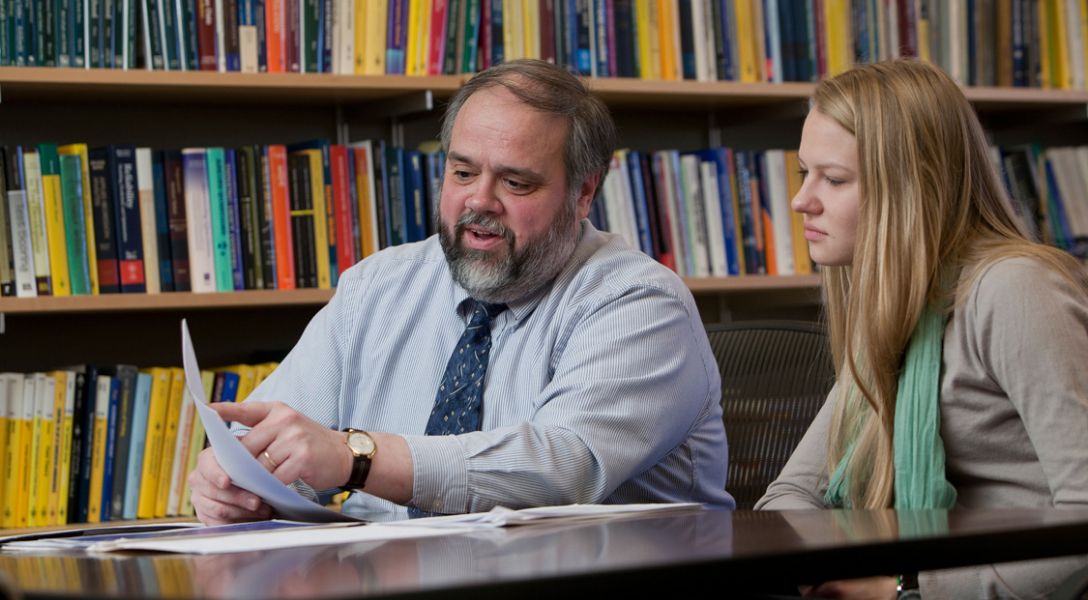
Kerstin Emily Frailey - “PRACTICAL DATA QUALITY FOR MODERN DATA & MODERN USES, WITH APPLICATIONS TO AMERICA’S COVID-19 DATA"
Dissertation Advisor: Martin Wells
Initial job placement: Co-Founder & CEO
David Kent - “Smoothness-Penalized Deconvolution: Rates of Convergence, Choice of Tuning Parameter, and Inference"
Dissertation Advisor: David Ruppert
Initial job placement: VISITING ASSISTANT PROFESSOR - Cornell University
Yuchen Xu - “Dynamic Atomic Column Detection in Transmission Electron Microscopy Videos via Ridge Estimation”
Dissertation Advisor: David Matteson
Initial job placement: Postdoctoral Fellow - UCLA
Siyi Deng - “Optimal and Safe Semi-supervised Estimation and Inference for High-dimensional Linear Regression"
Dissertation Advisor: Yang Ning
Initial job placement: Data Scientist - TikTok
Peter (Haoxuan) Wu - “Advances in adaptive and deep Bayesian state-space models”
Initial job placement: Quantitative Researcher - DRW
Grace Deng - “Generative models and Bayesian spillover graphs for dynamic networks”
Initial job placement: Data Scientist - Research at Google
Samriddha Lahiry - “Some problems of asymptotic quantum statistical inference”
Dissertation Advisor: Michael Nussbaum
Initial job placement: Postdoctoral Fellow - Harvard University
Yaosheng Xu - “WWTA load-balancing for parallel-server systems with heterogeneous servers and multi-scale heavy traffic limits for generalized Jackson networks”
Dissertation Advisor: Jim Dai
Initial job placement: Applied Scientist - Amazon
Seth Strimas-Mackey - “Latent structure in linear prediction and corpora comparison”
Dissertation Advisor: Marten Wegkamp and Florentina Bunea
Initial job placement: Data Scientist at Google
Tao Zhang - “Topics in modern regression modeling”
Dissertation Advisor: David Ruppert and Kengo Kato
Initial job placement: Quantitative Researcher - Point72
Wentian Huang - “Nonparametric and semiparametric approaches to functional data modeling”
Initial job placement: Ernst & Young
Binh Tang - “Deep probabilistic models for sequential prediction”
Initial job placement: Amazon
Yi Su - “Off-policy evaluation and learning for interactive systems"
Dissertation Advisor: Thorsten Joachims
Initial job placement: Berkeley (postdoc)
Ruqi Zhang - “Scalable and reliable inference for probabilistic modeling”
Dissertation Advisor: Christopher De Sa
Jason Sun - “Recent developments on Matrix Completion"
Initial job placement: LinkedIn

Indrayudh Ghosal - “Model combinations and the Infinitesimal Jackknife : how to refine models with boosting and quantify uncertainty”
Dissertation Advisor: Giles Hooker
Benjamin Ryan Baer - “Contributions to fairness and transparency”
Initial job placement: Rochester (postdoc)
Megan Lynne Gelsinger - “Spatial and temporal approaches to analyzing big data”
Dissertation Advisor: David Matteson and Joe Guinness
Initial job placement: Institute for Defense Analysis
Zhengze Zhou - “Statistical inference for machine learning : feature importance, uncertainty quantification and interpretation stability”
Initial job placement: Facebook
Huijie Feng - “Estimation and inference of high-dimensional individualized threshold with binary responses”
Initial job placement: Microsoft
Xiaojie Mao - “Machine learning methods for data-driven decision making : contextual optimization, causal inference, and algorithmic fairness”
Dissertation Advisor: Nathan Kallus and Madeleine Udell
Initial job placement: Tsinghua University, China
Xin Bing - “Structured latent factor models : Identifiability, estimation, inference and prediction”
Initial job placement: Cambridge (postdoc), University of Toronto
Yang Liu - “Nonparametric regression and density estimation on a network"
Dissertation Advisor: David Ruppert and Peter Frazier
Initial job placement: Research Analyst - Cubist Systematic Strategies
Skyler Seto - “Learning from less : improving and understanding model selection in penalized machine learning problems”
Initial job placement: Machine Learning Researcher - Apple
Jiekun Feng - “Markov chain, Markov decision process, and deep reinforcement learning with applications to hospital management and real-time ride-hailing”
Initial job placement:
Wenyu Zhang - “Methods for change point detection in sequential data”
Initial job placement: Research Scientist - Institute for Infocomm Research
Liao Zhu - “The adaptive multi-factor model and the financial market"
Initial job placement: Quantitative Researcher - Two Sigma
Xiaoyun Quan - “Latent Gaussian copula model for high dimensional mixed data, and its applications”
Dissertation Advisor: James Booth and Martin Wells
Praphruetpong (Ben) Athiwaratkun - "Density representations for words and hierarchical data"
Dissertation Advisor: Andrew Wilson
Initial job placement: AI Scientist - AWS AI Labs
Yiming Sun - “High dimensional data analysis with dependency and under limited memory”
Dissertation Advisor: Sumanta Basu and Madeleine Udell
Zi Ye - “Functional single index model and jensen effect"
Dissertation Advisor: Giles Hooker
Initial job placement: Data & Applied Scientist - Microsoft
Hui Fen (Sarah) Tan - “Interpretable approaches to opening up black-box models”
Dissertation Advisor: Giles Hooker and Martin Wells
Daniel E. Gilbert - “Luck, fairness and Bayesian tensor completion”
Yichen zhou - “asymptotics and interpretability of decision trees and decision tree ensemblesg”.
Initial job placement: Data Scientist - Google
Ze Jin - “Measuring statistical dependence and its applications in machine learning”
Initial job placement: Research Scientist, Facebook Integrity Ranking & ML - Facebook
Xiaohan Yan - “Statistical learning for structural patterns with trees”
Dissertation Advisor: Jacob Bien
Initial job placement: Senior Data Scientist - Microsoft
Guo Yu - “High-dimensional structured regression using convex optimization”
Dan kowal - "bayesian methods for functional and time series data".
Dissertation Advisor: David Matteson and David Ruppert
Initial job placement: assistant professor, Department of Statistics, Rice University
Keegan Kang - "Data Dependent Random Projections"
David sinclair - "model selection results for high dimensional graphical models on binary and count data with applications to fmri and genomics", liu, yanning – "statistical issues in the design and analysis of clinical trials".
Dissertation Advisor: Bruce Turnbull
Nicholson, William Bertil – "Tools for Modeling Sparse Vector Autoregressions"
Tupper, laura lindley – "topics in classification and clustering of high-dimensional data", chetelat, didier – "high-dimensional inference by unbiased risk estimation".
Initial Job Placement: Assistant Professor Universite de Montreal, Montreal, Canada
Gaynanova, Irina – "Estimation Of Sparse Low-Dimensional Linear Projections"
Dissertation Advisor: James Booth
Initial Job Placement: Assistant Professor, Texas A&M, College Station, TX
Mentch, Lucas – "Ensemble Trees and CLTS: Statistical Inference in Machine Learning"
Initial Job Placement: Assistant Professor, University of Pittsburgh, Pittsburgh, PA
Risk, Ben – "Topics in Independent Component Analysis, Likelihood Component Analysis, and Spatiotemporal Mixed Modeling"
Dissertation Advisors: David Matteson and David Ruppert
Initial Job Placement: Postdoctoral Fellow, University of North Carolina, Chapel Hill, NC
Zhao, Yue – "Contributions to the Statistical Inference for the Semiparametric Elliptical Copula Model"
Disseration Advisor: Marten Wegkamp
Initial Job Placement: Postoctoral Fellow, McGill University, Montreal, Canada
Chen, Maximillian Gene – "Dimension Reduction and Inferential Procedures for Images"
Dissertation Advisor: Martin Wells
Earls, Cecelia – Bayesian hierarchical Gaussian process models for functional data analysis
Dissertation Advisor: Giles Hooker
Initial Job Placement: Lecturer, Cornell University, Ithaca, NY
Li, James Yi-Wei – "Tensor (Multidimensional Array) Decomposition, Regression, and Software for Statistics and Machine Learning"
Initial Job Placement: Research Scientist, Yahoo Labs
Schneider, Matthew John – "Three Papers on Time Series Forecasting and Data Privacy"
Dissertation Advisor: John Abowd
Initial Job Placement: Assistant Professor, Northwestern University, Evanston, IL
Thorbergsson, Leifur – "Experimental design for partially observed Markov decision processes"
Initial Job Placement: Data Scientist, Memorial Sloan Kettering Cancer Center, New York, NY
Wan, Muting – "Model-Based Classification with Applications to High-Dimensional Data in Bioinformatics"
Initial Job Placement: Senior Associate, 1010 Data, New York, NY
Johnson, Lynn Marie – "Topics in Linear Models: Methods for Clustered, Censored Data and Two-Stage Sampling Designs"
Dissertation Advisor: Robert Strawderman
Initial Job Placement: Statistical Consultant, Cornell, Statistical Consulting Unit, Ithaca, NY
Tecuapetla Gomez, Inder Rafael – "Asymptotic Inference for Locally Stationary Processes"
Initial Job Placement: Postdoctoral Fellow, Georg-August-Universitat Gottigen, Gottigen, Germany.
Bar, Haim – "Parallel Testing, and Variable Selection -- a Mixture-Model Approach with Applications in Biostatistics"
Dissertation Advisor: James Booth
Initial Job Placement: Postdoc, Department of Medicine, Weill Medical Center, New York, NY
Cunningham, Caitlin – "Markov Methods for Identifying ChIP-seq Peaks"
Initial Job Placement: Assistant Professor, Le Moyne College, Syracuse, NY
Ji, Pengsheng – "Selected Topics in Nonparametric Testing and Variable Selection for High Dimensional Data"
Dissertation Advisor: Michael Nussbaum
Initial Job Placement: Assistant Professor, University of Georgia, Athens, GA
Morris, Darcy Steeg – "Methods for Multivariate Longitudinal Count and Duration Models with Applications in Economics"
Dissertation Advisor: Francesca Molinari
Initial Job Placement: Research Mathematical Statistician, Center for Statistical Research and Methodology, U.S. Census Bureau, Washington DC
Narayanan, Rajendran – "Shrinkage Estimation for Penalised Regression, Loss Estimation and Topics on Largest Eigenvalue Distributions"
Initial Job Placement: Visiting Scientist, Indian Statistical Institute, Kolkata, India
Xiao, Luo – "Topics in Bivariate Spline Smoothing"
Dissertation Advisor: David Ruppert
Initial Job Placement: Postdoc, Johns Hopkins University, Baltimore, MD
Zeber, David – "Extremal Properties of Markov Chains and the Conditional Extreme Value Model"
Dissertation Advisor: Sidney Resnick
Initial Job Placement: Data Analyst, Mozilla, San Francisco, CA
Clement, David – "Estimating equation methods for longitudinal and survival data"
Dissertation Advisor: Robert Strawderman
Initial Job Placement: Quantitative Analyst, Smartodds, London UK
Eilertson, Kirsten – "Estimation and inference of random effect models with applications to population genetics and proteomics"
Dissertation Advisor: Carlos Bustamante
Initial Job Placement: Biostatistician, The J. David Gladstone Institutes, San Francisco CA
Grabchak, Michael – "Tempered stable distributions: properties and extensions"
Dissertation Advisor: Gennady Samorodnitsky
Initial Job Placement: Assistant Professor, UNC Charlotte, Charlotte NC
Li, Yingxing – "Aspects of penalized splines"
Initial Job Placement: Assistant Professor, The Wang Yanan Institute for Studies in Economics, Xiamen University
Lopez Oliveros, Luis – "Modeling end-user behavior in data networks"
Dissertation Advisor: Sidney Resnick
Initial Job Placement: Consultant, Murex North America, New York NY
Ma, Xin – "Statistical Methods for Genome Variant Calling and Population Genetic Inference from Next-Generation Sequencing Data"
Initial Job Placement: Postdoc, Stanford University, Stanford CA
Kormaksson, Matthias – "Dynamic path analysis and model based clustering of microarray data"
Dissertation Advisor: James Booth
Initial Job Placement: Postdoc, Department of Public Health, Weill Cornell Medical College, New York NY
Schifano, Elizabeth – "Topics in penalized estimation"
Initial Job Placement: Postdoc, Department of Biostatistics, Harvard University, Boston MA
Hanlon, Bret – "High-dimensional data analysis"
Dissertation Advisor: Anand Vidyashankar
Shaby, Benjamin – "Tools for hard bayesian computations"
Initial Job Placement: Postdoc, SAMSI, Durham NC
Zipunnikov, Vadim – "Topics on generalized linear mixed models"
Initial Job Placement: Postdoc, Department of Biostatistics, Johns Hopkins University, Baltimore MD
Barger, Kathryn Jo-Anne – "Objective bayesian estimation for the number of classes in a population using Jeffreys and reference priors"
Dissertation Advisor: John Bunge
Initial Job Placement: Pfizer Incorporated
Chan, Serena Suewei – "Robust and efficient inference for linear mixed models using skew-normal distributions"
Initial Job Placement: Statistician, Takeda Pharmaceuticles, Deerfield IL
Lin, Haizhi – "Distressed debt prices and recovery rate estimation"
Dissertation Advisor: Martin Wells
Initial Job Placement: Associate, Fixed Income Department, Credit Suisse Securities (USA), New York, NY

Thesis/Capstone for Master's in Data Science | Northwestern SPS - Northwestern School of Professional Studies
- Post-baccalaureate
- Undergraduate
- Professional Development
- Pre-College
- Center for Public Safety
- Get Information
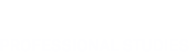
Data Science
Capstone and thesis overview.
Capstone and thesis are similar in that they both represent a culminating, scholarly effort of high quality. Both should clearly state a problem or issue to be addressed. Both will allow students to complete a larger project and produce a product or publication that can be highlighted on their resumes. Students should consider the factors below when deciding whether a capstone or thesis may be more appropriate to pursue.
A capstone is a practical or real-world project that can emphasize preparation for professional practice. A capstone is more appropriate if:
- you don't necessarily need or want the experience of the research process or writing a big publication
- you want more input on your project, from fellow students and instructors
- you want more structure to your project, including assignment deadlines and due dates
- you want to complete the project or graduate in a timely manner
A student can enroll in MSDS 498 Capstone in any term. However, capstone specialization courses can provide a unique student experience and may be offered only twice a year.
A thesis is an academic-focused research project with broader applicability. A thesis is more appropriate if:
- you want to get a PhD or other advanced degree and want the experience of the research process and writing for publication
- you want to work individually with a specific faculty member who serves as your thesis adviser
- you are more self-directed, are good at managing your own projects with very little supervision, and have a clear direction for your work
- you have a project that requires more time to pursue
Students can enroll in MSDS 590 Thesis as long as there is an approved thesis project proposal, identified thesis adviser, and all other required documentation at least two weeks before the start of any term.
From Faculty Director, Thomas W. Miller, PhD
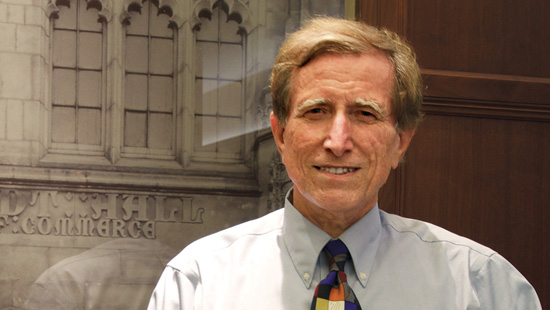
Capstone projects and thesis research give students a chance to study topics of special interest to them. Students can highlight analytical skills developed in the program. Work on capstone and thesis research projects often leads to publications that students can highlight on their resumes.”
A thesis is an individual research project that usually takes two to four terms to complete. Capstone course sections, on the other hand, represent a one-term commitment.
Students need to evaluate their options prior to choosing a capstone course section because capstones vary widely from one instructor to the next. There are both general and specialization-focused capstone sections. Some capstone sections offer in individual research projects, others offer team research projects, and a few give students a choice of individual or team projects.
Students should refer to the SPS Graduate Student Handbook for more information regarding registration for either MSDS 590 Thesis or MSDS 498 Capstone.
Capstone Experience
If students wish to engage with an outside organization to work on a project for capstone, they can refer to this checklist and lessons learned for some helpful tips.
Capstone Checklist
- Start early — set aside a minimum of one to two months prior to the capstone quarter to determine the industry and modeling interests.
- Networking — pitch your idea to potential organizations for projects and focus on the business benefits you can provide.
- Permission request — make sure your final project can be shared with others in the course and the information can be made public.
- Engagement — engage with the capstone professor prior to and immediately after getting the dataset to ensure appropriate scope for the 10 weeks.
- Teambuilding — recruit team members who have similar interests for the type of project during the first week of the course.
Capstone Lesson Learned
- Access to company data can take longer than expected; not having this access before or at the start of the term can severely delay the progress
- Project timeline should align with coursework timeline as closely as possible
- One point of contact (POC) for business facing to ensure streamlined messages and more effective time management with the organization
- Expectation management on both sides: (business) this is pro-bono (students) this does not guarantee internship or job opportunities
- Data security/masking not executed in time can risk the opportunity completely
Publication of Work
Northwestern University Libraries offers an option for students to publish their master’s thesis or capstone in Arch, Northwestern’s open access research and data repository.
Benefits for publishing your thesis:
- Your work will be indexed by search engines and discoverable by researchers around the world, extending your work’s impact beyond Northwestern
- Your work will be assigned a Digital Object Identifier (DOI) to ensure perpetual online access and to facilitate scholarly citation
- Your work will help accelerate discovery and increase knowledge in your subject domain by adding to the global corpus of public scholarly information
Get started:
- Visit Arch online
- Log in with your NetID
- Describe your thesis: title, author, date, keywords, rights, license, subject, etc.
- Upload your thesis or capstone PDF and any related supplemental files (data, code, images, presentations, documentation, etc.)
- Select a visibility: Public, Northwestern-only, Embargo (i.e. delayed release)
- Save your work to the repository
Your thesis manuscript or capstone report will then be published on the MSDS page. You can view other published work here .
For questions or support in publishing your thesis or capstone, please contact [email protected] .
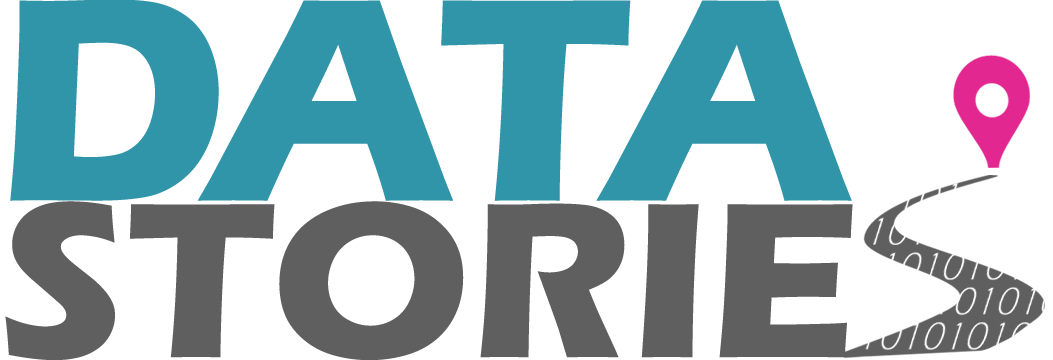
Diploma Theses
Diploma Theses Catalogue, 2023-24
The Data Science Lab offers a list of suggested diploma theses to undergraduate and postgraduate students in subjects relative to the research interests of the laboratory. For more information, contact the person in charge of each diploma thesis.
2023-24 theses proposals and preparation guidelines
Selected theses:
– Efstratios Karkanis: Prediction of Traffic Flow in Road Networks (in Greek). BSc thesis, Dept of Informatics, University of Piraeus, October 2023. [ pdf ]
– Giorgos Mimoglou: Detecting Statistically Significant Spatial Clusters in Vessel Data (in Greek). BSc thesis, Dept of Informatics, University of Piraeus, September 2021. [ pdf ]
– Giorgos Theofilopoulos: Predicting Vessels’ Estimated Time of Arrival using Machine Learning Techniques (in Greek). BSc thesis, Dept of Informatics, University of Piraeus, July 2021. [ pdf ]
– Nikos Galanakis: Streaming Data Clustering using D-Stream Algorithm (in Greek). BSc thesis, Dept of Informatics, University of Piraeus, October 2017. [ pdf ]
Privacy Overview
Cookie | Duration | Description |
---|---|---|
cookielawinfo-checkbox-analytics | 11 months | This cookie is set by GDPR Cookie Consent plugin. The cookie is used to store the user consent for the cookies in the category "Analytics". |
cookielawinfo-checkbox-functional | 11 months | The cookie is set by GDPR cookie consent to record the user consent for the cookies in the category "Functional". |
cookielawinfo-checkbox-necessary | 11 months | This cookie is set by GDPR Cookie Consent plugin. The cookies is used to store the user consent for the cookies in the category "Necessary". |
cookielawinfo-checkbox-others | 11 months | This cookie is set by GDPR Cookie Consent plugin. The cookie is used to store the user consent for the cookies in the category "Other. |
cookielawinfo-checkbox-performance | 11 months | This cookie is set by GDPR Cookie Consent plugin. The cookie is used to store the user consent for the cookies in the category "Performance". |
viewed_cookie_policy | 11 months | The cookie is set by the GDPR Cookie Consent plugin and is used to store whether or not user has consented to the use of cookies. It does not store any personal data. |
Cookie | Duration | Description |
---|---|---|
_ga | Google Analytics: Used to distinguish users. | |
_gat | Google Analytics: Used to throttle request rate. | |
_gid | Google Analytics: Used to distinguish users. |
- Harvard Library
- Research Guides
- Faculty of Arts & Sciences Libraries
Computer Science Library Research Guide
Find dissertations and theses.
- Get Started
- How to get the full-text
- What is Peer Review?
- Find Books in the SEC Library This link opens in a new window
- Find Conference Proceedings
- Find Patents This link opens in a new window
- Find Standards
- Find Technical Reports
- Find Videos
- Ask a Librarian This link opens in a new window
Engineering Librarian

How to search for Harvard dissertations
- DASH , Digital Access to Scholarship at Harvard, is the university's central, open-access repository for the scholarly output of faculty and the broader research community at Harvard. Most Ph.D. dissertations submitted from March 2012 forward are available online in DASH.
- Check HOLLIS, the Library Catalog, and refine your results by using the Advanced Search and limiting Resource Type to Dissertations
- Search the database ProQuest Dissertations & Theses Global Don't hesitate to Ask a Librarian for assistance.
How to search for Non-Harvard dissertations
Library Database:
- ProQuest Dissertations & Theses Global
Free Resources:
- Many universities provide full-text access to their dissertations via a digital repository. If you know the title of a particular dissertation or thesis, try doing a Google search.
Related Sites
- Formatting Your Dissertation - GSAS
- Ph.D. Dissertation Submission - FAS
- Empowering Students Before you Sign that Contract! - Copyright at Harvard Library
Select Library Titles
- << Previous: Find Conference Proceedings
- Next: Find Patents >>
- Last Updated: Feb 27, 2024 1:52 PM
- URL: https://guides.library.harvard.edu/cs
Harvard University Digital Accessibility Policy
Navigation Menu
Search code, repositories, users, issues, pull requests..., provide feedback.
We read every piece of feedback, and take your input very seriously.
Saved searches
Use saved searches to filter your results more quickly.
To see all available qualifiers, see our documentation .
undergraduate-thesis
Here are 32 public repositories matching this topic..., doublez0108 / tj-graduation-project-2021.
Tongji Univ. Undergraduate Graduation Project 2021. | 🎉含: 同济er毕设答辩PPT模板
- Updated Jun 8, 2023
zfengg / HUSTtex
A series of TeX templates for undergraduate thesis at HUST.
- Updated Apr 10, 2023
Gnomeek / GDUT-UndergraduateThesis
Latex/Word Template for GDUT Undergraduate Students' Thesis(广东工业大学本科毕业论文LaTex/Word模板)
- Updated Apr 16, 2022
isaacarroyov / thesis_undergrad
Documentation: Methodology and Exploratory Data Analysis
- Updated May 15, 2024
- Jupyter Notebook
avnishsachar / manipal_thesis_template
Thesis template for Manipal Institute of Technology (final year project/ bachelor's thesis)
- Updated May 5, 2020
dmgolembiowski / BW-Chaos-Thesis-FA2018
Undergraduate Mathematics thesis repository for programming chaotic plotting and numerical analysis
- Updated Apr 3, 2019
netotz / alpha-neighbor-p-center-problem
Heuristic algorithms for the alpha-neighbor p-center problem.
- Updated Apr 5, 2024
zewei-Zhang / zjgsuthesis
用于浙江工商大学本科生毕业论文的Latex模板。
- Updated Apr 26, 2022
wiknwo / FYP-COMP30910-MSCP
The data, code and thesis for my own undergraduate thesis in the "FYP: Design and Implementation (COMP30910)" module.
- Updated Jun 24, 2024
lukablaskovic / reverse-image-search
Reverse image search using Milvus.io and Towhee.io. Undergraduate Thesis, Faculty of informatics in Pula (FIPU).
- Updated Jun 20, 2023
deepshig / Digitalization-of-Offline-Handdrawn-Flow-Diagrams
This is a web based application which takes as input the image of a handdrawn flow chart with text and polygonal hapes, and digitailzes it.
- Updated Feb 14, 2022
thurbridi / cnn-facies-classifier
My computer science undergraduate thesis for a degree at UFSC
- Updated Dec 8, 2022
chez14 / undergrad-thesis
My undergraduate thesis on Parahyangan Catholic University
- Updated Feb 17, 2021
Jemtaly / SDU-Undergraduate-Thesis-Template
山东大学本科毕业论文(设计)的 LaTeX 模板,参考《山东大学本科毕业论文(设计)撰写规范》
- Updated May 31, 2024
KarinaRovani / NFC-Reader-in-React
Undergraduate Thesis project in Portuguese with the goal of developing an React application using NFC to read products
- Updated May 10, 2023
geraked / bams
BigBlueButton & AdobeConnect Monitoring Software
- Updated Nov 9, 2021
kevinjnguyen / Compatible-Item-Recommendation
Compatible Item Recommendation is a collection of research documents and resources developed by Kevin J Nguyen and Victoria Wei as they pursued their research as Undergraduate Research Scholars.
- Updated May 4, 2018
felipecustodio / undergraduate-thesis
🎓 Undergraduate Thesis - Bachelor of Computer Science
- Updated Jun 23, 2021
aeglon97 / Evaluate-Historical-Stock-Market-Forecasts
- Updated Jun 11, 2020
PaulTran47 / ECON190
Pomona College Senior Seminar in Economics, spring 2017.
- Updated Feb 16, 2022
Improve this page
Add a description, image, and links to the undergraduate-thesis topic page so that developers can more easily learn about it.
Curate this topic
Add this topic to your repo
To associate your repository with the undergraduate-thesis topic, visit your repo's landing page and select "manage topics."
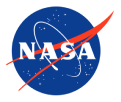
Suggested Searches
- Climate Change
- Expedition 64
- Mars perseverance
- SpaceX Crew-2
- International Space Station
- View All Topics A-Z
Humans in Space
Earth & climate, the solar system, the universe, aeronautics, learning resources, news & events.
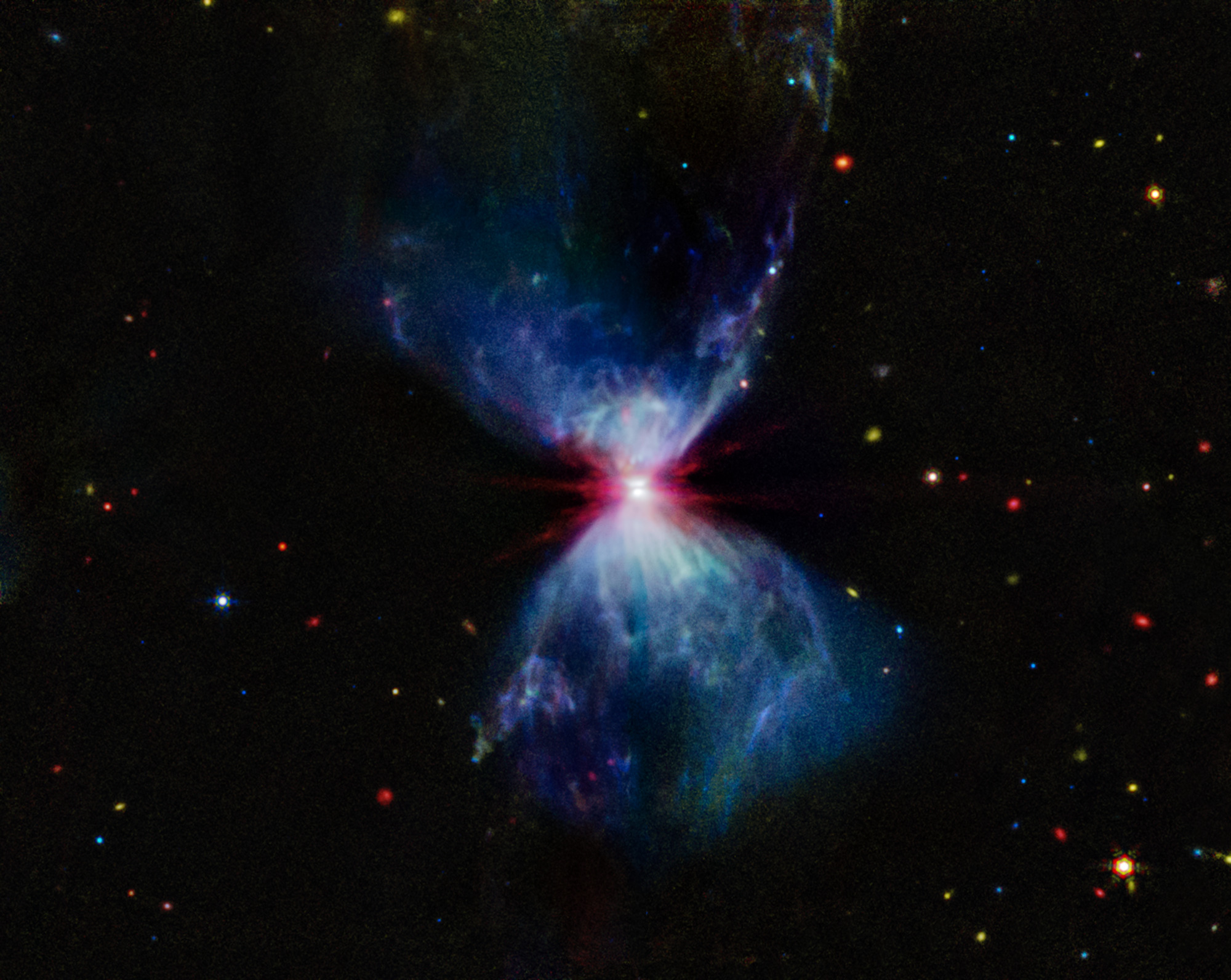
NASA’s Webb Captures Celestial Fireworks Around Forming Star

NASA Asteroid Experts Create Hypothetical Impact Scenario for Exercise
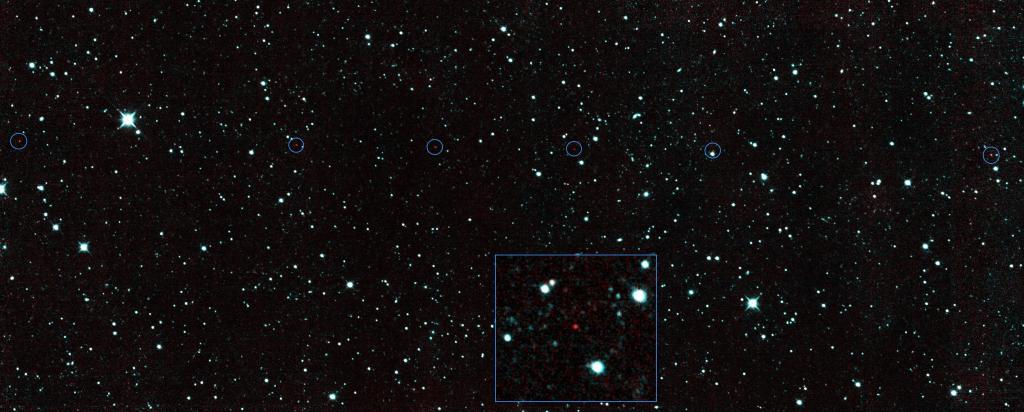
NASA’s NEOWISE Infrared Heritage Will Live On
- Search All NASA Missions
- A to Z List of Missions
- Upcoming Launches and Landings
- Spaceships and Rockets
- Communicating with Missions
- James Webb Space Telescope
- Hubble Space Telescope
- Why Go to Space
- Commercial Space
- Destinations
- Living in Space
- Explore Earth Science
- Earth, Our Planet
- Earth Science in Action
- Earth Multimedia
- Earth Science Researchers
- Pluto & Dwarf Planets
- Asteroids, Comets & Meteors
- The Kuiper Belt
- The Oort Cloud
- Skywatching
- The Search for Life in the Universe
- Black Holes
- The Big Bang
- Dark Energy & Dark Matter
- Earth Science
- Planetary Science
- Astrophysics & Space Science
- The Sun & Heliophysics
- Biological & Physical Sciences
- Lunar Science
- Citizen Science
- Astromaterials
- Aeronautics Research
- Human Space Travel Research
- Science in the Air
- NASA Aircraft
- Flight Innovation
- Supersonic Flight
- Air Traffic Solutions
- Green Aviation Tech
- Drones & You
- Technology Transfer & Spinoffs
- Space Travel Technology
- Technology Living in Space
- Manufacturing and Materials
- Science Instruments
- For Kids and Students
- For Educators
- For Colleges and Universities
- For Professionals
- Science for Everyone
- Requests for Exhibits, Artifacts, or Speakers
- STEM Engagement at NASA
- NASA's Impacts
- Centers and Facilities
- Directorates
- Organizations
- People of NASA
- Internships
- Our History
- Doing Business with NASA
- Get Involved
- Aeronáutica
- Ciencias Terrestres
- Sistema Solar
- All NASA News
- Video Series on NASA+
- Newsletters
- Social Media
- Media Resources
- Upcoming Launches & Landings
- Virtual Events
- Sounds and Ringtones
- Interactives
- STEM Multimedia

NASA’s ECOSTRESS Maps Burn Risk Across Phoenix Streets

NASA Shares Use Requirements with Commercial Destination Partners
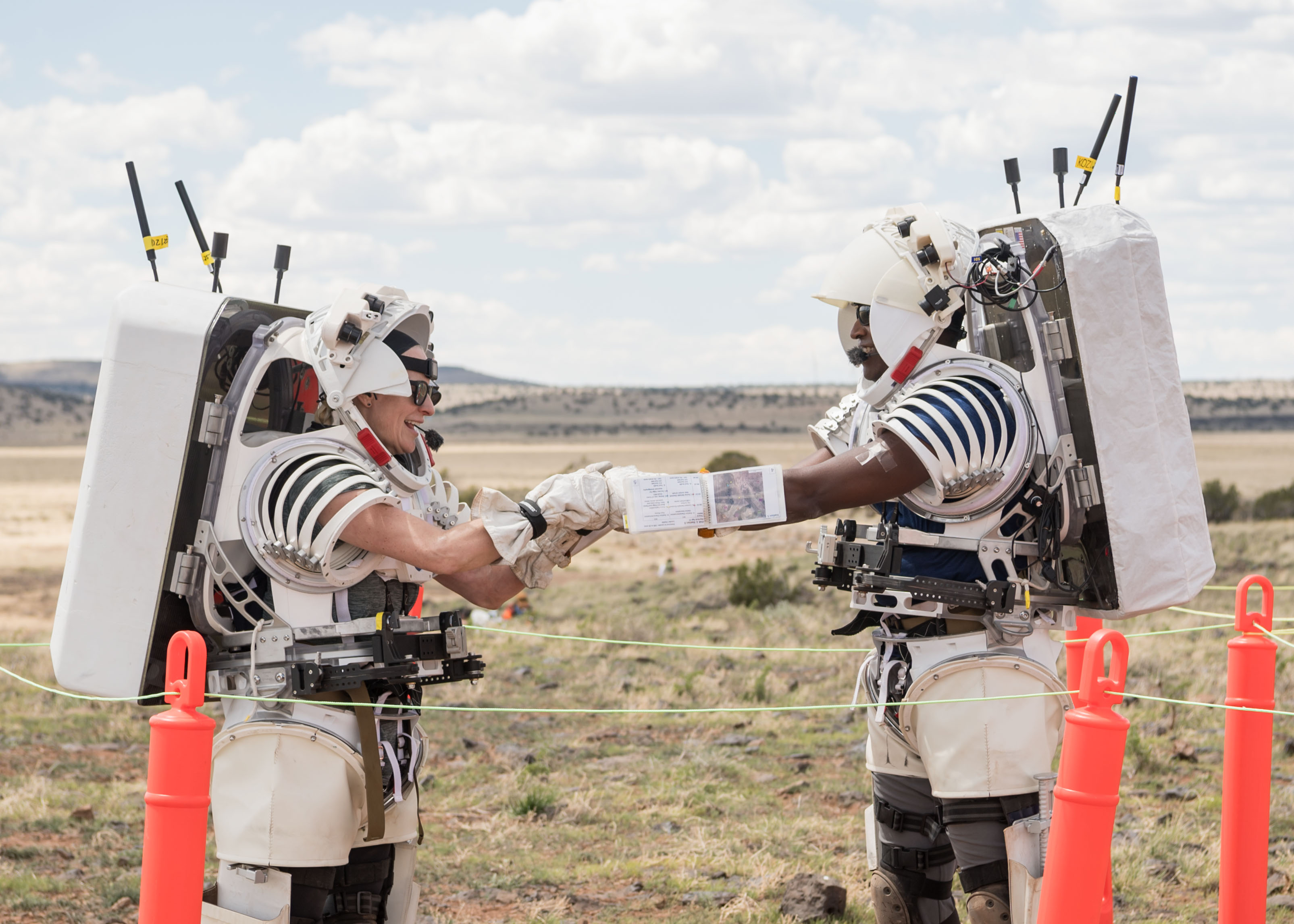
Behind the Scenes of a NASA ‘Moonwalk’ in the Arizona Desert
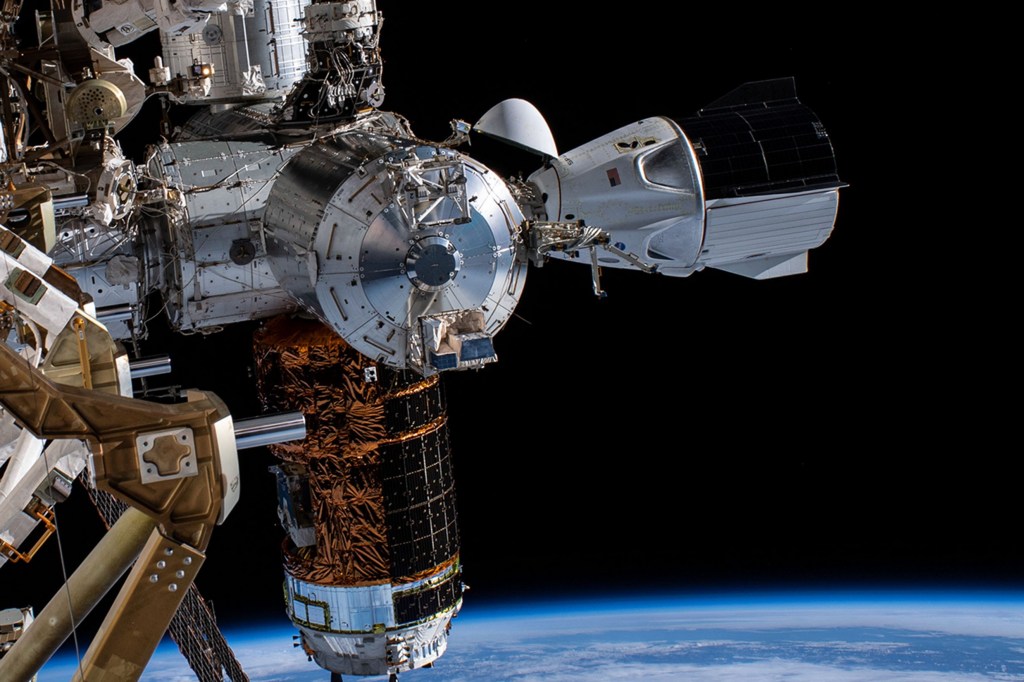
In Space Production Applications News
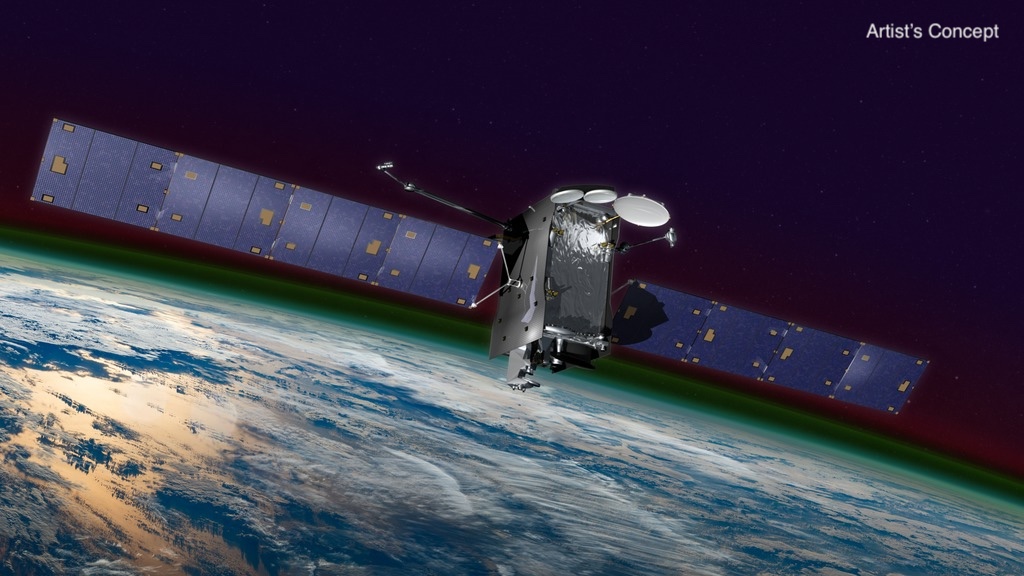
Alphabet Soup: NASA’s GOLD Finds Surprising C, X Shapes in Atmosphere

The 1998 Florida Firestorm and NASA’s Kennedy Space Center
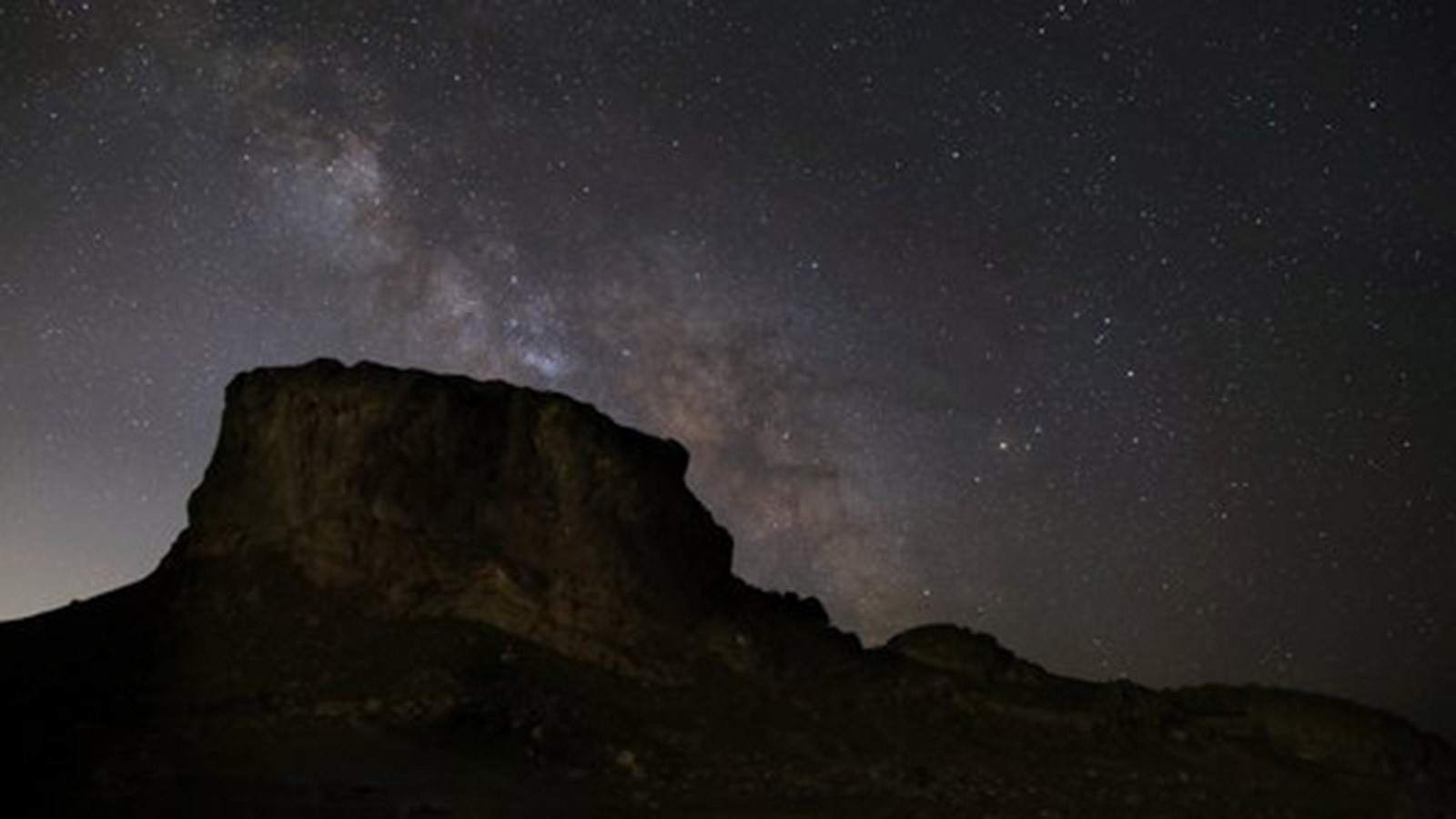
What’s Up: July 2024 Skywatching Tips from NASA

Bente Eegholm: Ensuring Space Telescopes Have Stellar Vision
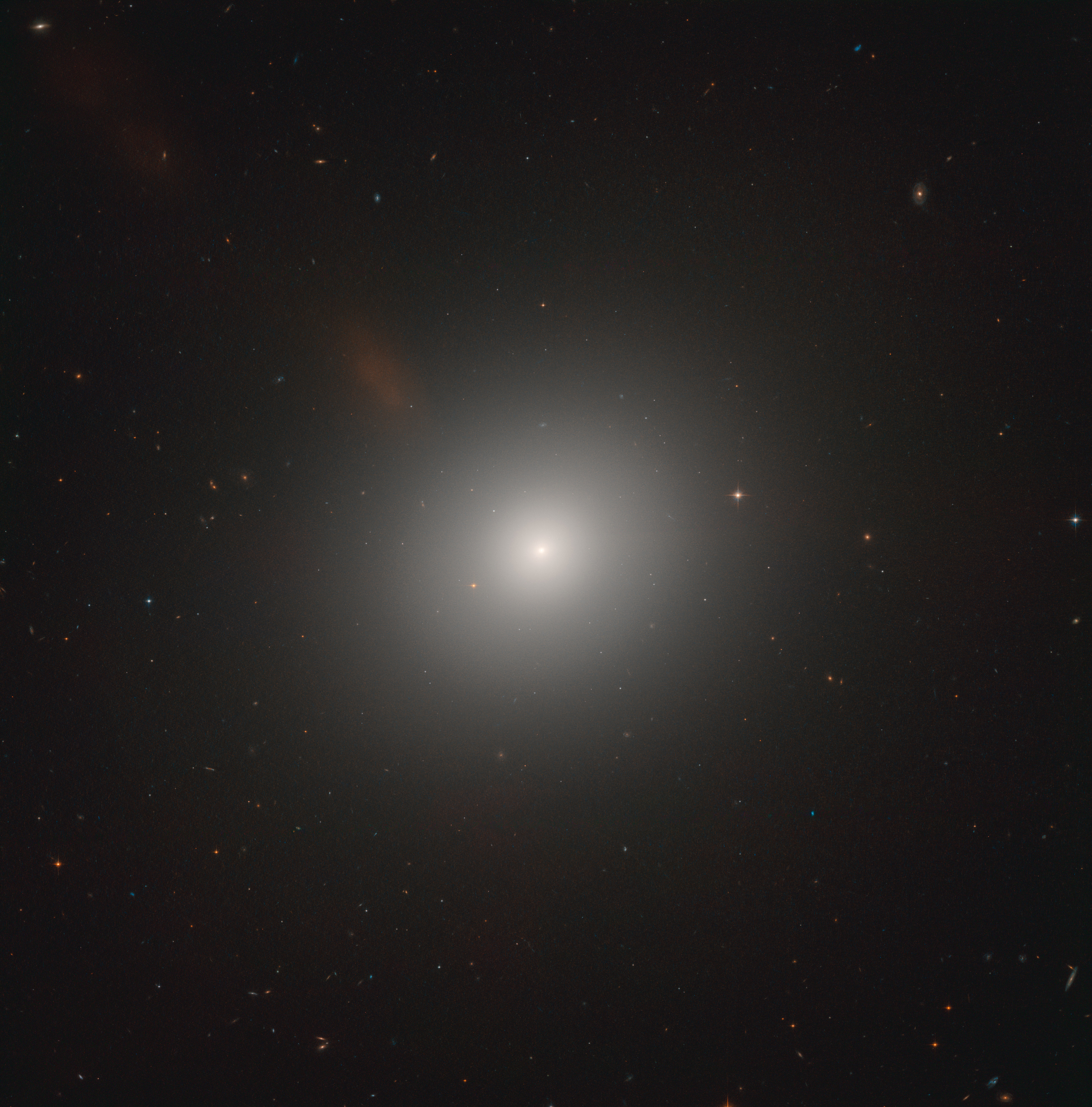
Hubble Examines an Active Galaxy Near the Lion’s Heart
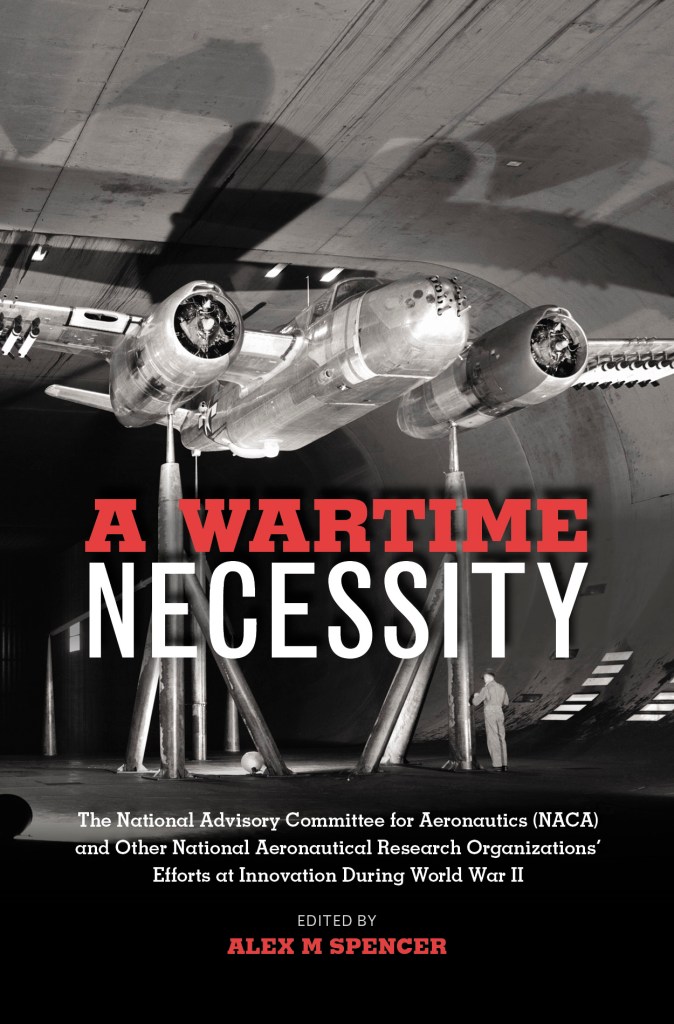
A Wartime Necessity
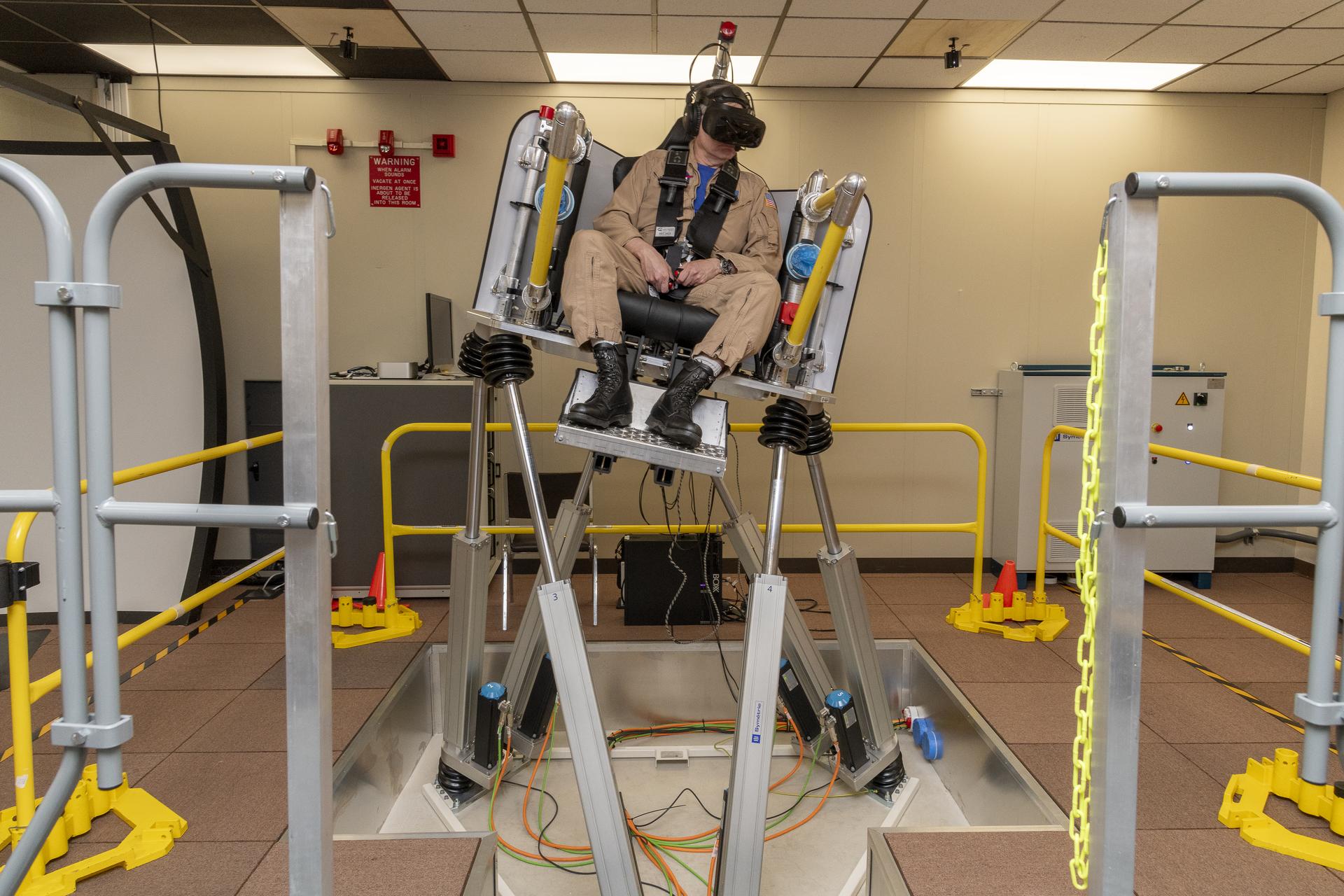
NASA Prepares for Air Taxi Passenger Comfort Studies
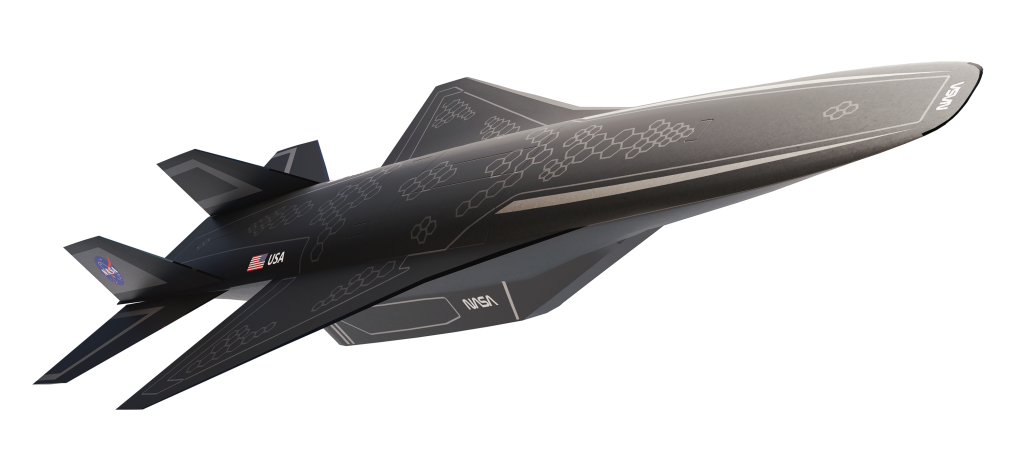
Hypersonic Technology Project
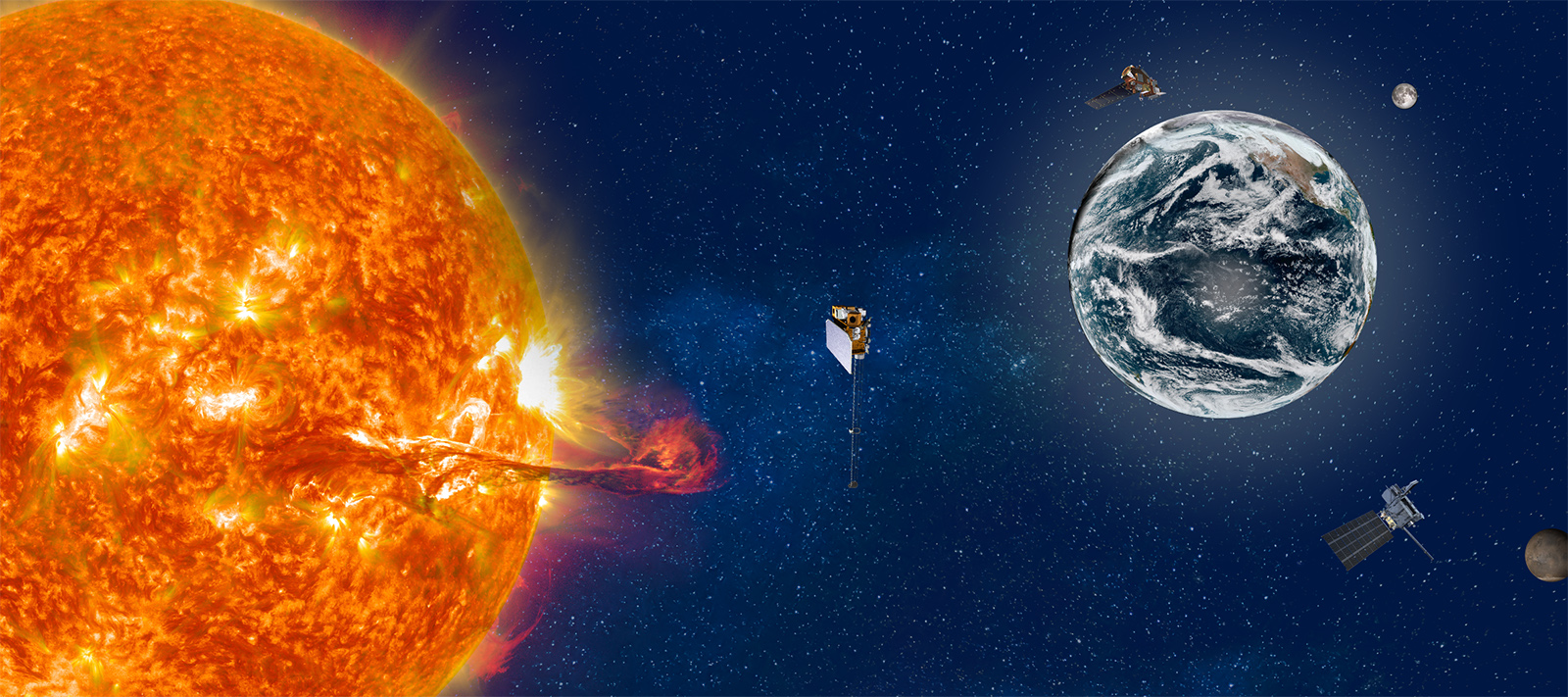
Amendment 22: Heliophysics Flight Opportunities in Research and Technology Final Text and Due Date
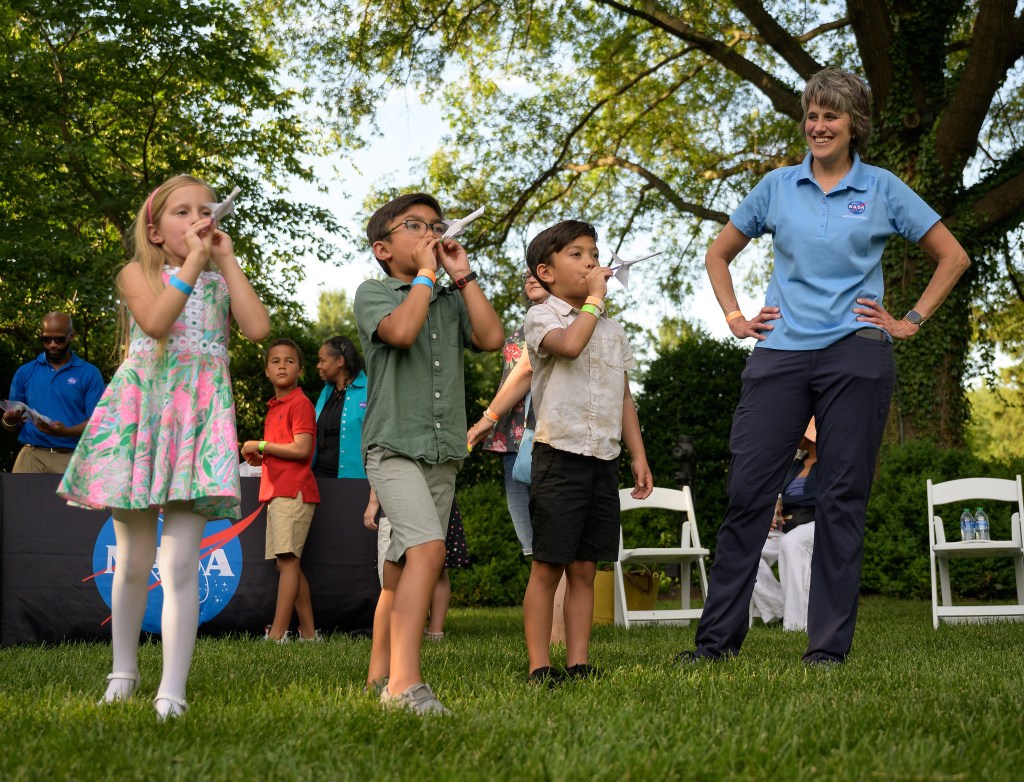
Slow Your Student’s ‘Summer Slide’ and Beat Boredom With NASA STEM

Mission Success: HERA Crew Successfully Completes 45-Day Simulated Journey to Mars
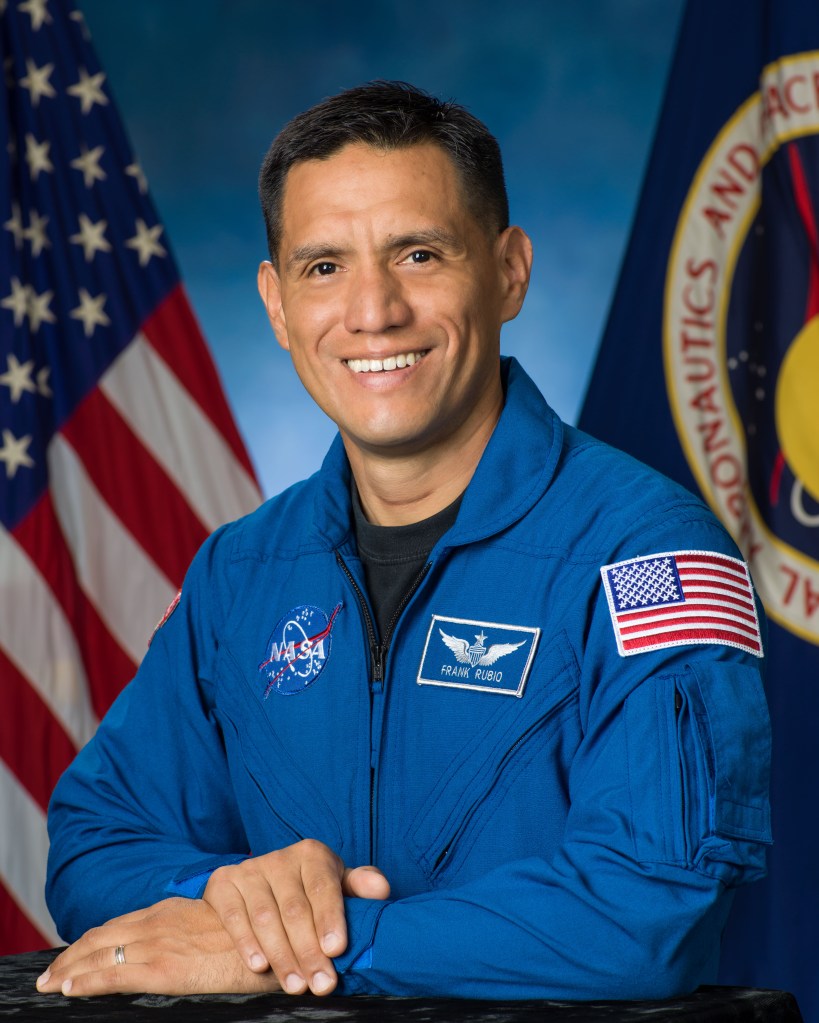
Astronauta de la NASA Frank Rubio
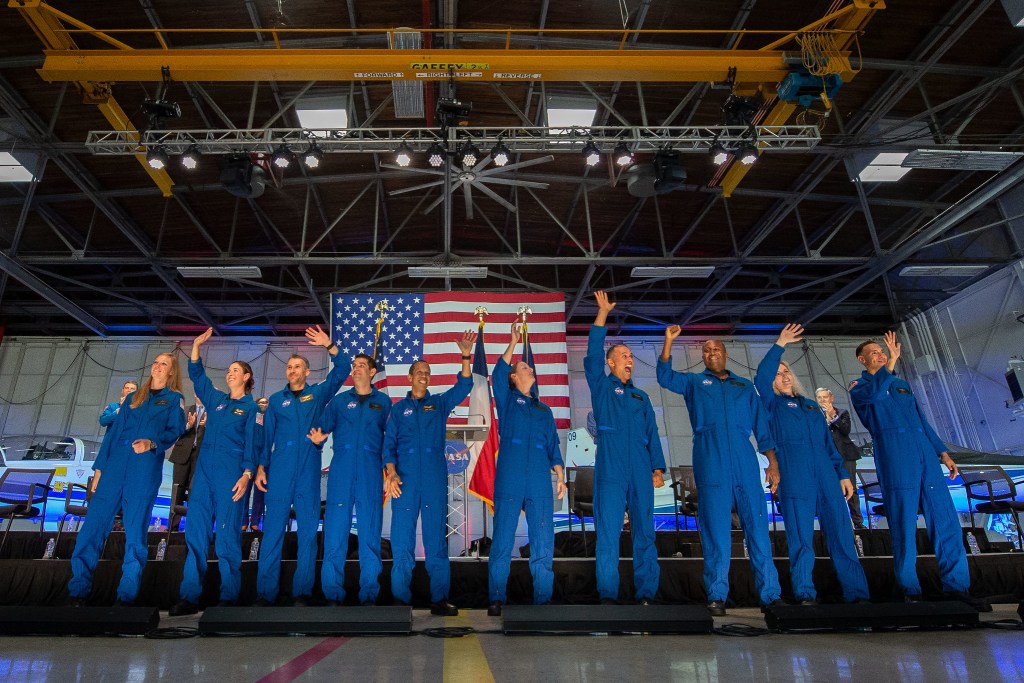
Diez maneras en que los estudiantes pueden prepararse para ser astronautas
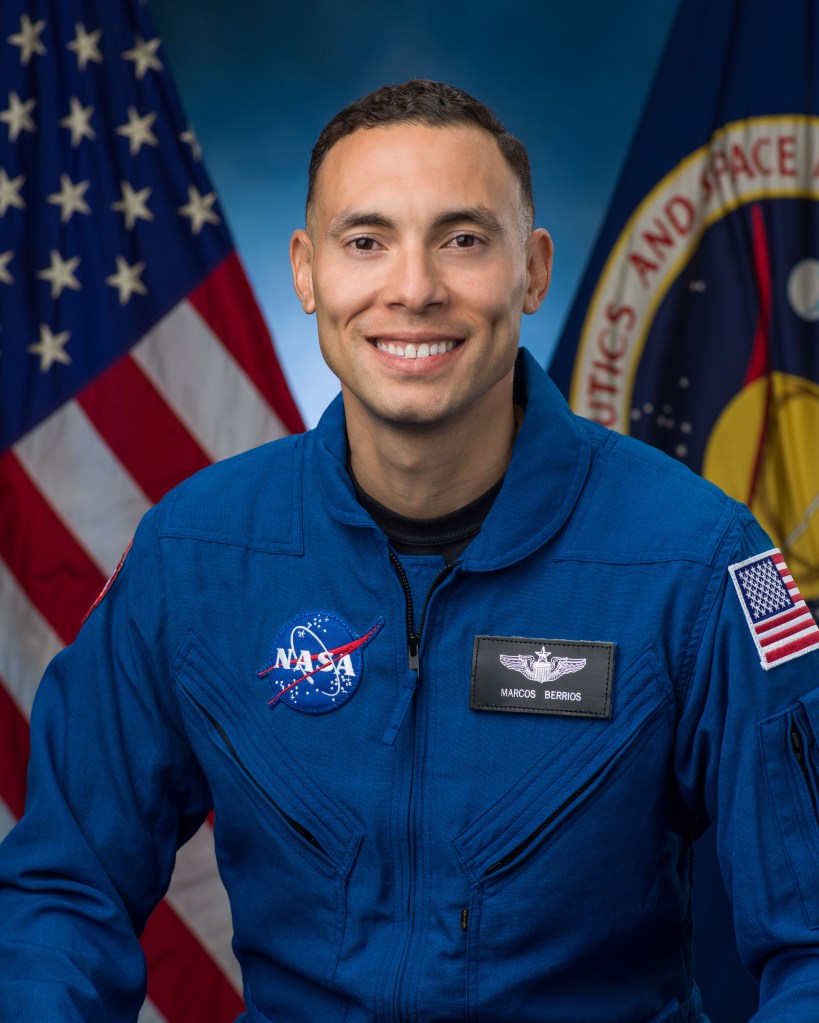
Astronauta de la NASA Marcos Berríos
21 min read
Interview with Xinchuan Huang
Ciara C. Fitzpatrick
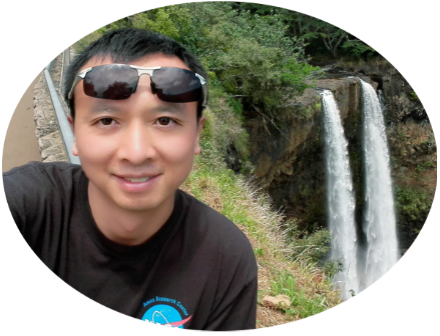
Let’s start with your childhood, where you were born, where you’re from, your young years, your family at the time, what your parents did, and how early it was in your life that you decided you’d like to pursue a career like the one you’re pursuing now?
I was born in a small town in Sichuan, China. It is not far from the famous Emei Mountain, and the beautiful Qingyi river runs through it. At the beginning, I lived with my grandmother’s family in a small village on the riverbank, called “Pond in heaven”. After I left there at four years old, I lived with my parents in Sichuan and Xinjiang provinces, alternatively, as my parents had been working apart. Luckily their reunion came after three years, and finally there was a real “home” for us. My parents were both high school teachers, they worked in the school system opened by a research institute for the children of their employees. It has elementary schools, middle schools, and high schools. That’s where I grew up and received my pre-college education.

Since I was young, my mother has taught me enlightenment and urged my study. While my father was not quite involved in my academics, he valued the importance of reading and cultivated my interest in books. Every time we walked into a bookstore together, I was just purely happy because it simply meant one or two new books were coming home with me. He encouraged me to keep expanding my knowledge and horizons by also subscribing to many educational magazines and newspapers for kids, among which I remember two of my most favorite magazines. Before elementary school it was the “Children’s Science Pictorial”, and in elementary school it was the “Youth Science”. Those magazines started and nurtured my interest in science and the universe.
In middle school, there was an advertisement for a simple and cheap monocular telescope. I told my dad about it and he helped me order one, even though all it could show was the craters on the moon. But I was so excited, I could lay on the cold ground, watching the moon for hours, as if a new world was unfolding in front of me. Seeing how much I enjoyed it, my father later ordered for me the astronomy volume of the Chinese Encyclopedia. It cost 20 Yuan, which was not a small amount at that time. I was so thrilled to have the book. Holding that hardcover book, I felt that I was holding the universe in my arms.
I can imagine!
But most contents in that encyclopedia were still too advanced for me at the time, so I was more obsessed with the colorful photos in the book. Along with my interest in space and the universe, I was also interested in the topics of UFOs and extraterrestrial civilizations. For example, I read a book called “The Mystery of Flying Saucers”, which was a collection of reports and discussions translated from French. In that book, it mentioned the Drake equation for estimating the likelihood of civilizations in the universe. It deeply impressed me. In 2009, after my postdoc at Ames, I had an opportunity to meet with Dr. Drake. He’s the author of the equation and the founder of the SETI Institute. I must say that not everyone has the opportunity or the luck to meet an idol from their childhood and truly chat with him.
Good luck indeed!
However, when I told Dr. Drake that my first time reading about his equation was in a book of UFOs, he laughed and said “(it) was in a wrong story!” (laughs)
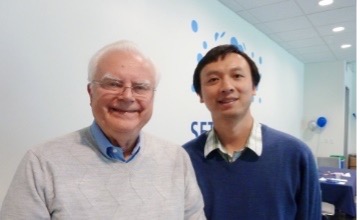
When I graduated from high school, I did consider a major in astronomy, but there were very few undergraduate astronomy majors in China universities. The only few available that year were either not recruiting in Sichuan or in a city I didn’t like. The famous Peking University did have astrophysics major, but each year they only recruited about 10 undergraduate students from the whole country, and few from Sichuan. Otherwise, I could have enrolled there thirty years ago.
Any idea why they didn’t place more emphasis on astronomy? China, as you know, has a strong reputation in space exploration.
There is tradition for astronomy in China, and people know of ancient records and scientists, but it likely wasn’t the focus at that time. The astronomy and astrophysics research of Peking university and other China institutions have expanded significantly in last 30 years.
That’s for sure.
Anyway, I was admitted to the Fudan University in Shanghai, to major in Applied Chemistry II. That’s an interesting name. Usually you see chemistry, applied chemistry, materials chemistry, etc. What does the “II” mean? Previously, it was the Radiochemistry major, but people adjusted its content to keep up with the growth of economy, and to make it easier for their students to find jobs. There was already a major of “Applied Chemistry” in the Chemistry department, so it became “Applied Chemistry II”. My undergraduate thesis was done in the Institute of Laser Chemistry at Fudan, on the UV dissociation of a small organic molecule under cryogenic matrix isolation conditions.
Well, you certainly were well served by both your parents, as they helped direct your focus and your education. I also looked it up because I had not remembered that you came to Ames as a postdoc when I was associated with the NPP program as the Ames representative.
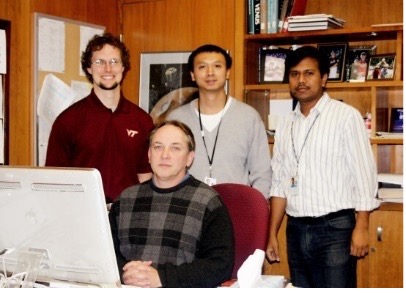
I don’t remember all of them of course as there were quite a few over that period of time, but I hope that was a good experience for you. You were working with Tim Lee as your advisor and I’d known him for a very long time.
I appreciated and enjoyed the opportunity of doing my postdoc at Ames. I had been thinking of other career choices right before Tim sent an email to Joel (my PhD advisor) asking if there was any student suited for a research project at Ames, about ammonia’s Infrared spectrum calculations. The target was to generate a complete IR line list which people can utilize to characterize the NH 3 related celestial environments and eliminate all the NH 3 features from the astronomical observations, such as those in Titan’s atmosphere. It was a very good match to my Ph.D. background on the potential energy surface and vibrational dynamics of water cluster ions.
You had another postdoc before you came to Ames? At Emory University?
Yes, that was more like a one-year extension after the thesis defense, to finish up my Ph.D. projects.
How did you get from China to the United States? Was it because of your educational pursuits?
During my undergraduate study, I had some interest in laser chemistry and spectroscopy. For example, photodissociation products were detected and characterized by their infrared spectrum, and we know the spectroscopic fingerprints of molecules are determined by their nature, or internal properties. After college, I became a graduate student at the Institute of Chemistry, Chinese Academy of Science, in Beijing. Supposedly I should learn how to use a femtosecond laser system to investigate some ultra-fast processes in chemical reactions, but my supervisor left the institute unexpectedly.
So, I applied to some graduate programs in United States, and later enrolled in the chemistry department of Emory University in Atlanta. The admission could be related to my background in laser chemistry labs, but I didn’t continue that path. Instead, I changed to theoretical chemistry and vibrational dynamics studies. But I always admired our colleague experimental spectroscopists working in the laboratories, perhaps because I have myself witnessed how difficult an experimental study could become. It may include sample preparation, optical path platform construction, vacuum pumps, laser tuning, circuit of detectors, hardware interface and software development, etc., so requiring a variety of knowledge and skills from chemistry, physics, to mechanics, electronics, and even materials and computer science. Compared to that, it is relatively simpler to do theoretical spectroscopic studies. But from our perspective, our work still belongs to the laboratory astrophysics. Our lab is set up inside computers, and our equipment and devices are computing programs and algorithms.
Did you come to Emory because of a connection or a contact with them? Or did they just have a good program in what you were studying?
I applied to several graduate programs in the US, and received admissions including Emory, but I had no connections with them before. I chose the physical chemistry graduate program at Emory, for their reputation in both experimental and theoretical research.
So, you applied to several programs and you chose and got admitted to Emory. And then what was your route to Ames? Was it your postdoc? You got a postdoc here and then you stayed?
That’s very straightforward.
Straight and simple.
Did you know Tim at all beforehand? From a conference or something like that?
Not personally, except that he was an expert in Coupled Cluster theory. After Tim contacted my advisor in the summer of 2005, I met him later that year in the ACS meeting at D.C.
You were going to tell us something about the work that you are doing, which I found very complicated. It had to do with something called a “potential energy surface” and some other things which I don’t even know what they are, but let’s go ahead because one of the reasons we asked this question is because we want to know why it is important enough that taxpayers should fund research into it.
Our research focuses on the Infrared and microwave spectrum ranges, provides high quality spectroscopic constants, or highly accurate Infrared line list predictions for small molecules in outer space. Those molecules play important roles in the interstellar medium, atmospheres of solar system objects, like Venus and Titan, and atmospheres of brown dwarfs and exoplanets. The IR spectroscopic constants and line lists will facilitate the detection of those molecules, help characterize the physical conditions of related environments, determine column densities or atmospheric concentrations, and improve the chemistry evolution models. Since a large part of the astronomical research involves spectrum data analysis and modeling, naturally more reliable and more accurate reference data will be needed to better support NASA strategic goals, help maximize the scientific output of various NASA missions, and eventually help us better understand what’s going on in the universe.
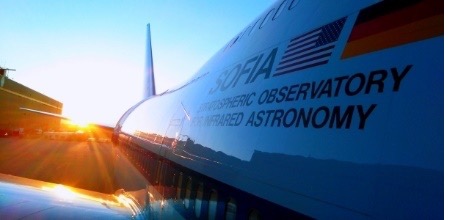
In the last two decades, the generation of more accurate reference data and predictions has required us to combine the advantages of experiments and theories. Our colleagues in Europe adopted similar strategies. For example, the latest Infrared line list we computed for hot carbon dioxide up to 3000 K has several components: high quality ab initio potential energy surface refined using reliable, high resolution experimental data or models, and the best dipole moment surfaces with accuracy already verified by recent highly accurate experiment IR intensities, and the most accurate line positions from the experiment based effective Hamiltonian models. In this way, the spectral line position and intensity accuracy from existing experiment data are integrated with the completeness, reliability and consistency from theoretical predictions. We hope the line list can improve the accuracy of CO 2 analysis and modeling for brown dwarf and hot exoplanet atmospheres, which include, but not limited to the recent CO 2 discoveries that JWST made on exoplanets.
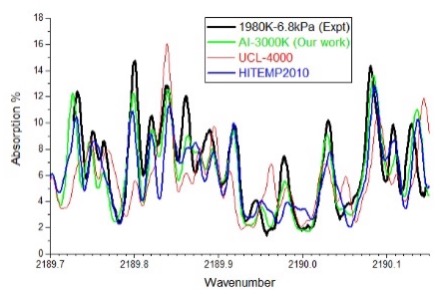
On the other hand, like I mentioned earlier, some molecules, like methyl cyanide, SO 2 , and ammonia, generate a plethora of spectral lines, appearing like wild grasses. That’s why some molecules were called “weeds”. They’re the “weeds” in the field of spectrum and may overshadow other important signals. Once I looked at a small segment of SOFIA EXES spectrum at 20 mm. Although I already knew it contained hundreds of sulfur dioxide bending mode transitions, I did not expect that so many very weak oscillations and tiny bumps in the observed spectrum could be excellently explained and reproduced until I ran the simulations by myself using SO 2 line lists. Without a reliable and complete line list, many weak features may go unnoticed and treated as noises. But when you have a good line list, you can identify all the features of a specific molecule, then try to remove them, like removing weeds, so more interesting features or molecules can be found. We may call them the “flowers”. From this angle, we are like farmers in the spectroscopy field, or treasure hunters in the jungle of spectrum.
That’s a good way of putting it. And this leads to a greater understanding of what elements of the NASA mission? How does this fit in with what NASA is trying to accomplish, which could be just exploration, or the search for life, or some of the other great questions that NASA is trying to help answer?
There are several potential impacts from the basic scientific research we have been doing. One is to identify those molecules for their existence in the universe, where they are, and how many they are. Second is to figure out what their environment looks like, e.g., the pressure and temperature. An accurate reference line list can help to extract that information from observed spectrum data. The third impact is about some potential biosignature molecules for habitable exoplanets. Like the one we worked on recently, the nitrous oxide or laughing gas, N 2 O, it is one of those molecules contributing to the transit spectrum of Earth. Another impact is on chemical evolution models. Because our reliable predictions have very high consistency across isotopologues, higher than experiments, we can help to determine more accurate isotopic ratios and evolution history in outer space. In summary, and in the larger picture, we are contributing to the exploration of the universe and the search for habitable planets by providing basic reference data and tools for all NASA missions related to Infrared astronomy, from past Herschel, SOFIA, to JWST, and future ARIEL and other missions.
You mentioned biosignatures, which caught my attention because we’re hoping to find some evidence that we’re not alone in the universe, that there is other biology going on somewhere out there. Almost all of our research focuses on trying to address that, at some level. And it has a lot of popular support, taxpayer support, because they want the answer to that question perhaps most of all.
The IR spectra based astronomical research involves many models and datasets from different sources, like the spectra modeling on the JWST observations of exoplanet atmospheres. Every piece of work has its own uncertainties, which will add up model by model, database by database. A recent study published in Nature Astronomy revealed that the abundance errors resulting from the opacity inaccuracies can be about one order of magnitude larger than those brought on from JWST-quality observations. This is a bottleneck. From this perspective, our study can help to reduce, or to minimize those uncertainties and errors associated with the opacity data. Compared to experimental measurements under certain conditions, we are trying to provide a complete picture for molecules in the full range of IR and MW spectra. The computed line lists can be used to generate more reliable opacity data at different target temperatures. Having more accurate opacity data with uncertainty reduced or minimized, scientists can determine more accurate properties for exoplanets and other objects in the universe.
Have there been any surprising or breakthrough findings or discoveries or something not expected that has come from your work?
Not expected? Let me think. We should be careful about the claims on the strengths and limitations of our work. On one side we should have enough confidence, but every molecule is unique, we also need to properly estimate the limitation of our line list predictions. With the synergy between experimental data and high-quality theoretical calculations, many improvements actually can be expected. If we know clearly what we can do and what our limits are, they are not real surprises. Some predictions may look surprising, but they need verifications from future experiments. If verified, the agreement is still expected. If rejected, it means something we need to explain or fix, not real breakthrough or findings.
If we really want to talk about “surprises”, I can name two kinds of them. One is that we find surprisingly good agreement or high accuracy verification between predictions and experiments. For example, our room temperature CO 2 line list. The IR intensity agreement with the best experiment measurement has reached the level of sub-half percent, for both accuracy and uncertainty, and towards 0.1 %, or permille level, 1‰. It was the best level ever achieved for CO 2 . That’s kind of a surprise because we were targeting a major upgrade, we knew we were doing better, but we didn’t know the improvements would be so good. That is a good surprise, but there could also be an opposite kind of surprise: a similar molecule or band, similar studies following the same track, so we had assumed it should come out as satisfactory as other molecules or bands, but it did not work out. Then we must figure out what’s going on, what we forgot or missed, or what’s the difference. For example, is that due to some unknown electronic state interference, sensitive resonances, potential defects in potential energy surface, or program bugs, etc.?
That is the science part of it.
Those are really the surprises.
You’re a very impressive and accomplished NASA research scientist, that’s obvious. And you’ve pursued that from youth, really, that line of work. Have you ever given any thought to, if you weren’t doing what you’re doing now, is there another dream job that you might like to have pursued if you had gone another way?
When people talk about a dream job, it usually means something that cannot be realized, except in our dreams. Maybe a contractor scientist without the need to worry about funding?
But still a scientist? OK, that’s good too. But what things would interest you if you couldn’t be a research scientist anymore? This is just to get into your personality and find out more about you.
Oh, if I forget the astronomer or scientist dream from childhood? My dream job has changed several times. Right now, I think it would be interesting to be a local tourist guide.
It would indeed. I like that.
It is also good for me, not only helps to get familiar with my neighborhood, community, the natural environment, but also gives me some good exercise! (laughs)
What advice might you give to a young aspiring student who would like to have a career like yours?
When I graduated from high school and went to Fudan University to study chemistry, I had never thought that one day I could still have the opportunity to work for NASA and become a scientist at SETI, Search for Extraterrestrial Intelligence Institute. I also met Dr. Drake and talked to him. In a way this was already infinitely close to my childhood dreams. In this life, I could not become a real astronomer, the most I can do is some basic and auxiliary research work in the field of astrochemistry and theoretical spectroscopy. But looking back from my childhood and my college, I can’t help thinking of a phrase that I read from Steve Jobs, the Apple founder. What he said was something like: “many seemingly unrelated and even useless points in your life may someday eventually connect together to form a path to your dreams. Every piece of past experience will have its meaning and function and role in your career. It Is only then that we can realize their meaning and their role” . This statement roughly applies to me, though of course my experience has been much simpler.
I like that quote because we don’t always realize as we’re living and moving forward, the significance of various things that happen. Something that’s just a coincidence can have quite an impact on one’s life or direction.
Yes. The universe is infinite, and all the Earth’s science and technology can be found useful in space explorations, sooner or later. If you are interested in the universe, in space sciences, but at the moment you cannot see how your specialty skills or major can be connected to space, don’t worry and don’t give up. Work hard on what you are doing now, whether it’s learning, research, or work, so that when the opportunity comes, you will be ready.
My second piece of advice was borrowed from Professor Yuan-Tseh Lee, a Nobel Prize winner in Chemistry. About 20 years ago I met him at a conference. At that time, people were talking about innovations everywhere, but I could not find out how to innovate at all, no matter where I looked, so I asked him for advice. Professor Lee said innovation is not like that; innovation comes from years of continuous accumulation and improvements. He said first you need to get very familiar with what you have at hand, get to the bottom, fully understand principles and techniques of what you are doing, and then try to make improvements. There is always room for improvements, and even a tiny improvement will count and will help. Keep improving, a little bit here, a little bit there. Over time, this will eventually lead to real innovation and breakthroughs. My understanding or take away from his replies, is just like the ancient Chinese saying: “ No accumulation of steps, no distance to thousands of miles; no accumulation of small streams, there will be no rivers and seas.” That’s it.
Very good answer, thought provoking and true. Thank you for sharing that. Would you like to tell us anything about your family? Are you married? Do you have children?
Yeah, I’m married, and my wife was also from the Chemistry Department of Emory. But she works in the field of organic chemistry, which I could never figure out since my college years. (laughs) And we have two daughters, one in elementary school and the older one in high school. Our daily lives are kind of routine. Like driving the kids to school, back home doing my work, sometimes accompanying kids doing their homework, taking them to extra-curricular activities, cooking, etc.
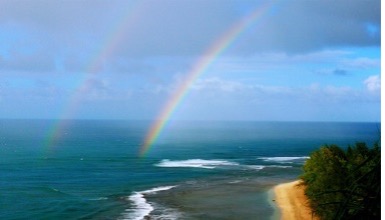
We have a favorite travel destination, the Kauai Island in Hawai’i. Our first visit to Kauai was in 2007, and we really, really like it. I went there more often than my family: I have been there seven times! (laughs) I enjoyed looking out to the west of Pacific Ocean at the end of the Waimea canyon and walking on the Ke’e beach at the east end of the Na Pali Trail. If there is a chance, I may think about living there after retirement.
You could do worse than that! In fact, that might be the answer to the next question, which is: with all your work and family responsibilities, and everything that you are involved in, what do you do for fun?
My interests include reading, like history, literature, and sci-fi books. I like sci-fi fictions and TV shows, such as “The Expanse” series, “The Peripheral” from last year, and the “Three-Body” TV series from China. For fun, I like Chinese Crosstalk, which is a comic dialogue between two people. Every year I also like to pick cherries and nectarines from farms in Brentwood.
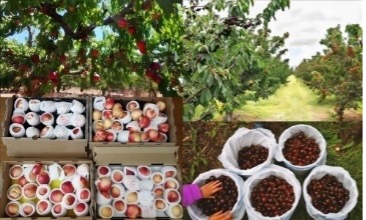
Because I use my phone or camera like a recorder, I took too many photos here and there, far more than truly memorable moments. Those photos are a big headache when compiling a family yearbook. After our first child was born, it’s great fun to make annual photobooks for each year.
It’s wonderful that you do that. That will pay dividends in the future, for sure.
Before the pandemic, I also liked to have lunch together with a few colleagues every couple of weeks in some Chinese restaurants nearby, and most of the time we order spicy Chinese food.
You like that? I like that too, although not too spicy! What has been a prime inspiration for you in your life? Something that motivated you to accomplish all that you’ve accomplished so far. Is there a person that you particularly liked? Drake, for example, and his work, that helped to inspire you going forward?
A major motivation has been my curiosity about nature and stars. For inspirational figures, there were many – yes, Dr. Drake was one, because his work inspired people to think more seriously about the relation between life and the universe, and motivated me to make my own contributions. There was also inspiration from Professor Lee. After he won the chemistry Nobel Prize in 1986, there was a lot of laser chemistry related research going on in China. That’s what inspired me too, and why I asked him for advice.
This has been wonderful. I’ve learned a lot about you and that is the whole purpose of this series. Thank you very much. We’ve enjoyed chatting with you.
Thank you. It is great to have this opportunity to chat with you, I enjoyed it too.

IMAGES
VIDEO
COMMENTS
MIT's DSpace contains more than 58,000 theses completed at MIT dating as far back as the mid 1800's. Theses in this collection have been scanned by the MIT Libraries or submitted in electronic format by thesis authors. Since 2004 all new Masters and Ph.D. theses are scanned and added to this collection after degrees are awarded.
They will stress the importance of structure, substance and style. They will urge you to write down your methodology and results first, then progress to the literature review, introduction and conclusions and to write the summary or abstract last. To write clearly and directly with the reader's expectations always in mind.
Although educational programs, conventions and thesis requirements vary wildly, I hope to offer some common guidelines for any student currently working on a Data Science thesis. The article offers five guidance points, but may effectively be summarized in a single line: "Write for your reader, not for yourself."
The Stanford Data Science Undergraduate Research Pathways program is an 8-week full-time research experience designed to provide students at institutions without access to research opportunities the chance to conduct a research project under the supervision of both a mentor and faculty member. This is an in-person experience held at Stanford ...
Data Science [ undergraduate program ... science to save some time from elective courses and devise a graduation schedule within six quarters by exercising the thesis option that enables them to apply data science techniques to the applied field of their original expertise, thus reducing the course load in the elective series. ...
BSc/MSc Thesis. Our research group offers various interesting topics for a BSc or MSc thesis, the latter both in Computer Science and Scientific Computing. These topics are typically closely related to ongoing research projects (see our Research Page and Publications ). Below, we outline the basic procedure you should follow when planning to do ...
Data Science-Related Research Topics. Developing machine learning models for real-time fraud detection in online transactions. The use of big data analytics in predicting and managing urban traffic flow. Investigating the effectiveness of data mining techniques in identifying early signs of mental health issues from social media usage.
An honors thesis provides an opportunity for eligible students to carry out faculty-supervised research in their senior year. The application process and requirements for the Statistics, Data Science, and Informatics honors programs are described on the department website. Students are encouraged to contribute their thesis to the archive of honors theses at the University of Michigan Library.
If you are an undergraduate honors student interested in submitting your thesis to DukeSpace, ... data, data analysis, data science, data sets, data visualization, sports analytics, statistical science, statistics. Contact Us. 411 Chapel Drive Durham, NC 27708 (919) 660-5870
Abstract. To drive progress in the field of data science, we propose 10 challenge areas for the research community to pursue. Since data science is broad, with methods drawing from computer science, statistics, and other disciplines, and with applications appearing in all sectors, these challenge areas speak to the breadth of issues spanning ...
In this article, we have listed 10 such research and thesis topic ideas to take up as data science projects in 2022. Handling practical video analytics in a distributed cloud: With increased dependency on the internet, sharing videos has become a mode of data and information exchange. The role of the implementation of the Internet of Things ...
Data Science Thesis Continuation. (4 Hours) Focuses on student continuing to prepare an undergraduate thesis under faculty supervision. DS 5010. Introduction to Programming for Data Science. (4 Hours) Offers an introductory course on fundamentals of programming and data structures. Covers lists, arrays, trees, hash tables, etc.; program design ...
To earn the Master of Science in Data Science, students must complete 12 courses. This requires students to be on campus for at least 3 semesters (one and a half academic years). Some students will choose to extend their studies for a fourth semester to take additional courses or complete a master's thesis research project.
Bachelor and Master Thesis. We offer a variety of cutting-edge and exciting research topics for Bachelor's and Master's theses. We cover a wide range of topics from Data Science, Natural Language Processing, Argument Mining, the Use of AI in Business, Ethics in AI and Multimodal AI. We are always open to suggestions for your own topics, so ...
This short guide is intended to give you a brief guideline on how to organise and conduct your thesis. This guide covers both, a master and a bachelor thesis. Managing your Thesis. A thesis is a complex task, similar to a software project has to be managed properly. It can be seen to have four stages, which are iterated several time.
The Harvard University Archives' collection of theses, dissertations, and prize papers document the wide range of academic research undertaken by Harvard students over the course of the University's history.. Beyond their value as pieces of original research, these collections document the history of American higher education, chronicling both the growth of Harvard as a major research ...
Apache Spark. Python Blaze. Technologies for constructing data processing pipelines. Luigi. Cloud analytics platforms that make machine learning "available to the masses". Amazon Machine Learning. Microsoft Azure Machine Learning. NB: The examples provided for a given topic above are not intended to be comprehensive.
Overview. A project-based seminar course in which students work individually or in small teams to tackle data science and machine learning problems, working with real-world datasets. The course emphasizes critical thinking about experiments and large dataset analysis and the ability to clearly communicate one's research.
Data Science. The listing of a course description here does not guarantee a course's being offered in a particular term. ... Undergraduate Prerequisites: CASMA581 or CDSDS122 AND CDSDS320 ... explore lab settings and industrial collaborations that may be relevant to their thesis work; (2) experience the diversity of applied and in-the-field ...
2015. 2014. 2013. 2012. 2011. 2010. 2009. 2008. This list of recent dissertation topics shows the range of research areas that our students are working on.
Thesis. A thesis is an academic-focused research project with broader applicability. A thesis is more appropriate if: you want to get a PhD or other advanced degree and want the experience of the research process and writing for publication; you want to work individually with a specific faculty member who serves as your thesis adviser
The Data Science Lab offers a list of suggested diploma theses to undergraduate and postgraduate students in subjects relative to the research interests of the laboratory. For more information, contact the person in charge of each diploma thesis. 2023-24 theses proposals and preparation guidelines. Selected theses:
How to search for Harvard dissertations. DASH, Digital Access to Scholarship at Harvard, is the university's central, open-access repository for the scholarly output of faculty and the broader research community at Harvard.Most Ph.D. dissertations submitted from March 2012 forward are available online in DASH.; Check HOLLIS, the Library Catalog, and refine your results by using the Advanced ...
The data, code and thesis for my own undergraduate thesis in the "FYP: Design and Implementation (COMP30910)" module. ... My computer science undergraduate thesis for a degree at UFSC. neural-networks undergraduate-thesis seismic-data Updated Dec 8, 2022; Jupyter Notebook;
My undergraduate thesis was done in the Institute of Laser Chemistry at Fudan, on the UV dissociation of a small organic molecule under cryogenic matrix isolation conditions. ... Since a large part of the astronomical research involves spectrum data analysis and modeling, naturally more reliable and more accurate reference data will be needed ...