When you choose to publish with PLOS, your research makes an impact. Make your work accessible to all, without restrictions, and accelerate scientific discovery with options like preprints and published peer review that make your work more Open.
- PLOS Biology
- PLOS Climate
- PLOS Complex Systems
- PLOS Computational Biology
- PLOS Digital Health
- PLOS Genetics
- PLOS Global Public Health
- PLOS Medicine
- PLOS Mental Health
- PLOS Neglected Tropical Diseases
- PLOS Pathogens
- PLOS Sustainability and Transformation
- PLOS Collections
- How to Write Discussions and Conclusions

The discussion section contains the results and outcomes of a study. An effective discussion informs readers what can be learned from your experiment and provides context for the results.

What makes an effective discussion?
When you’re ready to write your discussion, you’ve already introduced the purpose of your study and provided an in-depth description of the methodology. The discussion informs readers about the larger implications of your study based on the results. Highlighting these implications while not overstating the findings can be challenging, especially when you’re submitting to a journal that selects articles based on novelty or potential impact. Regardless of what journal you are submitting to, the discussion section always serves the same purpose: concluding what your study results actually mean.
A successful discussion section puts your findings in context. It should include:
- the results of your research,
- a discussion of related research, and
- a comparison between your results and initial hypothesis.
Tip: Not all journals share the same naming conventions.
You can apply the advice in this article to the conclusion, results or discussion sections of your manuscript.
Our Early Career Researcher community tells us that the conclusion is often considered the most difficult aspect of a manuscript to write. To help, this guide provides questions to ask yourself, a basic structure to model your discussion off of and examples from published manuscripts.

Questions to ask yourself:
- Was my hypothesis correct?
- If my hypothesis is partially correct or entirely different, what can be learned from the results?
- How do the conclusions reshape or add onto the existing knowledge in the field? What does previous research say about the topic?
- Why are the results important or relevant to your audience? Do they add further evidence to a scientific consensus or disprove prior studies?
- How can future research build on these observations? What are the key experiments that must be done?
- What is the “take-home” message you want your reader to leave with?
How to structure a discussion
Trying to fit a complete discussion into a single paragraph can add unnecessary stress to the writing process. If possible, you’ll want to give yourself two or three paragraphs to give the reader a comprehensive understanding of your study as a whole. Here’s one way to structure an effective discussion:
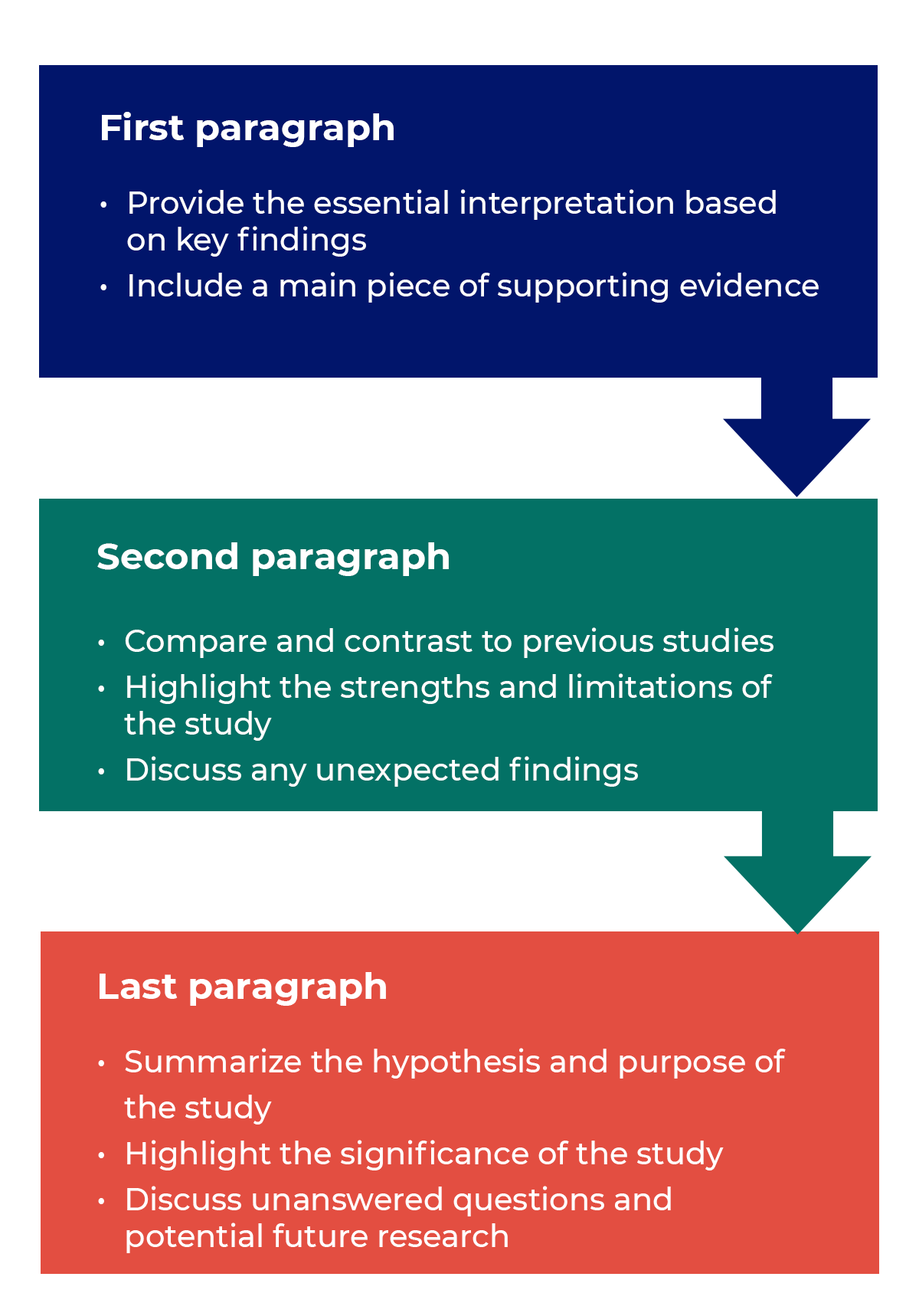
Writing Tips
While the above sections can help you brainstorm and structure your discussion, there are many common mistakes that writers revert to when having difficulties with their paper. Writing a discussion can be a delicate balance between summarizing your results, providing proper context for your research and avoiding introducing new information. Remember that your paper should be both confident and honest about the results!

- Read the journal’s guidelines on the discussion and conclusion sections. If possible, learn about the guidelines before writing the discussion to ensure you’re writing to meet their expectations.
- Begin with a clear statement of the principal findings. This will reinforce the main take-away for the reader and set up the rest of the discussion.
- Explain why the outcomes of your study are important to the reader. Discuss the implications of your findings realistically based on previous literature, highlighting both the strengths and limitations of the research.
- State whether the results prove or disprove your hypothesis. If your hypothesis was disproved, what might be the reasons?
- Introduce new or expanded ways to think about the research question. Indicate what next steps can be taken to further pursue any unresolved questions.
- If dealing with a contemporary or ongoing problem, such as climate change, discuss possible consequences if the problem is avoided.
- Be concise. Adding unnecessary detail can distract from the main findings.
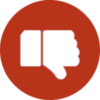
Don’t
- Rewrite your abstract. Statements with “we investigated” or “we studied” generally do not belong in the discussion.
- Include new arguments or evidence not previously discussed. Necessary information and evidence should be introduced in the main body of the paper.
- Apologize. Even if your research contains significant limitations, don’t undermine your authority by including statements that doubt your methodology or execution.
- Shy away from speaking on limitations or negative results. Including limitations and negative results will give readers a complete understanding of the presented research. Potential limitations include sources of potential bias, threats to internal or external validity, barriers to implementing an intervention and other issues inherent to the study design.
- Overstate the importance of your findings. Making grand statements about how a study will fully resolve large questions can lead readers to doubt the success of the research.
Snippets of Effective Discussions:
Consumer-based actions to reduce plastic pollution in rivers: A multi-criteria decision analysis approach
Identifying reliable indicators of fitness in polar bears
- How to Write a Great Title
- How to Write an Abstract
- How to Write Your Methods
- How to Report Statistics
- How to Edit Your Work
The contents of the Peer Review Center are also available as a live, interactive training session, complete with slides, talking points, and activities. …
The contents of the Writing Center are also available as a live, interactive training session, complete with slides, talking points, and activities. …
There’s a lot to consider when deciding where to submit your work. Learn how to choose a journal that will help your study reach its audience, while reflecting your values as a researcher…
- USC Libraries
- Research Guides
Organizing Your Social Sciences Research Paper
- 8. The Discussion
- Purpose of Guide
- Design Flaws to Avoid
- Independent and Dependent Variables
- Glossary of Research Terms
- Reading Research Effectively
- Narrowing a Topic Idea
- Broadening a Topic Idea
- Extending the Timeliness of a Topic Idea
- Academic Writing Style
- Applying Critical Thinking
- Choosing a Title
- Making an Outline
- Paragraph Development
- Research Process Video Series
- Executive Summary
- The C.A.R.S. Model
- Background Information
- The Research Problem/Question
- Theoretical Framework
- Citation Tracking
- Content Alert Services
- Evaluating Sources
- Primary Sources
- Secondary Sources
- Tiertiary Sources
- Scholarly vs. Popular Publications
- Qualitative Methods
- Quantitative Methods
- Insiderness
- Using Non-Textual Elements
- Limitations of the Study
- Common Grammar Mistakes
- Writing Concisely
- Avoiding Plagiarism
- Footnotes or Endnotes?
- Further Readings
- Generative AI and Writing
- USC Libraries Tutorials and Other Guides
- Bibliography
The purpose of the discussion section is to interpret and describe the significance of your findings in relation to what was already known about the research problem being investigated and to explain any new understanding or insights that emerged as a result of your research. The discussion will always connect to the introduction by way of the research questions or hypotheses you posed and the literature you reviewed, but the discussion does not simply repeat or rearrange the first parts of your paper; the discussion clearly explains how your study advanced the reader's understanding of the research problem from where you left them at the end of your review of prior research.
Annesley, Thomas M. “The Discussion Section: Your Closing Argument.” Clinical Chemistry 56 (November 2010): 1671-1674; Peacock, Matthew. “Communicative Moves in the Discussion Section of Research Articles.” System 30 (December 2002): 479-497.
Importance of a Good Discussion
The discussion section is often considered the most important part of your research paper because it:
- Most effectively demonstrates your ability as a researcher to think critically about an issue, to develop creative solutions to problems based upon a logical synthesis of the findings, and to formulate a deeper, more profound understanding of the research problem under investigation;
- Presents the underlying meaning of your research, notes possible implications in other areas of study, and explores possible improvements that can be made in order to further develop the concerns of your research;
- Highlights the importance of your study and how it can contribute to understanding the research problem within the field of study;
- Presents how the findings from your study revealed and helped fill gaps in the literature that had not been previously exposed or adequately described; and,
- Engages the reader in thinking critically about issues based on an evidence-based interpretation of findings; it is not governed strictly by objective reporting of information.
Annesley Thomas M. “The Discussion Section: Your Closing Argument.” Clinical Chemistry 56 (November 2010): 1671-1674; Bitchener, John and Helen Basturkmen. “Perceptions of the Difficulties of Postgraduate L2 Thesis Students Writing the Discussion Section.” Journal of English for Academic Purposes 5 (January 2006): 4-18; Kretchmer, Paul. Fourteen Steps to Writing an Effective Discussion Section. San Francisco Edit, 2003-2008.
Structure and Writing Style
I. General Rules
These are the general rules you should adopt when composing your discussion of the results :
- Do not be verbose or repetitive; be concise and make your points clearly
- Avoid the use of jargon or undefined technical language
- Follow a logical stream of thought; in general, interpret and discuss the significance of your findings in the same sequence you described them in your results section [a notable exception is to begin by highlighting an unexpected result or a finding that can grab the reader's attention]
- Use the present verb tense, especially for established facts; however, refer to specific works or prior studies in the past tense
- If needed, use subheadings to help organize your discussion or to categorize your interpretations into themes
II. The Content
The content of the discussion section of your paper most often includes :
- Explanation of results : Comment on whether or not the results were expected for each set of findings; go into greater depth to explain findings that were unexpected or especially profound. If appropriate, note any unusual or unanticipated patterns or trends that emerged from your results and explain their meaning in relation to the research problem.
- References to previous research : Either compare your results with the findings from other studies or use the studies to support a claim. This can include re-visiting key sources already cited in your literature review section, or, save them to cite later in the discussion section if they are more important to compare with your results instead of being a part of the general literature review of prior research used to provide context and background information. Note that you can make this decision to highlight specific studies after you have begun writing the discussion section.
- Deduction : A claim for how the results can be applied more generally. For example, describing lessons learned, proposing recommendations that can help improve a situation, or highlighting best practices.
- Hypothesis : A more general claim or possible conclusion arising from the results [which may be proved or disproved in subsequent research]. This can be framed as new research questions that emerged as a consequence of your analysis.
III. Organization and Structure
Keep the following sequential points in mind as you organize and write the discussion section of your paper:
- Think of your discussion as an inverted pyramid. Organize the discussion from the general to the specific, linking your findings to the literature, then to theory, then to practice [if appropriate].
- Use the same key terms, narrative style, and verb tense [present] that you used when describing the research problem in your introduction.
- Begin by briefly re-stating the research problem you were investigating and answer all of the research questions underpinning the problem that you posed in the introduction.
- Describe the patterns, principles, and relationships shown by each major findings and place them in proper perspective. The sequence of this information is important; first state the answer, then the relevant results, then cite the work of others. If appropriate, refer the reader to a figure or table to help enhance the interpretation of the data [either within the text or as an appendix].
- Regardless of where it's mentioned, a good discussion section includes analysis of any unexpected findings. This part of the discussion should begin with a description of the unanticipated finding, followed by a brief interpretation as to why you believe it appeared and, if necessary, its possible significance in relation to the overall study. If more than one unexpected finding emerged during the study, describe each of them in the order they appeared as you gathered or analyzed the data. As noted, the exception to discussing findings in the same order you described them in the results section would be to begin by highlighting the implications of a particularly unexpected or significant finding that emerged from the study, followed by a discussion of the remaining findings.
- Before concluding the discussion, identify potential limitations and weaknesses if you do not plan to do so in the conclusion of the paper. Comment on their relative importance in relation to your overall interpretation of the results and, if necessary, note how they may affect the validity of your findings. Avoid using an apologetic tone; however, be honest and self-critical [e.g., in retrospect, had you included a particular question in a survey instrument, additional data could have been revealed].
- The discussion section should end with a concise summary of the principal implications of the findings regardless of their significance. Give a brief explanation about why you believe the findings and conclusions of your study are important and how they support broader knowledge or understanding of the research problem. This can be followed by any recommendations for further research. However, do not offer recommendations which could have been easily addressed within the study. This would demonstrate to the reader that you have inadequately examined and interpreted the data.
IV. Overall Objectives
The objectives of your discussion section should include the following: I. Reiterate the Research Problem/State the Major Findings
Briefly reiterate the research problem or problems you are investigating and the methods you used to investigate them, then move quickly to describe the major findings of the study. You should write a direct, declarative, and succinct proclamation of the study results, usually in one paragraph.
II. Explain the Meaning of the Findings and Why They are Important
No one has thought as long and hard about your study as you have. Systematically explain the underlying meaning of your findings and state why you believe they are significant. After reading the discussion section, you want the reader to think critically about the results and why they are important. You don’t want to force the reader to go through the paper multiple times to figure out what it all means. If applicable, begin this part of the section by repeating what you consider to be your most significant or unanticipated finding first, then systematically review each finding. Otherwise, follow the general order you reported the findings presented in the results section.
III. Relate the Findings to Similar Studies
No study in the social sciences is so novel or possesses such a restricted focus that it has absolutely no relation to previously published research. The discussion section should relate your results to those found in other studies, particularly if questions raised from prior studies served as the motivation for your research. This is important because comparing and contrasting the findings of other studies helps to support the overall importance of your results and it highlights how and in what ways your study differs from other research about the topic. Note that any significant or unanticipated finding is often because there was no prior research to indicate the finding could occur. If there is prior research to indicate this, you need to explain why it was significant or unanticipated. IV. Consider Alternative Explanations of the Findings
It is important to remember that the purpose of research in the social sciences is to discover and not to prove . When writing the discussion section, you should carefully consider all possible explanations for the study results, rather than just those that fit your hypothesis or prior assumptions and biases. This is especially important when describing the discovery of significant or unanticipated findings.
V. Acknowledge the Study’s Limitations
It is far better for you to identify and acknowledge your study’s limitations than to have them pointed out by your professor! Note any unanswered questions or issues your study could not address and describe the generalizability of your results to other situations. If a limitation is applicable to the method chosen to gather information, then describe in detail the problems you encountered and why. VI. Make Suggestions for Further Research
You may choose to conclude the discussion section by making suggestions for further research [as opposed to offering suggestions in the conclusion of your paper]. Although your study can offer important insights about the research problem, this is where you can address other questions related to the problem that remain unanswered or highlight hidden issues that were revealed as a result of conducting your research. You should frame your suggestions by linking the need for further research to the limitations of your study [e.g., in future studies, the survey instrument should include more questions that ask..."] or linking to critical issues revealed from the data that were not considered initially in your research.
NOTE: Besides the literature review section, the preponderance of references to sources is usually found in the discussion section . A few historical references may be helpful for perspective, but most of the references should be relatively recent and included to aid in the interpretation of your results, to support the significance of a finding, and/or to place a finding within a particular context. If a study that you cited does not support your findings, don't ignore it--clearly explain why your research findings differ from theirs.
V. Problems to Avoid
- Do not waste time restating your results . Should you need to remind the reader of a finding to be discussed, use "bridge sentences" that relate the result to the interpretation. An example would be: “In the case of determining available housing to single women with children in rural areas of Texas, the findings suggest that access to good schools is important...," then move on to further explaining this finding and its implications.
- As noted, recommendations for further research can be included in either the discussion or conclusion of your paper, but do not repeat your recommendations in the both sections. Think about the overall narrative flow of your paper to determine where best to locate this information. However, if your findings raise a lot of new questions or issues, consider including suggestions for further research in the discussion section.
- Do not introduce new results in the discussion section. Be wary of mistaking the reiteration of a specific finding for an interpretation because it may confuse the reader. The description of findings [results section] and the interpretation of their significance [discussion section] should be distinct parts of your paper. If you choose to combine the results section and the discussion section into a single narrative, you must be clear in how you report the information discovered and your own interpretation of each finding. This approach is not recommended if you lack experience writing college-level research papers.
- Use of the first person pronoun is generally acceptable. Using first person singular pronouns can help emphasize a point or illustrate a contrasting finding. However, keep in mind that too much use of the first person can actually distract the reader from the main points [i.e., I know you're telling me this--just tell me!].
Analyzing vs. Summarizing. Department of English Writing Guide. George Mason University; Discussion. The Structure, Format, Content, and Style of a Journal-Style Scientific Paper. Department of Biology. Bates College; Hess, Dean R. "How to Write an Effective Discussion." Respiratory Care 49 (October 2004); Kretchmer, Paul. Fourteen Steps to Writing to Writing an Effective Discussion Section. San Francisco Edit, 2003-2008; The Lab Report. University College Writing Centre. University of Toronto; Sauaia, A. et al. "The Anatomy of an Article: The Discussion Section: "How Does the Article I Read Today Change What I Will Recommend to my Patients Tomorrow?” The Journal of Trauma and Acute Care Surgery 74 (June 2013): 1599-1602; Research Limitations & Future Research . Lund Research Ltd., 2012; Summary: Using it Wisely. The Writing Center. University of North Carolina; Schafer, Mickey S. Writing the Discussion. Writing in Psychology course syllabus. University of Florida; Yellin, Linda L. A Sociology Writer's Guide . Boston, MA: Allyn and Bacon, 2009.
Writing Tip
Don’t Over-Interpret the Results!
Interpretation is a subjective exercise. As such, you should always approach the selection and interpretation of your findings introspectively and to think critically about the possibility of judgmental biases unintentionally entering into discussions about the significance of your work. With this in mind, be careful that you do not read more into the findings than can be supported by the evidence you have gathered. Remember that the data are the data: nothing more, nothing less.
MacCoun, Robert J. "Biases in the Interpretation and Use of Research Results." Annual Review of Psychology 49 (February 1998): 259-287; Ward, Paulet al, editors. The Oxford Handbook of Expertise . Oxford, UK: Oxford University Press, 2018.
Another Writing Tip
Don't Write Two Results Sections!
One of the most common mistakes that you can make when discussing the results of your study is to present a superficial interpretation of the findings that more or less re-states the results section of your paper. Obviously, you must refer to your results when discussing them, but focus on the interpretation of those results and their significance in relation to the research problem, not the data itself.
Azar, Beth. "Discussing Your Findings." American Psychological Association gradPSYCH Magazine (January 2006).
Yet Another Writing Tip
Avoid Unwarranted Speculation!
The discussion section should remain focused on the findings of your study. For example, if the purpose of your research was to measure the impact of foreign aid on increasing access to education among disadvantaged children in Bangladesh, it would not be appropriate to speculate about how your findings might apply to populations in other countries without drawing from existing studies to support your claim or if analysis of other countries was not a part of your original research design. If you feel compelled to speculate, do so in the form of describing possible implications or explaining possible impacts. Be certain that you clearly identify your comments as speculation or as a suggestion for where further research is needed. Sometimes your professor will encourage you to expand your discussion of the results in this way, while others don’t care what your opinion is beyond your effort to interpret the data in relation to the research problem.
- << Previous: Using Non-Textual Elements
- Next: Limitations of the Study >>
- Last Updated: May 18, 2024 11:38 AM
- URL: https://libguides.usc.edu/writingguide

Library Guides
Dissertations 5: findings, analysis and discussion: home.
- Results/Findings
Alternative Structures
The time has come to show and discuss the findings of your research. How to structure this part of your dissertation?
Dissertations can have different structures, as you can see in the dissertation structure guide.
Dissertations organised by sections
Many dissertations are organised by sections. In this case, we suggest three options. Note that, if within your course you have been instructed to use a specific structure, you should do that. Also note that sometimes there is considerable freedom on the structure, so you can come up with other structures too.
A) More common for scientific dissertations and quantitative methods:
- Results chapter
- Discussion chapter
Example:
- Introduction
- Literature review
- Methodology
- (Recommendations)
if you write a scientific dissertation, or anyway using quantitative methods, you will have some objective results that you will present in the Results chapter. You will then interpret the results in the Discussion chapter.
B) More common for qualitative methods
- Analysis chapter. This can have more descriptive/thematic subheadings.
- Discussion chapter. This can have more descriptive/thematic subheadings.
- Case study of Company X (fashion brand) environmental strategies
- Successful elements
- Lessons learnt
- Criticisms of Company X environmental strategies
- Possible alternatives
C) More common for qualitative methods
- Analysis and discussion chapter. This can have more descriptive/thematic titles.
- Case study of Company X (fashion brand) environmental strategies
If your dissertation uses qualitative methods, it is harder to identify and report objective data. Instead, it may be more productive and meaningful to present the findings in the same sections where you also analyse, and possibly discuss, them. You will probably have different sections dealing with different themes. The different themes can be subheadings of the Analysis and Discussion (together or separate) chapter(s).
Thematic dissertations
If the structure of your dissertation is thematic , you will have several chapters analysing and discussing the issues raised by your research. The chapters will have descriptive/thematic titles.
- Background on the conflict in Yemen (2004-present day)
- Classification of the conflict in international law
- International law violations
- Options for enforcement of international law
- Next: Results/Findings >>
- Last Updated: Aug 4, 2023 2:17 PM
- URL: https://libguides.westminster.ac.uk/c.php?g=696975
CONNECT WITH US
How to Write the Discussion Section of a Research Paper
The discussion section of a research paper analyzes and interprets the findings, provides context, compares them with previous studies, identifies limitations, and suggests future research directions.
Updated on September 15, 2023

Structure your discussion section right, and you’ll be cited more often while doing a greater service to the scientific community. So, what actually goes into the discussion section? And how do you write it?
The discussion section of your research paper is where you let the reader know how your study is positioned in the literature, what to take away from your paper, and how your work helps them. It can also include your conclusions and suggestions for future studies.
First, we’ll define all the parts of your discussion paper, and then look into how to write a strong, effective discussion section for your paper or manuscript.
Discussion section: what is it, what it does
The discussion section comes later in your paper, following the introduction, methods, and results. The discussion sets up your study’s conclusions. Its main goals are to present, interpret, and provide a context for your results.
What is it?
The discussion section provides an analysis and interpretation of the findings, compares them with previous studies, identifies limitations, and suggests future directions for research.
This section combines information from the preceding parts of your paper into a coherent story. By this point, the reader already knows why you did your study (introduction), how you did it (methods), and what happened (results). In the discussion, you’ll help the reader connect the ideas from these sections.
Why is it necessary?
The discussion provides context and interpretations for the results. It also answers the questions posed in the introduction. While the results section describes your findings, the discussion explains what they say. This is also where you can describe the impact or implications of your research.
Adds context for your results
Most research studies aim to answer a question, replicate a finding, or address limitations in the literature. These goals are first described in the introduction. However, in the discussion section, the author can refer back to them to explain how the study's objective was achieved.
Shows what your results actually mean and real-world implications
The discussion can also describe the effect of your findings on research or practice. How are your results significant for readers, other researchers, or policymakers?
What to include in your discussion (in the correct order)
A complete and effective discussion section should at least touch on the points described below.
Summary of key findings
The discussion should begin with a brief factual summary of the results. Concisely overview the main results you obtained.
Begin with key findings with supporting evidence
Your results section described a list of findings, but what message do they send when you look at them all together?
Your findings were detailed in the results section, so there’s no need to repeat them here, but do provide at least a few highlights. This will help refresh the reader’s memory and help them focus on the big picture.
Read the first paragraph of the discussion section in this article (PDF) for an example of how to start this part of your paper. Notice how the authors break down their results and follow each description sentence with an explanation of why each finding is relevant.
State clearly and concisely
Following a clear and direct writing style is especially important in the discussion section. After all, this is where you will make some of the most impactful points in your paper. While the results section often contains technical vocabulary, such as statistical terms, the discussion section lets you describe your findings more clearly.
Interpretation of results
Once you’ve given your reader an overview of your results, you need to interpret those results. In other words, what do your results mean? Discuss the findings’ implications and significance in relation to your research question or hypothesis.
Analyze and interpret your findings
Look into your findings and explore what’s behind them or what may have caused them. If your introduction cited theories or studies that could explain your findings, use these sources as a basis to discuss your results.
For example, look at the second paragraph in the discussion section of this article on waggling honey bees. Here, the authors explore their results based on information from the literature.
Unexpected or contradictory results
Sometimes, your findings are not what you expect. Here’s where you describe this and try to find a reason for it. Could it be because of the method you used? Does it have something to do with the variables analyzed? Comparing your methods with those of other similar studies can help with this task.
Context and comparison with previous work
Refer to related studies to place your research in a larger context and the literature. Compare and contrast your findings with existing literature, highlighting similarities, differences, and/or contradictions.
How your work compares or contrasts with previous work
Studies with similar findings to yours can be cited to show the strength of your findings. Information from these studies can also be used to help explain your results. Differences between your findings and others in the literature can also be discussed here.
How to divide this section into subsections
If you have more than one objective in your study or many key findings, you can dedicate a separate section to each of these. Here’s an example of this approach. You can see that the discussion section is divided into topics and even has a separate heading for each of them.
Limitations
Many journals require you to include the limitations of your study in the discussion. Even if they don’t, there are good reasons to mention these in your paper.
Why limitations don’t have a negative connotation
A study’s limitations are points to be improved upon in future research. While some of these may be flaws in your method, many may be due to factors you couldn’t predict.
Examples include time constraints or small sample sizes. Pointing this out will help future researchers avoid or address these issues. This part of the discussion can also include any attempts you have made to reduce the impact of these limitations, as in this study .
How limitations add to a researcher's credibility
Pointing out the limitations of your study demonstrates transparency. It also shows that you know your methods well and can conduct a critical assessment of them.
Implications and significance
The final paragraph of the discussion section should contain the take-home messages for your study. It can also cite the “strong points” of your study, to contrast with the limitations section.
Restate your hypothesis
Remind the reader what your hypothesis was before you conducted the study.
How was it proven or disproven?
Identify your main findings and describe how they relate to your hypothesis.
How your results contribute to the literature
Were you able to answer your research question? Or address a gap in the literature?
Future implications of your research
Describe the impact that your results may have on the topic of study. Your results may show, for instance, that there are still limitations in the literature for future studies to address. There may be a need for studies that extend your findings in a specific way. You also may need additional research to corroborate your findings.
Sample discussion section
This fictitious example covers all the aspects discussed above. Your actual discussion section will probably be much longer, but you can read this to get an idea of everything your discussion should cover.
Our results showed that the presence of cats in a household is associated with higher levels of perceived happiness by its human occupants. These findings support our hypothesis and demonstrate the association between pet ownership and well-being.
The present findings align with those of Bao and Schreer (2016) and Hardie et al. (2023), who observed greater life satisfaction in pet owners relative to non-owners. Although the present study did not directly evaluate life satisfaction, this factor may explain the association between happiness and cat ownership observed in our sample.
Our findings must be interpreted in light of some limitations, such as the focus on cat ownership only rather than pets as a whole. This may limit the generalizability of our results.
Nevertheless, this study had several strengths. These include its strict exclusion criteria and use of a standardized assessment instrument to investigate the relationships between pets and owners. These attributes bolster the accuracy of our results and reduce the influence of confounding factors, increasing the strength of our conclusions. Future studies may examine the factors that mediate the association between pet ownership and happiness to better comprehend this phenomenon.
This brief discussion begins with a quick summary of the results and hypothesis. The next paragraph cites previous research and compares its findings to those of this study. Information from previous studies is also used to help interpret the findings. After discussing the results of the study, some limitations are pointed out. The paper also explains why these limitations may influence the interpretation of results. Then, final conclusions are drawn based on the study, and directions for future research are suggested.
How to make your discussion flow naturally
If you find writing in scientific English challenging, the discussion and conclusions are often the hardest parts of the paper to write. That’s because you’re not just listing up studies, methods, and outcomes. You’re actually expressing your thoughts and interpretations in words.
- How formal should it be?
- What words should you use, or not use?
- How do you meet strict word limits, or make it longer and more informative?
Always give it your best, but sometimes a helping hand can, well, help. Getting a professional edit can help clarify your work’s importance while improving the English used to explain it. When readers know the value of your work, they’ll cite it. We’ll assign your study to an expert editor knowledgeable in your area of research. Their work will clarify your discussion, helping it to tell your story. Find out more about AJE Editing.

Adam Goulston, PsyD, MS, MBA, MISD, ELS
Science Marketing Consultant
See our "Privacy Policy"
Ensure your structure and ideas are consistent and clearly communicated
Pair your Premium Editing with our add-on service Presubmission Review for an overall assessment of your manuscript.

How To Write The Discussion Chapter
A Simple Explainer With Examples + Free Template
By: Jenna Crossley (PhD) | Reviewed By: Dr. Eunice Rautenbach | August 2021
If you’re reading this, chances are you’ve reached the discussion chapter of your thesis or dissertation and are looking for a bit of guidance. Well, you’ve come to the right place ! In this post, we’ll unpack and demystify the typical discussion chapter in straightforward, easy to understand language, with loads of examples .
Overview: The Discussion Chapter
- What the discussion chapter is
- What to include in your discussion
- How to write up your discussion
- A few tips and tricks to help you along the way
- Free discussion template
What (exactly) is the discussion chapter?
The discussion chapter is where you interpret and explain your results within your thesis or dissertation. This contrasts with the results chapter, where you merely present and describe the analysis findings (whether qualitative or quantitative ). In the discussion chapter, you elaborate on and evaluate your research findings, and discuss the significance and implications of your results .
In this chapter, you’ll situate your research findings in terms of your research questions or hypotheses and tie them back to previous studies and literature (which you would have covered in your literature review chapter). You’ll also have a look at how relevant and/or significant your findings are to your field of research, and you’ll argue for the conclusions that you draw from your analysis. Simply put, the discussion chapter is there for you to interact with and explain your research findings in a thorough and coherent manner.
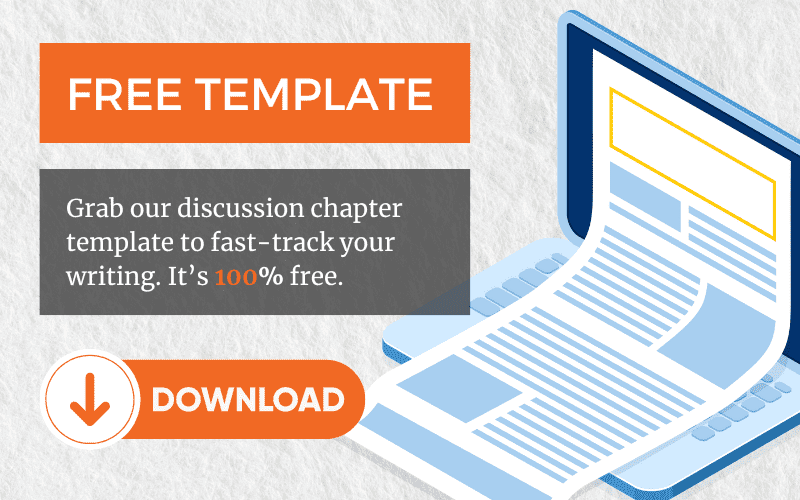
What should I include in the discussion chapter?
First things first: in some studies, the results and discussion chapter are combined into one chapter . This depends on the type of study you conducted (i.e., the nature of the study and methodology adopted), as well as the standards set by the university. So, check in with your university regarding their norms and expectations before getting started. In this post, we’ll treat the two chapters as separate, as this is most common.
Basically, your discussion chapter should analyse , explore the meaning and identify the importance of the data you presented in your results chapter. In the discussion chapter, you’ll give your results some form of meaning by evaluating and interpreting them. This will help answer your research questions, achieve your research aims and support your overall conclusion (s). Therefore, you discussion chapter should focus on findings that are directly connected to your research aims and questions. Don’t waste precious time and word count on findings that are not central to the purpose of your research project.
As this chapter is a reflection of your results chapter, it’s vital that you don’t report any new findings . In other words, you can’t present claims here if you didn’t present the relevant data in the results chapter first. So, make sure that for every discussion point you raise in this chapter, you’ve covered the respective data analysis in the results chapter. If you haven’t, you’ll need to go back and adjust your results chapter accordingly.
If you’re struggling to get started, try writing down a bullet point list everything you found in your results chapter. From this, you can make a list of everything you need to cover in your discussion chapter. Also, make sure you revisit your research questions or hypotheses and incorporate the relevant discussion to address these. This will also help you to see how you can structure your chapter logically.
Need a helping hand?
How to write the discussion chapter
Now that you’ve got a clear idea of what the discussion chapter is and what it needs to include, let’s look at how you can go about structuring this critically important chapter. Broadly speaking, there are six core components that need to be included, and these can be treated as steps in the chapter writing process.
Step 1: Restate your research problem and research questions
The first step in writing up your discussion chapter is to remind your reader of your research problem , as well as your research aim(s) and research questions . If you have hypotheses, you can also briefly mention these. This “reminder” is very important because, after reading dozens of pages, the reader may have forgotten the original point of your research or been swayed in another direction. It’s also likely that some readers skip straight to your discussion chapter from the introduction chapter , so make sure that your research aims and research questions are clear.
Step 2: Summarise your key findings
Next, you’ll want to summarise your key findings from your results chapter. This may look different for qualitative and quantitative research , where qualitative research may report on themes and relationships, whereas quantitative research may touch on correlations and causal relationships. Regardless of the methodology, in this section you need to highlight the overall key findings in relation to your research questions.
Typically, this section only requires one or two paragraphs , depending on how many research questions you have. Aim to be concise here, as you will unpack these findings in more detail later in the chapter. For now, a few lines that directly address your research questions are all that you need.
Some examples of the kind of language you’d use here include:
- The data suggest that…
- The data support/oppose the theory that…
- The analysis identifies…
These are purely examples. What you present here will be completely dependent on your original research questions, so make sure that you are led by them .
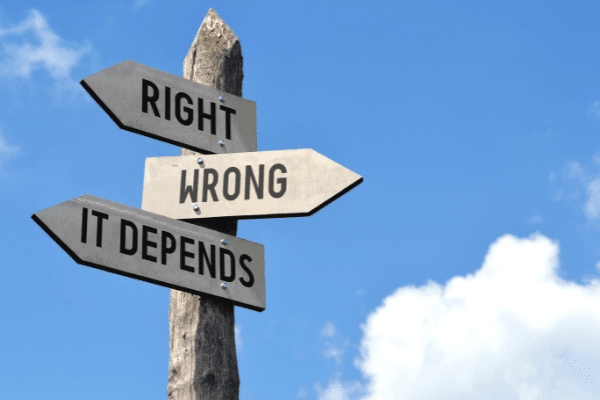
Step 3: Interpret your results
Once you’ve restated your research problem and research question(s) and briefly presented your key findings, you can unpack your findings by interpreting your results. Remember: only include what you reported in your results section – don’t introduce new information.
From a structural perspective, it can be a wise approach to follow a similar structure in this chapter as you did in your results chapter. This would help improve readability and make it easier for your reader to follow your arguments. For example, if you structured you results discussion by qualitative themes, it may make sense to do the same here.
Alternatively, you may structure this chapter by research questions, or based on an overarching theoretical framework that your study revolved around. Every study is different, so you’ll need to assess what structure works best for you.
When interpreting your results, you’ll want to assess how your findings compare to those of the existing research (from your literature review chapter). Even if your findings contrast with the existing research, you need to include these in your discussion. In fact, those contrasts are often the most interesting findings . In this case, you’d want to think about why you didn’t find what you were expecting in your data and what the significance of this contrast is.
Here are a few questions to help guide your discussion:
- How do your results relate with those of previous studies ?
- If you get results that differ from those of previous studies, why may this be the case?
- What do your results contribute to your field of research?
- What other explanations could there be for your findings?
When interpreting your findings, be careful not to draw conclusions that aren’t substantiated . Every claim you make needs to be backed up with evidence or findings from the data (and that data needs to be presented in the previous chapter – results). This can look different for different studies; qualitative data may require quotes as evidence, whereas quantitative data would use statistical methods and tests. Whatever the case, every claim you make needs to be strongly backed up.
Step 4: Acknowledge the limitations of your study
The fourth step in writing up your discussion chapter is to acknowledge the limitations of the study. These limitations can cover any part of your study , from the scope or theoretical basis to the analysis method(s) or sample. For example, you may find that you collected data from a very small sample with unique characteristics, which would mean that you are unable to generalise your results to the broader population.
For some students, discussing the limitations of their work can feel a little bit self-defeating . This is a misconception, as a core indicator of high-quality research is its ability to accurately identify its weaknesses. In other words, accurately stating the limitations of your work is a strength, not a weakness . All that said, be careful not to undermine your own research. Tell the reader what limitations exist and what improvements could be made, but also remind them of the value of your study despite its limitations.
Step 5: Make recommendations for implementation and future research
Now that you’ve unpacked your findings and acknowledge the limitations thereof, the next thing you’ll need to do is reflect on your study in terms of two factors:
- The practical application of your findings
- Suggestions for future research
The first thing to discuss is how your findings can be used in the real world – in other words, what contribution can they make to the field or industry? Where are these contributions applicable, how and why? For example, if your research is on communication in health settings, in what ways can your findings be applied to the context of a hospital or medical clinic? Make sure that you spell this out for your reader in practical terms, but also be realistic and make sure that any applications are feasible.
The next discussion point is the opportunity for future research . In other words, how can other studies build on what you’ve found and also improve the findings by overcoming some of the limitations in your study (which you discussed a little earlier). In doing this, you’ll want to investigate whether your results fit in with findings of previous research, and if not, why this may be the case. For example, are there any factors that you didn’t consider in your study? What future research can be done to remedy this? When you write up your suggestions, make sure that you don’t just say that more research is needed on the topic, also comment on how the research can build on your study.
Step 6: Provide a concluding summary
Finally, you’ve reached your final stretch. In this section, you’ll want to provide a brief recap of the key findings – in other words, the findings that directly address your research questions . Basically, your conclusion should tell the reader what your study has found, and what they need to take away from reading your report.
When writing up your concluding summary, bear in mind that some readers may skip straight to this section from the beginning of the chapter. So, make sure that this section flows well from and has a strong connection to the opening section of the chapter.
Tips and tricks for an A-grade discussion chapter
Now that you know what the discussion chapter is , what to include and exclude , and how to structure it , here are some tips and suggestions to help you craft a quality discussion chapter.
- When you write up your discussion chapter, make sure that you keep it consistent with your introduction chapter , as some readers will skip from the introduction chapter directly to the discussion chapter. Your discussion should use the same tense as your introduction, and it should also make use of the same key terms.
- Don’t make assumptions about your readers. As a writer, you have hands-on experience with the data and so it can be easy to present it in an over-simplified manner. Make sure that you spell out your findings and interpretations for the intelligent layman.
- Have a look at other theses and dissertations from your institution, especially the discussion sections. This will help you to understand the standards and conventions of your university, and you’ll also get a good idea of how others have structured their discussion chapters. You can also check out our chapter template .
- Avoid using absolute terms such as “These results prove that…”, rather make use of terms such as “suggest” or “indicate”, where you could say, “These results suggest that…” or “These results indicate…”. It is highly unlikely that a dissertation or thesis will scientifically prove something (due to a variety of resource constraints), so be humble in your language.
- Use well-structured and consistently formatted headings to ensure that your reader can easily navigate between sections, and so that your chapter flows logically and coherently.
If you have any questions or thoughts regarding this post, feel free to leave a comment below. Also, if you’re looking for one-on-one help with your discussion chapter (or thesis in general), consider booking a free consultation with one of our highly experienced Grad Coaches to discuss how we can help you.
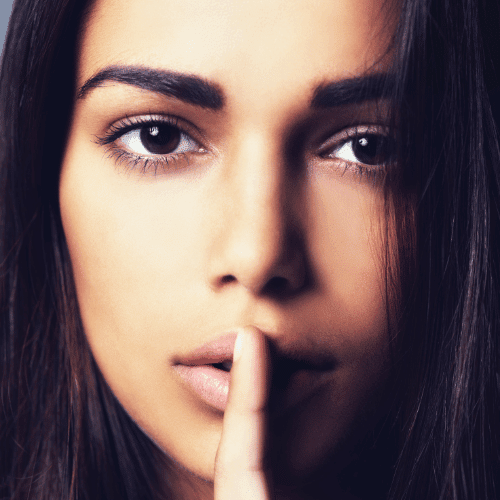
Psst... there’s more!
This post was based on one of our popular Research Bootcamps . If you're working on a research project, you'll definitely want to check this out ...
You Might Also Like:
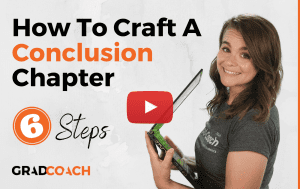
36 Comments
Thank you this is helpful!
This is very helpful to me… Thanks a lot for sharing this with us 😊
This has been very helpful indeed. Thank you.
This is actually really helpful, I just stumbled upon it. Very happy that I found it, thank you.
Me too! I was kinda lost on how to approach my discussion chapter. How helpful! Thanks a lot!
This is really good and explicit. Thanks
Thank you, this blog has been such a help.
Thank you. This is very helpful.
Dear sir/madame
Thanks a lot for this helpful blog. Really, it supported me in writing my discussion chapter while I was totally unaware about its structure and method of writing.
With regards
Syed Firoz Ahmad PhD, Research Scholar
I agree so much. This blog was god sent. It assisted me so much while I was totally clueless about the context and the know-how. Now I am fully aware of what I am to do and how I am to do it.
Thanks! This is helpful!
thanks alot for this informative website
Dear Sir/Madam,
Truly, your article was much benefited when i structured my discussion chapter.
Thank you very much!!!
This is helpful for me in writing my research discussion component. I have to copy this text on Microsoft word cause of my weakness that I cannot be able to read the text on screen a long time. So many thanks for this articles.
This was helpful
Thanks Jenna, well explained.
Thank you! This is super helpful.
Thanks very much. I have appreciated the six steps on writing the Discussion chapter which are (i) Restating the research problem and questions (ii) Summarising the key findings (iii) Interpreting the results linked to relating to previous results in positive and negative ways; explaining whay different or same and contribution to field of research and expalnation of findings (iv) Acknowledgeing limitations (v) Recommendations for implementation and future resaerch and finally (vi) Providing a conscluding summary
My two questions are: 1. On step 1 and 2 can it be the overall or you restate and sumamrise on each findings based on the reaerch question? 2. On 4 and 5 do you do the acknowlledgement , recommendations on each research finding or overall. This is not clear from your expalanattion.
Please respond.
This post is very useful. I’m wondering whether practical implications must be introduced in the Discussion section or in the Conclusion section?
Sigh, I never knew a 20 min video could have literally save my life like this. I found this at the right time!!!! Everything I need to know in one video thanks a mil ! OMGG and that 6 step!!!!!! was the cherry on top the cake!!!!!!!!!
Thanks alot.., I have gained much
This piece is very helpful on how to go about my discussion section. I can always recommend GradCoach research guides for colleagues.
Many thanks for this resource. It has been very helpful to me. I was finding it hard to even write the first sentence. Much appreciated.
Thanks so much. Very helpful to know what is included in the discussion section
this was a very helpful and useful information
This is very helpful. Very very helpful. Thanks for sharing this online!
it is very helpfull article, and i will recommend it to my fellow students. Thank you.
Superlative! More grease to your elbows.
Powerful, thank you for sharing.
Wow! Just wow! God bless the day I stumbled upon you guys’ YouTube videos! It’s been truly life changing and anxiety about my report that is due in less than a month has subsided significantly!
Simplified explanation. Well done.
The presentation is enlightening. Thank you very much.
Thanks for the support and guidance
This has been a great help to me and thank you do much
I second that “it is highly unlikely that a dissertation or thesis will scientifically prove something”; although, could you enlighten us on that comment and elaborate more please?
Sure, no problem.
Scientific proof is generally considered a very strong assertion that something is definitively and universally true. In most scientific disciplines, especially within the realms of natural and social sciences, absolute proof is very rare. Instead, researchers aim to provide evidence that supports or rejects hypotheses. This evidence increases or decreases the likelihood that a particular theory is correct, but it rarely proves something in the absolute sense.
Dissertations and theses, as substantial as they are, typically focus on exploring a specific question or problem within a larger field of study. They contribute to a broader conversation and body of knowledge. The aim is often to provide detailed insight, extend understanding, and suggest directions for further research rather than to offer definitive proof. These academic works are part of a cumulative process of knowledge building where each piece of research connects with others to gradually enhance our understanding of complex phenomena.
Furthermore, the rigorous nature of scientific inquiry involves continuous testing, validation, and potential refutation of ideas. What might be considered a “proof” at one point can later be challenged by new evidence or alternative interpretations. Therefore, the language of “proof” is cautiously used in academic circles to maintain scientific integrity and humility.
Submit a Comment Cancel reply
Your email address will not be published. Required fields are marked *
Save my name, email, and website in this browser for the next time I comment.
- Print Friendly

Writing the Dissertation - Guides for Success: The Results and Discussion
- Writing the Dissertation Homepage
- Overview and Planning
- The Literature Review
- The Methodology
- The Results and Discussion
- The Conclusion
- The Abstract
- The Difference
- What to Avoid
Overview of writing the results and discussion
The results and discussion follow on from the methods or methodology chapter of the dissertation. This creates a natural transition from how you designed your study, to what your study reveals, highlighting your own contribution to the research area.
Disciplinary differences
Please note: this guide is not specific to any one discipline. The results and discussion can vary depending on the nature of the research and the expectations of the school or department, so please adapt the following advice to meet the demands of your project and department. Consult your supervisor for further guidance; you can also peruse our Writing Across Subjects guide .
Guide contents
As part of the Writing the Dissertation series, this guide covers the most common conventions of the results and discussion chapters, giving you the necessary knowledge, tips and guidance needed to impress your markers! The sections are organised as follows:
- The Difference - Breaks down the distinctions between the results and discussion chapters.
- Results - Provides a walk-through of common characteristics of the results chapter.
- Discussion - Provides a walk-through of how to approach writing your discussion chapter, including structure.
- What to Avoid - Covers a few frequent mistakes you'll want to...avoid!
- FAQs - Guidance on first- vs. third-person, limitations and more.
- Checklist - Includes a summary of key points and a self-evaluation checklist.
Training and tools
- The Academic Skills team has recorded a Writing the Dissertation workshop series to help you with each section of a standard dissertation, including a video on writing the results and discussion (embedded below).
- The dissertation planner tool can help you think through the timeline for planning, research, drafting and editing.
- iSolutions offers training and a Word template to help you digitally format and structure your dissertation.
Introduction
The results of your study are often followed by a separate chapter of discussion. This is certainly the case with scientific writing. Some dissertations, however, might incorporate both the results and discussion in one chapter. This depends on the nature of your dissertation and the conventions within your school or department. Always follow the guidelines given to you and ask your supervisor for further guidance.
As part of the Writing the Dissertation series, this guide covers the essentials of writing your results and discussion, giving you the necesary knowledge, tips and guidance needed to leave a positive impression on your markers! This guide covers the results and discussion as separate – although interrelated – chapters, as you'll see in the next two tabs. However, you can easily adapt the guidance to suit one single chapter – keep an eye out for some hints on how to do this throughout the guide.
Results or discussion - what's the difference?
To understand what the results and discussion sections are about, we need to clearly define the difference between the two.
The results should provide a clear account of the findings . This is written in a dry and direct manner, simply highlighting the findings as they appear once processed. It’s expected to have tables and graphics, where relevant, to contextualise and illustrate the data.
Rather than simply stating the findings of the study, the discussion interprets the findings to offer a more nuanced understanding of the research. The discussion is similar to the second half of the conclusion because it’s where you consider and formulate a response to the question, ‘what do we now know that we didn’t before?’ (see our Writing the Conclusion guide for more). The discussion achieves this by answering the research questions and responding to any hypotheses proposed. With this in mind, the discussion should be the most insightful chapter or section of your dissertation because it provides the most original insight.
Across the next two tabs of this guide, we will look at the results and discussion chapters separately in more detail.
Writing the results
The results chapter should provide a direct and factual account of the data collected without any interpretation or interrogation of the findings. As this might suggest, the results chapter can be slightly monotonous, particularly for quantitative data. Nevertheless, it’s crucial that you present your results in a clear and direct manner as it provides the necessary detail for your subsequent discussion.
Note: If you’re writing your results and discussion as one chapter, then you can either:
1) write them as distinctly separate sections in the same chapter, with the discussion following on from the results, or...
2) integrate the two throughout by presenting a subset of the results and then discussing that subset in further detail.
Next, we'll explore some of the most important factors to consider when writing your results chapter.
How you structure your results chapter depends on the design and purpose of your study. Here are some possible options for structuring your results chapter (adapted from Glatthorn and Joyner, 2005):
- Chronological – depending on the nature of the study, it might be important to present your results in order of how you collected the data, such as a pretest-posttest design.
- Research method – if you’ve used a mixed-methods approach, you could isolate each research method and instrument employed in the study.
- Research question and/or hypotheses – you could structure your results around your research questions and/or hypotheses, providing you have more than one. However, keep in mind that the results on their own don’t necessarily answer the questions or respond to the hypotheses in a definitive manner. You need to interpret the findings in the discussion chapter to gain a more rounded understanding.
- Variable – you could isolate each variable in your study (where relevant) and specify how and whether the results changed.
Tables and figures
For your results, you are expected to convert your data into tables and figures, particularly when dealing with quantitative data. Making use of tables and figures is a way of contextualising your results within the study. It also helps to visually reinforce your written account of the data. However, make sure you’re only using tables and figures to supplement , rather than replace, your written account of the results (see the 'What to avoid' tab for more on this).
Figures and tables need to be numbered in order of when they appear in the dissertation, and they should be capitalised. You also need to make direct reference to them in the text, which you can do (with some variation) in one of the following ways:
Figure 1 shows…
The results of the test (see Figure 1) demonstrate…
The actual figures and tables themselves also need to be accompanied by a caption that briefly outlines what is displayed. For example:
Table 1. Variables of the regression model
Table captions normally appear above the table, whilst figures or other such graphical forms appear below, although it’s worth confirming this with your supervisor as the formatting can change depending on the school or discipline. The style guide used for writing in your subject area (e.g., Harvard, MLA, APA, OSCOLA) often dictates correct formatting of tables, graphs and figures, so have a look at your style guide for additional support.
Using quotations
If your qualitative data comes from interviews and focus groups, your data will largely consist of quotations from participants. When presenting this data, you should identify and group the most common and interesting responses and then quote two or three relevant examples to illustrate this point. Here’s a brief example from a qualitative study on the habits of online food shoppers:
Regardless of whether or not participants regularly engage in online food shopping, all but two respondents commented, in some form, on the convenience of online food shopping:
"It’s about convenience for me. I’m at work all week and the weekend doesn’t allow much time for food shopping, so knowing it can be ordered and then delivered in 24 hours is great for me” (Participant A).
"It fits around my schedule, which is important for me and my family” (Participant D).
"In the past, I’ve always gone food shopping after work, which has always been a hassle. Online food shopping, however, frees up some of my time” (Participant E).
As shown in this example, each quotation is attributed to a particular participant, although their anonymity is protected. The details used to identify participants can depend on the relevance of certain factors to the research. For instance, age or gender could be included.
Writing the discussion
The discussion chapter is where “you critically examine your own results in the light of the previous state of the subject as outlined in the background, and make judgments as to what has been learnt in your work” (Evans et al., 2014: 12). Whilst the results chapter is strictly factual, reporting on the data on a surface level, the discussion is rooted in analysis and interpretation , allowing you and your reader to delve beneath the surface.
Next, we will review some of the most important factors to consider when writing your discussion chapter.
Like the results, there is no single way to structure your discussion chapter. As always, it depends on the nature of your dissertation and whether you’re dealing with qualitative, quantitative or mixed-methods research. It’s good to be consistent with the results chapter, so you could structure your discussion chapter, where possible, in the same way as your results.
When it comes to structure, it’s particularly important that you guide your reader through the various points, subtopics or themes of your discussion. You should do this by structuring sections of your discussion, which might incorporate three or four paragraphs around the same theme or issue, in a three-part way that mirrors the typical three-part essay structure of introduction, main body and conclusion.

Figure 1: The three-part cycle that embodies a typical essay structure and reflects how you structure themes or subtopics in your discussion.
This is your topic sentence where you clearly state the focus of this paragraph/section. It’s often a fairly short, declarative statement in order to grab the reader’s attention, and it should be clearly related to your research purpose, such as responding to a research question.
This constitutes your analysis where you explore the theme or focus, outlined in the topic sentence, in further detail by interrogating why this particular theme or finding emerged and the significance of this data. This is also where you bring in the relevant secondary literature.
This is the evaluative stage of the cycle where you explicitly return back to the topic sentence and tell the reader what this means in terms of answering the relevant research question and establishing new knowledge. It could be a single sentence, or a short paragraph, and it doesn’t strictly need to appear at the end of every section or theme. Instead, some prefer to bring the main themes together towards the end of the discussion in a single paragraph or two. Either way, it’s imperative that you evaluate the significance of your discussion and tell the reader what this means.
A note on the three-part structure
This is often how you’re taught to construct a paragraph, but the themes and ideas you engage with at dissertation level are going to extend beyond the confines of a short paragraph. Therefore, this is a structure to guide how you write about particular themes or patterns in your discussion. Think of this structure like a cycle that you can engage in its smallest form to shape a paragraph; in a slightly larger form to shape a subsection of a chapter; and in its largest form to shape the entire chapter. You can 'level up' the same basic structure to accommodate a deeper breadth of thinking and critical engagement.
Using secondary literature
Your discussion chapter should return to the relevant literature (previously identified in your literature review ) in order to contextualise and deepen your reader’s understanding of the findings. This might help to strengthen your findings, or you might find contradictory evidence that serves to counter your results. In the case of the latter, it’s important that you consider why this might be and the implications for this. It’s through your incorporation of secondary literature that you can consider the question, ‘What do we now know that we didn’t before?’
Limitations
You may have included a limitations section in your methodology chapter (see our Writing the Methodology guide ), but it’s also common to have one in your discussion chapter. The difference here is that your limitations are directly associated with your results and the capacity to interpret and analyse those results.
Think of it this way: the limitations in your methodology refer to the issues identified before conducting the research, whilst the limitations in your discussion refer to the issues that emerged after conducting the research. For example, you might only be able to identify a limitation about the external validity or generalisability of your research once you have processed and analysed the data. Try not to overstress the limitations of your work – doing so can undermine the work you’ve done – and try to contextualise them, perhaps by relating them to certain limitations of other studies.
Recommendations
It’s often good to follow your limitations with some recommendations for future research. This creates a neat linearity from what didn’t work, or what could be improved, to how other researchers could address these issues in the future. This helps to reposition your limitations in a positive way by offering an action-oriented response. Try to limit the amount of recommendations you discuss – too many can bring the end of your discussion to a rather negative end as you’re ultimately focusing on what should be done, rather than what you have done. You also don’t need to repeat the recommendations in your conclusion if you’ve included them here.
What to avoid
This portion of the guide will cover some common missteps you should try to avoid in writing your results and discussion.
Over-reliance on tables and figures
It’s very common to produce visual representations of data, such as graphs and tables, and to use these representations in your results chapter. However, the use of these figures should not entirely replace your written account of the data. You don’t need to specify every detail in the data set, but you should provide some written account of what the data shows, drawing your reader’s attention to the most important elements of the data. The figures should support your account and help to contextualise your results. Simply stating, ‘look at Table 1’, without any further detail is not sufficient. Writers often try to do this as a way of saving words, but your markers will know!
Ignoring unexpected or contradictory data
Research can be a complex process with ups and downs, surprises and anomalies. Don’t be tempted to ignore any data that doesn’t meet your expectations, or that perhaps you’re struggling to explain. Failing to report on data for these, and other such reasons, is a problem because it undermines your credibility as a researcher, which inevitably undermines your research in the process. You have to do your best to provide some reason to such data. For instance, there might be some methodological reason behind a particular trend in the data.
Including raw data
You don’t need to include any raw data in your results chapter – raw data meaning unprocessed data that hasn’t undergone any calculations or other such refinement. This can overwhelm your reader and obscure the clarity of the research. You can include raw data in an appendix, providing you feel it’s necessary.
Presenting new results in the discussion
You shouldn’t be stating original findings for the first time in the discussion chapter. The findings of your study should first appear in your results before elaborating on them in the discussion.
Overstressing the significance of your research
It’s important that you clarify what your research demonstrates so you can highlight your own contribution to the research field. However, don’t overstress or inflate the significance of your results. It’s always difficult to provide definitive answers in academic research, especially with qualitative data. You should be confident and authoritative where possible, but don’t claim to reach the absolute truth when perhaps other conclusions could be reached. Where necessary, you should use hedging (see definition) to slightly soften the tone and register of your language.
Definition: Hedging refers to 'the act of expressing your attitude or ideas in tentative or cautious ways' (Singh and Lukkarila, 2017: 101). It’s mostly achieved through a number of verbs or adverbs, such as ‘suggest’ or ‘seemingly.’
Q: What’s the difference between the results and discussion?
A: The results chapter is a factual account of the data collected, whilst the discussion considers the implications of these findings by relating them to relevant literature and answering your research question(s). See the tab 'The Differences' in this guide for more detail.
Q: Should the discussion include recommendations for future research?
A: Your dissertation should include some recommendations for future research, but it can vary where it appears. Recommendations are often featured towards the end of the discussion chapter, but they also regularly appear in the conclusion chapter (see our Writing the Conclusion guide for more). It simply depends on your dissertation and the conventions of your school or department. It’s worth consulting any specific guidance that you’ve been given, or asking your supervisor directly.
Q: Should the discussion include the limitations of the study?
A: Like the answer above, you should engage with the limitations of your study, but it might appear in the discussion of some dissertations, or the conclusion of others. Consider the narrative flow and whether it makes sense to include the limitations in your discussion chapter, or your conclusion. You should also consult any discipline-specific guidance you’ve been given, or ask your supervisor for more. Be mindful that this is slightly different to the limitations outlined in the methodology or methods chapter (see our Writing the Methodology guide vs. the 'Discussion' tab of this guide).
Q: Should the results and discussion be in the first-person or third?
A: It’s important to be consistent , so you should use whatever you’ve been using throughout your dissertation. Third-person is more commonly accepted, but certain disciplines are happy with the use of first-person. Just remember that the first-person pronoun can be a distracting, but powerful device, so use it sparingly. Consult your lecturer for discipline-specific guidance.
Q: Is there a difference between the discussion and the conclusion of a dissertation?
A: Yes, there is a difference. The discussion chapter is a detailed consideration of how your findings answer your research questions. This includes the use of secondary literature to help contextualise your discussion. Rather than considering the findings in detail, the conclusion briefly summarises and synthesises the main findings of your study before bringing the dissertation to a close. Both are similar, particularly in the way they ‘broaden out’ to consider the wider implications of the research. They are, however, their own distinct chapters, unless otherwise stated by your supervisor.
The results and discussion chapters (or chapter) constitute a large part of your dissertation as it’s here where your original contribution is foregrounded and discussed in detail. Remember, the results chapter simply reports on the data collected, whilst the discussion is where you consider your research questions and/or hypothesis in more detail by interpreting and interrogating the data. You can integrate both into a single chapter and weave the interpretation of your findings throughout the chapter, although it’s common for both the results and discussion to appear as separate chapters. Consult your supervisor for further guidance.
Here’s a final checklist for writing your results and discussion. Remember that not all of these points will be relevant for you, so make sure you cover whatever’s appropriate for your dissertation. The asterisk (*) indicates any content that might not be relevant for your dissertation. To download a copy of the checklist to save and edit, please use the Word document, below.
- Results and discussion self-evaluation checklist

- << Previous: The Methodology
- Next: The Conclusion >>
- Last Updated: Apr 19, 2024 12:38 PM
- URL: https://library.soton.ac.uk/writing_the_dissertation
Jump to navigation

Cochrane Training
Chapter 14: completing ‘summary of findings’ tables and grading the certainty of the evidence.
Holger J Schünemann, Julian PT Higgins, Gunn E Vist, Paul Glasziou, Elie A Akl, Nicole Skoetz, Gordon H Guyatt; on behalf of the Cochrane GRADEing Methods Group (formerly Applicability and Recommendations Methods Group) and the Cochrane Statistical Methods Group
Key Points:
- A ‘Summary of findings’ table for a given comparison of interventions provides key information concerning the magnitudes of relative and absolute effects of the interventions examined, the amount of available evidence and the certainty (or quality) of available evidence.
- ‘Summary of findings’ tables include a row for each important outcome (up to a maximum of seven). Accepted formats of ‘Summary of findings’ tables and interactive ‘Summary of findings’ tables can be produced using GRADE’s software GRADEpro GDT.
- Cochrane has adopted the GRADE approach (Grading of Recommendations Assessment, Development and Evaluation) for assessing certainty (or quality) of a body of evidence.
- The GRADE approach specifies four levels of the certainty for a body of evidence for a given outcome: high, moderate, low and very low.
- GRADE assessments of certainty are determined through consideration of five domains: risk of bias, inconsistency, indirectness, imprecision and publication bias. For evidence from non-randomized studies and rarely randomized studies, assessments can then be upgraded through consideration of three further domains.
Cite this chapter as: Schünemann HJ, Higgins JPT, Vist GE, Glasziou P, Akl EA, Skoetz N, Guyatt GH. Chapter 14: Completing ‘Summary of findings’ tables and grading the certainty of the evidence. In: Higgins JPT, Thomas J, Chandler J, Cumpston M, Li T, Page MJ, Welch VA (editors). Cochrane Handbook for Systematic Reviews of Interventions version 6.4 (updated August 2023). Cochrane, 2023. Available from www.training.cochrane.org/handbook .
14.1 ‘Summary of findings’ tables
14.1.1 introduction to ‘summary of findings’ tables.
‘Summary of findings’ tables present the main findings of a review in a transparent, structured and simple tabular format. In particular, they provide key information concerning the certainty or quality of evidence (i.e. the confidence or certainty in the range of an effect estimate or an association), the magnitude of effect of the interventions examined, and the sum of available data on the main outcomes. Cochrane Reviews should incorporate ‘Summary of findings’ tables during planning and publication, and should have at least one key ‘Summary of findings’ table representing the most important comparisons. Some reviews may include more than one ‘Summary of findings’ table, for example if the review addresses more than one major comparison, or includes substantially different populations that require separate tables (e.g. because the effects differ or it is important to show results separately). In the Cochrane Database of Systematic Reviews (CDSR), all ‘Summary of findings’ tables for a review appear at the beginning, before the Background section.
14.1.2 Selecting outcomes for ‘Summary of findings’ tables
Planning for the ‘Summary of findings’ table starts early in the systematic review, with the selection of the outcomes to be included in: (i) the review; and (ii) the ‘Summary of findings’ table. This is a crucial step, and one that review authors need to address carefully.
To ensure production of optimally useful information, Cochrane Reviews begin by developing a review question and by listing all main outcomes that are important to patients and other decision makers (see Chapter 2 and Chapter 3 ). The GRADE approach to assessing the certainty of the evidence (see Section 14.2 ) defines and operationalizes a rating process that helps separate outcomes into those that are critical, important or not important for decision making. Consultation and feedback on the review protocol, including from consumers and other decision makers, can enhance this process.
Critical outcomes are likely to include clearly important endpoints; typical examples include mortality and major morbidity (such as strokes and myocardial infarction). However, they may also represent frequent minor and rare major side effects, symptoms, quality of life, burdens associated with treatment, and resource issues (costs). Burdens represent the impact of healthcare workload on patient function and well-being, and include the demands of adhering to an intervention that patients or caregivers (e.g. family) may dislike, such as having to undergo more frequent tests, or the restrictions on lifestyle that certain interventions require (Spencer-Bonilla et al 2017).
Frequently, when formulating questions that include all patient-important outcomes for decision making, review authors will confront reports of studies that have not included all these outcomes. This is particularly true for adverse outcomes. For instance, randomized trials might contribute evidence on intended effects, and on frequent, relatively minor side effects, but not report on rare adverse outcomes such as suicide attempts. Chapter 19 discusses strategies for addressing adverse effects. To obtain data for all important outcomes it may be necessary to examine the results of non-randomized studies (see Chapter 24 ). Cochrane, in collaboration with others, has developed guidance for review authors to support their decision about when to look for and include non-randomized studies (Schünemann et al 2013).
If a review includes only randomized trials, these trials may not address all important outcomes and it may therefore not be possible to address these outcomes within the constraints of the review. Review authors should acknowledge these limitations and make them transparent to readers. Review authors are encouraged to include non-randomized studies to examine rare or long-term adverse effects that may not adequately be studied in randomized trials. This raises the possibility that harm outcomes may come from studies in which participants differ from those in studies used in the analysis of benefit. Review authors will then need to consider how much such differences are likely to impact on the findings, and this will influence the certainty of evidence because of concerns about indirectness related to the population (see Section 14.2.2 ).
Non-randomized studies can provide important information not only when randomized trials do not report on an outcome or randomized trials suffer from indirectness, but also when the evidence from randomized trials is rated as very low and non-randomized studies provide evidence of higher certainty. Further discussion of these issues appears also in Chapter 24 .
14.1.3 General template for ‘Summary of findings’ tables
Several alternative standard versions of ‘Summary of findings’ tables have been developed to ensure consistency and ease of use across reviews, inclusion of the most important information needed by decision makers, and optimal presentation (see examples at Figures 14.1.a and 14.1.b ). These formats are supported by research that focused on improved understanding of the information they intend to convey (Carrasco-Labra et al 2016, Langendam et al 2016, Santesso et al 2016). They are available through GRADE’s official software package developed to support the GRADE approach: GRADEpro GDT (www.gradepro.org).
Standard Cochrane ‘Summary of findings’ tables include the following elements using one of the accepted formats. Further guidance on each of these is provided in Section 14.1.6 .
- A brief description of the population and setting addressed by the available evidence (which may be slightly different to or narrower than those defined by the review question).
- A brief description of the comparison addressed in the ‘Summary of findings’ table, including both the experimental and comparison interventions.
- A list of the most critical and/or important health outcomes, both desirable and undesirable, limited to seven or fewer outcomes.
- A measure of the typical burden of each outcomes (e.g. illustrative risk, or illustrative mean, on comparator intervention).
- The absolute and relative magnitude of effect measured for each (if both are appropriate).
- The numbers of participants and studies contributing to the analysis of each outcomes.
- A GRADE assessment of the overall certainty of the body of evidence for each outcome (which may vary by outcome).
- Space for comments.
- Explanations (formerly known as footnotes).
Ideally, ‘Summary of findings’ tables are supported by more detailed tables (known as ‘evidence profiles’) to which the review may be linked, which provide more detailed explanations. Evidence profiles include the same important health outcomes, and provide greater detail than ‘Summary of findings’ tables of both of the individual considerations feeding into the grading of certainty and of the results of the studies (Guyatt et al 2011a). They ensure that a structured approach is used to rating the certainty of evidence. Although they are rarely published in Cochrane Reviews, evidence profiles are often used, for example, by guideline developers in considering the certainty of the evidence to support guideline recommendations. Review authors will find it easier to develop the ‘Summary of findings’ table by completing the rating of the certainty of evidence in the evidence profile first in GRADEpro GDT. They can then automatically convert this to one of the ‘Summary of findings’ formats in GRADEpro GDT, including an interactive ‘Summary of findings’ for publication.
As a measure of the magnitude of effect for dichotomous outcomes, the ‘Summary of findings’ table should provide a relative measure of effect (e.g. risk ratio, odds ratio, hazard) and measures of absolute risk. For other types of data, an absolute measure alone (such as a difference in means for continuous data) might be sufficient. It is important that the magnitude of effect is presented in a meaningful way, which may require some transformation of the result of a meta-analysis (see also Chapter 15, Section 15.4 and Section 15.5 ). Reviews with more than one main comparison should include a separate ‘Summary of findings’ table for each comparison.
Figure 14.1.a provides an example of a ‘Summary of findings’ table. Figure 15.1.b provides an alternative format that may further facilitate users’ understanding and interpretation of the review’s findings. Evidence evaluating different formats suggests that the ‘Summary of findings’ table should include a risk difference as a measure of the absolute effect and authors should preferably use a format that includes a risk difference .
A detailed description of the contents of a ‘Summary of findings’ table appears in Section 14.1.6 .
Figure 14.1.a Example of a ‘Summary of findings’ table
Summary of findings (for interactive version click here )
a All the stockings in the nine studies included in this review were below-knee compression stockings. In four studies the compression strength was 20 mmHg to 30 mmHg at the ankle. It was 10 mmHg to 20 mmHg in the other four studies. Stockings come in different sizes. If a stocking is too tight around the knee it can prevent essential venous return causing the blood to pool around the knee. Compression stockings should be fitted properly. A stocking that is too tight could cut into the skin on a long flight and potentially cause ulceration and increased risk of DVT. Some stockings can be slightly thicker than normal leg covering and can be potentially restrictive with tight foot wear. It is a good idea to wear stockings around the house prior to travel to ensure a good, comfortable fit. Participants put their stockings on two to three hours before the flight in most of the studies. The availability and cost of stockings can vary.
b Two studies recruited high risk participants defined as those with previous episodes of DVT, coagulation disorders, severe obesity, limited mobility due to bone or joint problems, neoplastic disease within the previous two years, large varicose veins or, in one of the studies, participants taller than 190 cm and heavier than 90 kg. The incidence for the seven studies that excluded high risk participants was 1.45% and the incidence for the two studies that recruited high-risk participants (with at least one risk factor) was 2.43%. We have used 10 and 30 per 1000 to express different risk strata, respectively.
c The confidence interval crosses no difference and does not rule out a small increase.
d The measurement of oedema was not validated (indirectness of the outcome) or blinded to the intervention (risk of bias).
e If there are very few or no events and the number of participants is large, judgement about the certainty of evidence (particularly judgements about imprecision) may be based on the absolute effect. Here the certainty rating may be considered ‘high’ if the outcome was appropriately assessed and the event, in fact, did not occur in 2821 studied participants.
f None of the other studies reported adverse effects, apart from four cases of superficial vein thrombosis in varicose veins in the knee region that were compressed by the upper edge of the stocking in one study.
Figure 14.1.b Example of alternative ‘Summary of findings’ table
14.1.4 Producing ‘Summary of findings’ tables
The GRADE Working Group’s software, GRADEpro GDT ( www.gradepro.org ), including GRADE’s interactive handbook, is available to assist review authors in the preparation of ‘Summary of findings’ tables. GRADEpro can use data on the comparator group risk and the effect estimate (entered by the review authors or imported from files generated in RevMan) to produce the relative effects and absolute risks associated with experimental interventions. In addition, it leads the user through the process of a GRADE assessment, and produces a table that can be used as a standalone table in a review (including by direct import into software such as RevMan or integration with RevMan Web), or an interactive ‘Summary of findings’ table (see help resources in GRADEpro).
14.1.5 Statistical considerations in ‘Summary of findings’ tables
14.1.5.1 dichotomous outcomes.
‘Summary of findings’ tables should include both absolute and relative measures of effect for dichotomous outcomes. Risk ratios, odds ratios and risk differences are different ways of comparing two groups with dichotomous outcome data (see Chapter 6, Section 6.4.1 ). Furthermore, there are two distinct risk ratios, depending on which event (e.g. ‘yes’ or ‘no’) is the focus of the analysis (see Chapter 6, Section 6.4.1.5 ). In the presence of a non-zero intervention effect, any variation across studies in the comparator group risks (i.e. variation in the risk of the event occurring without the intervention of interest, for example in different populations) makes it impossible for more than one of these measures to be truly the same in every study.
It has long been assumed in epidemiology that relative measures of effect are more consistent than absolute measures of effect from one scenario to another. There is empirical evidence to support this assumption (Engels et al 2000, Deeks and Altman 2001, Furukawa et al 2002). For this reason, meta-analyses should generally use either a risk ratio or an odds ratio as a measure of effect (see Chapter 10, Section 10.4.3 ). Correspondingly, a single estimate of relative effect is likely to be a more appropriate summary than a single estimate of absolute effect. If a relative effect is indeed consistent across studies, then different comparator group risks will have different implications for absolute benefit. For instance, if the risk ratio is consistently 0.75, then the experimental intervention would reduce a comparator group risk of 80% to 60% in the intervention group (an absolute risk reduction of 20 percentage points), but would also reduce a comparator group risk of 20% to 15% in the intervention group (an absolute risk reduction of 5 percentage points).
‘Summary of findings’ tables are built around the assumption of a consistent relative effect. It is therefore important to consider the implications of this effect for different comparator group risks (these can be derived or estimated from a number of sources, see Section 14.1.6.3 ), which may require an assessment of the certainty of evidence for prognostic evidence (Spencer et al 2012, Iorio et al 2015). For any comparator group risk, it is possible to estimate a corresponding intervention group risk (i.e. the absolute risk with the intervention) from the meta-analytic risk ratio or odds ratio. Note that the numbers provided in the ‘Corresponding risk’ column are specific to the ‘risks’ in the adjacent column.
For the meta-analytic risk ratio (RR) and assumed comparator risk (ACR) the corresponding intervention risk is obtained as:

As an example, in Figure 14.1.a , the meta-analytic risk ratio for symptomless deep vein thrombosis (DVT) is RR = 0.10 (95% CI 0.04 to 0.26). Assuming a comparator risk of ACR = 10 per 1000 = 0.01, we obtain:

For the meta-analytic odds ratio (OR) and assumed comparator risk, ACR, the corresponding intervention risk is obtained as:

Upper and lower confidence limits for the corresponding intervention risk are obtained by replacing RR or OR by their upper and lower confidence limits, respectively (e.g. replacing 0.10 with 0.04, then with 0.26, in the example). Such confidence intervals do not incorporate uncertainty in the assumed comparator risks.
When dealing with risk ratios, it is critical that the same definition of ‘event’ is used as was used for the meta-analysis. For example, if the meta-analysis focused on ‘death’ (as opposed to survival) as the event, then corresponding risks in the ‘Summary of findings’ table must also refer to ‘death’.
In (rare) circumstances in which there is clear rationale to assume a consistent risk difference in the meta-analysis, in principle it is possible to present this for relevant ‘assumed risks’ and their corresponding risks, and to present the corresponding (different) relative effects for each assumed risk.
The risk difference expresses the difference between the ACR and the corresponding intervention risk (or the difference between the experimental and the comparator intervention).
For the meta-analytic risk ratio (RR) and assumed comparator risk (ACR) the corresponding risk difference is obtained as (note that risks can also be expressed using percentage or percentage points):

As an example, in Figure 14.1.b the meta-analytic risk ratio is 0.41 (95% CI 0.29 to 0.55) for diarrhoea in children less than 5 years of age. Assuming a comparator group risk of 22.3% we obtain:

For the meta-analytic odds ratio (OR) and assumed comparator risk (ACR) the absolute risk difference is obtained as (percentage points):

Upper and lower confidence limits for the absolute risk difference are obtained by re-running the calculation above while replacing RR or OR by their upper and lower confidence limits, respectively (e.g. replacing 0.41 with 0.28, then with 0.55, in the example). Such confidence intervals do not incorporate uncertainty in the assumed comparator risks.
14.1.5.2 Time-to-event outcomes
Time-to-event outcomes measure whether and when a particular event (e.g. death) occurs (van Dalen et al 2007). The impact of the experimental intervention relative to the comparison group on time-to-event outcomes is usually measured using a hazard ratio (HR) (see Chapter 6, Section 6.8.1 ).
A hazard ratio expresses a relative effect estimate. It may be used in various ways to obtain absolute risks and other interpretable quantities for a specific population. Here we describe how to re-express hazard ratios in terms of: (i) absolute risk of event-free survival within a particular period of time; (ii) absolute risk of an event within a particular period of time; and (iii) median time to the event. All methods are built on an assumption of consistent relative effects (i.e. that the hazard ratio does not vary over time).
(i) Absolute risk of event-free survival within a particular period of time Event-free survival (e.g. overall survival) is commonly reported by individual studies. To obtain absolute effects for time-to-event outcomes measured as event-free survival, the summary HR can be used in conjunction with an assumed proportion of patients who are event-free in the comparator group (Tierney et al 2007). This proportion of patients will be specific to a period of time of observation. However, it is not strictly necessary to specify this period of time. For instance, a proportion of 50% of event-free patients might apply to patients with a high event rate observed over 1 year, or to patients with a low event rate observed over 2 years.

As an example, suppose the meta-analytic hazard ratio is 0.42 (95% CI 0.25 to 0.72). Assuming a comparator group risk of event-free survival (e.g. for overall survival people being alive) at 2 years of ACR = 900 per 1000 = 0.9 we obtain:

so that that 956 per 1000 people will be alive with the experimental intervention at 2 years. The derivation of the risk should be explained in a comment or footnote.
(ii) Absolute risk of an event within a particular period of time To obtain this absolute effect, again the summary HR can be used (Tierney et al 2007):

In the example, suppose we assume a comparator group risk of events (e.g. for mortality, people being dead) at 2 years of ACR = 100 per 1000 = 0.1. We obtain:

so that that 44 per 1000 people will be dead with the experimental intervention at 2 years.
(iii) Median time to the event Instead of absolute numbers, the time to the event in the intervention and comparison groups can be expressed as median survival time in months or years. To obtain median survival time the pooled HR can be applied to an assumed median survival time in the comparator group (Tierney et al 2007):

In the example, assuming a comparator group median survival time of 80 months, we obtain:

For all three of these options for re-expressing results of time-to-event analyses, upper and lower confidence limits for the corresponding intervention risk are obtained by replacing HR by its upper and lower confidence limits, respectively (e.g. replacing 0.42 with 0.25, then with 0.72, in the example). Again, as for dichotomous outcomes, such confidence intervals do not incorporate uncertainty in the assumed comparator group risks. This is of special concern for long-term survival with a low or moderate mortality rate and a corresponding high number of censored patients (i.e. a low number of patients under risk and a high censoring rate).
14.1.6 Detailed contents of a ‘Summary of findings’ table
14.1.6.1 table title and header.
The title of each ‘Summary of findings’ table should specify the healthcare question, framed in terms of the population and making it clear exactly what comparison of interventions are made. In Figure 14.1.a , the population is people taking long aeroplane flights, the intervention is compression stockings, and the control is no compression stockings.
The first rows of each ‘Summary of findings’ table should provide the following ‘header’ information:
Patients or population This further clarifies the population (and possibly the subpopulations) of interest and ideally the magnitude of risk of the most crucial adverse outcome at which an intervention is directed. For instance, people on a long-haul flight may be at different risks for DVT; those using selective serotonin reuptake inhibitors (SSRIs) might be at different risk for side effects; while those with atrial fibrillation may be at low (< 1%), moderate (1% to 4%) or high (> 4%) yearly risk of stroke.
Setting This should state any specific characteristics of the settings of the healthcare question that might limit the applicability of the summary of findings to other settings (e.g. primary care in Europe and North America).
Intervention The experimental intervention.
Comparison The comparator intervention (including no specific intervention).
14.1.6.2 Outcomes
The rows of a ‘Summary of findings’ table should include all desirable and undesirable health outcomes (listed in order of importance) that are essential for decision making, up to a maximum of seven outcomes. If there are more outcomes in the review, review authors will need to omit the less important outcomes from the table, and the decision selecting which outcomes are critical or important to the review should be made during protocol development (see Chapter 3 ). Review authors should provide time frames for the measurement of the outcomes (e.g. 90 days or 12 months) and the type of instrument scores (e.g. ranging from 0 to 100).
Note that review authors should include the pre-specified critical and important outcomes in the table whether data are available or not. However, they should be alert to the possibility that the importance of an outcome (e.g. a serious adverse effect) may only become known after the protocol was written or the analysis was carried out, and should take appropriate actions to include these in the ‘Summary of findings’ table.
The ‘Summary of findings’ table can include effects in subgroups of the population for different comparator risks and effect sizes separately. For instance, in Figure 14.1.b effects are presented for children younger and older than 5 years separately. Review authors may also opt to produce separate ‘Summary of findings’ tables for different populations.
Review authors should include serious adverse events, but it might be possible to combine minor adverse events as a single outcome, and describe this in an explanatory footnote (note that it is not appropriate to add events together unless they are independent, that is, a participant who has experienced one adverse event has an unaffected chance of experiencing the other adverse event).
Outcomes measured at multiple time points represent a particular problem. In general, to keep the table simple, review authors should present multiple time points only for outcomes critical to decision making, where either the result or the decision made are likely to vary over time. The remainder should be presented at a common time point where possible.
Review authors can present continuous outcome measures in the ‘Summary of findings’ table and should endeavour to make these interpretable to the target audience. This requires that the units are clear and readily interpretable, for example, days of pain, or frequency of headache, and the name and scale of any measurement tools used should be stated (e.g. a Visual Analogue Scale, ranging from 0 to 100). However, many measurement instruments are not readily interpretable by non-specialist clinicians or patients, for example, points on a Beck Depression Inventory or quality of life score. For these, a more interpretable presentation might involve converting a continuous to a dichotomous outcome, such as >50% improvement (see Chapter 15, Section 15.5 ).
14.1.6.3 Best estimate of risk with comparator intervention
Review authors should provide up to three typical risks for participants receiving the comparator intervention. For dichotomous outcomes, we recommend that these be presented in the form of the number of people experiencing the event per 100 or 1000 people (natural frequency) depending on the frequency of the outcome. For continuous outcomes, this would be stated as a mean or median value of the outcome measured.
Estimated or assumed comparator intervention risks could be based on assessments of typical risks in different patient groups derived from the review itself, individual representative studies in the review, or risks derived from a systematic review of prognosis studies or other sources of evidence which may in turn require an assessment of the certainty for the prognostic evidence (Spencer et al 2012, Iorio et al 2015). Ideally, risks would reflect groups that clinicians can easily identify on the basis of their presenting features.
An explanatory footnote should specify the source or rationale for each comparator group risk, including the time period to which it corresponds where appropriate. In Figure 14.1.a , clinicians can easily differentiate individuals with risk factors for deep venous thrombosis from those without. If there is known to be little variation in baseline risk then review authors may use the median comparator group risk across studies. If typical risks are not known, an option is to choose the risk from the included studies, providing the second highest for a high and the second lowest for a low risk population.
14.1.6.4 Risk with intervention
For dichotomous outcomes, review authors should provide a corresponding absolute risk for each comparator group risk, along with a confidence interval. This absolute risk with the (experimental) intervention will usually be derived from the meta-analysis result presented in the relative effect column (see Section 14.1.6.6 ). Formulae are provided in Section 14.1.5 . Review authors should present the absolute effect in the same format as the risks with comparator intervention (see Section 14.1.6.3 ), for example as the number of people experiencing the event per 1000 people.
For continuous outcomes, a difference in means or standardized difference in means should be presented with its confidence interval. These will typically be obtained directly from a meta-analysis. Explanatory text should be used to clarify the meaning, as in Figures 14.1.a and 14.1.b .
14.1.6.5 Risk difference
For dichotomous outcomes, the risk difference can be provided using one of the ‘Summary of findings’ table formats as an additional option (see Figure 14.1.b ). This risk difference expresses the difference between the experimental and comparator intervention and will usually be derived from the meta-analysis result presented in the relative effect column (see Section 14.1.6.6 ). Formulae are provided in Section 14.1.5 . Review authors should present the risk difference in the same format as assumed and corresponding risks with comparator intervention (see Section 14.1.6.3 ); for example, as the number of people experiencing the event per 1000 people or as percentage points if the assumed and corresponding risks are expressed in percentage.
For continuous outcomes, if the ‘Summary of findings’ table includes this option, the mean difference can be presented here and the ‘corresponding risk’ column left blank (see Figure 14.1.b ).
14.1.6.6 Relative effect (95% CI)
The relative effect will typically be a risk ratio or odds ratio (or occasionally a hazard ratio) with its accompanying 95% confidence interval, obtained from a meta-analysis performed on the basis of the same effect measure. Risk ratios and odds ratios are similar when the comparator intervention risks are low and effects are small, but may differ considerably when comparator group risks increase. The meta-analysis may involve an assumption of either fixed or random effects, depending on what the review authors consider appropriate, and implying that the relative effect is either an estimate of the effect of the intervention, or an estimate of the average effect of the intervention across studies, respectively.
14.1.6.7 Number of participants (studies)
This column should include the number of participants assessed in the included studies for each outcome and the corresponding number of studies that contributed these participants.
14.1.6.8 Certainty of the evidence (GRADE)
Review authors should comment on the certainty of the evidence (also known as quality of the body of evidence or confidence in the effect estimates). Review authors should use the specific evidence grading system developed by the GRADE Working Group (Atkins et al 2004, Guyatt et al 2008, Guyatt et al 2011a), which is described in detail in Section 14.2 . The GRADE approach categorizes the certainty in a body of evidence as ‘high’, ‘moderate’, ‘low’ or ‘very low’ by outcome. This is a result of judgement, but the judgement process operates within a transparent structure. As an example, the certainty would be ‘high’ if the summary were of several randomized trials with low risk of bias, but the rating of certainty becomes lower if there are concerns about risk of bias, inconsistency, indirectness, imprecision or publication bias. Judgements other than of ‘high’ certainty should be made transparent using explanatory footnotes or the ‘Comments’ column in the ‘Summary of findings’ table (see Section 14.1.6.10 ).
14.1.6.9 Comments
The aim of the ‘Comments’ field is to help interpret the information or data identified in the row. For example, this may be on the validity of the outcome measure or the presence of variables that are associated with the magnitude of effect. Important caveats about the results should be flagged here. Not all rows will need comments, and it is best to leave a blank if there is nothing warranting a comment.
14.1.6.10 Explanations
Detailed explanations should be included as footnotes to support the judgements in the ‘Summary of findings’ table, such as the overall GRADE assessment. The explanations should describe the rationale for important aspects of the content. Table 14.1.a lists guidance for useful explanations. Explanations should be concise, informative, relevant, easy to understand and accurate. If explanations cannot be sufficiently described in footnotes, review authors should provide further details of the issues in the Results and Discussion sections of the review.
Table 14.1.a Guidance for providing useful explanations in ‘Summary of findings’ (SoF) tables. Adapted from Santesso et al (2016)
14.2 Assessing the certainty or quality of a body of evidence
14.2.1 the grade approach.
The Grades of Recommendation, Assessment, Development and Evaluation Working Group (GRADE Working Group) has developed a system for grading the certainty of evidence (Schünemann et al 2003, Atkins et al 2004, Schünemann et al 2006, Guyatt et al 2008, Guyatt et al 2011a). Over 100 organizations including the World Health Organization (WHO), the American College of Physicians, the American Society of Hematology (ASH), the Canadian Agency for Drugs and Technology in Health (CADTH) and the National Institutes of Health and Clinical Excellence (NICE) in the UK have adopted the GRADE system ( www.gradeworkinggroup.org ).
Cochrane has also formally adopted this approach, and all Cochrane Reviews should use GRADE to evaluate the certainty of evidence for important outcomes (see MECIR Box 14.2.a ).
MECIR Box 14.2.a Relevant expectations for conduct of intervention reviews
For systematic reviews, the GRADE approach defines the certainty of a body of evidence as the extent to which one can be confident that an estimate of effect or association is close to the quantity of specific interest. Assessing the certainty of a body of evidence involves consideration of within- and across-study risk of bias (limitations in study design and execution or methodological quality), inconsistency (or heterogeneity), indirectness of evidence, imprecision of the effect estimates and risk of publication bias (see Section 14.2.2 ), as well as domains that may increase our confidence in the effect estimate (as described in Section 14.2.3 ). The GRADE system entails an assessment of the certainty of a body of evidence for each individual outcome. Judgements about the domains that determine the certainty of evidence should be described in the results or discussion section and as part of the ‘Summary of findings’ table.
The GRADE approach specifies four levels of certainty ( Figure 14.2.a ). For interventions, including diagnostic and other tests that are evaluated as interventions (Schünemann et al 2008b, Schünemann et al 2008a, Balshem et al 2011, Schünemann et al 2012), the starting point for rating the certainty of evidence is categorized into two types:
- randomized trials; and
- non-randomized studies of interventions (NRSI), including observational studies (including but not limited to cohort studies, and case-control studies, cross-sectional studies, case series and case reports, although not all of these designs are usually included in Cochrane Reviews).
There are many instances in which review authors rely on information from NRSI, in particular to evaluate potential harms (see Chapter 24 ). In addition, review authors can obtain relevant data from both randomized trials and NRSI, with each type of evidence complementing the other (Schünemann et al 2013).
In GRADE, a body of evidence from randomized trials begins with a high-certainty rating while a body of evidence from NRSI begins with a low-certainty rating. The lower rating with NRSI is the result of the potential bias induced by the lack of randomization (i.e. confounding and selection bias).
However, when using the new Risk Of Bias In Non-randomized Studies of Interventions (ROBINS-I) tool (Sterne et al 2016), an assessment tool that covers the risk of bias due to lack of randomization, all studies may start as high certainty of the evidence (Schünemann et al 2018). The approach of starting all study designs (including NRSI) as high certainty does not conflict with the initial GRADE approach of starting the rating of NRSI as low certainty evidence. This is because a body of evidence from NRSI should generally be downgraded by two levels due to the inherent risk of bias associated with the lack of randomization, namely confounding and selection bias. Not downgrading NRSI from high to low certainty needs transparent and detailed justification for what mitigates concerns about confounding and selection bias (Schünemann et al 2018). Very few examples of where not rating down by two levels is appropriate currently exist.
The highest certainty rating is a body of evidence when there are no concerns in any of the GRADE factors listed in Figure 14.2.a . Review authors often downgrade evidence to moderate, low or even very low certainty evidence, depending on the presence of the five factors in Figure 14.2.a . Usually, certainty rating will fall by one level for each factor, up to a maximum of three levels for all factors. If there are very severe problems for any one domain (e.g. when assessing risk of bias, all studies were unconcealed, unblinded and lost over 50% of their patients to follow-up), evidence may fall by two levels due to that factor alone. It is not possible to rate lower than ‘very low certainty’ evidence.
Review authors will generally grade evidence from sound non-randomized studies as low certainty, even if ROBINS-I is used. If, however, such studies yield large effects and there is no obvious bias explaining those effects, review authors may rate the evidence as moderate or – if the effect is large enough – even as high certainty ( Figure 14.2.a ). The very low certainty level is appropriate for, but is not limited to, studies with critical problems and unsystematic clinical observations (e.g. case series or case reports).
Figure 14.2.a Levels of the certainty of a body of evidence in the GRADE approach. *Upgrading criteria are usually applicable to non-randomized studies only (but exceptions exist).
14.2.2 Domains that can lead to decreasing the certainty level of a body of evidence
We now describe in more detail the five reasons (or domains) for downgrading the certainty of a body of evidence for a specific outcome. In each case, if no reason is found for downgrading the evidence, it should be classified as 'no limitation or not serious' (not important enough to warrant downgrading). If a reason is found for downgrading the evidence, it should be classified as 'serious' (downgrading the certainty rating by one level) or 'very serious' (downgrading the certainty grade by two levels). For non-randomized studies assessed with ROBINS-I, rating down by three levels should be classified as 'extremely' serious.
(1) Risk of bias or limitations in the detailed design and implementation
Our confidence in an estimate of effect decreases if studies suffer from major limitations that are likely to result in a biased assessment of the intervention effect. For randomized trials, these methodological limitations include failure to generate a random sequence, lack of allocation sequence concealment, lack of blinding (particularly with subjective outcomes that are highly susceptible to biased assessment), a large loss to follow-up or selective reporting of outcomes. Chapter 8 provides a discussion of study-level assessments of risk of bias in the context of a Cochrane Review, and proposes an approach to assessing the risk of bias for an outcome across studies as ‘Low’ risk of bias, ‘Some concerns’ and ‘High’ risk of bias for randomized trials. Levels of ‘Low’. ‘Moderate’, ‘Serious’ and ‘Critical’ risk of bias arise for non-randomized studies assessed with ROBINS-I ( Chapter 25 ). These assessments should feed directly into this GRADE domain. In particular, ‘Low’ risk of bias would indicate ‘no limitation’; ‘Some concerns’ would indicate either ‘no limitation’ or ‘serious limitation’; and ‘High’ risk of bias would indicate either ‘serious limitation’ or ‘very serious limitation’. ‘Critical’ risk of bias on ROBINS-I would indicate extremely serious limitations in GRADE. Review authors should use their judgement to decide between alternative categories, depending on the likely magnitude of the potential biases.
Every study addressing a particular outcome will differ, to some degree, in the risk of bias. Review authors should make an overall judgement on whether the certainty of evidence for an outcome warrants downgrading on the basis of study limitations. The assessment of study limitations should apply to the studies contributing to the results in the ‘Summary of findings’ table, rather than to all studies that could potentially be included in the analysis. We have argued in Chapter 7, Section 7.6.2 , that the primary analysis should be restricted to studies at low (or low and unclear) risk of bias where possible.
Table 14.2.a presents the judgements that must be made in going from assessments of the risk of bias to judgements about study limitations for each outcome included in a ‘Summary of findings’ table. A rating of high certainty evidence can be achieved only when most evidence comes from studies that met the criteria for low risk of bias. For example, of the 22 studies addressing the impact of beta-blockers on mortality in patients with heart failure, most probably or certainly used concealed allocation of the sequence, all blinded at least some key groups and follow-up of randomized patients was almost complete (Brophy et al 2001). The certainty of evidence might be downgraded by one level when most of the evidence comes from individual studies either with a crucial limitation for one item, or with some limitations for multiple items. An example of very serious limitations, warranting downgrading by two levels, is provided by evidence on surgery versus conservative treatment in the management of patients with lumbar disc prolapse (Gibson and Waddell 2007). We are uncertain of the benefit of surgery in reducing symptoms after one year or longer, because the one study included in the analysis had inadequate concealment of the allocation sequence and the outcome was assessed using a crude rating by the surgeon without blinding.
(2) Unexplained heterogeneity or inconsistency of results
When studies yield widely differing estimates of effect (heterogeneity or variability in results), investigators should look for robust explanations for that heterogeneity. For instance, drugs may have larger relative effects in sicker populations or when given in larger doses. A detailed discussion of heterogeneity and its investigation is provided in Chapter 10, Section 10.10 and Section 10.11 . If an important modifier exists, with good evidence that important outcomes are different in different subgroups (which would ideally be pre-specified), then a separate ‘Summary of findings’ table may be considered for a separate population. For instance, a separate ‘Summary of findings’ table would be used for carotid endarterectomy in symptomatic patients with high grade stenosis (70% to 99%) in which the intervention is, in the hands of the right surgeons, beneficial, and another (if review authors considered it relevant) for asymptomatic patients with low grade stenosis (less than 30%) in which surgery appears harmful (Orrapin and Rerkasem 2017). When heterogeneity exists and affects the interpretation of results, but review authors are unable to identify a plausible explanation with the data available, the certainty of the evidence decreases.
(3) Indirectness of evidence
Two types of indirectness are relevant. First, a review comparing the effectiveness of alternative interventions (say A and B) may find that randomized trials are available, but they have compared A with placebo and B with placebo. Thus, the evidence is restricted to indirect comparisons between A and B. Where indirect comparisons are undertaken within a network meta-analysis context, GRADE for network meta-analysis should be used (see Chapter 11, Section 11.5 ).
Second, a review may find randomized trials that meet eligibility criteria but address a restricted version of the main review question in terms of population, intervention, comparator or outcomes. For example, suppose that in a review addressing an intervention for secondary prevention of coronary heart disease, most identified studies happened to be in people who also had diabetes. Then the evidence may be regarded as indirect in relation to the broader question of interest because the population is primarily related to people with diabetes. The opposite scenario can equally apply: a review addressing the effect of a preventive strategy for coronary heart disease in people with diabetes may consider studies in people without diabetes to provide relevant, albeit indirect, evidence. This would be particularly likely if investigators had conducted few if any randomized trials in the target population (e.g. people with diabetes). Other sources of indirectness may arise from interventions studied (e.g. if in all included studies a technical intervention was implemented by expert, highly trained specialists in specialist centres, then evidence on the effects of the intervention outside these centres may be indirect), comparators used (e.g. if the comparator groups received an intervention that is less effective than standard treatment in most settings) and outcomes assessed (e.g. indirectness due to surrogate outcomes when data on patient-important outcomes are not available, or when investigators seek data on quality of life but only symptoms are reported). Review authors should make judgements transparent when they believe downgrading is justified, based on differences in anticipated effects in the group of primary interest. Review authors may be aided and increase transparency of their judgements about indirectness if they use Table 14.2.b available in the GRADEpro GDT software (Schünemann et al 2013).
(4) Imprecision of results
When studies include few participants or few events, and thus have wide confidence intervals, review authors can lower their rating of the certainty of the evidence. The confidence intervals included in the ‘Summary of findings’ table will provide readers with information that allows them to make, to some extent, their own rating of precision. Review authors can use a calculation of the optimal information size (OIS) or review information size (RIS), similar to sample size calculations, to make judgements about imprecision (Guyatt et al 2011b, Schünemann 2016). The OIS or RIS is calculated on the basis of the number of participants required for an adequately powered individual study. If the 95% confidence interval excludes a risk ratio (RR) of 1.0, and the total number of events or patients exceeds the OIS criterion, precision is adequate. If the 95% CI includes appreciable benefit or harm (an RR of under 0.75 or over 1.25 is often suggested as a very rough guide) downgrading for imprecision may be appropriate even if OIS criteria are met (Guyatt et al 2011b, Schünemann 2016).
(5) High probability of publication bias
The certainty of evidence level may be downgraded if investigators fail to report studies on the basis of results (typically those that show no effect: publication bias) or outcomes (typically those that may be harmful or for which no effect was observed: selective outcome non-reporting bias). Selective reporting of outcomes from among multiple outcomes measured is assessed at the study level as part of the assessment of risk of bias (see Chapter 8, Section 8.7 ), so for the studies contributing to the outcome in the ‘Summary of findings’ table this is addressed by domain 1 above (limitations in the design and implementation). If a large number of studies included in the review do not contribute to an outcome, or if there is evidence of publication bias, the certainty of the evidence may be downgraded. Chapter 13 provides a detailed discussion of reporting biases, including publication bias, and how it may be tackled in a Cochrane Review. A prototypical situation that may elicit suspicion of publication bias is when published evidence includes a number of small studies, all of which are industry-funded (Bhandari et al 2004). For example, 14 studies of flavanoids in patients with haemorrhoids have shown apparent large benefits, but enrolled a total of only 1432 patients (i.e. each study enrolled relatively few patients) (Alonso-Coello et al 2006). The heavy involvement of sponsors in most of these studies raises questions of whether unpublished studies that suggest no benefit exist (publication bias).
A particular body of evidence can suffer from problems associated with more than one of the five factors listed here, and the greater the problems, the lower the certainty of evidence rating that should result. One could imagine a situation in which randomized trials were available, but all or virtually all of these limitations would be present, and in serious form. A very low certainty of evidence rating would result.
Table 14.2.a Further guidelines for domain 1 (of 5) in a GRADE assessment: going from assessments of risk of bias in studies to judgements about study limitations for main outcomes across studies
Table 14.2.b Judgements about indirectness by outcome (available in GRADEpro GDT)
Intervention:
Comparator:
Direct comparison:
Final judgement about indirectness across domains:
14.2.3 Domains that may lead to increasing the certainty level of a body of evidence
Although NRSI and downgraded randomized trials will generally yield a low rating for certainty of evidence, there will be unusual circumstances in which review authors could ‘upgrade’ such evidence to moderate or even high certainty ( Table 14.3.a ).
- Large effects On rare occasions when methodologically well-done observational studies yield large, consistent and precise estimates of the magnitude of an intervention effect, one may be particularly confident in the results. A large estimated effect (e.g. RR >2 or RR <0.5) in the absence of plausible confounders, or a very large effect (e.g. RR >5 or RR <0.2) in studies with no major threats to validity, might qualify for this. In these situations, while the NRSI may possibly have provided an over-estimate of the true effect, the weak study design may not explain all of the apparent observed benefit. Thus, despite reservations based on the observational study design, review authors are confident that the effect exists. The magnitude of the effect in these studies may move the assigned certainty of evidence from low to moderate (if the effect is large in the absence of other methodological limitations). For example, a meta-analysis of observational studies showed that bicycle helmets reduce the risk of head injuries in cyclists by a large margin (odds ratio (OR) 0.31, 95% CI 0.26 to 0.37) (Thompson et al 2000). This large effect, in the absence of obvious bias that could create the association, suggests a rating of moderate-certainty evidence. Note : GRADE guidance suggests the possibility of rating up one level for a large effect if the relative effect is greater than 2.0. However, if the point estimate of the relative effect is greater than 2.0, but the confidence interval is appreciably below 2.0, then some hesitation would be appropriate in the decision to rate up for a large effect. Another situation allows inference of a strong association without a formal comparative study. Consider the question of the impact of routine colonoscopy versus no screening for colon cancer on the rate of perforation associated with colonoscopy. Here, a large series of representative patients undergoing colonoscopy may provide high certainty evidence about the risk of perforation associated with colonoscopy. When the risk of the event among patients receiving the relevant comparator is known to be near 0 (i.e. we are certain that the incidence of spontaneous colon perforation in patients not undergoing colonoscopy is extremely low), case series or cohort studies of representative patients can provide high certainty evidence of adverse effects associated with an intervention, thereby allowing us to infer a strong association from even a limited number of events.
- Dose-response The presence of a dose-response gradient may increase our confidence in the findings of observational studies and thereby enhance the assigned certainty of evidence. For example, our confidence in the result of observational studies that show an increased risk of bleeding in patients who have supratherapeutic anticoagulation levels is increased by the observation that there is a dose-response gradient between the length of time needed for blood to clot (as measured by the international normalized ratio (INR)) and an increased risk of bleeding (Levine et al 2004). A systematic review of NRSI investigating the effect of cyclooxygenase-2 inhibitors on cardiovascular events found that the summary estimate (RR) with rofecoxib was 1.33 (95% CI 1.00 to 1.79) with doses less than 25mg/d, and 2.19 (95% CI 1.64 to 2.91) with doses more than 25mg/d. Although residual confounding is likely to exist in the NRSI that address this issue, the existence of a dose-response gradient and the large apparent effect of higher doses of rofecoxib markedly increase our strength of inference that the association cannot be explained by residual confounding, and is therefore likely to be both causal and, at high levels of exposure, substantial. Note : GRADE guidance suggests the possibility of rating up one level for a large effect if the relative effect is greater than 2.0. Here, the fact that the point estimate of the relative effect is greater than 2.0, but the confidence interval is appreciably below 2.0 might make some hesitate in the decision to rate up for a large effect
- Plausible confounding On occasion, all plausible biases from randomized or non-randomized studies may be working to under-estimate an apparent intervention effect. For example, if only sicker patients receive an experimental intervention or exposure, yet they still fare better, it is likely that the actual intervention or exposure effect is larger than the data suggest. For instance, a rigorous systematic review of observational studies including a total of 38 million patients demonstrated higher death rates in private for-profit versus private not-for-profit hospitals (Devereaux et al 2002). One possible bias relates to different disease severity in patients in the two hospital types. It is likely, however, that patients in the not-for-profit hospitals were sicker than those in the for-profit hospitals. Thus, to the extent that residual confounding existed, it would bias results against the not-for-profit hospitals. The second likely bias was the possibility that higher numbers of patients with excellent private insurance coverage could lead to a hospital having more resources and a spill-over effect that would benefit those without such coverage. Since for-profit hospitals are likely to admit a larger proportion of such well-insured patients than not-for-profit hospitals, the bias is once again against the not-for-profit hospitals. Since the plausible biases would all diminish the demonstrated intervention effect, one might consider the evidence from these observational studies as moderate rather than low certainty. A parallel situation exists when observational studies have failed to demonstrate an association, but all plausible biases would have increased an intervention effect. This situation will usually arise in the exploration of apparent harmful effects. For example, because the hypoglycaemic drug phenformin causes lactic acidosis, the related agent metformin was under suspicion for the same toxicity. Nevertheless, very large observational studies have failed to demonstrate an association (Salpeter et al 2007). Given the likelihood that clinicians would be more alert to lactic acidosis in the presence of the agent and over-report its occurrence, one might consider this moderate, or even high certainty, evidence refuting a causal relationship between typical therapeutic doses of metformin and lactic acidosis.
14.3 Describing the assessment of the certainty of a body of evidence using the GRADE framework
Review authors should report the grading of the certainty of evidence in the Results section for each outcome for which this has been performed, providing the rationale for downgrading or upgrading the evidence, and referring to the ‘Summary of findings’ table where applicable.
Table 14.3.a provides a framework and examples for how review authors can justify their judgements about the certainty of evidence in each domain. These justifications should also be included in explanatory notes to the ‘Summary of Findings’ table (see Section 14.1.6.10 ).
Chapter 15, Section 15.6 , describes in more detail how the overall GRADE assessment across all domains can be used to draw conclusions about the effects of the intervention, as well as providing implications for future research.
Table 14.3.a Framework for describing the certainty of evidence and justifying downgrading or upgrading
14.4 Chapter information
Authors: Holger J Schünemann, Julian PT Higgins, Gunn E Vist, Paul Glasziou, Elie A Akl, Nicole Skoetz, Gordon H Guyatt; on behalf of the Cochrane GRADEing Methods Group (formerly Applicability and Recommendations Methods Group) and the Cochrane Statistical Methods Group
Acknowledgements: Andrew D Oxman contributed to earlier versions. Professor Penny Hawe contributed to the text on adverse effects in earlier versions. Jon Deeks provided helpful contributions on an earlier version of this chapter. For details of previous authors and editors of the Handbook , please refer to the Preface.
Funding: This work was in part supported by funding from the Michael G DeGroote Cochrane Canada Centre and the Ontario Ministry of Health.
14.5 References
Alonso-Coello P, Zhou Q, Martinez-Zapata MJ, Mills E, Heels-Ansdell D, Johanson JF, Guyatt G. Meta-analysis of flavonoids for the treatment of haemorrhoids. British Journal of Surgery 2006; 93 : 909-920.
Atkins D, Best D, Briss PA, Eccles M, Falck-Ytter Y, Flottorp S, Guyatt GH, Harbour RT, Haugh MC, Henry D, Hill S, Jaeschke R, Leng G, Liberati A, Magrini N, Mason J, Middleton P, Mrukowicz J, O'Connell D, Oxman AD, Phillips B, Schünemann HJ, Edejer TT, Varonen H, Vist GE, Williams JW, Jr., Zaza S. Grading quality of evidence and strength of recommendations. BMJ 2004; 328 : 1490.
Balshem H, Helfand M, Schünemann HJ, Oxman AD, Kunz R, Brozek J, Vist GE, Falck-Ytter Y, Meerpohl J, Norris S, Guyatt GH. GRADE guidelines: 3. Rating the quality of evidence. Journal of Clinical Epidemiology 2011; 64 : 401-406.
Bhandari M, Busse JW, Jackowski D, Montori VM, Schünemann H, Sprague S, Mears D, Schemitsch EH, Heels-Ansdell D, Devereaux PJ. Association between industry funding and statistically significant pro-industry findings in medical and surgical randomized trials. Canadian Medical Association Journal 2004; 170 : 477-480.
Brophy JM, Joseph L, Rouleau JL. Beta-blockers in congestive heart failure. A Bayesian meta-analysis. Annals of Internal Medicine 2001; 134 : 550-560.
Carrasco-Labra A, Brignardello-Petersen R, Santesso N, Neumann I, Mustafa RA, Mbuagbaw L, Etxeandia Ikobaltzeta I, De Stio C, McCullagh LJ, Alonso-Coello P, Meerpohl JJ, Vandvik PO, Brozek JL, Akl EA, Bossuyt P, Churchill R, Glenton C, Rosenbaum S, Tugwell P, Welch V, Garner P, Guyatt G, Schünemann HJ. Improving GRADE evidence tables part 1: a randomized trial shows improved understanding of content in summary of findings tables with a new format. Journal of Clinical Epidemiology 2016; 74 : 7-18.
Deeks JJ, Altman DG. Effect measures for meta-analysis of trials with binary outcomes. In: Egger M, Davey Smith G, Altman DG, editors. Systematic Reviews in Health Care: Meta-analysis in Context . 2nd ed. London (UK): BMJ Publication Group; 2001. p. 313-335.
Devereaux PJ, Choi PT, Lacchetti C, Weaver B, Schünemann HJ, Haines T, Lavis JN, Grant BJ, Haslam DR, Bhandari M, Sullivan T, Cook DJ, Walter SD, Meade M, Khan H, Bhatnagar N, Guyatt GH. A systematic review and meta-analysis of studies comparing mortality rates of private for-profit and private not-for-profit hospitals. Canadian Medical Association Journal 2002; 166 : 1399-1406.
Engels EA, Schmid CH, Terrin N, Olkin I, Lau J. Heterogeneity and statistical significance in meta-analysis: an empirical study of 125 meta-analyses. Statistics in Medicine 2000; 19 : 1707-1728.
Furukawa TA, Guyatt GH, Griffith LE. Can we individualize the 'number needed to treat'? An empirical study of summary effect measures in meta-analyses. International Journal of Epidemiology 2002; 31 : 72-76.
Gibson JN, Waddell G. Surgical interventions for lumbar disc prolapse: updated Cochrane Review. Spine 2007; 32 : 1735-1747.
Guyatt G, Oxman A, Vist G, Kunz R, Falck-Ytter Y, Alonso-Coello P, Schünemann H. GRADE: an emerging consensus on rating quality of evidence and strength of recommendations. BMJ 2008; 336 : 3.
Guyatt G, Oxman AD, Akl EA, Kunz R, Vist G, Brozek J, Norris S, Falck-Ytter Y, Glasziou P, DeBeer H, Jaeschke R, Rind D, Meerpohl J, Dahm P, Schünemann HJ. GRADE guidelines: 1. Introduction-GRADE evidence profiles and summary of findings tables. Journal of Clinical Epidemiology 2011a; 64 : 383-394.
Guyatt GH, Oxman AD, Kunz R, Brozek J, Alonso-Coello P, Rind D, Devereaux PJ, Montori VM, Freyschuss B, Vist G, Jaeschke R, Williams JW, Jr., Murad MH, Sinclair D, Falck-Ytter Y, Meerpohl J, Whittington C, Thorlund K, Andrews J, Schünemann HJ. GRADE guidelines 6. Rating the quality of evidence--imprecision. Journal of Clinical Epidemiology 2011b; 64 : 1283-1293.
Iorio A, Spencer FA, Falavigna M, Alba C, Lang E, Burnand B, McGinn T, Hayden J, Williams K, Shea B, Wolff R, Kujpers T, Perel P, Vandvik PO, Glasziou P, Schünemann H, Guyatt G. Use of GRADE for assessment of evidence about prognosis: rating confidence in estimates of event rates in broad categories of patients. BMJ 2015; 350 : h870.
Langendam M, Carrasco-Labra A, Santesso N, Mustafa RA, Brignardello-Petersen R, Ventresca M, Heus P, Lasserson T, Moustgaard R, Brozek J, Schünemann HJ. Improving GRADE evidence tables part 2: a systematic survey of explanatory notes shows more guidance is needed. Journal of Clinical Epidemiology 2016; 74 : 19-27.
Levine MN, Raskob G, Landefeld S, Kearon C, Schulman S. Hemorrhagic complications of anticoagulant treatment: the Seventh ACCP Conference on Antithrombotic and Thrombolytic Therapy. Chest 2004; 126 : 287S-310S.
Orrapin S, Rerkasem K. Carotid endarterectomy for symptomatic carotid stenosis. Cochrane Database of Systematic Reviews 2017; 6 : CD001081.
Salpeter S, Greyber E, Pasternak G, Salpeter E. Risk of fatal and nonfatal lactic acidosis with metformin use in type 2 diabetes mellitus. Cochrane Database of Systematic Reviews 2007; 4 : CD002967.
Santesso N, Carrasco-Labra A, Langendam M, Brignardello-Petersen R, Mustafa RA, Heus P, Lasserson T, Opiyo N, Kunnamo I, Sinclair D, Garner P, Treweek S, Tovey D, Akl EA, Tugwell P, Brozek JL, Guyatt G, Schünemann HJ. Improving GRADE evidence tables part 3: detailed guidance for explanatory footnotes supports creating and understanding GRADE certainty in the evidence judgments. Journal of Clinical Epidemiology 2016; 74 : 28-39.
Schünemann HJ, Best D, Vist G, Oxman AD, Group GW. Letters, numbers, symbols and words: how to communicate grades of evidence and recommendations. Canadian Medical Association Journal 2003; 169 : 677-680.
Schünemann HJ, Jaeschke R, Cook DJ, Bria WF, El-Solh AA, Ernst A, Fahy BF, Gould MK, Horan KL, Krishnan JA, Manthous CA, Maurer JR, McNicholas WT, Oxman AD, Rubenfeld G, Turino GM, Guyatt G. An official ATS statement: grading the quality of evidence and strength of recommendations in ATS guidelines and recommendations. American Journal of Respiratory and Critical Care Medicine 2006; 174 : 605-614.
Schünemann HJ, Oxman AD, Brozek J, Glasziou P, Jaeschke R, Vist GE, Williams JW, Jr., Kunz R, Craig J, Montori VM, Bossuyt P, Guyatt GH. Grading quality of evidence and strength of recommendations for diagnostic tests and strategies. BMJ 2008a; 336 : 1106-1110.
Schünemann HJ, Oxman AD, Brozek J, Glasziou P, Bossuyt P, Chang S, Muti P, Jaeschke R, Guyatt GH. GRADE: assessing the quality of evidence for diagnostic recommendations. ACP Journal Club 2008b; 149 : 2.
Schünemann HJ, Mustafa R, Brozek J. [Diagnostic accuracy and linked evidence--testing the chain]. Zeitschrift für Evidenz, Fortbildung und Qualität im Gesundheitswesen 2012; 106 : 153-160.
Schünemann HJ, Tugwell P, Reeves BC, Akl EA, Santesso N, Spencer FA, Shea B, Wells G, Helfand M. Non-randomized studies as a source of complementary, sequential or replacement evidence for randomized controlled trials in systematic reviews on the effects of interventions. Research Synthesis Methods 2013; 4 : 49-62.
Schünemann HJ. Interpreting GRADE's levels of certainty or quality of the evidence: GRADE for statisticians, considering review information size or less emphasis on imprecision? Journal of Clinical Epidemiology 2016; 75 : 6-15.
Schünemann HJ, Cuello C, Akl EA, Mustafa RA, Meerpohl JJ, Thayer K, Morgan RL, Gartlehner G, Kunz R, Katikireddi SV, Sterne J, Higgins JPT, Guyatt G, Group GW. GRADE guidelines: 18. How ROBINS-I and other tools to assess risk of bias in nonrandomized studies should be used to rate the certainty of a body of evidence. Journal of Clinical Epidemiology 2018.
Spencer-Bonilla G, Quinones AR, Montori VM, International Minimally Disruptive Medicine W. Assessing the Burden of Treatment. Journal of General Internal Medicine 2017; 32 : 1141-1145.
Spencer FA, Iorio A, You J, Murad MH, Schünemann HJ, Vandvik PO, Crowther MA, Pottie K, Lang ES, Meerpohl JJ, Falck-Ytter Y, Alonso-Coello P, Guyatt GH. Uncertainties in baseline risk estimates and confidence in treatment effects. BMJ 2012; 345 : e7401.
Sterne JAC, Hernán MA, Reeves BC, Savović J, Berkman ND, Viswanathan M, Henry D, Altman DG, Ansari MT, Boutron I, Carpenter JR, Chan AW, Churchill R, Deeks JJ, Hróbjartsson A, Kirkham J, Jüni P, Loke YK, Pigott TD, Ramsay CR, Regidor D, Rothstein HR, Sandhu L, Santaguida PL, Schünemann HJ, Shea B, Shrier I, Tugwell P, Turner L, Valentine JC, Waddington H, Waters E, Wells GA, Whiting PF, Higgins JPT. ROBINS-I: a tool for assessing risk of bias in non-randomised studies of interventions. BMJ 2016; 355 : i4919.
Thompson DC, Rivara FP, Thompson R. Helmets for preventing head and facial injuries in bicyclists. Cochrane Database of Systematic Reviews 2000; 2 : CD001855.
Tierney JF, Stewart LA, Ghersi D, Burdett S, Sydes MR. Practical methods for incorporating summary time-to-event data into meta-analysis. Trials 2007; 8 .
van Dalen EC, Tierney JF, Kremer LCM. Tips and tricks for understanding and using SR results. No. 7: time‐to‐event data. Evidence-Based Child Health 2007; 2 : 1089-1090.
For permission to re-use material from the Handbook (either academic or commercial), please see here for full details.

An official website of the United States government
The .gov means it’s official. Federal government websites often end in .gov or .mil. Before sharing sensitive information, make sure you’re on a federal government site.
The site is secure. The https:// ensures that you are connecting to the official website and that any information you provide is encrypted and transmitted securely.
- Publications
- Account settings
Preview improvements coming to the PMC website in October 2024. Learn More or Try it out now .
- Advanced Search
- Journal List
- J Clin Transl Sci
- v.4(3); 2020 Jun

Communicating and disseminating research findings to study participants: Formative assessment of participant and researcher expectations and preferences
Cathy l. melvin.
1 College of Medicine, Medical University of South Carolina, Charleston, SC, USA
Jillian Harvey
2 College of Health Professions/Healthcare Leadership & Management, Medical University of South Carolina, Charleston, SC, USA
Tara Pittman
3 South Carolina Clinical & Translational Research Institute (CTSA), Medical University of South Carolina, Charleston, SC, USA
Stephanie Gentilin
Dana burshell.
4 SOGI-SES Add Health Study Carolina Population Center, University of North Carolina at Chapel Hill, Chapel Hill, NC, USA
Teresa Kelechi
5 College of Nursing, Medical University of South Carolina, Charleston, SC, USA
Introduction:
Translating research findings into practice requires understanding how to meet communication and dissemination needs and preferences of intended audiences including past research participants (PSPs) who want, but seldom receive, information on research findings during or after participating in research studies. Most researchers want to let others, including PSP, know about their findings but lack knowledge about how to effectively communicate findings to a lay audience.
We designed a two-phase, mixed methods pilot study to understand experiences, expectations, concerns, preferences, and capacities of researchers and PSP in two age groups (adolescents/young adults (AYA) or older adults) and to test communication prototypes for sharing, receiving, and using information on research study findings.
Principal Results:
PSP and researchers agreed that sharing study findings should happen and that doing so could improve participant recruitment and enrollment, use of research findings to improve health and health-care delivery, and build community support for research. Some differences and similarities in communication preferences and message format were identified between PSP groups, reinforcing the best practice of customizing communication channel and messaging. Researchers wanted specific training and/or time and resources to help them prepare messages in formats to meet PSP needs and preferences but were unaware of resources to help them do so.
Conclusions:
Our findings offer insight into how to engage both PSP and researchers in the design and use of strategies to share research findings and highlight the need to develop services and support for researchers as they aim to bridge this translational barrier.
Introduction
Since 2006, the National Institutes of Health Clinical and Translational Science Awards (CTSA) have aimed to advance science and translate knowledge into evidence that, if implemented, helps patients and providers make more informed decisions with the potential to improve health care and health outcomes [ 1 , 2 ]. This aim responded to calls by leaders in the fields of comparative effectiveness research, clinical trials, research ethics, and community engagement to assure that results of clinical trials were made available to participants and suggesting that providing participants with results both positive and negative should be the “ethical norm” [ 1 , 3 ]. Others noted that
on the surface, the concept of providing clinical trial results might seem straightforward but putting such a plan into action will be much more complicated. Communication with patients following participation in a clinical trial represents an important and often overlooked aspect of the patient-physician relationship. Careful exploration of this issue, both from the patient and clinician-researcher perspective, is warranted [ 4 ].
Authors also noted that no systematic approach to operationalizing this “ethical norm” existed and that evidence was lacking to describe either positive or negative outcomes of sharing clinical trial results with study participants and the community [ 4 ]. It was generally assumed, but not supported by research, that sharing would result in better patient–physician/researcher communication, improvement in patient care and satisfaction with care, better patient/participant understanding of clinical trials, and enhanced clinical trial accrual [ 4 ].
More recent literature informs these processes but also raises unresolved concerns about the communication and dissemination of research results. A 2008 narrative review of available data on the effects of communicating aggregate and individual research showed that
- research participants want aggregate and clinically significant individual study results made available to them despite the transient distress that communication of results sometimes elicits [ 3 , 5 ]. While differing in their preferences for specific channels of communication, they indicated that not sharing results fostered lack of participant trust in the health-care system, providers, and researchers [ 6 ] and an adverse impact on trial participation [ 5 ];
- investigators recognized their ethical obligation to at least offer to share research findings with recipients and the nonacademic community but differed on whether they should proactively re-contact participants, the type of results to be offered to participants, the need for clinical relevance before disclosure, and the stage at which research results should be offered [ 5 ]. They also reported not being well versed in communication and dissemination strategies known to be effective and not having funding sources to implement proven strategies for sharing with specific audiences [ 5 ];
- members of the research enterprise noted that while public opinion regarding participation in clinical trials is positive, clinical trial accrual remains low and that the failure to provide information about study results may be one of many factors negatively affecting accrual. They also called for better understanding of physician–researcher and patient attitudes and preferences and posit that development of effective mechanisms to share trial results with study participants should enhance patient–physician communication and improve clinical care and research processes [ 5 ].
A 2010 survey of CTSAs found that while professional and scientific audiences are currently the primary focus for communicating and disseminating research findings, it is equally vital to develop approaches for sharing research findings with other audiences, including individuals who participate in clinical trials [ 1 , 5 ]. Effective communication and dissemination strategies are documented in the literature [ 6 , 7 ], but most are designed to promote adoption of evidence-based interventions and lack of applicability to participants overall, especially to participants who are members of special populations and underrepresented minorities who have fewer opportunities to participate in research and whose preferences for receiving research findings are unknown [ 7 ].
Researchers often have limited exposure to methods that offer them guidance in communicating and disseminating study findings in ways likely to improve awareness, adoption, and use of their findings [ 7 ]. Researchers also lack expertise in using communication channels such as traditional journalism platforms, live or face-to-face events such as public festivals, lectures, and panels, and online interactions [ 8 ]. Few strategies provide guidance for researchers about how to develop communications that are patient-centered, contain plain language, create awareness of the influence of findings on participant or population health, and increase the likelihood of enrollment in future studies.
Consequently, researchers often rely on traditional methods (e.g., presentations at scientific meetings and publication of study findings in peer-reviewed journals) despite evidence suggesting their limited reach and/or impact among professional/scientific and/or lay audiences [ 9 , 10 ].
Input from stakeholders can enhance our understanding of how to assure that participants will receive understandable, useful information about research findings and, as appropriate, interpret and use this information to inform their decisions about changing health behaviors, interacting with their health-care providers, enrolling in future research studies, sharing their study experiences with others, or recommending to others that they participate in studies.
Purpose and Goal
This pilot project was undertaken to address issues cited above and in response to expressed concerns of community members in our area about not receiving information on research studies in which they participated. The project design, a two-phase, mixed methods pilot study, was informed by their subsequent participation in a committee of community-academic representatives to determine possible options for improving the communication and dissemination of study results to both study participants and the community at large.
Our goals were to understand the experiences, expectations, concerns, preferences, and capacities of researchers and past research participants (PSP) in two age groups (adolescents/young adults (AYA) aged 15–25 years and older adults aged 50 years or older) and to test communication prototypes for sharing, receiving, and using information on research study findings. Our long-term objectives are to stimulate new, interdisciplinary collaborative research and to develop resources to meet PSP and researcher needs.
This study was conducted in an academic medical center located in south-eastern South Carolina. Phase one consisted of surveying PSP and researchers. In phase two, in-person focus groups were conducted among PSP completing the survey and one-on-one interviews were conducted among researchers. Participants in either the interviews or focus groups responded to a set of questions from a discussion guide developed by the study team and reviewed three prototypes for communicating and disseminating study results developed by the study team in response to PSP and researcher survey responses: a study results letter, a study results email, and a web-based communication – Mail Chimp (Figs. 1 – 3 ).

Prototype 1: study results email prototype. MUSC, Medical University of South Carolina.

Prototype 3: study results MailChimp prototypes 1 and 2. MUSC, Medical University of South Carolina.

Prototype 2: study results letter prototype.
PSP and researcher surveys
A 42-item survey questionnaire representing seven domains was developed by a multidisciplinary team of clinicians, researchers, and PSP that evaluated the questions for content, ease of understanding, usefulness, and comprehensiveness [ 11 ]. Project principal investigators reviewed questions for content and clarity [ 11 ]. The PSP and researcher surveys contained screening and demographic questions to determine participant eligibility and participant characteristics. The PSP survey assessed prior experience with research, receipt of study information from the research team, intention to participate in future research, and preferences and opinions about receipt of information about study findings and next steps. Specific questions for PSP elicited their preferences for communication channels such as phone call, email, social or mass media, and public forum and included channels unique to South Carolina, such as billboards. PSP were asked to rank their preferences and experiences regarding receipt of study results using a Likert scale with the following measurements: “not at all interested” (0), “not very interested” (1), “neutral” (3), “somewhat interested” (3), and “very interested” (4).
The researcher survey contained questions about researcher decisions, plans, and actions regarding communication and dissemination of research results for a recently completed study. Items included knowledge and opinions about how to communicate and disseminate research findings, resources used and needed to develop communication strategies, and awareness and use of dissemination channels, message development, and presentation format.
A research team member administered the survey to PSP and researchers either in person or via phone. Researchers could also complete the survey online through Research Electronic Data Capture (REDCap©).
Focus groups and discussion guide content
The PSP focus group discussion guide contained questions to assess participants’ past experiences with receiving information about research findings; identify participant preferences for receiving research findings whether negative, positive, or equivocal; gather information to improve communication of research results back to participants; assess participant intention to enroll in future research studies, to share their study experiences with others, and to refer others to our institution for study participation; and provide comments and suggestions on prototypes developed for communication and dissemination of study results. Five AYA participated in one focus group, and 11 older adults participated in one focus group. Focus groups were conducted in an off-campus location with convenient parking and at times convenient for participants. Snacks and beverages were provided.
The researcher interview guide was designed to understand researchers’ perspectives on communicating and disseminating research findings to participants; explore past experiences, if any, of researchers with communication and dissemination of research findings to study participants; document any approaches researchers may have used or intend to use to communicate and disseminate research findings to study participants; assess researcher expectations of benefits associated with sharing findings with participants, as well as, perceived and actual barriers to sharing findings; and provide comments and suggestions on prototypes developed for communication and dissemination of study results.
Prototype materials
Three prototypes were presented to focus group participants and included (1) a formal letter on hospital letterhead designed to be delivered by standard mail, describing the purpose and findings of a fictional study and thanking the individual for his/her participation, (2) a text-only email including a brief thank you and a summary of major findings with a link to a study website for more information, and (3) an email formatted like a newsletter with detailed information on study purpose, method, and findings with graphics to help convey results. A mock study website was shown and included information about study background, purpose, methods, results, as well as, links to other research and health resources. Prototypes were presented either in paper or PowerPoint format during the focus groups and explained by a study team member who then elicited participant input using the focus group guide. Researchers also reviewed and commented on prototype content and format in one-on-one interviews with a study team member.
Protection of Human Subjects
The study protocol (No. Pro00067659) was submitted to and approved by the Institutional Review Board at the Medical University of South Carolina in 2017. PSP (or the caretakers for PSP under age 18), and researchers provided verbal informed consent prior to completing the survey or participating in either a focus group or interview. Participants received a verbal introduction prior to participating in each phase.
Recruitment and Interview Procedures
Past study participants.
A study team member reviewed study participant logs from five recently completed studies at our institution involving AYA or older adults to identify individuals who provided consent for contact regarding future studies. Subsequent PSP recruitment efforts based on these searches were consistent with previous contact preferences recorded in each study participant’s consent indicating desire to be re-contacted. The primary modes of contact were phone/SMS and email.
Efforts to recruit other PSP were made through placement of flyers in frequented public locations such as coffee shops, recreation complexes, and college campuses and through social media, Yammer, and newsletters. ResearchMatch, a web-based recruitment tool, was used to alert its subscribers about the study. Potential participants reached by these methods contacted our study team to learn more about the study, and if interested and pre-screened eligible, volunteered and were consented for the study. PSP completing the survey indicated willingness to share experiences with the study team in a focus group and were re-contacted to participate in focus groups.
Researcher recruitment
Researchers were identified through informal outreach by study investigators and staff, a flyer distributed on campus, use of Yammer and other institutional social media platforms, and internal electronic newsletters. Researchers responding to these recruitment efforts were invited to participate in the researcher survey and/or interview.
Incentives for participation
Researchers and PSP received a $25 gift card for completing the survey and $75 for completing the interview (researcher) or focus group (PSP) (up to $100 per researcher or PSP).
Data tables displaying demographic and other data from the PSP surveys (Table (Table1) 1 ) were prepared from the REDCap© database and responses reported as number and percent of respondents choosing each response option.
Post study participant (PSP) characteristics by Adolescents/Young Adults (AYA), Older Adults, and ALL (All participants regardless of age)
Age mean (SD) = 49.7 (18.6).
Focus group and researcher interview data were recorded (either via audio recording and/or notes taken by research staff) and analyzed via a general inductive qualitative approach, a method appropriate for program evaluation studies and aimed at condensing large amounts of textual data into frameworks that describe the underlying process and experiences under study [ 12 ]. Data were analyzed by our team’s qualitative expert who read the textual data multiple times, developed a coding scheme to identify themes in the textual data, and used group consensus methods with other team members to identify unique, key themes.
Sixty-one of sixty-five PSP who volunteered to participate in the PSP survey were screened eligible, fifty were consented, and forty-eight completed the survey questionnaire. Of the 48 PSP completing the survey, 15 (32%) were AYA and 33 (68%) older adults. The mean age of survey respondents was 49.7 years, 23.5 for AYA, and 61.6 for older adults. Survey respondents were predominantly White, non-Hispanic/Latino, female, and with some college or a college degree (Table (Table1). 1 ). The percentage of participants in each group never or rarely needing any help with reading/interpreting written materials was above 93% in both groups.
Over 90% of PSP responded that they would participate in another research study, and more than 75% of PSP indicated that study participants should know about study results. Most (68.8%) respondents indicated that they did not receive any communications from study staff after they finished a study .
PSP preferences for communication channel are summarized in Table Table2 2 and based on responses to the question “How do you want to receive information?.” Both AYA and older adults agree or completely agree that they prefer email to other communication channels and that billboards did not apply to them. Older adult preferences for communication channels as indicated by agreeing or completely agreeing were in ranked order of highest to lowest: use of mailed letters/postcards, newsletter, and phone. A majority (over 50%) of older adults completely disagreed or disagreed on texting and social media as options and had only slight preference for mass media, public forum, and wellness fairs or expos.
Communication preference by group: AYA * , older adult ** , and ALL ( n = 48)
ALL, total per column.
While AYA preferred email over all other options, they completely disagreed/disagreed with mailed letters/postcards, social media, and mass media options.
When communication formats were ranked overall by each group and by both groups combined, the ranking from most to least preferred was written materials, opportunities to interact with study teams and ask questions, visual charts, graphs, pictures, and videos, audios, and podcasts.
PSP Focus Groups
PSP want to receive and share information on study findings for studies in which he/she participated. Furthermore, participants stated their desire to share study results across social networks and highlighted opportunities to share communicated study results with their health-care providers, family members, friends, and other acquaintances with similar medical conditions.
Because of the things I was in a study for, it’s a condition I knew three other people who had the same condition, so as soon as it worked for me, I put the word out, this is great stuff. I would forward the email with the link, this is where you can go to also get in on this study, or I’d also tell them, you know, for me, like the medication. Here’s the medication. Here’s the name of it. Tell your doctor. I would definitely share. I’d just tell everyone without a doubt. Right when I get home, as soon as I walk in the door, and say Renee-that’s my daughter-I’ve got to tell you this.
Communication of study information could happen through several channels including social media, verbal communication, sharing of written documents, and forwarding emails containing a range of content in a range of formats (e.g., reports and pamphlets).
Word of mouth and I have no shame in saying I had head to toe psoriasis, and I used the drug being studied, and so I would just go to people, hey, look. So, if you had it in paper form, like a pamphlet or something, yeah I’d pass it on to them.
PSP prefer clear, simple messaging and highlighted multiple, preferred communication modalities for receiving information on study findings including emails, letters, newsletters, social media, and websites.
The wording is really simple, which I like. It’s to the point and clear. I really like the bullet points, because it’s quick and to the point. I think the [long] paragraphs-you get lost, especially when you are reading on your phone.
They indicated a clear preference for colorful, simple, easy to read communication. PSP also expressed some concern about difficulty opening emails with pictures and dislike lengthy written text. “I don’t read long emails. I tend to delete them”
PSP indicated some confusion about common research language. For example, one participant indicated that using the word “estimate” indicates the research findings were an approximation, “When I hear those words, I just think you’re guessing, estimate, you know? It sounds like an estimate, not a definite answer.”
Researcher Survey
Twenty-three of thirty-two researchers volunteered to participate in the researcher survey, were screened eligible, and two declined to participate, resulting in 19 who provided consent to participate and completed the survey. The mean age of survey respondents was 51.8 years. Respondents were predominantly White, non-Hispanic/Latino, and female, and all were holders of either a professional school degree or a doctoral degree. When asked if it is important to inform study participants of study results, 94.8% of responding researchers agreed that it was extremely important or important. Most researchers have disseminated findings to study participants or plan to disseminate findings.
Researchers listed a variety of reasons for their rating of the importance of informing study participants of study results including “to promote feelings of inclusion by participants and other community members”, “maintaining participant interest and engagement in the subject study and in research generally”, “allowing participants to benefit somewhat from their participation in research and especially if personal health data are collected”, “increasing transparency and opportunities for learning”, and “helping in understanding the impact of the research on the health issue under study”.
Some researchers view sharing study findings as an “ethical responsibility and/or a tenet of volunteerism for a research study”. For example, “if we (researchers) are obligated to inform participants about anything that comes up during the conduct of the study, we should feel compelled to equally give the results at the end of the study”.
One researcher “thought it a good idea to ask participants if they would like an overview of findings at the end of the study that they could share with others who would like to see the information”.
Two researchers said that sharing research results “depends on the study” and that providing “general findings to the participants” might be “sufficient for a treatment outcome study”.
Researchers indicated that despite their willingness to share study results, they face resource challenges such as a lack of funding and/or staff to support communication and dissemination activities and need assistance in developing these materials. One researcher remarked “I would really like to learn what are (sic) the best ways to share research findings. I am truly ignorant about this other than what I have casually observed. I would enjoy attending a workshop on the topic with suggested templates and communication strategies that work best” and that this survey “reminds me how important this is and it is promising that our CTSA seems to plan to take this on and help researchers with this important study element.”
Another researcher commented on a list of potential types of assistance that could be made available to assist with communicating and disseminating results, that “Training on developing lay friendly messaging is especially critically important and would translate across so many different aspects of what we do, not just dissemination of findings. But I’ve noticed that it is a skill that very few people have, and some people never can seem to develop. For that reason, I find as a principal investigator that I am spending a lot of my time working on these types of materials when I’d really prefer research assistant level folks having the ability to get me 99% of the way there.”
Most researchers indicated that they provide participants with personal tests or assessments taken from the study (60% n = 6) and final study results (72.7%, n = 8) but no other information such as recruitment and retention updates, interim updates or results, information on the impact of the study on either the health topic of the study or the community, information on other studies or provide tips and resources related to the health topic and self-help. Sixty percent ( n = 6) of researcher respondents indicated sharing planned next steps for the study team and information on how the study results would be used.
When asked about how they communicated results, phone calls were mentioned most frequently followed by newsletters, email, webpages, public forums, journal article, mailed letter or postcard, mass media, wellness fairs/expos, texting, or social media.
Researchers used a variety of communication formats to communicate with study participants. Written descriptions of study findings were most frequently reported followed by visual depictions, opportunities to interact with study staff and ask questions or provide feedback, and videos/audio/podcasts.
Seventy-three percent of researchers reported that they made efforts to make study findings information available to those with low levels of literacy, health literacy, or other possible limitations such as non-English-speaking populations.
In open-ended responses, most researchers reported wanting to increase their awareness and use of on-campus training and other resources to support communication and dissemination of study results, including how to get resources and budgets to support their use.
Researcher Interviews
One-on-one interviews with researchers identified two themes.
Researchers may struggle to see the utility of communicating small findings
Some researchers indicated hesitancy in communicating preliminary findings, findings from small studies, or highly summarized information. In addition, in comparison to research participants, researchers seemed to place a higher value on specific details of the study.
“I probably wouldn’t put it up [on social media] until the actual manuscript was out with the graphs and the figures, because I think that’s what people ultimately would be interested in.”
Researchers face resource and time limitations in communication and dissemination of study findings
Researchers expressed interest in communicating research results to study participants. However, they highlighted several challenges including difficulties in tracking current email and physical addresses for participants; compliance with literacy and visual impairment regulations; and the number of products already required in research that consume a considerable amount of a research team’s time. Researchers expressed a desire to have additional resources and templates to facilitate sharing study findings. According to one respondent, “For every grant there is (sic) 4-10 papers and 3-5 presentations, already doing 10-20 products.” Researchers do not want to “reinvent the wheel” and would like to pull from existing papers and presentations on how to share with participants and have boilerplate, writing templates, and other logistical information available for their use.
Researchers would also like training in the form of lunch-n-learns, podcasts, or easily accessible online tools on how to develop materials and approaches. Researchers are interested in understanding the “do’s and don’ts” of communicating and disseminating study findings and any regulatory requirements that should be considered when communicating with research participants following a completed study. For example, one researcher asked, “From beginning to end – the do’s and don’ts – are stamps allowed as a direct cost? or can indirect costs include paper for printing newsletters, how about designing a website, a checklist for pulling together a newsletter?”
The purpose of this pilot study was to explore the current experiences, expectations, concerns, preferences, and capacities of PSP including youth/young adult and older adult populations and researchers for sharing, receiving, and using information on research study findings. PSP and researchers agreed, as shown in earlier work [ 3 , 5 ], that sharing information upon study completion with participants was something that should be done and that had value for both PSP and researchers. As in prior studies [ 3 , 5 ], both groups also agreed that sharing study findings could improve ancillary outcomes such as participant recruitment and enrollment, use of research findings to improve health and health-care delivery, and build overall community support for research. In addition, communicating results acknowledges study participants’ contributions to research, a principle firmly rooted in respect for treating participants as not merely a means to further scientific investigation [ 5 ].
The majority of PSP indicated that they did not receive research findings from studies they participated in, that they would like to receive such information, and that they preferred specific communication methods for receipt of this information such as email and phone calls. While our sample was small, we did identify preferences for communication channels and for message format. Some differences and similarities in preferences for communication channels and message format were identified between AYA and older adults, thus reinforcing the best practice of customizing communication channel and messaging to each specific group. However, the preference for email and the similar rank ordering of messaging formats suggest that there are some overall communication preferences that may apply to most populations of PSP. It remains unclear whether participants prefer individual or aggregate results of study findings and depends on the type of study, for example, individual results of genotypes versus aggregate results of epidemiological studies [ 13 ]. A study by Miller et al suggests that the impact of receiving aggregate results, whether clinically relevant or not, may equal that of receiving individual results [ 14 ]. Further investigation warrants evaluation of whether, when, and how researchers should communicate types of results to study participants, considering multiple demographics of the populations such as age and ethnicity on preferences.
While researchers acknowledged that PSP would like to hear from them regarding research results and that they wanted to meet this expectation, they indicated needing specific training and/or time and resources to provide this information to PSP in a way that meets PSP needs and preferences. Costs associated with producing reports of findings were a concern of researchers in our study, similar to findings from a study conducted by Di Blasi and colleagues in which 15% (8 of 53 investigators) indicated that they wanted to avoid extra costs associated with the conduct of their studies and extra administrative work [ 15 ]. In this same study, the major reason for not informing participants about study results was that forty percent of investigators never considered this option. Researchers were unaware of resources available on existing platforms at their home institution or elsewhere to help them with communication and dissemination efforts [ 10 ].
Addressing Barriers to Implementation
Information from academic and other organizations on how to best communicate research findings in plain language is available and could be shared with researchers and their teams. The Cochrane Collaborative [ 16 ], the Centers for Disease Control and Prevention [ 17 ], and the Patient-Centered Outcomes Research Institute [ 18 ] have resources to help researchers develop plain language summaries using proven approaches to overcome literacy and other issues that limit participant access to study findings. Some academic institutions have electronic systems in place to confidentially share templated laboratory and other personal study information with participants and, if appropriate, with their health-care providers.
Limitations
Findings from the study are limited by several study and respondent characteristics. The sample was drawn from research records at one university engaging in research in a relatively defined geographic area and among two special populations: AYA and older adults. As such, participants were not representative of either the general population in the area, the population of PSP or researchers available in the area, or the racial and ethnic diversity of potential and/or actual participants in the geographic area. The small number of researcher participants did not represent the pool of researchers at the university, and the research studies from which participants were drawn were not representative of the broad range of clinical and translational research undertaken by our institution or within the geographic community it serves. The number of survey and focus group participants was insufficient to allow robust analysis of findings specific to participants’ race, ethnicity, gender, or membership in the target age groups of AYA or older adult. However, these data will inform a future trial with adequate representations from underrepresented and special population groups.
Since all PSP had participated in research, they may have been biased in favor of wanting to know more about study results and/or supportive/nonsupportive of the method of communication/dissemination they were exposed to through their participation in these studies.
Conclusions
Our findings provide information from PSP and researchers on their expectations about sharing study findings, preferences for how to communicate and disseminate study findings, and need for greater assistance in removing roadblocks to using proven communication and dissemination approaches. This information illustrates the potential to engage both PSP and researchers in the design and use of communication and dissemination strategies and materials to share research findings, engage in efforts to more broadly disseminate research findings, and inform our understanding of how to interpret and communicate research findings for members of special population groups. While several initial prototypes were developed in response to this feedback and shared for review by participants in this study, future research will focus on finalizing and testing specific communication and dissemination prototypes aimed at these special population groups.
Findings from our study support a major goal of the National Center for Advancing Translational Science Recruitment Innovation Center to engage and collaborate with patients and their communities to advance translation science. In response to the increased awareness of the importance of sharing results with study participants or the general public, a template for dissemination of research results is available in the Recruitment and Retention Toolbox through the CTSA Trial Innovation Network (TIN: trialinnovationnetwork.org ). We believe that our findings will inform resources for use in special populations through collaborations within the TIN.
Acknowledgment
This pilot project was supported, in part, by the National Center for Advancing Translational Sciences of the NIH under Grant Number UL1 TR001450. The content is solely the responsibility of the authors and does not necessarily represent the official views of the NIH.
Disclosures
The authors have no conflicts of interest to declare.
Ethical Approval
This study was reviewed, approved, and continuously overseen by the IRB at the Medical University of South Carolina (ID: Pro00067659). All procedures performed in studies involving human participants were in accordance with the ethical standards of the institutional and/or national research committee and with the 1964 Helsinki declaration and its later amendments or comparable ethical standards.
We use cookies on this site to enhance your experience
By clicking any link on this page you are giving your consent for us to set cookies.
A link to reset your password has been sent to your email.
Back to login
We need additional information from you. Please complete your profile first before placing your order.
Thank you. payment completed., you will receive an email from us to confirm your registration, please click the link in the email to activate your account., there was error during payment, orcid profile found in public registry, download history, how to write the analysis and discussion chapters in qualitative (ssah) research.
- Charlesworth Author Services
- 11 November, 2021
While it is more common for Science, Technology, Engineering and Mathematics (STEM) researchers to write separate, distinct chapters for their data/ results and analysis/ discussion , the same sections can feel less clearly defined for a researcher in Social Sciences, Arts and Humanities (SSAH). This article will look specifically at some useful approaches to writing the analysis and discussion chapters in qualitative/SSAH research.
Note : Most of the differences in approaches to research, writing, analysis and discussion come down, ultimately, to differences in epistemology – how we approach, create and work with knowledge in our respective fields. However, this is a vast topic that deserves a separate discussion.
Look for emerging themes and patterns
The ‘results’ of qualitative research can sometimes be harder to pinpoint than in quantitative research. You’re not dealing with definitive numbers and results in the same way as, say, a scientist conducting experiments that produce measurable data. Instead, most qualitative researchers explore prominent, interesting themes and patterns emerging from their data – that could comprise interviews, textual material or participant observation, for example.
You may find that your data presents a huge number of themes, issues and topics, all of which you might find equally significant and interesting. In fact, you might find yourself overwhelmed by the many directions that your research could take, depending on which themes you choose to study in further depth. You may even discover issues and patterns that you had not expected , that may necessitate having to change or expand the research focus you initially started off with.
It is crucial at this point not to panic. Instead, try to enjoy the many possibilities that your data is offering you. It can be useful to remind yourself at each stage of exactly what you are trying to find out through this research.
What exactly do you want to know? What knowledge do you want to generate and share within your field?
Then, spend some time reflecting upon each of the themes that seem most interesting and significant, and consider whether they are immediately relevant to your main, overarching research objectives and goals.
Suggestion: Don’t worry too much about structure and flow at the early stages of writing your discussion . It would be a more valuable use of your time to fully explore the themes and issues arising from your data first, while also reading widely alongside your writing (more on this below). As you work more intimately with the data and develop your ideas, the overarching narrative and connections between those ideas will begin to emerge. Trust that you’ll be able to draw those links and craft the structure organically as you write.
Let your data guide you
A key characteristic of qualitative research is that the researchers allow their data to ‘speak’ and guide their research and their writing. Instead of insisting too strongly upon the prominence of specific themes and issues and imposing their opinions and beliefs upon the data, a good qualitative researcher ‘listens’ to what the data has to tell them.
Again, you might find yourself having to address unexpected issues or your data may reveal things that seem completely contradictory to the ideas and theories you have worked with so far. Although this might seem worrying, discovering these unexpected new elements can actually make your research much richer and more interesting.
Suggestion: Allow yourself to follow those leads and ask new questions as you work through your data. These new directions could help you to answer your research questions in more depth and with greater complexity; or they could even open up other avenues for further study, either in this or future research.
Work closely with the literature
As you analyse and discuss the prominent themes, arguments and findings arising from your data, it is very helpful to maintain a regular and consistent reading practice alongside your writing. Return to the literature that you’ve already been reading so far or begin to check out new texts, studies and theories that might be more appropriate for working with any new ideas and themes arising from your data.
Reading and incorporating relevant literature into your writing as you work through your analysis and discussion will help you to consistently contextualise your research within the larger body of knowledge. It will be easier to stay focused on what you are trying to say through your research if you can simultaneously show what has already been said on the subject and how your research and data supports, challenges or extends those debates. By drawing from existing literature , you are setting up a dialogue between your research and prior work, and highlighting what this research has to add to the conversation.
Suggestion : Although it might sometimes feel tedious to have to blend others’ writing in with yours, this is ultimately the best way to showcase the specialness of your own data, findings and research . Remember that it is more difficult to highlight the significance and relevance of your original work without first showing how that work fits into or responds to existing studies.
In conclusion
The discussion chapters form the heart of your thesis and this is where your unique contribution comes to the forefront. This is where your data takes centre-stage and where you get to showcase your original arguments, perspectives and knowledge. To do this effectively needs you to explore the original themes and issues arising from and within the data, while simultaneously contextualising these findings within the larger, existing body of knowledge of your specialising field. By striking this balance, you prove the two most important qualities of excellent qualitative research : keen awareness of your field and a firm understanding of your place in it.
Charlesworth Author Services , a trusted brand supporting the world’s leading academic publishers, institutions and authors since 1928.
To know more about our services, visit: Our Services
Visit our new Researcher Education Portal that offers articles and webinars covering all aspects of your research to publication journey! And sign up for our newsletter on the Portal to stay updated on all essential researcher knowledge and information!
Register now: Researcher Education Portal
Maximise your publication success with Charlesworth Author Services.
Share with your colleagues
Related articles.
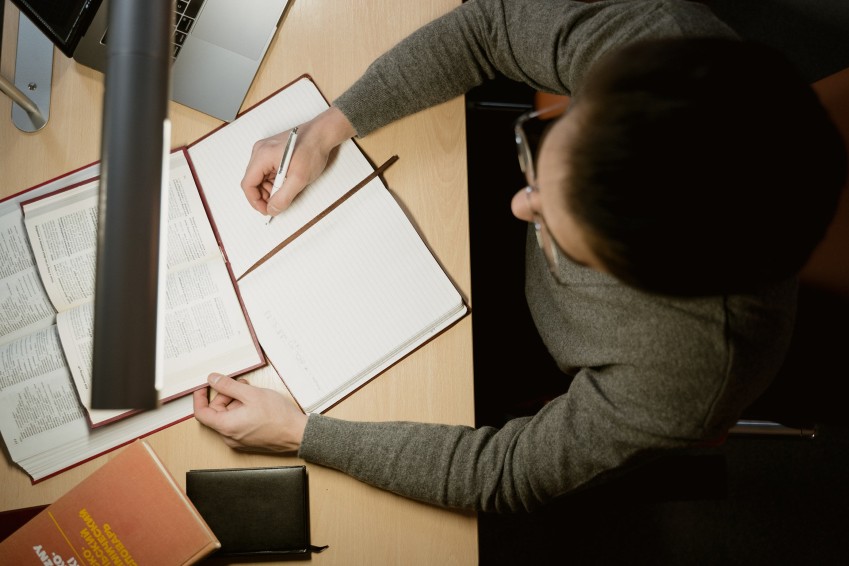

How to write an Introduction to an academic article
Charlesworth Author Services 17/08/2020 00:00:00

The best way to write the Study Background
Charlesworth Author Services 25/10/2021 00:00:00
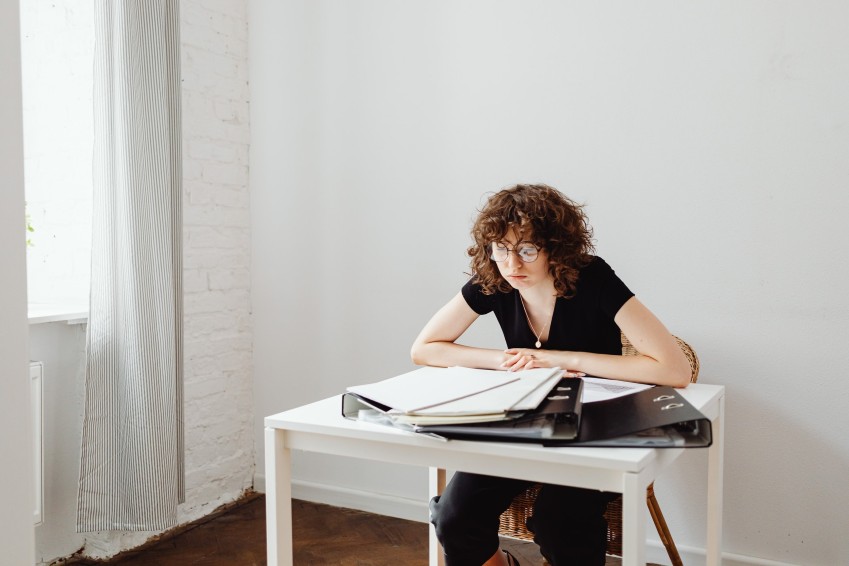
Conducting a Literature Review
Charlesworth Author Services 10/03/2021 00:00:00
Related webinars

Bitesize Webinar: How to write and structure your academic article for publication: Module 4: Prepare to write your academic paper
Charlesworth Author Services 04/03/2021 00:00:00

Bitesize Webinar: How to write and structure your academic article for publication: Module 5: Conduct a Literature Review

Bitesize Webinar: How to write and structure your academic article for publication: Module 6: Choose great titles and write strong abstracts
Charlesworth Author Services 05/03/2021 00:00:00

Bitesize Webinar: How to write and structure your academic article for publication: Module 7: Write a strong theoretical framework section
Literature review.
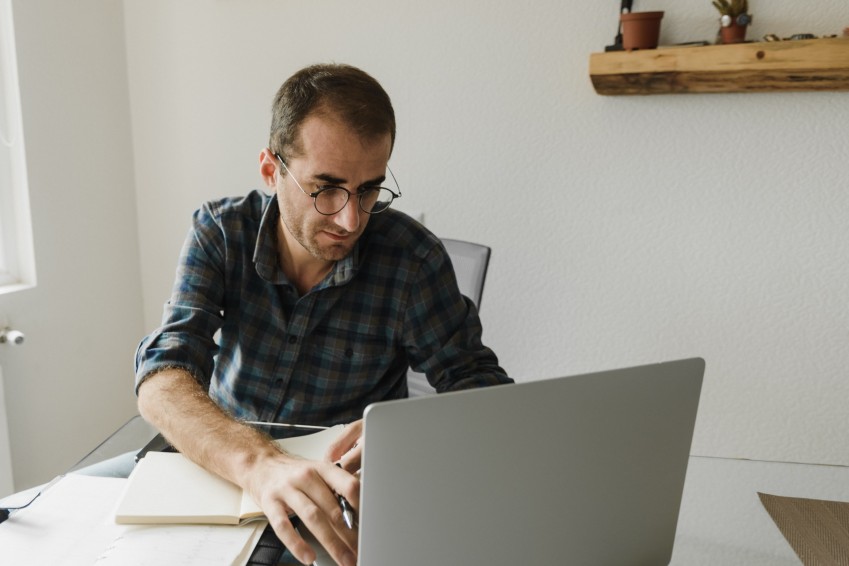
Important factors to consider as you Start to Plan your Literature Review
Charlesworth Author Services 06/10/2021 00:00:00
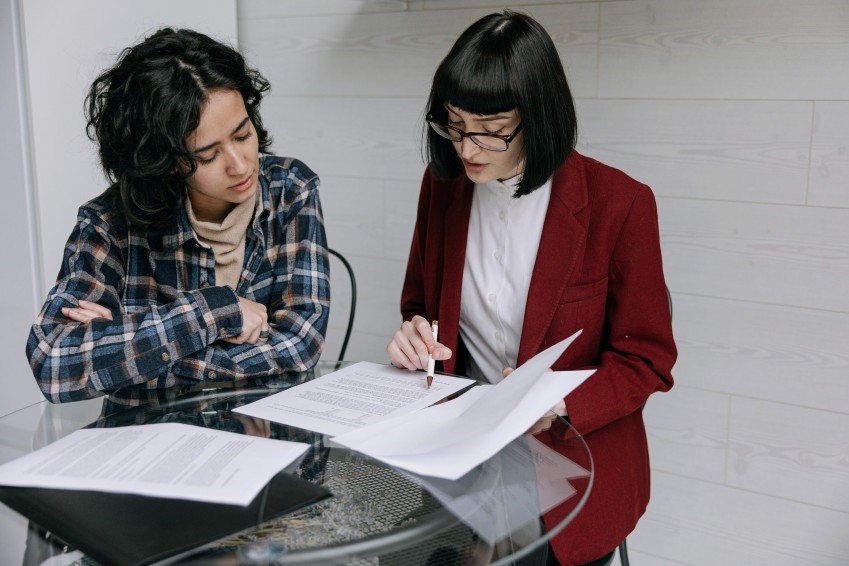
Difference between a Literature Review and a Critical Review
Charlesworth Author Services 08/10/2021 00:00:00
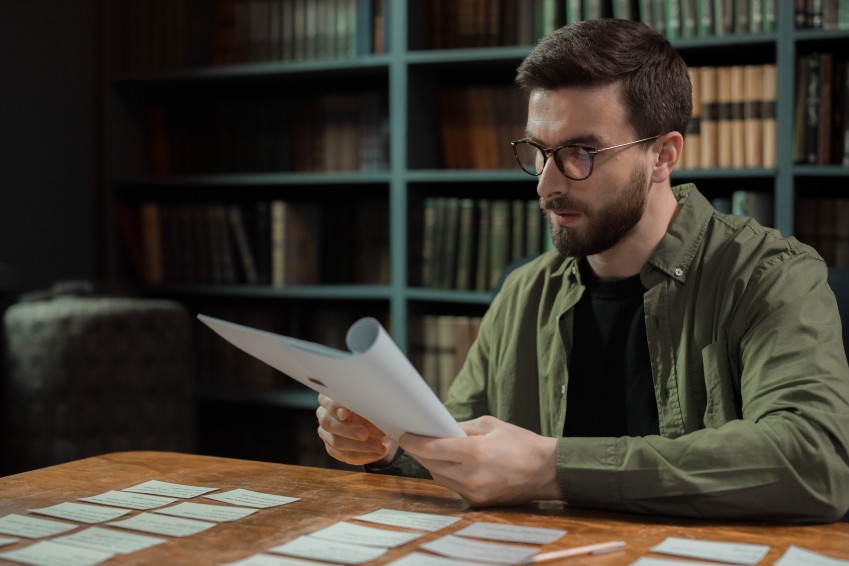
How to refer to other studies or literature in the different sections of a research paper
Charlesworth Author Services 07/10/2021 00:00:00
- Privacy Policy

Home » Research Findings – Types Examples and Writing Guide
Research Findings – Types Examples and Writing Guide
Table of Contents

Research Findings
Definition:
Research findings refer to the results obtained from a study or investigation conducted through a systematic and scientific approach. These findings are the outcomes of the data analysis, interpretation, and evaluation carried out during the research process.
Types of Research Findings
There are two main types of research findings:
Qualitative Findings
Qualitative research is an exploratory research method used to understand the complexities of human behavior and experiences. Qualitative findings are non-numerical and descriptive data that describe the meaning and interpretation of the data collected. Examples of qualitative findings include quotes from participants, themes that emerge from the data, and descriptions of experiences and phenomena.
Quantitative Findings
Quantitative research is a research method that uses numerical data and statistical analysis to measure and quantify a phenomenon or behavior. Quantitative findings include numerical data such as mean, median, and mode, as well as statistical analyses such as t-tests, ANOVA, and regression analysis. These findings are often presented in tables, graphs, or charts.
Both qualitative and quantitative findings are important in research and can provide different insights into a research question or problem. Combining both types of findings can provide a more comprehensive understanding of a phenomenon and improve the validity and reliability of research results.
Parts of Research Findings
Research findings typically consist of several parts, including:
- Introduction: This section provides an overview of the research topic and the purpose of the study.
- Literature Review: This section summarizes previous research studies and findings that are relevant to the current study.
- Methodology : This section describes the research design, methods, and procedures used in the study, including details on the sample, data collection, and data analysis.
- Results : This section presents the findings of the study, including statistical analyses and data visualizations.
- Discussion : This section interprets the results and explains what they mean in relation to the research question(s) and hypotheses. It may also compare and contrast the current findings with previous research studies and explore any implications or limitations of the study.
- Conclusion : This section provides a summary of the key findings and the main conclusions of the study.
- Recommendations: This section suggests areas for further research and potential applications or implications of the study’s findings.
How to Write Research Findings
Writing research findings requires careful planning and attention to detail. Here are some general steps to follow when writing research findings:
- Organize your findings: Before you begin writing, it’s essential to organize your findings logically. Consider creating an outline or a flowchart that outlines the main points you want to make and how they relate to one another.
- Use clear and concise language : When presenting your findings, be sure to use clear and concise language that is easy to understand. Avoid using jargon or technical terms unless they are necessary to convey your meaning.
- Use visual aids : Visual aids such as tables, charts, and graphs can be helpful in presenting your findings. Be sure to label and title your visual aids clearly, and make sure they are easy to read.
- Use headings and subheadings: Using headings and subheadings can help organize your findings and make them easier to read. Make sure your headings and subheadings are clear and descriptive.
- Interpret your findings : When presenting your findings, it’s important to provide some interpretation of what the results mean. This can include discussing how your findings relate to the existing literature, identifying any limitations of your study, and suggesting areas for future research.
- Be precise and accurate : When presenting your findings, be sure to use precise and accurate language. Avoid making generalizations or overstatements and be careful not to misrepresent your data.
- Edit and revise: Once you have written your research findings, be sure to edit and revise them carefully. Check for grammar and spelling errors, make sure your formatting is consistent, and ensure that your writing is clear and concise.
Research Findings Example
Following is a Research Findings Example sample for students:
Title: The Effects of Exercise on Mental Health
Sample : 500 participants, both men and women, between the ages of 18-45.
Methodology : Participants were divided into two groups. The first group engaged in 30 minutes of moderate intensity exercise five times a week for eight weeks. The second group did not exercise during the study period. Participants in both groups completed a questionnaire that assessed their mental health before and after the study period.
Findings : The group that engaged in regular exercise reported a significant improvement in mental health compared to the control group. Specifically, they reported lower levels of anxiety and depression, improved mood, and increased self-esteem.
Conclusion : Regular exercise can have a positive impact on mental health and may be an effective intervention for individuals experiencing symptoms of anxiety or depression.
Applications of Research Findings
Research findings can be applied in various fields to improve processes, products, services, and outcomes. Here are some examples:
- Healthcare : Research findings in medicine and healthcare can be applied to improve patient outcomes, reduce morbidity and mortality rates, and develop new treatments for various diseases.
- Education : Research findings in education can be used to develop effective teaching methods, improve learning outcomes, and design new educational programs.
- Technology : Research findings in technology can be applied to develop new products, improve existing products, and enhance user experiences.
- Business : Research findings in business can be applied to develop new strategies, improve operations, and increase profitability.
- Public Policy: Research findings can be used to inform public policy decisions on issues such as environmental protection, social welfare, and economic development.
- Social Sciences: Research findings in social sciences can be used to improve understanding of human behavior and social phenomena, inform public policy decisions, and develop interventions to address social issues.
- Agriculture: Research findings in agriculture can be applied to improve crop yields, develop new farming techniques, and enhance food security.
- Sports : Research findings in sports can be applied to improve athlete performance, reduce injuries, and develop new training programs.
When to use Research Findings
Research findings can be used in a variety of situations, depending on the context and the purpose. Here are some examples of when research findings may be useful:
- Decision-making : Research findings can be used to inform decisions in various fields, such as business, education, healthcare, and public policy. For example, a business may use market research findings to make decisions about new product development or marketing strategies.
- Problem-solving : Research findings can be used to solve problems or challenges in various fields, such as healthcare, engineering, and social sciences. For example, medical researchers may use findings from clinical trials to develop new treatments for diseases.
- Policy development : Research findings can be used to inform the development of policies in various fields, such as environmental protection, social welfare, and economic development. For example, policymakers may use research findings to develop policies aimed at reducing greenhouse gas emissions.
- Program evaluation: Research findings can be used to evaluate the effectiveness of programs or interventions in various fields, such as education, healthcare, and social services. For example, educational researchers may use findings from evaluations of educational programs to improve teaching and learning outcomes.
- Innovation: Research findings can be used to inspire or guide innovation in various fields, such as technology and engineering. For example, engineers may use research findings on materials science to develop new and innovative products.
Purpose of Research Findings
The purpose of research findings is to contribute to the knowledge and understanding of a particular topic or issue. Research findings are the result of a systematic and rigorous investigation of a research question or hypothesis, using appropriate research methods and techniques.
The main purposes of research findings are:
- To generate new knowledge : Research findings contribute to the body of knowledge on a particular topic, by adding new information, insights, and understanding to the existing knowledge base.
- To test hypotheses or theories : Research findings can be used to test hypotheses or theories that have been proposed in a particular field or discipline. This helps to determine the validity and reliability of the hypotheses or theories, and to refine or develop new ones.
- To inform practice: Research findings can be used to inform practice in various fields, such as healthcare, education, and business. By identifying best practices and evidence-based interventions, research findings can help practitioners to make informed decisions and improve outcomes.
- To identify gaps in knowledge: Research findings can help to identify gaps in knowledge and understanding of a particular topic, which can then be addressed by further research.
- To contribute to policy development: Research findings can be used to inform policy development in various fields, such as environmental protection, social welfare, and economic development. By providing evidence-based recommendations, research findings can help policymakers to develop effective policies that address societal challenges.
Characteristics of Research Findings
Research findings have several key characteristics that distinguish them from other types of information or knowledge. Here are some of the main characteristics of research findings:
- Objective : Research findings are based on a systematic and rigorous investigation of a research question or hypothesis, using appropriate research methods and techniques. As such, they are generally considered to be more objective and reliable than other types of information.
- Empirical : Research findings are based on empirical evidence, which means that they are derived from observations or measurements of the real world. This gives them a high degree of credibility and validity.
- Generalizable : Research findings are often intended to be generalizable to a larger population or context beyond the specific study. This means that the findings can be applied to other situations or populations with similar characteristics.
- Transparent : Research findings are typically reported in a transparent manner, with a clear description of the research methods and data analysis techniques used. This allows others to assess the credibility and reliability of the findings.
- Peer-reviewed: Research findings are often subject to a rigorous peer-review process, in which experts in the field review the research methods, data analysis, and conclusions of the study. This helps to ensure the validity and reliability of the findings.
- Reproducible : Research findings are often designed to be reproducible, meaning that other researchers can replicate the study using the same methods and obtain similar results. This helps to ensure the validity and reliability of the findings.
Advantages of Research Findings
Research findings have many advantages, which make them valuable sources of knowledge and information. Here are some of the main advantages of research findings:
- Evidence-based: Research findings are based on empirical evidence, which means that they are grounded in data and observations from the real world. This makes them a reliable and credible source of information.
- Inform decision-making: Research findings can be used to inform decision-making in various fields, such as healthcare, education, and business. By identifying best practices and evidence-based interventions, research findings can help practitioners and policymakers to make informed decisions and improve outcomes.
- Identify gaps in knowledge: Research findings can help to identify gaps in knowledge and understanding of a particular topic, which can then be addressed by further research. This contributes to the ongoing development of knowledge in various fields.
- Improve outcomes : Research findings can be used to develop and implement evidence-based practices and interventions, which have been shown to improve outcomes in various fields, such as healthcare, education, and social services.
- Foster innovation: Research findings can inspire or guide innovation in various fields, such as technology and engineering. By providing new information and understanding of a particular topic, research findings can stimulate new ideas and approaches to problem-solving.
- Enhance credibility: Research findings are generally considered to be more credible and reliable than other types of information, as they are based on rigorous research methods and are subject to peer-review processes.
Limitations of Research Findings
While research findings have many advantages, they also have some limitations. Here are some of the main limitations of research findings:
- Limited scope: Research findings are typically based on a particular study or set of studies, which may have a limited scope or focus. This means that they may not be applicable to other contexts or populations.
- Potential for bias : Research findings can be influenced by various sources of bias, such as researcher bias, selection bias, or measurement bias. This can affect the validity and reliability of the findings.
- Ethical considerations: Research findings can raise ethical considerations, particularly in studies involving human subjects. Researchers must ensure that their studies are conducted in an ethical and responsible manner, with appropriate measures to protect the welfare and privacy of participants.
- Time and resource constraints : Research studies can be time-consuming and require significant resources, which can limit the number and scope of studies that are conducted. This can lead to gaps in knowledge or a lack of research on certain topics.
- Complexity: Some research findings can be complex and difficult to interpret, particularly in fields such as science or medicine. This can make it challenging for practitioners and policymakers to apply the findings to their work.
- Lack of generalizability : While research findings are intended to be generalizable to larger populations or contexts, there may be factors that limit their generalizability. For example, cultural or environmental factors may influence how a particular intervention or treatment works in different populations or contexts.
About the author
Muhammad Hassan
Researcher, Academic Writer, Web developer
You may also like

Data Collection – Methods Types and Examples

Delimitations in Research – Types, Examples and...

Research Process – Steps, Examples and Tips

Research Design – Types, Methods and Examples

Institutional Review Board – Application Sample...

Evaluating Research – Process, Examples and...
Have a language expert improve your writing
Run a free plagiarism check in 10 minutes, automatically generate references for free.
- Knowledge Base
- Dissertation
- How to Write a Discussion Section | Tips & Examples
How to Write a Discussion Section | Tips & Examples
Published on 21 August 2022 by Shona McCombes . Revised on 25 October 2022.
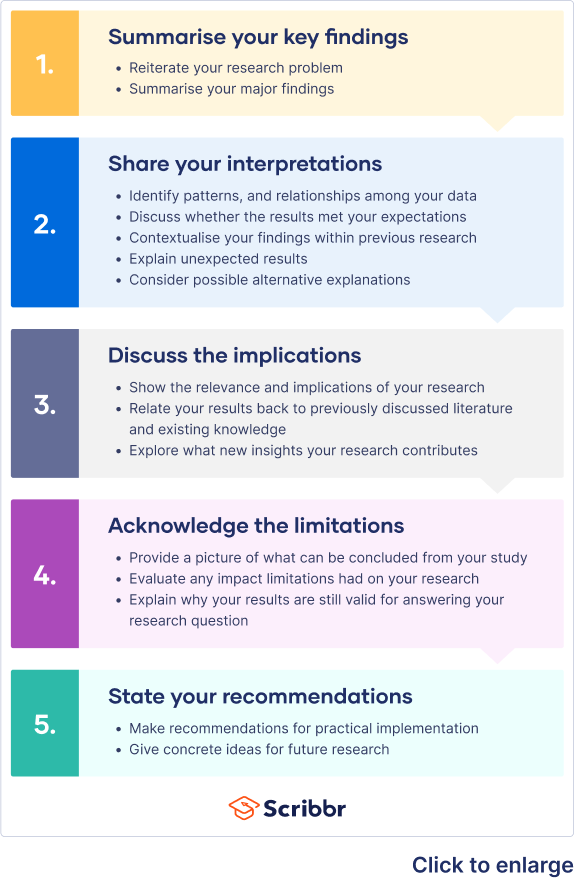
The discussion section is where you delve into the meaning, importance, and relevance of your results .
It should focus on explaining and evaluating what you found, showing how it relates to your literature review , and making an argument in support of your overall conclusion . It should not be a second results section .
There are different ways to write this section, but you can focus your writing around these key elements:
- Summary: A brief recap of your key results
- Interpretations: What do your results mean?
- Implications: Why do your results matter?
- Limitations: What can’t your results tell us?
- Recommendations: Avenues for further studies or analyses
Instantly correct all language mistakes in your text
Be assured that you'll submit flawless writing. Upload your document to correct all your mistakes.
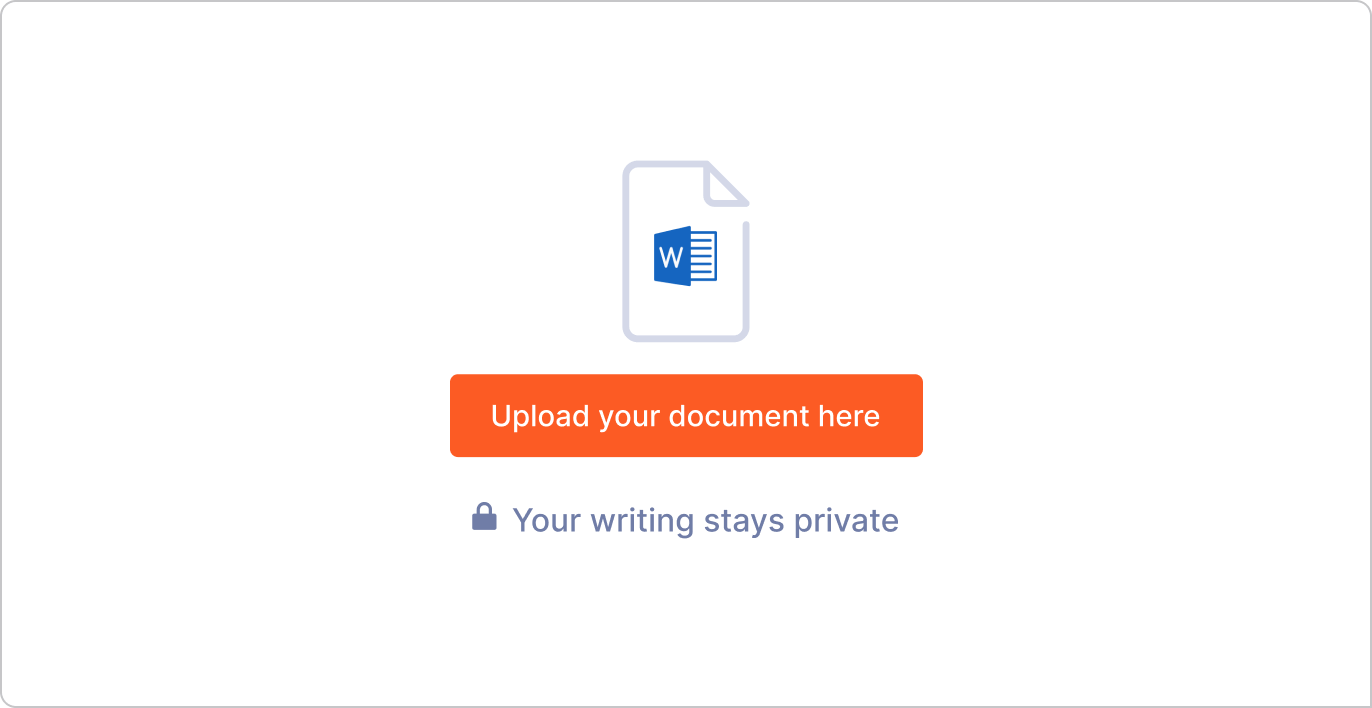
Table of contents
What not to include in your discussion section, step 1: summarise your key findings, step 2: give your interpretations, step 3: discuss the implications, step 4: acknowledge the limitations, step 5: share your recommendations, discussion section example.
There are a few common mistakes to avoid when writing the discussion section of your paper.
- Don’t introduce new results: You should only discuss the data that you have already reported in your results section .
- Don’t make inflated claims: Avoid overinterpretation and speculation that isn’t directly supported by your data.
- Don’t undermine your research: The discussion of limitations should aim to strengthen your credibility, not emphasise weaknesses or failures.
Prevent plagiarism, run a free check.
Start this section by reiterating your research problem and concisely summarising your major findings. Don’t just repeat all the data you have already reported – aim for a clear statement of the overall result that directly answers your main research question . This should be no more than one paragraph.
Many students struggle with the differences between a discussion section and a results section . The crux of the matter is that your results sections should present your results, and your discussion section should subjectively evaluate them. Try not to blend elements of these two sections, in order to keep your paper sharp.
- The results indicate that …
- The study demonstrates a correlation between …
- This analysis supports the theory that …
- The data suggest that …
The meaning of your results may seem obvious to you, but it’s important to spell out their significance for your reader, showing exactly how they answer your research question.
The form of your interpretations will depend on the type of research, but some typical approaches to interpreting the data include:
- Identifying correlations , patterns, and relationships among the data
- Discussing whether the results met your expectations or supported your hypotheses
- Contextualising your findings within previous research and theory
- Explaining unexpected results and evaluating their significance
- Considering possible alternative explanations and making an argument for your position
You can organise your discussion around key themes, hypotheses, or research questions, following the same structure as your results section. Alternatively, you can also begin by highlighting the most significant or unexpected results.
- In line with the hypothesis …
- Contrary to the hypothesised association …
- The results contradict the claims of Smith (2007) that …
- The results might suggest that x . However, based on the findings of similar studies, a more plausible explanation is x .
As well as giving your own interpretations, make sure to relate your results back to the scholarly work that you surveyed in the literature review . The discussion should show how your findings fit with existing knowledge, what new insights they contribute, and what consequences they have for theory or practice.
Ask yourself these questions:
- Do your results support or challenge existing theories? If they support existing theories, what new information do they contribute? If they challenge existing theories, why do you think that is?
- Are there any practical implications?
Your overall aim is to show the reader exactly what your research has contributed, and why they should care.
- These results build on existing evidence of …
- The results do not fit with the theory that …
- The experiment provides a new insight into the relationship between …
- These results should be taken into account when considering how to …
- The data contribute a clearer understanding of …
- While previous research has focused on x , these results demonstrate that y .
The only proofreading tool specialized in correcting academic writing
The academic proofreading tool has been trained on 1000s of academic texts and by native English editors. Making it the most accurate and reliable proofreading tool for students.
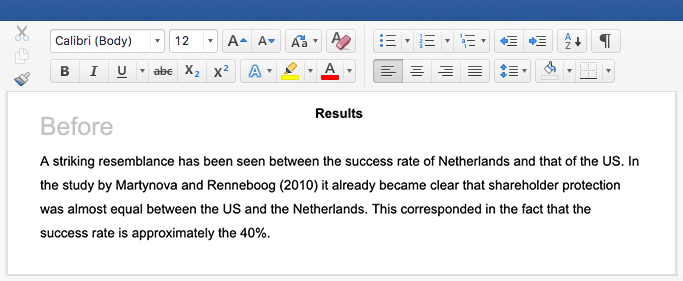
Correct my document today
Even the best research has its limitations. Acknowledging these is important to demonstrate your credibility. Limitations aren’t about listing your errors, but about providing an accurate picture of what can and cannot be concluded from your study.
Limitations might be due to your overall research design, specific methodological choices , or unanticipated obstacles that emerged during your research process.
Here are a few common possibilities:
- If your sample size was small or limited to a specific group of people, explain how generalisability is limited.
- If you encountered problems when gathering or analysing data, explain how these influenced the results.
- If there are potential confounding variables that you were unable to control, acknowledge the effect these may have had.
After noting the limitations, you can reiterate why the results are nonetheless valid for the purpose of answering your research question.
- The generalisability of the results is limited by …
- The reliability of these data is impacted by …
- Due to the lack of data on x , the results cannot confirm …
- The methodological choices were constrained by …
- It is beyond the scope of this study to …
Based on the discussion of your results, you can make recommendations for practical implementation or further research. Sometimes, the recommendations are saved for the conclusion .
Suggestions for further research can lead directly from the limitations. Don’t just state that more studies should be done – give concrete ideas for how future work can build on areas that your own research was unable to address.
- Further research is needed to establish …
- Future studies should take into account …
- Avenues for future research include …
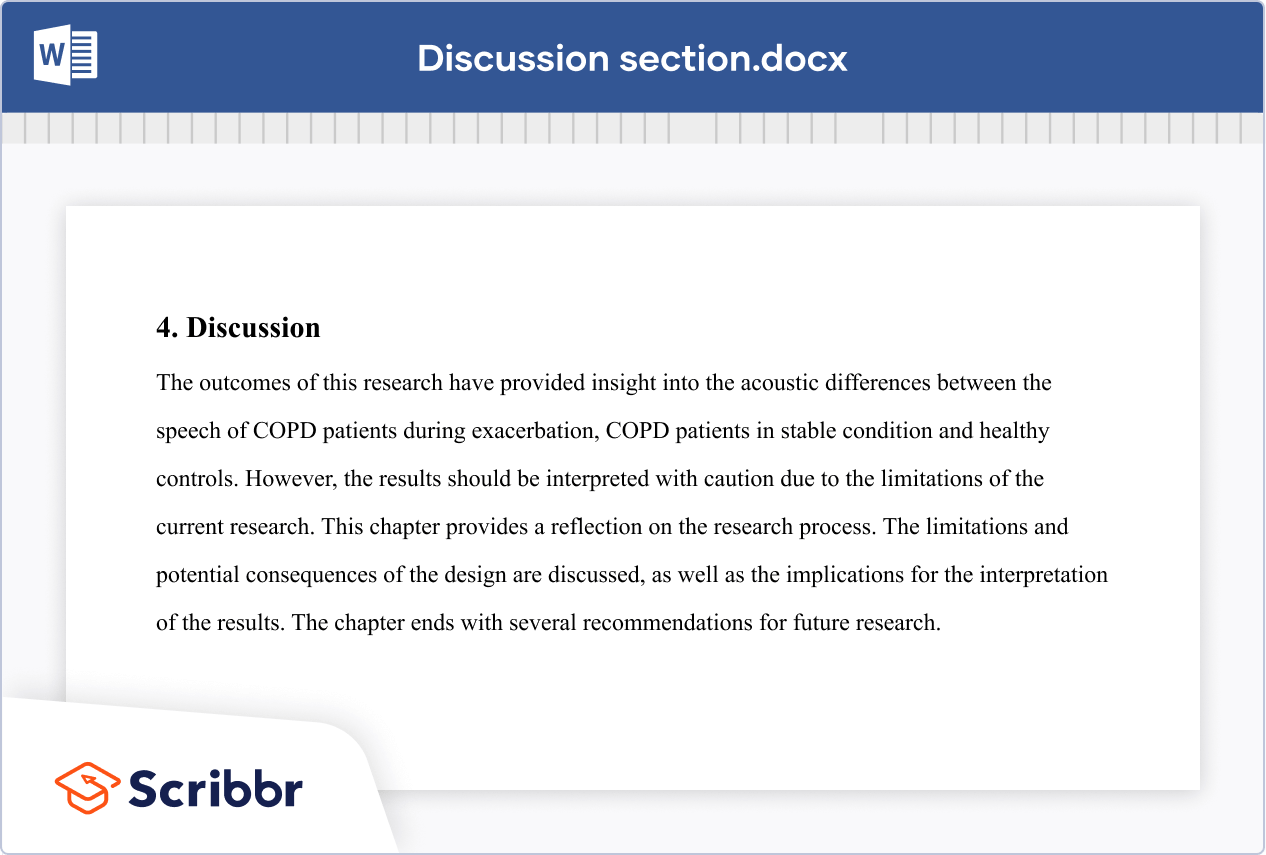
Cite this Scribbr article
If you want to cite this source, you can copy and paste the citation or click the ‘Cite this Scribbr article’ button to automatically add the citation to our free Reference Generator.
McCombes, S. (2022, October 25). How to Write a Discussion Section | Tips & Examples. Scribbr. Retrieved 14 May 2024, from https://www.scribbr.co.uk/thesis-dissertation/discussion/
Is this article helpful?
Shona McCombes
Other students also liked, how to write a results section | tips & examples, research paper appendix | example & templates, how to write a thesis or dissertation introduction.
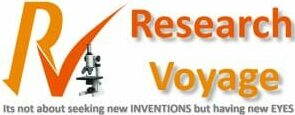
Research Voyage
Research Tips and Infromation
07 Easy Steps for Writing Discussion Section of a Research Paper

In my role as a journal reviewer, I’ve had the privilege (and sometimes the frustration) of reviewing numerous research papers.
I’ve encountered papers where authors neglect to discuss how their results benefit the problem at hand, the domain they are working in, and society at large. This oversight often fails to make a lasting impression on reviewers
One recurring issue that I’ve noticed, time and again, is the challenge of properly delineating the boundaries of the discussion section in research papers. It’s not uncommon to come across papers where authors blur the lines between various crucial sections in a research paper.
Some authors mistakenly present their results within the discussion section, failing to provide a clear delineation between the findings of their study and their interpretation. Even if the journal allows to combine the results and discussion section, adopting a structured approach, such as dedicating a paragraph for results and a paragraph for discussing the results, can enhance the flow and readability of the paper, ensuring that readers can easily follow the progression of the study and its implications.
I vividly recall one instance where an author proceeded to rehash the entire methodology, complete with block diagrams, within the discussion section—without ever drawing any substantive conclusions. It felt like wading through familiar territory, only to find myself back at square one.
And then there are those authors who seem more interested in speculating about future directions than analyzing the outcomes of their current work in the discussion section. While it’s important to consider the implications of one’s research, it shouldn’t overshadow the critical analysis of the results at hand.
In another instance, a researcher concealed all failures or limitations in their work, presenting only the best-case scenarios, which created doubts about the validity of their findings. As a reviewer, I promptly sent it back for a relook and suggested adding various scenarios to reflect the true behaviour of the experiment.
In this post, I’ll delve into practical strategies for crafting the discussion section of a research paper. Drawing from my experiences as a reviewer and researcher, I’ll explore the nuances of the discussion section and provide insights into how to engage in critical discussion.
Introduction
I. focus on the relevance.
- II. Highlight the Limitations
- III. Introduce New Discoveries
IV. Highlight the Observations
V. compare and relate with other research works.
- VI. Provide Alternate View Points
A. Future Directions
B. conclusion, how to validate the claims i made in the discussion section of my research paper, phrases that can be used in the discussion section of a research paper, phrases that can be used in the analysis part of the discussion section of a research paper.
- Your Next Move...
Whether charts and graphs are allowed in discussion section of my Research Paper?
Can i add citations in discussion section of my research paper, can i combine results and discussion section in my research paper, what is the weightage of discussion section in a research paper in terms of selection to a journal, whether literature survey paper has a discussion section.
The Discussion section of a research paper is where authors interpret their findings, contextualize their research, and propose future directions. It is a crucial section that provides the reader with insights into the significance and implications of the study.
Writing an effective discussion section is a crucial aspect of any research paper, as it allows researchers to delve into the significance of their findings and explore their implications. A well-crafted discussion section not only summarizes the key observations and limitations of the study but also establishes connections with existing research and opens avenues for future exploration. In this article, we will present a comprehensive guide to help you structure your discussion section in seven simple steps.
By following these steps, you’ll be able to write a compelling Discussion section that enhances the reader’s understanding of your research and contributes to the broader scientific community.
Please note, the discussion section usually follows after the Results Section. I have written a comprehensive article on ” How to Write Results Section of your Research Paper “. Please visit the article to enhance your write-up on the results section.
Which are these 07 steps for writing an Effective Discussion Section of a Research Paper?
Step 1: Focus on the Relevance : In the first step, we will discuss the importance of emphasizing the relevance of your research findings to the broader scientific context. By clearly articulating the significance of your study, you can help readers understand how your work contributes to the existing body of knowledge and why it matters.
Step 2: Highlight the Limitations : Every research study has its limitations, and it is essential to address them honestly and transparently. We will explore how to identify and describe the limitations of your study, demonstrating a thorough understanding of potential weaknesses and areas for improvement.
Step 3: Highlight the Observations : In this step, we will delve into the core findings of your study. We will discuss the key observations and results, focusing on their relevance to your research objectives. By providing a concise summary of your findings, you can guide readers through the main outcomes of your study.
Step 4: Compare and Relate with Other Research Works : Research is a collaborative and cumulative process, and it is vital to establish connections between your study and previous research. We will explore strategies to compare and relate your findings to existing literature, highlighting similarities, differences, and gaps in knowledge.
Step 5: Provide Alternate Viewpoints: Science thrives on the diversity of perspectives. Acknowledging different viewpoints and interpretations of your results fosters a more comprehensive understanding of the research topic. We will discuss how to incorporate alternative viewpoints into your discussion, encouraging a balanced and nuanced analysis.
Step 6: Show Future Directions : A well-crafted discussion section not only summarizes the present but also points towards the future. We will explore techniques to suggest future research directions based on the implications of your study, providing a roadmap for further investigations in the field.
Step 7: Concluding Thoughts : In the final step, we will wrap up the discussion section by summarizing the key points and emphasizing the overall implications of your research. We will discuss the significance of your study’s contributions and offer some closing thoughts to leave a lasting impression on your readers.
By following these seven steps, you can craft a comprehensive and insightful discussion section that not only synthesizes your findings but also engages readers in a thought-provoking dialogue about the broader implications and future directions of your research. Let’s delve into each step in detail to enhance the quality and impact of your discussion section.
The purpose of every research is to implement the results for the positive development of the relevant subject. In research, it is crucial to emphasize the relevance of your study to the field and its potential impact. Before delving into the details of how the research was conceived and the sequence of developments that took place, consider highlighting the following factors to establish the relevance of your work:
- Identifying a pressing problem or research gap: Example: “This research addresses the critical problem of network security in wireless communication systems. With the widespread adoption of wireless networks, the vulnerability to security threats has increased significantly. Existing security mechanisms have limitations in effectively mitigating these threats. Therefore, there is a pressing need to develop novel approaches that enhance the security of wireless communication systems.”
- Explaining the significance and potential impact of the research: Example: “By developing an intelligent intrusion detection system using machine learning algorithms, this research aims to significantly enhance the security of wireless networks. The successful implementation of such a system would not only protect sensitive data and communication but also ensure the reliability and integrity of wireless networks in various applications, including Internet of Things (IoT), smart cities, and critical infrastructure.”
- Establishing connections with previous research and advancements in the field: Example: “This study builds upon previous research on intrusion detection systems and machine learning techniques. By leveraging recent advancements in deep learning algorithms and anomaly detection methods, we aim to overcome the limitations of traditional rule-based intrusion detection systems and achieve higher detection accuracy and efficiency.”
By emphasizing the relevance of your research and articulating its potential impact, you set the stage for readers to understand the significance of your work in the broader context. This approach ensures that readers grasp the motivations behind your research and the need for further exploration in the field.
II. Highlight the Limitations
Many times the research is on a subject that might have legal limitations or restrictions. This limitation might have caused certain imperfections in carrying out research or in results. This issue should be acknowledged by the researcher before the work is criticized by others later in his/her discussion section.
In computer science research, it is important to identify and openly acknowledge the limitations of your study. By doing so, you demonstrate transparency and a thorough understanding of potential weaknesses, allowing readers to interpret the findings in a more informed manner. Here’s an example:
Example: “It is crucial to acknowledge certain limitations and constraints that have affected the outcomes of this research. In the context of privacy-sensitive applications such as facial recognition systems, there are legal limitations and ethical concerns that can impact the accuracy and performance of the developed algorithm. These limitations stem from regulations and policies that impose restrictions on data collection, access, and usage to protect individuals’ privacy rights. As a result, the algorithm developed in this study operates under these legal constraints, which may have introduced certain imperfections.”
In this example, the researcher is working on a facial recognition system and acknowledges the legal limitations and ethical concerns associated with privacy-sensitive applications. By openly addressing these limitations, the researcher demonstrates an understanding of the challenges imposed by regulations and policies. This acknowledgement sets the stage for a more nuanced discussion and prevents others from solely criticizing the work based on these limitations without considering the broader legal context.
By highlighting the limitations, researchers can also offer potential solutions or future directions to mitigate the impact of these constraints. For instance, the researcher may suggest exploring advanced privacy-preserving techniques or collaborating with legal experts to find a balance between privacy protection and system performance.
By acknowledging and addressing the limitations, researchers demonstrate their awareness of potential weaknesses in their study, maintaining credibility, and fostering a more constructive discussion of their findings within the context of legal and ethical considerations.
III. Introduce New Discoveries
Begin the discussion section by stating all the major findings in the course of the research. The first paragraph should have the findings mentioned, which is expected to be synoptic, naming and briefly describing the analysis of results.
Example: “In this study, several significant discoveries emerged from the analysis of the collected data. The findings revealed compelling insights into the performance of parallel computing architectures for large-scale data processing. Through comprehensive experimentation and analysis, the following key discoveries were made:
- Discovery 1: The proposed parallel computing architecture demonstrated a 30% improvement in processing speed compared to traditional sequential computing methods. This finding highlights the potential of parallel computing for accelerating data-intensive tasks.
- Discovery 2: A direct relationship between the number of processing cores and the overall system throughput was observed. As the number of cores increased, the system exhibited a near-linear scalability, enabling efficient utilization of available computational resources.
- Discovery 3: The analysis revealed a trade-off between processing speed and energy consumption. While parallel computing achieved faster processing times, it also resulted in higher energy consumption. This finding emphasizes the importance of optimizing energy efficiency in parallel computing systems.
These discoveries shed light on the performance characteristics and trade-offs associated with parallel computing architectures for large-scale data processing tasks. The following sections will delve into the implications of these findings, discussing their significance, limitations, and potential applications.”
In this example, the researcher presents a concise overview of the major discoveries made during the research. Each discovery is briefly described, highlighting the key insights obtained from the analysis. By summarizing the findings in a synoptic manner, the reader gains an immediate understanding of the notable contributions and can anticipate the subsequent detailed discussion.
This approach allows the discussion section to begin with a clear and impactful introduction of the major discoveries, capturing the reader’s interest and setting the stage for a comprehensive exploration of each finding in subsequent paragraphs.
Coming to the major part of the findings, the discussion section should interpret the key observations, the analysis of charts, and the analysis of tables. In the field of computer science, presenting and explaining the results in a clear and accessible manner is essential for readers to grasp the significance of the findings. Here are some examples of how to effectively highlight observations in computer science research:
Begin with explaining the objective of the research, followed by what inspired you as a researcher to study the subject:
In a study on machine learning algorithms for sentiment analysis, start by stating the goal of developing an accurate and efficient sentiment analysis model. Share your motivation for choosing this research topic, such as the increasing importance of sentiment analysis in various domains like social media, customer feedback analysis, and market research.
Example: The objective of this research was to develop a sentiment analysis model using machine learning algorithms. As sentiment analysis plays a vital role in understanding public opinion and customer feedback, we were motivated by the need for an accurate and efficient model that could be applied in various domains such as social media analysis, customer reviews, and market research.
Explain the meaning of the findings, as every reader might not understand the analysis of graphs and charts as easily as people who are in the same field as you:
If your research involves analyzing performance metrics of different algorithms, consider presenting the results in a visually intuitive manner, such as line graphs or bar charts. In the discussion section, explain the significance of the trends observed in the graphs. For instance, if a particular algorithm consistently outperforms others in terms of accuracy, explain why this finding is noteworthy and how it aligns with existing knowledge in the field.
Example: To present the performance evaluation of the algorithms, we analyzed multiple metrics, including precision, recall, and F1 score. The line graph in Figure 1 demonstrates the trends observed. It is noteworthy that Algorithm A consistently outperformed the other algorithms across all metrics. This finding indicates that Algorithm A has a higher ability to accurately classify sentiment in comparison to its counterparts. This aligns with previous studies that have also highlighted the robustness of Algorithm A in sentiment analysis tasks.
Ensure the reader can understand the key observations without being forced to go through the whole paper:
In computer science research, it is crucial to present concise summaries of your key observations to facilitate understanding for readers who may not have the time or expertise to go through the entire paper. For example, if your study compares the runtime performance of two programming languages for a specific task, clearly state the observed differences and their implications. Highlight any unexpected or notable findings that may challenge conventional wisdom or open up new avenues for future exploration.
Example: In this study comparing the runtime performance of Python and Java for a specific computational task, we observed notable differences. Python consistently showed faster execution times, averaging 20% less time than Java across varying input sizes. These results challenge the common perception that Java is the superior choice for computationally intensive tasks. The observed performance advantage of Python in this context suggests the need for further investigation into the underlying factors contributing to this discrepancy, such as differences in language design and optimization strategies.
By employing these strategies, researchers can effectively highlight their observations in the discussion section. This enables readers to gain a clear understanding of the significance of the findings and their implications without having to delve into complex technical details.
No one is ever the only person researching a particular subject. A researcher always has companions and competitors. The discussion section should have a detailed comparison of the research. It should present the facts that relate the research to studies done on the same subject.
Example: The table below compares some of the well-known prediction techniques with our fuzzy predictor with MOM defuzzification for response time, relative error and Environmental constraints. Based on the results obtained it can be concluded that the Fuzzy predictor with MOM defuzzification has a less relative error and quick response time as compared to other prediction techniques. The proposed predictor is more flexible, simple to implement and deals with noisy and uncertain data from real-life situations. The relative error of 5-10% is acceptable for our system as the predicted fuzzy region and the fuzzy region of the actual position remains the same.
Table 1 : Comparison of well-known Robot Motion prediction Techniques
VI. Provide Alternate View Points
Almost every time, it has been noticed that analysis of charts and graphs shows results that tend to have more than one explanation. The researcher must consider every possible explanation and potential enhancement of the study from alternative viewpoints. It is critically important that this is clearly put out to the readers in the discussion section.
In the discussion section of a research paper, it is important to acknowledge that data analysis often yields results that can be interpreted in multiple ways. By considering different viewpoints and potential enhancements, researchers can provide a more comprehensive and nuanced analysis of their findings. Here are some examples:
Example 1: “The analysis of our experimental data showed a decrease in system performance following the implementation of the proposed optimization technique. While our initial interpretation suggested that the optimization failed to achieve the desired outcome, an alternate viewpoint could be that the decrease in performance was influenced by an external factor, such as the configuration of the hardware setup. Further investigation into the hardware settings and benchmarking protocols is necessary to fully understand the observed results and identify potential enhancements.”
Example 2: “The analysis of user feedback revealed a mixed response to the redesigned user interface. While some participants reported improved usability and satisfaction, others expressed confusion and dissatisfaction. An alternate viewpoint could be that the diverse range of user backgrounds and preferences might have influenced these varied responses. Further research should focus on conducting user studies with a larger and more diverse sample to gain a deeper understanding of the underlying factors contributing to the contrasting user experiences.”
Example 3: “Our study found a positive correlation between the implementation of agile methodologies and project success rates. However, an alternate viewpoint suggests that other factors, such as team dynamics and project complexity, could have influenced the observed correlation. Future research should explore the interactions between agile methodologies and these potential confounding factors to gain a more comprehensive understanding of their impact on project success.”
In these examples, researchers present alternative viewpoints that offer different interpretations or explanations for the observed results. By acknowledging these alternate viewpoints, researchers demonstrate a balanced and comprehensive analysis of their findings. It is crucial to clearly communicate these alternative perspectives to readers in the discussion section, as it encourages critical thinking and highlights the complexity and potential limitations of the research.
By presenting alternate viewpoints, researchers invite further exploration and discussion, fostering a more comprehensive understanding of the research topic. This approach enriches the scientific discourse and promotes a deeper analysis of the findings, contributing to the overall advancement of knowledge in the field.
VII. Future Directions and Conclusion
The section must have suggestions for research that should be done to unanswered questions. These should be suggested at the beginning of the discussion section to avoid questions being asked by critics. Emphasizing the importance of following future directions can lead to new research as well.
Example: ” While this study provides valuable insights into the performance of the proposed algorithm, there are several unanswered questions and avenues for future research that merit attention. By identifying these areas, we aim to stimulate further exploration and contribute to the continuous advancement of the field. The following future directions are suggested:
- Future Direction 1: Investigating the algorithm’s performance under different dataset characteristics and distributions. The current study focused on a specific dataset, but it would be valuable to evaluate the algorithm’s robustness and generalizability across a broader range of datasets, including real-world scenarios and diverse data sources.
- Future Direction 2: Exploring the potential integration of additional machine learning techniques or ensemble methods to further enhance the algorithm’s accuracy and reliability. By combining the strengths of multiple models, it is possible to achieve better performance and handle complex patterns and outliers more effectively.
- Future Direction 3: Extending the evaluation to consider the algorithm’s scalability in large-scale deployment scenarios. As the volume of data continues to grow exponentially, it is crucial to assess the algorithm’s efficiency and scalability in handling big data processing requirements.
By suggesting these future directions, we hope to inspire researchers to explore new avenues and build upon the foundation laid by this study. Addressing these unanswered questions will contribute to a more comprehensive understanding of the algorithm’s capabilities and limitations, paving the way for further advancements in the field.”
In this example, the researcher presents specific future directions that can guide further research. Each future direction is described concisely, highlighting the specific area of investigation and the potential benefits of pursuing those directions. By suggesting these future directions early in the discussion section, the researcher proactively addresses potential questions or criticisms and demonstrates a proactive approach to knowledge expansion.
By emphasizing the importance of following future directions, researchers not only inspire others to continue the research trajectory but also contribute to the collective growth of the field. This approach encourages ongoing exploration, innovation, and collaboration, ensuring the continuous development and improvement of computer science research.
In the final step, wrap up the discussion section by summarizing the key points and emphasizing the overall implications of your research. We will discuss the significance of your study’s contributions and offer some closing thoughts to leave a lasting impression on your readers. This section serves as a crucial opportunity to reinforce the main findings and highlight the broader impact of your work. Here are some examples:
Example 1: “In conclusion, this research has made significant contributions to the field of natural language processing. By proposing a novel neural network architecture for language generation, we have demonstrated the effectiveness and versatility of the model in generating coherent and contextually relevant sentences. The experimental results indicate a significant improvement in language generation quality compared to existing approaches. The implications of this research extend beyond traditional applications, opening up new possibilities for automated content creation, chatbot systems, and dialogue generation in artificial intelligence.”
Example 2: “In summary, this study has provided valuable insights into the optimization of network routing protocols for wireless sensor networks. By proposing a novel hybrid routing algorithm that combines the advantages of both reactive and proactive protocols, we have demonstrated enhanced network performance in terms of latency, energy efficiency, and scalability. The experimental results validate the effectiveness of the proposed algorithm in dynamic and resource-constrained environments. These findings have implications for various applications, including environmental monitoring, industrial automation, and smart city infrastructure.”
Example 3: “In closing, this research sheds light on the security vulnerabilities of blockchain-based smart contracts. By conducting an extensive analysis of existing smart contract platforms and identifying potential attack vectors, we have highlighted the need for robust security measures to mitigate risks and protect user assets. The insights gained from this study can guide the development of more secure and reliable smart contract frameworks, ensuring the integrity and trustworthiness of blockchain-based applications across industries such as finance, supply chain, and decentralized applications.”
In these examples, the concluding thoughts summarize the main contributions and findings of the research. They emphasize the significance of the study’s implications and highlight the potential impact on various domains within computer science. By providing a succinct and impactful summary, the researcher leaves a lasting impression on readers, reinforcing the value and relevance of the research in the field.
Validating claims in the discussion section of a research paper is essential to ensure the credibility and reliability of your findings. Here are some strategies to validate the claims made in the discussion section:
- Referencing supporting evidence: Cite relevant sources from the existing literature that provide evidence or support for your claims. These sources can include peer-reviewed studies, research articles, and authoritative sources in your field. By referencing credible and reputable sources, you establish the validity of your claims and demonstrate that your interpretations are grounded in existing knowledge.
- Relating to the results: Connect your claims to the results presented in the earlier sections of your research paper. Clearly demonstrate how the findings support your claims and provide evidence for your interpretations. Refer to specific data, measurements, statistical analyses, or other evidence from your results section to substantiate your claims.
- Comparing with previous research: Discuss how your findings align with or diverge from previous research in the field. Reference relevant studies and explain how your results compare to or build upon existing knowledge. By contextualizing your claims within the broader research landscape, you provide further validation for your interpretations.
- Addressing limitations and alternative explanations: Acknowledge the limitations of your study and consider alternative explanations for your findings. By addressing potential counterarguments and alternative viewpoints, you demonstrate a thorough evaluation of your claims and increase the robustness of your conclusions.
- Seeking peer feedback: Prior to submitting your research paper, consider seeking feedback from colleagues or experts in your field. They can provide valuable insights and suggestions for further validating your claims or improving the clarity of your arguments.
- Inviting replication and further research: Encourage other researchers to replicate your study or conduct further investigations. By promoting replication and future research, you contribute to the ongoing validation and refinement of your claims.
Remember, the validation of claims in the discussion section is a critical aspect of scientific research. By employing rigorous methods and logical reasoning, you can strengthen the credibility and impact of your findings and contribute to the advancement of knowledge in your field.
Here are some common phrases that can be used in the discussion section of a paper or research article. I’ve included a table with examples to illustrate how these phrases might be used:
Here are some common academic phrases that can be used in the analysis section of a paper or research article. I have included a table with examples to illustrate how these phrases might be used:
Your Next Move…
I believe you will proceed to write conclusion section of your research paper. Conclusion section is the most neglected part of the research paper as many authors feel it is unnecessary but write in a hurry to submit the article to some reputed journal.
Please note, once your paper gets published , the readers decide to read your full paper based only on abstract and conclusion. They decide the relevance of the paper based on only these two sections. If they don’t read then they don’t cite and this in turn affects your citation score. So my sincere advice to you is not to neglect this section.
Visit my article on “How to Write Conclusion Section of Research Paper” for further details.
Please visit my article on “ Importance and Improving of Citation Score for Your Research Paper ” for increasing your visibility in research community and on Google Scholar Citation Score.
The Discussion section of a research paper is an essential part of any study, as it allows the author to interpret their results and contextualize their findings. To write an effective Discussion section, authors should focus on the relevance of their research, highlight the limitations, introduce new discoveries, highlight their observations, compare and relate their findings to other research works, provide alternate viewpoints, and show future directions.
By following these 7 steps, authors can ensure that their Discussion section is comprehensive, informative, and thought-provoking. A well-written Discussion section not only helps the author interpret their results but also provides insights into the implications and applications of their research.
In conclusion, the Discussion section is an integral part of any research paper, and by following these 7 steps, authors can write a compelling and informative discussion section that contributes to the broader scientific community.
Frequently Asked Questions
Yes, charts and graphs are generally allowed in the discussion section of a research paper. While the discussion section is primarily focused on interpreting and discussing the findings, incorporating visual aids such as charts and graphs can be helpful in presenting and supporting the analysis.
Yes, you can add citations in the discussion section of your research paper. In fact, it is highly recommended to support your statements, interpretations, and claims with relevant and credible sources. Citations in the discussion section help to strengthen the validity and reliability of your arguments and demonstrate that your findings are grounded in existing literature.
Combining the results and discussion sections in a research paper is a common practice in certain disciplines, particularly in shorter research papers or those with specific formatting requirements. This approach can help streamline the presentation of your findings and provide a more cohesive narrative. However, it is important to note that the decision to combine these sections should be based on the guidelines of the target journal or publication and the specific requirements of your field.
The weightage of the discussion section in terms of the selection of a research paper for publication in a journal can vary depending on the specific requirements and criteria of the journal. However, it is important to note that the discussion section is a critical component of a research paper as it allows researchers to interpret their findings, contextualize them within the existing literature, and discuss their implications.
In general, literature survey papers typically do not have a separate section explicitly labeled as “Discussion.” However, the content of a literature survey paper often incorporates elements of discussion throughout the paper. The focus of a literature survey paper is to review and summarize existing literature on a specific topic or research question, rather than presenting original research findings.
Upcoming Events
- Visit the Upcoming International Conferences at Exotic Travel Destinations with Travel Plan
- Visit for Research Internships Worldwide
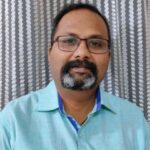
Leave a Reply Cancel reply
You must be logged in to post a comment.
Recent Posts
- Top 10 AI-Based Research Paper Abstract Generators
- EditPad Research Title Generator: Is It Helpful to Create a Title for Your Research?
- Are Postdoctoral Fellowships Taxable? A Guide to Understanding Tax Implications
- How to Get Off-Cycle Research/Academic Internships
- How to End Your Academic/Research Internship?
- All Blog Posts
- Research Career
- Research Conference
- Research Internship
- Research Journal
- Research Tools
- Uncategorized
- Research Conferences
- Research Journals
- Research Grants
- Internships
- Research Internships
- Email Templates
- Conferences
- Blog Partners
- Privacy Policy
Copyright © 2024 Research Voyage
Design by ThemesDNA.com

Thank you for visiting nature.com. You are using a browser version with limited support for CSS. To obtain the best experience, we recommend you use a more up to date browser (or turn off compatibility mode in Internet Explorer). In the meantime, to ensure continued support, we are displaying the site without styles and JavaScript.
- View all journals
- My Account Login
- Explore content
- About the journal
- Publish with us
- Sign up for alerts
- Open access
- Published: 11 May 2024
Enhancing cervical cancer detection and robust classification through a fusion of deep learning models
- Sandeep Kumar Mathivanan 1 ,
- Divya Francis 2 ,
- Saravanan Srinivasan 3 ,
- Vaibhav Khatavkar 4 ,
- Karthikeyan P 5 &
- Mohd Asif Shah 6 , 7
Scientific Reports volume 14 , Article number: 10812 ( 2024 ) Cite this article
407 Accesses
Metrics details
- Health care
- Medical research
Cervical cancer, the second most prevalent cancer affecting women, arises from abnormal cell growth in the cervix, a crucial anatomical structure within the uterus. The significance of early detection cannot be overstated, prompting the use of various screening methods such as Pap smears, colposcopy, and Human Papillomavirus (HPV) testing to identify potential risks and initiate timely intervention. These screening procedures encompass visual inspections, Pap smears, colposcopies, biopsies, and HPV-DNA testing, each demanding the specialized knowledge and skills of experienced physicians and pathologists due to the inherently subjective nature of cancer diagnosis. In response to the imperative for efficient and intelligent screening, this article introduces a groundbreaking methodology that leverages pre-trained deep neural network models, including Alexnet, Resnet-101, Resnet-152, and InceptionV3, for feature extraction. The fine-tuning of these models is accompanied by the integration of diverse machine learning algorithms, with ResNet152 showcasing exceptional performance, achieving an impressive accuracy rate of 98.08%. It is noteworthy that the SIPaKMeD dataset, publicly accessible and utilized in this study, contributes to the transparency and reproducibility of our findings. The proposed hybrid methodology combines aspects of DL and ML for cervical cancer classification. Most intricate and complicated features from images can be extracted through DL. Further various ML algorithms can be implemented on extracted features. This innovative approach not only holds promise for significantly improving cervical cancer detection but also underscores the transformative potential of intelligent automation within the realm of medical diagnostics, paving the way for more accurate and timely interventions.
Similar content being viewed by others
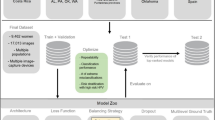
Reproducible and clinically translatable deep neural networks for cervical screening
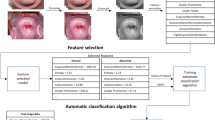
Comparison of machine and deep learning for the classification of cervical cancer based on cervicography images
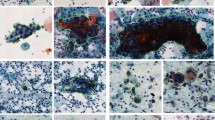
Artificial intelligence-assisted fast screening cervical high grade squamous intraepithelial lesion and squamous cell carcinoma diagnosis and treatment planning
Introduction.
Cervical cancer, a prevalent malignancy significantly affecting women, presents significant health challenges, particularly in underdeveloped nations with high morbidity and mortality rates 1 . In countries like India, cervical cancer constitutes approximately 6–29% of all female cancer diagnoses, primarily focusing on squamous cells. The disease classifies into three stages: CIN1, CIN2, and CIN3, representing mild, moderate, and severe stages, respectively 2 . Initiated by Human Papillomavirus (HPV), specifically high-risk strains, cervical cancer involves aberrant transformations in cervix cells, leading to the synthesis of E6 and E7 proteins 3 . These proteins, influencing tumor suppressor genes, play a pivotal role in cancer initiation. In the subjective field of cancer diagnosis, heavily reliant on pathologists and gynaecologists, artificial intelligence, particularly deep learning (DL), has streamlined the diagnostic process 4 . DL automates intricate feature extraction, excelling at recognizing inherent traits within images and enhancing performance 3 . As a favoured approach for cancer categorization, DL methodologies revolutionize image processing by eliminating the need for explicit feature extraction 5 . In cervical cancer diagnosis, tests like HPV testing, PAP testing, colposcopy, and biopsy are crucial, with AI increasingly playing a prominent role in prognosis and diagnostics 6 . DL’s ability to automatically classify images by learning high-level characteristics empowers pathologists in the challenging task of cancer diagnosis. Prevention and screening programs, encompassing various tests, are pivotal components in the ongoing fight against cervical cancer 7 .
While PAP smear image screening remains a primary method for addressing cervical cancer, it presents challenges. It demands a higher volume of microscopic examinations for both cancer and noncancer cases, is time-intensive, and necessitates the expertise of trained professionals 8 . Additionally, there exists a risk of overlooking positive cases when employing conventional screening techniques. Both PAP smears and HPV tests, despite their expense, offer limited sensitivity in cancer detection. Colposcopy screening serves as a vital complement to address the limitations of PAP smear images and HPV tests 9 . Early detection of cervical and other cancers becomes more feasible, even in the absence of discernible signs and symptoms. Successful screening programs hold the potential to prevent cervical cancer fatalities, ultimately reducing disease burden and suffering. Colposcopy, a widely employed surgical technique, plays a crucial role in cervical cancer screening 10 . Swift identification and categorization of this cancer type can significantly enhance the patient's overall clinical management. Numerous research publications have explored diverse methodologies within digital colposcopy to extract valuable insights from images 11 . The overarching aim of these studies is to equip healthcare practitioners with valuable resources during colposcopy examinations, catering to their varying levels of expertise. Previous research in diagnosis has harnessed computer-aided systems for a myriad of tasks, encompassing image quality enhancement and assessment, regional segmentation, image recognition, identification of unstable regions and patterns, classification of transition zone types (TZ), and cancer risk assessment. Computer-aided design (CAD) tools play a pivotal role in enhancing the quality of cervical colposcopy images, segmenting regions of concern, and pinpointing specific anomalies 12 . These strategies prove invaluable to physicians in their diagnostic processes, although they require a solid foundation of experience and skill to establish precise diagnoses. Detecting diseased areas, such as potential neoplasms, during a colposcopy examination assumes critical importance. Noteworthy examples of these abnormal regions include acetowhite areas, aberrant vascularization, mosaic patterns, and punctate lesions 13 .
Our proposed approach skilfully combines the power of DL with traditional machine-learning (ML) methods. We extract features by carefully collecting activation values from deep neural networks. Then, we use a different type of classifiers, such as Simple Logistic, Principal Component Analysis, and Random Tree techniques, to make accurate classifications. In our work with the SIPaKMeD dataset, we take a comprehensive approach, as shown in Fig. 1 , to provide a clear overview of our system. We use DL techniques for feature extraction and employ a wide range of ML methods for classification. We thoroughly tested our system on the SIPaKMeD dataset, using a range of pre-trained models like Alexnet, Resnet-101, Resnet-152, and InceptionV3 for feature extraction. Our innovative approach consistently outperforms other models, achieving higher accuracy with the Simple Logistic model on the testing set. In this article, we introduce a hybrid methodology that combines DL and ML elements, presenting a comprehensive comparison of different pre-trained DL models. This research sheds light on their effectiveness in addressing cervical cancer classification challenges.
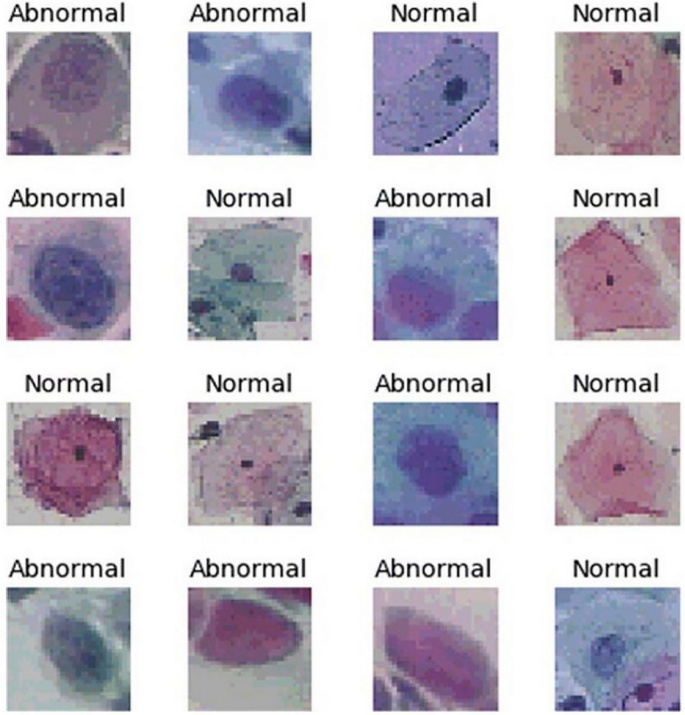
Sample normal and abnormal images from dataset.
The paper is structured as follows: section “ Introduction ” provides a concise overview of key concepts. Section “ Related work ” delves into existing research on ML and DL methods in cervical cancer. Section “ Materials and methods ” offers a detailed explanation of our novel approach. Section “ Experimental results and discussion ” outlines the datasets used. Section “ Conclusion ” discusses the outcomes of our experiments. Conclusion provides a summary of the paper’s key findings and insights.
Related work
The author introduced two deep learning convolutional neural network (CNN) architectures, namely the VGG19 (TL) model and CYENET, for the purpose of cervical cancer detection using colposcopy images. The VGG19 model is utilized as a transfer learning component within our CNN architecture. Our efforts in automatic cervical cancer classification through colposcopy images have culminated in the creation of a novel model known as the Colposcopy Ensemble Network, or CYENET. We have evaluated the predictive performance of this model, estimating its accuracy, specificity, and sensitivity. Specifically, the VGG19 (TL) model achieves a commendable 73.3% success rate in classifying the data, yielding satisfactory results overall. Furthermore, the VGG19 model, in the transfer learning context, exhibits a moderate kappa score for classification tasks. Our experimental findings underscore the excellence of the proposed CYENET model, which demonstrates remarkable sensitivity, specificity, and high kappa values, with percentages of 92.4%, 96.2%, and 88%, respectively. These results highlight the efficacy of our approach in cervical cancer detection using colposcopy images 14 . The author conducted a cervical cell categorization study using the publicly available SIPaKMeD dataset, which contains five cell classifications: superficial-intermediate, parabasal, koilocytotic, metaplastic, and dyskeratotic. The study employed a CNN to distinguish between healthy cervical cells, cells with precancerous abnormalities, and benign cells. Following the segmentation of Pap smear images, cervical cells in the resulting enhanced images were analyzed using a deep CNN with four convolutional layers and obtained an accuracy rate of 91.13% 15 .
The aim of author research was to leverage deep learning techniques to establish an integrated framework for automated cervix type classification and cervical cancer detection. To achieve this, we collected a comprehensive dataset consisting of 4005 colposcopy photos and 915 histopathology images from diverse clinical sources and publicly available databases. To enhance our approach, we developed a streamlined MobileNetv2-YOLOv3 model, which was trained and validated to identify the transformation region within cervix images. This region served as the Region of Interest (ROI) for subsequent classification. The ROI extraction process exhibited exceptional performance, with a mean average accuracy (mAP) of 99.88%. Furthermore, cervix type and cervical cancer classification tests achieved high accuracy rates, with scores of 96.84% and 94.50%, respectively. These results demonstrate the effectiveness of the approach in automating cervix type categorization and cervical cancer detection 16 . When LBCs and biopsies were initially tested using a modified general primer for HPV PCR, any samples that were found to be HPV-negative underwent subsequent whole genome sequencing. Out of the 1052 pre-CIN3+ LBC samples, HPV was detected in an impressive 97.0% (1020 samples) using the Cobas 4800 assay. Additionally, nine samples revealed HPV strains that were not specifically covered by the Cobas 4800 test. Remarkably, only 4 out of the 1052 samples (0.4%) showed no presence of HPV. In contrast, 91.6% of CIN3+ patients had previously tested positive for HPV using cytology. This underscores the high sensitivity of the standard HPV screening test within the context of the actual screening program, where it demonstrated an impressive sensitivity rate of 97.0% 17 . Author conducted a comprehensive study to assess the effectiveness of concurrent visual inspection with dilute acetic acid (VIA) or mobile colposcopy when compared to standalone high-risk human papillomavirus (hr-HPV) DNA testing (utilizing platforms like careHPV, GeneXpert, AmpFire, or MA-6000) in a real-world, resource-constrained setting. Additionally, author investigated the rate at which participants were subsequently lost to follow-up. The 'positivity' rates for EVA and VIA were 8.6% (95% CI, 6.7–10.6) and 2.1% (95% CI, 1.6–2.5), respectively, while the hr-HPV ‘positivity’ rate was 17.9% (95% CI, 16.7–19.0). It's noteworthy that a substantial majority of women in the cohort tested negative for both hr-HPV DNA and visual inspection (3588 out of 4482, or 80.1%). A smaller percentage, 2.1% (95% CI, 1.7–2.6), tested hr-HPV-negative but positive on visual inspection. In total, 51 women in the group tested positive on both measures. Out of the 274 individuals who tested positive for hr-HPV in a standalone test, a significant proportion, 191 (69.5%), returned for at least one follow-up visit 18 . Author study explores four distinct subsets: breast vs. cervical cancer, internal vs. external validation, comparing mammography, ultrasound, cytology, and colposcopy, and assessing the performance of deep learning (DL) algorithms versus human doctors. Based on a comprehensive analysis of 35 studies that met the inclusion criteria for this systematic review, author found that the pooled sensitivity stands at 88% (95% CI 85–90%), the specificity at 84% (79–87%), and the Area Under the Curve (AUC) at an impressive 0.92 (0.90–0.94) 19 .
To diagnose cervical cancer effectively, this study explores a wide array of both online and offline machine learning algorithms using benchmarked datasets. Additionally, hybrid techniques are employed to address segmentation challenges, and the feature count is optimized through the incorporation of tree classifiers. Remarkably, certain algorithms can attain accuracy, precision, recall, and F1 scores exceeding 100% as the training data percentage increases. While approaches such as logistic regression with L1 regularization can indeed achieve 100% accuracy, it's worth noting that they may come at a higher computational cost in terms of CPU time compared to other methods that can still deliver a commendable 99% accuracy with significantly lower computational demands 20 . The author used supervised machine learning to detect cervical cancer at an early stage. Author trained a machine learning model using a dataset from UCI that contains information related to cervical cancer. To assess how well our classifiers performed and how accurate they were, we trained them with and without a feature selection process. The author employed various feature selection methods, including Relief rank, the wrapper approach, and LASSO regression. Impressively, when we used all the features, the XG Boost model achieved a high accuracy rate of 94.94%. Interestingly, in some cases, the feature selection techniques performed even better 21 . Author proposed, deep learning plays a pivotal role in two distinct approaches. The first approach involves using pre-trained feature extractor models along with machine learning algorithms to classify cervical cancer images. In this case, ResNet-50 achieves the highest classification accuracy of 92.03%. The second approach employs transfer learning, where Google Net outperforms other models with a remarkable classification accuracy of 96.01% 22 . Author proposed a deep feature-fed MLP neural network and it incorporates four innovative ideas to adjust the number of neurons in its hidden layers. Additionally, the MLP is enhanced by inputting features extracted from ResNet-34, ResNet-50, and VGG-19 deep networks. The technique involves these two CNNs discarding their classification layers and passing their output through a flatten layer before inputting into the MLP. Both CNNs are trained with the Adam optimizer using relevant images to improve their performance. Remarkably, this proposed approach achieves outstanding results, with an accuracy of 99.23% for two classes and 97.65% for seven classes when evaluated on the Herlev benchmark database 23 . Table 1 , illustrates the detailed comparison information of various state-of-the-methods. In the realm of cervical cancer detection and classification, there exists a noticeable research gap that centers around the need for enhanced methodologies. Current approaches often face limitations in terms of accuracy and robustness. To address this gap, our proposed research aims to leverage the power of deep learning models and their fusion to create a more comprehensive and effective system. By amalgamating the strengths of different deep learning architectures, our research seeks to improve the precision and reliability of cervical cancer detection, ultimately contributing to early diagnosis and better patient outcomes. This exploration into the fusion of deep learning models represents a novel avenue in the pursuit of advancing cervical cancer detection and classification techniques.
Material and methods
Dataset description.
The dataset we used for this study is accessible through this link: https://www.cs.uoi.gr/~marina/sipakmed.html . It contains five different cell types, as detailed in 24 . In our research, we've transformed this dataset into a two-class system with two categories: normal and abnormal. Specifically, the normal category includes superficial intermediate cells and parabasal cells, while the aberrant category covers koilocytotic, dyskeratotic, and metaplastic cell types 25 . Within the normal category, we've further divided cells into two subcategories: superficial intermediate cells and parabasal cells. The essential dataset characteristics are summarized in Table 2 . The SIPaKMeD dataset comprises a total of 4068 images, with 3254 allocated for training (making up 80% of the total), and 813 set aside for testing (accounting for 20% of the total). This dataset consists of two distinct classes: normal photos, totalling 1618, and aberrant images, amounting to 2450. Figure 2 provides visual examples of photographs from these two different categories. The existing literature extensively covers different screening methods for cervical cancer, such as Pap smear, colposcopy, and HPV testing, emphasizing the importance of early detection. However, a significant gap exists in automated screening systems using pap smear images. Traditional methods rely on expert interpretation, but integrating deep learning (DL) and machine learning (ML) offers potential for intelligent automation. Despite this potential, few studies focus on developing and evaluating such systems specifically for cervical cancer prediction using pap smear images. This research addresses this gap by proposing a methodology that utilizes pre-trained deep neural network models for feature extraction and applies various ML algorithms for prediction. The study aims to contribute to advancing automated screening systems for cervical cancer, aiming to improve early detection and patient outcomes.
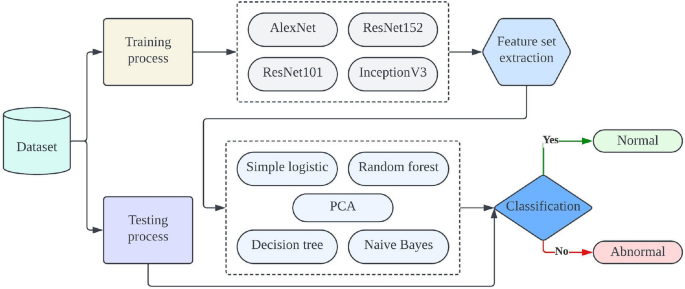
Proposed model cervical cancer classification.
The schematic representation of our proposed system can be observed in Fig. 2 . To facilitate the classification task for cervical cancer, we employ the SIPaKMeD dataset, which comprises images of pap smears. This dataset is categorized into two groups: abnormal and normal, with a distribution of 60% for training and 40% for testing. To extract relevant feature sets from well-established CNN architectures such as Alexnet, Resnet-101, Resnet-152, and InceptionV3, we initiate feature extraction from these pretrained CNN models. This step allows us to gather valuable information from the final layer activation values. For the task of classifying images into normal and abnormal categories, we leverage a variety of machine learning techniques, including Simple Logistic, Decision Tree, Random Forest, Naive Bayes, and Principal Component Analysis. Our approach is designed as a hybrid strategy, merging both DL and ML methodologies. The utilization of DL enables our model to capture intricate and complex features inherent in the data, while ML provides the necessary flexibility to handle diverse scenarios. By harnessing the last layer of pretrained models for feature extraction, we enable different machine learning algorithms to classify data based on these extracted attributes. This combination of DL and ML enhances our system's ability to effectively categorize cervical cancer cases.
Pre-trained neural networks
The pre-trained model has undergone training on a larger dataset, acquiring specific weights and biases that encapsulate the dataset's distinctive characteristics. This model has been effectively employed for making predictions based on data. The transferability of learned features to other datasets is possible because certain fundamental abstract properties remain consistent across various types of images. By utilizing pre-trained models, significant time and effort savings are achieved, as a substantial portion of the feature extraction process has already been completed. Noteworthy examples of pre-trained models include Resnet152, ResNet101, Inceptionv3, and Alexnet, which are summarized in Table 3 for reference.
The image classification framework based on ResNet-101 consists of two main parts: feature extraction and feature classification. In Fig. 3 , you can see how the feature extractor is built, comprising five main convolution modules with a total of one hundred convolution layers, an average pooling layer, and a fully connected layer 26 . Once the features are extracted, they are used to train a classifier with a Softmax structure. Table 4 lists the convolution layers and their configurations in the ResNet-101 backbone. Using shortcut connections to increase data dimensions, the ResNet-101 model significantly improves performance by increasing convolutional depth. These shortcut connections also address the problem of network depth causing degradation by enabling identity mapping. For most binary classification tasks, the loss function is applied using the logical cross-entropy function, as shown in Eq. ( 1 ).
where the ground truth value, \(\% {f_l}\) , and the predicted value, \(\% {q_l}\) , are respectively indicated as the l th training dataset's ground truth and predicted values. The value of the loss, \({k}_{({h_{l}}, \; {q_{l}})}^{b}\) , is then backpropagated through the CNN model. At the same time, the CNN model parameters (weights and biases) are gradually optimised during each epoch. This process continues until the loss is minimised and the CNN model converges to a solution.
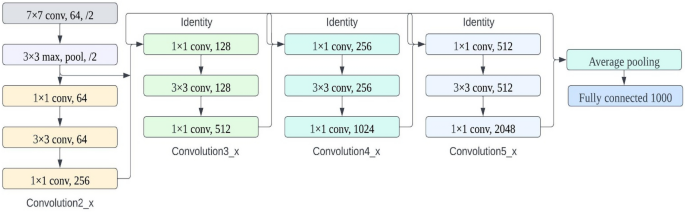
ResNet101 architecture.
The ResNet architecture is efficient, promoting the training of very deep neural networks (DNN) and enhancing accuracy. It addresses the challenge of accuracy degradation associated with increasing network depth. When depth is increased, accuracy often drops, which is a drawback. However, deeper networks can improve accuracy by avoiding the saturation of shallow networks, where errors remain minimal 27 . The key idea here is that information from one layer should easily flow to the next with the help of identity mapping. ResNet tackles the degradation problem, along with the gradient vanishing issue, using residual blocks. These blocks handle the remaining computation while considering the input and output of the block. Figure 4 , illustrates architecture of ResNet152. Table 5 , illustrates the configuration of ResNet152.
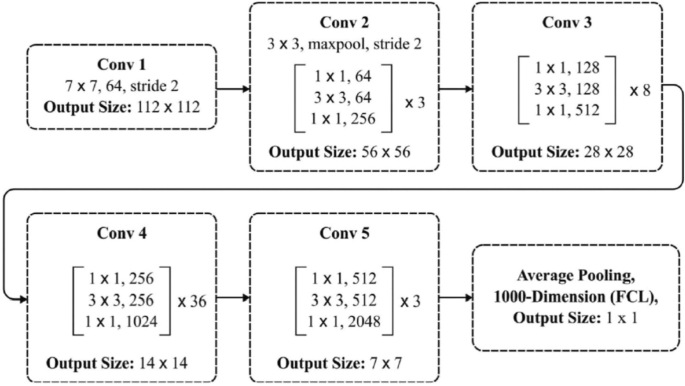
ResNet152 architecture.
InceptionV3
This advanced model has undergone training by one of the industry's most renowned hardware specialists, leveraging an impressive repertoire of over 20 million distinct parameters. The model's architecture is a harmonious blend of symmetrical and asymmetrical construction blocks, each meticulously crafted with its own unique set of convolutional, average, and maximum pooling layers, concatenation operations, and fully connected layers configurations. Furthermore, the model's design incorporates an activation layer that takes advantage of batch normalization, a widely adopted technique in the field. This technique helps stabilize and accelerate the training process, making the model more robust and efficient 28 . For the critical task of classification, the model employs the Softmax method, a popular and well-established approach in machine learning. Softmax is instrumental in producing probability distributions over multiple classes, which enables the model to make informed and precise predictions. To provide a visual understanding of the Inception-V3 model's intricate design, Fig. 5 serves as a diagrammatic representation, offering insights into the model's underlying architecture and the various components that make it a powerhouse in the realm of machine learning and artificial intelligence.
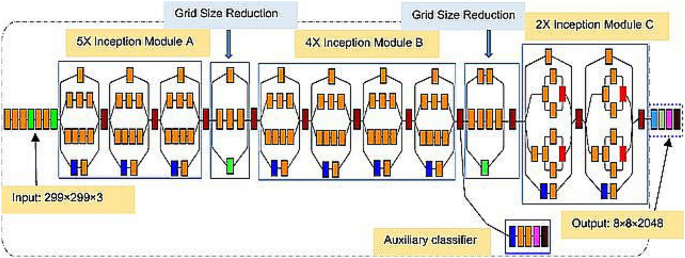
InceptionV3 architecture.
The field of machine learning, particularly in the domain of image processing, has witnessed a profound impact thanks to the advent of Alexnet. As suggested in Ref. 29 , this influential model boasts a preconfigured Convolutional Neural Network (CNN) with a total of eight distinct layers 29 . Its remarkable performance in the 2012 ImageNet Large Scale Visual Recognition Challenge (LSVRC-2012) competition marked a watershed moment, as it clinched victory with a substantial lead over its competitors. The architectural blueprint of Alexnet bears some resemblance to Yann Lecun's pioneering LeNet, highlighting its historical lineage and the evolutionary progress of convolutional neural networks.
Figure 6 provides an insightful visual representation of the holistic design of the Alexnet system. In the journey of data processing within Alexnet, input data traverse through an intricate sequence, comprising five convolution layers and three max-pooling layers, as vividly illustrated in Fig. 5 . These layers play a pivotal role in feature extraction and hierarchical representation, which are vital aspects of image analysis and understanding. The culmination of AlexNet's network journey is marked by the application of the SoftMax activation function in the final layer, enabling it to produce probabilistic class predictions. Along the way, the Rectified Linear Unit (ReLU) activation function is systematically employed across all the network's convolution layers, providing a nonlinear transformation that enhances the network's capacity to learn and extract features effectively. This combination of architectural elements and activation functions has played a significant role in solidifying AlexNet's position as a groundbreaking model in the domain of image processing and machine learning.
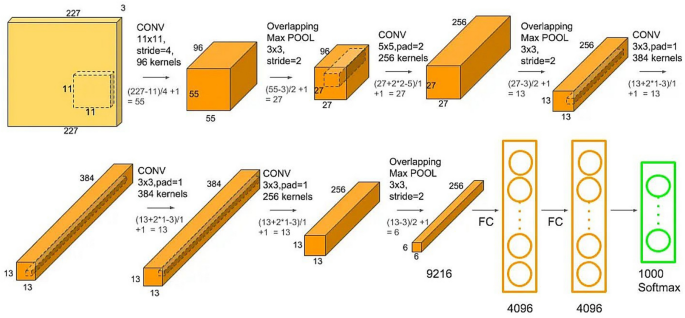
AlexNet architecture.
Simple logistic regression
Logistic regression serves as a powerful method for modelling the probability of a discrete outcome based on input variables, making the choice of input variables a pivotal aspect of this modelling process. The most common application of logistic regression involves modelling a binary outcome, which pertains to scenarios where the result can exclusively assume one of two possible values, such as true or false, yes or no, and the like. However, in situations where there are more than two discrete potential outcomes, multinomial logistic regression proves invaluable in capturing the complexity of the scenario. Logistic regression finds its primary utility in the realm of classification problems 30 . It becomes particularly valuable when the task at hand involves determining which category a new sample best aligns with. This becomes especially pertinent when dealing with substantial datasets, where the need to classify or categorize data efficiently and accurately is paramount. One noteworthy domain where logistic regression finds widespread application is in cybersecurity, where classification challenges are ubiquitous. A pertinent example is the detection of cyberattacks. Here, logistic regression plays a crucial role in identifying and categorizing potential threats, contributing significantly to bolstering the security of digital systems and networks.
Decision tree
In the realm of supervised learning algorithms, decision trees emerge as a highly versatile and powerful tool for both classification and regression tasks. They operate by constructing a tree-like structure, wherein internal nodes serve as decision points, branches represent the outcomes of attribute tests, and terminal nodes store class labels. The construction of a decision tree is an iterative process, continually dividing the training data into subsets based on attribute values until certain stopping conditions, such as reaching the maximum tree depth or the minimum sample size required for further division, are met. To guide this division process, the Decision Tree algorithm relies on metrics like entropy or Gini impurity, which gauge the level of impurity or unpredictability within the data subsets 31 . These metrics inform the algorithm’s choice of the most suitable attribute for data splitting during training, aiming to maximize information gain or minimize impurity. In essence, the central nodes of a decision tree represent the features, the branches encapsulate the decision rules, and the leaf nodes encapsulate the algorithm’s outcomes. This design accommodates both classification and regression challenges, making decision trees a flexible tool in supervised machine learning. One notable advantage of decision trees is their effectiveness in handling a wide range of problems. Moreover, their ability to be leveraged in ensembles, such as the Random Forest algorithm, enables the simultaneous training on multiple subsets of data, elevating their efficacy and robustness in real-world applications.
Random forest
A Random Forest is a powerful machine learning tool that handles both regression and classification tasks effectively. It works by combining the predictions of multiple decision trees to solve complex problems. Here's how it works: The Random Forest algorithm builds a “forest” of decision trees using a technique called bagging. Bagging improves the precision and reliability of machine learning ensembles 32 . The algorithm then makes predictions by averaging the results from these trees, determining the final outcome. What makes the Random Forest special is its scalability. Unlike single decision trees, it can adapt to complex data and improves its accuracy as you add more trees to the “forest.” The Random Forest also helps prevent overfitting, making it a valuable tool for real-world applications with noisy and complex datasets. Moreover, it reduces the need for extensive fine-tuning, making it an appealing choice for practitioners seeking effective and dependable machine learning models.
Naïve Bayes
Naïve Bayes theorem forms the fundamental principle underlying the Naive Bayes algorithm. In this method, a key assumption is that there's no interdependence among the feature pairs, resulting in two pivotal presumptions: feature independence and attribute equality. Naive Bayes classifiers are versatile, existing in three primary variants: Gaussian Naive Bayes, Bernoulli Naive Bayes, and Multinomial Naive Bayes 33 . The choice of variant depends on the nature of the data being analyzed. For binary data, Bernoulli Naïve Bayes is employed, while count data finds its match in Multinomial Naïve Bayes, and continuous data is aptly handled by Gaussian Naïve Bayes. Equation ( 2 ) serves as a proof of Bayes theorem, underpinning the mathematical foundations of this approach.
Principal component analysis
Principal Component Analysis (PCA) serves as a powerful technique designed to mitigate the impact of correlations among variables through an orthogonal transformation. PCA finds widespread use in both exploratory data analysis and machine learning for predictive modelling. In addition, PCA stands out as an unsupervised learning algorithm that offers a valuable approach for delving into the intricate relationships between variables. This method, also referred to as generic factor analysis, enables the discovery of the optimal line of fit through regression analysis 34 . What sets PCA apart is its ability to reduce the dimensionality of a dataset without prior knowledge of the target variables while preserving the most critical patterns and interdependencies among the variables. By doing so, PCA simplifies complex data, making it more amenable for various tasks, such as regression and classification. The result is a more streamlined subset of variables that encapsulates the essential essence of the data.
Experimental results and discussion
Feature extraction in this process relies on pre-trained models. Some notable examples of these models include ResNet101, ResNet152, InceptionV3, and AlexNet. For classification purposes, machine learning techniques like Simple Logistic, Decision Tree, Random Forest, Naive Bayes, and Principal Component Analysis (PCA) come into play. Among these models, the ResNet152 feature extraction method stands out for its exceptional performance, achieving the highest testing accuracy at 99.08%. When it comes to machine learning, the Simple Logistic model outperforms all the pre-trained models in terms of accuracy. It's worth noting that there's a slight but noticeable gap between training accuracy and testing accuracy for all the pre-trained models, with training accuracy consistently higher. This discrepancy underscores the complexities of classifying cells associated with cervical cancer.
Leveraging a variety of machine learning approaches proves significant, as it not only provides flexibility but also improves accuracy in tackling this challenging classification task. The subsequent sections will delve into the findings obtained using the suggested method, offering a comprehensive comparison of the employed pre-trained models in Table 6 . While ResNet152 achieved the highest accuracy, it's essential to highlight that the Simple Logistic Classifier, with the highest accuracy among all the pre-trained models, will be the focal point of this discussion. Additionally, Fig. 7 provides a visual comparative analysis of all the pre-trained models, revealing the performance of the Simple Logistic Classifier as the benchmark for reference.
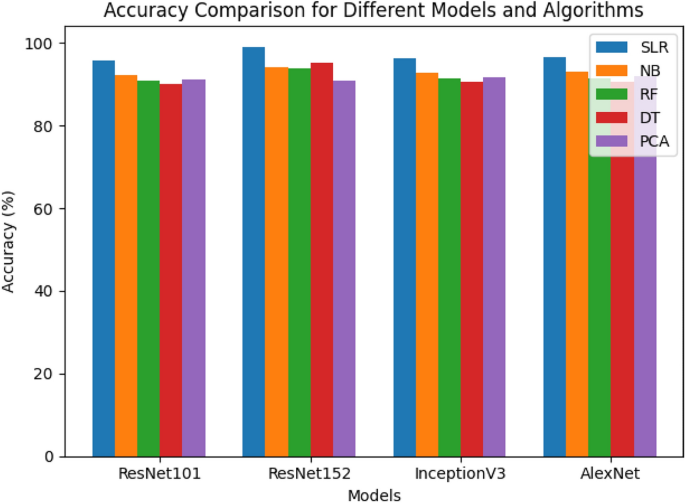
Pretrained models accuracy (%) comparison.
The ResNet-101 model plays a pivotal role in feature extraction. It's worth noting that a range of machine learning techniques, including Simple Logistic, Decision Tree, Random Forest, Naive Bayes, and Principal Component Analysis, were applied to both the test and training datasets. For a comprehensive view of the results, Table 7 present the outcomes for the training and testing sets, respectively. When compared to other classifiers, the Random Forest stood out, achieving the highest accuracy (Acc) (98.62%), precision (Pr) (98.64%), recall (98.64%), and the lowest mean absolute error (0.062%) for the training dataset. On the other hand, the test dataset yielded the best results with simple logistic regression, which delivered an accuracy of 95.81%, precision of 94.41%, recall of 94.32%, and a root mean square of 0.225.
The ResNet-152 model helps extract features. Various machine learning techniques, like Simple Logistic, Decision Tree, Random Forest, Naive Bayes, and Principal Component Analysis, were used on both the test and training data. Table 8 show the results for the training and testing sets. Among the classifiers, Random Forest performed the best, with the highest accuracy (98.98%), precision (99.64%), recall (99.42%), and the lowest mean absolute error (0.053%) for the training data. In contrast, for the test data, simple logistic regression delivered the best results with an accuracy of 98.08%, precision of 95.41%, recall of 94.21%, and a root mean square of 0.22.
The Inceptionv3 model handles feature extraction, and we used several machine learning techniques like Simple Logistic, Decision Tree, Random Forest, Naive Bayes, and Principal Component Analysis on both the training and test datasets. Table 9 show the results for both training and testing. Among these methods, the Random Forest performed the best for training, with the highest accuracy (97.74%), precision (97.75%), recall (97.73%), and the lowest mean absolute error (0.068%). On the other hand, for the test data, simple logistic regression delivered outstanding results with an accuracy of 98.08%, precision of 95.01%, recall of 93.42%, and a root mean square of 0.2349.
The task of feature extraction is managed by the AlexNet model, and we employed a range of machine learning techniques, such as Simple Logistic, Decision Tree, Random Forest, Naive Bayes, and Principal Component Analysis, on both the training and test datasets. Table 10 present the findings for both training and testing phases. Among these methodologies, the Random Forest excelled during training, achieving the highest levels of accuracy (99.12%), precision (98.83%), recall (98.81%), and the lowest mean absolute error (0.061%). Conversely, for the test data, simple logistic regression yielded remarkable results, attaining an accuracy of 96.31%, precision of 94.81%, recall of 94.72%, and a root mean square of 0.234. Figure 8 , illustrates the confusion matrix of all four pre-trained models.
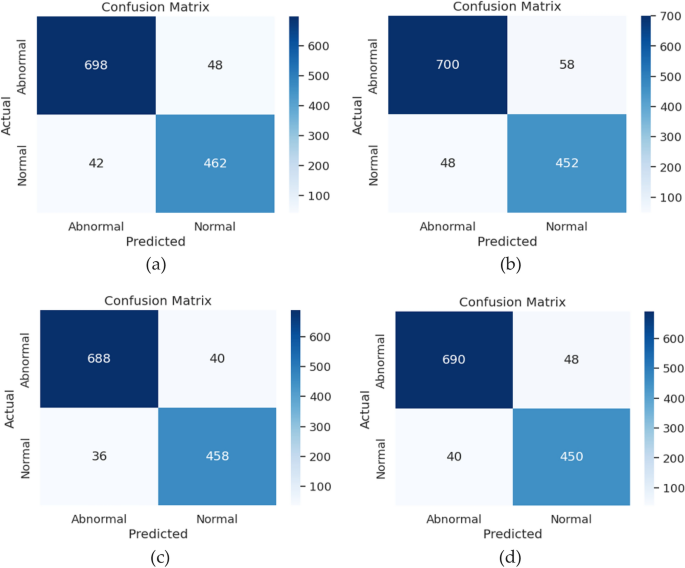
Confusion matrix for ( a ) ResNet101; ( b ) ResNet152; ( c ) InceptionV3; ( d ) AlexNet.
Table 11 illustrates a comprehensive comparison of classification accuracy between the proposed ResNet152 model with SLR and several state-of-the-art models. The table presents a detailed breakdown of the performance metrics, emphasizing the accuracy of these models in solving the specific task. The accuracy values are expressed as percentages, showcasing how effectively each model can correctly classify data points. The proposed ResNet152 with SLR stands out by achieving the highest classification accuracy of 98.08%. This remarkable result demonstrates the effectiveness of this model in comparison to other state-of-the-art models. Figure 9 complements the insights from Table 11 by offering a visual representation of the accuracy comparison. This bar chart clearly illustrates how the proposed ResNet152 with SLR outperforms other existing models in terms of accuracy. Each bar in the chart represents a different model, with its height corresponding to its accuracy percentage. The striking difference in the height of the bar for the proposed model highlights its superior performance, with an accuracy of 98.08%. This figure serves as a powerful visualization of the comparison, making it easy for readers to grasp the extent of the proposed model's excellence. Together, Table 11 and Fig. 9 provide a robust and easily interpretable analysis of the model comparison, emphasizing the outstanding performance of the proposed ResNet152 with SLR in the context of accuracy. These visual aids are essential in conveying the significance of the research findings to your readers and stakeholders.
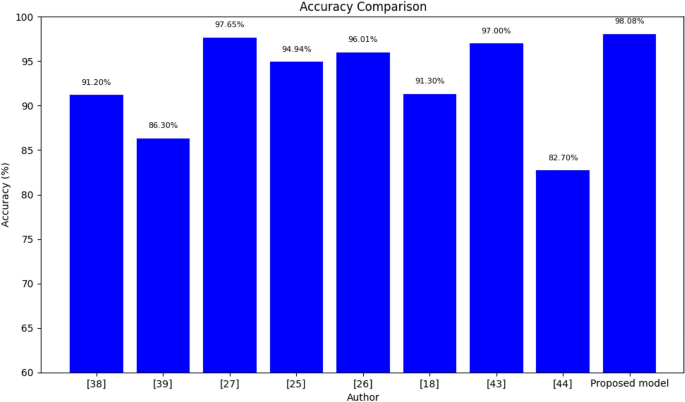
Graphical illustration of accuracy comparison of proposed and other models.
Cervical cancer classification is a challenging task within the medical field. Interpreting and diagnosing cervical cancer have historically been a complex process for pathologists. However, advancements in technology have led to a shift in how this task is approached. Specifically, the integration of Deep Learning (DL) and Machine Learning (ML) algorithms has emerged as a powerful tool, enhancing the precision of cervical cancer classification. Central to this progress is the use of pretrained models such as ResNet101, ResNet152, InceptionV3, and AlexNet. These models have been carefully fine-tuned through training on pap smear images, allowing them to effectively extract key features from the intricate world of Pap smear images. Our innovative approach, applied to the SIPaKMeD dataset, represents a pioneering method that combines the strengths of DL and ML in the field of cervical cancer classification. DL excels at extracting intricate features from images, providing a foundation for various ML algorithms to work with. This hybrid methodology holds promise for improving cervical cancer classification. The results obtained from our approach are indeed promising. For instance, ResNet101 achieved an accuracy of 95.81%. However, ResNet152 stands out as the leading model, achieving an impressive accuracy of 98.08%. Notably, these exceptional results were achieved using Simple Logistic classifiers, which outperformed other classification techniques. Furthermore, when Simple Logistic is combined with pretrained DL models, it reaffirms its effectiveness as the top-performing ML approach, highlighting the strength and efficiency of this combination in the context of cervical cancer classification. This not only confirms the potential of this model but also underscores the promising prospects of our hybrid DL-ML approach for advancing the field of cervical cancer diagnosis. In conclusion, these findings emphasize the transformative potential of our hybrid methodology. By skilfully combining DL and ML, we aim to contribute to the ongoing evolution of cervical cancer diagnosis, ultimately improving patient outcomes. Our results point toward a future where cervical cancer classification is more accurate, efficient, and accessible.
Data availability
The datasets used during the current study https://www.cs.uoi.gr/~marina/sipakmed.html .
Basak, H., Kundu, R., Chakraborty, S. & Das, N. Cervical cytology classification using PCA and GWO enhanced deep features selection. SN Comput. Sci. 2 , 369. https://doi.org/10.1007/s42979-021-00741-2 (2021).
Article Google Scholar
Bedell, S. L., Goldstein, L. S., Goldstein, A. R. & Goldstein, A. T. Cervical cancer screening: Past, present, and future. Sex Med. Rev. 8 (1), 28–37 (2020).
Article PubMed Google Scholar
Dong, N., Zhao, L., Wu, C. H. & Chang, J. F. Inception v3 based cervical cell classification combined with artificially extracted features. Appl. Soft Comput. 93 , 106311. https://doi.org/10.1016/j.asoc.2020.106311 (2020).
Akpudo, U. E. & Hur, J. W. J. D-dCNN: A novel hybrid deep learning-based tool for vibration-based diagnostics. Energies 14 (17), 5286. https://doi.org/10.3390/en14175286 (2021).
Zhang, T., Luo, Y. M. & Li, P. Cervical precancerous lesions classification using pre-trained densely connected convolutional networks with colposcopy images. Biomed. Signal Process. Control 55 , 101566 (2020).
Hua, W., Xiao, T. & Jiang, X. Lymph-vascular space invasion prediction in cervical cancer: Exploring radiomics and deep learning multilevel features of tumor and peritumor tissue on multiparametric MRI. Biomed. Signal Process. Control 58 , 101869 (2020).
Yusufaly, T. I., Kallis, K. & Simon, A. A knowledge-based organ dose prediction tool for brachytherapy treatment planning of patients with cervical cancer. Brachytherapy 19 (5), 624–634 (2020).
Kim, S. I. et al. Prediction of disease recurrence according to surgical approach of primary radical hysterectomy in patients with early-stage cervical cancer using machine learning methods. Gynecol. Oncol. 159 , 185–186 (2020).
Suriya, M., Chandran, V. & Sumithra, M. G. Enhanced deep convolutional neural network for malarial parasite classification. Int. J. Comput. Appl. 44 , 1113–1122 (2019).
Google Scholar
Meng, Q. Machine learning to predict local recurrence and distant metastasis of cervical cancer after definitive radiotherapy. Int. J. Radiat. Oncol. 108 (3), e767 (2020).
Fekri-Ershad, S. Pap smear classification using combination of global significant value, texture statistical features and time series features. Multimed. Tools Appl. 78 (22), 31121–31136 (2019).
Ali, T. M., Nawaz, A. & Rehman, A. U. A sequential machine learning-cum-attention mechanism for effective segmentation of brain tumor. Front. Oncol. 12 (1), 1–15 (2022).
CAS Google Scholar
Khamparia, A., Gupta, D., de Albuquerque, V. H. C., Sangaiah, A. K. & Jhaveri, R. H. Internet of health things-driven deep learning system for detection and classification of cervical cells using transfer learning. J. Supercomput. 76 (11), 8590–8608 (2020).
Chandran, V. et al. Diagnosis of cervical cancer based on ensemble deep learning network using colposcopy images. BioMed Res. Int. 5584004 , 1–15 (2021).
Alsubai, S., Alqahtani, A., Sha, M., Almadhor, A. & Abba, S. Privacy preserved cervical cancer detection using convolutional neural networks applied to pap smear images. Comput. Math. Methods Med. 9676206 , 1–8 (2023).
Habtemariam, L. W., Zewde, E. T. & Simegn, G. L. Cervix type and cervical cancer classification system using deep learning techniques. Med. Devices Evid. Res. 15 (1), 163–176 (2022).
Hortlund, M., Mühr, L. S. A., Lagheden, C. & Hjerpe, A. Audit of laboratory sensitivity of human papillomavirus and cytology testing in a cervical screening program. Int. J. Cancer 149 , 2083–2090 (2021).
Article CAS PubMed Google Scholar
Effah, K. et al. Concurrent HPV DNA testing and a visual inspection method for cervical precancer screening: A practical approach from Battor, Ghana. PLoS Glob. Public Health https://doi.org/10.1371/journal.pgph.0001830 (2023).
Article PubMed PubMed Central Google Scholar
Xue, P. et al. Deep learning in image-based breast and cervical cancer detection: A systematic review and meta-analysis. Digit. Med. 5 (19), 1–15 (2022).
Singh, S. K. & Goyal, A. Performance analysis of machine learning algorithms for cervical cancer detection. Res. Anthol. Med. Inform. Breast Cervic. Cancer https://doi.org/10.4018/978-1-6684-7136-4.ch019 (2023).
Kumawat, G. et al. Prognosis of cervical cancer disease by applying machine learning techniques. J. Circ. Syst. Comput. 32 (1), 1–14 (2023).
Kalbhor, M. M. & Shinde, S. V. Cervical cancer diagnosis using convolution neural network: Feature learning and transfer learning approaches. Soft Comput. 132 (1), 1–19 (2023).
Plissiti, M. E. & Dimitrakopoulos, P. Sipakmed: A new dataset for feature and image-based classification of normal and pathological cervical cells in pap smear images. In IEEE International Conference on Image Processing ( ICIP ), Athens (2015).
Plissiti, M. E. et al . Sipakmed: A new dataset for feature and image-based classification of normal and pathological cervical cells in pap smear images. In IEEE International Conference on Image Processing ( ICIP ) (2018).
Fekri-Ershad, S. & Alsaffar, M. F. Developing a tuned three-layer perceptron fed with trained deep convolutional neural networks for cervical cancer diagnosis. Diagnostics 13 (4), 1–18 (2023).
Kurita, Y. et al. Accurate deep learning model using semi-supervised learning and Noisy Student for cervical cancer screening in low magnification images. PLoS One 18 (5), e0285996 (2023).
Article CAS PubMed PubMed Central Google Scholar
Chen, J., Zhou, M., Zhang, D., Huang, H. & Zhang, F. Quantification of water inflow in rock tunnel faces via convolutional neural network approach. Autom. Constr. 123 (1), 1–14 (2021).
Attallah, O. CerCan·Net: Cervical cancer classification model via multi-layer feature ensembles of lightweight CNNs and transfer learning. Expert Syst. Appl. 229 (1), 1–19 (2023).
Pustokhin, D. A., Pustokhina, I. V., Dinh, P. N., Phan, S. V. & Nguyen, G. N. An effective deep residual network-based class attention layer with bidirectional LSTM for diagnosis and classification of COVID-19. J. Appl. Stat. https://doi.org/10.1080/02664763.2020.1849057 (2020).
Singh, T. & Vishwakarma, D. K. A deeply coupled ConvNet for human activity recognition using dynamic and RGB images. Neural Comput. Appl. 33 , 469–485 (2021).
Article CAS Google Scholar
Krizhevsky, A., Sutskever, I. & Hinton, G. E. ImageNet classification with deep convolutional neural networks. Commun. ACM 60 (6), 1–7 (2017).
Sperandei, S. Understanding logistic regression analysis. Biochem. Med. (Zagreb) 24 (1), 12–18 (2014).
AlMamun, M. H. & Keikhosrokiani, P. Chapter 23—Predicting onset (type-2) of diabetes from medical records using binary class classification. In Big Data Analytics for Healthcare 301–312 (Academic Press, 2022).
Chapter Google Scholar
Breiman, L. E. O. Random forests. Mach. Learn. 45 (1), 5–32 (2001).
Rish, I. An Empirical Study of the Naïve Bayes Classifier 1–7 (Watson Research Center, Corpus ID: 14891965, 2001).
Jolliffe, I. T. & Cadima, J. Principal component analysis: A review and recent developments. Trans. R. Soc. A 374 , 20150202 (2016).
ADS MathSciNet Google Scholar
Shareef, B., Vakanski, A., Freer, P. E. & Xian, M. ESTAN: Enhanced small tumor-aware network for breast ultrasound image segmentation. Healthcare (Basel) 10 (11), 1–14 (2022).
Shareef, B., Xian, M., Vakanski, A. & Wang, H. Breast ultrasound tumor classification using a hybrid multitask CNN-transformer network. Med. Image Comput. Comput. Assist. Interv. 14223 , 344–353 (2023).
PubMed PubMed Central Google Scholar
Download references
Author information
Authors and affiliations.
School of Computer Science and Engineering, Galgotias University, Greater Noida, 203201, India
Sandeep Kumar Mathivanan
Department of Electronics and Communication Engineering, PSNA College of Engineering and Technology, Dindigul, India
Divya Francis
Department of Computer Science and Engineering, Vel Tech Rangarajan Dr. Sagunthala R&D Institute of Science and Technology, Chennai, India
Saravanan Srinivasan
School of Computing Science and Engineering, VIT Bhopal University, Bhopal–Indore Highway Kothrikalan, Sehore, Madhya Pradesh, India
Vaibhav Khatavkar
Department of Computer Applications, School of Computer Science Engineering and Information Systems, Vellore Institute of Technology, Vellore, Tamil Nadu, 632014, India
Karthikeyan P
Kebri Dehar University, Kebri Dehar, Somali, 250, Ethiopia
Mohd Asif Shah
Division of Research and Development, Lovely Professional University, Phagwara, Punjab, 144001, India
You can also search for this author in PubMed Google Scholar
Contributions
Conceptualization, S.K.M. and D.F.; methodology, S.K.M.; validation, D.F. and V.K.; resources, M.A.S.; data curation, S.S. writing—original draft preparation, S.K.M. and D.F.; writing—review and editing, S.K.M., and M.A.S.; visualization, S.S. and P.K.; supervision S.K.M. and S.S.; project administration. S.K.M., S.S., P.K. and M.A.S.
Corresponding author
Correspondence to Mohd Asif Shah .
Ethics declarations
Competing interests.
The authors declare no competing interests.
Additional information
Publisher's note.
Springer Nature remains neutral with regard to jurisdictional claims in published maps and institutional affiliations.
Rights and permissions
Open Access This article is licensed under a Creative Commons Attribution 4.0 International License, which permits use, sharing, adaptation, distribution and reproduction in any medium or format, as long as you give appropriate credit to the original author(s) and the source, provide a link to the Creative Commons licence, and indicate if changes were made. The images or other third party material in this article are included in the article's Creative Commons licence, unless indicated otherwise in a credit line to the material. If material is not included in the article's Creative Commons licence and your intended use is not permitted by statutory regulation or exceeds the permitted use, you will need to obtain permission directly from the copyright holder. To view a copy of this licence, visit http://creativecommons.org/licenses/by/4.0/ .
Reprints and permissions
About this article
Cite this article.
Mathivanan, S., Francis, D., Srinivasan, S. et al. Enhancing cervical cancer detection and robust classification through a fusion of deep learning models. Sci Rep 14 , 10812 (2024). https://doi.org/10.1038/s41598-024-61063-w
Download citation
Received : 15 October 2023
Accepted : 30 April 2024
Published : 11 May 2024
DOI : https://doi.org/10.1038/s41598-024-61063-w
Share this article
Anyone you share the following link with will be able to read this content:
Sorry, a shareable link is not currently available for this article.
Provided by the Springer Nature SharedIt content-sharing initiative
- Cervical cancer
- Classification
- Deep neural network
- Machine learning
By submitting a comment you agree to abide by our Terms and Community Guidelines . If you find something abusive or that does not comply with our terms or guidelines please flag it as inappropriate.
Quick links
- Explore articles by subject
- Guide to authors
- Editorial policies
Sign up for the Nature Briefing: Cancer newsletter — what matters in cancer research, free to your inbox weekly.

- Introduction
- Conclusions
- Article Information
eTable 1. List of Codes Used for Identification of Medically Assisted Reproduction–Related Procedures
eTable 2. Grouping of ICD-10 Diagnostic Codes Used to Approximate the International Childhood Cancer Classification
eTable 3. Number of Cancers Among Children Born and Diagnosed in France Between 2010 and 2015 in the French National Registry of Childhood Cancers (RNCE) and in the Present Study
eTable 4. Birth Characteristics of Children Born in France in 2010 to 2021 by Cancer Status
eTable 5. Risk of Cancer in Children Born in France in 2010 to 2021 After Fresh Embryo Transfer Compared to Naturally Conceived Children
eTable 6. Risk of Cancer in Children Born in France in 2010 to 2021 After Frozen Embryo Transfer Compared to Naturally Conceived Children
eTable 7. Risk of Cancer in Children Born in France in 2010 to 2021 After Artificial Insemination Compared to Naturally Conceived Children
eTable 8. Number of Cases, Incidence Rate, and Risk of Cancer in Children Born After Natural Conception, Fresh Embryo Transfer, Frozen Embryo Transfer, or Artificial Insemination in Singletons Born in France in 2010 to 2021
eTable 9. Risk of Cancer in Children Born in France in 2010 to 2021 After Fresh Embryo Transfer, Frozen Embryo Transfer, or Artificial Insemination Compared to Naturally Conceived Children
eTable 10. Risk of Cancer in Children Born in France in 2010 to 2021 After Fresh Embryo Transfer, Frozen Embryo Transfer, or Artificial Insemination Compared to Naturally Conceived Children
eTable 11. Number of Children Born After Medically Assisted Reproduction in France Between 2010 and 2020 According to the French National Agency of Biomedicine (ABM) and in the Present Study
Data Sharing Statement
- Cancer Risk Among Children Born After Fertility Treatment JAMA Network Open Invited Commentary May 2, 2024 Marie Hargreave, PhD
See More About
Sign up for emails based on your interests, select your interests.
Customize your JAMA Network experience by selecting one or more topics from the list below.
- Academic Medicine
- Acid Base, Electrolytes, Fluids
- Allergy and Clinical Immunology
- American Indian or Alaska Natives
- Anesthesiology
- Anticoagulation
- Art and Images in Psychiatry
- Artificial Intelligence
- Assisted Reproduction
- Bleeding and Transfusion
- Caring for the Critically Ill Patient
- Challenges in Clinical Electrocardiography
- Climate and Health
- Climate Change
- Clinical Challenge
- Clinical Decision Support
- Clinical Implications of Basic Neuroscience
- Clinical Pharmacy and Pharmacology
- Complementary and Alternative Medicine
- Consensus Statements
- Coronavirus (COVID-19)
- Critical Care Medicine
- Cultural Competency
- Dental Medicine
- Dermatology
- Diabetes and Endocrinology
- Diagnostic Test Interpretation
- Drug Development
- Electronic Health Records
- Emergency Medicine
- End of Life, Hospice, Palliative Care
- Environmental Health
- Equity, Diversity, and Inclusion
- Facial Plastic Surgery
- Gastroenterology and Hepatology
- Genetics and Genomics
- Genomics and Precision Health
- Global Health
- Guide to Statistics and Methods
- Hair Disorders
- Health Care Delivery Models
- Health Care Economics, Insurance, Payment
- Health Care Quality
- Health Care Reform
- Health Care Safety
- Health Care Workforce
- Health Disparities
- Health Inequities
- Health Policy
- Health Systems Science
- History of Medicine
- Hypertension
- Images in Neurology
- Implementation Science
- Infectious Diseases
- Innovations in Health Care Delivery
- JAMA Infographic
- Law and Medicine
- Leading Change
- Less is More
- LGBTQIA Medicine
- Lifestyle Behaviors
- Medical Coding
- Medical Devices and Equipment
- Medical Education
- Medical Education and Training
- Medical Journals and Publishing
- Mobile Health and Telemedicine
- Narrative Medicine
- Neuroscience and Psychiatry
- Notable Notes
- Nutrition, Obesity, Exercise
- Obstetrics and Gynecology
- Occupational Health
- Ophthalmology
- Orthopedics
- Otolaryngology
- Pain Medicine
- Palliative Care
- Pathology and Laboratory Medicine
- Patient Care
- Patient Information
- Performance Improvement
- Performance Measures
- Perioperative Care and Consultation
- Pharmacoeconomics
- Pharmacoepidemiology
- Pharmacogenetics
- Pharmacy and Clinical Pharmacology
- Physical Medicine and Rehabilitation
- Physical Therapy
- Physician Leadership
- Population Health
- Primary Care
- Professional Well-being
- Professionalism
- Psychiatry and Behavioral Health
- Public Health
- Pulmonary Medicine
- Regulatory Agencies
- Reproductive Health
- Research, Methods, Statistics
- Resuscitation
- Rheumatology
- Risk Management
- Scientific Discovery and the Future of Medicine
- Shared Decision Making and Communication
- Sleep Medicine
- Sports Medicine
- Stem Cell Transplantation
- Substance Use and Addiction Medicine
- Surgical Innovation
- Surgical Pearls
- Teachable Moment
- Technology and Finance
- The Art of JAMA
- The Arts and Medicine
- The Rational Clinical Examination
- Tobacco and e-Cigarettes
- Translational Medicine
- Trauma and Injury
- Treatment Adherence
- Ultrasonography
- Users' Guide to the Medical Literature
- Vaccination
- Venous Thromboembolism
- Veterans Health
- Women's Health
- Workflow and Process
- Wound Care, Infection, Healing
Get the latest research based on your areas of interest.
Others also liked.
- Download PDF
- X Facebook More LinkedIn
Rios P , Herlemont P , Fauque P, et al. Medically Assisted Reproduction and Risk of Cancer Among Offspring. JAMA Netw Open. 2024;7(5):e249429. doi:10.1001/jamanetworkopen.2024.9429
Manage citations:
© 2024
- Permissions
Medically Assisted Reproduction and Risk of Cancer Among Offspring
- 1 EPI-PHARE Scientific Interest Group in Epidemiology of Health Products, French National Agency for Medicines and Health Products Safety, French National Health Insurance, Saint-Denis, France
- 2 Epidemiology of Childhood and Adolescent Cancers, Centre for Research in Epidemiology and Statistics, French National Institute for Health and Medical Research (INSERM) Joint Research Unit (UMR) 1153, Université Paris-Cité, Paris, France
- 3 INSERM UMR 1231, Université Bourgogne Franche-Comté, Dijon, France
- 4 French National Registry of Childhood Cancers, Assistance Publique–Hôpitaux de Paris, Centre Hospitalier Régional Universitaire (CHU) Paul Brousse, Villejuif, France
- 5 French National Registry of Childhood Solid Tumours, CHU de Nancy, Nancy, France
- 6 Université Paris-Cité, Paris, France
- Invited Commentary Cancer Risk Among Children Born After Fertility Treatment Marie Hargreave, PhD JAMA Network Open
Question Does cancer risk differ among children born after fresh embryo transfer (ET), frozen embryo transfer (FET), or artificial insemination (AI) and children conceived naturally?
Findings In this cohort study of 8 526 306 children, the overall risk of cancer did not differ among children born after fresh ET, FET, or AI and children conceived naturally. However, an increased risk of leukemia was observed among children born after fresh ET (especially those with the longest follow-up) and among children born after FET (particularly acute lymphoblastic leukemia).
Meaning These findings suggest that the risk of leukemia among children conceived by FET or fresh ET should be further monitored.
Importance Cancer is a leading cause of death among children worldwide. Treatments used for medically assisted reproduction (MAR) are suspected risk factors because of their potential for epigenetic disturbance and associated congenital malformations.
Objective To assess the risk of cancer, overall and by cancer type, among children born after MAR compared with children conceived naturally.
Design, Setting, and Participants For this cohort study, the French National Mother-Child Register (EPI-MERES) was searched for all live births that occurred in France between January 1, 2010, and December 31, 2021 (and followed up until June 30, 2022). The EPI-MERES was built from comprehensive data of the French National Health Data System. Data analysis was performed from December 1, 2021, to June 30, 2023.
Exposure Use of assisted reproduction technologies (ART), such as fresh embryo transfer (ET) or frozen ET (FET), and artificial insemination (AI).
Main Outcomes and Measures The risk of cancer was compared, overall and by cancer type, among children born after fresh ET, FET, or AI and children conceived naturally, using Cox proportional hazards regression models adjusted for maternal and child characteristics at birth.
Results This study included 8 526 306 children with a mean (SD) age of 6.4 (3.4) years; 51.2% were boys, 96.4% were singletons, 12.1% were small for gestational age at birth, and 3.1% had a congenital malformation. There were 260 236 children (3.1%) born after MAR, including 133 965 (1.6%) after fresh ET, 66 165 (0.8%) after FET, and 60 106 (0.7%) after AI. A total of 9256 case patients with cancer were identified over a median follow-up of 6.7 (IQR, 3.7-9.6) years; 165, 57, and 70 were born after fresh ET, FET, and AI, respectively. The overall risk of cancer did not differ between children conceived naturally and those born after fresh ET (hazard ratio [HR], 1.12 [95% CI, 0.96 to 1.31]), FET (HR, 1.02 [95% CI, 0.78 to 1.32]), or AI (HR, 1.09 [95% CI, 0.86 to 1.38]). However, the risk of acute lymphoblastic leukemia was higher among children born after FET (20 case patients; HR 1.61 [95% CI, 1.04 to 2.50]; risk difference [RD], 23.2 [95% CI, 1.5 to 57.0] per million person-years) compared with children conceived naturally. Moreover, among children born between 2010 and 2015, the risk of leukemia was higher among children born after fresh ET (45 case patients; HR, 1.42 [95% CI, 1.06 to 1.92]; adjusted RD, 19.7 [95% CI, 2.8 to 43.2] per million person-years).
Conclusions and Relevance The findings of this cohort study suggest that children born after FET or fresh ET had an increased risk of leukemia compared with children conceived naturally. This risk, although resulting in a limited number of cases, needs to be monitored in view of the continuous increase in the use of ART.
Childhood cancer is a leading cause of death among children worldwide. Overall, 1800 cases occur every year in France among children aged younger than 15 years, with half occurring before age 6 years. 1 The early onset after birth and the embryologic characteristics of some cancers 2 suggest that the causes may take place around conception. Treatments involved in medically assisted reproduction (MAR), 3 including assisted reproduction technologies (ART; eg, fresh embryo transfer [ET] or frozen ET [FET]) and artificial insemination (AI), have been suggested as potential risk factors for childhood cancer. 4 - 6
Children born after MAR represent a growing population worldwide. 7 Compared with children conceived naturally, children born after MAR have an increased risk of adverse perinatal outcomes, including preterm birth, low birth weight, and fetal growth anomalies. 8 , 9 However, little is known about the medium-term and long-term effects of fertility treatments on child health. Previous studies on the risk of childhood cancer among children born after ART, most of which were based on relatively small cohorts of exposed children 10 - 18 or interview-based case-control study designs, 19 - 24 provided contrasting results. With regard to findings from large cohorts including 100 000 or more exposed children, 25 - 29 no increase in overall cancer risk has been reported, except 1 study 29 that reported a marginal association with ART. Nevertheless, positive associations have been reported when investigating specific cancer types. 25 - 29 In addition, previous studies that assessed the risk of childhood cancer by fresh ET or FET 12 , 15 , 17 , 25 - 28 were based on limited numbers of case patients exposed to each ART modality.
We aimed to assess the risk of cancer, overall and by cancer type, among children born after ART use (fresh ET or FET) or after AI compared with children conceived naturally, considering all children born in France between 2010 and 2021.
This cohort study was authorized by decree 2016-1871 from December 26, 2016, relating to the processing of personal data from the National Health Data System and French law Art. 1461-13 and 14. The EPI-PHARE Scientific Interest Group in Epidemiology of Health Products, which has permanent regulatory access to the data from the French National Health Data System (SNDS; in application of the provisions of Art. R. 1461-12 et seq. 9 of the French Public Health Code and French Data Protection Authority decision CNIL-2016-10 316), was exempted from participant consent and institutional review board approval. The study was registered in the EPI-PHARE SNDS-based registry under reference T-2020-10-272. The study followed the Strengthening the Reporting of Observational Studies in Epidemiology ( STROBE ) reporting guideline.
This study was conducted using comprehensive data from the French National Mother-Child Register (EPI-MERES) for all children born alive in France between January 1, 2010, and December 31, 2021. The EPI-MERES database was built from the SNDS, 30 which provides comprehensive hospitalization and health insurance reimbursement data for more than 99% of the French population; it is an unselected register of all pregnancies from 2010 onward in France, identified by end-of-pregnancy diagnoses and procedure codes and linked to their offspring using a deterministic algorithm. Linkage of mother and infant records is available for 95% of children. As of today, the EPI-MERES includes data for more than 8.5 million liveborn children, accounting for 98% of all live births recorded in France since 2010. The EPI-MERES contains a wide set of information on mothers (medical history and sociodemographic characteristics), pregnancies (start and end dates, mode of delivery, and pregnancy outcome), and offspring (gestational age and birth weight, outpatient and inpatient health care use, and hospital discharge diagnoses and medical procedures coded according to the Classification Commune des Actes Médicaux [ CCAM ]). Patients with long-term diseases (LTDs), such as cancer, benefit from full coverage of their health expenditure, and the diagnosis is recorded in the Datamart Consommation Inter Régime (DCIR) using International Statistical Classification of Diseases, Tenth Revision ( ICD-10 ) codes. The DCIR is a French health care expenditure database that contains all of the individual data of health insurance beneficiaries. Data from the EPI-MERES have been used previously to conduct drug use and safety pharmacoepidemiologic studies. 31 - 34
Artificial insemination and ART are fully covered by French health insurance after medical validation and until a maternal age of 43 years. Procedures related to MAR and their dates were identified using CCAM codes for oocyte retrieval, ET, and AI (eTable 1 in Supplement 1 ). Children were considered as born after MAR when the conception date fell within 21 days of the MAR procedure recorded in the SNDS, and as naturally conceived otherwise. The recorded date of ET was retained as the ART procedure date. Children were considered as born after fresh ET when there were fewer than 21 days between the date of oocyte retrieval and the ET, and as born after FET when the delay was 21 days or longer.
Childhood cancers were identified using hospital discharge and LTD diagnoses, using algorithms constructed in collaboration with experts in cancer coding from the French National Registry of Childhood Cancers (RNCE). Children were considered case patients with cancer if they met at least 1 of the following criteria: (1) had at least 2 hospitalizations with a discharge diagnosis of cancer; (2) had at least 1 hospitalization with a discharge diagnosis of cancer in addition to 1 hospitalization for oncologic treatment (chemotherapy, radiotherapy, oncologic surgery, or bone marrow transplantation) or an LTD diagnosis of childhood cancer; or (3) had 1 hospitalization with a discharge diagnosis of cancer, followed by death within 6 months.
Cancer diagnoses were categorized into 12 groups as close as possible to those of the International Classification of Childhood Cancer, Third Edition ( ICCC-3 ; eTable 2 in Supplement 1 ), 35 using ICD-10 codes, in the absence of the morphology and topography codes of the International Classification of Diseases for Oncology, Third Edition ( ICD-O-3 ) required for ICCC-3 . For each child, the most frequently coded cancer diagnostic group among all their hospital stays was retained as the cancer diagnosis. Because of a lack of specificity, for malignant bone tumors and gonadic neoplasms, only case patients with identification of an oncologic treatment were retained. Because information available in the SNDS does not allow for the proper individual identification of same-sex siblings born of multiple pregnancies, only 1 case patient was retained in the case of identical cancer diagnosis in same-sex twins. Anonymized data provided by the RNCE for children born and diagnosed in the 2010 to 2015 period were used as the reference set for the distribution in large categories of cancer diagnoses (eTable 3 in Supplement 1 ).
Characteristics of children included year of birth, sex, singleton or multiple birth, birth weight (<1000, 1000-2499, 2500-3999, or ≥4000 g), gestational length (≤28, 29-32, 33-36, 37-41, or ≥42 weeks), and an indicator of fetal growth (small, adequate, or large for gestational age). Major congenital malformations were identified based on hospital discharge diagnoses within up to 12 months after birth, based on ICD-10 codes selected by the European Surveillance of Congenital Anomalies network. 36 Characteristics of mothers included maternal age at the index child birth (≤24, 25-29, 30-34, 35-39, or ≥40 years) and the French census-based deprivation index 37 of the municipality of residence at birth, used as a proxy for the household socioeconomic category. The French census-based deprivation index is categorized in quintiles, with the fifth quintile representing the most deprived municipalities.
Children contributed to person-years at risk from their date of birth until the date of first hospitalization with a discharge diagnosis of cancer, the date of death, or the end of the study on June 30, 2022, whichever occurred first. We compared the risk of cancer among children born after fresh ET, FET, or AI with that of children conceived naturally, taking all cancers together and by main cancer groups. The risk of leukemia was examined overall and by leukemia subtype (acute lymphoblastic leukemia [ALL] and acute myeloid leukemia [AML]).
Adjusted hazard ratios (HRs) and 95% CIs were estimated using Cox proportional hazards regression models including a priori selected covariates reported to be associated with childhood cancers and MAR in the literature (ie, sex, year of birth, multiple birth, maternal age at childbirth, and deprivation index). Additional models also adjusted for potential mediating factors including gestational length, birth weight, macrosomia, and congenital malformations. The percentages of missing data were small. To assess cancer risk among children followed over a longer period, complementary analyses restricted to children born between 2010 and 2015 were performed for most frequent childhood cancers. Partial likelihood tests were performed post hoc to determine whether the estimated HR differed across the various MAR modalities. Sensitivity analyses restricted to singletons were performed. Additional sensitivity analyses were performed using the first coded (instead of the most frequently coded) diagnosis group as the cancer diagnosis and restricting the case definition to children with chemotherapy exposure. The proportional hazards assumption was tested with Schoenfeld residuals, and there were no clear violations. Hazard ratios were not estimated for categories with fewer than 5 case patients. All P values were 2-sided and a significance level of .05 was applied. Data analysis was performed from December 1, 2021, to June 30, 2023, using SAS, version 9.4 (SAS Institute Inc).
The study cohort included 8 526 306 children, with a mean (SD) age of 6.4 (3.4) years. Boys comprised 51.2% of the cohort, and girls comprised 48.8%; 96.4% of children were singletons, 12.1% were small for gestational age at birth, and 3.1% had a congenital malformation. There were 260 236 children (3.1%) born after MAR, including 133 965 (1.6%) after fresh ET, 66 165 (0.8%) after FET, and 60 106 (0.7%) after AI. Although the number of births after fresh ET decreased from 2015 onward, a continuous increase in the number of children born after FET was observed over the study period and FET started to prevail over fresh ET in 2020. The annual number of children born after AI remained stable over the study period ( Figure ).
Compared with children conceived naturally, children born after MAR were more often part of a multiple birth, had lower birth weight and gestational age, were more often born small for gestational age, and were more often diagnosed with a congenital malformation. Children born after FET were more frequently born large for gestational age. Mothers whose children were born after MAR were older and their residence at birth was more often in the least deprived municipalities ( Table 1 ).
During a median follow-up of 6.7 (IQR, 3.7-9.6) years for children conceived naturally and 6.8 (IQR, 3.4-9.1) years, 4.4 (IQR, 2.4-7.3) years, and 6.5 (IQR, 3.6-9.4) years for children born after fresh ET, FET, and AI, respectively, 9256 case patients with cancer were identified. A total of 8964 cases were observed among children conceived naturally (incidence rate [IR], 164 per million person-years), 165 among children born after fresh ET (IR, 183 per million person-years), 57 among children born after FET (IR, 172 per million person-years), and 70 among children born after AI (IR, 179 per million person-years). Case patients with cancer more often were born preterm, were large for gestational age, and were diagnosed with congenital malformation compared with children without cancer (eTable 4 in Supplement 1 ). The overall risk of cancer did not differ between children conceived naturally and those born after fresh ET (HR, 1.12 [95% CI, 0.96 to 1.31]), FET (HR, 1.02 [95% CI, 0.78 to 1.32]), or AI (HR, 1.09 [95% CI, 0.86 to 1.38]) ( Table 2 ).
Leukemia was the most commonly diagnosed type of cancer, accounting for 29.4% of total cases. Among these case patients, 2635 were children conceived naturally (IR, 48 per million person-years), 52 were born after fresh ET (IR, 58 per million person-years), 23 were born after FET (IR, 69 per million person-years), and 19 were born after AI (IR, 49 per million person-years) ( Table 2 ). Acute lymphoblastic leukemia accounted for 79.1% of leukemia cases. Among these case patients, 2 083 were children conceived naturally (IR, 38 per million person-years), 39 were born after fresh ET (IR, 43 per million person-years), 20 were born after FET (IR, 60 per million person-years), and 16 were born after AI (IR, 41 per million person-years). Overall, the risk of leukemia for children born after MAR did not differ significantly from that of children conceived naturally (fresh ET: HR, 1.19 [95% CI, 0.90 to 1.56]; FET: HR, 1.42 [95% CI, 0.94 to 2.14; and AI: HR, 1.01 [95% CI, 0.64 to 1.58]). The risk of ALL was significantly increased after FET (HR, 1.61 [95% CI, 1.04 to 2.50]; adjusted risk difference [RD], 23.2 [95% CI, 1.5 to 57.0] per million person-years), but not after fresh ET (HR, 1.14 [95% CI, 0.83 to 1.57]) ( Table 2 ). The partial likelihood test was not statistically significant ( P = .07).
Besides leukemia, the most frequent groups of cancers were tumors of the central nervous system (CNS; n = 1875), embryonal tumors (n = 2481), and lymphomas (n = 823). Compared with children conceived naturally, the risk of each of these cancer groups did not differ among children born after fresh ET or among those born after FET. The risk of malignant CNS tumors tended to be higher among children born after AI, although estimates did not reach statistical significance (HR, 1.51 [95% CI, 0.92 to 2.38]; adjusted RD, 16.8 [95% CI, –2.6 to 45.5] per million person-years) ( Table 2 ).
In the analyses restricted to children born between 2010 and 2015, the median follow-up duration was longer and more homogeneous for the different modes of conception. These median durations were as follows: 9.4 (IQR, 7.8-10.9) years for natural conception, 9.4 (IQR, 7.9-10.9) years for fresh ET, 8.8 (IQR, 7.5-10.4) years for FET, and 9.3 (IQR, 7.9-10.8) years for AI. The risk of leukemia was significantly increased among children born after fresh ET (HR, 1.42 [95% CI, 1.06 to 1.92]; adjusted RD, 19.7 [95% CI, 2.8 to 43.2] per million person-years) but not after FET (HR, 1.27 [95% CI, 0.70 to 2.29]). The partial likelihood test was not statistically significant ( P = .06). The risk of ALL was increased, without reaching statistical significance, among children born after fresh ET (HR, 1.33 [95% CI, 0.93 to 1.89]; adjusted RD, 12.5 [95% CI, – 2.7 to 33.8] per million person-years) and FET (HR, 1.47 [95% CI, 0.79 to 2.74]; adjusted RD, 17.9 [95% CI, −8.0 to 66.1] per million person-years). No other association was observed ( Table 3 ).
Additional adjustments for birth characteristics and congenital malformations marginally modified the estimates (eTables 5-7 in Supplement 1 ). Results remained stable in sensitivity analyses restricted to singletons or based on alternative case definitions (eTables 8-10 in Supplement 1 ).
In this nationwide birth cohort study including more than 8.5 million children, the risk of any cancer was not increased among children born after MAR. However, the findings suggest that children born after fresh ET or FET had a higher risk of leukemia.
The absence of an overall association between cancer and MAR in this study is consistent with the findings of most large observational studies. 25 - 28 However, the grouping of all cancer types, although meaningful for detecting common risk factors, could miss subtype specificities. In line with our findings, 4 previous studies 13 , 17 , 27 , 28 reported an increased risk of leukemia among children born after ART. With regard to ART modalities such as fresh ET or FET, the literature is still sparse and results are conflicting. The data used in this study were obtained from one of the largest cohorts of children born after FET to date; thus, our results support the hypothesis of an increased risk of leukemia, mainly observable for ALL, with a limited number for AML. In our complementary analysis restricted to births between 2010 and 2015, the risk of leukemia was increased for children born after FET (although statistical significance was not reached because of reduced numbers of exposed case patients) and also for those born after fresh ET, probably as a result of more homogeneous follow-up between children born after fresh ET or FET and better coverage of the age range corresponding to the incidence peak of leukemia (2-6 years). Sargisian et al 28 conducted a study (median follow-up of 9.9 years) in Nordic countries, and they found an increased risk of leukemia among children born after FET vs children conceived naturally (HR, 2.22 [95% CI, 1.47 to 3.35]) but not among those born after fresh ET. In another study, Weng et al 17 found that the risk of leukemia was significantly increased among children born after fresh ET, but not among children born after FET. However, estimates were based on fewer than 5 exposed case patients by ART modality. A meta-analysis conducted by Zhang et al 38 reported no increased cancer risk among children born after ART based on 15 cohorts, but the authors found an increased risk of childhood cancer among children born after FET (pooled HR, 1.37 [95% CI, 1.04 to 1.81]) compared with children conceived naturally. However, the finding was not statistically significant when children born after fresh ET were considered as the reference group (pooled HR, 1.28 [95% CI, 0.96 to 1.69]), indicating that the increased incidence of cancer among children born after FET may be based on in vitro fertilization (IVF), intracytoplasmic sperm injection (ICSI), or any other ART. Differences between studies might be explained by heterogeneity across them in terms of sample size, follow-up duration, and study period, because substantial changes in ART practices occurred over time within the same laboratory and among different laboratories (eg, culture conditions: culture media used and oxygen tension, or embryo freezing processes).
Our findings do not provide evidence of an increased risk of other types of childhood cancer among children born after MAR. Based on 19 exposed case patients, the nonsignificantly increased risk of CNS tumors after AI reported here warrants further investigation. Furthermore, the number of hepatic tumors among exposed children was very small (<5 after fresh ET and 0 after FET), and the overall risk of embryonal tumors was not increased among children born after MAR.
The etiologic mechanisms behind a possible increased risk of cancer among children born after MAR are not known, but explanatory hypotheses consider epigenetic disturbance as a potential pathway. Previous studies have found that procedures involved in MAR, such as IVF, ICSI, and ovarian stimulation, can induce epigenetic changes in human embryos, cord blood, and placentae. 39 , 40 This finding is also supported by studies that suggest an association between MAR-related procedures and imprinting disorders, 41 such as Beckwith-Weidemann syndrome, 42 a pediatric overgrowth disorder involving a predisposition to tumor development.
Our study has major strengths. It is based on one of the largest cohorts published to date and on comprehensive data covering the entire French population, and we used reliable and systematically collected data, with no potential for recall bias and selection bias. We performed analyses for the most frequent childhood cancer groups and by MAR modality, and we had reliable individual information on major confounders and potential mediating factors.
Our findings must be interpreted in light of some limitations. We used reimbursement and hospitalization data to identify exposure to MAR procedures and childhood cancer occurrence. With regard to exposure assessment, MAR-related procedures are expensive treatments that benefit from full insurance coverage in France; therefore, they typically are not subject to nondeclaration. Compared with reported numbers from the French Agency of Biomedicine over the same period, our study was able to identify more than 90% of liveborn children conceived by MAR, and the distribution by fresh ET, FET, or AI was comparable (eTable 11 in Supplement 1 ). Still, information on the type of ART (ie, ICSI) or cross-border fertility care was not available.
With regard to outcome assessment, most pediatric cancers are life-threatening pathologies requiring hospitalization for diagnosis and treatment and are very unlikely to be underdiagnosed in France. The algorithms used in our study were able to identify the most frequent groups of childhood cancers with numbers close to that of the RNCE except for tumors of the adrenal glands, peripheral nerves, and autonomic nervous system, which were underestimated in our study based on ICD-10 diagnoses. Conversely, the number of bone tumors, hepatic tumors, and malignant CNS tumors was higher than that of the registry, possibly reflecting inclusions of metastatic localizations as primary sites. Exposure or outcome misclassification might have attenuated the associations.
Additionally, information on child emigration was not available. Given the rarity of childhood cancers, the number of potential undetected cases because of child emigration is likely to be small. Child emigration could also lead to overestimation of the follow-up time. However, these potential misclassification biases might not differ by conception mode; therefore, they should not have substantially biased our estimates.
Many potential confounders have been taken into account. However, as with most studies on the effects of fertility treatments on child health, our study was unable to distinguish between possible treatment effects and parental conditions underlying infertility 43 that might be associated with an increased risk of cancer in offspring. Therefore, residual confounding cannot be completely ruled out.
Finally, although this study included a large nationwide cohort, the absolute number of children with cancer was relatively small. Risk assessment of childhood cancers is challenging given their rarity, and results based on small numbers may be subject to both lack of power or the potential for spurious associations. We did not adjust for multiple comparisons. Therefore, these findings must be interpreted with caution.
In this cohort study, the overall risk of cancer was not increased after MAR but our findings suggest that children born after FET or fresh ET may have an increased risk of leukemia. This risk, although resulting in a limited number of cases, needs to be monitored in view of the continuous increase in the use of ART.
Accepted for Publication: February 12, 2024.
Published: May 2, 2024. doi:10.1001/jamanetworkopen.2024.9429
Open Access: This is an open access article distributed under the terms of the CC-BY License . © 2024 Rios P et al. JAMA Network Open .
Corresponding Author: Paula Rios, MD, PhD, EPI-PHARE Scientific Interest Group in Epidemiology of Health Products, French National Agency for Medicines and Health Products Safety, French National Health Insurance, 42 Bd de la Libération, 93200 Saint-Denis, France ( [email protected] ).
Author Contributions: Dr Rios and Mr Herlemont had full access to all of the data in the study and take responsibility for the integrity of the data and the accuracy of the data analysis. Drs Zureik, Clavel, and Dray-Spira contributed equally to this work.
Concept and design: Rios, Zureik, Clavel, Dray-Spira.
Acquisition, analysis, or interpretation of data: All authors.
Drafting of the manuscript: Rios, Herlemont, Zureik, Clavel.
Critical review of the manuscript for important intellectual content: Rios, Fauque, Lacour, Jouannet, Weill, Zureik, Clavel, Dray-Spira.
Statistical analysis: Rios, Clavel.
Supervision: Fauque, Lacour, Jouannet, Zureik, Clavel, Dray-Spira.
Conflict of Interest Disclosures: Dr Rios received grant funding from the French National Agency for Medicines and Health Products Safety during the conduct of the study. No other disclosures were reported.
Data Sharing Statement: See Supplement 2 .
- Register for email alerts with links to free full-text articles
- Access PDFs of free articles
- Manage your interests
- Save searches and receive search alerts
- Frontiers in Plant Science
- Sustainable and Intelligent Phytoprotection
- Research Topics
Plant Species Classification for Smart Phytoprotection
Total Downloads
Total Views and Downloads
About this Research Topic
The number of plant species is extremely vast, making it impractical and impossible for a botanist or expert to identify and classify all of them. Some plant species bear remarkable similarities to each other, making differentiation a time-consuming process. In addition, many plants are facing extinction. Both endangered and non-endangered plant species need proper preservation and conservation to reduce the risk of extinction. With the rapid development of information and communication technologies, it is possible to explore new research directions and practices for smart plant protection, “Smart Phytoprotection”, through emerging technologies. With the widespread application of intelligent technologies such as deep learning in plant species classification, plant recognition and classification have witnessed considerable improvement, aiding the preservation of endangered species and the advancement of agriculture and forestry disciplines. This Research Topic is designed to gather the most recent research on plant species classification for smart plant conservation, aiming to deepen our understanding of the various smart plant species classification techniques. We hope to address these issues through an interdisciplinary lens, facilitating discussion about intelligent image processing and the application of deep learning to plant recognition. Deep learning methods have surfaced as a promising alternative in plant recognition, displaying superiority over other manually crafted methods in feature extraction. Through scientific analysis and in-depth research on plant classification, we aspire to assist in plant protection, advance the sustainable development and utilization of biological resources, expand and enhance the bioeconomy, and foster harmonious coexistence between humans and nature. This topic discusses the latest advances in plant species classification based on deep learning, explores technological advancements in plant species classification, and addresses the challenges faced in practical applications. This topic explores the evolution of plant species classification, spanning from its historical roots to present advancements and future prospects. We welcome submissions of different types of manuscripts including original research papers, reviews, and methods, including but not limited to: ▪ Fast plant image recognition based on deep learning ▪ Research and implementation of plant image recognition methods ▪ Researching the optimization of deep learning algorithms for object detection ▪ Research on plant recognition algorithms considering complex backgrounds
Keywords : Phytoprotection, Deep Learning, Plant Recognition, Species Classification, Conservation
Important Note : All contributions to this Research Topic must be within the scope of the section and journal to which they are submitted, as defined in their mission statements. Frontiers reserves the right to guide an out-of-scope manuscript to a more suitable section or journal at any stage of peer review.
Topic Editors
Topic coordinators, submission deadlines, participating journals.
Manuscripts can be submitted to this Research Topic via the following journals:
total views
- Demographics
No records found
total views article views downloads topic views
Top countries
Top referring sites, about frontiers research topics.
With their unique mixes of varied contributions from Original Research to Review Articles, Research Topics unify the most influential researchers, the latest key findings and historical advances in a hot research area! Find out more on how to host your own Frontiers Research Topic or contribute to one as an author.
Epistemic Goals and Practices in Biology Curriculum—the Philippines and Japan
- Open access
- Published: 10 May 2024
Cite this article
You have full access to this open access article
- Denis Dyvee Errabo ORCID: orcid.org/0000-0002-4084-5142 1 , 2 , 3 ,
- Keigo Fujinami 2 na1 &
- Tetsuo Isozaki 2 na1
169 Accesses
Explore all metrics
Despite cultural differences, the Philippines–Japan partnership is developing an intentional teaching curriculum with parallel standards. However, disparities among their respective educational systems have prompted inequalities. As education plays a critical role in collaboration, we explored the Epistemic Goals (EGs) and Epistemic Practices (EPs) in the biology curriculum, with the research question: How do the epistemic goals and practices of the biology curriculum transmit knowledge and skills in the Philippines and Japan? Using an ethnographic design, we conducted two iterative explorations of EGs and EPs. First, we examined the curriculum policy to determine its EGs. Using the A-B-C-D protocol, we employed discourse analysis to evaluate knowledge and skills in the biology grade-level standards. Second, we examined the articulation of goals in classroom teaching practices. We conducted classroom immersion and observed classes to determine EPs and supported our observations through interviews, synthesizing the data using inductive content analysis. Our findings revealed that the Philippines’ EGs were to transmit factual knowledge enhanced by basic science skills, and their EPs were audio-visual materials, gamified instructions, guided inquiry, posing questions, and learning-by-doing. In comparison, Japan’s EGs were to provide a solid foundation of theoretical and metacognitive knowledge, integrated science skills, and positive attitudes. Its EPs involved cultivating lasting learning, observation, investigation, experimentation, collaborative discussion, and reflective thinking. Our study makes a meaningful contribution by shedding light on crucial ideologies and cultural identities embedded in Biology curricula and teaching traditions.
Similar content being viewed by others
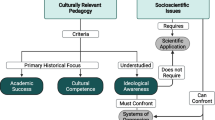
Re-envisioning Biology Curricula to Include Ideological Awareness
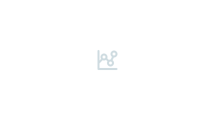
Aligning Teaching to Learning: A 3-Year Study Examining the Embedding of Language and Argumentation into Elementary Science Classrooms
Comparing the impact of two science-as-inquiry methods on the nos understanding of high-school biology students.
Avoid common mistakes on your manuscript.
Introduction
The cultural and educational connections within the Philippines-Japan collaboration establish the basis for developing long-lasting relationships between individuals. Despite cultural differences, both countries continue to develop an intentional teaching curriculum with parallel standards. According to Joseph ( 2010 ), the most effective way to demonstrate cultural ideology is through school curriculum. The term "curriculum" refers to different areas of education, such as the content taught in schools, learning methods, teacher approaches, and student progress assessment (Schiro, 2013 ). Understanding the basic components of an effective curriculum is critical to academic achievement.
Improving the Philippines’ curriculum is a significant and urgent matter given the considerable challenges they face in academic achievement. According to the Program for International Student Assessment (Organization for Economic Co-operation and Development, 2023 ), Filipino students exhibit relatively lower levels of achievement in critical academic domains such as science, mathematics, and reading (OECD, 2023 ). In contrast, the educational system in Japan is highly regarded for its exceptional quality and performance, consistently achieving top ranks among global academic systems. The 2022 PISA assessment shows that Japanese students consistently demonstrate superior performance compared with the average in their respective subject areas (OECD, 2023 ).
The disparities in outcomes and rankings between the education systems in Japan and the Philippines prompt an intriguing inquiry: what distinguishes Japanese education and how can we draw insights from its curricular practices to enhance the quality of education in the Philippines? This inquiry is of utmost importance as we aim to improve the educational outcomes and opportunities for Filipino students through an effective, quality curriculum. Moreover, it is essential to acknowledge the substantial research gap in curriculum studies regarding curricular benchmarks. This gap provides a valuable opportunity to gain insight into the unique educational system strategies.
Background of the Study
Examining the Epistemic Goals (EGs) and Epistemic Practices (EPs) of the biology curricula requires fundamental inquiries regarding the Nature of Science (NoS), the methodologies scientists employ in knowledge acquisition, and the scientific frameworks of understanding. Brock and Park ( 2022 ) argue that there has been a longstanding emphasis in science education on comprehending the NoS and the processes and undertakings of knowledge production. These essential elements are integrated as important learning goals in global science education curricula and policy documents (Leden & Hansson, 2019 ; Olson, 2018 ; Park et al., 2020 ).
EGs play a crucial role in establishing the fundamental and structural knowledge framework, including the required skills and attitudes. It encompasses the essential cognitive abilities that are pivotal for comprehension, academic engagement, and learning. It represents knowledge seeking, comprehension, and construction, particularly within the framework of the NoS (Chinn et al., 2011 ). Similarly, EGs enable individuals to explore their own beliefs about knowledge, as emphasized by Cho et al. ( 2011 ), with a significant influence on how individuals develop epistemic values and academic achievement. This includes improving advanced literacy skills, making informed decisions, and promoting a lifelong dedication to continuous learning.
Similarly, McDevitt et al. ( 1994 ) discuss how EPs involve various personal inquiry methods. The practices discussed by Hofer ( 2001 ) relate to the personal justification of knowledge acquisition. Personal justification of epistemic beliefs occurs through reliable processes when individual and social practices are considered within the epistemological framework (Chinn et al., 2011 ). According to Goldman ( 1999 ), considerable research has been dedicated to studying reliable belief formation processes, particularly concerning specific practices within scientific inquiry, arguing that practices, as opposed to errors and ignorance, have a relatively positive effect on knowledge. Furthermore, utilizing EPs include exploring external sources of information and engaging in active cognitive construction processes, as elucidated by Muis and Franco ( 2009 ). Hence, scientific inquiry is developed as a core emphasis to raise awareness, cultivate independent thinking skills, question assumptions, and make informed judgments.
Theoretical Framework
This study anchors its theoretical framework in the earlier work of Berland et al. ( 2016 ) on Epistemologies in Practice (EIP). Two epistemic folds define this framework.
First, the EIP defines epistemic goals for student knowledge acquisition, referring to the NoS as a means of understanding scientific development (Lederman, 2002 ). It entails an epistemological investigation of the fundamental features of reality such as the essence of truth, the process of justification, and the distinction between knowledge as a manifestation of capabilities and as a collection of factual information (Knight et al., 2014 ).
Moreover, defining goals is intimately connected to the epistemic dimensions; hence, this study examines how students use epistemic considerations when constructing scientific knowledge. This approach offers an analytical lens for understanding student involvement in scientific practices, which is vital to classroom and learning engagement. Berland et al. ( 2016 ) conducted a study identifying four noteworthy epistemic considerations: nature , generality , justification , and audience .
Nature explores an extensive range of knowledge. Fundamental to this consideration is the nature of knowledge (knowledge is) and that of knowing (knowledge acquisition) (Lederman, 2007 ; Schiefer et al., 2022 ). Generality delves into complex interconnections, forming an understanding using scientific concepts and facts. For instance, a phenomenon of interest can be comprehensively understood and explained within the scientific community by examining specific contexts and conditions utilizing scientific theories (Lewis & Belanger, 2015 ). Hence, this act of knowledge generation is crucial to thoroughly comprehending observed events and phenomena (Beeth & Hewson, 1999 ).
Next, justification underscores the necessity for logical reasoning to substantiate our conceptual comprehension. It is the systematic process that employs factual information and evidence, particularly that obtained from experiments, to substantiate assertions (Peffer & Ramezani, 2019 ). This practice links evidence with knowledge to assess essential claims and facilitates meaningful discussion (McNeill et al., 2006 ; Osborne et al., 2004 ). Finally, the audience dimension orients students' knowledge and the usefulness of their understanding (Berland et al., 2016 ). It is also relevant regarding how students perceive and derive meaning from the material, and how they develop a comprehensive understanding of it (Berland & Reiser, 2009 ; Paretti, 2009 ). The combined impact of these epistemic factors intricately shapes and defines the goals that guide the pursuit of epistemic knowledge.
Second, EIP includes essential practices in the classroom and learning community. In addition to acquiring discipline-specific knowledge, Peffer and Ramezani ( 2019 ) argue that demonstrating proficiency in scientific methodologies leads to developing a sophisticated epistemological understanding of concepts relevant to the NoS and scientific knowledge. Since the NoS is an essential element of inquiry in practice, epistemology and the NoS are inextricably linked (Deng et al., 2011 ). By exploring the NoS, we can gain insight into the fundamental elements that define scientific investigation, including its fundamental principles, underlying assumptions, and the methodologies of scientific pursuit.
According to Greene et al. ( 2016 ), NoS can be used interchangeably with concepts such as personal epistemology and epistemic cognition, which explore how individuals conceptualize knowledge. Personal epistemology reflects epistemological beliefs, reflective judgments, ways of knowing, and reflection (Hofer, 2001 ), whereas epistemic cognition is the examination of knowledge, particularly the evaluation of the essential components of justification and related concepts of objectivity, subjectivity, rationality, and truth (Moshman, 2014 ).
Furthermore, Lederman et al. ( 2002 ), referred NoS to the epistemology and sociology of science – understanding science as a way of knowing, and the values and beliefs inherent in scientific knowledge and its development. It encompasses various philosophical presuppositions, including values, development, conceptual inventions, consensus-building in the scientific community, and distinguishing scientific knowledge (Lederman, 1992 ; Smith & Wenk, 2006 ; Tsai, 2007 ). The close connection between an individual's cognitive framework and the philosophical foundations of the NoS becomes evident when we recognize that these concepts have a shared identity.
Research Question
In this study we analyzed the EGs and EPs in the Biology curriculum. Specifically, we address the question: How do the epistemic goals and practices of the Biology curriculum transmit knowledge and skills in the Philippines and Japan?
Research Design
We employed an ethnography design to examine the EGs and EPs of the biology curricula. Ethnography comprehensively explores the historical, cultural, and political aspects of knowledge evident in the educational traditions and practices of the countries under study (Hout, 2004 ). It involves systematically observing individuals, locations, concepts, written records, and behaviors (Savage, 2000 ) to document routine occurrences and identify opportunities for improvement (Dixon-Woods et al., 2019 ).
Research Strategies
We investigated two iterative cases of EGs and EPs. First, to determine the framework guiding the scope and implementation of EGs, we examined the Biology Grade Level Standards (BGLSs). In this context, EGs refer to the instructions’ specific statements and purposes that outline what students are expected to learn as they interact with the curriculum (Orr et al., 2022 ; Print, 1993 ).
According to Plowright ( 2011 ), the standards within a curriculum serve as its policies. A curriculum is inherently governed by the power and knowledge structures that stem from and circulate within sociocultural and political domains (Ball et al., 2012 ). As an artifact, it embodies culture, design, and learning (Hodder, 2000 ) and is associated with socio-material factors, discursive frameworks, policies, and performativity frameworks (Horan et al., 2014 ; Kalantzis & Cope, 2020 ; Maguire et al., 2011 ).
Second, we engaged in classroom immersion for observational (teaching) research (Sheal, 1989 ) to investigate the EPs. Teaching observation is an unbiased measure that allows us to gain a thorough, firsthand understanding of teaching practice (Desimone, 2009 ). Being physically present in the learning environment provides a unique opportunity to directly observe the teaching methods and strategies in real-time, including their application and usefulness (Granström et al., 2023 ). In addition to helping us identify opportunities for unique learning practices and ways to improve education (Sullivan et al., 2012 ), it provided a better understanding and appreciation of each country's cultural and pedagogical intricacies.
Data Collection and Gathering Procedures
This longitudinal study is part of an ongoing two-year community inquiry project. Our ongoing immersion began in the last quarter of 2022. The first iteration of the case focuses on the documented policies based on the BGLS. Policy materials were obtained from the websites of the Philippines Department of Education (DepEd) and the Ministry of Education, Culture, Sports, Science, and Technology (MEXT) ( 2006 ) in Japan. In the Philippines, science education goals are carefully designed with each grade level having its own standards that differentiate biology from other specialized areas of science, such as earth science, chemistry, and physics. The curriculum goals are divided into objectives customized for each grade level, thus ensuring a smooth and logical learning progression.
In contrast, science education in Japan follows a standardized set of overarching objectives that cover essential scientific concepts such as energy, particles (matter), life, and the earth. These objectives are outlined in the study course and provide a comprehensive framework that includes a range of knowledge, skills, and attitudes. The framework clearly outlines the overall objectives, making it possible to identify those specific to different scientific concepts.
The collected BGLSs were analyzed in the subsequent stages below.
Curriculum Matching and Mapping
Table 1 shows the curriculum matching results for both countries. DepEd and MEXT developed, implemented, and monitored the goals of the biology curriculum at the elementary (grades (G) 3–6), junior high (G7–G10), and lower secondary (G7–G9) levels. Employing Hale’s ( 2007 ) curriculum mapping protocol, to map EGs in the BGLS. Essential mapping was used to ascertain specific competencies, including detailed knowledge and abilities that students are expected to acquire.
Syntactic Analysis and Transformation
We expound upon these goals by examining their syntax. Syntax is a methodological analysis of the structure of sentences or statements (Foorman et al., 2016 ), including aspects such as word order, and structure. First, we investigated the verb-content-context and transformed it into Anderson and Krathwohl’s ( 2001 ) A-B-C-D protocol. As shown in Table 2 , a sample goal is divided into four distinct components.
Component A pertains to the intended audience , typically comprising students; component B relates to expected behavior or cognitive faculties component C pertains to the conditions necessary to demonstrate capabilities, and component D relates to the degree to which a behavior must be performed.
Classroom Immersion and Teaching Observation
We coordinated the immersion and teaching observation (IATO) with Philippine and Japanese school administrators. We were granted permission to conduct observations at three schools in Japan and two in the Philippines between January and December 2023. In August 2023, we conducted teaching observations in three classrooms in the Philippines. We further observed ten classrooms, which were predominantly held between November and December in Japan. Our observations encompass various aspects such as imparting subject knowledge, fostering skills, critical thinking abilities, and instilling specific values. Inside the classrooms, we were able to capture photographs and take detailed field notes, which allowed us to thoroughly document the interactions within each dynamic learning environment. By engaging in visual and observational documentation, we created a thorough record of the EPs. For ethical considerations, we deliberately chose not to incorporate any photographs of the students in this manuscript.
Interviews and Focus Group Discussions
After completing IATO, we conducted interviews with the educators to clarify the EPs. This dialogue dramatically improved our understanding of the factors influencing pedagogical decision-making by facilitating the exchange of ideas and perspectives. It also provided valuable context, enhancing our observations and enriching the quality of the observational data collected.
Data Analysis
Using discourse analysis (DA) and curriculum coding, we examined the explicit words that indicate EGs (knowledge and skills), which go beyond signs and signifiers by becoming “practices that methodically produce the objects of which they speak” (Foucault, 1972 , p. 49) at the expense of meaning formation (Khan & MacEachen, 2021 ).
We analyzed EGs based on the explicit BGLSs in the form of knowledge-using behavior and condition . Behavior referred to the knowledge dimension, and condition referred to content (scope of knowledge). To establish a connection between behavior and the cognitive domain, it is imperative to systematically categorize and classify individual cognitive verbs or processes based on their unique characteristics and underlying theoretical frameworks. This allows the development of personalized knowledge about cognitive tasks while contributing to a more organized understanding of cognitive functioning. Using Bloom’s Taxonomy of Objectives as revised by Anderson and Krathwohl ( 2001 ), we coded each behavior against the cognitive domains. Each cognitive domain uses active verbs arranged hierarchically. The first aspect is remembering , which facilitates quick recall (i.e., recognition). The second aspect is understanding , which allows one to make sense of knowledge/information (i.e., description). The third aspect is applying , which is a demonstration method/procedure (i.e., classification). The fourth aspect is analyzing , which enables breaking down the structure of one’s understanding into parts and pieces of information (i.e., differentiation). The fifth aspect is evaluating , which entails making use of one’s judgment based on parameters such as conditions (i.e., conclusion). Finally, the sixth aspect is creating , which involves putting together pieces of information to create cohesive and holistic knowledge (i.e., development).
Table 3 presents the coding of EGs using knowledge types. First, with the verb describe , we classified a wide range of behaviors from focus and recall to perception and processing to problem-solving and decision-making and compared and categorized the respective verbs based on characteristics derived from cognitive traits. In this context, the term be describes the understanding of information by employing the knowledge of principles. After determining behaviors using verbs, we further classified them into Anderson and Krathwohl’s ( 2001 ) types of knowledge (ToK). Each behavior is determined using the following: (1) familiarity with concepts, which necessitates acquiring factual knowledge (fk) , specifically knowledge of revealed facts; (2) conceptual knowledge (ck) encompassing the comprehension of ideas, associations, and operations; (3) procedural knowledge (pk), pertaining to the investigation methodology and knowledge acquisition within scientific inquiry; and (4) meta-cognitive knowledge (mck) , which denotes a more advanced level of comprehension pertaining to an individual’s understanding of cognition, self-awareness, and self-regulation. In Table 3 , remembering falls under fk , illustrating the knowledge of details/elements .
Similarly, we assessed EGs based on explicit standards in the form of practical skills (PSs) using condition and degree categories. Condition revealed the scope of knowledge and the degree of skill development. We examined the degree by selecting skills based on Gott and Duggan’s ( 1995 ) classification. These PSs were classified according to Finley ( 1983 ) Science skills . The first is Basic Science skills (BSs) , which cover fundamental scientific processes, including observation, classification, measurement, prediction, inference-making, and communication. Second, Integrated Science skills (ISs) are composites (two or more BSs) with fundamental scientific process competencies. Integrated science skills are uniformly identified as a control variable combined with interpreting, hypothesizing, and experimenting to form a cohesive approach.
Table 4 presents the coding of conditions and degrees. We underlined PSs (i.e., investigating) for ease of identification. Each skill is coded according to its degree of development. Finally, we classified the underlying skills as ISs .
Furthermore, we analyzed IATO data using inductive content analysis (ICA). ICA is a social inquiry method grounded in epistemology that depicts the reality of practice. For example, by examining learning delivery, one can identify replicable and valid strategies that can be used to draw inferences from the data (Krippendorff, 2019 ). We utilized Marying's ( 2000 ) ICA protocol to effectively organize, refine, and establish significant categories in teaching practice, ensuring that our observations and field notes were aligned.
Epistemic Goals and Practices – the Philippines
Table 5 presents the EGs and ToK in the Philippines context, utilizing behavior and condition . Regarding behavior , the data revealed a wide range of knowledge, primarily encompassing the domains of remembering and understanding. This trend indicates that the EGs emphasize acquiring crucial and foundational knowledge to develop fk , namely the specific details, elements, and principles of biology. Furthermore, this trend was consistently evident in G3, G4, G7, G8, and G9. However, we found variations in knowledge offerings for G5, G6, and G9. Higher order behavior incorporates mck in G5. This approach involves generating and cultivating strategic knowledge about health-promotion and hygienic practices. During G6, ck was presented to deliver life science principles, whereas during G9, more profound pk was presented. During G9, students were involved in the knowledge acquisition of scientific inquiry.
The condition suggests a progression of goals from elementary to junior high school. Fundamental principles of biology, such as the components and functions of living organisms, are systematically introduced in the early stages of education. For instance, as students progressed to higher grades, they were presented with more advanced concepts related to the organization and functioning of the human body.
Table 6 shows the degree-related goals and PSs in the Philippines. The data indicates that most elementary-level skills (G3–G6) involved classification, investigation, and communication. The acquisition of proficiency in classification and communication skills are imperative for developing a solid foundation for scientific literacy, commonly known as BSs . This investigation enabled a comprehensive scientific inquiry encompassing extensive processes. Investigative skills in G5 and advancements in classification improve the exploration and comprehension of biological phenomena, a combination of skills commonly referred to as ISs .
Additionally, we acknowledge the skills alignment with the proficiencies exhibited in junior high school. Where the use of condition and degree in the syntax did not effectively express practical skills, we resorted to observing behavior as an indicator of the skill dimension. Both the G7 and G8 levels of the curriculum employed the term recognize . In contrast, at the G9 level, the term familiar was used, implying the incorporation of students’ sensory abilities, such as sight or visual perception. These BSs enable students to cultivate their power of observation.
During our IATO, we identified recurring themes to indicate the EPs in the Philippines.
Audio-Visual Materials
We frequently noticed how adept educators were in using audio-visual materials (AVM) to leverage their instruction. Strategically integrating AVM materials led to more engaging and interactive multimedia content for students while stimulating their auditory and visual faculties. Interestingly, we found that the use of AVM also encourages inclusivity within the classroom. By supporting diverse learning preferences, AVM fostered wider understanding, retention, and promoted significant learning experiences.
Gamified Instruction
Several students actively participated in thrilling learning experiences. We observed a gamified strategy that effectively utilized game elements to optimize student engagement. Teachers incorporated gamified experiences, including quick recall sessions, critical thinking exercises, and formative assessments. The interactive nature of gamified experiences captured students’ attention, transforming ordinary learning activities into intellectually stimulating tasks. Therefore, sparked greater motivation, and consistent engagement.
Guided Inquiry
Students demonstrated scientific exploration consistent along with the structured guidance by their teachers. Curiosity prompted students to ask scientific questions and uncover practical solutions. This increased their interest and understanding to learning, while honing important abilities such as inquiry, critical thinking, and decision-making.
Posing Questions
We observed the art of posing thought-provoking questions. Posing questions tapped into students' inherent curiosity while stimulating their interest and motivation. Teachers often asked questions to probe student understanding and ask critical questions. Students learned self-regulation, critical inquiry, and advanced learning while providing relevant, accurate, and thorough knowledge through this guided process.
Learning-By-Doing
We witnessed a learning experience in which the students were active participants. They were engaged in dynamic discussions that provided them with first-hand encounters toward understanding. During this period, students actively engaged in observing phenomena and scientific processes. Through hands-on experiences, engaged learners assume responsibility for their own understanding. They skillfully implement acquired knowledge while effectively connecting theoretical ideas to real-life situations.
Epistemic Goals and Practices – Japan
Table 7 presents the EGs and ToK by incorporating behavior and condition . Japan has a standardized overall objective (goals) from elementary to lower secondary/junior high schools. The objective is to construct a layer: in elementary school science, each grade’s objectives fall under the subject’s overall objectives and that of lower secondary school science. Under the “objectives of science as a subject,” the first (energy and particles) and second (life and earth) fields have their own objectives, and each unit of the two fields has objectives based on the upper levels. This classification includes knowledge, abilities, and attitudes. We observed a comparable classification between the elementary and lower secondary levels. Within this categorization, there is remarkable uniformity in behavior, which illustrates the knowledge pattern. Students acquire knowledge, abilities, and attributes through higher cognitive learning, specifically in the form of creation. Each form of mck then contributes to the development of strategic knowledge, knowledge of cognitive tasks, and self-knowledge from G3–G9.
This condition entails a deeper understanding of living things, the structure of movement, the continuity of life, and the structure and function of the body. Various biology concepts facilitate scientific inquiry with the objective of advancing the understanding and acquisition of metacognitive knowledge. These objectives were designed to enhance proficiency in employing scientific methods, specifically in conducting scientific inquiry into natural objects, experiencing objects, and understanding phenomena. Furthermore, the process of developing student understanding is facilitated by their direct engagement with objects and phenomena, while honing their attitudes toward scientific inquiry.
Table 8 shows the degree-related EGs and PSs in Japan. The goals consist of knowledge, abilities, and attitude, and demonstrate the consistency of learning development across the elementary and lower secondary school levels. Irrespective of the concept being considered, skill development follows a standardized approach from G3 to G9. PSs are uniform across various learning domains, like all knowledge derived from active demonstration, including observations, experiments, and other scientific activities. Similarly, we noted that student abilities were centered around a repetitive mode of inquiry. The students employ and hone their skills to enhance their comprehension of biological principles. Furthermore, cultivating a positive attitude toward nature, life, and the environment requires consistent practice and refining one’s abilities. By employing observation, experimentation, and other practical work, students cultivate a positive disposition toward scientific inquiry and conducting scientific inquiries.
Our IATO in different schools, helped us determine recurring themes to indicate the EPs in Japan.
Cultivating Lasting Learning
Japanese teachers cultivate lasting learning. They began their lessons by writing the learning goals which are grounded on shared responsibility, to develop a sense of direction and purpose. They introduce real-world problems that allow students to connect their prior understanding. During active learning activities, the teachers gathered students’ observations and methodically arranged them on classroom boards. Such visual representations served as a valuable reference for ongoing discussions, reflection, and knowledge construction. It depicted patterns and variation that can elicit further scientific inquiries. Similarly, it promotes data-driven practice towards generating conclusions and generalizations. This approach bolstered students' capacity for analysis and cultivated a more profound comprehension of biology.
Observation, Investigation, and Experimentation
We observed learners utilizing their senses to examine organisms. They engaged in direct interactions under meticulously replicated conditions in the classroom or laboratory. They participated in a wide range of scientific activities and performed experiments. They diligently adhered to scientific methodologies and precisely recorded their discoveries to enhance understanding of diverse scientific phenomena and processes through practical activities.
Collaborative Discussion
All classes were encouraged to participate in micro-discussions. This allowed the students to ask questions, seek clarification, and enhance their understanding in a smaller and supportive environment. It was crucial for students with advanced understanding to take the lead and facilitate the discussion. Collaborative discussions were instrumental to learning from peers and affirming understanding, while expressing their thoughts and beliefs leading to collective empowerment and collaborative learning.
Reflective Thinking
The classes were adept in reflective thinking. This method encouraged students to carefully review what they had learned and evaluate if their present experiences met the learning objectives. Teachers designed purposeful queries to prompt reflection. While the students were provided ample time to ponder and participate in creating a tranquil environment for introspection.
Epistemic Goals – the Philippines and Japan
In the Philippines, EGs focus on transmitting fk . Both fk and ck are crucial for cognitive proficiency advancement (Schraw, 2006 ) and for helping students perform better in school (Idrus et al., 2022 ). Having a solid foundation of fk is essential for comprehending biological concepts. Thus, these goals aid in the development of critical thinking skills and enhancing students’ self-confidence. Moreover, this knowledge helps individuals navigate their surroundings, make informed choices, and contribute to a knowledgeable and enlightened society. Fk leverages ck , in contrast to the mere acquisition of information; fostering critical thinking skills and facilitating the transfer of learning, adaptability, and effective problem-solving.
The Philippines’ EGs mainly involve transmitting scientific skills essential for establishing scientific literacy and active participation in scientific investigations. Individuals with such skills can confidently observe, communicate, measure, hypothesize, analyze data, solve issues, and navigate the life sciences. Improving and refining these skills increases scientific comprehension and builds crucial life skills such as critical thinking, problem-solving, and communication.
In contrast, EGs in Japan center on transmitting mck , which is critical for cognitive development and learning. This knowledge can govern and regulate all aspects of knowledge or processes and can be applied to any cognitive pursuit, including learning (Flavell, 1979 ). This enables individuals to control their learning, adjust their strategies, participate in metacognitive processes, and apply their knowledge to new situations.
Japan’s EGs transmit highly integrated skills that provide a comprehensive and interdisciplinary approach to scientific inquiry. Such skills foster a holistic comprehension of broader issues and the cultivation of analytical and reasoning abilities, ideation, and advanced learning. Padilla ( 1990 ) posits that acquiring expertise is imperative for the development, experimentation, and execution of scientific research. Acquiring integrated scientific processing skills enables individuals to proficiently address complex challenges, contribute meaningfully to scientific advancement, and have a considerable impact on their understanding of biology.
Epistemic Practices – the Philippines and Japan
Epistemic practices in the Philippines capitalize on timely and relevant learner-centered pedagogy. The strategic integration of AVM resulted in an engaging and interactive classroom. AVM are designed to cater to diverse learning styles and stimulate learners’ auditory and visual faculties. AVM or multimedia inside the classroom consists of more than one medium aided by technology (Kapi et al., 2017 ; Abdulrahaman et al., 2020 ) and is used to improve understanding (Guan et al., 2018 ). Shaojie et al. ( 2022 ) found that AVM input can enrich learners' understanding of the content and motivate them to actively participate in listening comprehension activities by providing more authentic language input that is richer in multimodal cultural and situational contexts. Moreover, AVM promotes inclusivity by accommodating diverse learning preferences and enhancing comprehension and retention. This drives students’ eagerness to learn, while simplifying and adding excitement to the learning process (Rasul et al., 2011 ). AVM found to enhance student motivation and engagement (Dichev & Dicheva, 2017 ), as well as improve positive learning outcomes (Zainuddin, 2023 ), thus positively impacting student focus and concentration. Integrating gamified elements proved effective in capturing students' attention and foster a higher level of engagement.
It was also evident that the students exhibited a proactive and experiential approach toward scientific exploration. According to Kong ( 2021 ), this educational phenomenon promotes engagement and eventually leads to classroom success. The students demonstrated genuine and inherent curiosity and displayed a sincere interest in biology. Wang et al. ( 2022 ) argue that inquiries and epistemological beliefs form the foundation of scientific literacy. The teachers' adept organization and support effectively nurtured this curiosity. Students’ inherent inquisitiveness, under the guidance of the teacher's intentional mentorship, fostered an atmosphere conducive to purposeful inquiry and thus a heightened comprehension of biology. Based on Lin et al. ( 2011 ) and Jack et al. ( 2014 ), advancing toward scientific understanding and the application of scientific knowledge promotes interest in learning science.
Finally, educators' ability to pose thought-provoking questions has become important in the classroom. Each teacher's inquiries shaped classroom dynamics and fostered students' curiosity, critical thinking, and academic growth (Salmon & Barrera, 2021 ). Hilsdon ( 2010 ) states that insightful inquiries can lead to critical thinking by efficiently probing comprehension. Students actively participate in dynamic discussions and take responsibility for their learning.
Conversely, EPs in Japan use advanced methods to create a highly engaged and learning environment, outperforming traditional education. Teacher techniques included collaborative conversations, reflective thinking, and strategic use of thought-provoking questions throughout our classroom visits. This fostered active participation that encouraged students to critically engage and reflect on their learning. Higher-order thinking skills are essential for conceptual and disciplinary understanding (Heron & Palfreyman, 2023 ). These skills enable students to examine, synthesize, and evaluate information beyond fundamental knowledge.
Barlow et al. ( 2020 ) noted that in extensive research, empirical evidence is consistent, indicating that students who actively engage with learning materials and participate in the educational process demonstrate increased levels of engagement and achieve significantly greater learning outcomes. Similarly, Wang et al. ( 2022 ) argue that metacognitive skills help students learn and perform better. Furthermore, metacognition, or higher learning, also prepares learners for higher education (Stanton et al., 2021 ).
Reflective breaks were thoughtfully included in classroom immersion. Teachers set aside times for students to reflect. It reflects Japan's educational philosophy, which emphasizes learning, internalizing, and synthesizing knowledge to improve metacognition (Hanya et al., 2014 ). Kolb ( 1984 ) successfully linked reflection to experiential learning. The Japanese way of active learning transfer incorporates collaborative discussion and reflective dialogue. Dewey ( 1993 ) argues that reflective thinking examines beliefs, requiring careful examination of reporting, relating, reasoning, and reconstructing knowledge (Ryan, 2013 ).
We conducted ethnographic research examining two iterative cases of EGs and EPs of biology curriculum in the Philippines and Japan. We analyzed how these curricula effectively transmit valuable knowledge and skills. We found that the EGs in the Philippines were primarily grounded in disseminating factual knowledge with a specific emphasis on enhancing health and environmental awareness. Knowledge acquisition transitions from factual to conceptual as students progress to junior high school. EGs emphasize the utilization of basic science skills , particularly for exploring and comprehending various biological concepts. Alternatively, EPs prioritize learner-centered approaches that are both timely and relevant. These EPs include using AVM, gamified instruction, guided inquiry, thought-provoking questions, and hands-on learning experience.
However, EGs in Japan differed, focusing on a reliable means of imparting meta-cognitive knowledge . Students are equipped with problem-solving abilities and empowered to acquire integrated science skills to effectively engage in scientific inquiry. Implementing EPs fosters a sustainable learning environment and cultivates lasting learning, observation, investigation, experimentation, collaborative discussion, and reflective thinking.
Our findings shed light on the distinct and prioritized elements of biology standards and its EGs and EPs, making it a valuable addition to the current body of literature. Examining the realm of curriculum can improve comprehension, spark significant conversations, and enable informed decisions across cultures and borders. This research invites educators, policymakers, and stakeholders to embrace varied educational approaches to build a global community exploring knowledge and skills across national lines.
Limitations and Implications
The scope of this study is limited to a DA of the EGs and an ICA of the EPs. Our study provides insights into the development of policies and interventions that can address gaps in EGs and Eps. They can be used as a foundation for improving the biology curriculum in line with educational objectives and societal needs. Educators can also derive advantages from the findings of this study by engaging in professional development programs specifically designed to equip them with the essential skills and knowledge required to effectively implement learner-centric methodologies and integrate innovative teaching practices seamlessly. In addition, this study's cross-cultural benchmarks provide the potential for collaborative initiatives among educational institutions. Gaining insight into both commonalities and distinctions in EGs and EPs can foster cooperative endeavors aimed at improving global educational benchmarks.
Data Availability
The data have been made accessible in the results.
Abdulrahaman, M. D., Faruk, N., Oloyede, A. A., Surajudeen-Bakinde, N. T., Olawoyin, L. A., Mejabi, O. V., Imam Fulani, Y. O., Fahm, A. O., & Azeez, A. L. (2020). Multimedia tools in the teaching andlearningprocesses: A systematic review. Heliyon, 6 (11), e05312. https://doi.org/10.1016/j.heliyon.2020.e05312
Article Google Scholar
Anderson, L. W., & Krathwohl, D. R. (2001). A taxonomy for learning, teaching, and assessing: A revision of Bloom’s taxonomy of educational objectives . Longman.
Google Scholar
Ball, S. J., Maguire, M., & Braun, A. (2012). How schools do policy: Policy enactment in secondary schools . Routledge.
Barlow, A., Brown, S., Lutz, B., et al. (2020). Development of the Student Course Cognitive Engagement Instrument (SCCEI) for college engineering courses. International Journal of STEM Education, 7 (1), 22. https://doi.org/10.1186/s40594-020-00220-9
Beeth, M. E., & Hewson, P. W. (1999). Learning goals in an exemplary science teacher’s practice: Cognitive and social factors in teaching for conceptual change. Science Education, 83 , 738–760.
Berland, L. K., & Reiser, B. J. (2009). Making sense of argumentation and explanation. Science Education, 93 (1), 26–55.
Berland, L. K., Schwarz, C. V., Krist, C., Kenyon, L., Lo, A. S., & Reiser, B. J. (2016). Epistemologies in practice: Making scientific practices meaningful for students. Journal of Research in Science Teaching, 53 (7), 1082–1112. https://doi.org/10.1002/tea.21257
Brock, R., & Park, W. (2022). Distinguishing Nature of Science Beliefs, Knowledge, and Understandings. Science & Education. Advance Online Publication. https://doi.org/10.1007/s11191-022-00368-6
Chinn, C. A., Buckland, L. A., & Samarapungavan, A. (2011). Expanding the dimensions of epistemic cognition: Arguments from philosophy and psychology. Educational Psychologist, 46 (3), 141–167. https://doi.org/10.1080/00461520.2011.587722
Cho, M. H., Lankford, D. M., & Wescott, D. J. (2011). Exploring the relationships among epistemological beliefs, nature of science, and conceptual change in the learning of evolutionary theory. Evolution: Education and Outreach , 4(3), 313–322. https://doi.org/10.1007/s12052-011-0324-7
Deng, F., Chen, D. T., Tsai, C. C., & Chai, C. S. (2011). Students’ views of the nature of science: A critical review of research. Science Education, 95 , 961–999.
Department of Education (DepEd) (n.d.). Executive report . https://www.deped.gov.ph/2022/06/02/deped-to-launch-basic-educationdevelopment-plan-2030-as-strategic-roadmap-for-basic-education/
Desimone, L. M. (2009). Improving impact studies of teachers’ professional development: Toward better conceptualizations and measures. Educational Researcher , 38 (3), 181–199. https://www.jstor.org/stable/20532527
Dewey, J. (1993). How we think: A restatement of the relation of reflective thinking to the educativeprocess . D. C. Heath.
Dichev, C., & Dicheva, D. (2017). Gamifying education: What is known, what is believed and what remains uncertain: A critical review. International Journal of Educational Technology in Higher Education, 14 , 9. https://doi.org/10.1186/s41239-017-0042-5
Dixon-Woods, M., Campbell, A., Aveling, E. L., & Martin, G. (2019). An ethnographic study of improving data collection and completeness in large-scale data exercises. Wellcome Open Research , 4 , 203. https://doi.org/10.12688/wellcomeopenres.14993.1
Finley, F. N. (1983). Science processes. Journal of Research in Science Teaching, 20 (1), 47–54. https://doi.org/10.1002/tea.3660200105
Flavell, J. H. (1979). Metacognition and cognitive monitoring: A new area of cognitive–developmental inquiry. American Psychologist, 34 (10), 906–911. https://doi.org/10.1037/0003-066X.34.10.906
Foorman, B., Beyler, N., Borradaile, K., Coyne, M., Denton, C. A., Dimino, J., Furgeson, J., Hayes, L., Henke, J., Justice, L., Keating, B., Lewis, W., Sattar, S., Streke, A., Wagner, R., & Wissel, S. (2016). Foundational skills to support reading for understanding in kindergarten through 3rd grade (NCEE 2016–4008). National Center for Education Evaluation and Regional Assistance (NCEE), Institute of Education Sciences, U.S. Department of Education.
Foucault, M. (1972). The archaeology of knowledge (A. M. S. Smith, Trans.). Pantheon Books.
Goldman, A. I. (1999). Knowledge in a social world . Oxford University Press.
Book Google Scholar
Gott, R., & Duggan, S. (1995). Investigative work in the science curriculum . Open University Press.
Granström, M., Kikas, E., & Eisenschmidt, E. (2023). Classroom observations: How do teachers teachlearning strategies? Frontiers in Education, 8 , 1119519. https://doi.org/10.3389/feduc.2023.1119519
Greene, J. A., Sandoval, W. A., & Bråten, I. (2016). Handbook of epistemic cognition . Routledge Ltd. https://doi.org/10.4324/9781315795225
Guan, N., Song, J., & Li, D. (2018). On the advantages of computer multimedia-aided English teaching. Procedia Computer Science, 131 , 727–732. https://doi.org/10.1016/j.procs.2018.04.126
Hale, J. A. (2007). Guide to curriculum mapping: Planning, implementing, and sustaining the process . Sage.
Hanya, M., Yonei, H., Kurono, S., & Kamei, H. (2014). Development of reflective thinking in pharmacy students to improve their communication with patients through a process of role-playing, videoreviews, and transcript creation. Currents in Pharmacy Teaching and Learning, 6 (1), 122–129.
Heron, M., & Palfreyman, D. M. (2023). Exploring higher-order thinking in higher education seminar talk. College Teaching, 71 (4), 252–259. https://doi.org/10.1080/87567555.2021.2018397
Hilsdon, J. (2010). Critical thinking . Learning development with Plymouth University. Retrieved from http://www.plymouth.ac.uk/learn
Hodder, I. (2000). The interpretation of documents and material culture. In N. K. Denzin & Y. S. Lincoln (Eds.), Handbook of qualitative research (2 nd ed., pp. 703–715). Sage.
Hofer, B. K. (2001). Personal epistemology research: Implications for learning and teaching. EducationalPsychology Review, 13 , 353–383.
Horan, C., Finch, J., & Reid, E. (2014). The performativity of objects: The sociomaterial role of imaginal others [Conference presentation]. European Group for Organisation Studies (EGOS) Conference, Rotterdam, Netherlands.
Hout, S. (2004). Ethnography: Understanding occupation through an examination of culture. In S. Naylor & M. Stanley (Eds.), Qualitative research methodologies for occupational science and therapy (pp. 84–101). Taylor & Francis.
Idrus, H., Rahim, S. S. A., & Zulnaidi, H. (2022). Conceptual knowledge in area measurement for primary school students: A systematic review. STEM Education, 2 (1), 47–58. https://doi.org/10.3934/steme.2022003
Jack, B. M., Lin, H.-S., & Yore, L. D. (2014). The synergistic effect of affective factors on student learning outcomes. Journal of Research in Science Teaching, 51 (8), 1084–1101. https://doi.org/10.1002/tea.21153
Joseph, P. B. (Ed.). (2010). Cultures of curriculum (2nd ed.). Routledge.
Kalantzis, M., & Cope, B. (2020). Learning by design glossary: Artefacts . http://newlearningonline.com/learning-by-design/glossary/artefact
Kapi, A. Y., Osman, N., Ramli, R. Z., & Taib, J. M. (2017). Multimedia education tools for effective teaching and learning. Journal of Telecommunication, Electronic and Computer Engineering, 9 (2–8), 143–146.
Khan, T. H., & MacEachen, E. (2021). Foucauldian discourse analysis: Moving beyond a social constructionist analytic. International Journal of Qualitative Methods , 20 . https://doi.org/10.1177/16094069211018009
Knight, S., Buckingham Shum, S., & Littleton, K. (2014). Epistemology, assessment, pedagogy: Where learning meets analytics in the middle space. Journal of Learning Analytics , 1 (2), 23–47. https://doi.org/10.18608/jla.2014.12.3 .
Kolb, D. A. (1984). Experiential learning: Experience as the source of learning and development (Vol. 1). Prentice-Hall.
Kong, Y. (2021). The Role of Experiential Learning on Students’ Motivation and Classroom Engagement. Frontiers in Psychology, 12 , 771272. https://doi.org/10.3389/fpsyg.2021.771272
Krippendorff, K. (2019). Analytical constructs. In Content Analysis: An Introduction to Its Methodology (Fourth Edition ed., pp. 178–194). SAGE Publications, Inc., https://doi.org/10.4135/9781071878781
Leden, L., & Hansson, L. (2019). Nature of science progression in school year 1–9: A case study of teachers’ suggestions and rationales. Research in Science Education, 49 (2), 591–611. https://doi.org/10.1007/s11165-017-9628-0
Lederman, N. G. (1992). Students’ and teachers’ conceptions of the nature of science: A review of the research. Journal of Research in Science Teaching, 29 (4), 331–359.
Lederman, N. G. (2002). The state of science education: Subject matter without context. Electronic Journal of Science Education , 3 (2). Retrieved from http://unr.edu/homepage/jcannon/ejse/ejse.html
Lederman, N. G. (2007). Nature of science: Past, present, and future. In S. K. Abell & N. G. Lederman (Eds.), Handbook of research on science education (pp. 831–879). Lawrence Erlbaum Associates.
Lederman, N. G., Abd-El-Khalick, F., Bell, R. L., & Schwartz, R. S. (2002). Views of nature of science questionnaire: Toward valid and meaningful assessment of learners’ conceptions of nature of science. Journal of Research in Science Teaching, 39 (6), 497–521.
Lewis, C. T., & Belanger, C. (2015). The generality of scientific models: A measure theoretic approach. Synthese, 192 , 269–285. https://doi.org/10.1007/s11229-014-0567-2
Lin, H. S., Hong, Z. R., Chen, C. C., & Chou, C. H. (2011). The effect of integrating aesthetic understanding in reflective inquiry activities. International Journal of Science Education, 33 (9), 1199–1217. https://doi.org/10.1080/09500693.2010.504788
Maguire, M., Hoskins, K., & Ball, S. J. (2011). Policy discourses in school texts. Discourse: Studies in the Cultural Politics of Education, 32 (4), 597–609.
Mayring, P. (2000). Qualitative content analysis [28 paragraphs]. Forum Qualitative Sozialforschung / Forum: Qualitative Social Research, 1 (2), Article 20. http://nbn-resolving.de/urn:nbn:de:0114fqs0002204
McDevitt, T. M., Sheehan, E. P., Cooney, J. B., & Smith, H. V. (1994). Conceptions of listening, learning processes, and epistemologies held by American, Irish, and Australian university students. Learning & Individual Differences, 6 , 231–256.
McNeill, K. L., Lizotte, D. J., Krajcik, J., & Marx, R. W. (2006). Supporting students’ construction of scientific explanations by fading scaffolds in instructional materials. The Journal of the Learning Sciences, 15 (2), 153–191.
Ministry of Education, Culture, Sports, Science and Technology. (2006). Basic Act on Education . https://www.mext.go.jp/en/policy/education/lawandplan/title01/detail01/1373798.htm
Moshman, D. (2014). Epistemic domains of reasoning. In H. Markovits (Ed.), The developmental psychology of reasoning and decision-making (pp. 115–129). Psychology Press.
Muis, K. R., & Franco, G. M. (2009). Epistemic beliefs: Setting the standards for self-regulated learning. Contemporary Educational Psychology, 34 , 306–318.
Olson, J. K. (2018). The inclusion of the nature of science in nine recent international science education standards documents. Science & Education, 27 (7), 637–660.
Organization for Economic Co-operation and Development. (2023). PISA 2022 results (Volume I): The state of learning and equity in education . OECD Publishing. https://doi.org/10.1787/53f23881-en
Orr, R. B., Csikari, M. M., Freeman, S., & Rodriguez, M. C. (2022). Writing and using learning objectives. CBE Life Sciences Education , 21 (3), fe3. https://doi.org/10.1187/cbe.22-04-0073
Osborne, J. F., Erduran, S., & Simon, S. (2004). Enhancing the quality of argumentation in school science. Journal of Research in Science Teaching, 41 (10), 994–1020.
Padilla, M. J. (1990). The science process skills . National Association for Research in Science Teaching (NARST)
Paretti, M. (2009). When the teacher is the audience: Assignment design and assessment in the absence of “real” readers. In M. Weiser, B. Fehler, & A. Gonzalez (Eds.), Engaging audience: Writing in an age of new literacies. National Council of Teachers of English.
Park, W., Wu, J. Y., & Erduran, S. (2020). The nature of STEM disciplines in the science education standards documents from the USA, Korea, and Taiwan. Science & Education, 29 (4), 899–927.
Peffer, M. E., & Ramezani, N. (2019). Assessing epistemological beliefs of experts and novices via practices in authentic science inquiry. International Journal of STEM Education, 6 (1), 3. https://doi.org/10.1186/s40594-018-0157-9
Plowright, D. (2011). Using mixed methods: Frameworks for an integrated methodology . Sage.
Print, M. (1993). Curriculum development and design (2nd ed.). Routledge.
Rasul, S., Bukhsh, Q., & Batool, S. (2011). A study to analyze the effectiveness of audio visual aids in teaching learning process at Uvniversity level. Procedia Social and Behavioral Sciences, 28 , 78–81. https://doi.org/10.1016/j.sbspro.2011.11.016
Ryan, M. (2013). The pedagogical balancing act: Teaching reflection in higher education. Teaching in Higher Education, 18 (2), 144–151.
Salmon, A. K., & Barrera, M. X. (2021). Intentional questioning to promote thinking and learning. Thinking Skills and Creativity, 40 , 100822. https://doi.org/10.1016/j.tsc.2021.100822
Savage, J. (2000). Ethnography and health care. BMJ (Clinical Research Ed), 321 (7273), 1400–1402. https://doi.org/10.1136/bmj.321.7273.1400
Schiefer, J., Edelsbrunner, P. A., Bernholt, A., et al. (2022). Epistemic beliefs in science—a systematic integration of evidence from multiple studies. Educational Psychology Review, 34 (4), 1541–1575. https://doi.org/10.1007/s10648-022-09661-w
Schiro, M. (2013). Curriculum theory. Conflicting visions and enduring concerns (2nd ed.). Sage.
Schraw, G. (2006). Knowledge: Structures and processes. In P. A. Alexander & P. H. Winne (Eds.), Handbook of educational psychology (pp. 245–263). Lawrence Erlbaum.
Shaojie, T., Samad, A. A., & Ismail, L. (2022). Systematic literature review on audio-visual multimodal input in listening comprehension. Frontiers in Psychology, 13 , 980133. https://doi.org/10.3389/fpsyg.2022.980133
Sheal, P. (1989). Classroom observation: Training the observers. ELT Journal, 43 (2), 92–104. https://doi.org/10.1093/elt/43.2.92
Smith, C. L., & Wenk, L. (2006). Relations among three aspects of first-year college students’ epistemologies of science. Journal of Research in Science Teaching, 43 (8), 747–785.
Stanton, J. D., Sebesta, A. J., & Dunlosky, J. (2021). Fostering metacognition to support student learning and performance. CBE Life Sciences Education , 20(2), fe3. https://doi.org/10.1187/cbe.20-12-0289 .
Sullivan, P. B., Buckle, A., Nicky, G., et al. (2012). Peer observation of teaching as a faculty development tool. BMC Medical Education, 12 , 26. https://doi.org/10.1186/1472-6920-12-26
Tsai, C. C. (2007). Teachers’ scientific epistemological views: The coherence with instruction and students’ views. Science Education, 91 (2), 222–243. https://doi.org/10.1002/sce.20175
Wang, H. H., Hong, Z. R., & She, H. C. (2022). The role of structured inquiry, open inquiry, and epistemological beliefs in developing secondary students’ scientific and mathematical literacies. International Journal of STEM Education, 9 , 14. https://doi.org/10.1186/s40594-022-00329-z
Zainuddin, Z. (2023). Integrating ease of use and affordable gamification-based instruction into a remote learning environment . Advance online publication. https://doi.org/10.1007/s12564-023-09832-6
Download references
Open Access funding provided by Hiroshima University. This research was financially supported by the Japan Society for the Promotion of Science (JSPS) KAKENHI program under Grant Number 22KF0274.
Author information
Keigo Fujinami and Tetsuo Isozaki contributed equally to this work.
Authors and Affiliations
International Research Fellow, Japan Society for the Promotion of Science Postdoctoral Fellowship (Standard), Tokyo, Japan
Denis Dyvee Errabo
Graduate School of Humanities and Social Science, Hiroshima University, Hiroshima, Japan
Denis Dyvee Errabo, Keigo Fujinami & Tetsuo Isozaki
Department of Science Education, Bro. Andrew Gonzales FSC College of Education, De La Salle University, Manila, Philippines
You can also search for this author in PubMed Google Scholar
Corresponding author
Correspondence to Denis Dyvee Errabo .
Ethics declarations
Ethical standards.
This research conformed to the ethical standards approved by the institutional review board.
Conflict of Interest
The authors declared no conflict of interest.
Additional information
Publisher's note.
Springer Nature remains neutral with regard to jurisdictional claims in published maps and institutional affiliations.
Rights and permissions
Open Access This article is licensed under a Creative Commons Attribution 4.0 International License, which permits use, sharing, adaptation, distribution and reproduction in any medium or format, as long as you give appropriate credit to the original author(s) and the source, provide a link to the Creative Commons licence, and indicate if changes were made. The images or other third party material in this article are included in the article's Creative Commons licence, unless indicated otherwise in a credit line to the material. If material is not included in the article's Creative Commons licence and your intended use is not permitted by statutory regulation or exceeds the permitted use, you will need to obtain permission directly from the copyright holder. To view a copy of this licence, visit http://creativecommons.org/licenses/by/4.0/ .
Reprints and permissions
About this article
Errabo, D.D., Fujinami, K. & Isozaki, T. Epistemic Goals and Practices in Biology Curriculum—the Philippines and Japan. Res Sci Educ (2024). https://doi.org/10.1007/s11165-024-10170-9
Download citation
Accepted : 22 April 2024
Published : 10 May 2024
DOI : https://doi.org/10.1007/s11165-024-10170-9
Share this article
Anyone you share the following link with will be able to read this content:
Sorry, a shareable link is not currently available for this article.
Provided by the Springer Nature SharedIt content-sharing initiative
- Biology curriculum
- Epistemic goals
- Epistemic practices
- Science education
- Find a journal
- Publish with us
- Track your research
Artificial intelligence in strategy
Can machines automate strategy development? The short answer is no. However, there are numerous aspects of strategists’ work where AI and advanced analytics tools can already bring enormous value. Yuval Atsmon is a senior partner who leads the new McKinsey Center for Strategy Innovation, which studies ways new technologies can augment the timeless principles of strategy. In this episode of the Inside the Strategy Room podcast, he explains how artificial intelligence is already transforming strategy and what’s on the horizon. This is an edited transcript of the discussion. For more conversations on the strategy issues that matter, follow the series on your preferred podcast platform .
Joanna Pachner: What does artificial intelligence mean in the context of strategy?
Yuval Atsmon: When people talk about artificial intelligence, they include everything to do with analytics, automation, and data analysis. Marvin Minsky, the pioneer of artificial intelligence research in the 1960s, talked about AI as a “suitcase word”—a term into which you can stuff whatever you want—and that still seems to be the case. We are comfortable with that because we think companies should use all the capabilities of more traditional analysis while increasing automation in strategy that can free up management or analyst time and, gradually, introducing tools that can augment human thinking.
Joanna Pachner: AI has been embraced by many business functions, but strategy seems to be largely immune to its charms. Why do you think that is?
Subscribe to the Inside the Strategy Room podcast
Yuval Atsmon: You’re right about the limited adoption. Only 7 percent of respondents to our survey about the use of AI say they use it in strategy or even financial planning, whereas in areas like marketing, supply chain, and service operations, it’s 25 or 30 percent. One reason adoption is lagging is that strategy is one of the most integrative conceptual practices. When executives think about strategy automation, many are looking too far ahead—at AI capabilities that would decide, in place of the business leader, what the right strategy is. They are missing opportunities to use AI in the building blocks of strategy that could significantly improve outcomes.
I like to use the analogy to virtual assistants. Many of us use Alexa or Siri but very few people use these tools to do more than dictate a text message or shut off the lights. We don’t feel comfortable with the technology’s ability to understand the context in more sophisticated applications. AI in strategy is similar: it’s hard for AI to know everything an executive knows, but it can help executives with certain tasks.
When executives think about strategy automation, many are looking too far ahead—at AI deciding the right strategy. They are missing opportunities to use AI in the building blocks of strategy.
Joanna Pachner: What kind of tasks can AI help strategists execute today?
Yuval Atsmon: We talk about six stages of AI development. The earliest is simple analytics, which we refer to as descriptive intelligence. Companies use dashboards for competitive analysis or to study performance in different parts of the business that are automatically updated. Some have interactive capabilities for refinement and testing.
The second level is diagnostic intelligence, which is the ability to look backward at the business and understand root causes and drivers of performance. The level after that is predictive intelligence: being able to anticipate certain scenarios or options and the value of things in the future based on momentum from the past as well as signals picked in the market. Both diagnostics and prediction are areas that AI can greatly improve today. The tools can augment executives’ analysis and become areas where you develop capabilities. For example, on diagnostic intelligence, you can organize your portfolio into segments to understand granularly where performance is coming from and do it in a much more continuous way than analysts could. You can try 20 different ways in an hour versus deploying one hundred analysts to tackle the problem.
Predictive AI is both more difficult and more risky. Executives shouldn’t fully rely on predictive AI, but it provides another systematic viewpoint in the room. Because strategic decisions have significant consequences, a key consideration is to use AI transparently in the sense of understanding why it is making a certain prediction and what extrapolations it is making from which information. You can then assess if you trust the prediction or not. You can even use AI to track the evolution of the assumptions for that prediction.
Those are the levels available today. The next three levels will take time to develop. There are some early examples of AI advising actions for executives’ consideration that would be value-creating based on the analysis. From there, you go to delegating certain decision authority to AI, with constraints and supervision. Eventually, there is the point where fully autonomous AI analyzes and decides with no human interaction.
Because strategic decisions have significant consequences, you need to understand why AI is making a certain prediction and what extrapolations it’s making from which information.
Joanna Pachner: What kind of businesses or industries could gain the greatest benefits from embracing AI at its current level of sophistication?
Yuval Atsmon: Every business probably has some opportunity to use AI more than it does today. The first thing to look at is the availability of data. Do you have performance data that can be organized in a systematic way? Companies that have deep data on their portfolios down to business line, SKU, inventory, and raw ingredients have the biggest opportunities to use machines to gain granular insights that humans could not.
Companies whose strategies rely on a few big decisions with limited data would get less from AI. Likewise, those facing a lot of volatility and vulnerability to external events would benefit less than companies with controlled and systematic portfolios, although they could deploy AI to better predict those external events and identify what they can and cannot control.
Third, the velocity of decisions matters. Most companies develop strategies every three to five years, which then become annual budgets. If you think about strategy in that way, the role of AI is relatively limited other than potentially accelerating analyses that are inputs into the strategy. However, some companies regularly revisit big decisions they made based on assumptions about the world that may have since changed, affecting the projected ROI of initiatives. Such shifts would affect how you deploy talent and executive time, how you spend money and focus sales efforts, and AI can be valuable in guiding that. The value of AI is even bigger when you can make decisions close to the time of deploying resources, because AI can signal that your previous assumptions have changed from when you made your plan.
Joanna Pachner: Can you provide any examples of companies employing AI to address specific strategic challenges?
Yuval Atsmon: Some of the most innovative users of AI, not coincidentally, are AI- and digital-native companies. Some of these companies have seen massive benefits from AI and have increased its usage in other areas of the business. One mobility player adjusts its financial planning based on pricing patterns it observes in the market. Its business has relatively high flexibility to demand but less so to supply, so the company uses AI to continuously signal back when pricing dynamics are trending in a way that would affect profitability or where demand is rising. This allows the company to quickly react to create more capacity because its profitability is highly sensitive to keeping demand and supply in equilibrium.
Joanna Pachner: Given how quickly things change today, doesn’t AI seem to be more a tactical than a strategic tool, providing time-sensitive input on isolated elements of strategy?
Yuval Atsmon: It’s interesting that you make the distinction between strategic and tactical. Of course, every decision can be broken down into smaller ones, and where AI can be affordably used in strategy today is for building blocks of the strategy. It might feel tactical, but it can make a massive difference. One of the world’s leading investment firms, for example, has started to use AI to scan for certain patterns rather than scanning individual companies directly. AI looks for consumer mobile usage that suggests a company’s technology is catching on quickly, giving the firm an opportunity to invest in that company before others do. That created a significant strategic edge for them, even though the tool itself may be relatively tactical.
Joanna Pachner: McKinsey has written a lot about cognitive biases and social dynamics that can skew decision making. Can AI help with these challenges?
Yuval Atsmon: When we talk to executives about using AI in strategy development, the first reaction we get is, “Those are really big decisions; what if AI gets them wrong?” The first answer is that humans also get them wrong—a lot. [Amos] Tversky, [Daniel] Kahneman, and others have proven that some of those errors are systemic, observable, and predictable. The first thing AI can do is spot situations likely to give rise to biases. For example, imagine that AI is listening in on a strategy session where the CEO proposes something and everyone says “Aye” without debate and discussion. AI could inform the room, “We might have a sunflower bias here,” which could trigger more conversation and remind the CEO that it’s in their own interest to encourage some devil’s advocacy.
We also often see confirmation bias, where people focus their analysis on proving the wisdom of what they already want to do, as opposed to looking for a fact-based reality. Just having AI perform a default analysis that doesn’t aim to satisfy the boss is useful, and the team can then try to understand why that is different than the management hypothesis, triggering a much richer debate.
In terms of social dynamics, agency problems can create conflicts of interest. Every business unit [BU] leader thinks that their BU should get the most resources and will deliver the most value, or at least they feel they should advocate for their business. AI provides a neutral way based on systematic data to manage those debates. It’s also useful for executives with decision authority, since we all know that short-term pressures and the need to make the quarterly and annual numbers lead people to make different decisions on the 31st of December than they do on January 1st or October 1st. Like the story of Ulysses and the sirens, you can use AI to remind you that you wanted something different three months earlier. The CEO still decides; AI can just provide that extra nudge.
Joanna Pachner: It’s like you have Spock next to you, who is dispassionate and purely analytical.
Yuval Atsmon: That is not a bad analogy—for Star Trek fans anyway.
Joanna Pachner: Do you have a favorite application of AI in strategy?
Yuval Atsmon: I have worked a lot on resource allocation, and one of the challenges, which we call the hockey stick phenomenon, is that executives are always overly optimistic about what will happen. They know that resource allocation will inevitably be defined by what you believe about the future, not necessarily by past performance. AI can provide an objective prediction of performance starting from a default momentum case: based on everything that happened in the past and some indicators about the future, what is the forecast of performance if we do nothing? This is before we say, “But I will hire these people and develop this new product and improve my marketing”— things that every executive thinks will help them overdeliver relative to the past. The neutral momentum case, which AI can calculate in a cold, Spock-like manner, can change the dynamics of the resource allocation discussion. It’s a form of predictive intelligence accessible today and while it’s not meant to be definitive, it provides a basis for better decisions.
Joanna Pachner: Do you see access to technology talent as one of the obstacles to the adoption of AI in strategy, especially at large companies?
Yuval Atsmon: I would make a distinction. If you mean machine-learning and data science talent or software engineers who build the digital tools, they are definitely not easy to get. However, companies can increasingly use platforms that provide access to AI tools and require less from individual companies. Also, this domain of strategy is exciting—it’s cutting-edge, so it’s probably easier to get technology talent for that than it might be for manufacturing work.
The bigger challenge, ironically, is finding strategists or people with business expertise to contribute to the effort. You will not solve strategy problems with AI without the involvement of people who understand the customer experience and what you are trying to achieve. Those who know best, like senior executives, don’t have time to be product managers for the AI team. An even bigger constraint is that, in some cases, you are asking people to get involved in an initiative that may make their jobs less important. There could be plenty of opportunities for incorporating AI into existing jobs, but it’s something companies need to reflect on. The best approach may be to create a digital factory where a different team tests and builds AI applications, with oversight from senior stakeholders.
The big challenge is finding strategists to contribute to the AI effort. You are asking people to get involved in an initiative that may make their jobs less important.
Joanna Pachner: Do you think this worry about job security and the potential that AI will automate strategy is realistic?
Yuval Atsmon: The question of whether AI will replace human judgment and put humanity out of its job is a big one that I would leave for other experts.
The pertinent question is shorter-term automation. Because of its complexity, strategy would be one of the later domains to be affected by automation, but we are seeing it in many other domains. However, the trend for more than two hundred years has been that automation creates new jobs, although ones requiring different skills. That doesn’t take away the fear some people have of a machine exposing their mistakes or doing their job better than they do it.
Joanna Pachner: We recently published an article about strategic courage in an age of volatility that talked about three types of edge business leaders need to develop. One of them is an edge in insights. Do you think AI has a role to play in furnishing a proprietary insight edge?
Yuval Atsmon: One of the challenges most strategists face is the overwhelming complexity of the world we operate in—the number of unknowns, the information overload. At one level, it may seem that AI will provide another layer of complexity. In reality, it can be a sharp knife that cuts through some of the clutter. The question to ask is, Can AI simplify my life by giving me sharper, more timely insights more easily?
Joanna Pachner: You have been working in strategy for a long time. What sparked your interest in exploring this intersection of strategy and new technology?
Yuval Atsmon: I have always been intrigued by things at the boundaries of what seems possible. Science fiction writer Arthur C. Clarke’s second law is that to discover the limits of the possible, you have to venture a little past them into the impossible, and I find that particularly alluring in this arena.
AI in strategy is in very nascent stages but could be very consequential for companies and for the profession. For a top executive, strategic decisions are the biggest way to influence the business, other than maybe building the top team, and it is amazing how little technology is leveraged in that process today. It’s conceivable that competitive advantage will increasingly rest in having executives who know how to apply AI well. In some domains, like investment, that is already happening, and the difference in returns can be staggering. I find helping companies be part of that evolution very exciting.
Explore a career with us
Related articles.

Strategic courage in an age of volatility
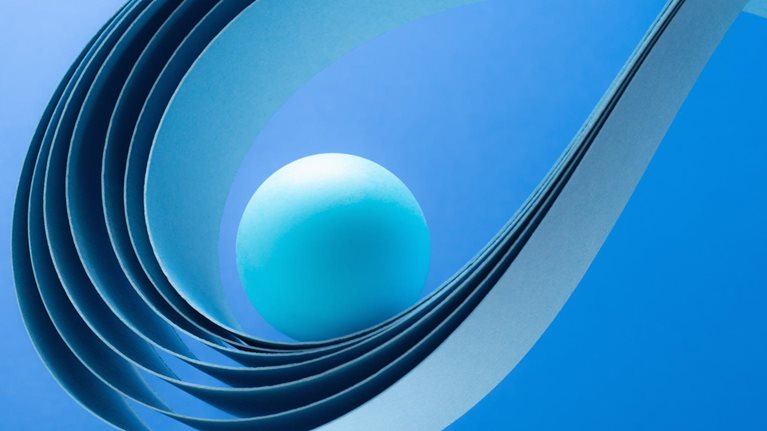
Bias Busters Collection

IMAGES
VIDEO
COMMENTS
Table of contents. What not to include in your discussion section. Step 1: Summarize your key findings. Step 2: Give your interpretations. Step 3: Discuss the implications. Step 4: Acknowledge the limitations. Step 5: Share your recommendations. Discussion section example. Other interesting articles.
Begin with a clear statement of the principal findings. This will reinforce the main take-away for the reader and set up the rest of the discussion. Explain why the outcomes of your study are important to the reader. Discuss the implications of your findings realistically based on previous literature, highlighting both the strengths and ...
The Discussion chapter brings an opportunity to write an academic argument that contains a detailed critical evaluation and analysis of your research findings. This chapter addresses the purpose and critical nature of the discussion, contains a guide to selecting key results to discuss, and details how best to structure the discussion with ...
The discussion section is often considered the most important part of your research paper because it: Most effectively demonstrates your ability as a researcher to think critically about an issue, to develop creative solutions to problems based upon a logical synthesis of the findings, and to formulate a deeper, more profound understanding of the research problem under investigation;
if you write a scientific dissertation, or anyway using quantitative methods, you will have some objective results that you will present in the Results chapter. You will then interpret the results in the Discussion chapter. B) More common for qualitative methods. - Analysis chapter. This can have more descriptive/thematic subheadings.
The discussion section provides an analysis and interpretation of the findings, compares them with previous studies, identifies limitations, and suggests future directions for research. This section combines information from the preceding parts of your paper into a coherent story. By this point, the reader already knows why you did your study ...
Step 1: Restate your research problem and research questions. The first step in writing up your discussion chapter is to remind your reader of your research problem, as well as your research aim (s) and research questions. If you have hypotheses, you can also briefly mention these.
present the findings of your research by 128 COMPLETING YOUR QUALITATIVE DISSERTATION 05-Bloomberg-45467.qxd 12/26/2007 11:59 AM Page 128. ... by way of structuring our discussion accord-ing to three interrelated activities: (a) seeking significant patterns among the findings,
The discussion chapter is a detailed consideration of how your findings answer your research questions. This includes the use of secondary literature to help contextualise your discussion. Rather than considering the findings in detail, the conclusion briefly summarises and synthesises the main findings of your study before bringing the ...
Accordingly, thematic analysis can result in a theory-driven or data-driven set of findings and engage a range of research questions (Braun & Clarke, 2006). Second, thematic analysis engages with analytic practices that are fairly common with other approaches to qualitative analysis. ... For instance, in Terry et al.'s (2017) discussion of ...
Chapter 4 Key Findings and Discussion. This chapter presents principal findings from the primary research. The findings can be. divided into two groups: qualitative and quantitative results. Figure 4.1 illustrates how. these two types of results are integrated.
These formats are supported by research that focused on improved understanding of the information they intend to convey (Carrasco-Labra et al 2016, Langendam et al 2016, Santesso et al 2016). ... Summary of findings ... Chapter 8 provides a discussion of study-level assessments of risk of bias in the context of a Cochrane Review, and proposes ...
Don't make the reader do the analytic work for you. Now, on to some specific ways to structure your findings section. 1). Tables. Tables can be used to give an overview of what you're about to present in your findings, including the themes, some supporting evidence, and the meaning/explanation of the theme.
The PSP focus group discussion guide contained questions to assess participants' past experiences with receiving information about research findings; identify participant preferences for receiving research findings whether negative, positive, or equivocal; gather information to improve communication of research results back to participants ...
The discussion chapters form the heart of your thesis and this is where your unique contribution comes to the forefront. This is where your data takes centre-stage and where you get to showcase your original arguments, perspectives and knowledge. To do this effectively needs you to explore the original themes and issues arising from and within ...
Discussion: This section interprets the results and explains what they mean in relation to the research question(s) and hypotheses. It may also compare and contrast the current findings with previous research studies and explore any implications or limitations of the study. ... Research findings are the result of a systematic and rigorous ...
The purpose of this chapter is to discuss and conceptualise the research findings, then to position the emergent theory, ... of professional experience that is illustrated by Fig. 6.1 and in detail by the concept map in in Appendix 7 and the discussion of findings in Chap. 5. ... According to this classification of competency frameworks, the ...
The middle sections of the research paper continue the narrow focus of the specific research being reported in the Methods and Results. This is pictured as the narrow central area of the hourglass. The Discussion usually begins with comments about the specific research and progressively connects the findings to the wider literature in the field.
The Discussion section is an important part of the research manuscript. that allows the authors to showcase the study. It is used to interpret. the results for readers, describe the virtues and ...
Table of contents. What not to include in your discussion section. Step 1: Summarise your key findings. Step 2: Give your interpretations. Step 3: Discuss the implications. Step 4: Acknowledge the limitations. Step 5: Share your recommendations. Discussion section example.
The Discussion section of a research paper is an essential part of any study, as it allows the author to interpret their results and contextualize their findings. To write an effective Discussion section, authors should focus on the relevance of their research, highlight the limitations, introduce new discoveries, highlight their observations ...
The findings are presented below in figure 4.2 where Accountants represents. 15.4%, IT administrator 7.7%, Internal Auditor 6.2%, Customer 68.5% and Social. W elfare Evaluator represents 2.3% ...
Qualitative data analysis is. concerned with transforming raw data by searching, evaluating, recogni sing, cod ing, mapping, exploring and describing patterns, trends, themes an d categories in ...
To date, Very few published studies investigate integrated approaches to care that involve primary care and palliative care services, and consider inequalities. 14,26-28 The findings of this study are in keeping with the findings of this limited previous research which suggest poor identification of patients with palliative care needs and ...
These visual aids are essential in conveying the significance of the research findings to your readers and stakeholders. Table 11 Classification accuracy comparison of proposed and state-of-the ...
Grouping of ICD-10 Diagnostic Codes Used to Approximate the International Childhood Cancer Classification. ... Centre for Research in Epidemiology and Statistics, French National Institute for Health and Medical Research ... Findings In this cohort study of 8 526 306 children, the overall risk of cancer did not differ among children born after ...
Keywords: Phytoprotection, Deep Learning, Plant Recognition, Species Classification, Conservation . Important Note: All contributions to this Research Topic must be within the scope of the section and journal to which they are submitted, as defined in their mission statements.Frontiers reserves the right to guide an out-of-scope manuscript to a more suitable section or journal at any stage of ...
Research Design. We employed an ethnography design to examine the EGs and EPs of the biology curricula. Ethnography comprehensively explores the historical, cultural, and political aspects of knowledge evident in the educational traditions and practices of the countries under study (Hout, 2004).It involves systematically observing individuals, locations, concepts, written records, and ...
Marvin Minsky, the pioneer of artificial intelligence research in the 1960s, talked about AI as a "suitcase word"—a term into which you can stuff whatever you want—and that still seems to be the case. ... is listening in on a strategy session where the CEO proposes something and everyone says "Aye" without debate and discussion. AI ...
The US real estate market is a complex ecosystem influenced by multiple factors, making it critical for stakeholders to understand its dynamics. This study uses Zillow Econ (monthly) data from January 2018 to October 2023 across 100 major regions gathered through Metropolitan Statistical Area (MSA) and advanced machine learning techniques, including radial kernel Support Vector Machines (SVMs ...