Harvard Dataverse
Harvard Dataverse is an online data repository where you can share, preserve, cite, explore, and analyze research data. It is open to all researchers, both inside and out of the Harvard community.
Harvard Dataverse provides access to a rich array of datasets to support your research. It offers advanced searching and text mining in over 2,000 dataverses, 75,000 datasets, and 350,000+ files, representing institutions, groups, and individuals at Harvard and beyond.
Explore Harvard Dataverse
The Harvard Dataverse repository runs on the open-source web application Dataverse , developed at the Institute for Quantitative Social Science . Dataverse helps make your data available to others, and allows you to replicate others' work more easily. Researchers, journals, data authors, publishers, data distributors, and affiliated institutions all receive academic credit and web visibility.

Why Create a Personal Dataverse?
- Easy set up
- Display your data on your personal website
- Brand it uniquely as your research program
- Makes your data more discoverable to the research community
- Satisfies data management plans
Terms to know
- A Dataverse repository is the software installation, which then hosts multiple virtual archives called dataverses .
- Each dataverse contains datasets, and each dataset contains descriptive metadata and data files (including documentation and code that accompany the data).
- As an organizing method, dataverses may also contain other dataverses.
Related Services and Tools
Research data services, qualitative research support.
Share your research data
Mendeley Data is a free and secure cloud-based communal repository where you can store your data, ensuring it is easy to share, access and cite, wherever you are.
Find out more about our institutional offering, Digital Commons Data
Search the repository
Recently published.
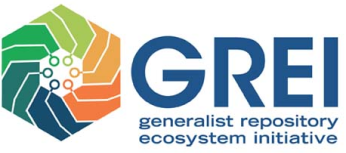
The Generalist Repository Ecosystem Initiative
Elsevier's Mendeley Data repository is a participating member of the National Institutes of Health (NIH) Office of Data Science Strategy (ODSS) GREI project. The GREI includes seven established generalist repositories funded by the NIH to work together to establish consistent metadata, develop use cases for data sharing, train and educate researchers on FAIR data and the importance of data sharing, and more.
Why use Mendeley Data?
The Mendeley Data communal data repository is powered by Digital Commons Data.
Digital Commons Data provides everything that your institution will need to launch and maintain a successful Research Data Management program at scale.
Data Monitor provides visibility on an institution's entire research data output by harvesting research data from 2000+ generalist and domain-specific repositories, including everything in Mendeley Data.

New re3data.org Editorial Board Members
The re3data Editorial Board is pleased to welcome seven new members: Dalal Hakim Rahme, Coordinator of Content Curation, United Nations Rene Faustino Gabriel Junior, Professor, Universidade Federal do Rio Grando do Sul Sandra Gisela Martín, Library...
re3data Call for Editorial Board
re3data Call for Editorial Board The re3data.org registry has been in operation for over 10 years and provides a curated index of over 3,000 research data repositories around the world from all disciplines. New repositories are identified and reviewed by an...
Releasing version 4.0 of the re3data Metadata Schema
re3data caters to a range of needs for diverse stakeholders (Vierkant et al., 2021). Detailed and precise repository descriptions are at the heart of the majority of these use cases, and repository metadata are given special attention at the re3data ...
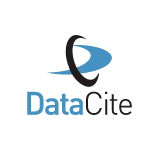
Helmholtz Open Science Office

Humbold-Universität zu Berlin
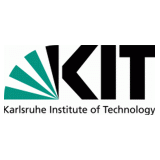
Karlsruher Institut für Technologie

Purdue University Libraries
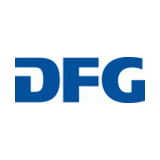
Deutsche Forschungsgemeinschaft
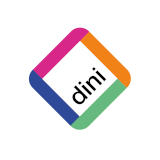
Deutsche Initiative für Netzwerkinformation

FAIRsharing
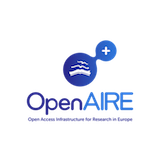
CoreTrustSeal
- Legal notice / Impressum
- Terms of Use & Privacy Policy
- Current projects
- EOSC FAIR-IMPACT
- re3data COREF

- Cite this service: re3data.org - Registry of Research Data Repositories. https://doi.org/10.17616/R3D last accessed: 2024-06-20
Data Repositories
- Harvard Dataverse
- IEEE DataPort
- Mendeley Data
- Open Science Framework
- Science Data Bank
- NIH and NCBI Repositories
- Manuscript Repositories
A key aspect of data sharing involves not only posting or publishing research articles on preprint servers or in scientific journals, but also making public the data, code, and materials that support the research. Data repositories are a centralized place to hold data, share data publicly, and organize data in a logical manner.
Benefits of Data Repositories
- manage your data
- organize and deposit your data
- cite your data by supplying a persistent identifier
- facilitate discovery of your data
- make your data more valuable for current and future research
- preserve your data for the long-run
Repository Comparison Grid
The number of available resources for data sharing and data publication has increased substantially in recent years. You can search the re3data.org global registry of research data repositories to find appropriate academic discipline repositories.
We have also created a resource to compare and contrast several of the general data repositories currently available for NIH and biomedical science researchers. Detailed feature descriptions of each platform are available on the subpages of this page.
Click on the Harvard Biomedical Repository Matrix below to view an enlarged version of the table on Zenodo.
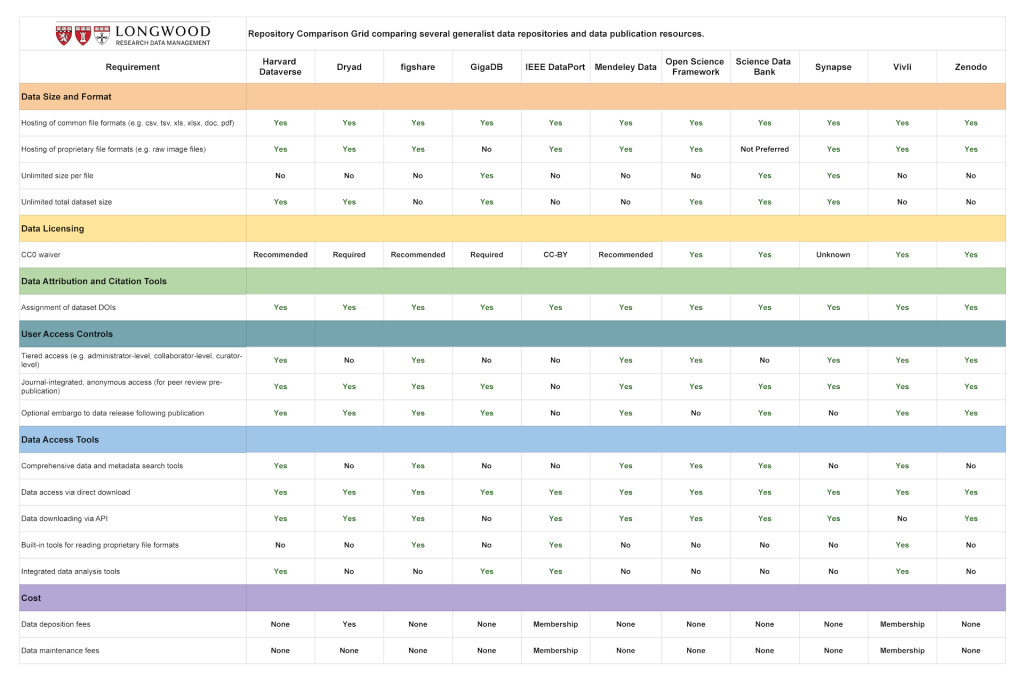
Accessible Repository Comparison
Repository comparison list.
A PDF version of this information is also available on Zenodo.
Harvard Dataverse Features & Specifications
Data size and format, hosting of common file formats (e.g. csv, tsv, xls, xlsx, doc, pdf).
- All file formats accepted (tabular, non-tabular, and compressed as a zip file bundle with file hierarchy feature to preserve directory structure).
Hosting of proprietary file formats (e.g. raw image files)
Unlimited size per file.
- To use the browser-based upload function, file can’t exceed 2.5GB. However, Harvard Dataverse is willing to work with Harvard researchers who have larger files.
Unlimited total dataset size
- 1TB per researcher. Harvard Dataverse will work with Harvard researchers who have larger datasets (>1 TB).
Data Licensing
- Recommended
- Harvard Dataverse strongly encourages use of a Creative Commons Zero (CC0) waiver for all public datasets, but dataset owners can specify other terms of use and restrict access to data.
Data Attribution and Citation Tools
Assignment of dataset dois.
- Harvard Dataverse assigns a DOI to each dataset and datafile within a dataverse.
- Dataset authors can identify themselves and other types of data contributors using the following types of unique IDs: ORCID, ISNI, LCNA, VIAF, GND, DAI, ResearcherID, Scopus ID.
User Access Controls
Tiered access (e.g. administrator-level, collaborator-level, curator-level).
- Harvard Dataverse allows draft, unpublished, and published (public) datasets. For draft and unpublished datasets, a variety of tiers of access can be assigned to different registered users.
Journal-integrated, anonymous access (for peer review pre-publication)
- The Harvard Dataverse Repository offers open access, restricted, and embargo options for all files, along with the ability to apply standard licenses and add custom terms of data access.
Optional embargo to data release following publication
Data access tools, comprehensive data and metadata search tools.
- Without logging in, users can browse a Dataverse installation and search for Dataverse collections, datasets, and files, view dataset descriptions and files for published datasets, and subset, analyze, and visualize data for published (restricted & not restricted) data files.
Data access via direct download
Data downloading via api.
- In addition to individual file downloading, Harvard Dataverse has multiple APIs for programmatic data and metadata access, as described in the Dataverse API Guide
Built-in tools for reading proprietary file formats
Integrated data analysis tools.
- Harvard Dataverse includes external tools that provide additional features that are not part of the Dataverse Software itself, such as data file previews, visualization, and curation.
Data deposition fees
- Harvard Dataverse Repository is free for all researchers worldwide (up to 1 TB).
Data maintenance fees
Dryad features & specifications.
- Prefers all data be submitted in non-proprietary, openly-documented formats that are preservation-friendly. Will accept other file types if they are "community-accepted" format. Do not accept submissions with personally identifiable information.
- No specified amount; no limit on storage space per researcher.
- 300GB/dataset
- Creative Commons Zero (CC0) Any data submitted will be published under the CC0 license. Does not currently support any other license types, nor allow for restrictions on data access or use.
- Each dataset published receives a DOI for the data submission as a whole. A suffix is added to the DOI when a data file or dataset is revised to enable version control.
- Can be shared anonymously and securely with editors and reviewers at the subset of journals that have integrated repository into their editorial workflow.
- Machine Readable metadata, primarily consists of keywords and information about the associated publication. Detailed, file-associated metadata are not records and thus not searchable. Uses the DataCite metadata schema.
- All datasets are free to download when published. Individual file downloading, multiple API's for programmatic data and metadata access.
- $120 data publishing charge unless funded by the institution, publishers or funders. Users can download datasets free of charge once they are published.
figshare Features & Specifications
- All file types (both compressed and non-compressed formats) are accepted. Some file types are rendered in the browser page. Files are tagged as follows: figures, datasets, media, code, paper, thesis, poster, presentation, and fileset.
- System-wide limit of 5TB per file.
- 20GB of private data files. Figshare+ (designed for larger datasets) offers storage in stages beginning with 100GB up to over 10TB per dataset. No limit on storage space per researcher.
- Code and software licenses include MIT, GPL-3.0, or Apache-2.0.
- All other files: CC-BY. These licensing rules apply to all individuals uploading files directly to figshare. Institutions and publishers are allowed to mandate alternative licenses.
- figshare assigns a DOI to each individual file at the point of publication. Authors can also add an ORCID ID, so all items are pushed to ORCID. Related files can be aggregated under a master DOI encompassing a Collection. A suffix is added to a file-level DOI when a file is revised or replaced to enable version control.
- Before the dataset is released publicly, a user can share it with a private sharing link or through a project/collaboration group.
- Publishers who have integrated their editorial workflow with figshare may have additional user access controls enabled to allow the journal's editors and reviewers to have anonymous and secure access to files before they are made public.
- Free-text search functionality is provided. Limited metadata, which consist of keywords and information about any associated publication, are recorded.
- Detailed, file-associated metadata are not recorded in the general-use figshare repository and thus are not searchable, but institutional figshare instances are allowed to develop custom metadata standards.
- In addition to individual file downloading, figshare has an API for programmatic data and metadata access.
- Figshare+ has a one-time fee based on the size of the dataset requested.
GigaDB Features & Specifications
- Only non-proprietary file types are accepted.
- An appropriate Open-Source Initiative (OSI) or other open-source license can be applied to software files, workflows, and virtual machines.
- Each dataset will be assigned a DOI that can be used as a citation in future articles and publications. No files present at the time of publication can be removed, but a versioning system allows authors to add new files after publication if needed. Detailed information about the data should be submitted by the authors in ISA-Tab.
- Through the GigaDB staging server, the journal's editors and reviewers have anonymous and secure access to files before they are made public, and authors can submit revised files during the peer-review process.
- Free-text search functionality is provided. Detailed, file-associated metadata are not recorded and thus are not searchable.
- Datasets may be downloaded via FTP or via a browser using GigaDB's Aspera server software. For larger datasets, GigaScience will copy data to a hard drive and ship it to a user ( at the user's expense ).
- Author-provided tools are hosted in GigaDB or on the GigaGalaxy server and linked to from the associated paper's GigaScience landing page.
- Data deposition costs for up to 1 TB of data are included in the standard article publication charge. All data provided by GigaDB is free to download and use.
IEEE DataPort Features & Specifications
- The following formats are currently supported: ZIP, GZ, gzip, CSV, JSON, TXT, SQL, XML, TSV, EBS, Avro, ORC, Parquet, HDF5, 7z, TBZ2, ISO, tar, BZ2, Z, XLS, XLSX, graph, properties, offsets, FLAC, OGG, WAV, AAC, MP3, GIF, JPG, JPEG, PNG, AVI, MOV, MP4, MPG, M4V, YAML, DAT, MAT, fig.
- No individual file size limit. For large datasets, upload a series of files that are 100 GB or less. For datasets with a large number of files (>100), compress your upload(s) using the ZIP and/or GZIP format.
- Up to 2TB of storage or 10 TB for Institutional Subscribers for each dataset.
- Datasets on IEEE DataPort are made available under Creative Commons Attribution (CC-BY) licenses which require attribution.
- Datasets on IEEE DataPort are made available under CC-BY licenses which require attribution. Any use requires citation. IEEE DataPort includes a Cite button on each dataset page so a user can easily obtain the proper citation for the dataset. The citation provided by using the Cite button is provided in multiple formats to facilitate easy attribution.
- IEEE DataPort is a self-monitoring system with feedback mechanisms so users can provide comments on datasets.
- To search, access, and analyze datasets on IEEE DataPort, you first need to create a free IEEE account or login with your existing account. After logging in you can search datasets by entering keywords in the search bar or by browsing the dataset categories. Once a desired dataset is located you can access it by simply clicking on the dataset.
- Users need to subscribe to access and/or download Standard datasets on IEEE DataPort. Open Access datasets are available to all registered users of IEEE DataPort.
- IEEE DataPort is data agnostic and will allow any format of dataset to be submitted for storage on IEEE DataPort. The provision of metadata, tools and/or supporting documentation allow the user to understand how each specific dataset can be analyzed.
- You can then view and analyze the dataset. If you do not have sufficient storage and computing capacity on your local system to perform the analysis, IEEE currently provides access to the dataset in the Cloud to facilitate the analysis. Once your analysis is complete, you can upload the analysis by clicking the “submit an analysis” button directly below the dataset image.
- An individual subscription allows you to view, download and/or access in the cloud all datasets, store your own research data at no cost, and access data management features. Individual subscriptions are free for all IEEE Society Members or $40/month.
Mendeley Data Features & Specifications
- 10GB per dataset
- Mendeley Data datasets for personal accounts have a maximum limit of 10 GB per dataset. However, if your Institution subscribes to Mendeley Data you will have the ability to create datasets up to a maximum size of 100GB. The maximum size will depend on the storage agreement that your institution has.
- Users can choose from a range of 16 licenses that can be applied to data, with the default being Creative Commons Zero (CC0) .
- Mendeley Data reserves a DOI when the dataset is created and mints it when the dataset is published.
- Mendeley Data provides PIDs for individual files and folders within a dataset.
- Each draft dataset has a share link which you can copy to send to collaborators; they’ll be able to access the dataset metadata and files prior to publishing.
- When publishing a dataset, a user may choose to defer the date at which the data becomes available (for example, so that it is available at the same time as an associated article).
- You can search for datasets by keyword, within dataset metadata and data files. You can perform an advanced search using field codes to target one or more specific fields and / or boolean operators.
- If the dataset owner has decided to allow the download of datasets, this can be done freely and only the download count is tracked.
- You can download all the files within the dataset using the “Download All” button in a Published dataset, which will give you the file size before you start the download and create a zip file with the Dataset name and version. You can see the same structure of the dataset including all the subfolders, within the zip file.
- The communal repository is available free of charge for individual researchers who want to publish relatively small datasets (up to 10GB each).
Open Science Framework (OSF) Features & Specifications
- Any type of file can be uploaded. Most files will render directly in the File Viewer. For example, if the file is an image, you can zoom in and out on details.
- 5GB/file upload limit for native OSF Storage. There is no limit imposed by OSF for the amount of storage used across add-ons connected to a given project.
- Users can choose from a long list of licenses. Users can also define a license in a .txt file and uploads to the project.
- OSF uses Globally Unique Identifiers (GUIDs) on all objects (users, files, projects, components, registrations, and preprints) across the platform, which are citable in scholarly communication. OSF also supports registration of DOIs for projects, components, and research registrations with DataCite, and for preprints with Crossref. OSF collects ORCID iDs for users and contributors, and provides those with metadata sent for DOI minting, as well as ROR identifiers when contributor affiliations are known.
- OSF does not currently support DOI versioning.
- OSF supports request access and private sharing settings, as well as view only link with ability to anonymize contributor list.
- Contributors are a group of collaborators within a project, component, registration, or preprint. Projects and components have individual contributor lists and permissions levels, so you can control who can access and modify your work.
- You can create a view-only link to share projects so those who have the link can view—but not edit—the project, and also the anonymize contributor list (e.g., for peer review).
- Free Text Search functionality provided. The OSF Search interface offers a few options for filtering search results. Tags are automatically indexed by search for public content.
- Access to view and download public content on OSF is free and does not require an account. You can download individual or multiple Quick Files to save and view them on your computer.
- Files stored on OSF can also be downloaded locally through the API.
- OSF is free to use by research producers and consumers. Signing up for an account on OSF is quick and easy, by providing a name, email, and password, or by using ORCID or institutional credentials (including Harvard Key).
Science Data Bank (ScienceDB) Features & Specifications
- They prefer non-proprietary file formats, which can be found in the Science Data Bank Preferred File Format table .
- Not preferred
- CC0 is the default license assigned to datasets.
- ScienceDB provides the following licensing options: CC0, CC-BY 4.0, CC BY-SA 4.0, CC BY-NC 4.0, CC BY-NC-SA 4.0, CC BY-ND 4.0, CC BY-NC-ND 4.0, and 3 licenses for database: PDDL, ODC-By, ODbL, as well as 12 software license agreements: MIT, Apache-2.0, AGPL-3.0, LGPL-2.1, GPL-2.0, GPL-3.0, BSD-2-Clause, BSD-3-Clause, MPL-2.0, BSL-1.0, EPL-2.0 and The Unlicense. For more information, see the Science Data Bank FAQ webpage.
- Once a submission is published, ScienceDB assigns a DOI to each dataset. A Commons Science and Technology Resource (CSTR) is also assigned to accepted datasets. Data depositors can select their preferred data citation format.
- At the time of data submission, users may select to an open access or embargo option. Files can be restricted to require users to request access through a Data Access Application.
- OPEN-API allows users to access datasets programmatically. All metadata is harvested via OAI-PMH.
- Currently, submitting data to ScienceDB is free. However, the Science Data Bank FAQ webpage states that they reserve the right to charge for submission, review, or storage in the future.
Synapse Features & Specifications
- Conditions for use are put in place to define/restrict how users who have permission to download data may use it.
- Conditions for use may include IRB approval or other restrictions that you define as the data contributor.
- You are responsible for determining if the data you would like to contribute is controlled data and therefore requires conditions for use.
- Conditions for use can be set at the project, folder, file and table level.
- Synapse items receive a unique identifier (SynID). Items that receive a SynID are files, folders, projects, tables, views, wikis, links, and Docker repositories. Each file also receives a MD5 checksum. DOIs are available in Synapse for projects, files, folders, tables, and views.
- There are a number of Synapse user access controls for public and private projects, and sharing settings can be managed at the project, folder, file, and table level. Data containing sensitive information (i.e., data with a de-identification risk) can be restricted to specific users; users wanting access to controlled data can request individual access. Project administrators can add and manage permissions for individual team members on private projects. Public projects can either be fully public or restricted to registered Synapse Users.
- Users are required to have a Synapse account and become a certified user in order to upload data. Data can be uploaded and downloaded via a web-based user interface or programmatically using Python, R, or the command line.
- Intended for individual researchers wanting to share small datasets (<100GB) for publication (including creating DOIs) and scientific, educational, and research collaborations.
Vivli Features & Specifications
- Data sets are not required to be standardized; however, we highly recommend that data sets be made available in CDISC SDTM (Standard Data Tabulation Model) format to support the most efficient data aggregation, re-use, and sharing. In the future, Vivli will explore the use of Common Data Elements within specific clinical domains.
- Vivli data contributors can share files up to 500 MB. Larger sizes of up to 100T can be accommodated. If your file is larger than 1T, please email Vivli Support .
- There is no limit per researcher, and each data contribution is reviewed by Vivli.
- All data contributors must sign and conform to the Data Contributor Agreement (available upon request), which includes language about intellectual property.
- All clinical research that is available for search and request on the Vivli platform is assigned a DataCite Digital Object Identifier (DOI) at the time the metadata for the clinical research data appears in the Vivli search and is available for request.
- The clinical research dataset is assigned a main DOI with a parent-child data object reference for all data and documents associated with a study’s data package to support data discovery.
- Datasets contributed to Vivli may be made available via various levels of access through download after signing of a Data Use Agreement.
- The Vivli platform allows users to search through listed studies using three search methods, including a Keyword Search, PICO Search, and Quick Study Look-up.
- To request data, a researcher or team must first create an account on Vivli and submit a research proposal.
- Yes, through Vivli’s secure research environment.
- Use robust analytical tools to combine and analyze multiple datasets. Vivli’s secure research environment is a virtual work-space within the Vivli platform where researchers who have been provided access to data, will have remote desktop access to conduct their analysis. Researchers will have access to SAS , STATA , R , Python , Jupyter , and the Microsoft Office suite to enable analysis of shared data sets. If desired, additional analytical tools, data and scripts may be included in a research team’s secure research environment.
- Access to metadata and data hosted by Vivli is free and accessible to all, subject to meeting a data contributor’s data sharing policies.
- If your academic institution is a member of Vivli there is no cost to deposit data in Vivli’s platform starting in 2023.
- Service fee for clinical trial datasets (<500GB): $4,000
- Larger clinical trial datasets (>500GB): $10,000
- Optional anonymization services provided by Privacy Analytics: $10,000 per dataset
Zenodo Features & Specifications
- All file types are accepted. Files are categorized as: publications, posters, presentations, datasets, images, software, videos/audio, and interactive materials. Zenodo also integrates with GitHub to deposit GitHub repositories for sharing and long-term preservation.
- Total files size limit per record is 50GB. Higher quotas can be requested and granted on a case-by-case basis
- Currently accept up to 50GB per dataset (you can have multiple datasets). There is no size limit on communities. Zenodo also encourages researchers to reach out to discuss use cases with larger dataset sizes.
- Users can choose from a long list of licenses, with the default being Creative Commons Zero (CC0) . Users must specify a license for all publicly available files.
- Zenodo assigns all publicly available uploads a Digital Object Identifier (DOI) to make the upload easily and uniquely citable. Zenodo further supports harvesting of all content via the OAI-PMH protocol. Also allows the user to enter a previously assigned DOI.
- Users can choose to deposit files under open, embargoed, restricted, or closed access. For embargoed files, the user can choose the length of the embargo period, and the content will become publicly available automatically at the end of the embargo period. Users may also deposit restricted files and grant access to specific individuals.
- Free-text search functionality is provided. Machine-readable metadata are also recorded, according to the Invenio Digital Library Framework . Zenodo communicates with existing services, such as Mendeley, ORCID, Crossref, and OpenAIRE for pre-filling metadata.
- In addition to individual file downloading, Zenodo has an OAI-PMH API for programmatic data and metadata access, as described in the Zenodo API documentation
© 2024 by the President and Fellows of Harvard College
Click through the PLOS taxonomy to find articles in your field.
For more information about PLOS Subject Areas, click here .
Recommended Repositories
All data, software and code underlying reported findings should be deposited in appropriate public repositories, unless already provided as part of the article. Repositories may be either subject-specific repositories that accept specific types of structured data and/or software, or cross-disciplinary generalist repositories that accept multiple data and/or software types.
If field-specific standards for data or software deposition exist, PLOS requires authors to comply with these standards. Authors should select repositories appropriate to their field of study (for example, ArrayExpress or GEO for microarray data; GenBank, EMBL, or DDBJ for gene sequences). PLOS has identified a set of established repositories, listed below, that are recognized and trusted within their respective communities. PLOS does not dictate repository selection for the data availability policy.
For further information on environmental and biomedical science repositories and field standards, we suggest utilizing FAIRsharing . Additionally, the Registry of Research Data Repositories ( Re3Data ) is a full scale resource of registered data repositories across subject areas. Both FAIRsharing and Re3Data provide information on an array of criteria to help researchers identify the repositories most suitable for their needs (e.g., licensing, certificates and standards, policy, etc.).
If no specialized community-endorsed public repository exists, institutional repositories that use open licenses permitting free and unrestricted use or public domain, and that adhere to best practices pertaining to responsible sharing, sustainable digital preservation, proper citation, and openness are also suitable for deposition.
If authors use repositories with stated licensing policies, the policies should not be more restrictive than the Creative Commons Attribution (CC BY) license .
Cross-disciplinary repositories
- Dryad Digital Repository
- Harvard Dataverse Network
- Network Data Exchange (NDEx)
- Open Science Framework
- Swedish National Data Service
Repositories by type
Biochemistry
* |
*Data entered in the STRENDA DB submission form are automatically checked for compliance and receive a fact sheet PDF with warnings for any missing information.
Biomedical Sciences
Marine Sciences
- SEA scieNtific Open data Edition (SEANOE)
Model Organisms
Neuroscience
- Functional Connectomes Project International Neuroimaging Data-Sharing Initiative (FCP/INDI)
- German Neuroinformatics Node/G-Node (GIN)
- NeuroMorpho.org
Physical Sciences
Social Sciences
- Inter-university Consortium for Political and Social Research (ICPSR)
- Qualitative Data Repository
- UK Data Service
Structural Databases
Taxonomic & Species Diversity
Unstructured and/or Large Data
PLOS would like to thank the Open Access Nature Publishing Group journal, Scientific Data , for their own list of recommended repositories .
Repository Criteria
The list of repositories above is not exhaustive and PLOS encourages the use of any repository that meet the following criteria:
Dataset submissions should be open to all researchers whose research fits the scientific scope of the repository. PLOS’ list does not include repositories that place geographical or affiliation restrictions on submission of datasets.
Repositories must assign a stable persistent identifier (PID) for each dataset at publication, such as a digital object identifier (DOI) or an accession number.
- Repositories must provide the option for data to be available under CC0 or CC BY licenses (or equivalents that are no less restrictive). Specifically, there must be no restrictions on derivative works or commercial use.
- Repositories should make datasets available to any interested readers at no cost, and with no registration requirements that unnecessarily restrict access to data. PLOS will not recommend repositories that charge readers access fees or subscription fees.
- Repositories must have a long-term data management plan (including funding) to ensure that datasets are maintained for the foreseeable future.
- Repositories should demonstrate acceptance and usage within the relevant research community, for example, via use of the repository for data deposition for multiple published articles.
- Repositories should have an entry in FAIRsharing.org to allow it to be linked to the PLOS entry .
Please note, the list of recommended repositories is not actively maintained. Please use the resources at the top of the page and the criteria above to help select an appropriate repository.
Unfortunately we don't fully support your browser. If you have the option to, please upgrade to a newer version or use Mozilla Firefox , Microsoft Edge , Google Chrome , or Safari 14 or newer. If you are unable to, and need support, please send us your feedback .
We'd appreciate your feedback. Tell us what you think! opens in new tab/window
Sharing research data
As a researcher, you are increasingly encouraged, or even mandated, to make your research data available, accessible, discoverable and usable.
Sharing research data is something we are passionate about too, so we’ve created this short video and written guide to help you get started.
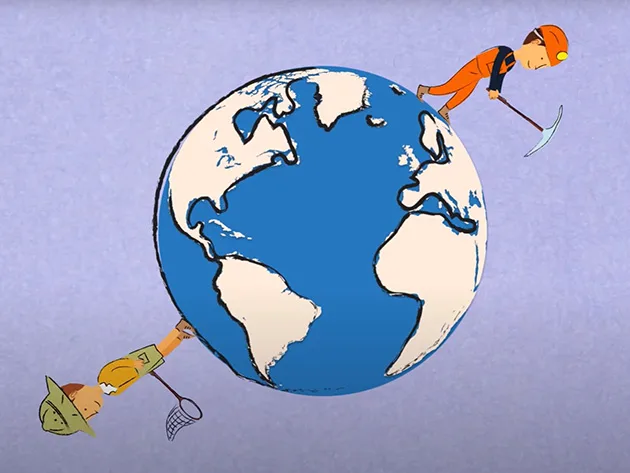
Research Data
What is research data.
While the definition often differs per field, generally, research data refers to the results of observations or experiments that validate your research findings. These span a range of useful materials associated with your research project, including:
Raw or processed data files
Research data does not include text in manuscript or final published article form, or data or other materials submitted and published as part of a journal article.
Why should I share my research data?
There are so many good reasons. We’ve listed just a few:
How you benefit
You get credit for the work you've done
Leads to more citations! 1
Can boost your number of publications
Increases your exposure and may lead to new collaborations
What it means for the research community
It's easy to reuse and reinterpret your data
Duplication of experiments can be avoided
New insights can be gained, sparking new lines of inquiry
Empowers replication
And society at large…
Greater transparency boosts public faith in research
Can play a role in guiding government policy
Improves access to research for those outside health and academia
Benefits the public purse as funding of repeat work is reduced
How do I share my research data?
The good news is it’s easy.
Yet to submit your research article? There are a number of options available. These may vary depending on the journal you have chosen, so be sure to read the Research Data section in its Guide for Authors before you begin.
Already published your research article? No problem – it’s never too late to share the research data associated with it.
Two of the most popular data sharing routes are:
Publishing a research elements article
These brief, peer-reviewed articles complement full research papers and are an easy way to receive proper credit and recognition for the work you have done. Research elements are research outputs that have come about as a result of following the research cycle – this includes things like data, methods and protocols, software, hardware and more.
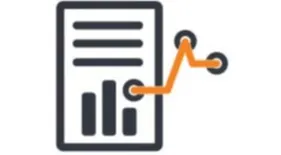
You can publish research elements articles in several different Elsevier journals, including our suite of dedicated Research Elements journals . They are easy to submit, are subject to a peer review process, receive a DOI and are fully citable. They also make your work more sharable, discoverable, comprehensible, reusable and reproducible.
The accompanying raw data can still be placed in a repository of your choice (see below).
Uploading your data to a repository like Mendeley Data
Mendeley Data is a certified, free-to-use repository that hosts open data from all disciplines, whatever its format (e.g. raw and processed data, tables, codes and software). With many Elsevier journals, it’s possible to upload and store your data to Mendeley Data during the manuscript submission process. You can also upload your data directly to the repository. In each case, your data will receive a DOI, making it independently citable and it can be linked to any associated article on ScienceDirect, making it easy for readers to find and reuse.
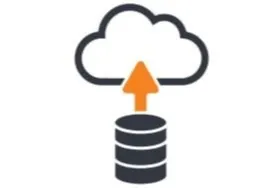
View an article featuring Mendeley data opens in new tab/window (just select the Research Data link in the left-hand bar or scroll down the page).
What if I can’t submit my research data?
Data statements offer transparency.
We understand that there are times when the data is simply not available to post or there are good reasons why it shouldn’t be shared. A number of Elsevier journals encourage authors to submit a data statement alongside their manuscript. This statement allows you to clearly explain the data you’ve used in the article and the reasons why it might not be available. The statement will appear with the article on ScienceDirect.
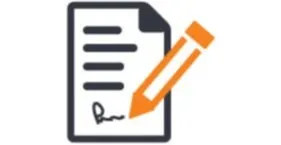
View a sample data statement opens in new tab/window (just select the Research Data link in the left-hand bar or scroll down the page).
Showcasing your research data on ScienceDirect
We have 3 top tips to help you maximize the impact of your data in your article on ScienceDirect.
Link with data repositories
You can create bidirectional links between any data repositories you’ve used to store your data and your online article. If you’ve published a data article, you can link to that too.
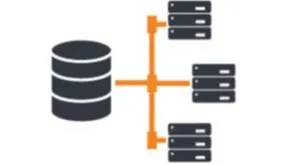
Enrich with interactive data visualizations
The days of being confined to static visuals are over. Our in-article interactive viewers let readers delve into the data with helpful functions such as zoom, configurable display options and full screen mode.
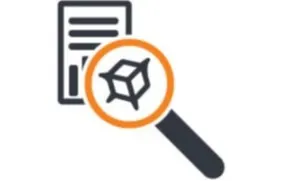
Cite your research data
Get credit for your work by citing your research data in your article and adding a data reference to the reference list. This ensures you are recognized for the data you shared and/or used in your research. Read the References section in your chosen journal’s Guide for Authors for more information.
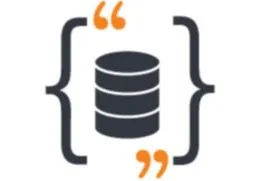
Ready to get started?
If you have yet to publish your research paper, the first step is to find the right journal for your submission and read the Guide for Authors .
Find a journal by matching paper title and abstract of your manuscript in Elsevier's JournalFinder opens in new tab/window
Find journal by title opens in new tab/window
Already published? Just view the options for sharing your research data above.
1 Several studies have now shown that making data available for an article increases article citations.
You are using an outdated browser. Please upgrade your browser to improve your experience.
Featured communities
Open repository for EU-funded research outputs from Horizon Europe, Euratom and earlier Framework Programmes.
Collection of items related to the Generic Mapping Tools software [www.generic-mapping-tools.org].
A community to share publications related to bio-systematics.
Generalist Repository Ecosystem Initiative (GREI) aims to develop collaborative approaches for data management and sharing through inclusion of the generalist repositories in the NIH data ecosystem.
Aurora is a University network platform for European university leaders, administrators, academics, and students to learn from and with each other. The projects we do and the results that can be shared publically will be put in this community page.
ECEMF is a Horizon 2020-funded project aiming to establish a European forum for energy and climate researchers and policy makers to achieve climate neutrality.
Recent uploads
Curated OpenFF PhAlkEthOH Dataset: 1000 conformer test set, version "nc_1000_v0": This provides a curated hdf5 file for a subset of the OpenFF PhAlkEthOH dataset designed to be compatible with modelforge, an infrastructure to implement and train NNPs. This dataset contains 1000 total conformers, for 101 unique molecules (a maximum of 10...
Uploaded on June 20, 2024
Part of modelforge: an infrastructure to implement and train NNPs
1 more version exist for this record
Curated OpenFF PhAlkEthOH Dataset: Full dataset, version "full_dataset_v0": This provides a curated hdf5 file for the OpenFF PhAlkEthOH dataset designed to be compatible with modelforge, an infrastructure to implement and train NNPs. This dataset contains 1,188,709 total conformers, for 12,271 unique molecules. When applicable, the units...
A .zip file containing the source data files & Jupyter notebook for analysis of the Prymnesium parvum 12B1 PKZILLA-detecting proteomic results (https://doi.org/10.5281/zenodo.10023441), and the resulting files from the workflow. See "Analysis of proteomic results" section of the manuscript Materials and Methods for further detail. Key...
Part of Brad S. Moore Lab
Source data files, executable code, and results for A-type PKZILLA PKS domain phylogenetic analysis. Difference from previous version: Difference between v1.0 to v1.1 of this Zenodo item series: is that KR11f0, KR20f0 were renamed in the plots to K11*, KR20*, respectively, based on new interpretations. Difference between v1.1 to v1.2:...
2 more versions exist for this record
DAO-Analyzer's dataset. Explore data from Decentralized Autonomous Organizations deployed on the DAOstack, DAOhaus and Aragon platforms.
Part of EU Open Research Repository (Pilot)
245 more versions exist for this record
What's Changed weaver: bump version to 5.1.0 + debug notebook output by @fmigneault in https://github.com/bird-house/birdhouse-deploy/pull/449 weaver: bump version to 5.6.0 by @fmigneault in https://github.com/bird-house/birdhouse-deploy/pull/463 Full Changelog: https://github.com/bird-house/birdhouse-deploy/compare/2.4.2...2.5.0
Part of Ouranos Consortium on Regional Climatology and Climate Change Adaptation Computer Research Institute of Montreal Birdhouse
64 more versions exist for this record
•Annual electricty use is approx. 67 kwh/capita one of the lowest in the world •Government anticipates electricity generation of 1000 MW by 2030 Research objectives •What is the best alternative technology for the expansion of the power sector in Sierra Leone? •What is the impact of natural gas power generation in the power sector? •How...
Uploaded on June 19, 2024
Part of Climate Compatible Growth
Slides for the EMI session at the World Biodiversity Forum 2024. https://worldbiodiversityforum2024.org/
Part of The Earth Metabolome Initiative
Submission ID: 157 H2IOSC (Humanities and cultural Heritage Italian Open Science Cloud) is a project funded by the National Recovery and Resilience Plan (PNRR) in Italy, aiming to create a federated cluster comprising the Italian branches of four European research infrastructures (RIs) - CLARIN, DARIAH, E-RIHS, OPERAS - operating in the...
Part of Workflows: Digital Methods for Reproducible Research Practices in the Arts and Humanities - DARIAH Annual Event 2024
The goal of scholarly editing is to reconstruct and publish texts by exploring philological phenomena and documenting them in critical apparatuses or editorial notes. Textual scholarship involves complex, multi-stage processes, and the digital landscape requires even additional effort from textual scholars, as it necessitates formal protocols...
This site uses cookies. Find out more on how we use cookies
What is a research repository, and why do you need one?
Last updated
31 January 2024
Reviewed by
Miroslav Damyanov
Without one organized source of truth, research can be left in silos, making it incomplete, redundant, and useless when it comes to gaining actionable insights.
A research repository can act as one cohesive place where teams can collate research in meaningful ways. This helps streamline the research process and ensures the insights gathered make a real difference.
- What is a research repository?
A research repository acts as a centralized database where information is gathered, stored, analyzed, and archived in one organized space.
In this single source of truth, raw data, documents, reports, observations, and insights can be viewed, managed, and analyzed. This allows teams to organize raw data into themes, gather actionable insights , and share those insights with key stakeholders.
Ultimately, the research repository can make the research you gain much more valuable to the wider organization.
- Why do you need a research repository?
Information gathered through the research process can be disparate, challenging to organize, and difficult to obtain actionable insights from.
Some of the most common challenges researchers face include the following:
Information being collected in silos
No single source of truth
Research being conducted multiple times unnecessarily
No seamless way to share research with the wider team
Reports get lost and go unread
Without a way to store information effectively, it can become disparate and inconclusive, lacking utility. This can lead to research being completed by different teams without new insights being gathered.
A research repository can streamline the information gathered to address those key issues, improve processes, and boost efficiency. Among other things, an effective research repository can:
Optimize processes: it can ensure the process of storing, searching, and sharing information is streamlined and optimized across teams.
Minimize redundant research: when all information is stored in one accessible place for all relevant team members, the chances of research being repeated are significantly reduced.
Boost insights: having one source of truth boosts the chances of being able to properly analyze all the research that has been conducted and draw actionable insights from it.
Provide comprehensive data: there’s less risk of gaps in the data when it can be easily viewed and understood. The overall research is also likely to be more comprehensive.
Increase collaboration: given that information can be more easily shared and understood, there’s a higher likelihood of better collaboration and positive actions across the business.
- What to include in a research repository
Including the right things in your research repository from the start can help ensure that it provides maximum benefit for your team.
Here are some of the things that should be included in a research repository:
An overall structure
There are many ways to organize the data you collect. To organize it in a way that’s valuable for your organization, you’ll need an overall structure that aligns with your goals.
You might wish to organize projects by research type, project, department, or when the research was completed. This will help you better understand the research you’re looking at and find it quickly.
Including information about the research—such as authors, titles, keywords, a description, and dates—can make searching through raw data much faster and make the organization process more efficient.
All key data and information
It’s essential to include all of the key data you’ve gathered in the repository, including supplementary materials. This prevents information gaps, and stakeholders can easily stay informed. You’ll need to include the following information, if relevant:
Research and journey maps
Tools and templates (such as discussion guides, email invitations, consent forms, and participant tracking)
Raw data and artifacts (such as videos, CSV files, and transcripts)
Research findings and insights in various formats (including reports, desks, maps, images, and tables)
Version control
It’s important to use a system that has version control. This ensures the changes (including updates and edits) made by various team members can be viewed and reversed if needed.
- What makes a good research repository?
The following key elements make up a good research repository that’s useful for your team:
Access: all key stakeholders should be able to access the repository to ensure there’s an effective flow of information.
Actionable insights: a well-organized research repository should help you get from raw data to actionable insights faster.
Effective searchability : searching through large amounts of research can be very time-consuming. To save time, maximize search and discoverability by clearly labeling and indexing information.
Accuracy: the research in the repository must be accurately completed and organized so that it can be acted on with confidence.
Security: when dealing with data, it’s also important to consider security regulations. For example, any personally identifiable information (PII) must be protected. Depending on the information you gather, you may need password protection, encryption, and access control so that only those who need to read the information can access it.
- How to create a research repository
Getting started with a research repository doesn’t have to be convoluted or complicated. Taking time at the beginning to set up the repository in an organized way can help keep processes simple further down the line.
The following six steps should simplify the process:
1. Define your goals
Before diving in, consider your organization’s goals. All research should align with these business goals, and they can help inform the repository.
As an example, your goal may be to deeply understand your customers and provide a better customer experience . Setting out this goal will help you decide what information should be collated into your research repository and how it should be organized for maximum benefit.
2. Choose a platform
When choosing a platform, consider the following:
Will it offer a single source of truth?
Is it simple to use
Is it relevant to your project?
Does it align with your business’s goals?
3. Choose an organizational method
To ensure you’ll be able to easily search for the documents, studies, and data you need, choose an organizational method that will speed up this process.
Choosing whether to organize your data by project, date, research type, or customer segment will make a big difference later on.
4. Upload all materials
Once you have chosen the platform and organization method, it’s time to upload all the research materials you have gathered. This also means including supplementary materials and any other information that will provide a clear picture of your customers.
Keep in mind that the repository is a single source of truth. All materials that relate to the project at hand should be included.
5. Tag or label materials
Adding metadata to your materials will help ensure you can easily search for the information you need. While this process can take time (and can be tempting to skip), it will pay off in the long run.
The right labeling will help all team members access the materials they need. It will also prevent redundant research, which wastes valuable time and money.
6. Share insights
For research to be impactful, you’ll need to gather actionable insights. It’s simpler to spot trends, see themes, and recognize patterns when using a repository. These insights can be shared with key stakeholders for data-driven decision-making and positive action within the organization.
- Different types of research repositories
There are many different types of research repositories used across organizations. Here are some of them:
Data repositories: these are used to store large datasets to help organizations deeply understand their customers and other information.
Project repositories: data and information related to a specific project may be stored in a project-specific repository. This can help users understand what is and isn’t related to a project.
Government repositories: research funded by governments or public resources may be stored in government repositories. This data is often publicly available to promote transparent information sharing.
Thesis repositories: academic repositories can store information relevant to theses. This allows the information to be made available to the general public.
Institutional repositories: some organizations and institutions, such as universities, hospitals, and other companies, have repositories to store all relevant information related to the organization.
- Build your research repository in Dovetail
With Dovetail, building an insights hub is simple. It functions as a single source of truth where research can be gathered, stored, and analyzed in a streamlined way.
1. Get started with Dovetail
Dovetail is a scalable platform that helps your team easily share the insights you gather for positive actions across the business.
2. Assign a project lead
It’s helpful to have a clear project lead to create the repository. This makes it clear who is responsible and avoids duplication.
3. Create a project
To keep track of data, simply create a project. This is where you’ll upload all the necessary information.
You can create projects based on customer segments, specific products, research methods , or when the research was conducted. The project breakdown will relate back to your overall goals and mission.
4. Upload data and information
Now, you’ll need to upload all of the necessary materials. These might include data from customer interviews , sales calls, product feedback , usability testing , and more. You can also upload supplementary information.
5. Create a taxonomy
Create a taxonomy to organize the data effectively by ensuring that each piece of information will be tagged and organized.
When creating a taxonomy, consider your goals and how they relate to your customers. Ensure those tags are relevant and helpful.
6. Tag key themes
Once the taxonomy is created, tag each piece of information to ensure you can easily filter data, group themes, and spot trends and patterns.
With Dovetail, automatic clustering helps quickly sort through large amounts of information to uncover themes and highlight patterns. Sentiment analysis can also help you track positive and negative themes over time.
7. Share insights
With Dovetail, it’s simple to organize data by themes to uncover patterns and share impactful insights. You can share these insights with the wider team and key stakeholders, who can use them to make customer-informed decisions across the organization.
8. Use Dovetail as a source of truth
Use your Dovetail repository as a source of truth for new and historic data to keep data and information in one streamlined and efficient place. This will help you better understand your customers and, ultimately, deliver a better experience for them.
Should you be using a customer insights hub?
Do you want to discover previous research faster?
Do you share your research findings with others?
Do you analyze research data?
Start for free today, add your research, and get to key insights faster
Editor’s picks
Last updated: 18 April 2023
Last updated: 27 February 2023
Last updated: 6 February 2023
Last updated: 15 January 2024
Last updated: 6 October 2023
Last updated: 5 February 2023
Last updated: 16 April 2023
Last updated: 7 March 2023
Last updated: 9 March 2023
Last updated: 12 December 2023
Last updated: 11 March 2024
Last updated: 13 May 2024
Latest articles
Related topics, .css-je19u9{-webkit-align-items:flex-end;-webkit-box-align:flex-end;-ms-flex-align:flex-end;align-items:flex-end;display:-webkit-box;display:-webkit-flex;display:-ms-flexbox;display:flex;-webkit-flex-direction:row;-ms-flex-direction:row;flex-direction:row;-webkit-box-flex-wrap:wrap;-webkit-flex-wrap:wrap;-ms-flex-wrap:wrap;flex-wrap:wrap;-webkit-box-pack:center;-ms-flex-pack:center;-webkit-justify-content:center;justify-content:center;row-gap:0;text-align:center;max-width:671px;}@media (max-width: 1079px){.css-je19u9{max-width:400px;}.css-je19u9>span{white-space:pre;}}@media (max-width: 799px){.css-je19u9{max-width:400px;}.css-je19u9>span{white-space:pre;}} decide what to .css-1kiodld{max-height:56px;display:-webkit-box;display:-webkit-flex;display:-ms-flexbox;display:flex;-webkit-align-items:center;-webkit-box-align:center;-ms-flex-align:center;align-items:center;}@media (max-width: 1079px){.css-1kiodld{display:none;}} build next, decide what to build next.

Users report unexpectedly high data usage, especially during streaming sessions.

Users find it hard to navigate from the home page to relevant playlists in the app.

It would be great to have a sleep timer feature, especially for bedtime listening.

I need better filters to find the songs or artists I’m looking for.
Log in or sign up
Get started for free
News: Teamscope joins StudyPages 🎉
Data collection in the fight against COVID-19
Data Sharing
6 repositories to share your research data.

Dear Diary, I have been struggling with an eating disorder for the past few years. I am afraid to eat and afraid I will gain weight. The fear is unjustified as I was never overweight. I have weighed the same since I was 12 years old, and I am currently nearing my 25th birthday. Yet, when I see my reflection, I see somebody who is much larger than reality. I told my therapist that I thought I was fat. She said it was 'body dysmorphia'. She explained this as a mental health condition where a person is apprehensive about their appearance and suggested I visit a nutritionist. She also told me that this condition was associated with other anxiety disorders and eating disorders. I did not understand what she was saying as I was in denial; I had a problem, to begin with. I wanted a solution without having to address my issues. Upon visiting my nutritionist, he conducted an in-body scan and told me my body weight was dangerously low. I disagreed with him. I felt he was speaking about a different person than the person I saw in the mirror. I felt like the elephant in the room- both literally and figuratively. He then made the simple but revolutionary suggestion to keep a food diary to track what I was eating. This was a clever way for my nutritionist and me to be on the same page. By recording all my meals, drinks, and snacks, I was able to see what I was eating versus what I was supposed to be eating. Keeping a meal diary was a powerful and non-invasive way for my nutritionist to walk in my shoes for a specific time and understand my eating (and thinking) habits. No other methodology would have allowed my nutritionist to capture so much contextual and behavioural information on my eating patterns other than a daily detailed food diary. However, by using a paper and pen, I often forgot (or intentionally did not enter my food entries) as I felt guilty reading what I had eaten or that I had eaten at all. I also did not have the visual flexibility to express myself through using photos, videos, voice recordings, and screen recordings. The usage of multiple media sources would have allowed my nutritionist to observe my behaviour in real-time and gain a holistic view of my physical and emotional needs. I confessed to my therapist my deliberate dishonesty in completing the physical food diary and why I had been reluctant to participate in the exercise. My therapist then suggested to my nutritionist and me to transition to a mobile diary study. Whilst I used a physical diary (paper and pen), a mobile diary study app would have helped my nutritionist and me reach a common ground (and to be on the same page) sooner rather than later. As a millennial, I wanted to feel like journaling was as easy as Tweeting or posting a picture on Instagram. But at the same time, I wanted to know that the information I provided in a digital diary would be as safe and private as it would have been as my handwritten diary locked in my bedroom cabinet. Further, a digital food diary study platform with push notifications would have served as a constant reminder to log in my food entries as I constantly check my phone. It would have also made the task of writing a food diary less momentous by transforming my journaling into micro-journaling by allowing me to enter one bite at a time rather than the whole day's worth of meals at once. Mainly, the digital food diary could help collect the evidence that I was not the elephant in the room, but rather that the elephant in the room was my denied eating disorder. Sincerely, The elephant in the room
Why share research data?
Sharing information stimulates science. When researchers choose to make their data publicly available, they are allowing their work to contribute far beyond their original findings.
The benefits of data sharing are immense. When researchers make their data public, they increase transparency and trust in their work, they enable others to reproduce and validate their findings, and ultimately, contribute to the pace of scientific discovery by allowing others to reuse and build on top of their data.
"If I have seen further it is by standing on the shoulders of Giants." Isaac Newton, 1675.
While the benefits of data sharing and open science are categorical, sadly 86% of medical research data is never reused . In a 2014 survey conducted by Wiley with over 2000 researchers across different fields, found that 21% of surveyed researchers did not know where to share their data and 16% how to do so.
In a series of articles on Data Sharing we seek to break down this process for you and cover everything you need to know on how to share your research outputs.
In this first article, we will introduce essential concepts of public data and share six powerful platforms to upload and share datasets.
What is a Research Data Repository?
The best way to publish and share research data is with a research data repository. A repository is an online database that allows research data to be preserved across time and helps others find it.
Apart from archiving research data, a repository will assign a DOI to each uploaded object and provide a web page that tells what it is, how to cite it and how many times other researchers have cited or downloaded that object.
What is a DOI?
When a researcher uploads a document to an online data repository, a digital object identifier (DOI) will be assigned. A DOI is a globally unique and persistent string (e.g. 10.6084/m9.figshare.7509368.v1) that identifies your work permanently.
A data repository can assign a DOI to any document, such as spreadsheets, images or presentation, and at different levels of hierarchy, like collection images or a specific chapter in a book.
The DOI contains metadata that provides users with relevant information about an object, such as the title, author, keywords, year of publication and the URL where that document is stored.
The International DOI Foundation (IDF) developed and introduced the DOI in 2000. Registration Agencies, a federation of independent organizations, register DOIs and provide the necessary infrastructure that allows researchers to declare and maintain metadata.

Key benefits of the DOI system:
- A more straightforward way to track research outputs
- Gives certainty to scientific work
- DOI's versioning system tracks changes to work overtime
- Can be assigned to any document
- Enables proper indexation and citation of research outputs
Once a document has a DOI, others can easily cite it. A handy tool to convert DOI's into a citation is DOI Citation Formatter .
Six repositories to share research data
Now that we have covered the role of a DOI and a data repository, below is a list of 6 data repositories for publishing and sharing research data.
1. figshare
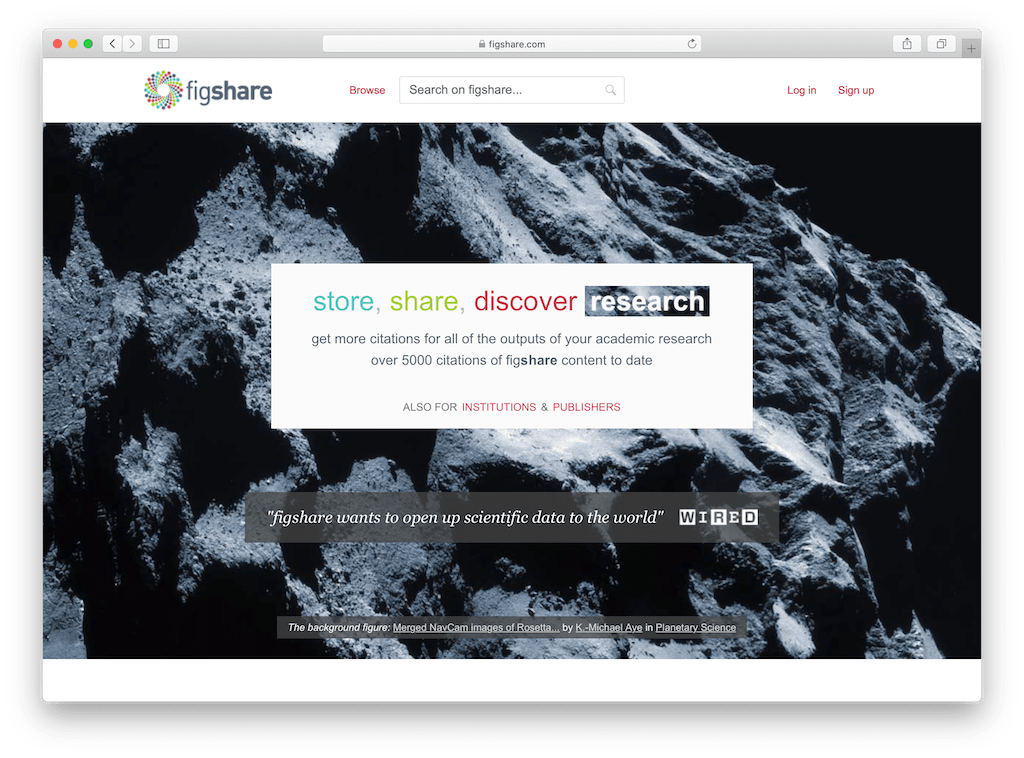
Figshare is an open access data repository where researchers can preserve their research outputs, such as datasets, images, and videos and make them discoverable.
Figshare allows researchers to upload any file format and assigns a digital object identifier (DOI) for citations.
Mark Hahnel launched Figshare in January 2011. Hahnel first developed the platform as a personal tool for organizing and publishing the outputs of his PhD in stem cell biology. More than 50 institutions now use this solution.
Figshare releases' The State of Open Data' every year to assess the changing academic landscape around open research.
Free accounts on Figshare can upload files of up to 5gb and get 20gb of free storage.
2. Mendeley Data

Mendeley Data is an open research data repository, where researchers can store and share their data. Datasets can be shared privately between individuals, as well as publicly with the world.
Mendeley's mission is to facilitate data sharing. In their own words, "when research data is made publicly available, science benefits:
- the findings can be verified and reproduced- the data can be reused in new ways
- discovery of relevant research is facilitated
- funders get more value from their funding investment."
Datasets uploaded to Mendeley Data go into a moderation process where they are reviewed. This ensures the content constitutes research data, is scientific, and does not contain a previously published research article.
Researchers can upload and store their work free of cost on Mendeley Data.
If appropriately used in the 21st century, data could save us from lots of failed interventions and enable us to provide evidence-based solutions towards tackling malaria globally. This is also part of what makes the ALMA scorecard generated by the African Leaders Malaria Alliance an essential tool for tracking malaria intervention globally. If we are able to know the financial resources deployed to fight malaria in an endemic country and equate it to the coverage and impact, it would be easier to strengthen accountability for malaria control and also track progress in malaria elimination across the continent of Africa and beyond.
Odinaka Kingsley Obeta
West African Lead, ALMA Youth Advisory Council/Zero Malaria Champion
There is a smarter way to do research.
Build fully customizable data capture forms, collect data wherever you are and analyze it with a few clicks — without any training required.
3. Dryad Digital Repository

Dryad is a curated general-purpose repository that makes data discoverable, freely reusable, and citable.
Most types of files can be submitted (e.g., text, spreadsheets, video, photographs, software code) including compressed archives of multiple files.
Since a guiding principle of Dryad is to make its contents freely available for research and educational use, there are no access costs for individual users or institutions. Instead, Dryad supports its operation by charging a $120US fee each time data is published.
4. Harvard Dataverse

Harvard Dataverse is an online data repository where scientists can preserve, share, cite and explore research data.
The Harvard Dataverse repository is powered by the open-source web application Dataverse, developed by Insitute of Quantitative Social Science at Harvard.
Researchers, journals and institutions may choose to install the Dataverse web application on their own server or use Harvard's installation. Harvard Dataverse is open to all scientific data from all disciplines.
Harvard Dataverse is free and has a limit of 2.5 GB per file and 10 GB per dataset.
5. Open Science Framework

OSF is a free, open-source research management and collaboration tool designed to help researchers document their project's lifecycle and archive materials. It is built and maintained by the nonprofit Center for Open Science.
Each user, project, component, and file is given a unique, persistent uniform resource locator (URL) to enable sharing and promote attribution. Projects can also be assigned digital object identifiers (DOIs) if they are made publicly available.
OSF is a free service.

Zenodo is a general-purpose open-access repository developed under the European OpenAIRE program and operated by CERN.
Zenodo was first born as the OpenAire orphan records repository, with the mission to provide open science compliance to researchers without an institutional repository, irrespective of their subject area, funder or nation.
Zenodo encourages users to early on in their research lifecycle to upload their research outputs by allowing them to be private. Once an associated paper is published, datasets are automatically made open.
Zenodo has no restriction on the file type that researchers may upload and accepts dataset of up to 50 GB.
Research data can save lives, help develop solutions and maximise our knowledge. Promoting collaboration and cooperation among a global research community is the first step to reduce the burden of wasted research.
Although the waste of research data is an alarming issue with billions of euros lost every year, the future is optimistic. The pressure to reduce the burden of wasted research is pushing journals, funders and academic institutions to make data sharing a strict requirement.
We hope with this series of articles on data sharing that we can light up the path for many researchers who are weighing the benefits of making their data open to the world.
The six research data repositories shared in this article are a practical way for researchers to preserve datasets across time and maximize the value of their work.
Cover image by Copernicus Sentinel data (2019), processed by ESA, CC BY-SA 3.0 IG .
References:
“Harvard Dataverse,” Harvard Dataverse, https://library.harvard.edu/services-tools/harvard-dataverse
“Recommended Data Repositories.” Nature, https://go.nature.com/2zdLYTz
“DOI Marketing Brochure,” International DOI Foundation, http://bit.ly/2KU4HsK
“Managing and sharing data: best practice for researchers.” UK Data Archive, http://bit.ly/2KJHE53
Wikipedia contributors, “Figshare,” Wikipedia, The Free Encyclopedia, https://en.wikipedia.org/w/index.php?title=Figshare&oldid=896290279 (accessed August 20, 2019).
Walport, M., & Brest, P. (2011). Sharing research data to improve public health. The Lancet, 377(9765), 537–539. https://doi.org/10.1016/s0140-6736(10)62234-9
Foster, E. D., & Deardorff, A. (2017). Open Science Framework (OSF). Journal of the Medical Library Association : JMLA , 105 (2), 203–206. doi:10.5195/jmla.2017.88
Wikipedia contributors, "Zenodo," Wikipedia, The Free Encyclopedia, https://en.wikipedia.org/w/index.php?title=Zenodo&oldid=907771739 (accessed August 20, 2019).
Wikipedia contributors, "Dryad (repository)," Wikipedia, The Free Encyclopedia, https://en.wikipedia.org/w/index.php?title=Dryad_(repository)&oldid=879494242 (accessed August 20, 2019).
“How and Why Researchers Share Data (and Why They don't),” The Wiley Network, Liz Ferguson , http://bit.ly/31TzVHs
“Frequently Asked Questions,” Mendeley Data, https://data.mendeley.com/faq
Dear Digital Diary, I realized that there is an unquestionable comfort in being misunderstood. For to be understood, one must peel off all the emotional layers and be exposed. This requires both vulnerability and strength. I guess by using a physical diary (a paper and a pen), I never felt like what I was saying was analyzed or judged. But I also never thought I was understood. Paper does not talk back.Using a daily digital diary has required emotional strength. It has required the need to trust and the need to provide information to be helped and understood. Using a daily diary has needed less time and effort than a physical diary as I am prompted to interact through mobile notifications. I also no longer relay information from memory, but rather the medical or personal insights I enter are real-time behaviours and experiences. The interaction is more organic. I also must confess this technology has allowed me to see patterns in my behaviour that I would have otherwise never noticed. I trust that the data I enter is safe as it is password protected. I also trust that I am safe because my doctor and nutritionist can view my records in real-time. Also, with the data entered being more objective and diverse through pictures and voice recordings, my treatment plan has been better suited to my needs. Sincerely, No more elephants in this room
Diego Menchaca
Diego is the founder and CEO of Teamscope. He started Teamscope from a scribble on a table. It instantly became his passion project and a vehicle into the unknown. Diego is originally from Chile and lives in Nijmegen, the Netherlands.
More articles on
How to successfully share research data.
Understanding and using data repositories
What is a data repository.
A data repository is a storage space for researchers to deposit data sets associated with their research. And if you’re an author seeking to comply with a journal data sharing policy , you’ll need to identify a suitable repository for your data.
An open access data repository openly stores data in a way that allows immediate user access to anyone. There are no limitations to the repository access.
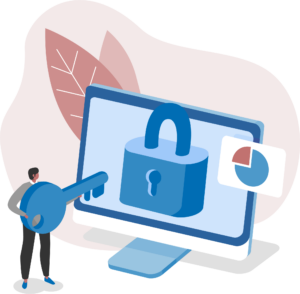
Publishing tips, direct to your inbox
Expert tips and guidance on getting published and maximizing the impact of your research. Register now for weekly insights direct to your inbox.
How should I choose a data repository?
First we recommend speaking to your institutional librarian, funder or colleagues at your institution for guidance on choosing a repository that is relevant to your discipline. You can also use FAIRsharing and re3data.org to search for a suitable repository – both provide a list of certified data repositories.
For cases where there is no subject-specific repository, you may wish to consider some of the generalist data repository types below.
4TU.ResearchData
ANDS contributing repositories
Dryad Digital Repository
Harvard Dataverse
Mendeley Data
Open Science Framework
Science Data Bank
Code Ocean (with code)
We encourage authors to select a data repository that issues a persistent identifier, preferably a Digital Object Identifier (DOI), and has established a robust preservation plan to ensure the data is preserved in perpetuity. Additionally, we highly encourage researchers to consider the FAIR Data Principles when depositing data.
Taylor & Francis Online supports ScholeXplorer data linking, helping you to establish a permanent link between your published article and its associated data . If you deposit your data in a ScholeXplorer recognized repository a link to your data will automatically appear on Taylor & Francis Online when your associated article is published.
Checklist for choosing a data repository
Use the Instructions for Authors to find out which data sharing policy your chosen journal adheres to.
Speak to your librarian for a recommendation that’s relevant to your discipline. There may be an institutional repository that is suitable.
Use FAIRsharing and re3data.org if you still haven’t found a suitable repository.
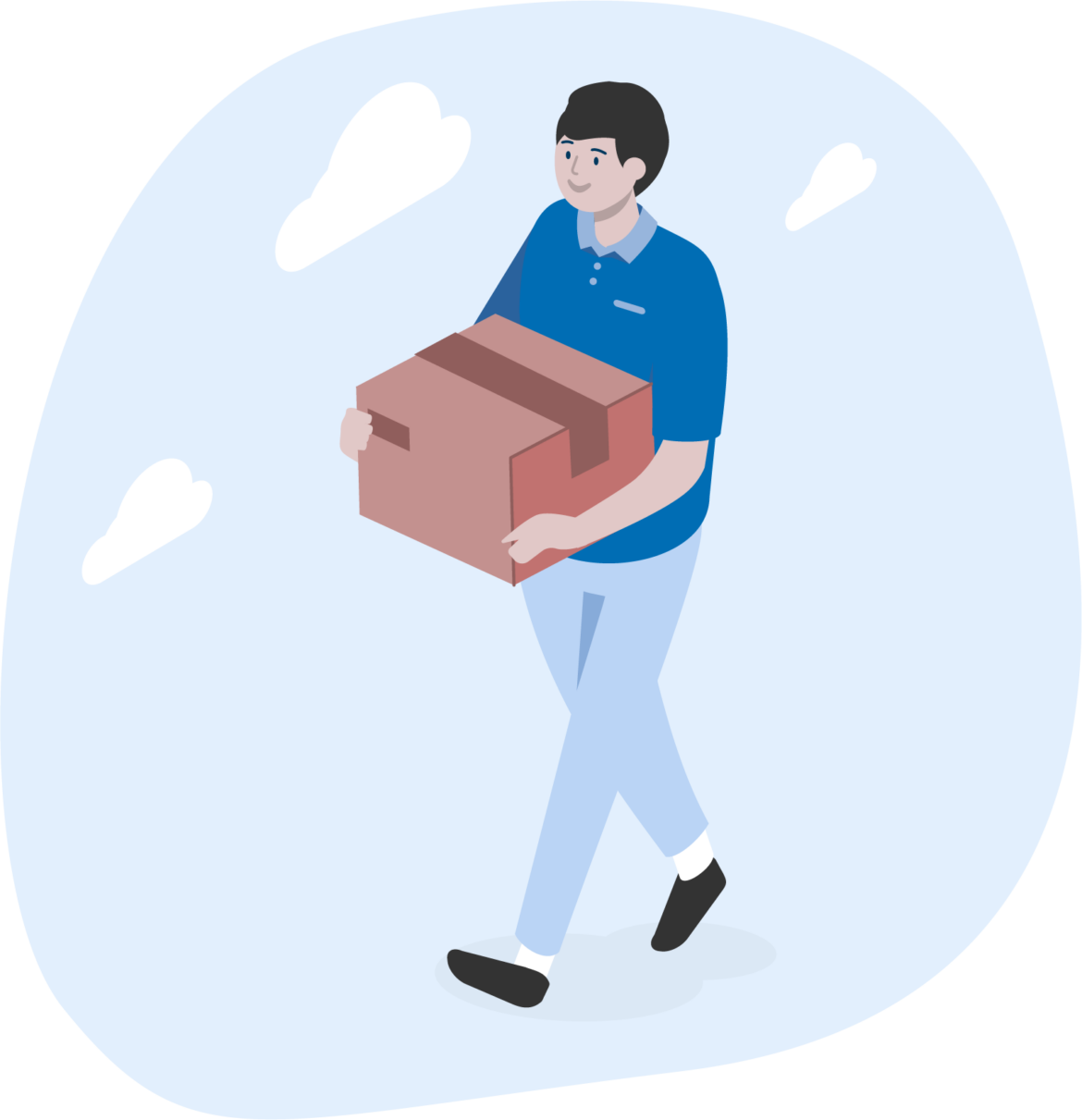
Frequently asked questions about using data repositories
The journal i’m submitting to is double-anonymous. what repository should i use.
If you’re submitting your article to a journal with a double-anonymous peer review policy and a data policy that mandates sharing, then you will need to deposit your data in a repository that preserves anonymity, i.e. removes the details of the authors.
You can use the repository Figshare to generate a ‘private sharing link’ for free. This can be sent via email and the recipient can access the data without logging in or having a Figshare account. This feature is especially for anonymous peer review; you can generate a private sharing link to anonymize data for reviewers. It does not include the Author field or any non-Figshare branding. It is important to note that these links expire after one year however; therefore you should not cite them in publications.
Dryad is another (paid for) alternative which allows you to make your data temporarily “private for peer review.” Dryad uses professional curators to ensure the validity of the files and descriptive information.
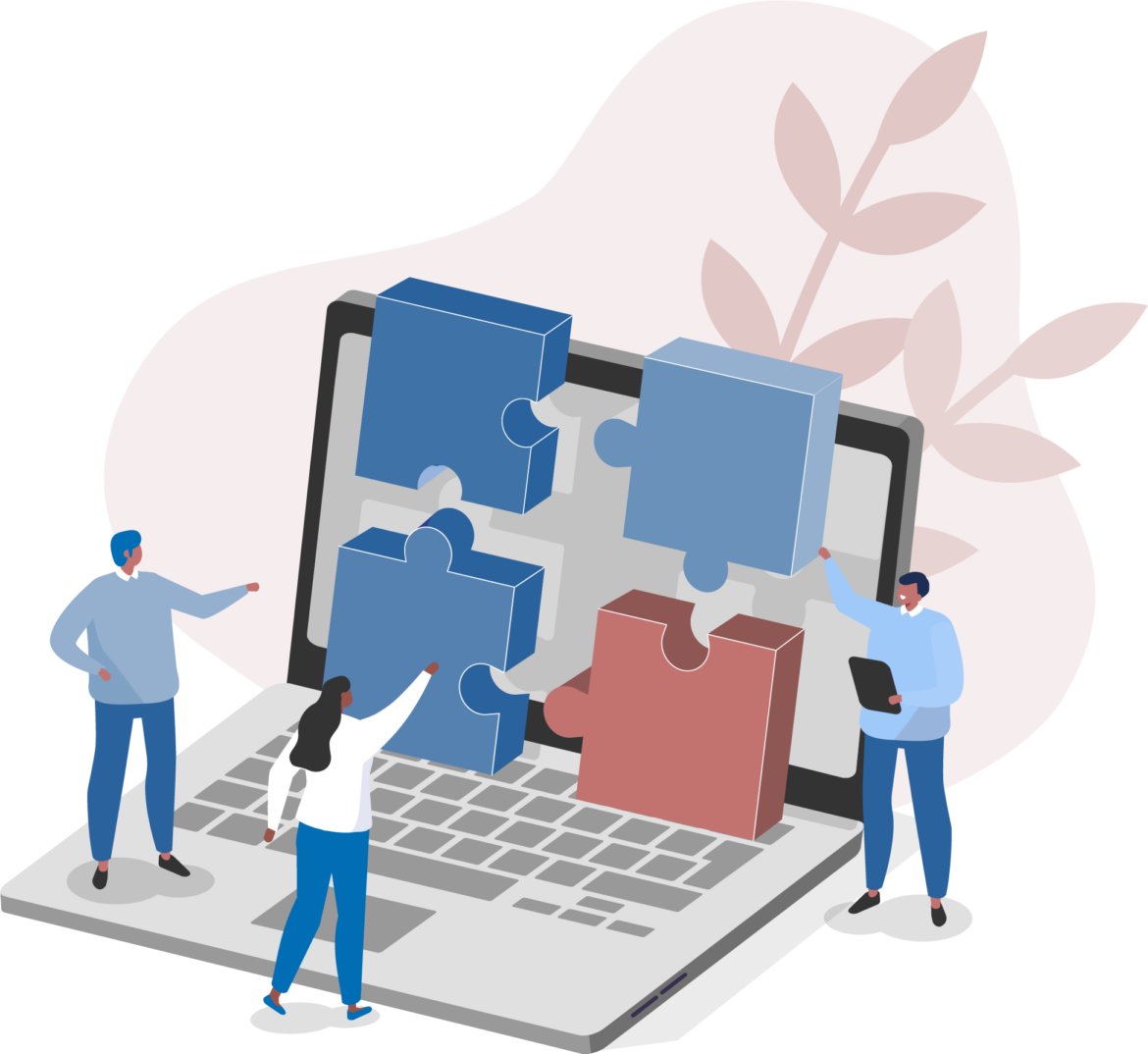
The policy states I need to share my data in a ‘FAIR aligned’ repository. What repository should I use?
The repository finder tool, developed by DataCite allows you to search for repositories which are certified and support the FAIR data principles.
Read on to find out how you can choose a FAIR aligned repository .
I need to limit access to my data in a repository. How can I do this?
There are a number of generalist repositories which allow you to limit access to your data, whether permanently or following an embargo period. Some of the repositories offering this functionality include:
Figshare – You can generate a ‘private sharing link’ for free. This can be sent via email address and the recipient can access the data without logging in or having a Figshare account.
Zenodo – Users may deposit restricted files with the ability to share access with others if certain requirements are met. These files will not be publicly available. The depositor of the original file will need to approve sharing of the data. You can also deposit content under an embargo status and provide an end date for the embargo; at the end date the content will become publicly available automatically.
OSF – You can make your project private or public and alternate between the two settings. You can have different privacy settings on your project and components, controlling which parts are public or private.
You may choose to limit access to your data if the journal you’re submitting your article to has a ‘ share upon reasonable request ‘ data sharing policy.

Research Data Repositories: Home
What is a research data repository.
A research data repository is a virtual place to store and preserve research data. Depositing research data in a repository increases data transparency and exposure and promotes research collaboration opportunities. There are multidisciplinary, subject-based, and special purpose repositories available to researchers worldwide. For example, iDEA houses digital resources produced by the Drexel University community.
Repository Selection Criteria
Although Drexel University Libraries does not currently recommend specific repositories, we advise reviewing the following considerations before selecting a repository for your research data.
- Does the Repository support FAIR principles?
FAIR means that data publishing platforms should enable data to be Findable, Accessible, Interoperable, and Re-usable. Many organizations, including the NIH, place considerable emphasis on data sharing that meets these principles.
- Data is Findable if it is uniquely and persistently identifiable. Does the repository register your data to create a persistent identifier (such as a DOI)? Does the repository provide for rich metadata that will enable discovery? Is your research output and data indexed in Google or subject databases?
- Data is Accessible if it can be understood and obtained by machines or humans, through a standard protocol that allows for authorization and authentication, where necessary.
- Data Objects are Interoperable if metadata and data are machine-accessible and utilize shared terminology.
- Data Objects are Re-Usable if the data can be automatically linked or integrated with other data sources, with proper citation of the source. Are data use agreements and/or licensing clearly presented, to allow depositors to state explicitly up front what uses they would be willing to allow?
- What is the cost structure? Are there ongoing costs after deposit? Have you accounted for these costs in your grant budget?
- Does the repository meet a set of certification standards? Check to see if a repository follows certification standards such as the Core Trust Seal of Approval or the Trustworthy Repositories audit & Certification: Criteria and Checklist (TRAC). Although certification criteria are informative, repository certification is still in its infancy.Most repositories have not achieved certification.
*University of Iowa Libraries. Research Data Services Data Repositories. Retrieved August 27, 2019 http://www.lib.uiowa.edu/data/share/data-repositories/
Finding Repositories
As the number of research data repositories increases, so do registries and directories of repositories. The following registries are useful for locating a repository for your research data:
- re3data.org : global registry that includes data repositories from various academic disciplines
- DataONE : DataONE is a community driven project providing access to data across multiple member repositories, supporting enhanced search and discovery of Earth and environmental data.
- NIH : These repositories include NIH-supported data accessible for reuse. Most accept submissions of appropriate data from NIH-funded investigators (and others), but some restrict data submission to only those researchers involved in a specific research network.
- PLOS Recommended Repositories : PLOS has identified a set of established repositories that are trusted and recognized within their respective communities
Scientific Data Recommended Data Repositories : repositories recommended by Scientific Data . Repositories on this list have been evaluated by Scientific Data , to ensure that they meet their requirements for data access, preservation, and stability.
Popular Research Data Repositories
Listed below are some popular research data repositories and details regarding each one.
Dryad https://www.datadryad.org/
- Subject/Discipline: multidisciplinary, initially biosciences
- Data Types/Data Status: Unstandardized file types found in most research. Final, from accepted peer-reviewed journal articles, or dissertations & books.
- File Format/File Size: any that follow accepted community standards and follow preservation-friendly file formats.
- Deposit Size Limitations: excess storage fees for data packages totaling over 20GB
- Accepted Metadata Schemas: any, so long as relevant details about data collection, processing, and analysis are included in a README document.
- Persistent Identifiers provided: DOI
- Levels of Access to Deposited Data: Unpublished, Published
- Fees: yes, base charge per data package is $120
- Data Curation Services (yes/no): yes
- Where Indexed: DCI , OAD , OpenDOAR , re3data
- Supports OAI (yes/no): yes
- API available (yes/no): yes
Figshare https://knowledge.figshare.com/ Figshare Features Sharing Data with FigShare
- Subject/Discipline: multidisciplinary
- Data Types/Data Status: quantitative/any status
- File Format/File Size: File formats, Figures, videos, posters,peer reviewed papers, diagrams, preprints, codes , scripts, slides, thesis
- Deposit Size Limitations: Upload files up to 5 GB, 20 GB of free private space, unlimited free public space
- Accepted Metadata Schemas: Dublin Core, Datacite, RDF
- Persistent Identifiers provided: DOI
- Levels of Access to Deposited Data: Embargoed , confidential, private public, unpublished, published
- Data Curation Services (yes/no): yes with subscription
- Where Indexed : DCI , OAD , OpenDOAR , re3data
- API available (yes/no): yes
Harvard Dataverse
Harvard Dataverse https://dataverse.harvard.edu/
- Subject/Discipline: multidisciplinary
- Data Types/Data Status: quantitative, qualitative/any
- File Format/File Size: any/ varies
- Deposit Size Limitations: varies
- Accepted Metadata Schemas: various
- Persistent Identifiers provided: DOI/Handle
- Levels of Access to Deposited Data: Closed, Unpublished, Published (restricted, not restricted)
- Fees : none
- Data Curation Services (yes/no): no
- Where Indexed : OAD , OpenDOAR , re3data
ICPSR Inter-university Consortium for Political and Social Science Research
ICPSR https://www.icpsr.umich.edu/icpsrweb/ ACCESS NOTE: User must create free MyData Login while on-campus or using VPN in order to download data. After the Login is created you will be able to download from anywhere.
- Subject/Discipline: social and behavioral sciences, with specialized collections in education, aging, criminal justice, substance abuse, terrorism, and other fields.
- Data Types/Data Status: accepts quantitative, qualitative and GIS data; final project status
- File Format/File Size: SAS, SPSS, or Stata files preferred. ASCII and other file formats also accepted.
- Deposit Size Limitations: no reported limit for curated ICPSR deposit; 2GB free self-deposit in OpenICSPSR
- Accepted Metadata Schemas: Recommended: DDI metadata schema
- Persistent Identifiers provided: DOI
- Levels of Access to Deposited Data: Open; Secure Online Analysis (public or password-protected); Restricted Use Data Agreement; Virtual Data Enclave; Physical Data Enclave
- Fees: no fee for self-deposit of data up to 2GB in OpenICPSR; fee for fully-curated deposit. Drexel institutional membership discounts curation fees.
- Data Curation Services (yes/no): none for OpenICPSR; full OIAS-based curation services for curated deposit.
- Where Indexed: DCI , OAD , re3data
- Supports OAI (yes/no): yes
- API available (yes/no): yes
QDR https://qdr.syr.edu/
- Subject/Discipline: social sciences, related traditions that use cognate methods
- Data Types/Data Status: qualitative , multi-method/ various
- File Format/File Size: alphanumeric, audio, video, photographic/less than 2 MB
- Deposit Size Limitations: not listed
- Accepted Metadata Schemas: DDI
- Levels of Access to Deposited Data: Standard, Conditional Online, Depositor Approved, Restricted Offline, Embargoed
- Fees: no fees to access or deposit data, but institutional membership available
- Data Curation Services (yes/no): yes (and more comprehensive curation services for QDR institutional members)
- Where Indexed : re3data
- Supports OAI (yes/no): no
Zenodo https://zenodo.org/
- Data Types/Data Status: qualitative, quantitative and mixed method
- File Format/File Size: text, spreadsheets, audio, video, images, source code.
- Deposit Size Limitations: 50 GB per data set, unlimited number of data sets.
- Accepted Metadata Schem . as: Marc, Dublin Core, Data Cite
- Levels of Access to Deposited Data: Opened, embargoed, restricted and closed
- Data Curation Services (yes/no): no
- Where Indexed: DCI , OAD , OpenDOAR , re3data
- Supports OAI (yes/no): yes
- API available (yes/no): yes
Citing Data from Research Data Repositories
Data should be cited within a publication just as other literature are cited. Use the appropriate format based on the citation style you're using (eg. APA, Chicago, Turabian, etc.). The minimum amount of information that should be included in a dataset citation is the following:
- Date published
- Universal, persistent identifier (PID)
- Some way to resolve the PID (DOIs and ARKs both have resolution services)
- Date accessed
Source:
https://libguides.utk.edu/dataservices/citation
Many data repositories have specific guidelines on how the data they host should be attributed. These citation guidelines are often included in the dataset’s metadata or linked from the repository’s web site.
Example of citing data from the Dryad repository:
How do I cite data from Dryad?
When citing data found in Dryad, please cite both the original article, as well as the Dryad data package. You can see both of these citations on the Dryad page for each data package.
Westbrook JW, Kitajima K, Burleigh JG, Kress WJ, Erickson DL, Wright SJ (2011) Data from: What makes a leaf tough? Patterns of correlated evolution between leaf toughness traits and demographic rates among 197 shade-tolerant woody species in a neotropical forest. Dryad Digital Repository. https://doi.org/10.5061/dryad.8525
Source: https://datadryad.org//pages/faq#using
Related Websites:
How to Cite Datasets and Link to Publications (Digital Curation Centre)
Why and How Should I Cite Data? (ICPSR)
Related Library Guides:
- Citation Style Manuals by Kathleen Turner Last Updated May 24, 2024 431 views this year
For Further Assistance
Please contact [email protected] to request assistance with finding a research data repository or uploading research data to a repository.
- Last Updated: Sep 16, 2022 1:35 PM
- URL: https://libguides.library.drexel.edu/data

Redirect Notice

Selecting a Data Repository
Learn how to evaluate and select appropriate data repositories.
As outlined in NIH's Supplemental Policy Information: Selecting a Repository for Data Resulting from NIH-Supported Research , using a quality data repository generally improves the FAIRness (Findable, Accessible, Interoperable, and Re-usable) of the data. For that reason, NIH strongly encourages the use of established repositories to the extent possible for preserving and sharing scientific data.
While NIH supports many data repositories, there are also many biomedical data repositories and generalist repositories supported by other organizations, both public and private. Researchers may wish to consult experts in their own institutions (e.g., librarians, data managers) for assistance in selecting an appropriate data repository.
NIH encourages researchers to select data repositories that exemplify the desired characteristics below, including when a data repository is supported or provided by a cloud-computing or high-performance computing platform. These desired characteristics aim to ensure that data are managed and shared in ways that are consistent with FAIR data principles.
- For data generated from research subject to such policies or funded under such opportunities, researchers should use the designated data repository(ies).
- Primary consideration should be given to data repositories that are discipline or data-type specific to support effective data discovery and reuse. For a list of NIH-supported repositories, visit Repositories for Sharing Scientific Data .
- Small datasets (up to 2 GB in size) may be included as supplementary material to accompany articles submitted to PubMed Central ( instructions ).
- Data repositories, including generalist repositories or institutional repositories, that make data available to the larger research community, institutions, or the broader public.
- Large datasets may benefit from cloud-based data repositories for data access, preservation, and sharing.
See Repositories for Sharing Scientific Data for a listing of NIH-supported data repositories.
Desirable Characteristics for All Data Repositories
When choosing a repository to manage and share data resulting from Federally funded research, here are some desirable characteristics to look for:
- Unique Persistent Identifiers: Assigns datasets a citable, unique persistent identifier, such as a digital object identifier (DOI) or accession number, to support data discovery, reporting, and research assessment. The identifier points to a persistent landing page that remains accessible even if the dataset is de-accessioned or no longer available.
- Long-Term Sustainability: Has a plan for long-term management of data, including maintaining integrity, authenticity, and availability of datasets; building on a stable technical infrastructure and funding plans; and having contingency plans to ensure data are available and maintained during and after unforeseen events.
- Metadata: Ensures datasets are accompanied by metadata to enable discovery, reuse, and citation of datasets, using schema that are appropriate to, and ideally widely used across, the community(ies) the repository serves. Domain-specific repositories would generally have more detailed metadata than generalist repositories.
- Curation and Quality Assurance: Provides, or has a mechanism for others to provide, expert curation and quality assurance to improve the accuracy and integrity of datasets and metadata.
- Free and Easy Access: Provides broad, equitable, and maximally open access to datasets and their metadata free of charge in a timely manner after submission, consistent with legal and ethical limits required to maintain privacy and confidentiality, Tribal sovereignty, and protection of other sensitive data.
- Broad and Measured Reuse: Makes datasets and their metadata available with broadest possible terms of reuse; and provides the ability to measure attribution, citation, and reuse of data (i.e., through assignment of adequate metadata and unique PIDs).
- Clear Use Guidance: Provides accompanying documentation describing terms of dataset access and use (e.g., particular licenses, need for approval by a data use committee).
- Security and Integrity: Has documented measures in place to meet generally accepted criteria for preventing unauthorized access to, modification of, or release of data, with levels of security that are appropriate to the sensitivity of data.
- Confidentiality: Has documented capabilities for ensuring that administrative, technical, and physical safeguards are employed to comply with applicable confidentiality, risk management, and continuous monitoring requirements for sensitive data.
- Common Format: Allows datasets and metadata downloaded, accessed, or exported from the repository to be in widely used, preferably non-proprietary, formats consistent with those used in the community(ies) the repository serves.
- Provenance: Has mechanisms in place to record the origin, chain of custody, and any modifications to submitted datasets and metadata.
- Retention Policy: Provides documentation on policies for data retention within the repository.
Additional Considerations for Human Data
When working with human participant data, including de-identified human data, here are some additional characteristics to look for:
- Fidelity to Consent: Uses documented procedures to restrict dataset access and use to those that are consistent with participant consent and changes in consent.
- Restricted Use Compliant: Uses documented procedures to communicate and enforce data use restrictions, such as preventing reidentification or redistribution to unauthorized users.
- Privacy: Implements and provides documentation of measures (for example, tiered access, credentialing of data users, security safeguards against potential breaches) to protect human subjects’ data from inappropriate access.
- Plan for Breach: Has security measures that include a response plan for detected data breaches.
- Download Control: Controls and audits access to and download of datasets (if download is permitted).
- Violations: Has procedures for addressing violations of terms-of-use by users and data mismanagement by the repository.
- Request Review: Makes use of an established and transparent process for reviewing data access requests.
Repositories for Scientific Data
See Repositories for Sharing Scientific Data for a listing of NIH-affiliated data repositories.
Related Resources
Repositories for Sharing Scientific Data
Thank you for visiting nature.com. You are using a browser version with limited support for CSS. To obtain the best experience, we recommend you use a more up to date browser (or turn off compatibility mode in Internet Explorer). In the meantime, to ensure continued support, we are displaying the site without styles and JavaScript.
- View all journals
- Explore content
- About the journal
- Publish with us
- Sign up for alerts
- Open access
- Published: 14 May 2020
The TRUST Principles for digital repositories
- Dawei Lin ORCID: orcid.org/0000-0002-5506-0030 1 ,
- Jonathan Crabtree ORCID: orcid.org/0000-0002-0139-7025 2 ,
- Ingrid Dillo ORCID: orcid.org/0000-0001-5654-2392 3 ,
- Robert R. Downs ORCID: orcid.org/0000-0002-8595-5134 4 ,
- Rorie Edmunds 5 ,
- David Giaretta ORCID: orcid.org/0000-0001-8414-7509 6 ,
- Marisa De Giusti ORCID: orcid.org/0000-0003-2422-6322 7 ,
- Hervé L’Hours ORCID: orcid.org/0000-0001-5137-3032 8 ,
- Wim Hugo ORCID: orcid.org/0000-0002-0255-5101 9 ,
- Reyna Jenkyns ORCID: orcid.org/0000-0001-6975-6816 10 ,
- Varsha Khodiyar ORCID: orcid.org/0000-0002-2743-6918 11 ,
- Maryann E. Martone ORCID: orcid.org/0000-0002-8406-3871 12 ,
- Mustapha Mokrane ORCID: orcid.org/0000-0002-0925-7983 3 ,
- Vivek Navale ORCID: orcid.org/0000-0002-7110-8946 13 ,
- Jonathan Petters ORCID: orcid.org/0000-0002-0853-5814 14 ,
- Barbara Sierman ORCID: orcid.org/0000-0002-8190-3409 15 ,
- Dina V. Sokolova ORCID: orcid.org/0000-0001-8510-819X 16 ,
- Martina Stockhause ORCID: orcid.org/0000-0001-6636-4972 17 &
- John Westbrook ORCID: orcid.org/0000-0002-6686-5475 18
Scientific Data volume 7 , Article number: 144 ( 2020 ) Cite this article
41k Accesses
158 Citations
152 Altmetric
Metrics details
- Genetic databases
As information and communication technology has become pervasive in our society, we are increasingly dependent on both digital data and repositories that provide access to and enable the use of such resources. Repositories must earn the trust of the communities they intend to serve and demonstrate that they are reliable and capable of appropriately managing the data they hold.
Following a year-long public discussion and building on existing community consensus 1 , several stakeholders, representing various segments of the digital repository community, have collaboratively developed and endorsed a set of guiding principles to demonstrate digital repository trustworthiness. T ransparency, R esponsibility, U ser focus, S ustainability and T echnology: the TRUST Principles provide a common framework to facilitate discussion and implementation of best practice in digital preservation by all stakeholders.
Context and History
For over sixty years, digital data stewardship and preservation have been central to the mission of academic institutions such as libraries, archives, and domain repositories 2 with many other stakeholders involved, including researchers, funders, infrastructure, and service providers. Scientific data management is receiving increasing attention inside and outside of the scientific community, particularly in the contemporary Open Science discourse. Consensus on ‘good’ data management practice is beginning to form, but there is still insufficient implementation in some scientific domains.
The FAIR Data Principles 3 highlight the need to embrace good practice by defining essential characteristics of data objects to ensure that data are reusable by humans and machines: they should be F indable, A ccessible, I nteroperable, and R eusable, i.e. FAIR. However, to make data FAIR whilst preserving them over time requires trustworthy digital repositories (TDRs) with sustainable governance and organizational frameworks, reliable infrastructure, and comprehensive policies supporting community-agreed practices. TDRs, with their clear remit to actively preserve data in response to changes in both technology and stakeholder requirements, play an important role in maintaining the value of data. They are held in a position of trust by their users as they accept the responsibilities of data stewardship. To fulfill this role, TDRs must demonstrate essential and enduring capabilities necessary to enable access and reuse of data over time for the communities they serve. TDRs support data curation and preservation of data holdings with different levels of reusability. In certain instances, lower-quality data, which cannot reasonably be improved or made more interoperable, may still retain high value to its user community and so require trustworthy stewardship. A TDR must identify and seek to meet community-accepted criteria and communicate the achieved level of data quality.
The Open Archival Information System (OAIS) reference model 4 provides recommendations on setting up archives delivering long-term preservation of and access to information (in particular, digital information) and creating preservation packages. It offers a coherent and comprehensive framework of principles and terminology for the management of archival information systems. However, conforming to the OAIS reference model does not guarantee trustworthiness. In order to assess trustworthiness, additional elements of the repository need to be addressed, including appropriate governance, resources, and security. Furthermore, since OAIS is a reference model and does not provide a detailed implementation guideline, there are different interpretations and implementations necessitating audit and certification mechanisms as recognized in the 1996 report, Preserving Digital Information 5 . The authors of the report recommended that “repositories claiming to serve an archival function must be able to demonstrate that they are who they say they are by meeting or exceeding the standards and criteria of an independently-administered program for archival certification”.
Trustworthiness is demonstrated through evidence, which depends on transparency, and thus repositories must provide transparent, honest, and verifiable evidence of their practice. In this way, stakeholders can be confident that repositories ensure data integrity, authenticity, accuracy, reliability, and accessibility over extended time frames. Trustworthiness is not a one-off achievement; it cannot be taken for granted without regular audit and certification.
Certification makes an objective and important contribution to the confidence of the various stakeholders of a repository. To assess and improve the quality of their professional practices, repositories rely on a range of international certification standards covering core, extended or formal level certification. These standards such as the CoreTrustSeal 6 , DIN31644/NESTOR 7 , and ISO16363 8 focus on four major assessment areas: organization, digital object management, technical infrastructure, and security risk management. The standards vary in the number and complexity of their requirements, with the intensity of assessments ranging from a peer review of a self-assessment to a more involved on-site visit by an external audit team. The choice of certification mechanism depends on the need, willingness, and ability of a repository to invest in its further professionalization and trustworthiness.
The adoption of the CoreTrustSeal Trustworthy Data Repositories Requirements by many data repositories serves as an example of the improvements made to ensure that their capabilities attain the properties of the TRUST Principles 6 . Many data repositories have obtained CoreTrustSeal certification and become members of the International Science Council’s World Data System (WDS). The attainment of certification and the completion of audits by many digital repositories demonstrates the desire for repositories to be perceived as trustworthy.
Repository managers and their teams are the primary audience for the existing OAIS reference model and trustworthiness certification mechanisms discussed above. In an Open Science context, however, we expect that a broader audience, including funders and repository users, will benefit from the framework encapsulated by the TRUST Principles, especially given the increasing attention given to scientific data stewardship (Box 1 ).
Box 1 The TRUST Principles
Transparency.
In order to select the most appropriate repository for a particular use case, all potential users benefit from being able to easily find and access information on the scope, target user community, policies, and capabilities of the data repository. Transparency in these areas offers an opportunity to learn about the repository and consider its suitability for users’ specific requirements, including data deposition, data preservation, and data discovery. To be compliant with this principle, repositories should ensure that, at a minimum, the mission statement and scope of the repository are clearly stated. In addition, the following aspects should be transparently declared:
Terms of use, both for the repository and for the data holdings.
Minimum digital preservation timeframe for the data holdings.
Any pertinent additional features or services, for example the capacity to responsibly steward sensitive data.
Clearly communicating repository policies and, in particular, the terms of use for data holdings, informs users about any limitations that may restrict their use of the data or the repository. Likewise, being able to easily assess whether a repository can handle sensitive data in a responsible manner would also inform their decision on whether to utilize the available data services.
Responsibility
TRUSTworthy repositories take responsibility for the stewardship of their data holdings and for serving their user community. Responsibility is demonstrated by:
Adhering to the designated community’s metadata and curation standards, along with providing stewardship of the data holdings e.g. technical validation, documentation, quality control, authenticity protection, and long-term persistence.
Providing data services e.g. portal and machine interfaces, data download or server-side processing.
Managing the intellectual property rights of data producers, the protection of sensitive information resources, and the security of the system and its content.
Repository users should have confidence that data depositors are prompted to provide all metadata compliant with the community norms, as this greatly enhances the discoverability and usefulness of the data. Knowing that a repository verifies the integrity of available data and metadata assures potential users that the data holdings are more likely to be interoperable with other relevant datasets. Both depositors and users must have confidence that the data will remain accessible over time, and thus can be cited and referenced in scholarly publications.
Responsibility may be clarified through some legal means (right to preserve) or may take the form of voluntary compliance with some norm (ethical standards).
A TRUSTworthy repository needs to focus on serving its target user community. Each user community likely has differing expectations from their community repositories, depending in part on the community’s maturity regarding data management and sharing. A TRUSTworthy repository is embedded in its target user community’s data practices, and so can respond to evolving community requirements. We take a broad view of ‘user community’ as these could include users depositing or accessing data; those accessing data holdings computationally; and indirect stakeholders such as funders, journal editors, other institutional partners or citizens.
Use and reuse of research data is an integral part of the scientific process, and therefore TRUSTworthy repositories should enable their community to find, explore, and understand their data holdings with regard to potential (re)use. Repositories should encourage users to fully describe data at the time of deposition and facilitate feedback on any issues with the data (e.g. quality or fitness for use) that may become apparent after the data have been made available.
Repositories have a vital role in applying and enforcing the target user community norms and standards as compliance facilitates data interoperability and reusability. Data standards that TRUSTworthy repositories should enforce include metadata schema, data file formats, controlled vocabularies, ontologies, and other semantics where these exist in the user community. A TRUSTworthy repository may demonstrate adherence to this principle by:
Implementing relevant data metrics and making these available to users.
Providing (or contributing to) community catalogues to facilitate data discovery.
Monitoring and identifying evolving community expectations and responding as required to meet these changing needs.
Sustainability
Ensuring sustainability of a TRUSTworthy repository is necessary to ensure uninterrupted access to its valuable data holdings for current and future user communities. Continued access to data is dependent upon the ability of the repository to provide services over time, and to respond with new or improved services to meet evolving user community requirements.
A TRUSTworthy repository may demonstrate the sustainability of its holdings by:
Planning sufficiently for risk mitigation, business continuity, disaster recovery, and succession.
Securing funding to enable ongoing usage and to maintain the desirable properties of the data resources that the repository has been entrusted with preserving and disseminating.
Providing governance for necessary long-term preservation of data so that data resources remain discoverable, accessible, and usable in the future.
A repository depends on the interaction of people, processes, and technologies to support secure, persistent, and reliable services. Its activities and functions are supported by software, hardware, and technical services. Together, these provide the tools to enable the delivery of the TRUST Principles.
A TRUSTworthy repository may demonstrate the fitness of its technological capabilities by:
Implementing relevant and appropriate standards, tools, and technologies for data management and curation.
Having plans and mechanisms in place to prevent, detect, and respond to cyber or physical security threats.
Impact of the TRUST Principles
The TRUST Principles in their abstract, non-technical formulation facilitate communication and thus impact stakeholders both within and outside the data user community. When data repositories, funders, and data creators adopt FAIR Principles and implement the TRUST Principles, repository users benefit directly through continuing and improved capabilities for efficient and effective use of data. Together, the stakeholders of the TRUST Principles contribute to a cultural change in research towards a data and information ecosystem that has been evolving during the information age but has been an essential part of the scientific process for centuries.
Various studies have found that transparency is associated with trust of digital repositories 9 . For example, for users of video data, “transparency of repository practices, and especially data curation practices, are important for trust” 10 . Studying the data repository staff perceptions of repository certification, Donaldson, et al . 11 , found that the process of acquiring certification contributed to the transparency of their repository, among other benefits.
The OAIS Reference Model describes the responsibilities of archival information systems that are entrusted with the stewardship of information resources. Describing challenges of effective data stewardship, Peng et al . 12 stated that “Defining roles and responsibilities in every level of stewardship and every stage of the data product lifecycle will help facilitate this challenge”. Furthermore, upon surveying research data practices throughout the data lifecycle, Kowalczyk 13 reported that “[t]he probability of long-term data management for research collections is low when the ongoing responsibility lies with an individual researcher or graduate student”.
Studying how users’ experiences influenced their perceptions of trust in data repositories, Yoon 14 found that “users’ awareness of repositories’ roles or functions can be one factor for developing users’ trust”. Users often trust repositories based on their own experiences, repository practices and reputation, and on the experiences of other community members 9 , 14 , 15 . Users’ trust in data is also associated with their trust in the archive from which the content was obtained 16 .
The report of a study on the sustainability of digital repositories that was conducted by the Organization for Economic Co-operation and Development (OECD) concluded that “Research data repositories are an essential part of the infrastructure for open science…” [and that it] “is important to ensure the sustainability of research data repositories” 17 . The importance of the sustainability of research data infrastructure has been identified in studies describing the needs of archaeologists 9 , 18 . In the absence of effective sustainability strategies and continuity plans, data repositories and their holdings could disappear, like many former biological databases 19 . Ironically, York et al . 20 observed that “despite the large number of data repositories, stewardship initiatives, and policies across the research data landscape, we know relatively little about the total amount, characteristics, or sustainability of stewarded research data”.
The adoption of technological capabilities should be completed in conjunction with the organizational, managerial and stewardship capabilities that facilitate the continuing use of a data repository’s holdings 10 , 21 . Describing the needs for earning public trust of health data, Van Staa et al . 22 called for capabilities that would “combine new technologies with clear accountability, transparent operations, and public trust …”, stating that “data stewardship is not just about physical and digital security: staff training, standard operating procedures, and the skills and attitudes of staff are also important” 22 .
Conclusions
The TRUST Principles provide a mnemonic to remind data repository stakeholders of the need to develop and maintain the infrastructure to foster continuing stewardship of data and enable future use of their data holdings. The TRUST Principles, however, are not an end in themselves, rather a means to facilitate communication with all stakeholders, providing repositories with guidance to demonstrate transparency, responsibility, user focus, sustainability, and technology.
RDA/WDS Certification of Digital Repositories IG. The TRUST Principles for Trustworthy Data Repositories – An Update. Research Data Alliance (RDA) , https://www.rd-alliance.org/trust-principles-trustworthy-data-repositories-–-update (2019).
Mokrane, M. & Parsons, M. Learning from the International Polar Year to Build the Future of Polar Data Management. Data Sci. J. 13 , IFPDA–15 (2014).
Article Google Scholar
Wilkinson, M. D. et al . The FAIR Guiding Principles for scientific data management and stewardship. Sci. Data 3 , 160018 (2016).
Consultative Committee for Space Data Systems. Reference Model for an Open Archival Information System (OAIS). Recommended Practice CCSDS 650.0-M-2. Consultative Committee for Space Data Systems , https://public.ccsds.org/Pubs/650x0m2.pdf (2012).
Waters, D. & Garrett, J. Preserving Digital Information, Report of the Task Force on Archiving of Digital Information . 1400 16th St., NW, Suite 740, Washington, DC 20036-2217. 59 pp, https://www.clir.org/pubs/reports/pub63/ (1996).
CoreTrustSeal. CoreTrustSeal Certified Repositories. CoreTrustSeal , https://www.coretrustseal.org/why-certification/certified-repositories/ (2020).
Harmsen, H. et al . Explanatory notes on the Nestor seal for trustworthy digital archives. Nestor Certification Working Group , http://nbn-resolving.de/urn:nbn:de:0008-2013100901 (2013).
Audit and Certification of Trustworthy Digital Repositories. ISO 16363/CCSDS 652.0-M-1, https://public.ccsds.org/Pubs/652x0m1.pdf (2011).
Yakel, E., Faniel, I. M., Kriesberg, A. & Yoon, A. Trust in Digital Repositories. Int. J. Digit. Curation 8 , 143–156 (2013).
Frank, R. D., Chen, Z., Crawford, E., Suzuka, K. & Yakel, E. Trust in qualitative data repositories. In Proceedings of the Association for Information Science and Technology 54 102–111 Association for Information Science and Technology (2017).
Donaldson, D. R., Dillo, I., Downs, R. & Ramdeen, S. The Perceived Value of Acquiring Data Seals of Approval. Int. J. Digit. Curation 12 , 130–151 (2017).
Peng, G. et al . A Conceptual Enterprise Framework for Managing Scientific Data Stewardship. Data Sci. J. 17 , 15 (2018).
Kowalczyk, S. T. Modelling the Research Data Lifecycle. Int. J. Digit. Curation 12 , 331–361 (2017).
Yoon, A. End users’ trust in data repositories: definition and influences on trust development. Arch. Sci. 14 , 17–34 (2014).
Downs, R. & Chen, R. Organizational needs for managing and preserving geospatial data and related electronic records. Data Sci. J. 4 , 255–271 (2006).
Donaldson, D. R. Trust in Archives–Trust in Digital Archival Content Framework. Archivaria 88 , 50–83 (2019).
Google Scholar
OECD. Business models for sustainable research data repositories . 58 , https://doi.org/10.1787/302b12bb-en (2017).
Williams, J. P. & Williams, R. D. Information science and North American archaeology: examining the potential for collaboration. Inf. Res . 24 , paper 820. Retrieved from, http://InformationR.net/ir/24-2/paper820.html (Archived by WebCite® at, http://www.Webcitation.Org/78mnvhrti ) (2019).
Attwood, T. K., Agit, B. & Ellis, L. B. M. Longevity of Biological Databases. EMBnet. journal 21 , 803 (2015).
York, J., Gutmann, M. & Berman, F. What Do We Know about the Stewardship Gap. Data Sci. J. 17 , 19 (2018).
Corrado, E. M. Repositories, Trust, and the CoreTrustSeal. Tech. Serv. Q. 36 , 61–72 (2019).
Staa, T.-P., van, Goldacre, B., Buchan, I. & Smeeth, L. Big health data: the need to earn public trust. BMJ 354 , i3636 (2016).
Download references
Acknowledgements
The authors very much appreciate the suggestions for improving this work that were offered by the members of the CoreTrustSeal Standards and Certification Board who did not contribute as authors, by participants of the Research Data Alliance Plenary 13 session, “Build TRUST to be FAIR - Emerging Needs of Certification in Life Sciences, Geosciences and Humanities”, which was convened by the RDA/WDS Certification of Digital Repositories Interest Group, and by participants of the NIH Workshop on Trustworthy Data Repositories for Biomedical Sciences (NIH Workshop, 2019) sponsored by NIH Office of Data Science Strategy, the first instance the TRUST framework was used to discuss trustworthy data repositories. We are grateful for thoughtful discussions with Shelley Stall, Robert S. Chen, Mark Conrad, Peter Doorn, Eliane Fankhauser, Elizabeth Hull, Siri Jodha Singh Khalsa, Micky Lindlar, Limor Peer, Philipp Conzett, and Rachel Drysdale. We would like to thank Anupama Gururaj for proof-reading the article.
Author information
Authors and affiliations.
Division of Allergy, Immunology, and Transplantation, National Institute of Allergy and Infectious Diseases, National Institutes of Health, Maryland, USA
HW Odum Institute for Research in Social Science, University of North Carolina at Chapel Hill, North Carolina, USA
Jonathan Crabtree
Data Archiving and Networked Services (DANS), The Hague, The Netherlands
Ingrid Dillo & Mustapha Mokrane
Center for International Earth Science Information Network (CIESIN), The Earth Institute, Columbia University, New York, USA
Robert R. Downs
World Data System of the International Science Council (WDS), WDS International Programme Office, Tokyo, Japan
Rorie Edmunds
PTAB Ltd, Dorset, UK
David Giaretta
Universidad Nacional de La Plata, Comisión de Investigaciones Científicas de la Provincia de Buenos Aires, La Plata, Argentina
Marisa De Giusti
UK Data Archive, UK Data Service, University of Essex, Colchester, UK
Hervé L’Hours
South African Environmental Observation Network, Cape Town, South Africa
Ocean Networks Canada, University of Victoria, Victoria, Canada
Reyna Jenkyns
Springer Nature, London, UK
Varsha Khodiyar
University of California, San Diego, California, USA and SciCrunch Inc., San Diego, USA
Maryann E. Martone
Center for Information Technology, National Institutes of Health, Maryland, USA
Vivek Navale
Data Services, University Libraries, Virginia Tech, Virginia, USA
Jonathan Petters
KB National Library of the Netherlands, The Hague, The Netherlands
Barbara Sierman
University Libraries, Columbia University, New York, USA
Dina V. Sokolova
German Climate Computing Center (DKRZ), Hamburg, Germany
Martina Stockhause
RCSB, Protein Data Bank, Rutgers, The State University of New Jersey, Institute for Quantitative Biomedicine at Rutgers, New Jersey, USA
John Westbrook
You can also search for this author in PubMed Google Scholar
Corresponding author
Correspondence to Dawei Lin .
Ethics declarations
Competing interests.
V.K.K. works for Springer Nature, the publishers of Scientific Data . Until February 2020, VKK held an editorial position at Scientific Data . The authors declare that V.K.K. was not involved in the editorial and refereeing process for this manuscript. Several of the authors are involved in the standards and certification efforts discussed in the manuscript, including D.L., J.C., I.D., R.R.D., R.E., H.L.H., W.H., R.J., and M.M., who are members of the CoreTrustSeal Standards and Certification Board and DG, who is a member of the Primary Trustworthy Digital Repository Authorization Body (PTAB). All other authors declare no competing interests.
Additional information
Publisher’s note Springer Nature remains neutral with regard to jurisdictional claims in published maps and institutional affiliations.
Rights and permissions
Open Access This article is licensed under a Creative Commons Attribution 4.0 International License, which permits use, sharing, adaptation, distribution and reproduction in any medium or format, as long as you give appropriate credit to the original author(s) and the source, provide a link to the Creative Commons license, and indicate if changes were made. The images or other third party material in this article are included in the article’s Creative Commons license, unless indicated otherwise in a credit line to the material. If material is not included in the article’s Creative Commons license and your intended use is not permitted by statutory regulation or exceeds the permitted use, you will need to obtain permission directly from the copyright holder. To view a copy of this license, visit http://creativecommons.org/licenses/by/4.0/ .
Reprints and permissions
About this article
Cite this article.
Lin, D., Crabtree, J., Dillo, I. et al. The TRUST Principles for digital repositories. Sci Data 7 , 144 (2020). https://doi.org/10.1038/s41597-020-0486-7
Download citation
Received : 06 March 2020
Accepted : 22 April 2020
Published : 14 May 2020
DOI : https://doi.org/10.1038/s41597-020-0486-7
Share this article
Anyone you share the following link with will be able to read this content:
Sorry, a shareable link is not currently available for this article.
Provided by the Springer Nature SharedIt content-sharing initiative
This article is cited by
Association between ehealth literacy and health outcomes in german athletes using the gr-eheals questionnaire: a validation and outcome study.
- Sheila Geiger
- Anna Julia Esser
- Alexander Bäuerle
BMC Sports Science, Medicine and Rehabilitation (2024)
Semantic units: organizing knowledge graphs into semantically meaningful units of representation
- Tobias Kuhn
- Robert Hoehndorf
Journal of Biomedical Semantics (2024)
Biomedical Data Repository Concepts and Management Principles
- Matthew McAuliffe
- Susan N. Wright
Scientific Data (2024)
The O3 guidelines: open data, open code, and open infrastructure for sustainable curated scientific resources
- Charles Tapley Hoyt
- Benjamin M. Gyori
A maturity model for catalogues of semantic artefacts
- Oscar Corcho
- Fajar J. Ekaputra
- Emanuele Storti
Quick links
- Explore articles by subject
- Guide to authors
- Editorial policies
Sign up for the Nature Briefing newsletter — what matters in science, free to your inbox daily.

Share, find and cite research data produced at Arizona State University.
The Arizona State University (ASU) Research Data Repository provides a platform for ASU-affiliated researchers to share, preserve, cite, and make research data accessible and discoverable. The ASU Research Data Repository provides a permanent digital identifier for research data, which complies with data sharing policies. The repository is powered by the Dataverse open-source application, developed and used by Harvard University. Both the ASU Research Data Repository and the KEEP Institutional Repository are managed by the ASU Library to ensure research produced at Arizona State University is discoverable and accessible to the global community.
In order to use this feature you must have at least one published or linked dataverse.
Are you sure you want to publish your dataverse? Once you do so it must remain published.
This dataverse cannot be published because the dataverse it is in has not been published.
Are you sure you want to delete your dataverse? You cannot undelete this dataverse.
- Research Project (8)
- Research Group (6)
- Researcher (4)
- Laboratory (3)
- Department (2)
- CC0 1.0 (55)
- Custom Terms (13)
- CC BY 4.0 (8)
- Meerow, Sara (8)
- Pendyala, Ram (6)
- Salon, Deborah (6)
- Simeone, Michael (6)
- Roy, Malini (5)
- Social Sciences (42)
- Medicine, Health and Life Sciences (36)
- Engineering (16)
- Computer and Information Science (13)
- Earth and Environmental Sciences (10)
- Animal Shelters (4)
- Animal Welfare (4)
- Human-animal Interaction (4)
- flooding (4)
Filter Results
Jun 17, 2024 - , ASU Library Research Data Repository, V1, UNF:6:SLTfKkxHbIrYr2g2fRKYgA== [fileUNF] Replication data for the manuscript titled "Raman thermometry for temperature assessment of inorganic transformations under microwave heating" for submission to the Journal of Raman Spectroscopy. The raw experimental data and MATLAB (R2024) scripts for analysis are included for v... |
Jun 17, 2024 - , ASU Library Research Data Repository, V1, UNF:6:6QmgMUd1OMhlJMSnH2JgWg== [fileUNF] The data are project results for current and future post-fire debris flow assessment of the state of California. The project model was developed largely in 2019-2020 using current and future fire and precipitation data from CalAdapt, USGS watershed geography and geologic characte... |
Jun 13, 2024 - , ASU Library Research Data Repository, V1 Dataset of manuscript, SLEAPing with cockroaches: low-cost approaches to teaching machine learning in neuroscience. |
(Arizona State University) Jun 13, 2024 |
Jun 13, 2024 - , ASU Library Research Data Repository, V1, UNF:6:o4iDo4neMSlg6IY9o9eEJQ== [fileUNF] The San Francisco Bay Area is one of the most progressive transportation regions in the deployment of high-capacity transit and the use of policies to encourage active transportation. Yet, there remains a dearth of knowledge on the abundance and location of parking infrastructure... |
May 16, 2024 - , ASU Library Research Data Repository, V1, UNF:6:Db7BzVSCZ5lO56FQg9rFGg== [fileUNF] A parking inventory for metropolitan Phoenix, Arizona, USA is developed by cross-referencing geospatial cadastral and roadway data with minimum parking requirements. Historical growth of parking is also estimated by linking year of property development to required off-street and... |
May 6, 2024 - , ASU Library Research Data Repository, V1, UNF:6:X1KQlMUspW62ALKL6kRxXA== [fileUNF] In 2019, four universities comprising the TOMNET (Transformative Transportation Technologies) and D-STOP Tier 1 University Transportation Centers, namely, Arizona State University, Georgia Tech, The University of Texas at Austin, and University of South Florida, conducted a surve... |
(Arizona State University) May 6, 2024 |
Apr 29, 2024 - , ASU Library Research Data Repository, V1, UNF:6:rP9zyE6Tz3ohv1Amr60GZQ== [fileUNF] Replication data for the 2015 article "Parking Infrastructure: A Constraint on or Opportunity for Urban Redevelopment? A Study of Los Angeles County Parking Supply and Growth." We estimate how parking has grown in Los Angeles County from 1950 to 2010. We find that since 1975 the... |
(Arizona State University) Apr 29, 2024 |
- < Previous
- 1 (Current)
Log in to create a dataverse or add a dataset.
Share this dataverse on your favorite social media networks.
Are you sure you want to reset the selected metadata fields? If you do this, any customizations (hidden, required, optional) you have done will no longer appear.
ASU Library Research Data Repository Support
Please fill this out to prove you are not a robot.
The Qualitative Data Repository
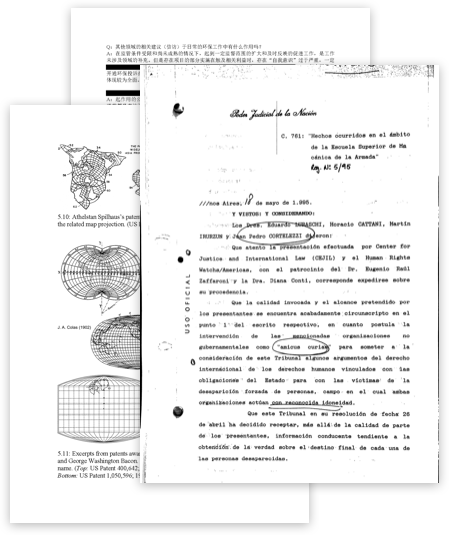
Featured Data Projects
Managing data.
QDR offers a series of web pages addressing key topics in managing research data from data management planning to formatting and depositing data, all with a focus on the needs of qualitative researchers.
Types of Qualitative Data
Qualitative data can take many different forms. This is a non-exhaustive list of the types of materials that can make up a qualitative data project.
Deposit Process
How do you deposit data with QDR? Learn about the steps of a data deposit, deposit fees and waivers, and typically turnaround times.
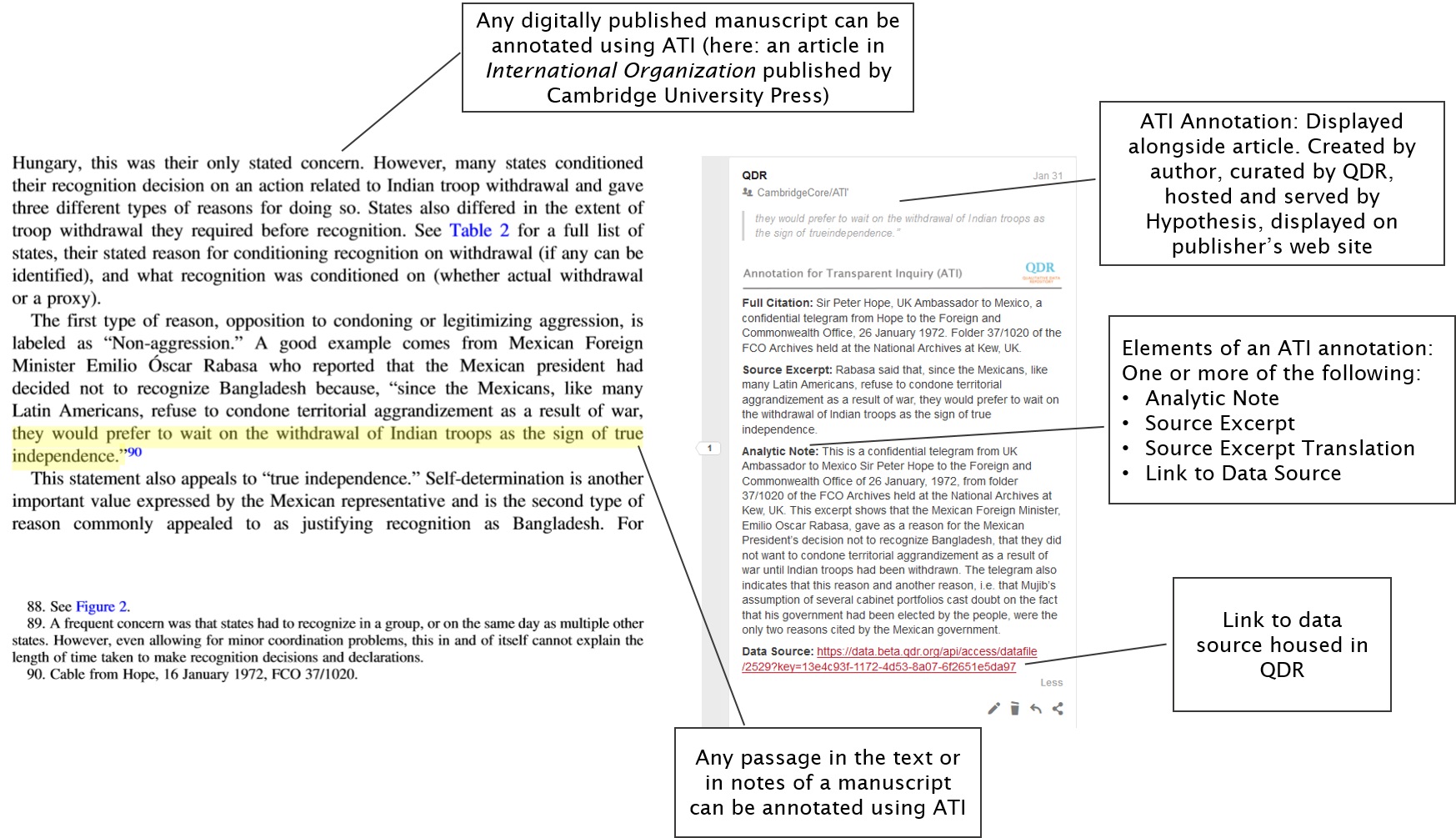
Annotation for Transparent Inquiry
Annotation for Transparent Inquiry (ATI) facilitates transparency in qualitative research by allowing scholars to “annotate” specific passages in an article. Annotations amplify the text and, when possible, include a link to one or more data sources underlying a claim; data sources are housed in a repository.
Learn more about ATI ‣
Sharing data and its documentation for secondary analysis
Empowering qualitative and multi-method inquiry through guidance and consultation
Providing data and materials to enrich and enliven teaching
Developing innovative approaches for enriching publications with data and analysis
Our Mission
QDR curates, stores, preserves, publishes, and enables the download of digital data generated through qualitative and multi-method research in the social sciences. The repository develops and disseminates guidance for managing, sharing, citing, and reusing qualitative data, and contributes to the generation of common standards for doing so. QDR’s overarching goals are to make sharing qualitative data customary in the social sciences, to broaden access to social science data, and to strengthen qualitative and multi-method research.
Learn more about us ‣

An official website of the United States government
Here's how you know
The .gov means it’s official. Federal government websites often end in .gov or .mil. Before sharing sensitive information, make sure you’re on a federal government site.
The site is secure. The https:// ensures that you are connecting to the official website and that any information you provide is encrypted and transmitted securely.
Finding Datasets, Data Repositories, and Data Standards
This online guide contains resources for finding data repositories for data preservation and access and locating datasets for reuse. The guide was developed as an online companion for the class Resources for Finding and Sharing Research Data . If you are NIH or HHS staff, please check out the NIH Library training schedule for upcoming classes.
If you need a one-on-one or group consultation on locating data repositories and datasets, please contact the NIH Library .
Some content of this guide is adapted from:
- Read, Kevin; Surkis, Alisa (2018): Research Data Management Teaching Toolkit. figshare. ( https://figshare.com/articles/Research_Data_Management_Teaching_Toolkit/5042998 ) This work is licensed under Attribution 4.0 International (CC BY 4.0).
Navigation:
Resources to Locate Data Repositories
Resources for data sharing for intramural nih researchers, issues to consider with data repositories, searching across data repositories, generalist repositories, data journals, databases linked to datasets, issues to consider with datasets, data standards and common data elements (cdes), data repositories.
- Domain-specific repositories
- Generalist repositories
- Information from the BMIC tables described above, listing repositories for sharing scientific data and repositories for accessing scientific data , can also be found at Sharing.nih.gov .
- The portal covers data registries from across many academic disciplines.
- Users can search by keyword or browse repositories by subject , content type , or country .
- Choose Databases to search and browse data repositories.
- Choose Collections to view data repositories, standards, and policies related to various topics.
- Submit a Data Management and Sharing plan (DMSP) outlining how scientific data and any accompanying metadata will be managed and shared, taking into account any potential restrictions or limitations.
- Comply with the Data Management and Sharing plan approved by the funding Institute or Center (IC).
- Data Management & Sharing Policy Overview : Learn more about the 2023 Data Management & Sharing Policy, and find resources to assist with compliance.
- Allowable Costs for Data Management and Sharing
- Elements of an NIH Data Management and Sharing Plan
- Selecting a Repository for Data Resulting from NIH-Supported Research
- Protecting Privacy When Sharing Human Research Participant Data
- Responsible Management and Sharing of American Indian/Alaska Native Participant Data
- Research associated with a ZIA
- Research associated with a clinical protocol that will undergo IC Initial Scientific Review
- The plans will address the elements indicated in the Intramural Research Program Data Management and Sharing (IRP DMS) Plan template. The template addresses six NIH-recommended core elements , and allows for the inclusion of IC-specific elements: Intramural Data Management and Sharing Plan Template (PDF)
- See the 2023 NIH Data Management and Sharing Policy page in the OIR Sourcebook for additional guidance and resources.
- See the library guide Data Management and Sharing Plan Resources for a detailed list of DMSP resources and IC-specific contacts.
- Genomic Data Sharing Policy
- NIH Institute and Center Data Sharing Policies
- Intramural Human Data Sharing Policy
- Other Sharing Policies
- Find more information on Intramural Data Sharing from the NIH Office of Intramural Research.
- Visit Sharing.nih.gov for guidance on Selecting a Data Repository and a list of potential Repositories for Sharing Scientific Data .
Issues to consider when finding a data repository to preserve and share data:
- Required Repositories: Check the funder/publisher policies to see if there are required repositories where the data must be deposited.
- You may need to anonymize and/or aggregate the data before sharing, or access to the data may need to be limited to researchers with specific permissions.
- Intellectual Property: Be aware of who owns the intellectual property and if there are any licensing restrictions.
- Required Data Standards: Be aware of the data standards (such as metadata and data formats) required for depositing the data in the repository.
- Deposit and Storage Costs: Be aware of any costs associated with depositing/storing the data.
Find additional guidance at Sharing.nih.gov for Selecting a Data Repository .
- Indexes datasets using the metadata descriptions that come directly from the dataset web pages using schema.org structure.
- Contains more than 31 million datasets from more than 4,600 internet domains.
- About half of these datasets come from .com domains, but .org and governmental domains also well represented.
- Dataset results are now also listed in general Google search results, according to February 2023 blog post .
- Filter results by date range, data type, source type (article or data repository), and source.
- NLM also offers Center for Clinical Observational Investigations (CCOI) Dataset Profiles , for exploring large-scale clinical datasets
Here’s a closer look at a few major cross-disciplinary repositories highlighted on the NIH Data Sharing Resources: Generalist Repositories page.
- Browse or search and filter datasets by geographical location, subject, journal, or institution.
- Filter by Item Type: Dataset.
- Filter by Type: Dataset to view only dataset results.
The NIH Office of Data Science Strategy (ODSS) announced the Generalist Repository Ecosystem Initiative (GREI) , which includes seven established generalist repositories that will work together to establish consistent metadata, develop use cases for data sharing, train and educate researchers on FAIR data and the importance of data sharing, and more. A series of recorded webinars is offered to learn about GREI and generalist repositories.
- Some will also store the dataset.
- Others provide recommendations of where to store the data.
- Usually peer-reviewed.
- GigaScience : An open access, open data, open peer-review journal from Oxford University Press focusing on “big data” research from the life and biomedical sciences.
- Scientific Data : Scientific Data is a peer-reviewed, open-access journal from Springer Nature that publishes descriptions of scientifically valuable datasets and research that advances the sharing and reuse of scientific data.
- Sources of Dataset Peer Review : University of Edinburgh maintains a list of peer-reviewed data publications.
- The EU-funded FOSTER portal (e-learning platform for training resources on Open Science) provides a list of Open Data Journals .
- Walters, William H. 2020. “ Data Journals: Incentivizing Data Access and Documentation Within the Scholarly Communication System ”. Insights 33 (1): 18. DOI: http://doi.org/10.1629/uksg.510 : Provides list of data journals.
- PubMed : Use the filter option “Article Attribute” > “Associated Data” to only view results with related data links. Data filters were originally added to PubMed and PubMed central in 2018.
- Web of Science : When viewing search results in Web of Science (All Databases), choose the Associated Data option under Quick Filters to view only search results that mention a data set, data study, or data repository in the Data Citation Index . The Data Citation Index includes records on over 14 million research data sets, 1.6 million data studies, and 405 thousand software from over 450 international data repositories in the sciences, social sciences, and arts and humanities.
Issues to consider when re-using datasets include:
- Who is the author of the dataset? What is their institutional affiliation?
- Is there a peer-reviewed publication associated with the dataset?
- Licensing : Check any license restrictions for the data. Many repositories will list the type of license the data is covered by (usually Creative Commons or Open Data Commons licenses ).
- Use the format defined by a style guide, like APA (See APA style manual examples for datasets ).
- In EndNote, you can define a reference as a dataset. EndNote will then format the reference into the correct dataset citation format for the selected style.
- Learn more: NYU Libraries, Data Sources: How to Cite Data & Statistics
See the ELIXIR Research Data Management Kit (RDMkit) guide on Existing Data for additional considerations and resources when locating existing datasets for reuse.
Data/metadata standards and CDEs can help to make data more FAIR (findable, accessible, interoperable, and re-usable – see FORCE11 The FAIR Data Principles ).
- DCC Disciplinary Metadata : Collections of metadata standards organized by discipline.
- FAIRsharing.org : An online catalog that includes over 1750 data and metadata standards.
- NIH CDE Repository : The NIH Common Data Elements (CDE) Repository provides access to structured human and machine-readable definitions of data elements that have been recommended or required by NIH Institutes and Centers and other organizations for use in research and for other purposes.
.png)
How to build a research repository: a step-by-step guide to getting started
Research repositories have the potential to be incredibly powerful assets for any research-driven organisation. But when it comes to building one, it can be difficult to know where to start. In this post, we provide some practical tips to define a clear vision and strategy for your repository.
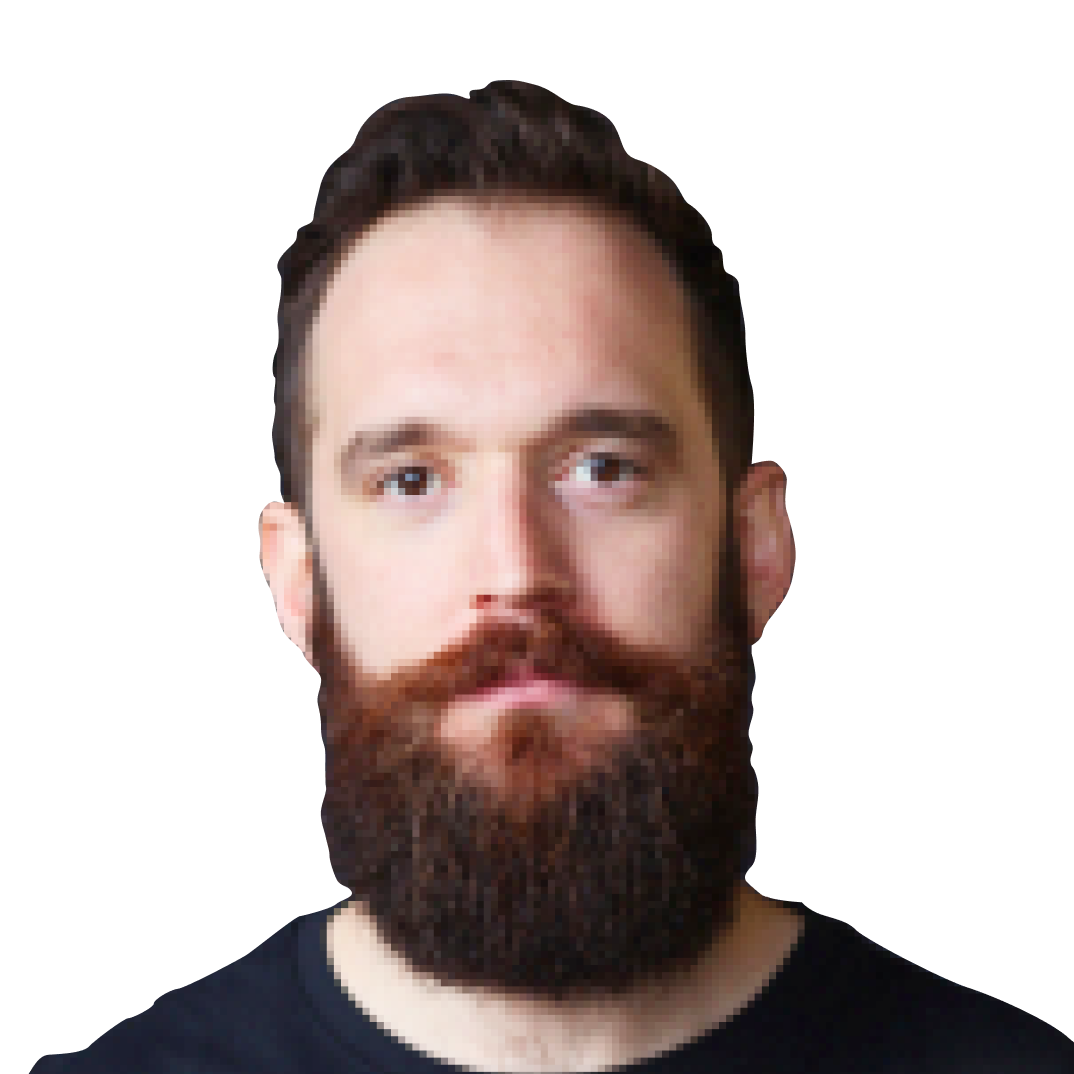
Done right, research repositories have the potential to be incredibly powerful assets for any research-driven organisation. But when it comes to building one, it can be difficult to know where to start.
As a result, we see tons of teams jumping in without clearly defining upfront what they actually hope to achieve with the repository, and ending up disappointed when it doesn't deliver the results.
Aside from being frustrating and demoralising for everyone involved, building an unused repository is a waste of money, time, and opportunity.
So how can you avoid this?
In this post, we provide some practical tips to define a clear vision and strategy for your repository in order to help you maximise your chances of success.
🚀 This post is also available as a free, interactive Miro template that you can use to work through each exercise outlined below - available for download here .
Defining the end goal for your repository
To start, you need to define your vision.
Only by setting a clear vision, can you start to map out the road towards realising it.
Your vision provides something you can hold yourself accountable to - acting as a north star. As you move forward with the development and roll out of your repository, this will help guide you through important decisions like what tool to use, and who to engage with along the way.
The reality is that building a research repository should be approached like any other product - aiming for progress, over perfection with each iteration of the solution.
Starting with a very simple question like "what do we hope to accomplish with our research repository within the first 12 months?" is a great starting point.
You need to be clear on the problems that you’re looking to solve - and the desired outcomes from building your repository - before deciding on the best approach.
Building a repository is an investment, so it’s important to consider not just what you want to achieve in the next few weeks or months, but also in the longer term to ensure your repository is scalable.
Whatever the ultimate goal (or goals), capturing the answer to this question will help you to focus on outcomes over output .
🔎 How to do this in practice…
1. complete some upfront discovery.
In a previous post we discussed how to conduct some upfront discovery to help with understanding today’s biggest challenges when it comes to accessing and leveraging research insights.
⏰ You should aim to complete your upfront discovery within a couple of hours, spending 20-30 mins interviewing each stakeholder (we recommend talking with at least 5 people, both researchers and non-researchers).
2. Prioritise the problems you want to solve
Start by spending some time reviewing the current challenges your team and organisation are facing when it comes to leveraging research and insights.
You can run a simple affinity mapping exercise to highlight the common themes from your discovery and prioritise the top 1-3 problems that you’d like to solve using your repository.
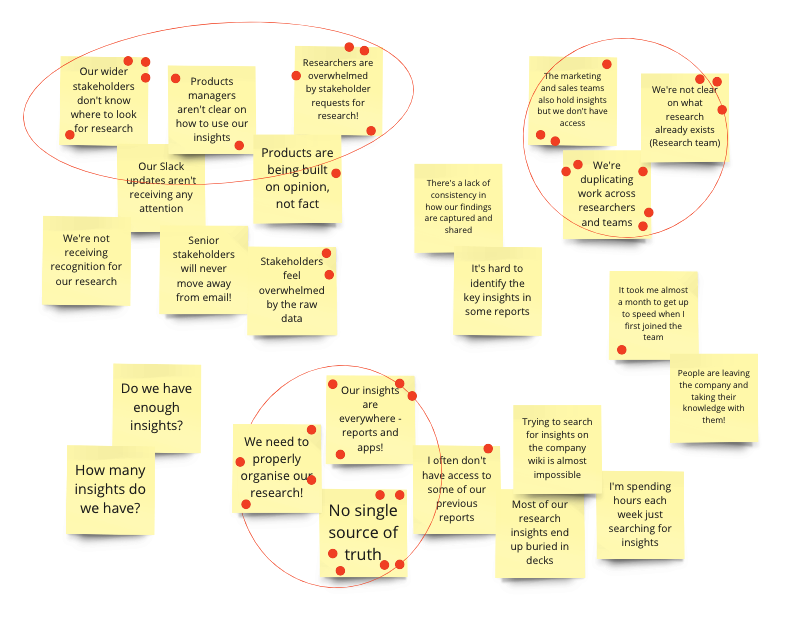
💡 Example challenges might include:
Struggling to understand what research has already been conducted to-date, leading to teams repeating previous research
Looking for better ways to capture and analyse raw data e.g. user interviews
Spending lots of time packaging up research findings for wider stakeholders
Drowning in research reports and artefacts, and in need of a better way to access and leverage existing insights
Lacking engagement in research from key decision makers across the organisation
⏰ You should aim to confirm what you want to focus on solving with your repository within 45-60 mins (based on a group of up to 6 people).
3. Consider what future success looks like
Next you want to take some time to think about what success looks like one year from now, casting your mind to the future and capturing what you’d like to achieve with your repository in this time.
A helpful exercise is to imagine the headline quotes for an internal company-wide newsletter talking about the impact that your new research repository has had across the business.
The ‘ Jobs to be done ’ framework provides a helpful way to format the outputs for this activity, helping you to empathise with what the end users of your repository might expect to experience by way of outcomes.

💡 Example headlines might include:
“When starting a new research project, people are clear on the research that’s already been conducted, so that we’re not repeating previous research” Research Manager
“During a study, we’re able to quickly identify and share the key insights from our user interviews to help increase confidence around what our customers are currently struggling with” Researcher
“Our designers are able to leverage key insights when designing the solution for a new user journey or product feature, helping us to derisk our most critical design decisions” Product Design Director
“Our product roadmap is driven by customer insights, and building new features based on opinion is now a thing of the past” Head of Product
“We’ve been able to use the key research findings from our research team to help us better articulate the benefits of our product and increase the number of new deals” Sales Lead
“Our research is being referenced regularly by C-level leadership at our quarterly townhall meetings, which has helped to raise the profile of our team and the research we’re conducting” Head of Research
Ask yourself what these headlines might read and add these to the front page of a newspaper image.
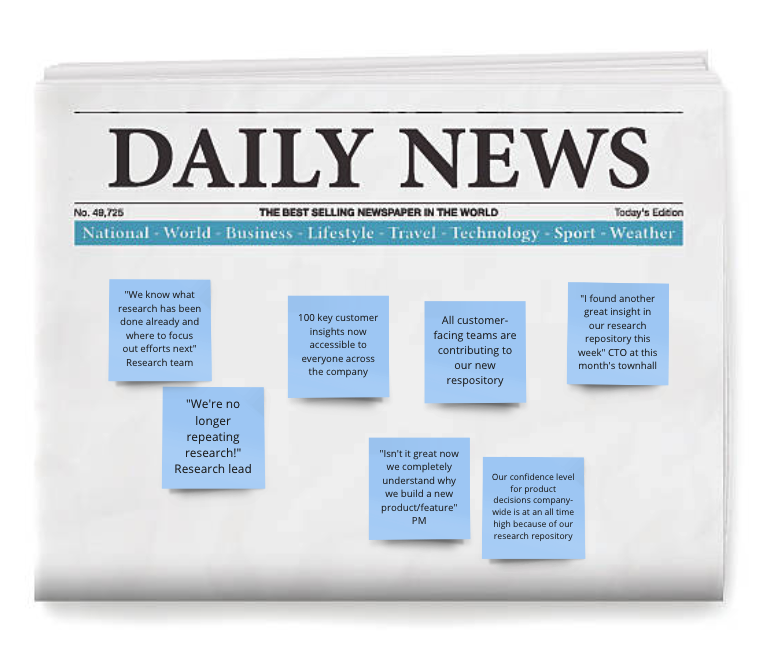
You then want to discuss each of these headlines across the group and fold these into a concise vision statement for your research repository - something memorable and inspirational that you can work towards achieving.
💡Example vision statements:
‘Our research repository makes it easy for anyone at our company to access the key learnings from our research, so that key decisions across the organisation are driven by insight’
‘Our research repository acts as a single source of truth for all of our research findings, so that we’re able to query all of our existing insights from one central place’
‘Our research repository helps researchers to analyse and synthesise the data captured from user interviews, so that we’re able to accelerate the discovery of actionable insights’
‘Our research repository is used to drive collaborative research across researchers and teams, helping to eliminate data silos, foster innovation and advance knowledge across disciplines’
‘Our research repository empowers people to make a meaningful impact with their research by providing a platform that enables the translation of research findings into remarkable products for our customers’
⏰ You should aim to agree the vision for your repository within 45-60 mins (based on a group of up to 6 people).
Creating a plan to realise your vision
Having a vision alone isn't going to make your repository a success. You also need to establish a set of short-term objectives, which you can use to plan a series of activities to help you make progress towards this.
Focus your thinking around the more immediate future, and what you want to achieve within the first 3 months of building your repository.
Alongside the short-term objectives you’re going to work towards, it’s also important to consider how you’ll measure your progress, so that you can understand what’s working well, and what might require further attention.
Agreeing a set of success metrics is key to holding yourself accountable to making a positive impact with each new iteration. This also helps you to demonstrate progress to others from as early on in the process as possible.
1. Establish 1-3 short term objectives
Take your vision statement and consider the first 1-3 results that you want to achieve within the first 3 months of working towards this.
These objectives need to be realistic and achievable given the 3 month timeframe, so that you’re able to build some momentum and set yourself up for success from the very start of the process.
💡Example objectives:
Improve how insights are defined and captured by the research team
Revisit our existing research to identify what data we want to add to our new research repository
Improve how our research findings are organised, considering how our repository might be utilised by researchers and wider teams
Initial group of champions bought-in and actively using our research repository
Improve the level of engagement with our research from wider teams and stakeholders
Capture your 3 month objectives underneath your vision, leaving space to consider the activities that you need to complete in order to realise each of these.
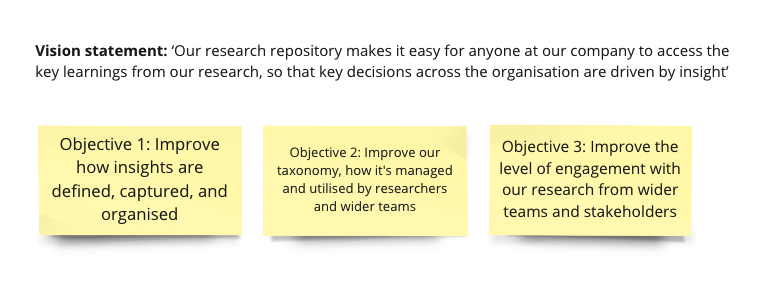
2. Identify how to achieve each objective
Each activity that you commit to should be something that an individual or small group of people can comfortably achieve within the first 3 months of building your repository.
Come up with some ideas for each objective and then prioritise completing the activities that will result in the biggest impact, with the least effort first.
💡Example activities:
Agree a definition for strategic and tactical insights to help with identifying the previous data that we want to add to our new research repository
Revisit the past 6 months of research and capture the data we want to add to our repository as an initial body of knowledge
Create the first draft taxonomy for our research repository, testing this with a small group of wider stakeholders
Launch the repository with an initial body of knowledge to a group of wider repository champions
Start distributing a regular round up of key insights stored in the repository
You can add your activities to a simple kanban board , ordering your ‘To do’ column with the most impactful tasks up top, and using this to track your progress and make visible who’s working on which tasks throughout the initial build of your repository.
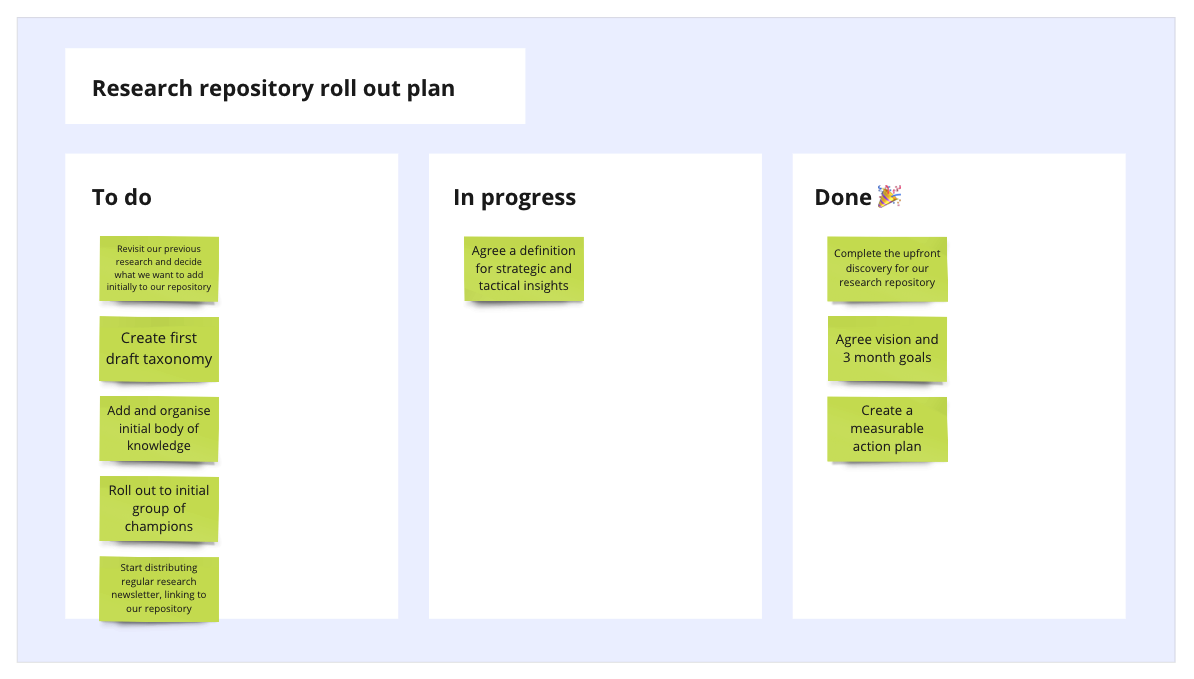
This is something you can come back to a revisit as you move throughout the wider roll out of your repository - adding any new activities into the board and moving these through to ‘Done’ as they’re completed.
⚠️ At this stage it’s also important to call out any risks or dependencies that could derail your progress towards completing each activity, such as capacity, or requiring support from other individuals or teams.
3. Agree how you’ll measure success
Lastly, you’ll need a way to measure success as you work on the activities you’ve associated with each of your short term objectives.
We recommend choosing 1-3 metrics that you can measure and track as you move forward with everything, considering ways to capture and review the data for each of these.
⚠️ Instead of thinking of these metrics as targets, we recommend using them to measure your progress - helping you to identify any activities that aren’t going so well and might require further attention.
💡Example success metrics:
Usage metrics - Number of insights captured, Active users of the repository, Number of searches performed, Number of insights viewed and shared
User feedback - Usability feedback for your repository, User satisfaction ( CSAT ), NPS aka how likely someone is to recommend using your repository
Research impact - Number of stakeholder requests for research, Time spent responding to requests, Level of confidence, Repeatable value of research, Amount of duplicated research, Time spent onboarding new joiners
Wider impact - Mentions of your research (and repository) internally, Links to your research findings from other initiatives e.g. discovery projects, product roadmaps, Customers praising solutions that were fuelled by your research
Think about how often you want to capture and communicate this information to the rest of the team, to help motivate everyone to keep making progress.
By establishing key metrics, you can track your progress and determine whether your repository is achieving its intended goals.
⏰ You should aim to create a measurable action plan for your repository within 60-90 mins (based on a group of up to 6 people).
🚀 Why not use our free, downloadable Miro template to start putting all of this into action today - available for download here .
To summarise
As with the development of any product, the cost of investing time upfront to ensure you’re building the right thing for your end users, is far lower than the cost of building the wrong thing - repositories are no different!
A well-executed research repository can be an extremely valuable asset for your organisation, but building one requires consideration and planning - and defining a clear vision and strategy upfront will help to maximise your chances of success.
It’s important to not feel pressured to nail every objective that you set in the first few weeks or months. Like any product, the further you progress, the more your strategy will evolve and shift. The most important thing is getting started with the right foundations in place, and starting to drive some real impact.
We hope this practical guide will help you to get started on building an effective research repository for your organisation. Thanks and happy researching!

Work with our team of experts
At Dualo we help teams to define a clear vision and strategy for their research repository as part of the ‘Discover, plan and set goals’ module facilitated by our Dualo Academy team. If you’re interested in learning more about how we work with teams, book a short call with us to discuss how we can support you with the development of your research repository and knowledge management process.
Nick Russell
I'm one of the Co-Founders of Dualo, passionate about research, design, product, and AI. Always open to chatting with others about these topics.
Insights to your inbox
Join our growing community and be the first to see fresh content.
Repo Ops ideas worth stealing
Interviews with leaders
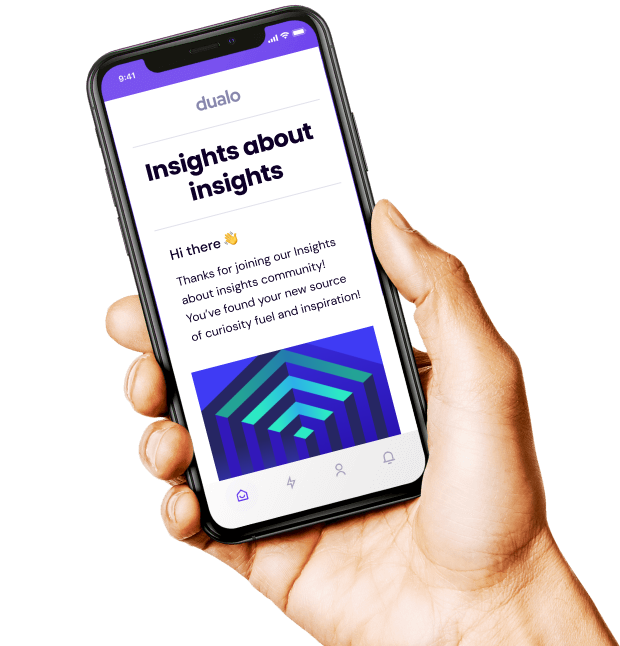
Related Articles
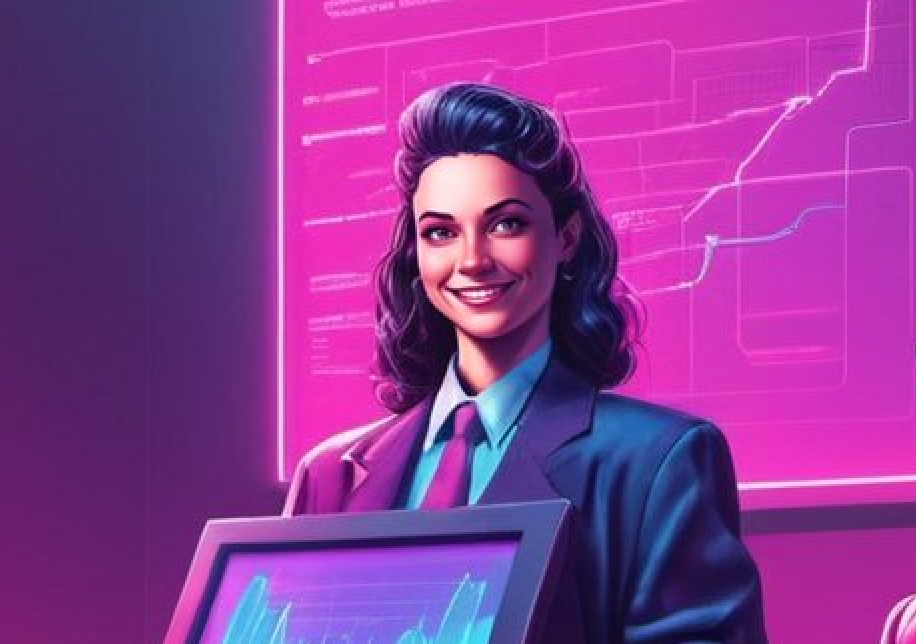
How top 1% researchers build UXR case studies
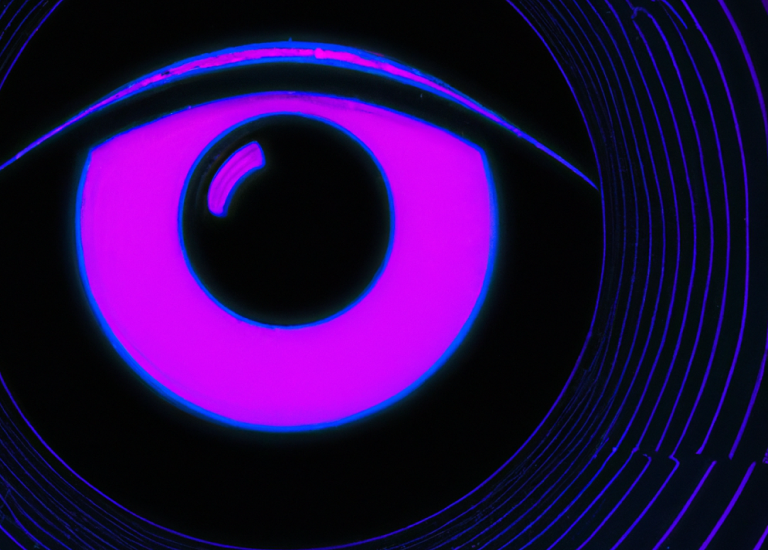
Navigating generative AI in UX Research: a deep dive into data privacy
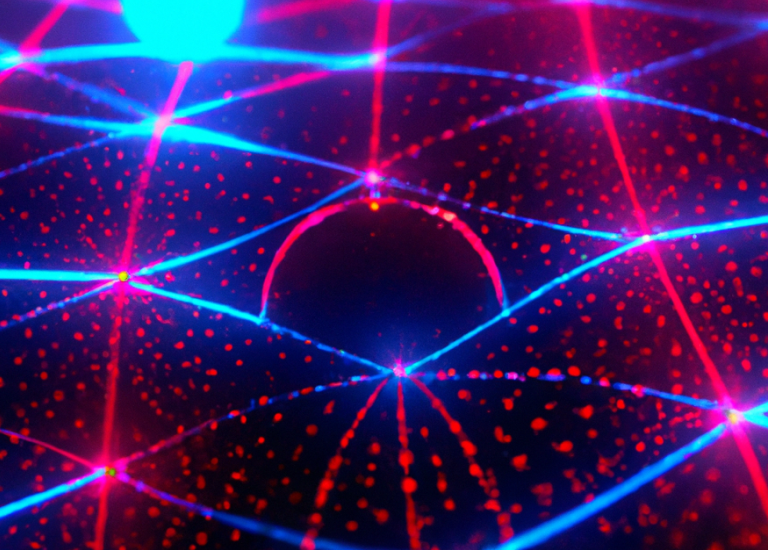
Welcoming a new age of knowledge management for user research
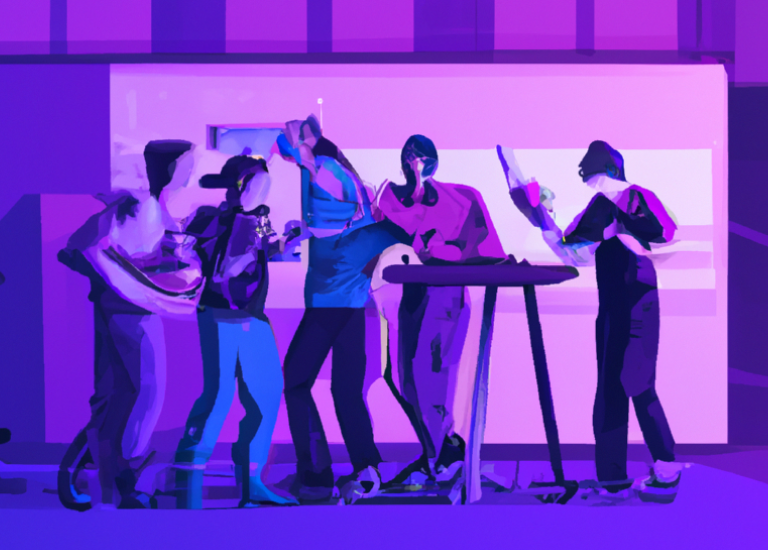
Building a research repository? Avoid these common pitfalls
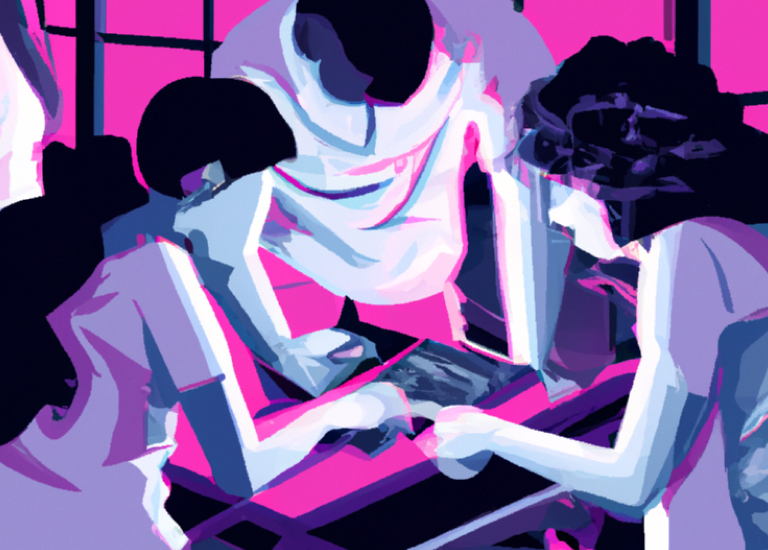
Unlocking hidden insights: why research teams must conduct meta-analysis
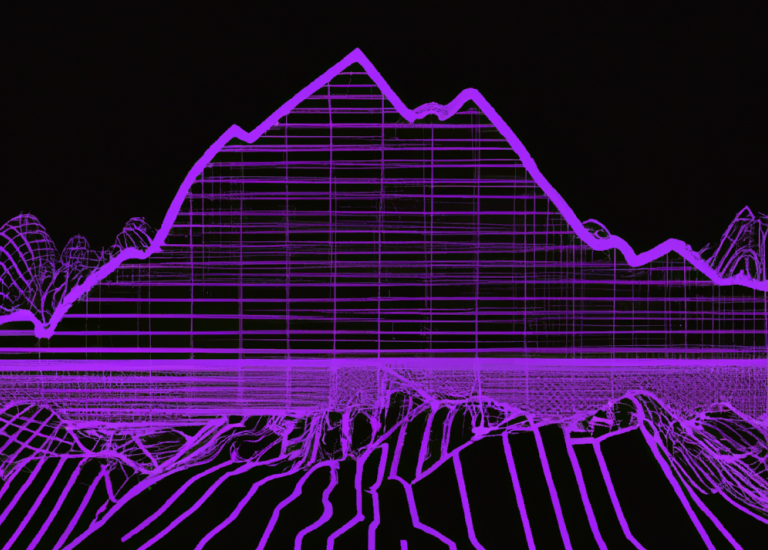
Unlocking the exponential power of insights – an interview with Zachary Heinemann

About Us Subscribe to our Newsletter

The University of Arizona Research Data Repository (ReDATA) is the institution's official repository for publicly archiving and sharing research materials (e.g., data, code, images, videos, etc.) created by University of Arizona researchers. ReDATA helps the UArizona community:
- Comply with funder and journal data sharing policies
- Comply with university data retention policies for primary data
- Archive data associated with published articles, theses/dissertations, and completed research projects
In support of the FAIR (findable, accessible, interoperable, reusable) data principles, all submissions are assigned a Digital Object Identifier (DOI) for citation purposes and undergo a curatorial review by a ReDATA team member prior to publication.
How to include ReDATA in grant applications
How to prepare and deposit materials
Tutorials, General Information, FAQs
Guidance for submitting data associated with journal publications
About the ReDATA team
You can contact the ReDATA team by scheduling a consultation or you may email us directly at [email protected]
Follow us on LinkedIn , Instagram , or Mastodon
Was this page useful?
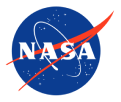
NASA’s Repository Supports Research of Commercial Astronaut Health

NASA’s Open Science Data Repository provides valuable information to researchers studying the impact of space on the human body. Nearly three years after the Inspiration4 commercial crew launch, biological data from the mission represents the first comprehensive, open-access database to include commercial astronaut health information.
Access to astronaut research data from astronauts has historically been limited, due to privacy regulations and concerns, but the field of astronauts is changing as commercial spaceflight becomes feasible for civilians.
“Open-access data is fundamentally transforming our approach to spaceflight research,” said Dr. Sylvain Costes, project manager of the Open Science Data Repository. “The repository is instrumental in this transformation, ensuring that all space-related biological and biomedical data are accessible to everyone. This broad access is vital for driving innovation across fields from astronaut health to terrestrial medical sciences.”
The collaborative efforts in opening data to researchers has led to multiple scientific papers on astronaut health published in Nature in June. The papers represents research to better understand the impact of spaceflight on the human body, how viruses might spread in a zero-gravity environment, and how countermeasures may protect humans on future long-duration missions.
Ongoing access to the data captured by commercial astronauts means the research can continue long after the crew returns to Earth, impacting the future of research beyond spaceflight, including cancer and genetic diseases and bone health.
“This series of inspiring articles enabled by the repository and enriched by new data generously shared by commercial astronauts aboard the Inspiration4 mission exemplifies our commitment to open science,” said Costes. “By making our data fully accessible and usable, we're enabling researchers worldwide to explore new frontiers in space biology.”
NASA’s Open Science Data Repository is based out of the agency's Ames Research Center in California’s Silicon Valley. NASA continues to pursue the best methods and technologies to support safe, productive human space travel. Through science conducted in laboratories, ground-based analogs, and missions to the International Space Station, NASA continues to research innovative ways to keep astronauts healthy as space explorations continues to the Moon, Mars, and beyond.
About the Author
Tara Friesen
Related terms.
Ames Research Center
- Ames Research Center's Science Directorate
Commercial Space
- Humans in Space
- Open Science
Explore More

NASA Announces New System to Aid Disaster Response

Lakita Lowe: Leading Space Commercialization Innovations and Fostering STEM Engagement
Lakita Lowe is at the forefront of space commercialization, seamlessly merging scientific expertise with visionary leadership to propel NASA’s commercial ambitions and ignite a passion for STEM in future generations. As a project integrator for NASA’s Commercial Low Earth Orbit Development Program (CLDP), Lowe leverages her extensive background in scientific research and biomedical studies to […]

NASA Ames Astrogram – May/June 2024
Reimagined NASA Ames Visitor Center Opens at Chabot Space & Science Center A complete transformation is now underway at the NASA Ames Visitor Center at Chabot Space & Science Center in Oakland, California. Significant changes will create a fully reimagined 360-degree experience, featuring new exhibits, models, and more. This transformation will be open to the public on […]
Discover More Topics From NASA

Open Science at NASA
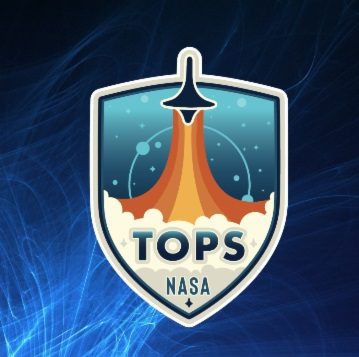
Humans In Space
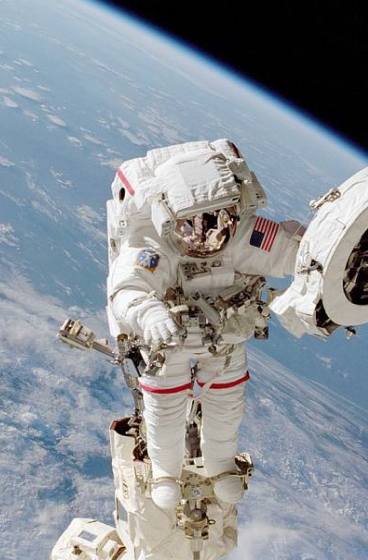

IMAGES
VIDEO
COMMENTS
Harvard Dataverse is an online data repository where you can share, preserve, cite, explore, and analyze research data. It is open to all researchers, both inside and out of the Harvard community. Harvard Dataverse provides access to a rich array of datasets to support your research.
Whether data is deposited in a purpose-built repository or published as Supporting Information alongside a research article, Open Data practices ensure that data remains accessible and discoverable. For verification, replication, reuse, and enhanced understanding of research.
Share your research data. Mendeley Data is a free and secure cloud-based communal repository where you can store your data, ensuring it is easy to share, access and cite, wherever you are. Create a Dataset. Find out more about our institutional offering, Digital Commons Data
re3data Call for Editorial Board The re3data.org registry has been in operation for over 10 years and provides a curated index of over 3,000 research data repositories around the world from all disciplines. New repositories are identified and reviewed by an... Read more.
Data repositories are a centralized place to hold data, share data publicly, and organize data in a logical manner. Benefits of Data Repositories. manage your data; organize and deposit your data; cite your data by supplying a persistent identifier; facilitate discovery of your data; make your data more valuable for current and future research
Repositories need to meet our requirements for anonymous peer-review, data access, preservation, resource stability, licences and suitability for use by all researchers with the appropriate types...
Authors should select repositories appropriate to their field of study (for example, ArrayExpress or GEO for microarray data; GenBank, EMBL, or DDBJ for gene sequences). PLOS has identified a set of established repositories, listed below, that are recognized and trusted within their respective communities.
Biomedical data repository: Systems that accept submissions of relevant data from the research community to store, organize, validate, archive, preserve, and distribute the data, in compliance ...
For more than ten years, re3data, a global registry of research data repositories (RDRs), has been helping scientists, funding agencies, libraries, and data centers with finding,...
Definition of research data and overview of the different options to share data: store, link, enrich, publish, declare.
Dataset. Open. Room Impulse Responses for Low-Frequency Sound Field Control. Cadavid, José Møller, Martin Bo van Waterschoot, Toon. About A dataset of room impulse responses (RIRs) measured in the low frequency range, for different measurement signal lengths, in two rooms with different acoustic conditions.
A research repository acts as a centralized database where information is gathered, stored, analyzed, and archived in one organized space. In this single source of truth, raw data, documents, reports, observations, and insights can be viewed, managed, and analyzed.
A repository is an online database that allows research data to be preserved across time and helps others find it. Apart from archiving research data, a repository will assign a DOI to each uploaded object and provide a web page that tells what it is, how to cite it and how many times other researchers have cited or downloaded that object.
A data repository is a storage space for researchers to deposit data sets associated with their research. And if you’re an author seeking to comply with a journal data sharing policy, you’ll need to identify a suitable repository for your data. An open access data repository openly stores data in a way that allows immediate user access to anyone.
What is a Research Data Repository? A research data repository is a virtual place to store and preserve research data. Depositing research data in a repository increases data transparency and exposure and promotes research collaboration opportunities.
Data repositories, including generalist repositories or institutional repositories, that make data available to the larger research community, institutions, or the broader public. Large datasets may benefit from cloud-based data repositories for data access, preservation, and sharing.
Use and reuse of research data is an integral part of the scientific process, and therefore TRUSTworthy repositories should enable their community to find, explore, and understand their...
The ASU Research Data Repository provides a permanent digital identifier for research data, which complies with data sharing policies. The repository is powered by the Dataverse open-source application, developed and used by Harvard University.
The repository develops and disseminates guidance for managing, sharing, citing, and reusing qualitative data, and contributes to the generation of common standards for doing so.
The Data Citation Index includes records on over 14 million research data sets, 1.6 million data studies, and 405 thousand software from over 450 international data repositories in the sciences, social sciences, and arts and humanities.
Research repositories have the potential to be incredibly powerful assets for any research-driven organisation. But when it comes to building one, it can be difficult to know where to start. In this post, we provide some practical tips to define a clear vision and strategy for your repository. Nick Russell. June 16, 2023.
The University of Arizona Research Data Repository (ReDATA) is the institution's official repository for publicly archiving and sharing research materials (e.g., data, code, images, videos, etc.) created by University of Arizona researchers. ReDATA helps the UArizona community: Comply with funder and journal data sharing policies.
NASA’s Open Science Data Repository provides valuable information to researchers studying the impact of space on the human body. Nearly three years after the Inspiration4 commercial crew launch, biological data from the mission represents the first comprehensive, open-access database to include commercial astronaut health information. Access to astronaut research data from astronauts has ...
Topics / Series / Thematic data collections / Data-related publications. Studies with online analysis. Self-published data, including replication datasets. Studies with learning guides. New/Updated Data Releases: In the last week. In the last month. In the last quarter. In the last year.