Automated Scoring of Writing
- Open Access
- First Online: 15 September 2023

Cite this chapter
You have full access to this open access chapter
- Stephanie Link ORCID: orcid.org/0000-0002-5586-1495 8 &
- Svetlana Koltovskaia 9
4713 Accesses
For decades, automated essay scoring (AES) has operated behind the scenes of major standardized writing assessments to provide summative scores of students’ writing proficiency (Dikli in J Technol Learn Assess 5(1), 2006). Today, AES systems are increasingly used in low-stakes assessment contexts and as a component of instructional tools in writing classrooms. Despite substantial debate regarding their use, including concerns about writing construct representation (Condon in Assess Writ 18:100–108, 2013; Deane in Assess Writ 18:7–24, 2013), AES has attracted the attention of school administrators, educators, testing companies, and researchers and is now commonly used in an attempt to reduce human efforts and improve consistency issues in assessing writing (Ramesh and Sanampudi in Artif Intell Rev 55:2495–2527, 2021). This chapter introduces the affordances and constraints of AES for writing assessment, surveys research on AES effectiveness in classroom practice, and emphasizes implications for writing theory and practice.
You have full access to this open access chapter, Download chapter PDF
- Automated essay scoring
- Summative assessment
Automated essay scoring (AES) is used internationally to rapidly assess writing and provide summative holistic scores and score descriptors for formal and informal assessments. The ease of using AES for response to writing is especially attractive for large-scale essay evaluation, providing also a low-cost supplement to human scoring and feedback provision. Additionally, intended benefits of AES include the elimination of human bias, such as rater fatigue, expertise, severity/leniency, inconsistency, and Halo effect. While AES developers also commonly suggest that their engines perform as reliably as human scorers (e.g., Burstein & Chodorow, 2010 ; Riordan et al., 2017 ; Rudner et al., 2006 ), AES is not free of critique. Automated scoring is frequently under scrutiny for use with university-level composition students in the United States (Condon, 2013 ) and second language writers (Crusan, 2010 ), with some writing practitioners discouraging its replacement of adequate literacy education because of its inability to evaluate meaning from a humanistic, socially-situated perspective (Deane, 2013 ; NCTE, 2013 ). AES also suffers from biases, such as imperfections in the quality and representation of training data to develop the systems and inform feedback generation. These biases question the fairness of AES (Loukina et al., 2019 ), especially if scores are modeled based on data that does not adequately represent a user population—a particular concern for use of AES with minoritized populations.
Despite reservations, the utility of AES in writing practices has increased significantly in recent years (Ramesh & Sanampudi, 2021 ), partially due to its integration into classroom-based tools (see Cotos, “ Automated Feedback on Writing ” for a review of automated writing evaluation). Thus, the affordances of AES for language testing are now readily available to writing practitioners and researchers, and the time is ripe for better understanding its potential impact on the pedagogical approaches to writing studies by first better understanding the history that drives AES development.
Dating back to the 1960s, AES started with the advent of Project Essay Grade (Page, 1966 ). Since then, automated scoring has advanced into leading technologies, including e-rater by the Educational Testing Service (ETS) (Attali & Burstein, 2006 ), Intelligent Essay Assessor (IEA) by Knowledge Analysis Technologies (Landauer et al., 2003 ), Intellimetric by Vantage Learning (Elliot, 2003 ), and a large number of prospective newcomers (e.g., Nguyen & Dery, 2016 ; Riordan et al., 2017 ). These AES engines are used for tests like the Test of English as a Foreign Language (TOEFL iBT), Graduate Management Admissions Test (GMAT), and the Pearson Test of English (PTE). In such tests, AES researchers not only found the scores reliable, but some argued that they also allowed for reproducibility, tractability, consistency, objectivity, item specification, granularity, and efficiency (William et al., 1999 ), characteristics that human raters can lack (Williamson et al., 2012 ).
The immediate AES response to writing is without much question a salient feature of automated scoring for testing contexts. However, research on classroom-based implementation has suggested that instructors can utilize the AES feedback to flag students’ writing that requires teachers’ special attention (Li et al., 2014 ), highlighting its potential for constructing individual development plans or conducting analysis of students’ writing needs. AES also provides constant, individualized feedback to lighten instructors’ feedback load (Kellogg et al., 2010 ), enhance student autonomy (Wang et al., 2013 ), and stimulate editing and revision (Li et al., 2014 ).
2 Core Idea of the Technology
Automated essay scoring involves automatic assessment of a students’ written work, usually in response to a writing prompt. This assessment generally includes (1) a holistic score of students’ performance, knowledge, and/or skill and (2) a score descriptor on how the student can improve the text. For example, e-rater by ETS ( 2013 ) scores essays on a scale from 0 to 6. A score of 6 may include the following feedback:
Score of 6: Excellent
Looks at the topic from a number of angles and responds to all aspects.
Responds thoughtfully and insightfully to the issues in the topic.
Develops with a superior structure and apt reasons or examples.
Uses sentence styles and language that have impact and energy.
Demonstrates that you know the mechanics of correct sentence structure.
AES engine developers over the years have undertaken a core goal of making the assessment of writing accurate, unbiased, and fair (Madnani & Cahill, 2018 ). The differences in score generation, however, are stark given the variation in philosophical foundations, intended purposes, extraction of features for scoring writing, and criteria used to test the systems (Yang et al., 2002 ). To this end, it is important to understand the prescribed use of automated systems so that they are not implemented inappropriately. For instance, if a system is meant to measure students’ writing proficiency, the system should not be used to assess students’ aptitude. Thus, scoring models for developing AES engines are valuable and effective in distinct ways and for their specific purposes.
Because each engine may be designed to assess different levels, genres, and/or skills of writing, developers utilize different natural language processing (NLP) techniques for establishing construct validity, or the extent to which an AES scoring engine measures what it intends to measure—a common concern for AES critics (Condon, 2013 ; Perelman, 2014 , 2020 ). NLP helps computers understand human input (text and speech) by starting with human and/or computer analysis of textual features so that a computer can process the textual input and offer reliable output (e.g., a holistic score and score descriptor) on new text. These features may include statistical features (e.g., essay length, word co-occurrences also known as n-grams), style-based features (e.g., sentence structure, grammar, part-of-speech), and content-based features (e.g., cohesion, semantics, prompt relevance) (see Ramesh & Sanampudi, 2021 , for an overview of features). Construct validity should thus be interpreted in relation to feature extraction of a given AES system to adequately appreciate (or challenge) the capabilities that system offers writing studies.
In addition to a focus on a variety of textual features, AES developers have utilized varied machine learning (ML) techniques to establish construct validity and efficient score modeling. Machine learning is a category of artificial intelligence (AI) that helps computers recognize patterns in data and continuously learn from the data to make accurate holistic score predictions and adjustments without further programming (IBM, 2020 ). Early AES research utilized standard multiple regression analysis to predict holistic scores based on a set of rater-defined textual features. This approach was utilized in the early 1960s for developing Project Essay Grade by Page ( 1966 ), but it has been criticized for its bias in favor of longer texts (Hearst, 2000 ) and its ignorance towards content and domain knowledge (Ramesh & Sanampudi, 2021 ).
In subsequent years, classification models, such as the bag of words approach (BOW), were common (e.g., Chen et al., 2010 ; Leacock & Chodorow, 2003 ). BOW models extract features in writing using NLP by counting the occurrences and co-occurrences of words within and across texts. Texts with multiple shared word strings are classified into similar holistic score categories (e.g., low, medium, high) (Chen et al., 2010 ; Zhang et al., 2010 ). E-rater by ETS is a good example of this approach. The aforementioned approaches are human-labor intensive. Latent semantic analysis (LSA) is advantageous in this regard; it is also strong in evaluating semantics. In LSA, the semantic representation of a text is compared to the semantic representation of other similarly scored responses. This analysis is done by training the computer on specific corpora that mimics a given writing prompt. Landauer et al. ( 2003 ) used LSA in Intelligent Essay Grade.
Advances in NLP and progress in ML have motivated AES researchers to move away from statistical regression-based modeling and classification approaches to advanced models involving neural network approaches (Dong et al., 2017 ; Kumar & Boulanger, 2020 ; Riordan et al., 2017 ). To develop these AES models, data undergoes a process of supervised learning, where the computer is provided with labeled data that enables it to produce a score as a human would. The supervised learning process often starts with a training set—a large corpus of representative, unbiased writing that is typically human- or auto-coded for specific linguistic features with each text receiving a holistic score. Models are then generated to teach a computer to identify and extract these features and provide a holistic score that correlates with the human rating. The models are evaluated on a testing set that the computer has never seen previously. Accuracy of algorithms is then evaluated by using testing set scores and human scores to determine human–computer consistency and reliability. Common evaluations are quadrated weighted kappa, Mean Absolute Error, and Pearson Correlation Coefficient.
Once accuracy results meet an industry standard (Powers et al., 2015 ), which varies across disciplines (Weigle, 2013 ), the algorithms are made public through user-friendly interfaces for testing contexts (i.e., to provide summative feedback, formal assessments to assess students’ performance or proficiency) and direct classroom use (i.e., informal assessments to improve students’ learning). For the classroom, teachers should be active in evaluating the feedback to determine whether it is reasonably accurate in assessing a learning goal, does not lead students away from the goal, and encourages students to engage in different ways with their text and/or the course content. Effective evaluation of AES should start with an awareness of AES affordances that can impact writing practice and then continue with the training of students in the utility of these affordances.
3 Functional Specifications
The overall functionality of AES for classroom use is to provide summative assessment of writing quality. AES accomplishes this through two key affordances: a holistic score and score descriptor.
Holistic score: The summative score provides an overall, generic assessment of writing quality. For example, Grammarly provides a holistic score or “performance” score out of 100%. The score represents the quality of writing (as determined by features, such as word count, readability statistics, vocabulary usage). If a student receives a score below 60–70%, this means that it could be understood by a reader who has a 9th grade education. For the text to be readable by 80% of English speakers, Grammarly suggests getting at least 60–70%.
Score descriptor: The holistic score is typically accompanied by a descriptor that indicates what the score represents. This characterization of the score meaning can be used to interpret the feedback, evaluate the feedback, and make decisions regarding editing and revising.
That is, these key affordances can be utilized to complete several main activities.
Interpreting feedback : Once students receive the holistic score along with the descriptor, they should interpret the score. Information provided for adequate score interpretation varies across AES systems, so students may need help in interpreting the meaning of this feedback.
Evaluating feedback : After interpreting the score and the descriptor, students need to think critically about how the feedback applies to their writing. That is, students need to determine whether the computer feedback is an adequate representation of their writing weaknesses. Evaluating feedback thus entails noticing the gap or problem found in one’s own writing and becoming consciously aware of how the feedback might be used to increase the quality of writing through self-editing (Ferris, 2011 ).
Making a decision about action : Once students evaluate their writing based on a given score and descriptor, they then need to decide whether to address the issues highlighted in the descriptor or seek additional feedback. Making and executing a revision plan can ensure that the student is being critical towards the feedback rather than accepting it outright.
Revising/editing : The student then revises the paper and resubmits it to the system to see if the score improves—an indicator of higher quality writing. If needed, the student can repeat the above actions or move on to editing of surface-level writing concerns.
4 Research on AES
AES research can be categorized along two lines: system-centric research that evaluates the system itself and user-centric research that evaluates use/impact of a system on learning. From a system-centric perspective, various studies have been conducted to validate AES-system-generated scores for the testing context. The majority have focused on reliability, or the extent to which results can be considered consistent or stable (Brown, 2005 ). They often evaluate reliability based on agreement between human and computer scoring (e.g., Burstein & Chodorow, 1999 ; Elliot, 2003 ; Streeter et al., 2011 ). (See Table 1 for a summary of reliability statistics from three major AES developers.)
The process of establishing validity should not start and stop with inter-coder reliability; however, automated scoring presents some distinctive validity challenges, such as “the potential to under- or misrepresent the construct of interest, vulnerability to cheating, impact on examinee behavior, and score users’ interpretation and use of scores” (Williamson et al., 2012 , p. 3). Thus, some researchers have also demonstrated reliability by using alternative measures, such as the association with independent measures (Attali et al., 2010 ) and the generalizability of scores (Attali et al., 2010 ). Others have gone a step further and suggested a unified approach to AES validation (Weigle, 2013 , Williamson et al., 2012 ). In general, results reveal promising developments in AES with modest correlations between AES and external criteria, such as independent proficiency assessments (Attali et al., 2010 ; Powers et al., 2015 , suggesting that automated scores can relate in a similar manner to select assessment criteria and that both have the potential to reflect similar constructs, although results across AES systems can vary, and not all data are readily available to the public.
While much research has focused on reliability of AES, little is known about the quality of holistic scores in testing or classroom contexts as well as teachers’ and students’ use and perceptions of automatically generated scores. In a testing context, James ( 2006 ) compared the IntelliMetric scores of the ACCUPLACER OnLine WritePlacer Plus test to the scores of “untrained” faculty raters. Results revealed a relatively high level of correspondence between the two. In a similar study with a group of developmental writing students in a two-year college in South Texas, Wang and Brown ( 2007 ) found that ACCUPLACER’s overall holistic mean score showed significant difference between IntelliMetric and human raters, indicating that IntelliMetric tends to assign higher scores than human raters do. Li et al. ( 2014 ) investigated the correlation between Criterion’s numeric scores with the English as a second language instructors’ numeric grades and analytic ratings for classroom-based assessment. The results showed low to moderate positive correlations between Criterion’s scores and instructors’ scores and analytic ratings. Taken together, these studies suggest limited continuity of findings on AES reliability across tools.
Results of multiple studies demonstrate varied uses for holistic scores and varied teachers’ and students’ perceptions toward the scores. For example, Li et al. ( 2014 ) found that Criterion’s holistic scores in the English as a second language classroom were used in three ways. First, instructors used the scores as a forewarning. That is, the scores alerted instructors to problematic writing. Second, the scores were used as a pre-submission benchmark. That is, the students were required to obtain a certain score before submitting a final draft to their teacher. Finally, Criterion's scores were utilized as an assessment tool—scores were part of course grading. Similar findings were reported in Chen and Cheng’s ( 2008 ) study that focused on EFL Tawainese teachers’ and students’ use and perception of My Access! While one teacher used My Access! as a pre-submission benchmark, the other used it for both formative and summative assessment, heavily relying on the scores to assessing writing performance. The third teacher did not make My Access! a requirement and asked the students to use it if they needed to.
In terms of teachers’ perceptions of holistic scores, holistic scores seem to be motivators for promoting student revision (Li et al. 2014 ; Scharber et al., 2008 ) although a few teachers in Maeng ( 2010 ) commented that the score caused some stress albeit was still helpful for facilitating the feedback process (i.e., for providing sample writing and revising). Teachers also tend to have mixed confidence in holistic scores (Chen & Cheng, 2008 ; Li et al, 2014 ). For example, in Li et al.’s ( 2014 ) study, English as a second language instructors had high trust in Criterion’s low holistic scores as the essays Criterion scored low were, in fact, poor essays. However, instructors possessed low levels of trust when Criterion assigned high scores to writing as instructors judged such writing lower.
Students also tend to have low trust in holistic scores (Chen & Cheng, 2008 ; Scharber et al., 2008 ). For example, Chen and Cheng ( 2008 ) found that EFL Taiwanese students’ low level of trust in holistic scores was influenced by teachers’ low level of trust in the scores as well as discrepancies in teachers’ scores and holistic scores of My Access! that students noticed. Similar findings were reported in Scharber et al.’s ( 2008 ) study that focused on Educational Theory into Practice Software’s (ETIPS) automated scorer implemented in a post-baccalaureate program at a large public Midwestern US university. The students in their study experienced negative emotions due to discrepancies in teachers’ and ETIPS’ holistic scores. ETIPS scores were one point lower than teachers’ scores. Additionally, the students found holistic scores with the short descriptor insufficient in guiding them as to how to actually improve their essays.
5 Implications of This Technology for Writing Theory and Practice
The rapid advancement of NLP and ML approaches to automated scoring lends well to theoretical contributions that help to (re-)define traditional notions of how learning takes place and the phenomena that underscores language development. Social- and cognitive-based theories to writing studies can be expanded with the integration of AES technology by offering new, socially-situated learning opportunities in online environments that can impact how students respond to feedback. These digitally-rich learning opportunities can thus significantly impact the writing process, offering a new mode of feedback that can be meaningful, constant, timely, and manageable while addressing individual learner needs. From a traditional pen-and-paper approach, these benefits are known to contribute significantly to writing accuracy (Hartshorn et al., 2010 ), and so the addition of rapid technology has the potential to add new knowledge to writing development research.
AES research can also contribute to practice. Due to its instantaneous nature, AES holistic scores could be used for placement purposes (e.g., by using ACCUPLACER) at schools, colleges, and universities. However, relying on the AES holistic score alone may not be adequate. Therefore, just like in large-scale tests, it is important that students’ writing is double-rated to enhance reliability, with a third rater used if there is a discrepancy in AES holistic score and a human rater’s score. Similarly, AES holistic scores could be used for diagnostic assessment. Diagnostic assessment is given prior to or at the start of the semester/course to get information about students’ language proficiency as well as their strengths and weaknesses in writing. Finally, AES scoring could be used for summative classroom assessment. For example, teachers could use AES scores as a pre-submission benchmark and require students to revise their essays until they get a predetermined score, or teachers could use the AES score for partial (rather than sole) assessment of goal attainment (Li et al., 2014 ; Weigle, 2013 ). Overall, in order to avoid pitfalls such as students focusing too intensively on obtaining high scores without actually improving their writing skills, teachers and students need to be trained or seek training on the different merits and demerits of a selected AES scoring system.
6 Concluding Remarks
While traditional approaches to written corrective feedback are still leading writing studies research, the ever-changing digitalization of the writing process shines light on new opportunities for enhancing the nature of feedback provision. The evolution of AI will undoubtedly expand the affordances of AES so that writing in digital spaces can be supplemented by computer-based feedback that is increasingly accurate and reliable. For now, these technologies are only foregrounding what can come from technological advancements, and in the meantime, it is the task of researchers and practitioners to cast a critical eye while also remaining open to the potential for AES technologies to promote autonomous, lifelong learning and writing development.
7 Tool List
List of well-known Automated Essay Scoring (AES) Tools
Attali, Y., Bridgeman, B., & Trapani, C. (2010). Performance of a generic approach in automated essay scoring. Journal of Technology , Learning, and Assessment, 10 (3). http://www.jtla.org
Attali, Y., & Burstein, J. (2006). Automated essay scoring with e-rater V.2. Journal of Technology, Learning, and Assessment, 4 (3), 1–30.
Google Scholar
Brown, J. D. (2005). Testing in language programs. A comprehensive guide to English language assessment . McGraw Hill.
Burstein, J., & Chodorow, M. (1999). Automated essay scoring for nonnative English speakers . Proceedings of the ACL99 Workshop on Computer-Mediated Language Assessment and Evaluation of Natural Language Processing. http://www.ets.org/Media/Research/pdf/erater_acl99rev.pdf
Burstein, J., & Chodorow, M. (2010). Progress and new directions in technology for automated essay evaluation. In R. Kaplan (Ed.), The Oxford handbook of applied linguistics (2nd ed., pp. 487–497). Oxford University Press.
Chen, C., & Cheng, W. (2008). Beyond the design of automated writing evaluation: Pedagogical practices and perceived learning effectiveness in EFL writing classes. Language Learning & Technology, 12 (2), 94–112.
Chen, Y. Y., Liu, C. L., Chang, T. H., & Lee, C. H. (2010). An unsupervised automated essay scoring system. IEEE Intelligent Systems, 25 (5), 61–67. https://doi.org/10.1109/MIS.2010.3
Article Google Scholar
Condon, W. (2013). Large-scale assessment, locally-developed measures, and automated scoring of essays: Fishing for red herrings? Assessing Writing, 18 , 100–108. https://doi.org/10.1016/j.asw.2012.11.001
Crusan, D. (2010). Assessment in the second language writing classroom . University of Michigan Press.
Book Google Scholar
Deane, P. (2013). On the relation between automated essay scoring and modern views of the writing construct. Assessing Writing, 18 , 7–24.
Dexter, S. (2007). Educational theory into practice software. In D. Gibson, C. Aldrich, & M. Prensky (Eds.), Games and simulations in online learning: Research and development frameworks (pp. 223–238). IGI Global. https://doi.org/10.4018/978-1-59904-304-3.ch011
Dikli, S. (2006). An overview of automated scoring of essays. The Journal of Technology, Learning and Assessment, 5 (1). https://ejournals.bc.edu/index.php/jtla/article/view/1640
Dong, F., Zhang, Y., & Yang, J. (2017). Attention-based recurrent convolutional neural network for automatic essay scoring . Proceedings of the 21st Conference on Computational Natural Language Learning (CoNLL 2017). https://aclanthology.org/K17-1017.pdf
Elliot, S. (2003). IntelliMetric: From here to validity. In M. D. Shermis & J. C. Burstein (Eds.), Automatic essay scoring: A cross-disciplinary perspective (pp. 71–86). Lawrence Erlbaum Associates.
ETS. (2013). Criterion scoring guide . Retrieved September 27, 2013, from http://www.ets.org/Media/Products/Criterion/topics/co-1s.htm
Ferris, D. R. (2011). Treatment of errors in second language student writing (2nd ed.). The University of Michigan Press.
Hartshorn, K. J., Evans, N. W., Merrill, P. F., Sudweeks, R. R., Strong-Krause, D., & Anderson, N. J. (2010). Effects of dynamic corrective feedback on ESL writing accuracy. TESOL Quarterly, 44 , 84–109.
Hearst, M. (2000). The debate on automated essay grading. IEEE Intelligent Systems and their Applications, 15 (5), 22–37. https://doi.org/10.1109/5254.889104
IBM. (2020). Machine learning . IBM Cloud Education. https://www.ibm.com/cloud/learn/machine-learning
James, C. (2006). Validating a computerized scoring system for assessing writing and placing students in composition courses. Assessing Writing, 11 (3), 167–178.
Kellogg, R., Whiteford, A., & Quinlan, T. (2010). Does automated feedback help students learn to write? Journal of Educational Computing Research, 42 , 173–196.
Kumar, V., & Boulanger, D. (2020). Explainable automated essay scoring: Deep learning really has pedagogical value. Frontiers in Education (Lausanne) , 5 . https://doi.org/10.3389/feduc.2020.572367
Landauer, T. K., Laham, D., & Foltz, P. (2003). Automatic essay assessment. Assessment in Education, 10 (3), 295–308.
Leacock, C., & Chodorow, M. (2003). C-rater: Automated scoring of short-answer questions. Computers and the Humanities, 37 , 389–405.
Li, Z., Link, S., Ma, H., Yang, H., & Hegelheimer, V. (2014). The role of automated writing evaluation holistic scores in the ESL classroom. System, 44 , 66–78. https://doi.org/10.1016/j.system.2014.02.007
Loukina, A., et al. (2019). The many dimensions of algorithmic fairness in educational applications . BEA@ACL.
Madnani, N., & Cahill, A. (2018). Automated scoring: Beyond natural language processing . COLING.
Maeng, U. (2010). The effect and teachers’ perception of using an automated essay scoring system in L2 writing. English Language and Linguistics, 16 (1), 247–275.
NCTE. (2013, April 20). NCTE position statement on machine scoring . National Council of Teachers of English. https://ncte.org/statement/machine_scoring/
Nguyen, H., & Dery, L. (2016). Neural networks for automated essay grading (pp. 1–11). CS224d Stanford Reports.
Page, E. B. (1966). The imminence of grading essays by computer. Phi Delta Kappan, 48 , 238–243.
Perelman, L. (2014). When “the state of the art” is counting words. Assessing Writing, 21 , 104–111.
Perelman, L. (2020). The BABEL generator and E-rater: 21st century writing constructs and automated essay scoring (AES). Journal of Writing Assessment, 13 (1).
Powers, D. E., Escoffery, D. S., & Duchnowski, M. P. (2015). Validating automated essay scoring: A (modest) refinement of the “gold standard.” Applied Measurement in Education, 28 (2), 130–142. https://doi.org/10.1080/08957347.2014.1002920
Ramesh, D., & Sanampudi, S. K. (2021). An automated essay scoring systems: A systematic literature review. The Artificial Intelligence Review, 55 (3), 2495–2527. https://doi.org/10.1007/s10462-021-10068-2
Riordan, B., Horbach, A., Cahill, A., Zesch, T., & Lee, C. M. (2017). Investigating neural architectures for short answer scoring . Proceedings of the 12th Workshop on Innovative Use of NLP for Building Educational Applications. https://aclanthology.org/W17-5017.pdf
Rudner, L., Garcia, V., & Welch, C. (2006). An evaluation of IntelliMetricTM essay scoring system. Journal of Technology, Learning, and Assessment, 4 (4). http://escholarship.bc.edu/ojs/index.php/jtla/article/view/1651/1493
Scharber, C., Dexter, S., & Riedel, E. (2008). Students’ experiences with an automated essay scorer. Journal of Technology, Learning and Assessment, 7 (1), 1–45. https://ejournals.bc.edu/index.php/jtla/article/view/1628
Streeter, L., Bernstein, J., Foltz, P., & DeLand, D. (2011). Pearson’s automated scoring of writing, speaking, and mathematics . White Paper. http://images.pearsonassessments.com/images/tmrs/PearsonsAutomatedScoringofWritingSpeakingandMathematics.pdf
Wang, J., & Brown, M. S. (2007). Automated essay scoring versus human scoring: A comparative study. Journal of Technology, Learning, and Assessment, 6 (2). http://www.jtla.org
Wang, Y., Shang, H., & Briody, P. (2013). Exploring the impact of using automated writing evaluation in English as a foreign language university students’ writing. Computer Assisted Language Learning, 26 (3), 1–24.
Weigle, S. C. (2013). English as a second language writing and automated essay evaluation. In M. D. Shermis & J. C. Burstein (Eds.), Handbook of automated essay evaluation: Current applications and new directions (pp. 36–54). Routledge.
William, D. M., Bejar, I. I., & Hone, A. S. (1999). ’Mental model’ comparison of automated and human scoring. Journal of Educational Measurement, 35 (2), 158–184.
Williamson, D., Xi, X., & Breyer, F. J. (2012). A framework for evaluation and use of automated scoring. Educational Measurement: Issues and Practice, 31 (1), 2–13.
Yang, Y., Buckendahl, C. W., Juszkiewicz, P. J., & Bhola, D. S. (2002). A review of strategies for validating computer-automated scoring. Applied Measurement in Education, 15 (4), 391–412. https://doi.org/10.1207/S15324818AME1504_04
Zhang, Y., Jin, R., & Zhou, Z. H. (2010). Understanding bag-of-words model: A statistical framework. International Journal of Machine Learning and Cybernetics, 1 , 43–52.
Download references
Author information
Authors and affiliations.
Oklahoma State University, 205 Morrill Hall, Stillwater, OK, 74078, USA
Stephanie Link
Department of Languages and Literature, Northeastern State University, Tahlequah, OK, 74464, USA
Svetlana Koltovskaia
You can also search for this author in PubMed Google Scholar
Corresponding author
Correspondence to Stephanie Link .
Editor information
Editors and affiliations.
School of Applied Linguistics, Zurich University of Applied Sciences, Winterthur, Switzerland
School of Management and Law, Center for Innovative Teaching and Learning, Zurich University of Applied Sciences, Winterthur, Switzerland
Christian Rapp
North Carolina State University, Raleigh, NC, USA
Chris M. Anson
TECFA, Faculty of Psychology and Educational Sciences, University of Geneva, Geneva, Switzerland
Kalliopi Benetos
English Department, Iowa State University, Ames, IA, USA
Elena Cotos
School of Education, Trinity College Dublin, Dublin, Ireland
TD School, University of Technology Sydney, Sydney, NSW, Australia
Antonette Shibani
Rights and permissions
Open Access This chapter is licensed under the terms of the Creative Commons Attribution 4.0 International License ( http://creativecommons.org/licenses/by/4.0/ ), which permits use, sharing, adaptation, distribution and reproduction in any medium or format, as long as you give appropriate credit to the original author(s) and the source, provide a link to the Creative Commons license and indicate if changes were made.
The images or other third party material in this chapter are included in the chapter's Creative Commons license, unless indicated otherwise in a credit line to the material. If material is not included in the chapter's Creative Commons license and your intended use is not permitted by statutory regulation or exceeds the permitted use, you will need to obtain permission directly from the copyright holder.
Reprints and permissions
Copyright information
© 2023 The Author(s)
About this chapter
Link, S., Koltovskaia, S. (2023). Automated Scoring of Writing. In: Kruse, O., et al. Digital Writing Technologies in Higher Education . Springer, Cham. https://doi.org/10.1007/978-3-031-36033-6_21
Download citation
DOI : https://doi.org/10.1007/978-3-031-36033-6_21
Published : 15 September 2023
Publisher Name : Springer, Cham
Print ISBN : 978-3-031-36032-9
Online ISBN : 978-3-031-36033-6
eBook Packages : Education Education (R0)
Share this chapter
Anyone you share the following link with will be able to read this content:
Sorry, a shareable link is not currently available for this article.
Provided by the Springer Nature SharedIt content-sharing initiative
- Publish with us
Policies and ethics
- Find a journal
- Track your research
- Readers Read
- Screenwriting
- Songwriting
- Writing Contests
Karlie Kloss to Relaunch Life Magazine at Bedford Media
NBF Expands National Book Awards Eligibility Criteria
Striking Writers and Actors March Together on Hollywood Streets
Vice Media Files for Chapter 11 Bankruptcy
Oprah Selects The Covenant of Water as 101st Book Club Pick
- Self-publishing
- Technical Writing
- Writing Prompts

Cookie Policy
Our website uses cookies to understand content and feature usage to drive site improvements over time. To learn more, review our Terms of Use and Privacy Policy .
Project Score: Write Like a Historian
Build structured, rubric-based support into your writing instruction. Provide additional support where needed based on Score, the OER Project’s automated essay-scoring service.
Every history teacher deserves a writing assistant that helps identify and focus on areas for student improvement. Project Score includes lessons, tools, prompts, and an automated essay-scoring service proven to help students write better and improve their historical writing and literacy skills.
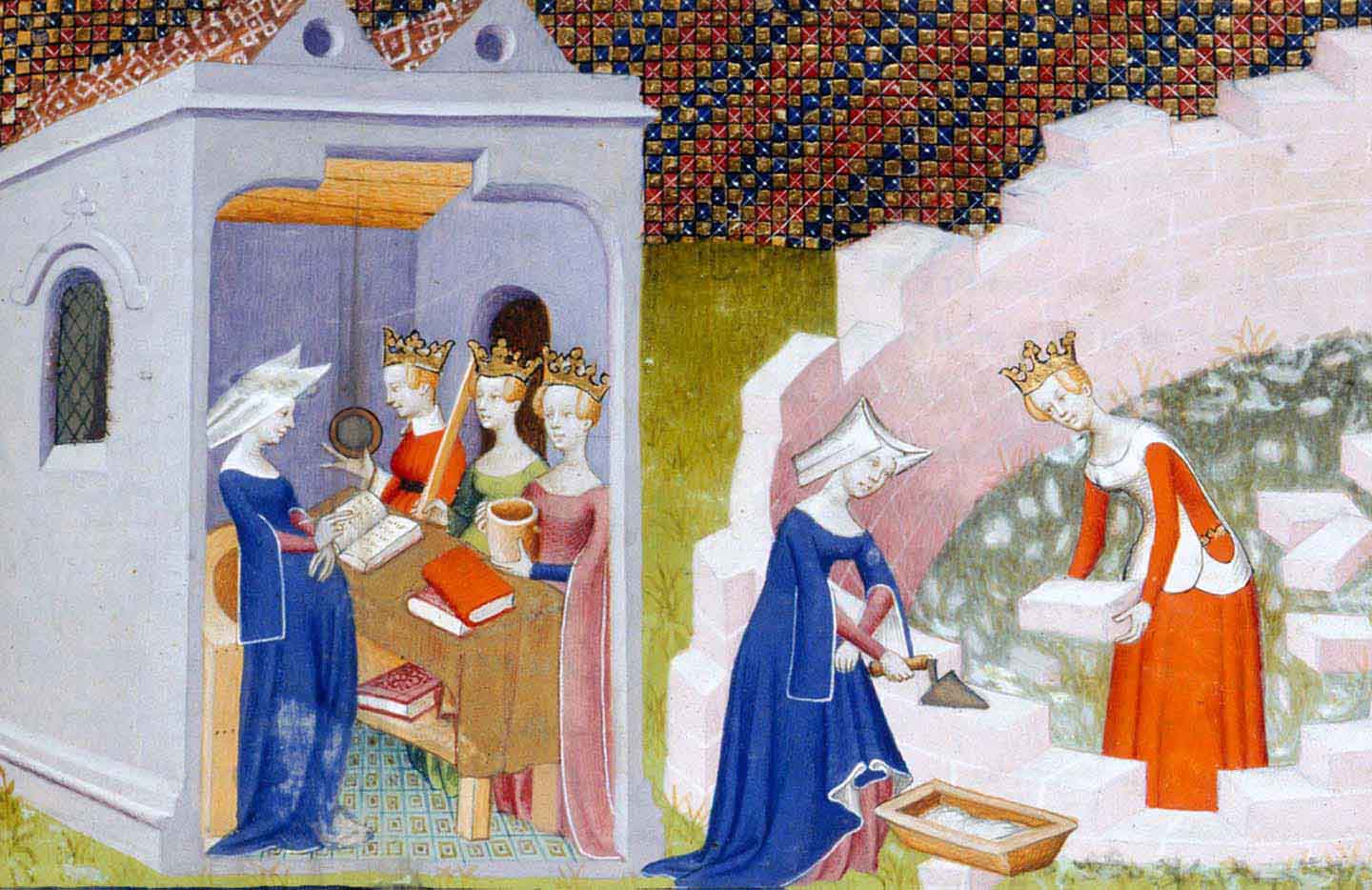
Why Teach with Project Score?
This course features Score, the free OER Project essay-scoring service. When combined with writing prompts and scaffolded pre- and post-writing activities, this powerful tool provides consistent formative feedback and loads of data to inform instruction and discussion.
Prompts: A variety of writing prompts address enduring historical questions about our planet, life, and humanity.
Prewriting: Scaffolded warm-up activities help prepare students for writing by introducing them to key elements of the Score Writing Rubric and giving them ample opportunity to prepare for successful writing.
Postwriting: Getting students to revise their writing can be a challenge. Scaffolded revision activities, focused on the Score Writing Rubric, give students the guidance and structure they need.
Consistent, instant feedback: Students can independently check their writing and receive immediate feedback to help inform their writing.
Informs instruction: Teachers receive individual and class data to help identify who needs extra help or where a class is struggling.
Sparks discussion: Score is not a grading tool—it’s a conversation starter for you and your students and a way for you to make data-driven instructional decisions.
Flexible. Ready to Use. Fully Supported.
Project Score can be taught as a two-week course, or you can use the activities anywhere within the Big History Project or World History Project courses for additional writing instruction. Find comprehensive support from the OER Project team and our vibrant teacher community.
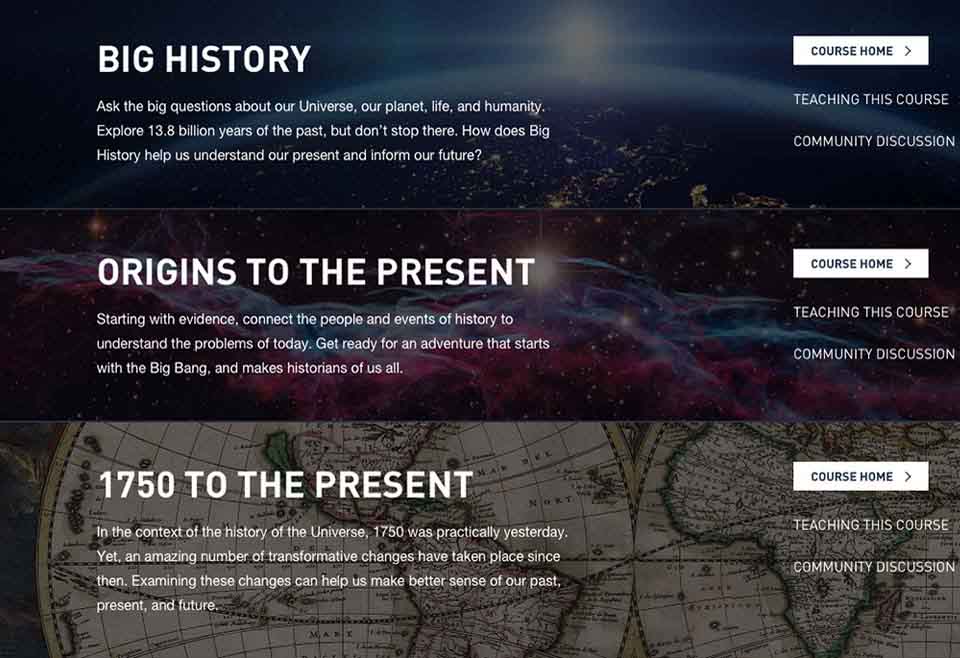
Help yourself to everything our free, online history courses have to offer: mind-blowing content balanced with critical skills development and lots of support.
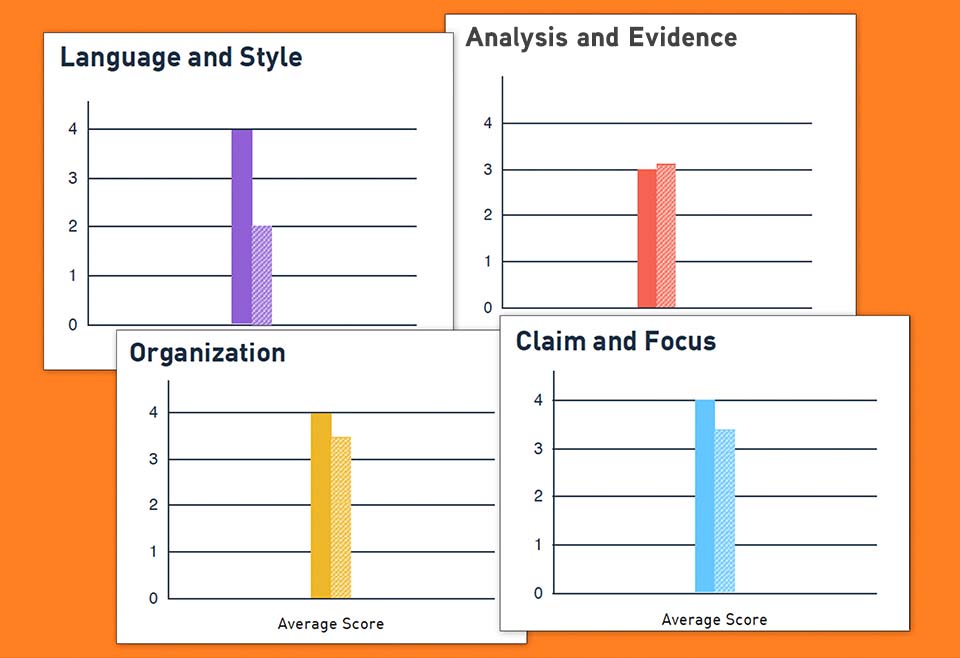
Get started building student writing capabilities—with confidence.
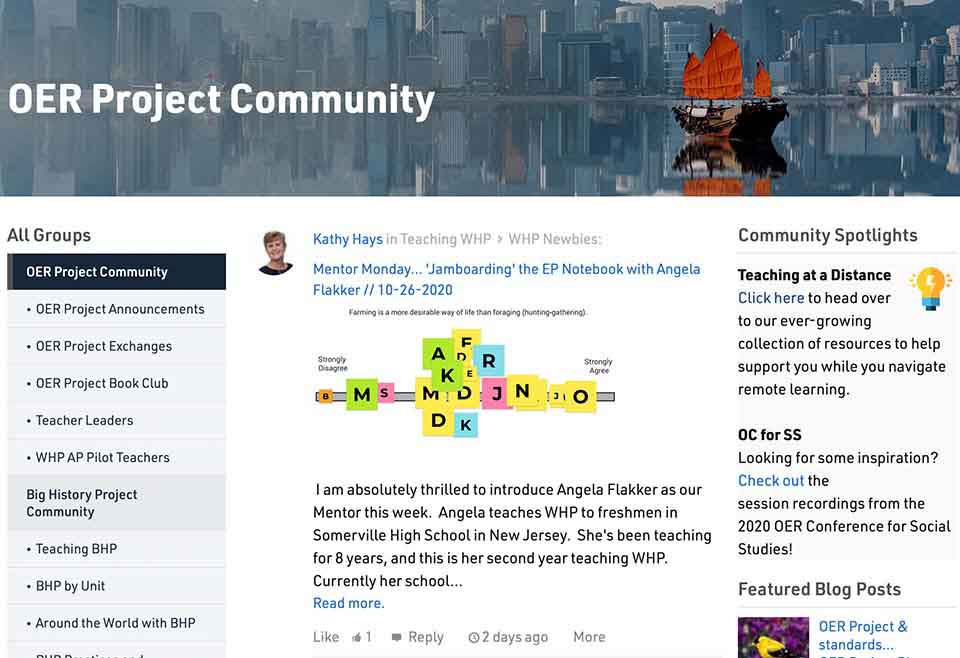
Not sure how you will use this in the classroom? Connect with fellow teachers and scholars in the OER Project Online Teacher Community to get your questions answered.
Extend Student Learning with our Supplemental Units
Try an OER Project supplemental unit to boost skill development in your classroom. These extension materials are the perfect supplement to an existing history or language arts curriculum.
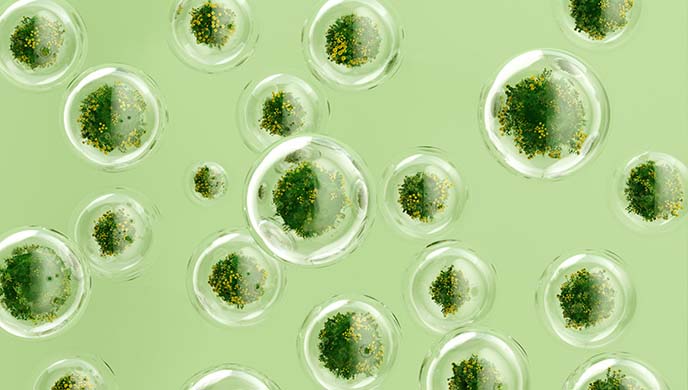
Climate Project Extension
A supplemental unit that starts with evidence and ends with student-developed plans to reach net carbon zero by 2050.
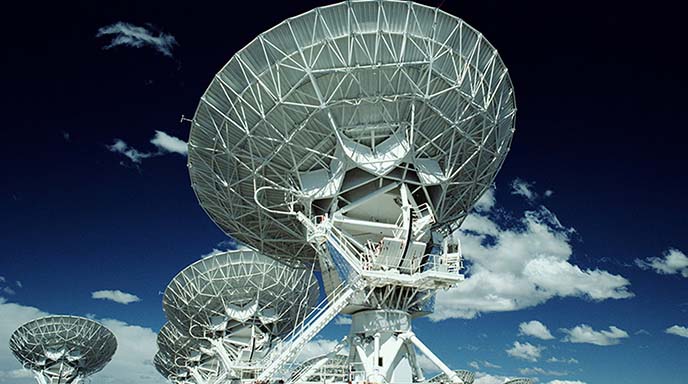
Help students understand and use data to confront urgent world topics such as poverty, democracy, and climate. This 2-week supplement is designed for high-school students and includes 10 data-exploration exercises that lead up to a final class presentation.
Access this course
Join OER Project to get instant access to the Project Score course for FREE with no hidden catches.

An official website of the United States government
The .gov means it’s official. Federal government websites often end in .gov or .mil. Before sharing sensitive information, make sure you’re on a federal government site.
The site is secure. The https:// ensures that you are connecting to the official website and that any information you provide is encrypted and transmitted securely.
- Publications
- Account settings
Preview improvements coming to the PMC website in October 2024. Learn More or Try it out now .
- Advanced Search
- Journal List
- Springer Nature - PMC COVID-19 Collection

An automated essay scoring systems: a systematic literature review
Dadi ramesh.
1 School of Computer Science and Artificial Intelligence, SR University, Warangal, TS India
2 Research Scholar, JNTU, Hyderabad, India
Suresh Kumar Sanampudi
3 Department of Information Technology, JNTUH College of Engineering, Nachupally, Kondagattu, Jagtial, TS India
Associated Data
Assessment in the Education system plays a significant role in judging student performance. The present evaluation system is through human assessment. As the number of teachers' student ratio is gradually increasing, the manual evaluation process becomes complicated. The drawback of manual evaluation is that it is time-consuming, lacks reliability, and many more. This connection online examination system evolved as an alternative tool for pen and paper-based methods. Present Computer-based evaluation system works only for multiple-choice questions, but there is no proper evaluation system for grading essays and short answers. Many researchers are working on automated essay grading and short answer scoring for the last few decades, but assessing an essay by considering all parameters like the relevance of the content to the prompt, development of ideas, Cohesion, and Coherence is a big challenge till now. Few researchers focused on Content-based evaluation, while many of them addressed style-based assessment. This paper provides a systematic literature review on automated essay scoring systems. We studied the Artificial Intelligence and Machine Learning techniques used to evaluate automatic essay scoring and analyzed the limitations of the current studies and research trends. We observed that the essay evaluation is not done based on the relevance of the content and coherence.
Supplementary Information
The online version contains supplementary material available at 10.1007/s10462-021-10068-2.
Introduction
Due to COVID 19 outbreak, an online educational system has become inevitable. In the present scenario, almost all the educational institutions ranging from schools to colleges adapt the online education system. The assessment plays a significant role in measuring the learning ability of the student. Most automated evaluation is available for multiple-choice questions, but assessing short and essay answers remain a challenge. The education system is changing its shift to online-mode, like conducting computer-based exams and automatic evaluation. It is a crucial application related to the education domain, which uses natural language processing (NLP) and Machine Learning techniques. The evaluation of essays is impossible with simple programming languages and simple techniques like pattern matching and language processing. Here the problem is for a single question, we will get more responses from students with a different explanation. So, we need to evaluate all the answers concerning the question.
Automated essay scoring (AES) is a computer-based assessment system that automatically scores or grades the student responses by considering appropriate features. The AES research started in 1966 with the Project Essay Grader (PEG) by Ajay et al. ( 1973 ). PEG evaluates the writing characteristics such as grammar, diction, construction, etc., to grade the essay. A modified version of the PEG by Shermis et al. ( 2001 ) was released, which focuses on grammar checking with a correlation between human evaluators and the system. Foltz et al. ( 1999 ) introduced an Intelligent Essay Assessor (IEA) by evaluating content using latent semantic analysis to produce an overall score. Powers et al. ( 2002 ) proposed E-rater and Intellimetric by Rudner et al. ( 2006 ) and Bayesian Essay Test Scoring System (BESTY) by Rudner and Liang ( 2002 ), these systems use natural language processing (NLP) techniques that focus on style and content to obtain the score of an essay. The vast majority of the essay scoring systems in the 1990s followed traditional approaches like pattern matching and a statistical-based approach. Since the last decade, the essay grading systems started using regression-based and natural language processing techniques. AES systems like Dong et al. ( 2017 ) and others developed from 2014 used deep learning techniques, inducing syntactic and semantic features resulting in better results than earlier systems.
Ohio, Utah, and most US states are using AES systems in school education, like Utah compose tool, Ohio standardized test (an updated version of PEG), evaluating millions of student's responses every year. These systems work for both formative, summative assessments and give feedback to students on the essay. Utah provided basic essay evaluation rubrics (six characteristics of essay writing): Development of ideas, organization, style, word choice, sentence fluency, conventions. Educational Testing Service (ETS) has been conducting significant research on AES for more than a decade and designed an algorithm to evaluate essays on different domains and providing an opportunity for test-takers to improve their writing skills. In addition, they are current research content-based evaluation.
The evaluation of essay and short answer scoring should consider the relevance of the content to the prompt, development of ideas, Cohesion, Coherence, and domain knowledge. Proper assessment of the parameters mentioned above defines the accuracy of the evaluation system. But all these parameters cannot play an equal role in essay scoring and short answer scoring. In a short answer evaluation, domain knowledge is required, like the meaning of "cell" in physics and biology is different. And while evaluating essays, the implementation of ideas with respect to prompt is required. The system should also assess the completeness of the responses and provide feedback.
Several studies examined AES systems, from the initial to the latest AES systems. In which the following studies on AES systems are Blood ( 2011 ) provided a literature review from PEG 1984–2010. Which has covered only generalized parts of AES systems like ethical aspects, the performance of the systems. Still, they have not covered the implementation part, and it’s not a comparative study and has not discussed the actual challenges of AES systems.
Burrows et al. ( 2015 ) Reviewed AES systems on six dimensions like dataset, NLP techniques, model building, grading models, evaluation, and effectiveness of the model. They have not covered feature extraction techniques and challenges in features extractions. Covered only Machine Learning models but not in detail. This system not covered the comparative analysis of AES systems like feature extraction, model building, and level of relevance, cohesion, and coherence not covered in this review.
Ke et al. ( 2019 ) provided a state of the art of AES system but covered very few papers and not listed all challenges, and no comparative study of the AES model. On the other hand, Hussein et al. in ( 2019 ) studied two categories of AES systems, four papers from handcrafted features for AES systems, and four papers from the neural networks approach, discussed few challenges, and did not cover feature extraction techniques, the performance of AES models in detail.
Klebanov et al. ( 2020 ). Reviewed 50 years of AES systems, listed and categorized all essential features that need to be extracted from essays. But not provided a comparative analysis of all work and not discussed the challenges.
This paper aims to provide a systematic literature review (SLR) on automated essay grading systems. An SLR is an Evidence-based systematic review to summarize the existing research. It critically evaluates and integrates all relevant studies' findings and addresses the research domain's specific research questions. Our research methodology uses guidelines given by Kitchenham et al. ( 2009 ) for conducting the review process; provide a well-defined approach to identify gaps in current research and to suggest further investigation.
We addressed our research method, research questions, and the selection process in Sect. 2 , and the results of the research questions have discussed in Sect. 3 . And the synthesis of all the research questions addressed in Sect. 4 . Conclusion and possible future work discussed in Sect. 5 .
Research method
We framed the research questions with PICOC criteria.
Population (P) Student essays and answers evaluation systems.
Intervention (I) evaluation techniques, data sets, features extraction methods.
Comparison (C) Comparison of various approaches and results.
Outcomes (O) Estimate the accuracy of AES systems,
Context (C) NA.
Research questions
To collect and provide research evidence from the available studies in the domain of automated essay grading, we framed the following research questions (RQ):
RQ1 what are the datasets available for research on automated essay grading?
The answer to the question can provide a list of the available datasets, their domain, and access to the datasets. It also provides a number of essays and corresponding prompts.
RQ2 what are the features extracted for the assessment of essays?
The answer to the question can provide an insight into various features so far extracted, and the libraries used to extract those features.
RQ3, which are the evaluation metrics available for measuring the accuracy of algorithms?
The answer will provide different evaluation metrics for accurate measurement of each Machine Learning approach and commonly used measurement technique.
RQ4 What are the Machine Learning techniques used for automatic essay grading, and how are they implemented?
It can provide insights into various Machine Learning techniques like regression models, classification models, and neural networks for implementing essay grading systems. The response to the question can give us different assessment approaches for automated essay grading systems.
RQ5 What are the challenges/limitations in the current research?
The answer to the question provides limitations of existing research approaches like cohesion, coherence, completeness, and feedback.
Search process
We conducted an automated search on well-known computer science repositories like ACL, ACM, IEEE Explore, Springer, and Science Direct for an SLR. We referred to papers published from 2010 to 2020 as much of the work during these years focused on advanced technologies like deep learning and natural language processing for automated essay grading systems. Also, the availability of free data sets like Kaggle (2012), Cambridge Learner Corpus-First Certificate in English exam (CLC-FCE) by Yannakoudakis et al. ( 2011 ) led to research this domain.
Search Strings : We used search strings like “Automated essay grading” OR “Automated essay scoring” OR “short answer scoring systems” OR “essay scoring systems” OR “automatic essay evaluation” and searched on metadata.
Selection criteria
After collecting all relevant documents from the repositories, we prepared selection criteria for inclusion and exclusion of documents. With the inclusion and exclusion criteria, it becomes more feasible for the research to be accurate and specific.
Inclusion criteria 1 Our approach is to work with datasets comprise of essays written in English. We excluded the essays written in other languages.
Inclusion criteria 2 We included the papers implemented on the AI approach and excluded the traditional methods for the review.
Inclusion criteria 3 The study is on essay scoring systems, so we exclusively included the research carried out on only text data sets rather than other datasets like image or speech.
Exclusion criteria We removed the papers in the form of review papers, survey papers, and state of the art papers.
Quality assessment
In addition to the inclusion and exclusion criteria, we assessed each paper by quality assessment questions to ensure the article's quality. We included the documents that have clearly explained the approach they used, the result analysis and validation.
The quality checklist questions are framed based on the guidelines from Kitchenham et al. ( 2009 ). Each quality assessment question was graded as either 1 or 0. The final score of the study range from 0 to 3. A cut off score for excluding a study from the review is 2 points. Since the papers scored 2 or 3 points are included in the final evaluation. We framed the following quality assessment questions for the final study.
Quality Assessment 1: Internal validity.
Quality Assessment 2: External validity.
Quality Assessment 3: Bias.
The two reviewers review each paper to select the final list of documents. We used the Quadratic Weighted Kappa score to measure the final agreement between the two reviewers. The average resulted from the kappa score is 0.6942, a substantial agreement between the reviewers. The result of evolution criteria shown in Table Table1. 1 . After Quality Assessment, the final list of papers for review is shown in Table Table2. 2 . The complete selection process is shown in Fig. Fig.1. 1 . The total number of selected papers in year wise as shown in Fig. Fig.2. 2 .
Quality assessment analysis
Final list of papers

Selection process

Year wise publications
What are the datasets available for research on automated essay grading?
To work with problem statement especially in Machine Learning and deep learning domain, we require considerable amount of data to train the models. To answer this question, we listed all the data sets used for training and testing for automated essay grading systems. The Cambridge Learner Corpus-First Certificate in English exam (CLC-FCE) Yannakoudakis et al. ( 2011 ) developed corpora that contain 1244 essays and ten prompts. This corpus evaluates whether a student can write the relevant English sentences without any grammatical and spelling mistakes. This type of corpus helps to test the models built for GRE and TOFEL type of exams. It gives scores between 1 and 40.
Bailey and Meurers ( 2008 ), Created a dataset (CREE reading comprehension) for language learners and automated short answer scoring systems. The corpus consists of 566 responses from intermediate students. Mohler and Mihalcea ( 2009 ). Created a dataset for the computer science domain consists of 630 responses for data structure assignment questions. The scores are range from 0 to 5 given by two human raters.
Dzikovska et al. ( 2012 ) created a Student Response Analysis (SRA) corpus. It consists of two sub-groups: the BEETLE corpus consists of 56 questions and approximately 3000 responses from students in the electrical and electronics domain. The second one is the SCIENTSBANK(SemEval-2013) (Dzikovska et al. 2013a ; b ) corpus consists of 10,000 responses on 197 prompts on various science domains. The student responses ladled with "correct, partially correct incomplete, Contradictory, Irrelevant, Non-domain."
In the Kaggle (2012) competition, released total 3 types of corpuses on an Automated Student Assessment Prize (ASAP1) (“ https://www.kaggle.com/c/asap-sas/ ” ) essays and short answers. It has nearly 17,450 essays, out of which it provides up to 3000 essays for each prompt. It has eight prompts that test 7th to 10th grade US students. It gives scores between the [0–3] and [0–60] range. The limitations of these corpora are: (1) it has a different score range for other prompts. (2) It uses statistical features such as named entities extraction and lexical features of words to evaluate essays. ASAP + + is one more dataset from Kaggle. It is with six prompts, and each prompt has more than 1000 responses total of 10,696 from 8th-grade students. Another corpus contains ten prompts from science, English domains and a total of 17,207 responses. Two human graders evaluated all these responses.
Correnti et al. ( 2013 ) created a Response-to-Text Assessment (RTA) dataset used to check student writing skills in all directions like style, mechanism, and organization. 4–8 grade students give the responses to RTA. Basu et al. ( 2013 ) created a power grading dataset with 700 responses for ten different prompts from US immigration exams. It contains all short answers for assessment.
The TOEFL11 corpus Blanchard et al. ( 2013 ) contains 1100 essays evenly distributed over eight prompts. It is used to test the English language skills of a candidate attending the TOFEL exam. It scores the language proficiency of a candidate as low, medium, and high.
International Corpus of Learner English (ICLE) Granger et al. ( 2009 ) built a corpus of 3663 essays covering different dimensions. It has 12 prompts with 1003 essays that test the organizational skill of essay writing, and13 prompts, each with 830 essays that examine the thesis clarity and prompt adherence.
Argument Annotated Essays (AAE) Stab and Gurevych ( 2014 ) developed a corpus that contains 102 essays with 101 prompts taken from the essayforum2 site. It tests the persuasive nature of the student essay. The SCIENTSBANK corpus used by Sakaguchi et al. ( 2015 ) available in git-hub, containing 9804 answers to 197 questions in 15 science domains. Table Table3 3 illustrates all datasets related to AES systems.
ALL types Datasets used in Automatic scoring systems
Features play a major role in the neural network and other supervised Machine Learning approaches. The automatic essay grading systems scores student essays based on different types of features, which play a prominent role in training the models. Based on their syntax and semantics and they are categorized into three groups. 1. statistical-based features Contreras et al. ( 2018 ); Kumar et al. ( 2019 ); Mathias and Bhattacharyya ( 2018a ; b ) 2. Style-based (Syntax) features Cummins et al. ( 2016 ); Darwish and Mohamed ( 2020 ); Ke et al. ( 2019 ). 3. Content-based features Dong et al. ( 2017 ). A good set of features appropriate models evolved better AES systems. The vast majority of the researchers are using regression models if features are statistical-based. For Neural Networks models, researches are using both style-based and content-based features. The following table shows the list of various features used in existing AES Systems. Table Table4 4 represents all set of features used for essay grading.
Types of features
We studied all the feature extracting NLP libraries as shown in Fig. Fig.3. that 3 . that are used in the papers. The NLTK is an NLP tool used to retrieve statistical features like POS, word count, sentence count, etc. With NLTK, we can miss the essay's semantic features. To find semantic features Word2Vec Mikolov et al. ( 2013 ), GloVe Jeffrey Pennington et al. ( 2014 ) is the most used libraries to retrieve the semantic text from the essays. And in some systems, they directly trained the model with word embeddings to find the score. From Fig. Fig.4 4 as observed that non-content-based feature extraction is higher than content-based.

Usages of tools

Number of papers on content based features
RQ3 which are the evaluation metrics available for measuring the accuracy of algorithms?
The majority of the AES systems are using three evaluation metrics. They are (1) quadrated weighted kappa (QWK) (2) Mean Absolute Error (MAE) (3) Pearson Correlation Coefficient (PCC) Shehab et al. ( 2016 ). The quadratic weighted kappa will find agreement between human evaluation score and system evaluation score and produces value ranging from 0 to 1. And the Mean Absolute Error is the actual difference between human-rated score to system-generated score. The mean square error (MSE) measures the average squares of the errors, i.e., the average squared difference between the human-rated and the system-generated scores. MSE will always give positive numbers only. Pearson's Correlation Coefficient (PCC) finds the correlation coefficient between two variables. It will provide three values (0, 1, − 1). "0" represents human-rated and system scores that are not related. "1" represents an increase in the two scores. "− 1" illustrates a negative relationship between the two scores.
RQ4 what are the Machine Learning techniques being used for automatic essay grading, and how are they implemented?
After scrutinizing all documents, we categorize the techniques used in automated essay grading systems into four baskets. 1. Regression techniques. 2. Classification model. 3. Neural networks. 4. Ontology-based approach.
All the existing AES systems developed in the last ten years employ supervised learning techniques. Researchers using supervised methods viewed the AES system as either regression or classification task. The goal of the regression task is to predict the score of an essay. The classification task is to classify the essays belonging to (low, medium, or highly) relevant to the question's topic. Since the last three years, most AES systems developed made use of the concept of the neural network.
Regression based models
Mohler and Mihalcea ( 2009 ). proposed text-to-text semantic similarity to assign a score to the student essays. There are two text similarity measures like Knowledge-based measures, corpus-based measures. There eight knowledge-based tests with all eight models. They found the similarity. The shortest path similarity determines based on the length, which shortest path between two contexts. Leacock & Chodorow find the similarity based on the shortest path's length between two concepts using node-counting. The Lesk similarity finds the overlap between the corresponding definitions, and Wu & Palmer algorithm finds similarities based on the depth of two given concepts in the wordnet taxonomy. Resnik, Lin, Jiang&Conrath, Hirst& St-Onge find the similarity based on different parameters like the concept, probability, normalization factor, lexical chains. In corpus-based likeness, there LSA BNC, LSA Wikipedia, and ESA Wikipedia, latent semantic analysis is trained on Wikipedia and has excellent domain knowledge. Among all similarity scores, correlation scores LSA Wikipedia scoring accuracy is more. But these similarity measure algorithms are not using NLP concepts. These models are before 2010 and basic concept models to continue the research automated essay grading with updated algorithms on neural networks with content-based features.
Adamson et al. ( 2014 ) proposed an automatic essay grading system which is a statistical-based approach in this they retrieved features like POS, Character count, Word count, Sentence count, Miss spelled words, n-gram representation of words to prepare essay vector. They formed a matrix with these all vectors in that they applied LSA to give a score to each essay. It is a statistical approach that doesn’t consider the semantics of the essay. The accuracy they got when compared to the human rater score with the system is 0.532.
Cummins et al. ( 2016 ). Proposed Timed Aggregate Perceptron vector model to give ranking to all the essays, and later they converted the rank algorithm to predict the score of the essay. The model trained with features like Word unigrams, bigrams, POS, Essay length, grammatical relation, Max word length, sentence length. It is multi-task learning, gives ranking to the essays, and predicts the score for the essay. The performance evaluated through QWK is 0.69, a substantial agreement between the human rater and the system.
Sultan et al. ( 2016 ). Proposed a Ridge regression model to find short answer scoring with Question Demoting. Question Demoting is the new concept included in the essay's final assessment to eliminate duplicate words from the essay. The extracted features are Text Similarity, which is the similarity between the student response and reference answer. Question Demoting is the number of repeats in a student response. With inverse document frequency, they assigned term weight. The sentence length Ratio is the number of words in the student response, is another feature. With these features, the Ridge regression model was used, and the accuracy they got 0.887.
Contreras et al. ( 2018 ). Proposed Ontology based on text mining in this model has given a score for essays in phases. In phase-I, they generated ontologies with ontoGen and SVM to find the concept and similarity in the essay. In phase II from ontologies, they retrieved features like essay length, word counts, correctness, vocabulary, and types of word used, domain information. After retrieving statistical data, they used a linear regression model to find the score of the essay. The accuracy score is the average of 0.5.
Darwish and Mohamed ( 2020 ) proposed the fusion of fuzzy Ontology with LSA. They retrieve two types of features, like syntax features and semantic features. In syntax features, they found Lexical Analysis with tokens, and they construct a parse tree. If the parse tree is broken, the essay is inconsistent—a separate grade assigned to the essay concerning syntax features. The semantic features are like similarity analysis, Spatial Data Analysis. Similarity analysis is to find duplicate sentences—Spatial Data Analysis for finding Euclid distance between the center and part. Later they combine syntax features and morphological features score for the final score. The accuracy they achieved with the multiple linear regression model is 0.77, mostly on statistical features.
Süzen Neslihan et al. ( 2020 ) proposed a text mining approach for short answer grading. First, their comparing model answers with student response by calculating the distance between two sentences. By comparing the model answer with student response, they find the essay's completeness and provide feedback. In this approach, model vocabulary plays a vital role in grading, and with this model vocabulary, the grade will be assigned to the student's response and provides feedback. The correlation between the student answer to model answer is 0.81.
Classification based Models
Persing and Ng ( 2013 ) used a support vector machine to score the essay. The features extracted are OS, N-gram, and semantic text to train the model and identified the keywords from the essay to give the final score.
Sakaguchi et al. ( 2015 ) proposed two methods: response-based and reference-based. In response-based scoring, the extracted features are response length, n-gram model, and syntactic elements to train the support vector regression model. In reference-based scoring, features such as sentence similarity using word2vec is used to find the cosine similarity of the sentences that is the final score of the response. First, the scores were discovered individually and later combined two features to find a final score. This system gave a remarkable increase in performance by combining the scores.
Mathias and Bhattacharyya ( 2018a ; b ) Proposed Automated Essay Grading Dataset with Essay Attribute Scores. The first concept features selection depends on the essay type. So the common attributes are Content, Organization, Word Choice, Sentence Fluency, Conventions. In this system, each attribute is scored individually, with the strength of each attribute identified. The model they used is a random forest classifier to assign scores to individual attributes. The accuracy they got with QWK is 0.74 for prompt 1 of the ASAS dataset ( https://www.kaggle.com/c/asap-sas/ ).
Ke et al. ( 2019 ) used a support vector machine to find the response score. In this method, features like Agreeability, Specificity, Clarity, Relevance to prompt, Conciseness, Eloquence, Confidence, Direction of development, Justification of opinion, and Justification of importance. First, the individual parameter score obtained was later combined with all scores to give a final response score. The features are used in the neural network to find whether the sentence is relevant to the topic or not.
Salim et al. ( 2019 ) proposed an XGBoost Machine Learning classifier to assess the essays. The algorithm trained on features like word count, POS, parse tree depth, and coherence in the articles with sentence similarity percentage; cohesion and coherence are considered for training. And they implemented K-fold cross-validation for a result the average accuracy after specific validations is 68.12.
Neural network models
Shehab et al. ( 2016 ) proposed a neural network method that used learning vector quantization to train human scored essays. After training, the network can provide a score to the ungraded essays. First, we should process the essay to remove Spell checking and then perform preprocessing steps like Document Tokenization, stop word removal, Stemming, and submit it to the neural network. Finally, the model will provide feedback on the essay, whether it is relevant to the topic. And the correlation coefficient between human rater and system score is 0.7665.
Kopparapu and De ( 2016 ) proposed the Automatic Ranking of Essays using Structural and Semantic Features. This approach constructed a super essay with all the responses. Next, ranking for a student essay is done based on the super-essay. The structural and semantic features derived helps to obtain the scores. In a paragraph, 15 Structural features like an average number of sentences, the average length of sentences, and the count of words, nouns, verbs, adjectives, etc., are used to obtain a syntactic score. A similarity score is used as semantic features to calculate the overall score.
Dong and Zhang ( 2016 ) proposed a hierarchical CNN model. The model builds two layers with word embedding to represents the words as the first layer. The second layer is a word convolution layer with max-pooling to find word vectors. The next layer is a sentence-level convolution layer with max-pooling to find the sentence's content and synonyms. A fully connected dense layer produces an output score for an essay. The accuracy with the hierarchical CNN model resulted in an average QWK of 0.754.
Taghipour and Ng ( 2016 ) proposed a first neural approach for essay scoring build in which convolution and recurrent neural network concepts help in scoring an essay. The network uses a lookup table with the one-hot representation of the word vector of an essay. The final efficiency of the network model with LSTM resulted in an average QWK of 0.708.
Dong et al. ( 2017 ). Proposed an Attention-based scoring system with CNN + LSTM to score an essay. For CNN, the input parameters were character embedding and word embedding, and it has attention pooling layers and used NLTK to obtain word and character embedding. The output gives a sentence vector, which provides sentence weight. After CNN, it will have an LSTM layer with an attention pooling layer, and this final layer results in the final score of the responses. The average QWK score is 0.764.
Riordan et al. ( 2017 ) proposed a neural network with CNN and LSTM layers. Word embedding, given as input to a neural network. An LSTM network layer will retrieve the window features and delivers them to the aggregation layer. The aggregation layer is a superficial layer that takes a correct window of words and gives successive layers to predict the answer's sore. The accuracy of the neural network resulted in a QWK of 0.90.
Zhao et al. ( 2017 ) proposed a new concept called Memory-Augmented Neural network with four layers, input representation layer, memory addressing layer, memory reading layer, and output layer. An input layer represents all essays in a vector form based on essay length. After converting the word vector, the memory addressing layer takes a sample of the essay and weighs all the terms. The memory reading layer takes the input from memory addressing segment and finds the content to finalize the score. Finally, the output layer will provide the final score of the essay. The accuracy of essay scores is 0.78, which is far better than the LSTM neural network.
Mathias and Bhattacharyya ( 2018a ; b ) proposed deep learning networks using LSTM with the CNN layer and GloVe pre-trained word embeddings. For this, they retrieved features like Sentence count essays, word count per sentence, Number of OOVs in the sentence, Language model score, and the text's perplexity. The network predicted the goodness scores of each essay. The higher the goodness scores, means higher the rank and vice versa.
Nguyen and Dery ( 2016 ). Proposed Neural Networks for Automated Essay Grading. In this method, a single layer bi-directional LSTM accepting word vector as input. Glove vectors used in this method resulted in an accuracy of 90%.
Ruseti et al. ( 2018 ) proposed a recurrent neural network that is capable of memorizing the text and generate a summary of an essay. The Bi-GRU network with the max-pooling layer molded on the word embedding of each document. It will provide scoring to the essay by comparing it with a summary of the essay from another Bi-GRU network. The result obtained an accuracy of 0.55.
Wang et al. ( 2018a ; b ) proposed an automatic scoring system with the bi-LSTM recurrent neural network model and retrieved the features using the word2vec technique. This method generated word embeddings from the essay words using the skip-gram model. And later, word embedding is used to train the neural network to find the final score. The softmax layer in LSTM obtains the importance of each word. This method used a QWK score of 0.83%.
Dasgupta et al. ( 2018 ) proposed a technique for essay scoring with augmenting textual qualitative Features. It extracted three types of linguistic, cognitive, and psychological features associated with a text document. The linguistic features are Part of Speech (POS), Universal Dependency relations, Structural Well-formedness, Lexical Diversity, Sentence Cohesion, Causality, and Informativeness of the text. The psychological features derived from the Linguistic Information and Word Count (LIWC) tool. They implemented a convolution recurrent neural network that takes input as word embedding and sentence vector, retrieved from the GloVe word vector. And the second layer is the Convolution Layer to find local features. The next layer is the recurrent neural network (LSTM) to find corresponding of the text. The accuracy of this method resulted in an average QWK of 0.764.
Liang et al. ( 2018 ) proposed a symmetrical neural network AES model with Bi-LSTM. They are extracting features from sample essays and student essays and preparing an embedding layer as input. The embedding layer output is transfer to the convolution layer from that LSTM will be trained. Hear the LSRM model has self-features extraction layer, which will find the essay's coherence. The average QWK score of SBLSTMA is 0.801.
Liu et al. ( 2019 ) proposed two-stage learning. In the first stage, they are assigning a score based on semantic data from the essay. The second stage scoring is based on some handcrafted features like grammar correction, essay length, number of sentences, etc. The average score of the two stages is 0.709.
Pedro Uria Rodriguez et al. ( 2019 ) proposed a sequence-to-sequence learning model for automatic essay scoring. They used BERT (Bidirectional Encoder Representations from Transformers), which extracts the semantics from a sentence from both directions. And XLnet sequence to sequence learning model to extract features like the next sentence in an essay. With this pre-trained model, they attained coherence from the essay to give the final score. The average QWK score of the model is 75.5.
Xia et al. ( 2019 ) proposed a two-layer Bi-directional LSTM neural network for the scoring of essays. The features extracted with word2vec to train the LSTM and accuracy of the model in an average of QWK is 0.870.
Kumar et al. ( 2019 ) Proposed an AutoSAS for short answer scoring. It used pre-trained Word2Vec and Doc2Vec models trained on Google News corpus and Wikipedia dump, respectively, to retrieve the features. First, they tagged every word POS and they found weighted words from the response. It also found prompt overlap to observe how the answer is relevant to the topic, and they defined lexical overlaps like noun overlap, argument overlap, and content overlap. This method used some statistical features like word frequency, difficulty, diversity, number of unique words in each response, type-token ratio, statistics of the sentence, word length, and logical operator-based features. This method uses a random forest model to train the dataset. The data set has sample responses with their associated score. The model will retrieve the features from both responses like graded and ungraded short answers with questions. The accuracy of AutoSAS with QWK is 0.78. It will work on any topics like Science, Arts, Biology, and English.
Jiaqi Lun et al. ( 2020 ) proposed an automatic short answer scoring with BERT. In this with a reference answer comparing student responses and assigning scores. The data augmentation is done with a neural network and with one correct answer from the dataset classifying reaming responses as correct or incorrect.
Zhu and Sun ( 2020 ) proposed a multimodal Machine Learning approach for automated essay scoring. First, they count the grammar score with the spaCy library and numerical count as the number of words and sentences with the same library. With this input, they trained a single and Bi LSTM neural network for finding the final score. For the LSTM model, they prepared sentence vectors with GloVe and word embedding with NLTK. Bi-LSTM will check each sentence in both directions to find semantic from the essay. The average QWK score with multiple models is 0.70.
Ontology based approach
Mohler et al. ( 2011 ) proposed a graph-based method to find semantic similarity in short answer scoring. For the ranking of answers, they used the support vector regression model. The bag of words is the main feature extracted in the system.
Ramachandran et al. ( 2015 ) also proposed a graph-based approach to find lexical based semantics. Identified phrase patterns and text patterns are the features to train a random forest regression model to score the essays. The accuracy of the model in a QWK is 0.78.
Zupanc et al. ( 2017 ) proposed sentence similarity networks to find the essay's score. Ajetunmobi and Daramola ( 2017 ) recommended an ontology-based information extraction approach and domain-based ontology to find the score.

Speech response scoring
Automatic scoring is in two ways one is text-based scoring, other is speech-based scoring. This paper discussed text-based scoring and its challenges, and now we cover speech scoring and common points between text and speech-based scoring. Evanini and Wang ( 2013 ), Worked on speech scoring of non-native school students, extracted features with speech ratter, and trained a linear regression model, concluding that accuracy varies based on voice pitching. Loukina et al. ( 2015 ) worked on feature selection from speech data and trained SVM. Malinin et al. ( 2016 ) used neural network models to train the data. Loukina et al. ( 2017 ). Proposed speech and text-based automatic scoring. Extracted text-based features, speech-based features and trained a deep neural network for speech-based scoring. They extracted 33 types of features based on acoustic signals. Malinin et al. ( 2017 ). Wu Xixin et al. ( 2020 ) Worked on deep neural networks for spoken language assessment. Incorporated different types of models and tested them. Ramanarayanan et al. ( 2017 ) worked on feature extraction methods and extracted punctuation, fluency, and stress and trained different Machine Learning models for scoring. Knill et al. ( 2018 ). Worked on Automatic speech recognizer and its errors how its impacts the speech assessment.
The state of the art
This section provides an overview of the existing AES systems with a comparative study w. r. t models, features applied, datasets, and evaluation metrics used for building the automated essay grading systems. We divided all 62 papers into two sets of the first set of review papers in Table Table5 5 with a comparative study of the AES systems.
State of the art
Comparison of all approaches
In our study, we divided major AES approaches into three categories. Regression models, classification models, and neural network models. The regression models failed to find cohesion and coherence from the essay because it trained on BoW(Bag of Words) features. In processing data from input to output, the regression models are less complicated than neural networks. There are unable to find many intricate patterns from the essay and unable to find sentence connectivity. If we train the model with BoW features in the neural network approach, the model never considers the essay's coherence and coherence.
First, to train a Machine Learning algorithm with essays, all the essays are converted to vector form. We can form a vector with BoW and Word2vec, TF-IDF. The BoW and Word2vec vector representation of essays represented in Table Table6. 6 . The vector representation of BoW with TF-IDF is not incorporating the essays semantic, and it’s just statistical learning from a given vector. Word2vec vector comprises semantic of essay in a unidirectional way.
Vector representation of essays
In BoW, the vector contains the frequency of word occurrences in the essay. The vector represents 1 and more based on the happenings of words in the essay and 0 for not present. So, in BoW, the vector does not maintain the relationship with adjacent words; it’s just for single words. In word2vec, the vector represents the relationship between words with other words and sentences prompt in multiple dimensional ways. But word2vec prepares vectors in a unidirectional way, not in a bidirectional way; word2vec fails to find semantic vectors when a word has two meanings, and the meaning depends on adjacent words. Table Table7 7 represents a comparison of Machine Learning models and features extracting methods.
Comparison of models
In AES, cohesion and coherence will check the content of the essay concerning the essay prompt these can be extracted from essay in the vector from. Two more parameters are there to access an essay is completeness and feedback. Completeness will check whether student’s response is sufficient or not though the student wrote correctly. Table Table8 8 represents all four parameters comparison for essay grading. Table Table9 9 illustrates comparison of all approaches based on various features like grammar, spelling, organization of essay, relevance.
Comparison of all models with respect to cohesion, coherence, completeness, feedback
comparison of all approaches on various features
What are the challenges/limitations in the current research?
From our study and results discussed in the previous sections, many researchers worked on automated essay scoring systems with numerous techniques. We have statistical methods, classification methods, and neural network approaches to evaluate the essay automatically. The main goal of the automated essay grading system is to reduce human effort and improve consistency.
The vast majority of essay scoring systems are dealing with the efficiency of the algorithm. But there are many challenges in automated essay grading systems. One should assess the essay by following parameters like the relevance of the content to the prompt, development of ideas, Cohesion, Coherence, and domain knowledge.
No model works on the relevance of content, which means whether student response or explanation is relevant to the given prompt or not if it is relevant to how much it is appropriate, and there is no discussion about the cohesion and coherence of the essays. All researches concentrated on extracting the features using some NLP libraries, trained their models, and testing the results. But there is no explanation in the essay evaluation system about consistency and completeness, But Palma and Atkinson ( 2018 ) explained coherence-based essay evaluation. And Zupanc and Bosnic ( 2014 ) also used the word coherence to evaluate essays. And they found consistency with latent semantic analysis (LSA) for finding coherence from essays, but the dictionary meaning of coherence is "The quality of being logical and consistent."
Another limitation is there is no domain knowledge-based evaluation of essays using Machine Learning models. For example, the meaning of a cell is different from biology to physics. Many Machine Learning models extract features with WordVec and GloVec; these NLP libraries cannot convert the words into vectors when they have two or more meanings.
Other challenges that influence the Automated Essay Scoring Systems.
All these approaches worked to improve the QWK score of their models. But QWK will not assess the model in terms of features extraction and constructed irrelevant answers. The QWK is not evaluating models whether the model is correctly assessing the answer or not. There are many challenges concerning students' responses to the Automatic scoring system. Like in evaluating approach, no model has examined how to evaluate the constructed irrelevant and adversarial answers. Especially the black box type of approaches like deep learning models provides more options to the students to bluff the automated scoring systems.
The Machine Learning models that work on statistical features are very vulnerable. Based on Powers et al. ( 2001 ) and Bejar Isaac et al. ( 2014 ), the E-rater was failed on Constructed Irrelevant Responses Strategy (CIRS). From the study of Bejar et al. ( 2013 ), Higgins and Heilman ( 2014 ), observed that when student response contain irrelevant content or shell language concurring to prompt will influence the final score of essays in an automated scoring system.
In deep learning approaches, most of the models automatically read the essay's features, and some methods work on word-based embedding and other character-based embedding features. From the study of Riordan Brain et al. ( 2019 ), The character-based embedding systems do not prioritize spelling correction. However, it is influencing the final score of the essay. From the study of Horbach and Zesch ( 2019 ), Various factors are influencing AES systems. For example, there are data set size, prompt type, answer length, training set, and human scorers for content-based scoring.
Ding et al. ( 2020 ) reviewed that the automated scoring system is vulnerable when a student response contains more words from prompt, like prompt vocabulary repeated in the response. Parekh et al. ( 2020 ) and Kumar et al. ( 2020 ) tested various neural network models of AES by iteratively adding important words, deleting unimportant words, shuffle the words, and repeating sentences in an essay and found that no change in the final score of essays. These neural network models failed to recognize common sense in adversaries' essays and give more options for the students to bluff the automated systems.
Other than NLP and ML techniques for AES. From Wresch ( 1993 ) to Madnani and Cahill ( 2018 ). discussed the complexity of AES systems, standards need to be followed. Like assessment rubrics to test subject knowledge, irrelevant responses, and ethical aspects of an algorithm like measuring the fairness of student response.
Fairness is an essential factor for automated systems. For example, in AES, fairness can be measure in an agreement between human score to machine score. Besides this, From Loukina et al. ( 2019 ), the fairness standards include overall score accuracy, overall score differences, and condition score differences between human and system scores. In addition, scoring different responses in the prospect of constructive relevant and irrelevant will improve fairness.
Madnani et al. ( 2017a ; b ). Discussed the fairness of AES systems for constructed responses and presented RMS open-source tool for detecting biases in the models. With this, one can change fairness standards according to their analysis of fairness.
From Berzak et al.'s ( 2018 ) approach, behavior factors are a significant challenge in automated scoring systems. That helps to find language proficiency, word characteristics (essential words from the text), predict the critical patterns from the text, find related sentences in an essay, and give a more accurate score.
Rupp ( 2018 ), has discussed the designing, evaluating, and deployment methodologies for AES systems. They provided notable characteristics of AES systems for deployment. They are like model performance, evaluation metrics for a model, threshold values, dynamically updated models, and framework.
First, we should check the model performance on different datasets and parameters for operational deployment. Selecting Evaluation metrics for AES models are like QWK, correlation coefficient, or sometimes both. Kelley and Preacher ( 2012 ) have discussed three categories of threshold values: marginal, borderline, and acceptable. The values can be varied based on data size, model performance, type of model (single scoring, multiple scoring models). Once a model is deployed and evaluates millions of responses every time for optimal responses, we need a dynamically updated model based on prompt and data. Finally, framework designing of AES model, hear a framework contains prompts where test-takers can write the responses. One can design two frameworks: a single scoring model for a single methodology and multiple scoring models for multiple concepts. When we deploy multiple scoring models, each prompt could be trained separately, or we can provide generalized models for all prompts with this accuracy may vary, and it is challenging.
Our Systematic literature review on the automated essay grading system first collected 542 papers with selected keywords from various databases. After inclusion and exclusion criteria, we left with 139 articles; on these selected papers, we applied Quality assessment criteria with two reviewers, and finally, we selected 62 writings for final review.
Our observations on automated essay grading systems from 2010 to 2020 are as followed:
- The implementation techniques of automated essay grading systems are classified into four buckets; there are 1. regression models 2. Classification models 3. Neural networks 4. Ontology-based methodology, but using neural networks, the researchers are more accurate than other techniques, and all the methods state of the art provided in Table Table3 3 .
- The majority of the regression and classification models on essay scoring used statistical features to find the final score. It means the systems or models trained on such parameters as word count, sentence count, etc. though the parameters extracted from the essay, the algorithm are not directly training on essays. The algorithms trained on some numbers obtained from the essay and hear if numbers matched the composition will get a good score; otherwise, the rating is less. In these models, the evaluation process is entirely on numbers, irrespective of the essay. So, there is a lot of chance to miss the coherence, relevance of the essay if we train our algorithm on statistical parameters.
- In the neural network approach, the models trained on Bag of Words (BoW) features. The BoW feature is missing the relationship between a word to word and the semantic meaning of the sentence. E.g., Sentence 1: John killed bob. Sentence 2: bob killed John. In these two sentences, the BoW is "John," "killed," "bob."
- In the Word2Vec library, if we are prepared a word vector from an essay in a unidirectional way, the vector will have a dependency with other words and finds the semantic relationship with other words. But if a word has two or more meanings like "Bank loan" and "River Bank," hear bank has two implications, and its adjacent words decide the sentence meaning; in this case, Word2Vec is not finding the real meaning of the word from the sentence.
- The features extracted from essays in the essay scoring system are classified into 3 type's features like statistical features, style-based features, and content-based features, which are explained in RQ2 and Table Table3. 3 . But statistical features, are playing a significant role in some systems and negligible in some systems. In Shehab et al. ( 2016 ); Cummins et al. ( 2016 ). Dong et al. ( 2017 ). Dong and Zhang ( 2016 ). Mathias and Bhattacharyya ( 2018a ; b ) Systems the assessment is entirely on statistical and style-based features they have not retrieved any content-based features. And in other systems that extract content from the essays, the role of statistical features is for only preprocessing essays but not included in the final grading.
- In AES systems, coherence is the main feature to be considered while evaluating essays. The actual meaning of coherence is to stick together. That is the logical connection of sentences (local level coherence) and paragraphs (global level coherence) in a story. Without coherence, all sentences in a paragraph are independent and meaningless. In an Essay, coherence is a significant feature that is explaining everything in a flow and its meaning. It is a powerful feature in AES system to find the semantics of essay. With coherence, one can assess whether all sentences are connected in a flow and all paragraphs are related to justify the prompt. Retrieving the coherence level from an essay is a critical task for all researchers in AES systems.
- In automatic essay grading systems, the assessment of essays concerning content is critical. That will give the actual score for the student. Most of the researches used statistical features like sentence length, word count, number of sentences, etc. But according to collected results, 32% of the systems used content-based features for the essay scoring. Example papers which are on content-based assessment are Taghipour and Ng ( 2016 ); Persing and Ng ( 2013 ); Wang et al. ( 2018a , 2018b ); Zhao et al. ( 2017 ); Kopparapu and De ( 2016 ), Kumar et al. ( 2019 ); Mathias and Bhattacharyya ( 2018a ; b ); Mohler and Mihalcea ( 2009 ) are used content and statistical-based features. The results are shown in Fig. Fig.3. 3 . And mainly the content-based features extracted with word2vec NLP library, but word2vec is capable of capturing the context of a word in a document, semantic and syntactic similarity, relation with other terms, but word2vec is capable of capturing the context word in a uni-direction either left or right. If a word has multiple meanings, there is a chance of missing the context in the essay. After analyzing all the papers, we found that content-based assessment is a qualitative assessment of essays.
- On the other hand, Horbach and Zesch ( 2019 ); Riordan Brain et al. ( 2019 ); Ding et al. ( 2020 ); Kumar et al. ( 2020 ) proved that neural network models are vulnerable when a student response contains constructed irrelevant, adversarial answers. And a student can easily bluff an automated scoring system by submitting different responses like repeating sentences and repeating prompt words in an essay. From Loukina et al. ( 2019 ), and Madnani et al. ( 2017b ). The fairness of an algorithm is an essential factor to be considered in AES systems.
- While talking about speech assessment, the data set contains audios of duration up to one minute. Feature extraction techniques are entirely different from text assessment, and accuracy varies based on speaking fluency, pitching, male to female voice and boy to adult voice. But the training algorithms are the same for text and speech assessment.
- Once an AES system evaluates essays and short answers accurately in all directions, there is a massive demand for automated systems in the educational and related world. Now AES systems are deployed in GRE, TOEFL exams; other than these, we can deploy AES systems in massive open online courses like Coursera(“ https://coursera.org/learn//machine-learning//exam ”), NPTEL ( https://swayam.gov.in/explorer ), etc. still they are assessing student performance with multiple-choice questions. In another perspective, AES systems can be deployed in information retrieval systems like Quora, stack overflow, etc., to check whether the retrieved response is appropriate to the question or not and can give ranking to the retrieved answers.
Conclusion and future work
As per our Systematic literature review, we studied 62 papers. There exist significant challenges for researchers in implementing automated essay grading systems. Several researchers are working rigorously on building a robust AES system despite its difficulty in solving this problem. All evaluating methods are not evaluated based on coherence, relevance, completeness, feedback, and knowledge-based. And 90% of essay grading systems are used Kaggle ASAP (2012) dataset, which has general essays from students and not required any domain knowledge, so there is a need for domain-specific essay datasets to train and test. Feature extraction is with NLTK, WordVec, and GloVec NLP libraries; these libraries have many limitations while converting a sentence into vector form. Apart from feature extraction and training Machine Learning models, no system is accessing the essay's completeness. No system provides feedback to the student response and not retrieving coherence vectors from the essay—another perspective the constructive irrelevant and adversarial student responses still questioning AES systems.
Our proposed research work will go on the content-based assessment of essays with domain knowledge and find a score for the essays with internal and external consistency. And we will create a new dataset concerning one domain. And another area in which we can improve is the feature extraction techniques.
This study includes only four digital databases for study selection may miss some functional studies on the topic. However, we hope that we covered most of the significant studies as we manually collected some papers published in useful journals.
Below is the link to the electronic supplementary material.
Not Applicable.
Publisher's Note
Springer Nature remains neutral with regard to jurisdictional claims in published maps and institutional affiliations.
Contributor Information
Dadi Ramesh, Email: moc.liamg@44hsemaridad .
Suresh Kumar Sanampudi, Email: ni.ca.hutnj@idupmanashserus .
- Adamson, A., Lamb, A., & December, R. M. (2014). Automated Essay Grading.
- Ajay HB, Tillett PI, Page EB (1973) Analysis of essays by computer (AEC-II) (No. 8-0102). Washington, DC: U.S. Department of Health, Education, and Welfare, Office of Education, National Center for Educational Research and Development
- Ajetunmobi SA, Daramola O (2017) Ontology-based information extraction for subject-focussed automatic essay evaluation. In: 2017 International Conference on Computing Networking and Informatics (ICCNI) p 1–6. IEEE
- Alva-Manchego F, et al. (2019) EASSE: Easier Automatic Sentence Simplification Evaluation.” ArXiv abs/1908.04567 (2019): n. pag
- Bailey S, Meurers D (2008) Diagnosing meaning errors in short answers to reading comprehension questions. In: Proceedings of the Third Workshop on Innovative Use of NLP for Building Educational Applications (Columbus), p 107–115
- Basu S, Jacobs C, Vanderwende L. Powergrading: a clustering approach to amplify human effort for short answer grading. Trans Assoc Comput Linguist (TACL) 2013; 1 :391–402. doi: 10.1162/tacl_a_00236. [ CrossRef ] [ Google Scholar ]
- Bejar, I. I., Flor, M., Futagi, Y., & Ramineni, C. (2014). On the vulnerability of automated scoring to construct-irrelevant response strategies (CIRS): An illustration. Assessing Writing, 22, 48-59.
- Bejar I, et al. (2013) Length of Textual Response as a Construct-Irrelevant Response Strategy: The Case of Shell Language. Research Report. ETS RR-13-07.” ETS Research Report Series (2013): n. pag
- Berzak Y, et al. (2018) “Assessing Language Proficiency from Eye Movements in Reading.” ArXiv abs/1804.07329 (2018): n. pag
- Blanchard D, Tetreault J, Higgins D, Cahill A, Chodorow M (2013) TOEFL11: A corpus of non-native English. ETS Research Report Series, 2013(2):i–15, 2013
- Blood, I. (2011). Automated essay scoring: a literature review. Studies in Applied Linguistics and TESOL, 11(2).
- Burrows S, Gurevych I, Stein B. The eras and trends of automatic short answer grading. Int J Artif Intell Educ. 2015; 25 :60–117. doi: 10.1007/s40593-014-0026-8. [ CrossRef ] [ Google Scholar ]
- Cader, A. (2020, July). The Potential for the Use of Deep Neural Networks in e-Learning Student Evaluation with New Data Augmentation Method. In International Conference on Artificial Intelligence in Education (pp. 37–42). Springer, Cham.
- Cai C (2019) Automatic essay scoring with recurrent neural network. In: Proceedings of the 3rd International Conference on High Performance Compilation, Computing and Communications (2019): n. pag.
- Chen M, Li X (2018) "Relevance-Based Automated Essay Scoring via Hierarchical Recurrent Model. In: 2018 International Conference on Asian Language Processing (IALP), Bandung, Indonesia, 2018, p 378–383, doi: 10.1109/IALP.2018.8629256
- Chen Z, Zhou Y (2019) "Research on Automatic Essay Scoring of Composition Based on CNN and OR. In: 2019 2nd International Conference on Artificial Intelligence and Big Data (ICAIBD), Chengdu, China, p 13–18, doi: 10.1109/ICAIBD.2019.8837007
- Contreras JO, Hilles SM, Abubakar ZB (2018) Automated essay scoring with ontology based on text mining and NLTK tools. In: 2018 International Conference on Smart Computing and Electronic Enterprise (ICSCEE), 1-6
- Correnti R, Matsumura LC, Hamilton L, Wang E. Assessing students’ skills at writing analytically in response to texts. Elem Sch J. 2013; 114 (2):142–177. doi: 10.1086/671936. [ CrossRef ] [ Google Scholar ]
- Cummins, R., Zhang, M., & Briscoe, E. (2016, August). Constrained multi-task learning for automated essay scoring. Association for Computational Linguistics.
- Darwish SM, Mohamed SK (2020) Automated essay evaluation based on fusion of fuzzy ontology and latent semantic analysis. In: Hassanien A, Azar A, Gaber T, Bhatnagar RF, Tolba M (eds) The International Conference on Advanced Machine Learning Technologies and Applications
- Dasgupta T, Naskar A, Dey L, Saha R (2018) Augmenting textual qualitative features in deep convolution recurrent neural network for automatic essay scoring. In: Proceedings of the 5th Workshop on Natural Language Processing Techniques for Educational Applications p 93–102
- Ding Y, et al. (2020) "Don’t take “nswvtnvakgxpm” for an answer–The surprising vulnerability of automatic content scoring systems to adversarial input." In: Proceedings of the 28th International Conference on Computational Linguistics
- Dong F, Zhang Y (2016) Automatic features for essay scoring–an empirical study. In: Proceedings of the 2016 Conference on Empirical Methods in Natural Language Processing p 1072–1077
- Dong F, Zhang Y, Yang J (2017) Attention-based recurrent convolutional neural network for automatic essay scoring. In: Proceedings of the 21st Conference on Computational Natural Language Learning (CoNLL 2017) p 153–162
- Dzikovska M, Nielsen R, Brew C, Leacock C, Gi ampiccolo D, Bentivogli L, Clark P, Dagan I, Dang HT (2013a) Semeval-2013 task 7: The joint student response analysis and 8th recognizing textual entailment challenge
- Dzikovska MO, Nielsen R, Brew C, Leacock C, Giampiccolo D, Bentivogli L, Clark P, Dagan I, Trang Dang H (2013b) SemEval-2013 Task 7: The Joint Student Response Analysis and 8th Recognizing Textual Entailment Challenge. *SEM 2013: The First Joint Conference on Lexical and Computational Semantics
- Educational Testing Service (2008) CriterionSM online writing evaluation service. Retrieved from http://www.ets.org/s/criterion/pdf/9286_CriterionBrochure.pdf .
- Evanini, K., & Wang, X. (2013, August). Automated speech scoring for non-native middle school students with multiple task types. In INTERSPEECH (pp. 2435–2439).
- Foltz PW, Laham D, Landauer TK (1999) The Intelligent Essay Assessor: Applications to Educational Technology. Interactive Multimedia Electronic Journal of Computer-Enhanced Learning, 1, 2, http://imej.wfu.edu/articles/1999/2/04/ index.asp
- Granger, S., Dagneaux, E., Meunier, F., & Paquot, M. (Eds.). (2009). International corpus of learner English. Louvain-la-Neuve: Presses universitaires de Louvain.
- Higgins D, Heilman M. Managing what we can measure: quantifying the susceptibility of automated scoring systems to gaming behavior” Educ Meas Issues Pract. 2014; 33 :36–46. doi: 10.1111/emip.12036. [ CrossRef ] [ Google Scholar ]
- Horbach A, Zesch T. The influence of variance in learner answers on automatic content scoring. Front Educ. 2019; 4 :28. doi: 10.3389/feduc.2019.00028. [ CrossRef ] [ Google Scholar ]
- https://www.coursera.org/learn/machine-learning/exam/7pytE/linear-regression-with-multiple-variables/attempt
- Hussein, M. A., Hassan, H., & Nassef, M. (2019). Automated language essay scoring systems: A literature review. PeerJ Computer Science, 5, e208. [ PMC free article ] [ PubMed ]
- Ke Z, Ng V (2019) “Automated essay scoring: a survey of the state of the art.” IJCAI
- Ke, Z., Inamdar, H., Lin, H., & Ng, V. (2019, July). Give me more feedback II: Annotating thesis strength and related attributes in student essays. In Proceedings of the 57th Annual Meeting of the Association for Computational Linguistics (pp. 3994-4004).
- Kelley K, Preacher KJ. On effect size. Psychol Methods. 2012; 17 (2):137–152. doi: 10.1037/a0028086. [ PubMed ] [ CrossRef ] [ Google Scholar ]
- Kitchenham B, Brereton OP, Budgen D, Turner M, Bailey J, Linkman S. Systematic literature reviews in software engineering–a systematic literature review. Inf Softw Technol. 2009; 51 (1):7–15. doi: 10.1016/j.infsof.2008.09.009. [ CrossRef ] [ Google Scholar ]
- Klebanov, B. B., & Madnani, N. (2020, July). Automated evaluation of writing–50 years and counting. In Proceedings of the 58th Annual Meeting of the Association for Computational Linguistics (pp. 7796–7810).
- Knill K, Gales M, Kyriakopoulos K, et al. (4 more authors) (2018) Impact of ASR performance on free speaking language assessment. In: Interspeech 2018.02–06 Sep 2018, Hyderabad, India. International Speech Communication Association (ISCA)
- Kopparapu SK, De A (2016) Automatic ranking of essays using structural and semantic features. In: 2016 International Conference on Advances in Computing, Communications and Informatics (ICACCI), p 519–523
- Kumar, Y., Aggarwal, S., Mahata, D., Shah, R. R., Kumaraguru, P., & Zimmermann, R. (2019, July). Get it scored using autosas—an automated system for scoring short answers. In Proceedings of the AAAI Conference on Artificial Intelligence (Vol. 33, No. 01, pp. 9662–9669).
- Kumar Y, et al. (2020) “Calling out bluff: attacking the robustness of automatic scoring systems with simple adversarial testing.” ArXiv abs/2007.06796
- Li X, Chen M, Nie J, Liu Z, Feng Z, Cai Y (2018) Coherence-Based Automated Essay Scoring Using Self-attention. In: Sun M, Liu T, Wang X, Liu Z, Liu Y (eds) Chinese Computational Linguistics and Natural Language Processing Based on Naturally Annotated Big Data. CCL 2018, NLP-NABD 2018. Lecture Notes in Computer Science, vol 11221. Springer, Cham. 10.1007/978-3-030-01716-3_32
- Liang G, On B, Jeong D, Kim H, Choi G. Automated essay scoring: a siamese bidirectional LSTM neural network architecture. Symmetry. 2018; 10 :682. doi: 10.3390/sym10120682. [ CrossRef ] [ Google Scholar ]
- Liua, H., Yeb, Y., & Wu, M. (2018, April). Ensemble Learning on Scoring Student Essay. In 2018 International Conference on Management and Education, Humanities and Social Sciences (MEHSS 2018). Atlantis Press.
- Liu J, Xu Y, Zhao L (2019) Automated Essay Scoring based on Two-Stage Learning. ArXiv, abs/1901.07744
- Loukina A, et al. (2015) Feature selection for automated speech scoring.” BEA@NAACL-HLT
- Loukina A, et al. (2017) “Speech- and Text-driven Features for Automated Scoring of English-Speaking Tasks.” SCNLP@EMNLP 2017
- Loukina A, et al. (2019) The many dimensions of algorithmic fairness in educational applications. BEA@ACL
- Lun J, Zhu J, Tang Y, Yang M (2020) Multiple data augmentation strategies for improving performance on automatic short answer scoring. In: Proceedings of the AAAI Conference on Artificial Intelligence, 34(09): 13389-13396
- Madnani, N., & Cahill, A. (2018, August). Automated scoring: Beyond natural language processing. In Proceedings of the 27th International Conference on Computational Linguistics (pp. 1099–1109).
- Madnani N, et al. (2017b) “Building better open-source tools to support fairness in automated scoring.” EthNLP@EACL
- Malinin A, et al. (2016) “Off-topic response detection for spontaneous spoken english assessment.” ACL
- Malinin A, et al. (2017) “Incorporating uncertainty into deep learning for spoken language assessment.” ACL
- Mathias S, Bhattacharyya P (2018a) Thank “Goodness”! A Way to Measure Style in Student Essays. In: Proceedings of the 5th Workshop on Natural Language Processing Techniques for Educational Applications p 35–41
- Mathias S, Bhattacharyya P (2018b) ASAP++: Enriching the ASAP automated essay grading dataset with essay attribute scores. In: Proceedings of the Eleventh International Conference on Language Resources and Evaluation (LREC 2018).
- Mikolov T, et al. (2013) “Efficient Estimation of Word Representations in Vector Space.” ICLR
- Mohler M, Mihalcea R (2009) Text-to-text semantic similarity for automatic short answer grading. In: Proceedings of the 12th Conference of the European Chapter of the ACL (EACL 2009) p 567–575
- Mohler M, Bunescu R, Mihalcea R (2011) Learning to grade short answer questions using semantic similarity measures and dependency graph alignments. In: Proceedings of the 49th annual meeting of the association for computational linguistics: Human language technologies p 752–762
- Muangkammuen P, Fukumoto F (2020) Multi-task Learning for Automated Essay Scoring with Sentiment Analysis. In: Proceedings of the 1st Conference of the Asia-Pacific Chapter of the Association for Computational Linguistics and the 10th International Joint Conference on Natural Language Processing: Student Research Workshop p 116–123
- Nguyen, H., & Dery, L. (2016). Neural networks for automated essay grading. CS224d Stanford Reports, 1–11.
- Palma D, Atkinson J. Coherence-based automatic essay assessment. IEEE Intell Syst. 2018; 33 (5):26–36. doi: 10.1109/MIS.2018.2877278. [ CrossRef ] [ Google Scholar ]
- Parekh S, et al (2020) My Teacher Thinks the World Is Flat! Interpreting Automatic Essay Scoring Mechanism.” ArXiv abs/2012.13872 (2020): n. pag
- Pennington, J., Socher, R., & Manning, C. D. (2014, October). Glove: Global vectors for word representation. In Proceedings of the 2014 conference on empirical methods in natural language processing (EMNLP) (pp. 1532–1543).
- Persing I, Ng V (2013) Modeling thesis clarity in student essays. In:Proceedings of the 51st Annual Meeting of the Association for Computational Linguistics (Volume 1: Long Papers) p 260–269
- Powers DE, Burstein JC, Chodorow M, Fowles ME, Kukich K. Stumping E-Rater: challenging the validity of automated essay scoring. ETS Res Rep Ser. 2001; 2001 (1):i–44. [ Google Scholar ]
- Powers DE, Burstein JC, Chodorow M, Fowles ME, Kukich K. Stumping e-rater: challenging the validity of automated essay scoring. Comput Hum Behav. 2002; 18 (2):103–134. doi: 10.1016/S0747-5632(01)00052-8. [ CrossRef ] [ Google Scholar ]
- Ramachandran L, Cheng J, Foltz P (2015) Identifying patterns for short answer scoring using graph-based lexico-semantic text matching. In: Proceedings of the Tenth Workshop on Innovative Use of NLP for Building Educational Applications p 97–106
- Ramanarayanan V, et al. (2017) “Human and Automated Scoring of Fluency, Pronunciation and Intonation During Human-Machine Spoken Dialog Interactions.” INTERSPEECH
- Riordan B, Horbach A, Cahill A, Zesch T, Lee C (2017) Investigating neural architectures for short answer scoring. In: Proceedings of the 12th Workshop on Innovative Use of NLP for Building Educational Applications p 159–168
- Riordan B, Flor M, Pugh R (2019) "How to account for misspellings: Quantifying the benefit of character representations in neural content scoring models."In: Proceedings of the Fourteenth Workshop on Innovative Use of NLP for Building Educational Applications
- Rodriguez P, Jafari A, Ormerod CM (2019) Language models and Automated Essay Scoring. ArXiv, abs/1909.09482
- Rudner, L. M., & Liang, T. (2002). Automated essay scoring using Bayes' theorem. The Journal of Technology, Learning and Assessment, 1(2).
- Rudner, L. M., Garcia, V., & Welch, C. (2006). An evaluation of IntelliMetric™ essay scoring system. The Journal of Technology, Learning and Assessment, 4(4).
- Rupp A. Designing, evaluating, and deploying automated scoring systems with validity in mind: methodological design decisions. Appl Meas Educ. 2018; 31 :191–214. doi: 10.1080/08957347.2018.1464448. [ CrossRef ] [ Google Scholar ]
- Ruseti S, Dascalu M, Johnson AM, McNamara DS, Balyan R, McCarthy KS, Trausan-Matu S (2018) Scoring summaries using recurrent neural networks. In: International Conference on Intelligent Tutoring Systems p 191–201. Springer, Cham
- Sakaguchi K, Heilman M, Madnani N (2015) Effective feature integration for automated short answer scoring. In: Proceedings of the 2015 conference of the North American Chapter of the association for computational linguistics: Human language technologies p 1049–1054
- Salim, Y., Stevanus, V., Barlian, E., Sari, A. C., & Suhartono, D. (2019, December). Automated English Digital Essay Grader Using Machine Learning. In 2019 IEEE International Conference on Engineering, Technology and Education (TALE) (pp. 1–6). IEEE.
- Shehab A, Elhoseny M, Hassanien AE (2016) A hybrid scheme for Automated Essay Grading based on LVQ and NLP techniques. In: 12th International Computer Engineering Conference (ICENCO), Cairo, 2016, p 65-70
- Shermis MD, Mzumara HR, Olson J, Harrington S. On-line grading of student essays: PEG goes on the World Wide Web. Assess Eval High Educ. 2001; 26 (3):247–259. doi: 10.1080/02602930120052404. [ CrossRef ] [ Google Scholar ]
- Stab C, Gurevych I (2014) Identifying argumentative discourse structures in persuasive essays. In: Proceedings of the 2014 Conference on Empirical Methods in Natural Language Processing (EMNLP) p 46–56
- Sultan MA, Salazar C, Sumner T (2016) Fast and easy short answer grading with high accuracy. In: Proceedings of the 2016 Conference of the North American Chapter of the Association for Computational Linguistics: Human Language Technologies p 1070–1075
- Süzen, N., Gorban, A. N., Levesley, J., & Mirkes, E. M. (2020). Automatic short answer grading and feedback using text mining methods. Procedia Computer Science, 169, 726–743.
- Taghipour K, Ng HT (2016) A neural approach to automated essay scoring. In: Proceedings of the 2016 conference on empirical methods in natural language processing p 1882–1891
- Tashu TM (2020) "Off-Topic Essay Detection Using C-BGRU Siamese. In: 2020 IEEE 14th International Conference on Semantic Computing (ICSC), San Diego, CA, USA, p 221–225, doi: 10.1109/ICSC.2020.00046
- Tashu TM, Horváth T (2019) A layered approach to automatic essay evaluation using word-embedding. In: McLaren B, Reilly R, Zvacek S, Uhomoibhi J (eds) Computer Supported Education. CSEDU 2018. Communications in Computer and Information Science, vol 1022. Springer, Cham
- Tashu TM, Horváth T (2020) Semantic-Based Feedback Recommendation for Automatic Essay Evaluation. In: Bi Y, Bhatia R, Kapoor S (eds) Intelligent Systems and Applications. IntelliSys 2019. Advances in Intelligent Systems and Computing, vol 1038. Springer, Cham
- Uto M, Okano M (2020) Robust Neural Automated Essay Scoring Using Item Response Theory. In: Bittencourt I, Cukurova M, Muldner K, Luckin R, Millán E (eds) Artificial Intelligence in Education. AIED 2020. Lecture Notes in Computer Science, vol 12163. Springer, Cham
- Wang Z, Liu J, Dong R (2018a) Intelligent Auto-grading System. In: 2018 5th IEEE International Conference on Cloud Computing and Intelligence Systems (CCIS) p 430–435. IEEE.
- Wang Y, et al. (2018b) “Automatic Essay Scoring Incorporating Rating Schema via Reinforcement Learning.” EMNLP
- Zhu W, Sun Y (2020) Automated essay scoring system using multi-model Machine Learning, david c. wyld et al. (eds): mlnlp, bdiot, itccma, csity, dtmn, aifz, sigpro
- Wresch W. The Imminence of Grading Essays by Computer-25 Years Later. Comput Compos. 1993; 10 :45–58. doi: 10.1016/S8755-4615(05)80058-1. [ CrossRef ] [ Google Scholar ]
- Wu, X., Knill, K., Gales, M., & Malinin, A. (2020). Ensemble approaches for uncertainty in spoken language assessment.
- Xia L, Liu J, Zhang Z (2019) Automatic Essay Scoring Model Based on Two-Layer Bi-directional Long-Short Term Memory Network. In: Proceedings of the 2019 3rd International Conference on Computer Science and Artificial Intelligence p 133–137
- Yannakoudakis H, Briscoe T, Medlock B (2011) A new dataset and method for automatically grading ESOL texts. In: Proceedings of the 49th annual meeting of the association for computational linguistics: human language technologies p 180–189
- Zhao S, Zhang Y, Xiong X, Botelho A, Heffernan N (2017) A memory-augmented neural model for automated grading. In: Proceedings of the Fourth (2017) ACM Conference on Learning@ Scale p 189–192
- Zupanc K, Bosnic Z (2014) Automated essay evaluation augmented with semantic coherence measures. In: 2014 IEEE International Conference on Data Mining p 1133–1138. IEEE.
- Zupanc K, Savić M, Bosnić Z, Ivanović M (2017) Evaluating coherence of essays using sentence-similarity networks. In: Proceedings of the 18th International Conference on Computer Systems and Technologies p 65–72
- Dzikovska, M. O., Nielsen, R., & Brew, C. (2012, June). Towards effective tutorial feedback for explanation questions: A dataset and baselines. In Proceedings of the 2012 Conference of the North American Chapter of the Association for Computational Linguistics: Human Language Technologies (pp. 200-210).
- Kumar, N., & Dey, L. (2013, November). Automatic Quality Assessment of documents with application to essay grading. In 2013 12th Mexican International Conference on Artificial Intelligence (pp. 216–222). IEEE.
- Wu, S. H., & Shih, W. F. (2018, July). A short answer grading system in chinese by support vector approach. In Proceedings of the 5th Workshop on Natural Language Processing Techniques for Educational Applications (pp. 125-129).
- Agung Putri Ratna, A., Lalita Luhurkinanti, D., Ibrahim I., Husna D., Dewi Purnamasari P. (2018). Automatic Essay Grading System for Japanese Language Examination Using Winnowing Algorithm, 2018 International Seminar on Application for Technology of Information and Communication, 2018, pp. 565–569. 10.1109/ISEMANTIC.2018.8549789.
- Sharma A., & Jayagopi D. B. (2018). Automated Grading of Handwritten Essays 2018 16th International Conference on Frontiers in Handwriting Recognition (ICFHR), 2018, pp 279–284. 10.1109/ICFHR-2018.2018.00056
Automated Essay Scoring: Writing Assessment and Instruction AES PEG ELL TOEFL iBT TPO
- http://www.sciencedirect.com/science/article/pii/B9780080448947002335
(Stanford users can avoid this Captcha by logging in.)
- Send to text email RefWorks EndNote printer
Automated essay scoring : a cross-disciplinary perspective
Available online, at the library.

SAL3 (off-campus storage)
More options.
- Find it at other libraries via WorldCat
- Contributors
Description
Creators/contributors, contents/summary.
- Part 1 Teaching of Writing: What Can Computers Contribute to a K-12 Writing Programme? M. Myers. Part 2 Psychometric Issues in Performance Assessment: Issues in the Reliability and Validity of Automated Scoring of Constructed Responses, G.K.W.K. Chung and E.L. Baker. Part 3 Automated Test Scorers: Project Essay Grade - PEG, E.B. Page
- A Text Categorization Approach to Automated Essay Grading, L.S. Larkey and W.B. Croft
- Intellimetric T - from here to validity, S. Elliot
- Automated Scoring and Annotation of Essays With the Intelligent Essay Assessor T, T.K. Landauer, D. Laham and P.W. Foltz
- the E-Rater T Scoring Engine - Automated Essay Scoring with Natural Language Processing, J. Burstein. Part 4 Psychometric Issues in Automated Essay Scoring: The Concept of Reliability in the Context of Automated Essay Scoring, G.J. Cizek and B.A. Page
- Validity of Automated Essay Scoring Systems, T.Z. Keith
- Norming and Scaling for Automated Essay Scoring, M.D. Shermis and K.E. Daniels
- Bayesian Analysis of Essay Grading, S. Ponisciak and V. Johnson
- Automated Grammatical Error Detection, C. Leacock and M. Chodorow
- Developing Technology for Automated Evaluation of Discourse Structure in Student Essays, J. Burnstein and D. Marcu.
- (source: Nielsen Book Data)
Bibliographic information
Browse related items.

- Stanford Home
- Maps & Directions
- Search Stanford
- Emergency Info
- Terms of Use
- Non-Discrimination
- Accessibility
© Stanford University , Stanford , California 94305 .
Psychometric & Assessment Services
We offer a full range of psychometric services in addition to comprehensive test development services.
Psychometric Services
- field test planning
- operational test design
- classical item analysis
- IRT analysis
- differential item functioning analysis
- alignment studies
- reliability and validity analyses
- technical report production
- standard setting
Our psychometric team also designs, analyzes, and presents findings of wide-ranging psychometric research. See the research page for details.
Assessment Services
Item and test development services.
We offer comprehensive test development services for standards-based assessments. In addition to developing items and test forms that illuminate instruction, our capabilities include developing item and test specifications, performance and oral assessments, observation checklists, administration manuals, scoring criteria, and interpretation guides. Our test development staff spans content specialists, project directors, editors, graphic artists, and support staff, who are advised by our psychometric team.
Project and Program Management
MI believes in building and maintaining relationships with customers and employees alike through effective, honest, and ongoing communication. To this end, we employ a distributed leadership model—rather than a regimented, hierarchical structure—in which our project and program managers can lead proactively, make decisions independently, and guarantee we exceed client expectations. MI’s team of project and program managers has diverse knowledge around multiple aspects of assessment, including holding previous roles in scoring, test development, and psychometrics. In addition, some individuals also have prior classroom experience and hold graduate degrees or are PMP-certified. This expansive range of backgrounds has provided ample opportunities for several team members to work effectively on numerous state-wide programs. To promote continual growth and expand on their experience, MI’s project and program managers are supported by our program management office, which provides protocols, tools, feedback, and professional development. This approach ensures our project and program managers have both the skills and the resources necessary to consistently meet client needs. Finally, we recognize that all projects are dynamic and that they can require give and take when leading many aspects of successful program management.
Document Production, Distribution, Receipt, and Storage
MI is capable of producing millions of test booklets annually. In addition to our in-house digital printing equipment, we maintain relationships with several local printers.
We have developed a proprietary Order-Pack-Ship (OPS) application used to scan and track test materials. In addition to allowing us to efficiently manage the selection and shipping of orders, our OPS system is built around extensive quality control procedures to ensure that the correct numbers and types of materials are shipped to schools and districts.
We have similarly developed a sophisticated internal tracking system used for logging and processing all materials returned from the field. This system is capable of locating individual test documents at any time they are in our possession.
We maintain a host of high-volume scanners and imaging equipment and use several time-tested processes that ensure all necessary precautions are taken during scanner and document setup, scanner calibration, data validation, and data export. We utilize a double-blind data correction process to achieve the most accurate reporting of student information and test results.
In addition to secure electronic delivery of assessment results, we are capable of producing reports in-house—using a variety of high-speed printers—and shipping to schools or districts.
Scoring Services
Handscoring.
MI handscores tens of millions of student responses annually. Our unified handscoring system allows us to conduct all hiring, training, qualifying, scoring, monitoring, communicating, and reporting tasks remotely. At the heart of this system is our state-of-the-art Virtual Scoring Center (VSC), comprised of VSC Capture (a system for acquiring images and decoding response data from paper tests), VSC Train (a secure training and practice application for raters and scoring leadership), and VSC Score (a secure user management, scoring, and reporting application).
At the company’s inception, MI developed an outstanding training method for the scoring of student constructed responses which has become the industry model. On this foundation, we use our scoring technologies to monitor rater performance effectively and efficiently. In addition to traditional measures of rater accuracy and agreement, we employ a host of automated quality-assurance score verifications to ensure the most appropriate score has been assigned to each response.
MI’s handscoring service offerings include conducting rangefinding proceedings, developing scoring tools and training materials, evaluating prompts and constructed-response items, recruiting and hiring scoring personnel, performing training activities, and supervising scoring efforts.
Automated Scoring
MI has led the field in automated scoring solutions since first adopted by schools, districts, and states in formative and summative contexts. MI’s Project Essay Grade (PEG) automated scoring engine currently provides nearly 10 million summative scores for students across the US.
PEG and the MI team have dominated public competitions testing the state of the art of automated scoring. These contests have spanned essay scoring (the Hewlett Foundation-sponsored Automated Student Assessment Prize [ASAP] phase one), short constructed response English language arts and science scoring (ASAP phase two) and reading constructed response scoring (the National Center for Education Statistics [NCES]-sponsored National Assessment of Educational Progress [NAEP] Automated Scoring Challenge).
In most operational assessment program contexts we recommend a hybrid scoring approach, in which an automated scoring engine is used alongside human raters. This approach is designed to leverage the respective strengths of automated and hand-scoring while mitigating their respective limitations.
Evaluation & School Improvement Services
Program evaluation.
Our team of accomplished researchers assists organizations in all aspects of the research/evaluation process, including leveraging data for program improvement and sustainability. Whether it is evaluating new programs, conducting statewide survey research, or disseminating resources and information on diverse topics, we offer objective, accurate, and sound information that clients can trust.
Technical Assistance and Professional Development Services
- Special Education Systems and Practices
- Multi-tiered Systems of Support (RtI and PBIS)
- Early Literacy
- Leadership Development
- Bullying Prevention
- School Safety and Healthy School Climate
- Career & Technical Education
- Student Support Services
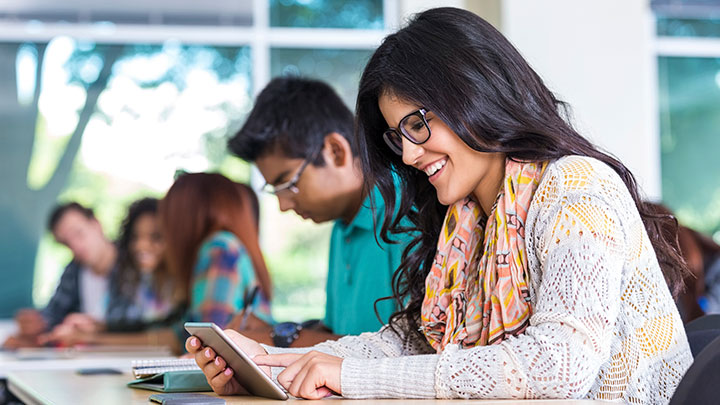
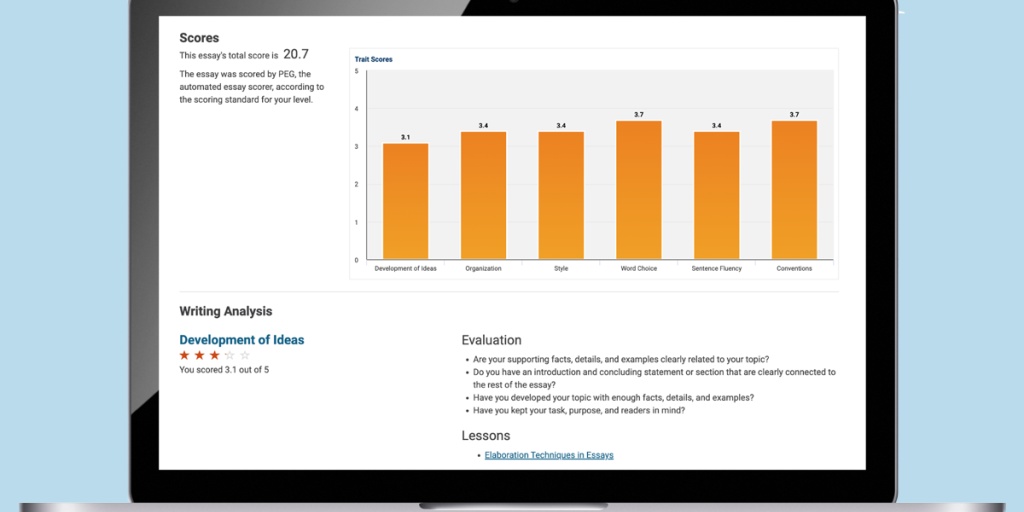
ERB Launches Writing Practice

ERB announced today the availability of ERB Writing Practice , an online program designed to help students improve their writing skills through practice, feedback, and guided support backed by the industry’s most accurate automated scoring algorithm . ERB Writing Practice helps educators and families save valuable time while giving students immediate and individualized feedback and opportunities for growth.
Why ERB Writing Practice?
Our mission is to help students reach their full potential. To achieve this, we are continually improving our programs for educators and families, so they have the right tools to activate and track student growth. In this spirit, we asked users of ERB’s previous writing program, WPP, to tell us about their experience with the former program, their needs, and how we can best support them.
The new Writing Practice includes all of the existing features of WPP, but with a refreshed design, improved user experience, and exciting new features and enhancements.
We also looked at the important aspects of effective learning: practice and feedback. We designed Writing Practice around this approach, incorporating instant feedback and extensive opportunities for students to practice in a low-stakes environment. To round it out, teachers have access to comprehensive reporting to track growth and learning year-round.
The redesigned program includes over 500 prompts as well as the flexibility for teachers to write their own prompts. Students can practice these prompts at any time to hone their writing skills. Additionally, educators can select from over 100 lessons on-demand, saving them valuable time teaching the core skills that develop great writers.
The Practice – Feedback – Practice Approach
In addition to the robust content library, there are multiple ways to review a student’s work and provide instant, actionable feedback, further enriching the student learning experience. These include teacher feedback, peer reviews, and the Project Essay Grade® (PEG®) scoring algorithm , an automated essay scoring solution based on more than 40 years of research by Dr. Ellis Batten Page. The PEG scoring algorithm is the “gold standard.” Its scoring results have been validated in more independent studies than all other essay scoring solutions combined. This automated, AI-based tool provides students with real-time feedback, allowing them to review and practice again. Students who used PEG feedback and traditional writing instruction showed a 22% improvement in writing over those who did not.
“The most gratifying aspect of offering members ERB Writing Practice is that we have the research to demonstrate it works” says ERB’s Chief Program Officer, Glenn Milewski; “at $18 per student, it’s a small investment for a big, year-round return.”
Saving Valuable Time
Educators using the Writing Practice program can look forward to more control and a seamless user interface. This includes the new Self-Rostering feature , which enables schools to roster their students rather than wait days for that to be handled for them. This saves valuable time, allowing teachers more time to focus on their students.
“Writing Practice is the perfect tool to improve a student’s writing skills. I have seen the tool increase writing abilities from a sixth-grade to a ninth-grade level in under a year. It is versatile, like a Swiss army knife. The program can be utilized across subjects and grade levels. The best part is seeing a student’s growth over time.” — Bob Doss, Bob Doss Coaching
Availability and Pricing Information
Member schools.
ERB Writing Practice is a considerable upgrade from the WPP program, and we are excited to offer it to schools today. For ERB Member schools, Writing Practice is $18 per student for the 2022-23 school year. Schools can request a free demo for more information and purchase through the Shopping Cart at ordering.erblearn.org .
Families can purchase through the Family Store.
About ERB ERB is a not-for-profit educational advisory group that provides educators and families with a complete understanding of the whole student. K-12 schools worldwide rely on ERB to provide them with an integrated suite of assessments, insights, and analytics that track the complete student journey—assessing academic ability, learning achievement, and social and emotional learning competencies. To learn more, visit www.erblearn.org.

ERB and Kahoot! Forge Partnership to Enhance Student Learning Experiences
ERB, a not-for-profit trusted leader in K-12 assessment and data-driven insights for independent school educators and families, and Kahoot!, a global leader in interactive learning and engagement, announced a partnership to elevate the teaching and learning experience for educators and students. […] read more
Stay in touch

Want to create or adapt books like this? Learn more about how Pressbooks supports open publishing practices.
9 10 Academic Goals Examples to Supercharge Your Student Success
Becoming a successful student involves more than just going to classes and remembering facts for the test. It is about establishing a mentality of learning and evolving continuously, and distinct academic goals are a key aspect of that. These are the lights that lead the way and help you stay on track as you navigate through the trials and tribulations of your studies, whether it’s the demands of testing or the stuff that life throws at you. But, just as importantly, by clearly defining and outlining your academic objectives, you give your study a sense of intention and purpose. Whether it’s achieving a particular GPA, mastering a challenging topic, or participating in enriching extracurricular, strong academic Goals are the foundation of both short- and long-term academic success.
Establishing concrete goals and the quest of excellence are frequently linked in the academic sphere. Before delving into these Academic Goals Examples, it is important to recognize services like Scholarly Help that provide workable ways to handle several Tasks. With options like pay someone to do my online class , Scholarly Help ensures you stay on track without compromising other responsibilities. This comprehensive guide explores ten powerful academic goals examples designed to elevate your student success to unprecedented heights. Whether your academic goals are designed to improve your critical thinking habits, advance your time management skills, or explore interdisciplinary thinking to further your academic career, they should provide students with a path toward overall intellectual and personal development. Rounding up different students through carefully structured college academic goals. All must necessarily form the basis of individual reality and opportunities.
Mastering Time Management
One of the building blocks for academic achievement is the effective management of time. The ability allows students to combine studies with other activities, namely work, daily life, or personal life. To manage time effectively, learners are recommended to:
- Plan a Weekly Schedule: set certain hours and days to work, study, and engage in other activities;
- Set Priorities: determine poses that are urgent and important, then focus on a solution;
- Do not Get Distracted: if some activities or processes are distracting, generate disadvantages.
Enhancing Study Skills
Improving study abilities might result in better comprehension and recall of course material. Students should focus on:
- Active Learning Techniques: Engage with the material such as holding discussions, teaching others, and transforming what one learned to real-life application.
- Effective Note-Taking: Employ methods like Cornell Note-taking System to organize and refresh notes.
- Regular Review Sessions: Set regular study dates to refresh one’s memory and prepare for exams.
Setting Specific Academic Targets
Setting clear, specific targets helps students stay motivated and measure progress. Examples of specific academic goals include:
- Achieve Specific GPA: Aim to reach or maintain a specific grade point average each semester.
- Improving Grades in Challenging Subjects: Identify subjects where improvement is needed and set goals accordingly.
- Completing Assignments Ahead of Deadlines: Plan to finish assignments before the due date to allow time for revisions.
Expanding Knowledge beyond the Classroom
Gaining knowledge outside the classroom can enhance academic performance and provide a broader perspective. Students can achieve this by:
- Reading Extensively: Explore Books, Journals, and articles related to their field of Study.
- Attending Seminars and Workshops: Participate in events offering additional insights and networking opportunities.
- Engaging in Research Projects: Collaborate with professors or peers on research projects to deepen understanding of specific topics.
Developing Critical Thinking Skills
Critical thinking is vital for problem-solving and making informed decisions. Students can cultivate these skills by:
- Questioning Assumptions: Always ask why and consider alternative viewpoints.
- Analyzing Arguments: Evaluate the evidence and logic in different arguments.
- Reflecting on Learning: Regularly review what has been learned and how it applies to real-world situations.
Building Effective Communication Skills
Strong communication skills are essential for academic and professional success. Students can enhance these skills by:
- Participating in Class Discussions: Engage actively in discussions to practice articulating thoughts clearly.
- Writing Regularly: Practice writing essays, reports, and articles to improve writing abilities.
- Presenting Projects: Take opportunities to present work in front of an audience to build confidence and clarity.
Fostering Collaboration and Teamwork
Collaboration with peers can lead to better understanding and innovative solutions. Students should focus on:
- Joining Study Groups: Collaborate with classmates to discuss topics and solve problems together.
- Participating in Group Projects: Develop teamwork and leadership skills by working on group assignments.
- Engaging in Extracurricular Activities: Join clubs and organizations that encourage teamwork and collective problem-solving.
Seeking Feedback and Continuous Improvement
Constructive feedback helps identify areas for improvement and guide academic growth. Students should:
- Ask for Feedback: Request feedback from professors and peers on assignments and presentations.
- Reflect on Criticism: Use feedback to identify strengths and weaknesses, developing action plans for improvement.
- Commit to Lifelong Learning: Embrace continuous learning and improvement in all aspects of life.
Utilizing Academic Resources
Taking full advantage of available academic resources can enhance learning and performance. Students should:
- Visit the Library Regularly: Utilize resources for research and study.
- Use Online Databases: Access academic journals and articles online to support studies.
- Seek Academic Support Services: Utilize tutoring, writing centers, and academic advising offered by the institution.
Preparing for Future Careers with Academic Goals
Setting academic goals with future careers in mind provides direction and motivation. Students should:
- Identify Career Goals: Determine career aspirations and align academic goals accordingly.
- Gain Relevant Experience: Pursue internships, part-time jobs, and volunteer opportunities related to the field of study.
- Develop Professional Skills: Focus on skills like resume writing, interviewing, and networking to prepare for the job market.
Setting and meeting academic goals necessitates dedication, strategic planning, and consistent effort. By focusing on these ten academic goal examples, students can improve their learning experience, and performance, and set themselves up for future success. Remember that the key to academic success is to set specific, attainable goals and work hard to meet them.
Education Copyright © by john44. All Rights Reserved.
Share This Book

IMAGES
VIDEO
COMMENTS
PEG, or Project Essay Grade, is the automated scoring system at the core of ERB Writing Practice. It was invented in the 1960s by Ellis Batten Page, a former high school English teacher, who spent "many long weekends sifting through stacks of papers wishing for some help."
The effects of automated essay scoring as a high school classroom intervention. This quasi-experimental, mixed methods study investigated whether students writing development and proficiency, in combination with teacher-led instruction, are significantly affected by the use of an automated essay scoring (AES) system.
automated essay scoring a practical possibility. In 2003, MI acquired Project Essay Grade™ (PEG™)1 from Dr. Page and his associates. Eleven years later, MI has re-engineered, enhanced and extended the PEG system using the latest techniques and technologies in the field of
This chapter describes the evolution of Project Essay Grade (PEG), which was the first of the automated essay scorers. The purpose is to detail some of the history of automated essay grading, why it was impractical when first created, what reenergized development and research in automated essay scoring, how PEG works, and to report recent research involving PEG.
The Project Essay Grade® (PEG®) software is an automated essay scoring solution that builds upon Dr. Ellis Batten Page's research in computational linguistics spanning over 40 years. PEG analyzes written prose, measures writing characteristics like fluency and grammar, and models the decision-making process of expert readers, ultimately ...
Automated essay scoring (AES) is the use of specialized computer programs to assign grades to essays written in an educational setting.It is a form of educational assessment and an application of natural language processing.Its objective is to classify a large set of textual entities into a small number of discrete categories, corresponding to the possible grades, for example, the numbers 1 to 6.
This chapter describes the evolution of Project Essay Grade (PEG), which was the first of the automated essay scorers. The purpose is to detail some of the history of automated essay grading, why it was impractical when first created, what reenergized development and research in automated essay scoring, how PEG works, and to report recent research involving PEG.
Project Essay Grader™ (PEG) Ellis Page developed the PEG in 1966. PEG is considered the earliest AES system that has been built in this field. ... Project essay grade: PEG; pp. 43-54. [Google Scholar] Peng, Ke & Xu (2012) Peng X, Ke D, Xu B. Automated essay scoring based on finite state transducer: towards ASR transcription of oral English ...
Automated essay scoring involves automatic assessment of a students' written work, usually in response to a writing prompt. This assessment generally includes (1) a holistic score of students' performance, knowledge, and/or skill and (2) a score descriptor on how the student can improve the text. For example, e-rater by ETS ( 2013) scores ...
Project Essay Grade (PEG) PEG is one of the earliest and longest-lived implementations of automated essay grading. It was devel-oped by Page and others (Hearst, 2000; Page, 1994, 1996) and primarily relies on style analysis of sur-face linguistic features of a block of text.
PEG Writing is a web-based formative assessment program developed by Measurement Incorporated (MI). PEG is an acronym for Project Essay Grade, which was developed by Ellis Page and colleagues (Page, 1966, Page, 2003). Since its acquisition by MI in 2003, PEG has undergone continuous redesign and enhancement for use in both formative and ...
The main concept behind PEG Writing is to automate this process. PEG uses the automated essay scoring engine from Measurement Incorporated. Measuremnt Inc. (MI) acquired PEG from Dr. Ellis Batten Page and his associates in 2002. PEG stands for Project Essay Grade. There have been a few research studies comparing automated essay scoring (AES ...
This course features Score, the free OER Project essay-scoring service. When combined with writing prompts and scaffolded pre- and post-writing activities, this powerful tool provides consistent formative feedback and loads of data to inform instruction and discussion. Prompts: A variety of writing prompts address enduring historical questions ...
This new volume is the first to focus entirely on automated essay scoring and evaluation. It is intended to provide a comprehensive overview of the evolution and state-of-the-art of automated essay scoring and evaluation technology across several disciplines, including education, testing and measurement, cognitive science, computer science, and computational linguistics.
Automated essay scoring (AES) is a computer-based assessment system that automatically scores or grades the student responses by considering appropriate features. The AES research started in 1966 with the Project Essay Grader (PEG) by Ajay et al. . PEG evaluates the writing characteristics such as grammar, diction, construction, etc., to grade ...
One side of the argument contends that allowing computers to assume a greater role in grading student written work provides many benefits to both the student and the instructor. Students benefit from increased response time for both grades and feedback. In many cases, an essay can be 'graded' and feedback provided within minutes [26].
An Overview of Automated Essay Scoring As a relatively young field, AES has only a 40-year history. Ellis Page is generally regarded as the pioneer of AES (Bereiter, 2003; Kukich, 2000; Wresch, 1993). In 1966, Page designed a computer grading program named Project Essay Grader (PEG). Utilizing the statistical capabilities of
7. Subject/Key Words: Automated Essay Scoring (AES), Writing Assessment, Writing Instruction, Project Essay Grade (PEG), Criterion Online Writing Evaluation, Reliability, Validity, English Language Learning (ELL), Text Adaptor, Test of English as a Foreign Language (TOEFL), Internet Based Testing (iBT), TOEFL Practice Online (TPO), SpeechRater ...
Page and colleagues ' Project Essay Grade (PEG; see Page, 1966). While technological develo pments have increased the complexity and accuracy of AES, core concepts in Page's wor k remain relevant.
Part 3 Automated Test Scorers: Project Essay Grade - PEG, E.B. Page; A Text Categorization Approach to Automated Essay Grading, L.S. Larkey and W.B. Croft; Intellimetric T - from here to validity, S. Elliot; Automated Scoring and Annotation of Essays With the Intelligent Essay Assessor T, T.K. Landauer, D. Laham and P.W. Foltz
The present study extended research on the effectiveness of automated writing evaluation (AWE) systems. Sixth graders were randomly assigned by classroom to an AWE condition that used Project Essay Grade Writing (n = 56) or a word-processing condition that used Google Docs (n = 58).Effectiveness was evaluated using multiple metrics: writing self-efficacy, holistic writing quality, performance ...
MI's Project Essay Grade (PEG) automated scoring engine currently provides nearly 10 million summative scores for students across the US. PEG and the MI team have dominated public competitions testing the state of the art of automated scoring. These contests have spanned essay scoring (the Hewlett Foundation-sponsored Automated Student ...
These include teacher feedback, peer reviews, and the Project Essay Grade® (PEG®) scoring algorithm, an automated essay scoring solution based on more than 40 years of research by Dr. Ellis Batten Page. The PEG scoring algorithm is the "gold standard." Its scoring results have been validated in more independent studies than all other ...
Examples of specific academic goals include: Achieve Specific GPA: Aim to reach or maintain a specific grade point average each semester. Improving Grades in Challenging Subjects: Identify subjects where improvement is needed and set goals accordingly. Completing Assignments Ahead of Deadlines: Plan to finish assignments before the due date to ...
A new tool called Writable, which uses ChatGPT to help grade student writing assignments, is being offered widely to teachers in grades 3-12.. Why it matters: Teachers have quietly used ChatGPT to grade papers since it first came out — but now schools are sanctioning and encouraging its use. Driving the news: Writable, which is billed as a time-saving tool for teachers, was purchased last ...