

Research Skills Framework
Patterns of practice and a set of tools for researchers and their teams to grow—, 1. learn skills & practices.

2. Build Projects & Playbooks

3. Map Progress & Goals

4. New Frontiers & Exploration

Driven by volunteers in the ReOps community, and organizers and participants around the world
.png)

University Library
Robert S. Swanson Library and Learning Center

Teaching Research at UW-Stout: RESEARCH SKILLS DEVELOPMENT (RSD) FRAMEWORK
- RESEARCH SKILLS DEVELOPMENT (RSD) FRAMEWORK
- INFORMATION LITERACY This link opens in a new window
Nakatani Teaching and Learning Center

The Nakatani Teaching and Learning Center (NTLC) supports teaching, learning and SoTL research at UW-STout. NTLC facilitates conversations, coordinates events, training and offers stipends for instructors.
The Community of Practice (CoP) program is a voluntary, year-long program focusing on a chosen topic or issue about enhancing teaching and learning. Guidance for studying a topic is provided with participants meeting on a regular basis conducting research, analyzing results, and disseminating their findings. A financial incentive is provided to instructors who complete the program.
Teaching Research at UW-Stout
Introduction to the research skills development (rsd) framework.
The Research Skills Development (RSD) Framework, initiated by John Willison and Kerry O'Regan (Adelaide, AU), is a tool used to analyze and plan curriculum for the systematic development of students' research skills. The framework is descriptive, scalable, and useful for assignment, course or program planning purposes.

- Articles Related to RSD Includes the originating article (Willison & O'Regan, 2007), RSD applications, other undergraduate research frameworks, and papers critiquing RSD.
- Examples of RSD Applications Examples of the RSD applied organized by discipline, task type and other.
John Willison and Dorothy Missingham (University of Adelaide, AU) spoke at the Student Research Skills Symposium (UW-Stout, Dec 2015).
Research Skills Classroom Activities
2014-2015 ntlc cop cohort.
- Final Reports
- Classroom Resources
- Application to Create RSD CoP, Group
- RSD at UW-Stout CoP Handbook 2014 -'15 Handbook outlining the impetus, expectations and schedule of meetings and tasks for the cohort.
- Invitation to Join CoP
- Application to Join RSD CoP, Individual
- Invitation to Read CoP Blog, Email
- Stages of Concern for RSD CoP The Stages of Concern Survey was used to assess the Community of Practice.
- "Key Lessons from Our Experiences Co-Facilitating the RSD CoP" Submitted by facilitators Sylvia Tiala, Kitrina Carlson, and Anne Kerber.
Willison Workshop | July 2, 2014 | UW-Stout
- Workshop Agenda
OPID Conference Presentation | April 16-17, 2015 | Green Lake, WI
- OPID 2015 Conference Information
- OPID 2015 Conference Presentation Proposal
RSD Spring Workshop | May 26, 2015 | UW-Stout
- Workshop Agenda (Planning Document)
- Edenborg Final Report, Part 1
- Kerber Final Report
- Navarre Final Report
- Polzer Final Report
- Tiala Final Report 2015
- Wilson Final Report
- Zagorski Final Report 2015
2015-2016 NTLC CoP Cohort

- RSD at UW-Stout CoP Handbook 2015 -'16
- UW-System Undergraduate and Discovery Grant Application The 2015 -'16 Undergraduate Research CoP was funded by the UW-System Undergraduate and Discovery Grant.
- UW-System Undergraduate Research and Discovery Grant Award Letter
- Application to Join RSD CoP-Advanced, Individual
RSD Workshop and CoP Kickoff | August 25, 2015 | UW-Stout
- Workshop & Community of Practice Kickoff Announcement
Student Research Skills Symposium | December 3-4, 2015 | UW-Stout
- Symposium Announcement
- Symposium Summary and Documents
- Aschenbrener Final Report
- Aschenbremer Report Addendum: Survey Tool
- Edenborg Final Report, Part 2
- Kotenko Final Report
- Kotenko Report Addendum: Survey Tool
- Olson Final Report
- Olson Report Addendum: Assignment Prompt
- Tiala Final Report 2016
- Zagorski Final Report 2016
- << Previous: Teaching Research
- Next: INFORMATION LITERACY >>
- Last Updated: Aug 10, 2023 3:07 PM
- URL: https://library.uwstout.edu/teachingresearch
Search form
Research and innovation menu, research and innovation, research skills development.
Willison & O’Regan (2007) believe that the development of research skills occurs on a continuum of knowledge production, from that which is new to the learner to fringe research that is new to everyone, or to move from “the commonly known, to the commonly not known, to the totally unknown" (p. 394). From this perspective, they developed a Research Skills Development Framework that outlines six facets of research and describes how each skill/activity manifests along a continuum based on the level of student autonomy.
Key Terms*:
- Prescribed Research – highly structured directions and modeling from faculty
- Bounded Research – boundaries set by and limited directions from faculty
- Scaffolded Research – scaffolds placed by faculty shape the students’ research
- Student-initiated Research – student-initiated research under the guidance of a faculty member
- Open Research – independent student research that is guided by disciplinary standards
* Please see the RSD Framework for a full description.
The Research Skills Development Framework is useful as both a conceptual and planning tool as well as an assessment mechanism. It can be used to develop course and program activities that are appropriate for the level of research being conducted, it can help clarify learning outcomes, develop assessment measurements, and track student progress and development.
Equipped with an understanding of student development and research skills development, one can begin to conceptualize and plan undergraduate research activities that best suit the characteristics of the student population you are working with and/or the students that you are targeting to conduct research with – whether it be in a course or extra-curricular activity.
Next – Strategies
- Willison, J.W. & O’Regan, K. (2007). Commonly known, commonly not known, totally unknown: A framework for students becoming researchers. Higher Education Research and Development , 26, 393-409.
Mentoring Undergraduate Research Directory
Developing Scientific Thinking and Research Skills Through the Research Thesis or Dissertation
- First Online: 22 September 2019
Cite this chapter
- Gina Wisker 3 , 4
1279 Accesses
2 Citations
This chapter explores higher level scientific thinking skills that research students need to develop during their research learning journeys towards their dissertation/thesis at postgraduate levels, and also final year undergraduate (Australian honours year) dissertation. A model of four quadrants is introduced. Practice and experience-informed examples are presented to show how higher order skills can be realised and embedded so that they become established ways of thinking, researching, creating, and expressing knowledge and understanding.
This is a preview of subscription content, log in via an institution to check access.
Access this chapter
- Available as PDF
- Read on any device
- Instant download
- Own it forever
- Available as EPUB and PDF
- Compact, lightweight edition
- Dispatched in 3 to 5 business days
- Free shipping worldwide - see info
- Durable hardcover edition
Tax calculation will be finalised at checkout
Purchases are for personal use only
Institutional subscriptions
Aitchison, C., & Guerin, C. (Eds.). (2014). Writing groups for doctoral education and beyond: Innovations in practice and theory . Abingdon: Routledge.
Google Scholar
Archer, L. (2008). Younger academics’ constructions of ‘authenticity’, ‘success’ and professional identity. Studies in Higher Education, 33 (4), 385–403. https://doi.org/10.1080/03075070802211729 .
Article Google Scholar
Baker, V. L., & Lattuca, L. R. (2010). Developmental networks and learning: Toward an interdisciplinary perspective on identity development during doctoral study. Studies in Higher Education, 35 (7), 807–827. https://doi.org/10.1080/03075070903501887 .
Bell, J. (2010). Doing your research project: A guide for first-time researchers in education, health and social science . Maidenhead: McGraw-Hill Open University Press.
Boud, D. (Ed.). (1988). Developing student autonomy in learning (2nd ed.). New York: Nichols Publishing Company.
Boud, D., & Walker, D. (1998). Promoting reflection in professional courses: The challenge of context. Studies in Higher Education, 23 (2), 191–206. https://doi.org/10.1080/03075079812331380384 .
Brew, A. (2001). Conceptions of research: A phenomenographic study. Studies in Higher Education, 26 (3), 271–285. https://doi.org/10.1080/03075070120076255 .
Bruce, N. (1995). Practising what we preach: Creating the conditions for student autonomy. Hong Kong Papers in Linguistics & Language Teaching , 18 , 73–88. Retrieved from http://hkjo.lib.hku.hk/archive/files/30cc63ad3122e0ad29590de2006ba6d0.pdf .
Butler, S. (1999). Catalysing student autonomy through action research in a problem centered learning environment. Research in Science Education, 29 (1), 127–140. https://doi.org/10.1007/BF02461184 .
Carver, C., & Scheier, M. (2005). Optimism. In C. R. Snyder & S. J. Lopez (Eds.), Handbook of positive psychology (pp. 231–256). New York, NY: Oxford University Press.
Castello, M., Kobayashi, S., McGinn, M. K., Pechar, H., Vekkaila, J., & Wisker, G. (2017). Researcher identity in transition: Signals to identify and manage spheres of activity in a risk-career. Frontline Learning Research , 3 (3), 19–33. https://doi.org/10.14786/flr.v3i3.149 .
Davies, A., & Danaher, P. A. (2014). Capacity-building for western expatriate nurses and Australian early career researchers. Procedia—Social and Behavioral Sciences, 112, 373–381. https://doi.org/10.1016/j.sbspro.2014.01.1177 .
Elbow, P. (1973). Writing without teachers . Oxford: Oxford University Press.
Fazey, D. M. A., & Fazey, J. A. (2001). The potential for autonomy in learning: Perceptions of competence, motivation and locus of control in first-year undergraduate students. Studies in Higher Education, 26 (3), 345–361. https://doi.org/10.1080/03075070120076309 .
Flanagan, M. (2018). Michael Thomas Flanagan, University College London, London . Retrieved April 4, 2019, from https://www.ee.ucl.ac.uk/~mflanaga .
Grant, B. M. (2008). Agonistic struggle: Master-slave dialogues in humanities supervision. Arts and Humanities in Higher Education, 7 (1), 9–27. https://doi.org/10.1177/1474022207084880 .
Haksever, A. M., & Manisali, E. (2000). Assessing supervision requirements of PhD students: The case of construction management and engineering in the UK. European Journal of Engineering Education, 25 (1), 19–32. https://doi.org/10.1080/030437900308616 .
Healey, M., Bovill, C., & Jenkins, A. (2015). Students as partners in learning. In J. Lea (Ed.), Enhancing learning and teaching in higher education: Engaging with the dimensions of practice (pp. 141–172). Maidenhead: Open University Press.
Healey, M., Flint, A., & Harrington, K. (2014). Engagement through partnership: Students as partners in learning and teaching in higher education . York: HE Academy. Retrieved from https://www.heacademy.ac.uk/knowledge-hub/engagement-through-partnership-students-partners-learning-and-teaching-higher .
Healey, M., Flint, A., & Harrington, K. (2016). Students as partners: Reflections on a conceptual model. Teaching and Learning Inquiry , 4 (2), 1–13. https://doi.org/10.20343/teachlearninqu.4.2.3 .
Holbrook, A., Shaw, K., Scevak, J., Bourke, S., Cantwell, R., & Budd, J. (2014). PhD candidate expectations: Exploring mismatch with experience. International Journal of Doctoral Studies , 9 , 329–346. https://doi.org/10.28945/2078 .
Ivanic, R. (1998). Writing and identity: The discoursal construction of identity in academic writing . Amsterdam: John Benjamins.
Book Google Scholar
Jenkins, E. W. (2001). Research in science education in Europe: Retrospect and prospect. In H. Behrendt (Ed.), Research in science education: Past, present and future (pp. 17–26). Dordrecht, The Netherlands: Kluwer Academic. https://doi.org/10.1007/0-306-47639-8_2 .
Johansson, T., Wisker, G., Claesson, S., Strandler, O., & Saalman, E. (2013). PhD supervision as an emotional process—Critical situations and emotional boundary work. Pertanika: Journal of Social Science and Humanities , 22 , 605–622.
Kiley, M., & Wisker, G. (2009). Threshold concepts in research education and evidence of threshold crossing. Higher Education Research and Development, 28 (4), 431–441. https://doi.org/10.1080/07294360903067930 .
Kobayashi, S., Grout, B., & Rump, C. Ø. (2013). Interaction and learning in PhD supervision—A qualitative study of supervision with multiple supervisors. Dansk Universitetspaedagogisk Tidsskrift , 8 (14), 13–25. Retrieved from https://tidsskrift.dk/dut/article/download/7174/6653 .
Kobayashi, S., Grout, B. W., & Rump, C. Ø. (2015). Opportunities to learn scientific thinking in joint doctoral supervision. Innovations in Education and Teaching International, 52 (1), 41–51. https://doi.org/10.1080/14703297.2014.981837 .
Li, S., & Seale, C. (2007). Learning to do qualitative data analysis: An observational study of doctoral work. Qualitative Health Research, 17 (10), 1442–1452. https://doi.org/10.1177/1049732307306924 .
Land, R., Meyer, J., & Smith, J. (Eds.). (2008). Threshold concepts within the disciplines . Rotterdam: Sense.
Leibowitz, B., Wisker, G., & Lamberti, P. (2018). ‘Crossing over’ into research on teaching and learning. In E. Bitzer (Ed.), Spaces, journeys and new horizons for postgraduate supervision (pp. 149–162). Stellenbosch, South Africa: SUN Press.
Lombardo, T. (2006). The evolution of future consciousness: The nature and historical development of the human capacity to think about the future . Bloomington, IN: AuthorHouse.
Lombardo, T. (2007). Developing constructive and creative attitudes and behaviors about the future: Part III—The self‐narrative, optimism and self‐efficacy, and the evolving future self. World Futures Study Federation : Futures Bulletin , 32 (2). Retrieved from https://www.centerforfutureconsciousness.com/pdf_files/WFSFArticles/Bulletins/FutConsArticle3.pdf .
Lombardo, T., & Richter, J. (2004). Evolving future consciousness through the pursuit of virtue. In H. Didsbury (Ed.), Thinking creatively in turbulent times . Bethesda, MD: World Future Society.
Meyer, J. H. F., & Land, R. (2003). Threshold concepts and troublesome knowledge: Linkages to ways of thinking and practising within the disciplines. In C. Rust (Ed.), Improving student learning: Improving student learning theory and practice—Ten years on . Oxford: Oxford Centre for Staff and Learning Development.
McAlpine, L. (2012). Shining a light on doctoral reading: Implications for doctoral identities and pedagogies. Innovations in Education and Teaching International, 49 (4), 351–361. https://doi.org/10.1080/14703297.2012.728875 .
McAlpine, L., Amundsen, C., & Turner, G. (2013). Identity-trajectory: Reframing early career academic experience. British Educational Research Journey, 40 (6), 952–969. https://doi.org/10.1002/berj.3123 .
Morris, C., & Wisker, G. (2011). Troublesome encounters: Strategies for managing the wellbeing of master’s and doctoral education students during their learning processes (HEA ESCalate Subject Centre Report). Retrieved from http://escalate.ac.uk/6828 .
Muurlink, O., & Poyatos Matas, C. (2010). A higher degree of stress: Academic wellbeing. In L. Marshall & C. Morris (Eds.), Taking wellbeing forward in higher education: Reflections on theory and practice (pp. 60–71). Falmer: University of Brighton Press.
Murray, R. (2016). How to write a thesis (4th ed.). Maidenhead: Open University Press.
Murtonen, M. (2005). University students’ research orientations: Do negative attitudes exist toward quantitative methods? Scandinavian Journal of Educational Research, 49 (3), 263–280. https://doi.org/10.1080/00313830500109568 .
Murtonen, M. (2015). University students’ understanding of the concepts empirical, theoretical, qualitative, and quantitative research. Teaching in Higher Education, 20 (7), 684–698. https://doi.org/10.1080/13562517.2015.1072152 .
Murtonen, M., Olkinuora, E., Tynjälä, P., & Lehtinen, E. (2008). “Do I need research skills in working life?”: University students’ motivation and difficulties in quantitative methods courses. Higher Education, 56 (5), 599–612. https://doi.org/10.1007/s10734-008-9113-9 .
Phillips, E., & Pugh, D. S. (2010). How to get a PhD: A handbook for students and their supervisors . Maidenhead: McGraw-Hill Open University Press.
Poyatos Matas, C. (2008, July). An innovative approach to research supervision . Paper presented at the University of Brighton Learning and Teaching Conference, Brighton, UK.
Poyatos Matas, C. (2009). A new approach to research supervision. In J. Barlow, G. Louw, & M. Rice (Eds.), Social purpose and creativity—Integrating learning in the real world (pp. 28–32). Falmer: University of Brighton Press.
Pyhältö, K., Nummenmaa, A. R., Soini, T., Stubb, J., & Lonka, K. (2012). Research on scholarly communities and development of scholarly identity in Finnish doctoral education. In T. S. Ahola & D. M. Hoffman (Eds.), Higher education research in Finland: Emerging structures and contemporary issues (pp. 337–357). Jyväskylä, Finland: Jyväskylä University Press.
Schulze, S. (2012). Empowering and disempowering students in student-supervisor relationships. Koers-Bulletin for Christian Scholarship, 77 (2), 1–8. https://doi.org/10.4102/koers.v77i2.47 .
Strandler, O., Johansson, T., Wisker, G., & Claesson, S. (2014). Supervisor or counsellor? Emotional boundary work in supervision. International Journal for Researcher Development, 5 (2), 70–82. https://doi.org/10.1108/IJRD-03-2014-0002 .
Trafford, V., & Leshem, S. (2009). Doctorateness as a threshold concept. Innovations in Education and Teaching International , 46 (3), 305–316. https://doi.org/10.1080/14703290903069027 .
Vekkaila, J., Pyhältö, K., & Lonka, K. (2013). Focusing on doctoral students’ experiences of engagement in thesis work. Frontline Learning Research, 1 (2), 10–32. https://doi.org/10.14786/flr.v1i2.43 .
Walliman, N. S. R. (2011). Your research project: Designing and planning your work . London: Sage.
Willison, J. (2009). Multiple contexts, multiple outcomes, one conceptual framework for research skill development in the undergraduate curriculum. CUR Quarterly , 29 (3), 10–14. Retrieved from https://www.adelaide.edu.au/rsd/evidence/related-articles/willison_2009_CURQ_29_3_.pdf .
Willison, J. (2012). When academics integrate research skill development in the curriculum. Higher Education Research & Development, 31 (6), 905–919. https://doi.org/10.1080/07294360.2012.658760 .
Willison, J., & O’Regan, K. (2005). 2020 Vision: An information literacy continuum for students primary school to post graduation. In A. Brew & C. Asmar (Eds.), Higher education in a changing world: Proceedings of the 28th HERDSA annual conference (pp. 633–641). Sydney, NSW, Australia: Higher Education Research and Development Society of Australasia.
Willison, J., & O’Regan, K. (2006). Research skill development framework . Retrieved April 4, 2019, from www.adelaide.edu.au/rsd .
Willison, J., Sabir, F., & Thomas, J. (2017). Shifting dimensions of autonomy in students’ research and employment. Higher Education Research and Development, 36 (2), 430–443. https://doi.org/10.1080/07294360.2016.1178216 .
Wisker, G. (2001). The postgraduate research handbook . Basingstoke: Palgrave Macmillan.
Wisker, G. (2009/2018). The undergraduate research handbook. Basingstoke: Palgrave Macmillan.
Wisker, G. (2012). The good supervisor: Supervising postgraduate and undergraduate research for doctoral theses and dissertations . Basingstoke: Palgrave Research Skills.
Wisker, G. (2014). Voice, vision and articulation: Conceptual threshold crossing in academic writing. In C. O’Mahony, A. Buchanan, M. O’Rourke, & B. Higgs (Eds.), Proceedings of the National Academy’s sixth annual conference and the fourth biennial threshold concepts conference (pp. 159–164). Cork, Ireland: NAIRTL. Retrieved from https://eric.ed.gov/?id=ED558533 .
Wisker, G. (2015). Getting published . London: Palgrave Macmillan.
Wisker, G. (2016). Toil and trouble: Professional and personal expectations and identities in academic writing for publication. In J. Smith (Ed.), Identity work in the contemporary university: Exploring an uneasy profession (pp. 143–154). Rotterdam: Sense.
Wisker, G. (2018). Frameworks and freedoms: Supervising research learning and the undergraduate dissertation. Journal of University Teaching and Learning Practice , 15 (4), 1–14. Retrieved from https://ro.uow.edu.au/jutlp/vol15/iss4/2 .
Wisker, G., & Kiley, M. (2017). Helping students demonstrate mastery of doctoral threshold concepts. In S. Carter & D. Laurs (Eds.), Developing research writing: A handbook for supervisors and advisers (pp. 173–178). Abingdon: Routledge.
Chapter Google Scholar
Wisker, G., Kiley, M., & Aiston, S. (2006, March). Making the learning leap: Research students crossing conceptual thresholds . Paper presented at the quality in postgraduate research conference, Adelaide, Australia.
Wisker, G., Morris, C., Cheng, M., Masika, R., Warnes, M., Lilly, J. …, Robinson, G. (2010). Doctoral learning journeys—Final report of the NTFS-funded project . Retrieved from http://www.heacademy.ac.uk/resources/detail/ntfs/Projects/Doctoral_Learning_Journeys .
Wisker, G., & Robinson, G. (2013). Doctoral ‘orphans’: Nurturing and supporting the success of postgraduates who have lost their supervisors. Higher Education Research & Development, 32 (2), 300–313. https://doi.org/10.1080/07294360.2012.657160 .
Wisker, G., Robinson, G., & Kiley, M. (2008, March). Crossing liminal spaces: Encouraging postgraduate students to cross conceptual thresholds and achieve threshold concepts in their research . Paper presented at the quality in postgraduate research: Research education in the new global environment—Part 2. Canberra, Australia.
Wisker, G., Robinson, G., Trafford, V., Warnes, M., & Creighton, E. (2003). From supervisory dialogues to successful PhDs: Strategies supporting and enabling the learning conversations of staff and students at postgraduate level. Teaching in Higher Education, 8 (3), 383–397. https://doi.org/10.1080/13562510309400 .
Wisker, G., & Savin-Baden, M. (2009). Priceless conceptual thresholds: Beyond the ‘stuck place’ in writing. London Review of Education, 7 (3), 235–247. https://doi.org/10.1080/14748460903290207 .
Download references
Author information
Authors and affiliations.
University of Brighton, Brighton, UK
Gina Wisker
University of Johannesburg, Johannesburg, South Africa
You can also search for this author in PubMed Google Scholar
Corresponding author
Correspondence to Gina Wisker .
Editor information
Editors and affiliations.
Faculty of Education and Culture, Tampere University, Tampere, Finland
Mari Murtonen
Department of Higher Education, University of Surrey, Guildford, UK
Kieran Balloo
Rights and permissions
Reprints and permissions
Copyright information
© 2019 The Author(s)
About this chapter
Wisker, G. (2019). Developing Scientific Thinking and Research Skills Through the Research Thesis or Dissertation. In: Murtonen, M., Balloo, K. (eds) Redefining Scientific Thinking for Higher Education. Palgrave Macmillan, Cham. https://doi.org/10.1007/978-3-030-24215-2_9
Download citation
DOI : https://doi.org/10.1007/978-3-030-24215-2_9
Published : 22 September 2019
Publisher Name : Palgrave Macmillan, Cham
Print ISBN : 978-3-030-24214-5
Online ISBN : 978-3-030-24215-2
eBook Packages : Education Education (R0)
Share this chapter
Anyone you share the following link with will be able to read this content:
Sorry, a shareable link is not currently available for this article.
Provided by the Springer Nature SharedIt content-sharing initiative
- Publish with us
Policies and ethics
- Find a journal
- Track your research

- < Previous
Home > Open Educational Resources > 6
Open Educational Resources
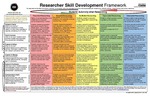
Researcher Skill Development Framework (US English Edition)
John Willison , The University of Adelaide Kerry O'Regan , The University of Adelaide Sara K. Kuhn , University of North Dakota Follow
Download Full Text (183 KB)
Download Researcher Skill Development Framework (US English Edition) DOC (Editable) (62 KB)
Description
Created by John Willison and Kerry O'Regan.
www.rsd.edu.au
Adapted for the US context by Sara K. Kuhn.
"Research Skill Development (RSD) is about making explicit and coherent in regular university coursework the incremental attainment of research skills in a specific discipline. In the RSD, there are six facets of the research process, identified from the literature and modified according to Bloom’s taxonomy and our experiences of using the framework in the disciplines. The meaning of ‘research’ in this context is: students actively finding information new to themselves. Underlying this notion is the ‘degree of knowness’ of knowledge: whether research involves developing knowledge that is commonly known to humanity, commonly unknown or totally unknown. We see that even inquiry into the commonly known is all part of the process of research skill development. Indeed, to overlook the development of skills in earlier years of education (such as First Year university) is to miss the potential development of skills required of ‘ blue-sky’ researchers or by industry and employment." -- https://www.adelaide.edu.au/rsd/framework/explanation/
Description adapted from: Willison, J., & O’Regan, K. (2007). Commonly known, commonly not known, totally unknown: A framework for students becoming researchers. Higher Education Research & Development, 26(4), 393-409. Retrieved from https://www.adelaide.edu.au/rsd/evidence/related-articles/RSD_article_web.doc
Publication Date
Document type.
Course Material
research skills, assessment, curriculum, knowledge development, scholarly communication, educational frameworks, educational methods
- Disciplines
Educational Assessment, Evaluation, and Research | Scholarly Communication
Creative Commons License

Recommended Citation
Willison, John; O'Regan, Kerry; and Kuhn, Sara K., "Researcher Skill Development Framework (US English Edition)" (2018). Open Educational Resources . 6. https://commons.und.edu/oers/6
Since July 27, 2018
Included in
Educational Assessment, Evaluation, and Research Commons , Scholarly Communication Commons
Advanced Search
- Notify me via email or RSS
- Collections
Author Corner
- Author Submission Agreement
- About the Library
Home | About | FAQ | My Account | Accessibility Statement
Privacy Copyright

- Subject Guides
Open Research Skills Framework: a Practical Guide
- Benefits of Open Research
- Open Research Lifecycle
- Open Research Community

The Open Research Skills Framework provides an overview of open research practices and principles which can be applied to projects across all disciplines.

This framework was created in partnership with practitioners and is intended for researchers of all levels, and across all disciplines.
The Open Research team are keen to hear if you have any questions or thoughts on the framework, and particularly interested to hear from any researchers who are considering adapting the framework to suit their discipline: [email protected]
What is open research?

'Open research' or 'open science'?
These terms are sometimes used interchangeably, but are based on the same principles of collaboration and accountability. Open research is relevant to all researchers (not just scientists), but its applications will differ between disciplines.
Open research values
Open research is built on accessible , reusable , reproducible , collaborative and transparent practices.

Accessibility
Research should allow anyone, whether from the academic community or general public, to access knowledge.

Reusability
Research data and publications can be interrogated, built upon and adapted.
Reproducibility
Research methods and data are made available for others to replicate and validate.

Collaboration
Research should be promoted across borders, cultures and disciplines to create benefits for all.

Transparency
Research should be open as possible, as closed as necessary.
Training sessions for postgraduate researchers and staff
The Open Research team offer training to postgraduate researchers and staff through the Building Research and Innovation Capacity (BRIC) programme.
To book a session go to: SkillsForge - Postgraduate Researchers or LMS - Staff (login required).
Next sessions:
If you need information, take a look at the:
- Bibliometrics: a practical guide
- Copyright: a practical guide
- Creative Commons for researchers: a practical guide
- Open access publishing: a practical guide
- Open research: a practical guide
- Research data management: a practical guide or complete the RDM 101 online tutorial in the VLE
You can also contact the team by email [email protected] to:
- ask a question
- request training for your department, research group, staff and/or postgraduate researchers
Alternatively, book an Open Research Online Appointment to discuss your individual needs.
What are OER?

OER can be built on the findings and outputs of open research, and encompass a range of formats including courseware, textbooks, games and videos.
The White Rose Libraries OER Toolkit provides information and tools to help academic and library staff to understand, engage with, and sustain OER in their work and practice.

Illustrations by Manfred Steger , licensed for reuse under a Pixabay Content licence .
- Next: Benefits of Open Research >>
- Last Updated: Jan 29, 2024 1:13 PM
- URL: https://subjectguides.york.ac.uk/openresearch
Defining Deeper Learning and 21st Century Skills
Life in the 21st century requires people to be prepared to fill a variety of roles—as workers, parents, citizens, and consumers—in which they will need to apply their knowledge and skills effectively to rapidly changing situations. Recognizing this need, business, political, and educational leaders are increasingly asking schools to teach students the competencies they will need to navigate a changing world—skills such as problem solving, critical thinking, and collaboration. Such skills are often referred to as “21st century skills,” “soft skills,” or “deeper learning.”
Publications
Education for Life and Work: Developing Transferable Knowledge and Skills in the 21st Century
Americans have long recognized that investments in public education contribute to the common good, enhancing national prosperity and supporting stable families, neighborhoods, and communities. Education is even more critical today, in the face of economic, environmental, and social challenges. Today's children can meet future challenges if their schooling and informal learning activities prepare them for adult roles as citizens, employees, managers, parents, volunteers, and entrepreneurs. To achieve their full potential as adults, young people need to develop a range of skills and knowledge that facilitate mastery and application of English, mathematics, and other school subjects. At the same time, business and political leaders are increasingly asking schools to develop skills such as problem solving, critical thinking, communication, collaboration, and self-management - often referred to as "21st century skills."
Education for Life and Work: Developing Transferable Knowledge and Skills in the 21st Century describes this important set of key skills that increase deeper learning, college and career readiness, student-centered learning, and higher order thinking. These labels include both cognitive and non-cognitive skills- such as critical thinking, problem solving, collaboration, effective communication, motivation, persistence, and learning to learn. 21st century skills also include creativity, innovation, and ethics that are important to later success and may be developed in formal or informal learning environments.
This report also describes how these skills relate to each other and to more traditional academic skills and content in the key disciplines of reading, mathematics, and science. Education for Life and Work: Developing Transferable Knowledge and Skills in the 21st Century summarizes the findings of the research that investigates the importance of such skills to success in education, work, and other areas of adult responsibility and that demonstrates the importance of developing these skills in K-16 education. In this report, features related to learning these skills are identified, which include teacher professional development, curriculum, assessment, after-school and out-of-school programs, and informal learning centers such as exhibits and museums.
Read Full Description
- Press Release
- Education for Life and Work: Guide for Practitioners
- Policy Highlights
- Report Brief
Description
An ad hoc committee will review and synthesize current research on the nature of deeper learning and 21st century skills and will address the following:
- Define the set of key skills that are referenced by the labels “deeper learning,” “21st century skills,” “college and career readiness,” “student centered learning,” “next generation learning,” “new basic skills,” and “higher order thinking.” These labels are typically used to include both cognitive and non cognitive skills - such as critical thinking, problem solving, collaboration, effective communication, motivation, persistence, and learning to learn that can be demonstrated within core academic content areas and that are important to success in education, work, and other areas of adult responsibility. The labels are also sometimes used to include other important capacities - such as creativity, innovation, and ethics - that are important to later success and may also be developed in formal or informal learning environments.
- Describe how these skills relate to each other and to more traditional academic skills and content in the key disciplines of reading, mathematics, and science. In particular, consider these skills in the context of the work of the National Governors Association and the Council of Chief State School Officers in specifying Common Core State Standards for English language arts and mathematics, and the work of the NRC in specifying a Conceptual Framework for New Science Education Standards.
- Summarize the findings of the research that investigates the importance of such skills to success in education, work, and other areas of adult responsibility and that demonstrates the importance of developing these skills in K-16 education.
- Summarize what is known - and what research is needed - about how these skills can be learned, taught, and assessed. This summary should include both the cognitive foundations of these skills in learning theory and research about effective approaches to teaching and learning these skills, including approaches using digital media.
- Identify features of educational interventions that research suggests could be used as indicators that an intervention is likely to develop the key skills in a substantial and meaningful way. In particular, for learning in formal school-based environments, identify features related to learning these skills in educational interventions in a) teacher professional development, b) curriculum, and c) assessment For learning in informal environments, identify features related to learning these skills in educational interventions in d) after-school and out-of-school programs and e) exhibits, museums, and other informal learning centers. For learning in both formal and informal environments, identify features related to learning these skills in education interventions in f) digital media.
The conclusions and recommendations of the report will provide a common foundation for further research and policy work that seeks to improve the way these skills are developed in K-16 education. The primary messages from the report will be distilled into a short report brief that could be broadly disseminated.
- Division of Behavioral and Social Sciences and Education
- Board on Testing and Assessment
Center for Education
Consensus Study
Contact the Public Access Records Office to make an inquiry, request a list of the public access file materials, or obtain a copy of the materials found in the file.

Past Events
Multiday Event | September 13-14, 2011
[Closed] Defining Deeper Learning and 21st Century Skills Third Committee Meeting
Multiday Event | June 16-17, 2011
[Closed] Second Committee Meeting
Multiday Event | March 26-27, 2011
[Closed] Defining Deeper Learning and 21st Century Skills
Responsible Staff Officers
- Margaret Hilton
Additional Project Staff
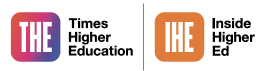
A framework to teach library research skills
Embedding library skills within disciplines allows teachers and students to link them to learning outcomes and research requirements. Here, a team of engagement librarians explain how to put a map in place
.css-76pyzs{margin-right:0.25rem;} ,
Karen rolfe, siân furmage, michael latham.
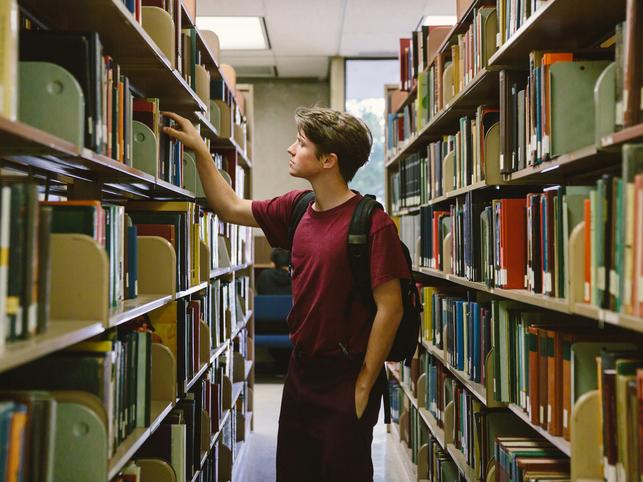
Created in partnership with
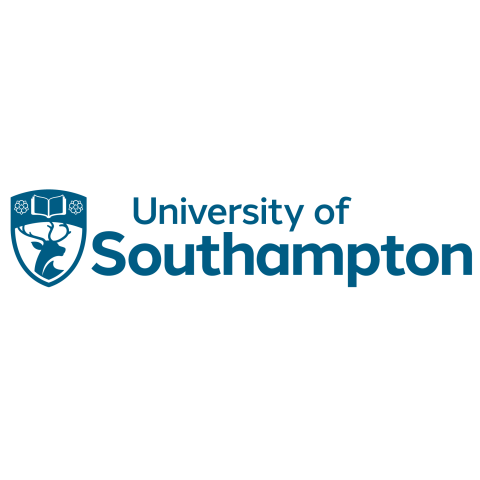
You may also like
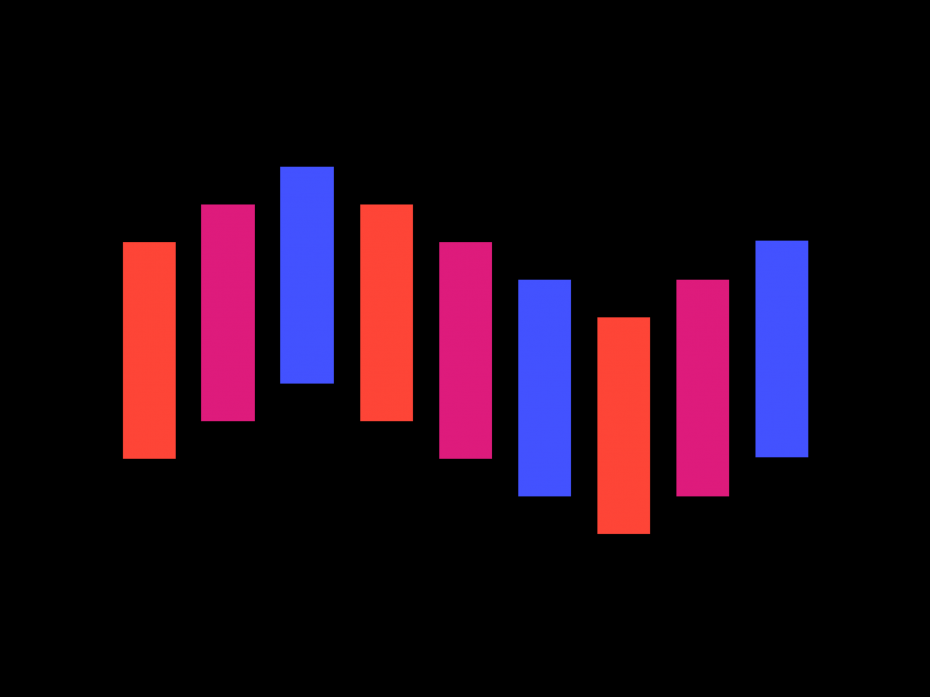
Popular resources
.css-1txxx8u{overflow:hidden;max-height:81px;text-indent:0px;} Analytical testing is the key to industry collaborations
Is it time to turn off turnitin, use ai to get your students thinking critically, taming anxiety around public speaking, emotions and learning: what role do emotions play in how and why students learn.
Teaching library skills might be ill placed during induction weeks. Rather, evidence suggests that embedding library research and other transferable skills within disciplines supports students’ learning and retention of skills. Using a framework to support embedded library skills teaching in this way is in line with evidence-based practice .
Library inductions at the start of a new academic year are also time and staff intensive. As library educators, we want to be consistent with the content we cover with students at all levels, providing our teaching at a point of need. We want to link the library skills students are developing to their subjects, learning outcomes and assessments that need library research skills (such as finding and citing literature).
- THE podcast: how the university library is an agent of change
- Tips (from a librarian) for creating assignment prompts
- Open access is inevitable – only the ‘how’ remains up for discussion
A library research skills framework provides values, standards and skills for students at all levels (and educators) to work towards; it establishes learner expectations and allows for year-on-year development. A framework can offer a structure for up-to-date and consistent skills training for students. It also gives library and academic staff a clear reference for curriculum mapping to highlight priorities and actions across qualifications, and to identify and open conversations around gaps in students’ library skills.
How we created our library research skills framework
Our library research skills framework describes six areas:
- Transition skills
- Online study
- Finding information
- Evaluating information
- Citing and referencing
- Digital literacy.
Learners should be supported to develop these skills during their undergraduate or taught postgraduate degree programmes. Our framework describes their expected progression, from being a new undergraduate to final-year student and into employment.
Our framework was created after benchmarking other national and international skills frameworks. We asked academic and library colleagues to comment on an initial draft, then incorporated their feedback and piloted the framework with two academic schools. We gathered more feedback from the teaching staff, librarians and students, and incorporated their input into the next iteration. We now have a process to continuously review and develop our framework.
How do we use our library research skills framework?
Our library research skills framework has been instrumental in helping our librarians to create curriculum maps. Librarians have identified the core or compulsory modules within each programme and mapped the learning outcomes to the skill areas of the framework. This allows our librarians to align their teaching, and learners can understand the link between library research skills and their subjects. As a result, librarians’ teaching is, as a whole, more sustainable and provides better quality support for our students.
Ultimately, we want to map out the best path for our learners to acquire library research skills throughout their degrees and into their lives post-qualification. The framework helps us to have conversations with our academics about creating relevant learning outcomes and touch points in their programmes and modules. It includes content, resources and activities pitched at the appropriate level for the learners and which librarians can use in their teaching or academic staff can use independently with their students.
What academics, students and librarians say about our framework
The University of Southampton’s education and student experience committee has endorsed our new approach to embedded teaching of library research skills. Feedback from academics in our pilots indicates that they fully support our approach.
“The library research skills framework is a welcome approach to improving students’ information literacy and awareness of library resources and support…We are keen to move away from overloading students with information during induction and move towards support at the point of need throughout the academic journey,” said a principal teaching fellow for education development in the Southampton Business School.
Our librarians have found the framework a helpful tool in combination with curriculum mapping the programmes they teach on.
“Curriculum mapping has ensured that I teach the library session at the appropriate time and align it with the learning outcomes of the course. Using the framework enabled me to pitch my training session at the right level, and its flexibility means that I can choose the relevant elements according to the experience of the students,“ said an engagement librarian to the School of Psychology.
How to create a library research skills framework
To create a library research skills framework at your university, first decide what skills your learners will need as they progress through their courses and once they go on to employment. Benchmark against other comparable frameworks and find out if you can use any in their entirety or adapt one that is appropriately licensed under Creative Commons as your starting point. Get feedback on your framework from your academics, librarians and students to make sure it meets the needs of your learners and allow it to evolve.
Our framework is a living, evolving document that incorporates developments our learners encounter in their studies, such as generative artificial intelligence. We review and update it twice a year. We are developing our equity, diversity and inclusivity content, for example, working in collaboration with academics to embed teaching on diversifying sources. We are in the early stages of planning a piece of research to evaluate the impact of our framework.
Anna Hvass is head of curriculum engagement ; Karen Rolfe, Siân Furmage and Michael Latham are engagement librarians. All are at the University of Southampton.
If you would like advice and insight from academics and university staff delivered direct to your inbox each week, sign up for the Campus newsletter .
Analytical testing is the key to industry collaborations
Contextual learning: linking learning to the real world, how hard can it be testing ai detection tools, chatgpt’s impact on nursing education and assessments, how to tackle the phd dissertation.
Register for free
and unlock a host of features on the THE site
Skip to content. | Skip to navigation
Personal tools

- Log in/Register Register

https://www.vitae.ac.uk/researchers-professional-development/about-the-vitae-researcher-development-framework/about-the-vitae-researcher-development-framework
This page has been reproduced from the Vitae website (www.vitae.ac.uk). Vitae is dedicated to realising the potential of researchers through transforming their professional and career development.
- Vitae members' area
About the Vitae Researcher Development Framework
The Vitae Researcher Development Framework (RDF) is for you if you are doing a doctorate, are a member of research staff, pursuing an academic career or thinking about applying the skills developed during your PhD in another career.

What is the RDF?
The framework describes the knowledge, behaviour and attributes of successful researchers.
Download the RDF (PDF)
More about the RDF's structure and content.

Some ways to use the RDF
- Identify your strengths and gaps in development
- Prioritise development needs
- Monitor progress
How other researchers have used the RDF

Conditions of use
We want all researchers in the world to benefit from the RDF so we are making it available to use subject to Conditions of Use on a non commercial basis.
All we ask is you let us know how you are using it by filling in this simple form.

RDF Planner app
Our online app based on the RDF is an approach to planning your professional development.
Use it to identify strengths, plan actions, review achievements and create a portfolio of evidence.

How can the RDF help you?
Find out how PGRs have benefitted from using RDF
Using the RDF for researchers
The RDF for researcher developers
For the supervisor, Research Director or PI
Are you a senior decision-maker?

Links and documents
Search RDF-related documents
Find RDF graphics
List of top RDF resources
Focus on a specific context with RDF lenses
RDF development cards
Download an accessible version of the RDF graphic
Bookmark & Share

Thank you for visiting nature.com. You are using a browser version with limited support for CSS. To obtain the best experience, we recommend you use a more up to date browser (or turn off compatibility mode in Internet Explorer). In the meantime, to ensure continued support, we are displaying the site without styles and JavaScript.
- View all journals
- My Account Login
- Explore content
- About the journal
- Publish with us
- Sign up for alerts
- Open access
- Published: 06 May 2024
An adaptable and personalized framework for top-N course recommendations in online learning
- Samina Amin 1 ,
- M. Irfan Uddin 1 ,
- Ala Abdulsalam Alarood 2 ,
- Wali Khan Mashwani 3 ,
- Ahmed Omar Alzahrani 4 &
- Hamdan Ahmed Alzahrani 5
Scientific Reports volume 14 , Article number: 10382 ( 2024 ) Cite this article
229 Accesses
Metrics details
- Computational science
- Computer science
- Information technology
- Scientific data
In recent years, the proliferation of Massive Open Online Courses (MOOC) platforms on a global scale has been remarkable. Learners can now meet their learning demands with the help of MOOC. However, learners might not understand the course material well if they have access to a lot of information due to their inadequate expertise and cognitive ability. Personalized Recommender Systems (RSs), a cutting-edge technology, can assist in addressing this issue. It greatly increases resource acquisition through personalized availability for various people of all ages. Intelligent learning methods, such as machine learning and Reinforcement Learning (RL) can be used in RS challenges. However, machine learning needs supervised data and classical RL is not suitable for multi-task recommendations in online learning platforms. To address these challenges, the proposed framework integrates a Deep Reinforcement Learning (DRL) and multi-agent approach. This adaptive system personalizes the learning experience by considering key factors such as learner sentiments, learning style, preferences, competency, and adaptive difficulty levels. We formulate the interactive RS problem using a DRL-based Actor-Critic model named DRR, treating recommendations as a sequential decision-making process. The DRR enables the system to provide top-N course recommendations and personalized learning paths, enriching the student's experience. Extensive experiments on a MOOC dataset such as the 100 K Coursera course review validate the proposed DRR model, demonstrating its superiority over baseline models in major evaluation metrics for long-term recommendations. The outcomes of this research contribute to the field of e-learning technology, guiding the design and implementation of course RSs, to facilitate personalized and relevant recommendations for online learning students.
Similar content being viewed by others
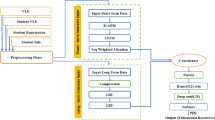
Dynamic educational recommender system based on Improved LSTM neural network
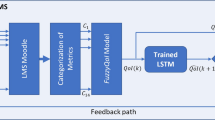
DeepLMS: a deep learning predictive model for supporting online learning in the Covid-19 era
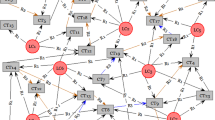
Interpretable early warning recommendations in interactive learning environments: a deep-neural network approach based on learning behavior knowledge graph
Introduction.
With the advancement of information technology, online learning has rapidly become a necessary platform for knowledge acquisition. Massive Open Online Courses (MOOC) platforms are recent advancements of this online learning movement. They have received a lot of attention from the academic and public domains. This development of numerous MOOC platforms, including Udemy, edX, Coursera, Udacity, and others. More than 100 million students worldwide have access to convenient education through these platforms. As they offer an affordable way to take appropriate courses at many prestigious universities 1 . Due to COVID-19's influence in the past two years, 2021 has witnessed a rise in MOOC, making it more challenging to execute traditional teaching methods in many regions 1 . MOOC has quickly replaced the traditional mode of classroom learning. It enabled the idea of being able to study anywhere and whenever possible. Learners frequently use online learning platforms to increase their knowledge, develop new abilities or skills, and conduct academic research.
Despite the many advantages that come with MOOC, it also leads to the extremely key challenge of information overload 1 . In order to assist learners in choosing appropriate courses from the thousands of courses accessible for learning, it is also required to consider the learner's career decisions and other factors. To address this, personalized Recommender Systems (RSs) 2 , 3 have emerged as a solution, aiming to match learners' preferences with relevant content and mitigate information overload.
While existing RSs have employed techniques like content-based filtering, Collaborative Filtering (CF), and hybrid methods 4 , 5 . Recently, motivated by the quick development of online learning, various recommendation methods have been developed 6 , 7 . They applied supervised learning and data mining techniques to develop RSs for MOOC. However, these existing RSs usually neglect interactions between a user and the recommendation algorithm. They often fall short in capturing user interactions and adapting to new circumstances, which leads to poor recommendation outcomes.
The nature of learner interaction with RSs turns the recommendation problem into not just a classification/prediction issue but also a sequential decision problem. The machine learning domain focuses on how intelligent agents communicate with their surroundings 6 , 7 . Whereas RL 8 proposes methods for modeling user-agent interactions. It adopts the policy through trial-and-error search, which is helpful for sequential decision-making. Due to the evaluation of overall actions, the conventional RL approach has the drawback of potentially being inefficient if the action space is too big 9 . While DRL 10 , 11 offers more advanced learning algorithms and function approximation features to tackle challenges in artificial intelligence. However, it has been applied in many different applications; e.g., games 12 , trading 13 , robotics 14 , 15 , disruption risk identification 16 , and the Internet of Things (IoT) 17 , 18 , 19 , 20 . Recently, a new research trend in recommendation research is the use of RL to address recommendation problems 4 . In practice, RL-based RSs have been used for a variety of specialized applications, including movie recommendations 21 , news recommendations 22 , treatment recommendations 23 , 24 , opinion analysis 25 , and e-learning 26 , 27 .
Nowadays, the majority of students conduct their studies online. Students are having a very hard time picking appropriate content to come to this online learning system. Since the internet has a massive, varied, and dynamic data collection. A study of the user's profile and interests should be used to develop a personalized RS for the learners. In MOOC platforms, the learner’s browsing pattern, efficiency, and domain relevancy should all be acquired by intelligent agents; e.g., the way learners navigate varies from one another. The RL agent can learn from dynamically changing browsing activity and then make recommendations to the learner. The learner's feedback is collected by the agent. The agents should dynamically modify information based on the user's preferences as well as from time to time in online course recommendations. Due to the passage of time, one piece of information becomes outdated. MOOC systems should dynamically update content and topics. Course content should be updated regularly based on learner feedback and temporal variations. To address the drawbacks, it is necessary to create a multi-agent-based intelligent learning environment that automatically tracks the factors, which influence learning in various contexts and then updates recommended learning paths and content from one learner to the next.
To the best of our knowledge, none of the current studies have applied multi-agent DRL techniques for MOOC RS 10 . The advances in RL have had phenomenal achievements across many domains. Although the multi-agent domain has been dominated by its single-agent counterpart throughout this development, multi-agent RL is making headway quickly, and the most recent achievements deal with issues that are challenging in real-world applications. Inspired by recent advancements in AI and DRL, in this work, a novel multi-agent DRL intelligent framework is proposed for personalized learning and an optimizing RS for multipath navigation adaptively in MOOC. Another challenge is to analyze how the agents learn by themselves and select optimal actions according to deviations in their environment. If any agent fully grasps the policy, it will be able to decide what is the best approach for action in any given state. For example, if the Agent has acquired the best policy, it can accurately determine whether a relevant course is present to be recommended to the learner. Even humans do not understand how to recommend suitable course content; we simply recognize them when we see them. This makes the task difficult for a machine to complete and unfeasible when performed with state-of-the-art methods. These challenges will be framed within the Markov Decision Process (MDP) framework 8 . Then, the interactive RS problem will be formulated using a DRL recommendation framework such as an Actor-Critic named DRR.
The prime contributions and technical developments of the proposed approach are outlined as follows.
To propose an intelligent agent based on DRR such as Actor-Critic for top-N course recommendations in MOOC.
To develop an effective technique for the proposed smart learning environment to encourage students to benefit from the learning materials and become independent learners.
To accommodate potential dynamic changes in students' states and learning preference levels, by leveraging MDP terminologies where the agent is solely concerned with the present state of the process and lacks curiosity about the entire historical context or previous records when a new learner is registered, or new information is added to the system.
To evaluate the proposed model, experiments are conducted through simulations and compare its performance with current state-of-the-art solutions in sequential recommendation tasks for optimal results.
The experimental findings verify the effectiveness of the proposed model in providing top-N course recommendations in MOOCs by computing evaluation metrics.
The rest of this article is categorized as follows: Literature review based on traditional RS, RL, and DRL will be discussed in “ Literature review ”. “ Research gap ” presents research gaps. A detailed methodology of the proposed framework will be demonstrated in “ Overall study framework ”. Experimental results and analysis will be presented in “ Experiments ”. Finally, the conclusions, limitations, and future work are presented in “ Conclusions ”.
Literature review
Researchers have done significant work in RSs analysis and provided effective solutions for the recommendation of items using supervised learning and RL methods 4 . Shin et al., 2 focused on introducing an RS that can compute the optimal number and schedule of examinations for every learner based on the RL method. Wacharawan et al. 27 developed an RL-based online recommendation approach. The authors designed an RL agent based on state-action-reward-state-action performing dynamically and continuously in action space. To determine optimal policy, the agent selects the collection of actions. Then the performance of their model is evaluated with the real dataset from an OL system. However, the predictions’ accuracy still needs improvement, as the square root of the mean squared error is relatively high. Along with the approach to determine the ideal values of greedy, learning rate, and discount rate, it is important to investigate the other RL methods. Similarly, Lalitha et al., 7 proposed an agent-based recommendation for e-learning by utilizing machine learning and knowledge discovery techniques. In other related work, Zheng et al., 22 applied a DRL-based model for news recommendation, while Esfahaani et al., proposed RS for online advertising with the help of DRL. Using RL techniques, some other research studies have been carried out on movie recommendations 21 , 28 . To perform recommendations, they created an RL-based single agent, but the models are unable to adapt multiple users appropriately if different patterns are observed for distinct user groups. Since they experience fundamental problems while deploying a single agent to carry out many tasks, such as feature space. Employing multiple agents to execute similar tasks in parallel is an alternate approach to improving learning performance. As a result, to make the recommendations more accurate, the existing studies need to be extended.
Moreover, Lin et al. 29 developed a hierarchical RL with a dynamic recurrent mechanism to provide individualized course recommendations. They suggested a policy gradient method to solve the trade-off between exploration and exploitation while building user profiles. While applying a dynamic baseline to investigate the user’s future preferences, it implemented a recurrent approach through context-aware learning to use the present information. Vedavathi et al., 30 proposed a hybrid Elman similarity-based e-learning course RS framework using sentiment analysis. Using similarity measures, their proposed model was utilized to classify the sentiment. Yuan et al., 31 designed MOOC recommendations for personalized course RS based on integrating multi-granularity sessions and multi-type interests. Furthermore, by combining network structured features with graph neural networks and user interactive activities with tensor factorization. Zhu et al., 32 created a hybrid RS approach. First, a network with a graph structure for teaching assessment was suggested to characterize students, courses, and other entities utilizing the ratings, commentary text, grades, and personal connections of the students. The vectorized representation of the students was then produced by a neural network built on a random walk algorithm after each student learned their unique relational pattern. Finally, a Bayesian probabilistic-based tensor factorization is used to learn and predict students’ ratings for lectures they have not yet attended. This is done by identifying these personalization features as the third dimension of the rating tensor.
Likewise, Campos et al., 33 created RS for MOOC ecosystems, suggesting sections of courses from various MOOC platforms (such as Khan Academy, Udemy, and edX). In order to balance the ecological environment and strengthen connections, their suggested model relied on the student profile and the MOOC ecosystems approach. The results of an experiment were carried out using a dataset with 19 students, three MOOC platforms showed that the adopted methods are more reliable than other methods. Furthermore, it was determined that their recommendations are 62,24% correct, 68.89% valuable, 72.81% dependable, and present new content in 99.12% of cases. These outcomes demonstrate that the strategy helps students close their knowledge gaps. Through the application of machine learning techniques, Nilashi et al. 34 suggested an RS to promote courses in MOOCs based on the preferences and behavior of students. The approach was created employing multi-criteria ratings taken from learners’ reviews posted online. For text mining, they utilized Latent Dirichlet Allocation; for decision rule creation, they employed decision trees; for learner’s reviews of courses, they applied a self-organizing map; and for preference prediction, they adopted a fuzzy rule-based approach. They also used a feature selection mechanism to pick the most crucial factors for predicting learners’ preferences. The approach was assessed using data gathered from MOOC platforms such as Udemy. The findings demonstrated that the approach is capable of accurately offering learners relevant courses that are catered to their interests.
Symeonidis and Malakoudis 35 built an xSVD++-based RS model. The “x” stands for a multi-dimensional matrix factorization structure combined with the CF method, which utilizes data from external sources (such as users’ skills, course features, etc.) to forecast course patterns and carry out rating predictions in accordance with them. Additionally, Boratto et al., 36 explored how RS works in the context of MOOC, going beyond prediction accuracy. While Lin et al., 37 proposed a novel course RS based on dynamic attention mechanism and hierarchical RL, to increase the adaptivity of the RS. J. Using a graph neural network, Wang et al., 38 designed a Top-N personalized RS in the MOOC by exploring two different aggregate functions to compact with the learners’ sequence neighbors and then apply an attention algorithm to produce the final course suggestions. Yuanguo 39 designed a hierarchical and recurrent RL-based context-aware RL technique. Their model can effectively rebuild user profiles for course recommendation. Madani et al., 40 developed RS for directing learners into appropriate courses. The suggested approach relies on CF (using the concepts of sentiment analysis) and social filtering to determine the optimal manner for the student to study and to suggest courses that complement their profile and social media material.
Research gap
The existing landscape of RSs reveals several gaps that hinder their effectiveness. Firstly, prevalent RSs commonly adopt static techniques for recommendations, lacking an understanding that recommendation actions are sequential decision-making problems. This oversight contributes to shortcomings in adapting to new circumstances and addressing the cold-start problem. Additionally, these conventional RSs frequently overlook user interactions with the recommendation algorithm. This leads to a failure in a timely recording of user feedback, resulting in suboptimal recommendations. Furthermore, existing methods, while competent in classifying learners’ intelligence levels, often fall short in regulating actions for specific topics. The reliance on RL-based single agents in these methods becomes problematic when adapting to distinct user groups with varying patterns.
While these techniques are competent in terms of recommendations to some point; however, they have the flaw of ignoring the learner’s shifting preferences in personalized adaptive sequential learning ability/activities. Furthermore, these methods may not precisely capture the learner’s evolving preferences for individual content, especially when the learners’ preferences are being changed over time across various courses over time, which results in inadequate recommendations in MOOC scenarios.
To address these gaps, this study proposes a novel multi-agent DRL framework for top-N MOOC recommendations. The proposed framework aims to optimize RS for adaptive multipath navigation in the MOOC environment. The primary contribution lies in exploring how agents, through DRL, can maximize learner satisfaction and minimize interactions to create personalized learning paths. In addition, the proposed work studies the challenge of understanding how agents autonomously learn and select optimal actions based on environmental deviations. By empowering agents to grasp policies, the proposed framework seeks to enable them to make informed decisions for optimal actions in any given state.
Overall study framework
In this section, the proposed model based on DRR is introduced, which is a top-n list-wise model for implicit feedback. First, the problem formulation is discussed. Then, the proposed model is presented in detail. Finally, it is proved that the discussed models have the potential to be streamlined into a top-N coarse recommendations model, by achieving promising results in terms of recommendation accuracies.
Problem formulation
The proposed framework can be divided into two main components: the learner agent and the recommendation agent. The learner agent is responsible for modelling the learner's behaviors, learning style, preferences, competency, adaptive difficulty levels, and knowledge level over time. It uses DRL algorithms with MDP terminologies to learn from the learner's interactions with the system and adapt to their changing needs or preferences during the study. The recommendation agent uses the information learned by the learner agent to recommend top-N course content to a learner based on their preferences, adaptive difficulty levels, behavior, knowledge level, etc.
The proposed DRR framework for personalized learning and top-N course recommendation can be seen in Fig. 2 . The environment is designed by learners and course material and the roles of the agents are handled by recommendation algorithms. Here, the state is shown as a feature depiction for learners, and action is defined as a feature depiction of course content. When a student wants a course, the agents are given a state representation (i.e., learner's features) and a collection of action representations (i.e., the course competitors' features). The agents will choose the best course of action (e.g., recommend a list of course content to a learner) and reward the learner with feedback. The reward is made up of click labels and an estimate of the learner's activity level. All these recommendations and feedback reports will be saved in the agents' database (memory). The agent will use the data in memory to update its recommendation algorithm at every iteration. This problem can be formulated as follows:
Agent \(\left(\mathcal{A}{\varvec{g}}\right)\) : A program (algorithm) that decides what to display next in a collection of MOOC.
Environment \(\left({\varvec{E}}\right)\) : The learning framework.
Action \(\left(\mathcal{A}\right)\) : action being the next suitable course to be recommended or recommending a new course. Or it is a rating score vector. The action is a perfect course content that the learner would enjoy studying/reading.
State \(\left(\mathcal{S}\right)\) : A learner's interaction features are depicted as a state. In other words, it is considered as the positive interaction records of the learners. The state-value \({\mathbbm{v}} \left(\mathcal{S}\right)\) can be defined to evaluate the goodness of the current state as shown in Eq. ( 1 ).
State Transitions \({\mathcal{P}}({s}_{\mathcal{T}}+1 \left| {s}_{\mathcal{T}}, {\partial }_{\mathcal{T}}\right)\) : The state is modelled as a depiction of a learner's interactions history. As a result, once the learner's response has been gathered, the state transition can be determined at an action \(\partial\) in state \(\mathcal{S}\) at time \(\mathcal{T}\) to \({s}_{\mathcal{T}+1}\) at \(\mathcal{T}+1\) , as follows in Eq. ( 2 ).
Reward \({\mathcal{R}}\left({s}_{\mathcal{T}+1}+{\partial }_{\mathcal{T}}, {s}_{\mathcal{T}}, {\partial }_{\mathcal{T}}\right)\) : to be the user satisfaction/ conversion or review. For instance, the reward will be positive if the learner is satisfied with the course and rates it as approximately 4; the reward is more positive if the learner rated the course as 5; if the learner becomes bored, the result will be negative and course rating can be as below 2. Furthermore, in response to the recommendation based on the action and the learner states s, the learner will give their input by clicking, not clicking, rating the course, etc. Following the learner's response, the recommender is given an immediate reward, R(s, a). The immediate reward for the agent transmitting from \(s {\text{to}} {s}_{\mathcal{T}+1}\) is generated by this function. The expectation of cumulative future reward will be maximized via the following formula (Eq. 3 ).
Discount rate ϒ : used to calculate the current value of future rewards or long-term rewards, which ranges from 0 to 1.
For example, Fig. 1 shows recommendation processes in RL, during the student-agent interaction, the model will assign an agent to each student. An agent will recommend the content to the student and the student will give feedback to the agent.
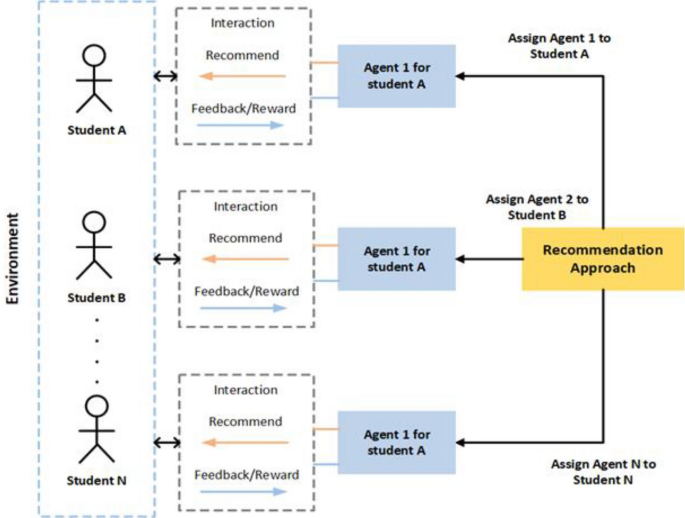
Student-agent interaction for top-N course RS.
Proposed DRR model
This study aims to propose an effective top-N course recommendation framework for RL-based RS. The suggested framework employs many strategies to construct a reliable RS model. To achieve this goal, the interactive RS problem will be formulated using a DRL recommendation framework such as an Actor-Critic named DRR. In this framework, various state representation schemes for training the recommendation policy (actor) and value function (critic) are briefly discussed. The proposed DRR framework for top-N course recommendation can be seen in Fig. 2 . Moreover, to remove the noise in the MOOC data, pre-processing is first carried out. The required features are then extracted utilizing the improved TFIDF of feature extraction. To provide robust course recommendations, the DRR-based recommendation agents (Actor-Critic) are designed as follows.
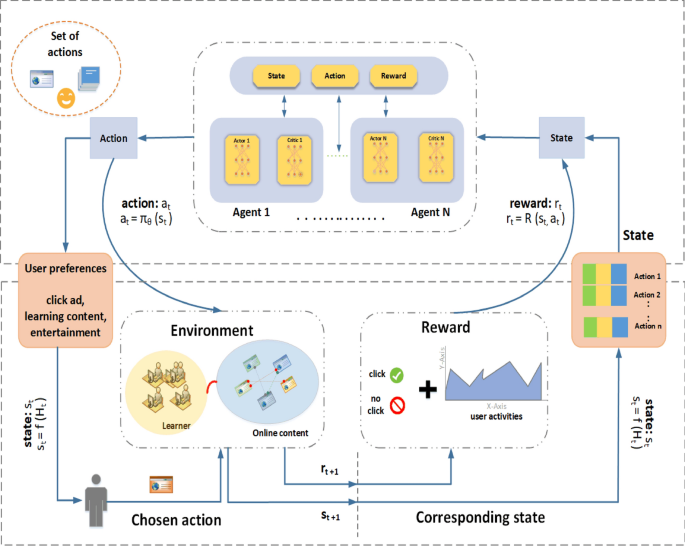
A proposed adaptable and personalized framework for top-N course recommendations in online learning.
Actor-Critic is a DRL-based model that combines elements of value-based and policy-based mechanisms. In this instance, the Actor oversees formulating recommendations (policy-based), and the Critic assesses the effectiveness of the recommendations (value-based). Sequential recommendations can be made using the Actor-Critic model, in which the Actor comes up with the suggestions and the Critic evaluates them. To enhance the suggestions over time, the model is updated using the value function and the policy gradient.
To train the model, we used 100 K Coursera course review data consisting of learners’ reviews for a specific course and their ratings. This data is then input into the Actor Network, which is responsible for making decisions about what course content to recommend next. The actor network generates an ideal course embedding, representing the desired content. To find similarities, these embeddings can be compared to those of other courses. The course material that best suits the student’s needs can then be suggested to them. The Critic Network is essential to this process. It evaluates each decision the Actor Network makes and directs it to find any possible flaws. While the Critic Network helps forecast how learners will behave in the future. For example, if RS recommends that a student read a course material or complete an assignment, and the student complies, they will instantly receive 100. But the student might get bored or want to use social media instead, which would result in a -100 penalty. This is where the Q-Network (Critic) becomes crucial: considering all potential future actions is essential.
Proposed architecture
The actor and critic are the two layers that design the network. Here the Critic uses a Q-learning mechanism and is primarily concerned with rewards and the Actor focuses on learning policies, which are the probabilities of choosing the next action. The process begins with course embeddings being directed into the Actor’s state representation module, where these embeddings are transformed into encoded representations. Subsequently, an action is formulated in the form of a vector. This action is then combined with course embeddings, resulting in a concatenated input that is fed into the Critic Module. The main objective of the Critic architecture is to analyze and predict the potential of the upcoming reward. First, a substantial amount of course embeddings are provided to the actor’s state representation module, where they are encoded. Then, a decision is taken (resulting in an action represented by a vector). The action is passed into the Critic Module along with item embeddings to estimate how excellent the reward will be.
In conclusion, this framework enhances the recommendation process by using a two-layered architecture. The Actor layer is responsible for learning the likelihood of selecting specific actions, while the Critic layer is dedicated to assessing the potential rewards. Through a combination of reward evaluations and learned policies, the proposed framework maximizes the decision-making process for personalized recommendations.
The design of the proposed framework can make an adaptable and personalized class environment more valuable. With the help of recommendations, the learner can benefit from more effective learning, and the system can benefit from more effective advertising.
Experiments
This section outlines the experimental procedure used in this study to evaluate the effectiveness of the DRR model (Actor-Critic), including the dataset description, parameter setting, evaluation metrics, and findings.
First, the dataset is introduced and employed in the experiments. Then, the baseline models are discussed and compared with the proposed models and the metrics that are adopted for evaluation.
Data description
We first briefly introduce the MOOC dataset that is applied:
100 K Coursera’s Course Reviews : to train the proposed models, a 100 K Coursera’s Course Reviews dataset was extracted from the publicly accessible Kaggle repository 41 . This data contains around 120k reviews from learners on Coursera’s MOOC for multiple courses. Each student has an integer rate between [1, 5]. The courses are rated based on the learner’s sentiments, learning style, preferences, competency, and adaptive difficulty levels, the student may have. Table 1 represents an example of the course review dataset that is rated based on these factors.
Data preprocessing : The dataset is pre-processed as follows for data preparation: precisely, we limit each review to 300 words (Fig. 3 ). The datasets are filtered to remove any courses with blank course descriptions. Figure 4 shows the distribution of token counts in review Lengths
Word embedding-based feature : it involves employing the Glove technique to represent text data (posts, reviews, etc.) as real-valued N-dimensional vectors in a pre-defined vector space. Glove is a technique for unsupervised learning that extracts explicit data on word-to-word co-occurrence from text corpora 42 , 43 . Every review is converted into corresponding labels (numerical values) using the Glove embedding model for creating the vocabulary. These numerical values are used as input for the machine learning and advanced DRL will help to better understand the learners’ attitudes, behavior, and actions during the recommendations.
Data splitting : For training and testing purposes, we divided the dataset in an 80:20 ratio for the training and testing model, respectively.
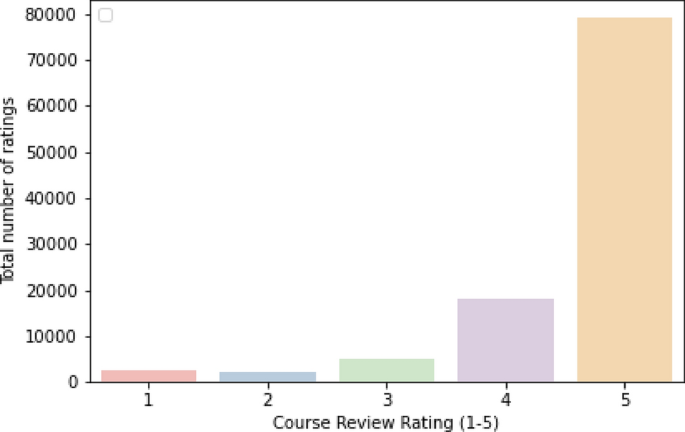
Distribution of Coursera course review into five ratings; rate 5 has a total of 79,173 ratings;4 (18,054); 3 (5071); 1 (2469) and 2 (2251).
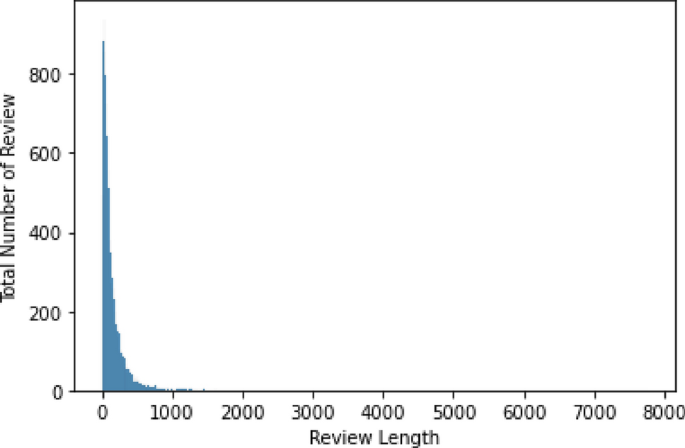
Distribution of token counts in review lengths.
Baseline models
To assess the accuracy of the proposed DRL models, we leveraged various baseline models which include a rating-based method matrix factorization (MF), multi-layer perceptron (MLP), SVD++, non-negative matrix factorization (NMF), and neural collaborative filtering (NCF). A detailed explanation of the baseline models is as follows:
MLP 44 the recommendation probability is produced using an MLP on a pair of user and course embeddings.
MF 45 is the commonly accepted baseline technique for CF-based RS. The recommendation algorithm is entirely rating-based and uses just rating data. In order to precisely examine the effects of using textual materials for RS, we employ this baseline methodology.
NMF 46 represents a set of algorithms in the domain of multivariate analysis and linear algebra. By breaking a matrix down into non-negative factors, using NMF in RS enables better interpretability of the resulting components. When dealing with data like user-item interactions, where the lack of negativity is consistent with the underlying dynamics, this strategy is particularly helpful. This approach enables RS to efficiently identify patterns and latent features in the data, resulting in recommendations that are more precise and insightful.
SVD++ 47 is an extension of SVD considering implicit ratings such as learner and course bias. It adds a factor vector for each course, and these course factors are used to define the features of the course, regardless of whether it has been assessed. This introduces the implicit feedback information based on SVD.
NCF 44 is a hybrid approach that integrates matrix factorization and MLP features for simulating learner-course latent frameworks, and it learns the likelihood of proposing target courses to associated learners.
DQN 48 is a well-liked method for sequential decision-making problems. DQN can be used in RS to provide sequential recommendations when the sequence of the course is important. The suggestion of the next course is the action in this scenario, and the state could be the learners’ previous interactions or the current context. The suggestions are based on the actions with the greatest Q-values determined by the DQN model, which learns a Q-value function to predict the expected reward for each action.
Parameter settings
A final dense layer with just one node is utilized to forecast the reward for the critic. The critic learns at a rate of 0.001, whereas the actor learns at a rate of 0.0001. Only 0.1% of the actors and critics are updated when the network is upgraded. We designed learning_rate = 0.001, γ = 0.99 (discount factor), epsilon = 0.1 (epsilon-greedy exploration factor). Based on the exploration probability, a student decides whether to explore or exploit. A course is picked at random if it opts to explore (based on a random number). Alternatively, depending on the Q-values (action probabilities), the actor framework estimates the probability of choosing each course. Moreover, a reward is determined for the student, following the course they selected and their interests. A reward also calculates whether the selected course aligns with the student’s interests. The proposed DRR model is subjected to rigorous training over 1000 episodes. Then, for each episode, a random student is chosen from the list of students.
Evaluation metrics
The major evaluation metrics, including Hit Ratio (HR@N), Normalized Discounted Cumulative Gain (NDCG@N), recall@N, and precision@N 28 , 49 are employed for evaluating the quality of recommendations. Where precision@N is the proportion of recommended items in the top-N set that are relevant (Eq. 4 ); HR@N is a recall-based metric that measures the percentage of the ground truth instances that are successfully recommended in top-N as determined in Eq. ( 5 ); recall@N denotes the percentage of preferred items in the top-N recommended list as denoted in Eq. ( 6 ); and NDCG@N assesses the position of a successful match in the recommendation list, assigning higher scores to hits at superior ranks within the list. The mathematical modelling of NDCG@N can be seen in Eq. ( 7 ). The top-N recommendations list is set as 5, 10, 15, and 20. We use the offline test method described in 49 , 50 to evaluate the proposed models.
It can be determined that the model score for a given course j for student i is computed as the inner product.
where \({Hits}_{u}@N\) stands for the number of courses in the top-N recommendation list for the u-th student pertaining to the testing set, |.| stands for the size of a set, and GT refers to the set of ground truths for all students in the testing set.
where R shows the total number of relevant courses for the given input. Precision@N and recall@N are binary metrics that can only be calculated for courses that a student has actually given a rating. The precision@N metric counts the proportion of generated recommendations that the student actually chose. Recall@N, on the other hand, gauges the proportion of all pertinent courses that the proposed model recommended.
where \(re{l}_{i}u\) is the graded relevance of the recommendation result listed at position i to the u-th student, and IDCGk@N represents the ideal discounted cumulative gain obtained through the best top-N recommendation list for the u-th student.
Experimental results, and analysis
Table 2 lists the overall performance of all the baselines and proposed methods. The proposed DRR model performs better than the comparison baselines in HR@10. The learner-to-content-based CF models, including MLP, NMF, MF, NCF, and SVD++ perform the worst among all the methods because, in the dataset, most of the learners participated in the review and only enrolled in a few courses. Thus, the embeddings for many students can not be sufficiently inferred from the sparse data. MF and NMF perform the worst of all the course-to-course-based CF algorithms because they consider all previously enrolled courses equally, which limits their ability to capture preferences. The effects of various previous courses are distinguished by MLP, NCF, and SVD++ by giving them various attention coefficients. When students enrol in numerous different courses, the effects of the useful courses in the past will be diminished. The proposed techniques, DRR, and DQN perform best because they compel the noisy courses to be removed.
Among the various CF models that are designed based on course-to-course interactions, MF and NMF tend to exhibit weaker performance compared to other models. This is due to their uniform treatment of all historically taken courses, which restricts their ability to accurately represent user preferences. On the other hand, models like MLP, NCF, and SVD++ with explicit feedback excel in distinguishing the impacts of different previously registered courses. They achieve this by assigning distinct attention coefficients to each course, thereby enhancing their predictive accuracy.
However, a challenge arises when students have enrolled in a multitude of diverse courses, causing less relevant courses to overshadow the effects of valuable ones from the past. To address this issue, the proposed approach DRR, as well as the DQN model, prove to be the most effective performers. These models adopt a strategy of deliberately eliminating irrelevant or noisy courses from consideration. This strategic course selection significantly enhances the overall predictive capability of these models and consequently leads to their superior performance.
Table 3 reports a comprehensive overview of the performance of both the baseline and the proposed approaches, where the best results are marked in bold type. Notably, the DRR model proposed in this study demonstrates superior performance compared to the baseline methods, specifically excelling in the recall@10 metric. It is important to highlight that the learner-to-course-based CF approaches, including MLP, NMF, MF, NCF, and SVD++, exhibit suboptimal performance. This is attributed to the dataset’s characteristics where most learners engage in providing reviews but enrol in only a limited number of courses. Consequently, the sparse data hinders the accurate inference of embeddings for many students.
Among the course-to-course-based CF models, both MF and NMF exhibit relatively weaker performance compared to their counterparts. This can be attributed to their uniform treatment of historically enrolled courses, limiting their ability to effectively represent preferences. In contrast, MLP, NCF, and SVD++ stand out by differentiating the impact of distinct historical courses through varying attention coefficients. Nevertheless, when users are enrolled in a diverse array of courses, the presence of less useful courses can dilute the effects of more valuable ones in their history.
Graphical representations of the experimental outcomes are depicted in Figs. 5 , 6 , 7 and 8 . Figure 5 underscores the superior performance of the DRR model on the MOOC dataset in comparison to the baseline models, particularly evident in its higher HR@N metric. This highlights the model’s effectiveness. Besides, a comparison with the DQN model, which employs straightforward fully connected neural networks for state modeling, reveals a significant performance gap, showcasing the clear advantages of the proposed DRR model. The effectiveness of the DRR model is consistently demonstrated across multiple metrics, including NDCG@N, recall@N, and precision@N. These contrasts (NDCG@N, recall@N, and precision@N) are intensely presented in Figs. 6 , 7 and 8 , respectively.
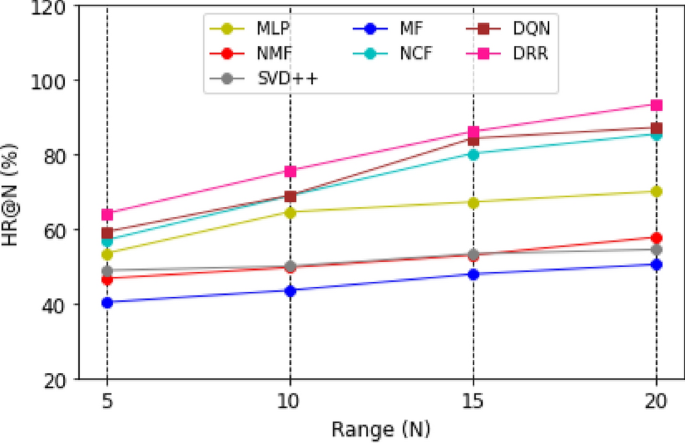
Evaluation index HR (%) for each model with the top (N = 5, 10, 15, 20).
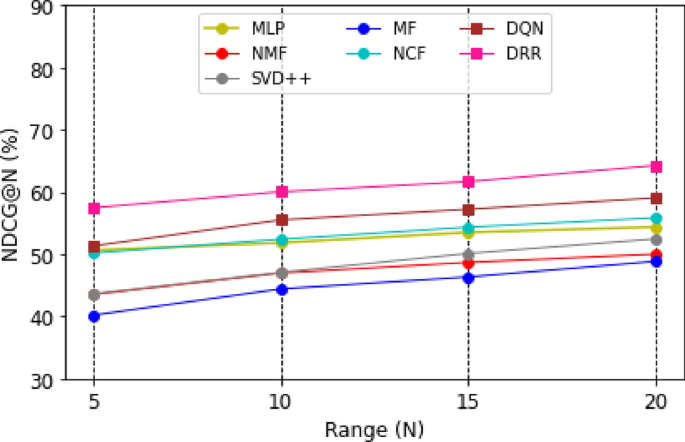
Evaluation index NDCG (%) for each model with top (N = 5, 10, 15, 20).
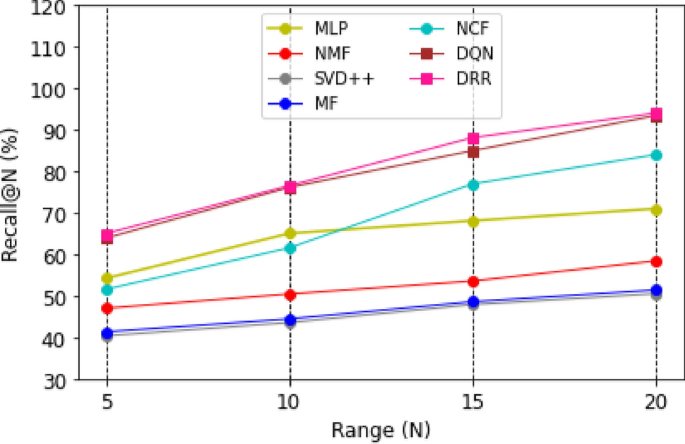
Evaluation index recall (%) for each model with a top (N = 5, 10, 15, 20).
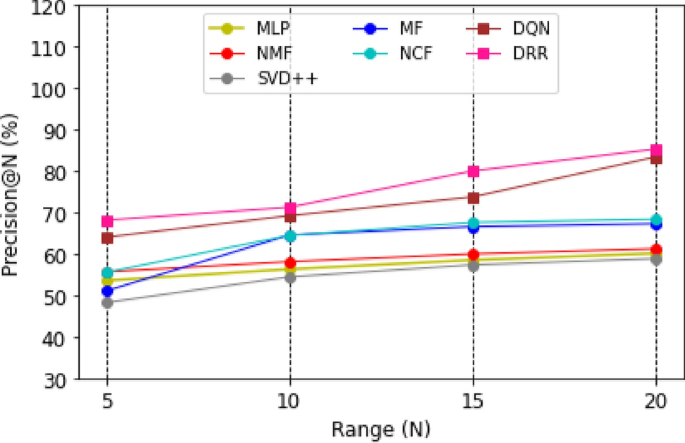
Evaluation index precision (%) for each model with the top (N = 5, 10, 15, 20).
Three conclusions can be drawn from the assessments. (1) Personalized adaptation and long-term planning are features of the suggested solutions that both traditional approaches and the NCF approach based on deep learning disregard. (2) Unlike DQN, which merely concatenates fully linked layers, the proposed state representation module effectively captures the complicated dynamic interactions between students and courses. This prevents information loss and subpar learning outcomes.
Likewise, compared with NCF, MF, MLP, NMF, and SVD + + , it can be found that the DRR performs the best in terms of HR@N (Fig. 5 ), NDCG@N (Fig. 6 ), recall@N (Fig. 7 ), and precision@N (Fig. 8 ). The other performs less favorably. The reasons are as follows: the DRR outperforms because it captures not only the interactions between the learners’ historical courses but also the learner’s preferences for them. The experimental results demonstrate that the suggested DRR model has better recommendation performance than the other six models.
DRL-based methods use neural networks to approximate the value function and can handle high-dimensional and complex state spaces. These methods have been successfully applied to various problems and have demonstrated significant improvement in performance over traditional tabular CF-based RS methods. The integration of DRL and RS points towards a promising avenue for the development of more advanced and personalized recommendation systems.
Conclusions
The significant growth in online learning platforms in recent years has revolutionized global education accessibility. Even though MOOCs let students complete their coursework, the abundance of course content available on the internet can make it challenging for certain learners to understand, especially those with low skills and cognitive abilities. The proposed solution to this problem is to use an adaptable and personalized RS integrated with an adaptive e-learning framework. Subsequently, a top-N MOOC course recommendation framework based on the DRR model is designed, trained, and evaluated using an Actor-Critic simulator. Through multiple iterations, a robust framework for training these models has been built, enabling the effective deployment of both DQN and Actor-Critic methodologies. The commonly used measures HR@N, Recall@k, Precision@k, and NDCG@N are used to evaluate each approach. The findings of this study demonstrate how new methods based on DRL can be used to recommend top-N courses in MOCC. Both DQN and DRR can reach the goal by suggesting the top-N courses, although DRR (Actor-Critic) obtains better performance and recommendations are more personalized to the learners’ preferences and interests. Our suggested framework, which is based on DRL for top-N course recommendations, is intended to act as a strong baseline for RSs in MOOCs. This framework has been rigorously evaluated within practical simulated environments, solidifying its contribution to the online learning domain.
Implications
The DRR framework is designed to intelligently assess students’ acceptance of technology and well-being while recommending relevant learning content. It enables continuous learning by adapting recommended courses to changing learner interests, ensuring an adaptable and personalized system. This streamlined learning process benefits both students and teachers. The proposed adaptable and personalized DRR model has the potential to significantly impact e-learning technology, improving the design and execution of course RS. In order to support students’ interests and preferences, it also automates administrative tasks and helps teachers better understand the unique needs of each student.
In addition, the enhanced adaptable and personalized RS framework for introducing online course content is not limited to academic course recommendations. It can also be employed for efficient information retrieval on e-commerce and news websites, providing suggestions for things like headlines on news sites or products on Amazon, Ali Express, etc.
Limitations and future work
One of the foremost challenges encountered during the exploration of this subject was the identification of suitable learning environments. The availability of open-source environments was limited. In certain RS, the signal for rewards is weak. As a result, the agent might not get paid for many actions. The agent may find it challenging to understand the best course of action as a result.
The study has many motivations for future research developments that we will explore can be: in the future, we aim to extend this study by incorporating multiple MOOC datasets encompassing a larger number of students and courses. The work can be enhanced further by using other DRL techniques like Double DQN, Dueling DQN, and Deep Deterministic Policy Gradient (DDPG). We also aim to evaluate the effectiveness of various approaches for top-N courses and quantify their effects on recommendation quality. This comprehensive assessment will be carried out through experiments conducted in both offline and simulated online evaluation contexts.
Data availability
The datasets generated and/or analysed during the current study are available in the Kaggle repository https://www.kaggle.com/datasets/septa97/100k-courseras-course-reviews-dataset .
Salta, K., Paschalidou, K., Tsetseri, M. & Koulougliotis, D. Shift from a traditional to a distance learning environment during the COVID-19 pandemic. Sci. Educ. 31 (1), 93–122. https://doi.org/10.1007/s11191-021-00234-x (2022).
Article Google Scholar
Shin, J. & Bulut, O. Building an intelligent recommendation system for personalized test scheduling in computerized assessments: A reinforcement learning approach. Behav. Res. Methods. 54 (1), 216–232. https://doi.org/10.3758/s13428-021-01602-9 (2022).
Article PubMed Google Scholar
Amin, S. et al. Developing a personalized E-learning and MOOC recommender system in IoT-enabled smart education. IEEE Access. 1 , 1. https://doi.org/10.1109/ACCESS.2023.3336676 (2023).
Mehdi, M., Trafford, A., & Behrouz, C. F. Reinforcement learning based recommender systems: A survey. arXiv:210106286v1. Published online 1–37 (2021).
Wayesa, F., Leranso, M., Asefa, G. & Kedir, A. Pattern-based hybrid book recommendation system using semantic relationships. Sci. Rep. 13 (1), 3693. https://doi.org/10.1038/s41598-023-30987-0 (2023).
Article ADS CAS PubMed PubMed Central Google Scholar
Ali, S. et al. Enabling recommendation system architecture in virtualized environment for e-learning. Egypt Inf. J. 23 (1), 33–45 (2022).
Google Scholar
Shahbazi, Z. Agent-based recommendation in E-learning environment using knowledge discovery and machine learning approaches. Mathematics. 10 (7), 1192 (2022).
Amin, S. et al. Smart E-learning framework for personalized adaptive learning and sequential path recommendations using reinforcement learning. IEEE Access. 11 , 89769–89790. https://doi.org/10.1109/ACCESS.2023.3305584 (2023).
Chen, X., Yao, L., McAuley, J., Zhou, G. & Wang, X. Deep reinforcement learning in recommender systems: A survey and new perspectives. Knowl. Based Syst. 264 , 110335. https://doi.org/10.1016/j.knosys.2023.110335 (2023).
Nguyen, T. T., Nguyen, N. D. & Nahavandi, S. Deep reinforcement learning for multiagent systems: A review of challenges, solutions, and applications. IEEE Trans. Cybern. 50 (9), 3826–3839. https://doi.org/10.1109/TCYB.2020.2977374 (2020).
Ahmadian, M., Ahmadian, S. & Ahmadi, M. RDERL: Reliable deep ensemble reinforcement learning-based recommender system. Knowl. Based Syst. 263 , 110289. https://doi.org/10.1016/j.knosys.2023.110289 (2023).
Xin, X. et al. Online reinforcement learning multiplayer non-zero sum games of continuous-time Markov jump linear systems. Appl. Math. Comput. 412 (1), 126537. https://doi.org/10.1016/j.amc.2021.126537 (2022).
Article MathSciNet Google Scholar
Taghian, M., Asadi, A. & Safabakhsh, R. Learning financial asset-specific trading rules via deep reinforcement learning. Expert. Syst. Appl. 195 (6), 116523. https://doi.org/10.1016/j.eswa.2022.116523 (2022).
Ibarz, J. et al. How to train your robot with deep reinforcement learning: Lessons we have learned. Int. J. Rob. Res. 40 (4–5), 698–721 (2021).
Zhang, J., Yan, Q., Zhu, X. & Yu, K. Smart industrial IoT empowered crowd sensing for safety monitoring in coal mine. Digit Commun. Netw. 9 (2), 296–305 (2023).
Aboutorab, H., Hussain, O. K., Saberi, M. & Hussain, F. K. A reinforcement learning-based framework for disruption risk identification in supply chains. Futur Gen. Comput. Syst. 126 (1), 110–122. https://doi.org/10.1016/j.future.2021.08.004 (2022).
Dake, D. K., Gadze, J. D., Klogo, G. S. & Nunoo-mensah, H. Multi-agent reinforcement learning framework in SDN-IoT for transient load detection and prevention. Technologies. 9 (44), 1–22 (2021).
Jiang, B., Zhao, Y., Dong, J. & Hu, J. Analysis of the influence of trust in opposing opinions: An inclusiveness-degree based Signed Deffuant–Weisbush model. Infor. Fusion , 104 , 102173. https://doi.org/10.1016/j.inffus.2023.102173 (2024)
Tan, L., Shi, N., Yu, K., Aloqaily, M. & Jararweh, Y. A blockchain-empowered access control framework for smart devices in green internet of things. ACM Trans. Internet Technol. 21 (3), 1–20 (2021).
Zhang, S. et al. Drl-based partial offloading for maximizing sum computation rate of wireless powered mobile edge computing network. IEEE Trans. Wirel. Commun. 21 (12), 10934–10948 (2022).
Yuyan, Z., Xiayao, S., & Yong, L. A novel movie recommendation system based on deep reinforcement learning with prioritized experience replay. In 2019 IEEE 19th International Conference on Communication Technology (ICCT) . 1496–1500. https://doi.org/10.1109/ICCT46805.2019.8947012 (2019).
Zheng, G., Zhang, F., Zheng, Z., et al. DRN: A deep reinforcement learning framework for news recommendation. In Proceedings of the 2018 World Wide Web Conference, Lyon, France. ACM, New York, NY, USA . 167–176 (2018).
Oh, S. H., Park, J., Lee, S. J., Kang, S. & Mo, J. Reinforcement learning-based expanded personalized diabetes treatment recommendation using South Korean electronic health records. Expert Syst. Appl. 206 , 117932. https://doi.org/10.1016/j.eswa.2022.117932 (2022).
Jha, R. K., Bag, S., Koley, D., Bojja, G. R. & Barman, S. An appropriate and cost-effective hospital recommender system for a patient of rural area using deep reinforcement learning. Intell. Syst. Appl. 18 , 200218. https://doi.org/10.1016/j.iswa.2023.200218 (2023).
Dong, J., Hu, J., Zhao, Y. & Peng, Y. Opinion formation analysis for Expressed and Private Opinions (EPOs) models: Reasoning private opinions from behaviors in group decision-making systems. Expert Syst. Appl. 236 , 121292. https://doi.org/10.1016/j.eswa.2023.121292 (2024).
Tzeng, J.-W., Huang, N.-F., Chuang, A.-C., Huang, T.-W. & Chang, H.-Y. Massive open online course recommendation system based on a reinforcement learning algorithm. Neural Comput. Appl. https://doi.org/10.1007/s00521-023-08686-8 (2023).
Lyu, W., Huang, Y. & Liu, J. The multifaceted influence of multidisciplinary background on placement and academic progression of faculty. Humanit. Soc. Sci. Commun. 11 (1), 350. https://doi.org/10.1057/s41599-024-02818-8 (2024).
Huang, L. et al. A deep reinforcement learning based long-term recommender system. Knowl. Based Syst. 213 (1), 106706. https://doi.org/10.1016/j.knosys.2020.106706 (2021).
Lin, Y. et al. Hierarchical reinforcement learning with dynamic recurrent mechanism for course recommendation. Knowl. Based Syst. 244 , 108546 (2022).
Vedavathi, N. & Anil Kumar, K. M. E-learning course recommendation based on sentiment analysis using hybrid Elman similarity. Knowl. Based Syst. 259 , 110086. https://doi.org/10.1016/j.knosys.2022.110086 (2023).
Liu, Y., Dong, Y., Yin, C., Chen, C. & Jia, R. A personalized course recommendation model integrating multi-granularity sessions and multi-type interests. Educ. Inf. Technol. https://doi.org/10.1007/s10639-023-12028-5 (2023).
Zhu, Y. et al. Heterogeneous teaching evaluation network based offline course recommendation with graph learning and tensor factorization. Neurocomputing. 415 , 84–95 (2020).
Campos, R., dos Santos, R. P. & Oliveira, J. Providing recommendations for communities of learners in MOOCs ecosystems. Expert Syst. Appl. 205 , 117510. https://doi.org/10.1016/j.eswa.2022.117510 (2022).
Nilashi, M. et al. Knowledge discovery for course choice decision in Massive Open Online Courses using machine learning approaches. Expert Syst. Appl. 199 , 117092. https://doi.org/10.1016/j.eswa.2022.117092 (2022).
Symeonidis, P. & Malakoudis, D. Multi-modal matrix factorization with side information for recommending massive open online courses. Expert Syst. Appl. 118 (15), 261–271 (2019).
Boratto, L., Fenu, G. & Marras, M. The Effect of Algorithmic Bias on Recommender Systems for Massive Open Online Courses. In Advances in Information Retrieval (eds Azzopardi, L. et al. ) 457–472 (Springer International Publishing, 2019).
Chapter Google Scholar
Lin, Y. et al. Adaptive course recommendation in MOOCs. Knowl. Based Syst. 224 , 107085 (2021).
Wang, J., Xie, H., Wang, F. L., Lee, L.-K. & Au, O. T. S. Top-N personalized recommendation with graph neural networks in MOOCs. Comput. Educ. Artif. Intell. 2 , 100010. https://doi.org/10.1016/j.caeai.2021.100010 (2021).
Article CAS Google Scholar
Lin, Y. et al. Context-aware reinforcement learning for course recommendation. Appl. Soft Comput. 125 , 109189. https://doi.org/10.1016/j.asoc.2022.109189 (2022).
Madani, Y., Ezzikouri, H., Erritali, M. & Hssina, B. Finding optimal pedagogical content in an adaptive e-learning platform using a new recommendation approach and reinforcement learning. J. Ambient Intell. Hum. Comput. 11 (10), 3921–3936. https://doi.org/10.1007/s12652-019-01627-1 (2020).
Jan charles maghirang adona. 100K Coursera’s Course Reviews Dataset. Accessed July 31, 2023. https://www.kaggle.com/datasets/septa97/100k-courseras-course-reviews-dataset .
Pennington, J., Socher, R., Manning, C. & Glove: Global Vectors for Word Representation. Proc,. Conf Empir Methods Nat Lang Process (EMNLP), Doha, Qatar. Published online 2014 , 1532–1543. https://doi.org/10.3115/v1/D14-1162 (2014).
Amin, S. et al. Detecting information on the spread of dengue on twitter using artificial neural networks. C Mater. Contin. 67 (1), 1317–1332. https://doi.org/10.32604/cmc.2021.014733 (2021).
He, X., Liao, L., Zhang, H., Nie, L., Hu, X., & Chua, T.-S. Neural collaborative filtering. In Proceedings of the 26th International Conference on World Wide Web . 173–182 (2017).
Zhang, H., Luo, F., Wu, J., He, X. & Li, Y. LightFR: Lightweight federated recommendation with privacy-preserving matrix factorization. ACM Trans. Inf. Syst. 41 (4), 1–28 (2023).
Muepu, D. M., Watanobe, Y., & Rahman, M. M. Collaborative filtering based on non-negative matrix factorization for programming problem recommendation. In International Conference on Industrial, Engineering and Other Applications of Applied Intelligent Systems . pp. 241–250 (Springer, 2023).
Liu, Y. & Zhang, W. Design and simulation of precision marketing recommendation system based on the NSSVD++ algorithm. Neural Comput. Appl. https://doi.org/10.1007/s00521-023-08302-9 (2023).
Article PubMed PubMed Central Google Scholar
Zhao, X., Zhang, L., Ding, Z., Xia, L., Tang, J., & Yin, D. Recommendations with negative feedback via pairwise deep reinforcement learning. In Proceedings of the 24th ACM SIGKDD International Conference on Knowledge Discovery & Data Mining . Pp. 1040–1048 (2018).
Liu, F. et al. State representation modeling for deep reinforcement learning based recommendation. Knowl. Based Syst. 205 , 106170. https://doi.org/10.1016/j.knosys.2020.106170 (2020).
Cao, B., Zhao, J., Lv, Z. & Yang, P. Diversified personalized recommendation optimization based on mobile data. In IEEE transactions on intelligent transportation systems 22 (4), 2133–2139. https://doi.org/10.1109/TITS.2020.3040909 (2021).
Download references
Acknowledgements
The authors acknowledge Project Number HU23K17736, Hosei University, Japan.
Author information
Authors and affiliations.
Institute of Computing, Kohat University of Science and Technology (KUST), Kohat, 26000, Pakistan
Samina Amin & M. Irfan Uddin
College of Computer Science and Engineering, University of Jeddah, Jeddah, Saudi Arabia
Ala Abdulsalam Alarood
Institute of Numerical Sciences, Kohat University of Science and Technology (KUST), Kohat, 26000, Pakistan
Wali Khan Mashwani
Faculty of Computer Science and Engineering, University of Jeddah, Jeddah, Saudi Arabia
Ahmed Omar Alzahrani
College of Computing and Informatics, Saudi Electronic University, Makkah, Saudi Arabia
Hamdan Ahmed Alzahrani
You can also search for this author in PubMed Google Scholar
Contributions
Samina Amin: conceptualization, data collection, data pre-processing, development of the research methodology, writing the original draft. M. Irfan Uddin: Methodology, design, data analysis, result analysis, writing, review and editing of the manuscript. Ala Abdulsalam Alarood: components development, gather of relevant data and experiments, data visualization, graphical representation, writing, review and editing of the manuscript. Wali Khan Mashwani: conceptualization, literature review, writing, review and editing of the manuscript. Ahmed Omar Alzahrani: data collection, data organization, experimentation and data analysis, writing the original draft of the manuscript. Safa Habibullah: project supervision, methodology refinement, writing, review and editing of the manuscript.
Corresponding author
Correspondence to M. Irfan Uddin .
Ethics declarations
Competing interests.
The authors declare no competing interests.
Additional information
Publisher's note.
Springer Nature remains neutral with regard to jurisdictional claims in published maps and institutional affiliations.
Rights and permissions
Open Access This article is licensed under a Creative Commons Attribution 4.0 International License, which permits use, sharing, adaptation, distribution and reproduction in any medium or format, as long as you give appropriate credit to the original author(s) and the source, provide a link to the Creative Commons licence, and indicate if changes were made. The images or other third party material in this article are included in the article's Creative Commons licence, unless indicated otherwise in a credit line to the material. If material is not included in the article's Creative Commons licence and your intended use is not permitted by statutory regulation or exceeds the permitted use, you will need to obtain permission directly from the copyright holder. To view a copy of this licence, visit http://creativecommons.org/licenses/by/4.0/ .
Reprints and permissions
About this article
Cite this article.
Amin, S., Uddin, M.I., Alarood, A.A. et al. An adaptable and personalized framework for top-N course recommendations in online learning. Sci Rep 14 , 10382 (2024). https://doi.org/10.1038/s41598-024-56497-1
Download citation
Received : 27 September 2023
Accepted : 07 March 2024
Published : 06 May 2024
DOI : https://doi.org/10.1038/s41598-024-56497-1
Share this article
Anyone you share the following link with will be able to read this content:
Sorry, a shareable link is not currently available for this article.
Provided by the Springer Nature SharedIt content-sharing initiative
- Reinforcement learning
- Deep reinforcement learning
- Online learning
- Recommender system
By submitting a comment you agree to abide by our Terms and Community Guidelines . If you find something abusive or that does not comply with our terms or guidelines please flag it as inappropriate.
Quick links
- Explore articles by subject
- Guide to authors
- Editorial policies
Sign up for the Nature Briefing: AI and Robotics newsletter — what matters in AI and robotics research, free to your inbox weekly.

- Coronavirus Updates
- Education at MUSC
- Adult Patient Care
- Hollings Cancer Center
- Children's Health
Biomedical Research
- Research Matters Blog
- NIH Peer Review
NIH announces the Simplified Framework for Peer Review
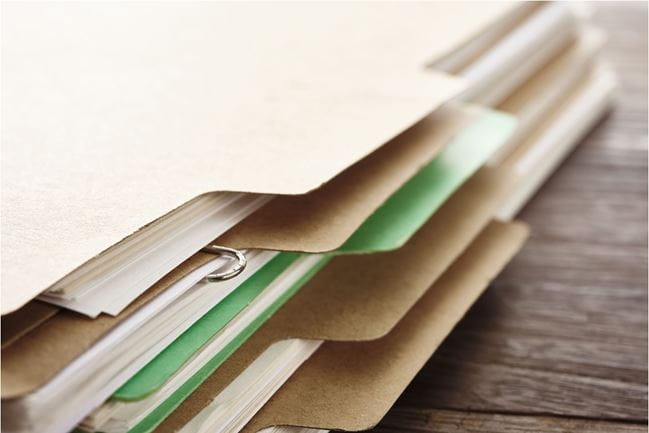
The mission of NIH is to seek fundamental knowledge about the nature and behavior of living systems and to apply that knowledge to enhance health, lengthen life, and reduce illness and disability . In support of this mission, Research Project Grant (RPG) applications to support biomedical and behavioral research are evaluated for scientific and technical merit through the NIH peer review system.
The Simplified Framework for NIH Peer Review initiative reorganizes the five regulatory criteria (Significance, Investigators, Innovation, Approach, Environment; 42 C.F.R. Part 52h.8 ) into three factors – two will receive numerical criterion scores and one will be evaluated for sufficiency. All three factors will be considered in determining the overall impact score. The reframing of the criteria serves to focus reviewers on three central questions they should be evaluating: 1) how important is the proposed research? 2) how rigorous and feasible are the methods? 3) do the investigators and institution have the expertise/resources necessary to carry out the project?
• Factor 1: Importance of the Research (Significance, Innovation), scored 1-9
• Factor 2: Rigor and Feasibility (Approach), scored 1-9
• Factor 3: Expertise and Resources (Investigator, Environment), to be evaluated with a selection from a drop-down menu
o Appropriate (no written explanation needed)
o Identify need for additional expertise and/or resources (requires reviewer to briefly address specific gaps in expertise or resources needed to carry out the project)
Simplifying Review of Research Project Grant Applications
NIH Activity Codes Affected by the Simplified Review Framework.
R01, R03, R15, R16, R21, R33, R34, R36, R61, RC1, RC2, RC4, RF1, RL1, RL2, U01, U34, U3R, UA5, UC1, UC2, UC4, UF1, UG3, UH2, UH3, UH5, (including the following phased awards: R21/R33, UH2/UH3, UG3/UH3, R61/R33).
Changes Coming to NIH Applications and Peer Review in 2025
• Simplified Review Framework for Most Research Project Grants (RPGs )
• Revisions to the NIH Fellowship Application and Review Process
• Updates to NRSA Training Grant Applications (under development)
• Updated Application Forms and Instructions
• Common Forms for Biographical Sketch and Current and Pending (Other) Support (coming soon)
Webinars, Notices, and Resources
Apr 17, 2024 - NIH Simplified Review Framework for Research Project Grants (RPG): Implementation and Impact on Funding Opportunities Webinar Recording & Resources
Nov 3, 2023 - NIH's Simplified Peer Review Framework for NIH Research Project Grant (RPG) Applications: for Applicants and Reviewers Webinar Recording & Resources
Oct 19, 2023 - Online Briefing on NIH’s Simplified Peer Review Framework for NIH Research Project Grant (RPG) Applications: for Applicants and Reviewers. See NOT-OD-24-010
Simplifying Review FAQs
Upcoming Webinars
Learn more and ask questions at the following upcoming webinars:
June 5, 2024 : Webinar on Updates to NIH Training Grant Applications (registration open)
September 19, 2024 : Webinar on Revisions to the Fellowship Application and Review Pro
Categories: NIH Policies , Research Education , Science Communications

IMAGES
VIDEO
COMMENTS
The model for research growth is a value chain that shows how themes ladder up toward organizational needs. • Page: Craft & Human Skills. The first step to using the framework with your team is to review the skills, define a shared understanding, and customize the list of skills relevant to your team context. • Tool: Skills & Themes Inventory.
The Research Skills Development (RSD) Framework, initiated by John Willison and Kerry O'Regan (Adelaide, AU), is a tool used to analyze and plan curriculum for the systematic development of students' research skills. The framework is descriptive, scalable, and useful for assignment, course or program planning purposes. ...
For educators Research Skill Development. to facilitate the explicit, coherent, incremental and cyclic development of the skills associated with researching, Framework. Creative Commons 4.0. problem solving, critical thinking and clinical reasoning. Scope for Student Autonomy.
The Research Skills Development Framework is useful as both a conceptual and planning tool as well as an assessment mechanism. It can be used to develop course and program activities that are appropriate for the level of research being conducted, it can help clarify learning outcomes, develop assessment measurements, and track student progress ...
Created by John Willison and Kerry O'Regan. www.rsd.edu.au Adapted for the US context by Sara K. Kuhn. "The seven-level Researcher Skill Development framework extends the RSD's original 5 levels of student autonomy to include the degree of autonomy required for a successful research career. It therefore addresses not only students, but also early, middle and late career researchers.
The quadrants can also be seen in relation to Willison and O'Regan's Research Skills Development (RSD) framework , which maps a development from early dependence and thinking to more complex work and enhanced autonomy for undergraduate researchers in particular.
Created by John Willison and Kerry O'Regan. www.rsd.edu.au Adapted for the US context by Sara K. Kuhn. "Research Skill Development (RSD) is about making explicit and coherent in regular university coursework the incremental attainment of research skills in a specific discipline. In the RSD, there are six facets of the research process, identified from the literature and modified according to ...
Research Skills Framework 2019 Literacies Novice At this level, individuals have developed an understanding and application of basic research skills. Intermediate At this level, individuals have progressed beyond the basics and have the research skills to find, evaluate, interpret, manage and use information for assignments using a variety of ...
The explicit and coherent development of student research skills has been an enduring educational concern at least since Dewey's (1908) call for student discovery learning and Vygotsky's work on ... Table 1: The 2018 version of the Research Skill Development framework (Willison & O'Regan, 2006/2018). The RSD framework was designed from ...
developed. Specify ESC issues. conducting and communicating. each relevant context. emerge broadly. Research Skill Development (RSD), a conceptual framework for Primary school to PhD, developed by John Willison and Kerry O'Regan ©, October, 2006/February, 2013, with much trialling by Eleanor Peirce and Mario Ricci.
Appendix 2: Work Skills Development framework ... A Research Skills Support Session, run in conjunction with staff from CLPD (Centre for Learning and Professional Development) will .
1. Introduction. The nature and effectiveness of research training including the place of generic skills development and academic writing, is currently much debated in high-income countries. 1, 2 Less is known about research training in low and middle-income countries in Asia, including the Philippines 3 It is clear that universities in the Philippines are 'at a critical stage in their ...
The Open Research Skills Framework provides an overview of open research practices and principles which can be applied to projects across all disciplines. This framework was created in partnership with practitioners and is intended for researchers of all levels, and across all disciplines.
Recognizing this need, business, political, and educational leaders are increasingly asking schools to teach students the competencies they will need to navigate a changing world—skills such as problem solving, critical thinking, and collaboration. Such skills are often referred to as "21st century skills," "soft skills," or "deeper ...
Research Skills Development (RSD) framework is about explicit and coherent development of research literacy and skills in a systematic manner from the undergraduate to postgraduate levels in University curricula. Integration of research literacy and skills in USP curricula is intended to produce graduates who are 'discerning consumers' of ...
A library research skills framework provides values, standards and skills for students at all levels (and educators) to work towards; it establishes learner expectations and allows for year-on-year development. A framework can offer a structure for up-to-date and consistent skills training for students. It also gives library and academic staff ...
Our online app based on the RDF is an approach to planning your professional development. Use it to identify strengths, plan actions, review achievements and create a portfolio of evidence. Find out how PGRs have benefitted from using RDF. Using the RDF for researchers. The RDF for researcher developers. For the supervisor, Research Director or PI.
The outcomes of this research contribute to the field of e-learning technology, guiding the design and implementation of course RSs, to facilitate personalized and relevant recommendations for ...
The mission of NIH is to seek fundamental knowledge about the nature and behavior of living systems and to apply that knowledge to enhance health, lengthen life, and reduce illness and disability.In support of this mission, Research Project Grant (RPG) applications to support biomedical and behavioral research are evaluated for scientific and technical merit through the NIH peer review system.