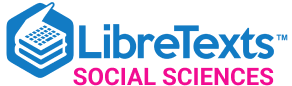
- school Campus Bookshelves
- menu_book Bookshelves
- perm_media Learning Objects
- login Login
- how_to_reg Request Instructor Account
- hub Instructor Commons

Margin Size
- Download Page (PDF)
- Download Full Book (PDF)
- Periodic Table
- Physics Constants
- Scientific Calculator
- Reference & Cite
- Tools expand_more
- Readability
selected template will load here
This action is not available.
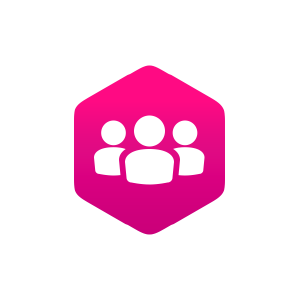
8.2: Relationship Formation
- Last updated
- Save as PDF
- Page ID 66589

- Jason S. Wrench, Narissra M. Punyanunt-Carter & Katherine S. Thweatt
- SUNY New Paltz & SUNY Oswego via OpenSUNY
\( \newcommand{\vecs}[1]{\overset { \scriptstyle \rightharpoonup} {\mathbf{#1}} } \)
\( \newcommand{\vecd}[1]{\overset{-\!-\!\rightharpoonup}{\vphantom{a}\smash {#1}}} \)
\( \newcommand{\id}{\mathrm{id}}\) \( \newcommand{\Span}{\mathrm{span}}\)
( \newcommand{\kernel}{\mathrm{null}\,}\) \( \newcommand{\range}{\mathrm{range}\,}\)
\( \newcommand{\RealPart}{\mathrm{Re}}\) \( \newcommand{\ImaginaryPart}{\mathrm{Im}}\)
\( \newcommand{\Argument}{\mathrm{Arg}}\) \( \newcommand{\norm}[1]{\| #1 \|}\)
\( \newcommand{\inner}[2]{\langle #1, #2 \rangle}\)
\( \newcommand{\Span}{\mathrm{span}}\)
\( \newcommand{\id}{\mathrm{id}}\)
\( \newcommand{\kernel}{\mathrm{null}\,}\)
\( \newcommand{\range}{\mathrm{range}\,}\)
\( \newcommand{\RealPart}{\mathrm{Re}}\)
\( \newcommand{\ImaginaryPart}{\mathrm{Im}}\)
\( \newcommand{\Argument}{\mathrm{Arg}}\)
\( \newcommand{\norm}[1]{\| #1 \|}\)
\( \newcommand{\Span}{\mathrm{span}}\) \( \newcommand{\AA}{\unicode[.8,0]{x212B}}\)
\( \newcommand{\vectorA}[1]{\vec{#1}} % arrow\)
\( \newcommand{\vectorAt}[1]{\vec{\text{#1}}} % arrow\)
\( \newcommand{\vectorB}[1]{\overset { \scriptstyle \rightharpoonup} {\mathbf{#1}} } \)
\( \newcommand{\vectorC}[1]{\textbf{#1}} \)
\( \newcommand{\vectorD}[1]{\overrightarrow{#1}} \)
\( \newcommand{\vectorDt}[1]{\overrightarrow{\text{#1}}} \)
\( \newcommand{\vectE}[1]{\overset{-\!-\!\rightharpoonup}{\vphantom{a}\smash{\mathbf {#1}}}} \)
Learning Outcomes
- Understand attraction.
- Ascertain reasons for attraction.
- Realize the different types of attraction.
Have you ever wondered why people pick certain relationships over others? We can’t pick our family members, although I know some people wish they could. We can, however, select who our friends and significant others are in our lives. Throughout our lives, we pick and select people that we build a connection to and have an attraction towards. We tend to avoid certain people who we don’t find attractive.
Understanding Attraction
Researchers have identified three primary types of attraction: physical, social, and task. Physical attraction refers to the degree to which you find another person aesthetically pleasing. What is deemed aesthetically pleasing can alter greatly from one culture to the next. We also know that pop culture can greatly define what is considered to be physically appealing from one era to the next. Think of the curvaceous ideal of Marilyn Monroe and Elizabeth Taylor in the 1950s as compared to the thin Halle Barry or Anne Hathaway. Although discussions of male physical attraction occur less often, they are equally impacted by pop culture. In the 1950s, you had solid men like Robert Mitchum and Marlon Brando as compared to the heavily muscled men of today like Joe Manganiello or Zac Efron.
The second type of attraction is social attraction , or the degree to which an individual sees another person as entertaining, intriguing, and fun to be around. We all have finite sources when it comes to the amount of time we have in a given day. We prefer to socialize with people that we think are fun. These people may entertain us or they may just fascinate us. No matter the reason, we find some people more socially desirable than others. Social attraction can also be a factor of power, for example, in situations where there are kids in the “in-group” and those that are not. In this case, those that are considered popular hold more power and are perceived as being more socially desirable to associate with. This relationship becomes problematic when these individuals decide to use this social desirability as a tool or weapon against others.
The final type of attraction is task attraction , or people we are attracted to because they possess specific knowledge and/or skills that help us accomplish specific goals. The first part of this definition requires that the target of task attraction possess specific knowledge and/or skills. Maybe you have a friend who is good with computers who will always fix your computer when something goes wrong. Maybe you have a friend who is good in math and can tutor you. Of course, the purpose of these relationships is to help you accomplish your own goals. In the first case, you have the goal of not having a broken down computer. In the second case, you have the goal of passing math. This is not to say that an individual may only be viewed as task attractive, but many relationships we form are because of task attraction in our lives.
Reasons for Attraction
Now that we’ve looked at the basics of what attraction is, let’s switch gears and talk about why we are attracted to each other. There are several reasons researchers have found for our attraction to others, including proximity, physicality, perceived gain, similarities and differences, and disclosure.
Physical Proximity
When you ask some people how they met their significant other, you will often hear proximity is a factor in how they met. Perhaps, they were taking the same class or their families went to the same grocery store. These common places create opportunities for others to meet and mingle. We are more likely to talk to people that we see frequently.
Physical Attractiveness
In day-to-day interactions, you are more likely to pay attention to someone you find more attractive than others. Research shows that males place more emphasis on physical attractiveness than females. 5 Appearance is very important at the beginning of the relationship.
Perceived Gain
This type of relationship might appear to be like an economic model and can be explained by exchange theory . 6 In other words, we will form relationships with people who can offer us rewards that outweigh the costs. Rewards are the things we want to acquire. They could be tangible (e.g., food, money, clothes) or intangible (support, admiration, status). Costs are undesirable things that we don’t want to expend a lot of energy to do. For instance, we don’t want to have to constantly nag the other person to call us or spend a lot of time arguing about past items. A good relationship will have fewer costs and more rewards. A bad relationship will have more costs and fewer rewards. Often, when people decide to stay or leave a relationship, they will consider the costs and rewards in the relationship.
Costs and rewards are not the only factors in a relationship. Partners also consider alternatives in the relationship. For instance, Becky and Alan have been together for a few years. Becky adores Alan and wants to marry him, but she feels that there are some problems in the relationship. Alan has a horrible temper; he is pessimistic; and he is critical of her. Becky has gained some weight, and Alan has said some hurtful things to her. Becky knows that every relationship will have issues. She doesn’t know whether to continue this relationship and take it further or if she should end it.
Her first alternative is called the comparison level (CL), which is the minimum standard that she is willing to tolerate. If Becky believes that it is ok for a person to say hurtful things to her or get angry, then Alan is meeting or exceeding her CL. However, if past romantic partners have never said anything hurtful towards her, then she would have a lower CL.
Becky will also consider another alternative, which is the comparison level of alternatives (CL alt ), or the comparison between current relationship rewards and what she might get in another relationship. If she doesn’t want to be single, then she might have a lower CL of alternatives. If she has another potential mate who would probably treat her better, then she would have a higher level of alternatives. We use this calculation all the time in relationships. Often when people are considering the possibility to end a relationship, they will consider all alternatives rather than just focusing on costs and rewards.
Similarities and Differences
It feels comforting when someone who appears to like the same things you like also has other similarities to you. Thus, you don’t have to explain yourself or give reasons for doing things a certain way. People with similar cultural, ethnic, or religious backgrounds are typically drawn to each other for this reason. It is also known as similarity thesis . The similarity thesis basically states that we are attracted to and tend to form relationships with others who are similar to us. 7 There are three reasons why similarity thesis works: validation, predictability, and affiliation. First, it is validating to know that someone likes the same things that we do. It confirms and endorses what we believe. In turn, it increases support and affection. Second, when we are similar to another person, we can make predictions about what they will like and not like. We can make better estimations and expectations about what the person will do and how they will behave. The third reason is due to the fact that we like others that are similar to us and thus they should like us because we are the same. Hence, it creates affiliation or connection with that other person.
However, there are some people who are attracted to someone completely opposite from who they are. This is where differences come into play. Differences can make a relationship stronger, especially when you have a relationship that is complementary . In complementary relationships, each person in the relationship can help satisfy the other person’s needs. For instance, one person likes to talk, and the other person likes to listen. They get along great because they can be comfortable in their communication behaviors and roles. In addition, they don’t have to argue over who will need to talk. Another example might be that one person likes to cook, and the other person likes to eat. This is a great relationship because both people are getting what they like, and it complements each other’s talents. Usually, friction will occur when there are differences of opinion or control issues. For example, if you have someone who loves to spend money and the other person who loves to save money, it might be very hard to decide how to handle financial issues.
Sometimes we form relationships with others after we have disclosed something about ourselves to others. Disclosure increases liking because it creates support and trust between you and this other person. We typically don’t disclose our most intimate thoughts to a stranger. We do this behavior with people we are close to because it creates a bond with the other person.
Disclosure is not the only factor that can lead to forming relationships. Disclosure needs to be appropriate and reciprocal 8 . In other words, if you provide information, it must be mutual. If you reveal too much or too little, it might be regarded as inappropriate and can create tension. Also, if you disclose information too soon or too quickly in the relationship, it can create some negative outcomes.
Key Takeaways
- We can be attracted to another person via various ways. It might be due to physical proximity, physical appearance, perceived gain, similarity/differences, and disclosure.
- The deepening of relationships can occur through disclosure and mutual trust.
- Relationships end through some form of separation or dissolution.
- Take a poll of the couples that you know and how they met. Which category does it fall into? Is there a difference among your couples and how they met?
- What are some ways that you could form a relationship with others? Discuss your findings with the class. How is it different/similar to what we talked about in this chapter?
- Discuss how and why a certain relationship that you know dissolved. What were the reasons or factors that caused the separation?
- Media Center
Similarity Hypothesis
The basic idea, theory, meet practice.
TDL is an applied research consultancy. In our work, we leverage the insights of diverse fields—from psychology and economics to machine learning and behavioral data science—to sculpt targeted solutions to nuanced problems.
Consider the closest friends you meet while backpacking abroad. You likely share many similarities; perhaps a thrill for spontaneity, hobbies, appreciation for culture, music preferences, or food choices. During the trip, you find yourself effortlessly interacting with other backpackers: sharing a relatively-unknown scenic route, a local exhibition to visit, or the best bed and breakfast in town. We often relate and empathize easily with similar individuals – this is a result of the similarity hypothesis.
The similarity hypothesis suggests that we tend to be drawn towards those who are similar to ourselves. Similarities can refer to shared attitudes and values, as well as political opinions, cultural background, or even minute details like posture. 1
The experience of interacting with similar individuals jumpstarts cognitive processing, like learning, memory, attention, and reasoning. An aspiring musician might remember all the lyrics to their favorite band’s albums. An employee might pick up skills more quickly when assisted by a mentor they admire or identify with. Even when it comes to making comparisons with others, we tend to look for individuals who share similar attitudes and beliefs because it can be difficult to make accurate comparisons when others are too different from us. 2
Why do we tend to be drawn towards individuals who share similar attitudes and values?
Similarity Hypothesis: A hypothesis which states that we tend to be attracted towards individuals who share similar important traits, such as attitudes and values.
Cognitive Processing: A general term to describe any mental function involved in acquiring, storing, interpreting and manipulating information. These functions can be conscious or unconscious, such as attention, memory storage, learning, and reasoning.
Empathy: Understanding an individual from their point of view and experiencing that individual’s feelings, thoughts and perceptions.
In 1954, Leon Festinger proposed in his social comparison theory: when individuals are uncertain of their abilities and opinions, they tend to make comparisons with other similar individuals to assess the accuracy of their own opinion. Festinger’s influential social comparison theory introduced the similarity hypothesis. Since its introduction in A Theory of Social Comparison Processes , a large amount of evidence has supported the hypothesis. 3
Festinger’s hypothesis has been used to explain phenomena in a diverse array of fields, from political science to marketing. For instance, in the 1971 The Attraction Paradigm , psychologist Donn Byrne introduced the similarity-attraction theory. Byrne’s theory was based on the similarity hypothesis. He suggested that individuals who share similar “important attitudes” (opinions on family and values) are generally more likely to be attracted to each other, compared to individuals who share similar “less important” attitudes (opinions on a specific type of sink). 4 This holds for friendships as well as romantic partners. Byrne further outlined that individuals associate with those who have similar personality characteristics, such as self-esteem, optimism, and conscientiousness.
According to Byrne, personality similarity has a key role to play in the longevity and happiness of a marriage. 5 Byrne’s similarity-attraction theory stated that individuals are generally romantically attracted to others who share similar physical characteristics and levels of physical attractiveness. Byrne’s work on similarity-attraction was so influential that further research has supported his theory, with individuals’ preference for similarity being demonstrated in various other aspects such as social habits and socioeconomic status. 5
The similarity hypothesis then made its way into the field of economics and decision-making in Amos Tversky’s 1972 book, Elimination by Aspects: A Theory of Choice . 6 Tversky influenced choice theory in economics by applying the similarity hypothesis to decision-making, changing the way modern economists approached the field. Based on the hypothesis, he suggested that when a new product enters a market, it will take more demand from the share of a similar product than a dissimilar one. This has important implications for brands: when creating a new line of products, they should make it as dissimilar as possible from their current offering to prevent market cannibalization. Tversky’s work influenced marketing managers, who started adopting his use of the similarity hypothesis to help make marketing entry decisions. 7
Leon Festinger
An influential American social psychologist, most renowned for his work on social comparison theory in his 1954 book, A Theory of Social Comparison Processes . Festinger introduced the similarity hypothesis in this book, which has been followed by an enormous amount of data which has provided evidence to support the hypothesis. Several of Festinger’s theories and research also renounced previously dominant behaviorist views of social psychology.
An American psychologist and influential contributor of foundational theory in interpersonal attraction. His work on similarity-attraction theory, based on the similarity hypothesis, was groundbreaking for exploring the relationship between similar attitudes and attraction. Byrne was also an early contributor on the psychology of human sexuality. 8
Amos Tversky
One of the founders of behavioral science who helped revolutionize the field of economics and decision-making. Tversky was an influential psychologist who applied the similarity hypothesis to decision-making and choice theory in economics. Along with Daniel Kahneman , Tversky was also a pioneer in loss aversion and prospect theory .
Consequences
When it comes to attraction, Byrne’s similarity-attraction theory remains relevant today as it provides reassurance that an individual is not alone in their belief. Being attracted to individuals with similar attitudes also enables one to more accurately predict the other’s behaviors in different scenarios, providing an insight into the other’s predilections and “pet peeves” based on similarity. 5
Similarly, when we empathize with a target, such as a novel, our enhanced cognitive processing enables us to facilitate reading comprehension. Our reading accelerates and our memory increases. Likewise, when we fail to empathize with a target, such as a film, we evoke a perception of dissimilarity. This creates the opposite effect, and our cognitive processing is inhibited: we lose focus easily, finding it difficult to recall the plot of the film. 1
Our enhanced cognitive processing is a result of empathy, which arises from our perception of similarity. This affects the way we interact with other individuals, as the perception of similarity can implicitly evoke empathy between two individuals. The perception of similarity is the reason why an employee may be able to learn new techniques more quickly when assisted by a mentor they empathize with.
Understanding the similarity hypothesis can allow us to better design inclusive educational curricula, particularly in scenarios where it is important to understand individuals or experiences which are not necessarily similar to most learners. This can be especially useful in cross-cultural education, history, minority education, and special-needs classes. 1 Applying the similarity hypothesis in these fields of education can help overcome the effort involved in understanding experiences or individuals which are dissimilar.
Controversies
Despite the repeated evidence upholding the similarity hypothesis, one criticism is that individuals frequently seek novelty and difference, with such experiences providing just as much certainty when it comes to self-evaluation. 3
Scholars who disagree with the similarity-attraction theory tend to adopt the complementarity view of attraction. This view states that individuals are more likely to prefer partners who have attributes that are complementary, rather than those who possess replicating attributes. This can be seen when an individual with a certain perceived negative attribute, such as impatience, is more attracted to someone who does not possess that same attribute. The complementarity view of attraction suggests that individuals prefer not to be reminded of their faults by being with someone similar, and therefore they are more attracted to those who will complement and bring out the best in them. 5
Emerging studies are also starting to define more clearly that it is perceived similarity, rather than actual similarity, that influences attraction. A 2012 study by American psychologists at Texas A&M and Northwestern University found that, unlike previous findings, actual similarity did not predict romantic attraction as effectively as previously thought. 9
There are alternative views when addressing how the similarity hypothesis influences opinion comparisons between individuals. Some argue that comparisons with other similar individuals depend on the type of opinion being evaluated. A study in 2000 by Jerry Suls, René Martin, and Ladd Wheeler highlights results which suggest that we prefer comparing with other similar individuals when it comes to the evaluation of preferences. Think about how you are more likely to care about what your best friend thinks of your outfit, compared to the Lyft driver who dropped you off this morning. In contrast, other studies have suggested that we prefer to compare ourselves with dissimilar individuals when it comes to belief assessment, 3 such as evaluating whether a certain statement or proposition is true.
The effects of the similarity hypothesis on memory retrieval.
In 2015, Hidetsugu Komeda conducted a study to observe memory retrieval in typically developing (TD) individuals and individuals with Autism Spectrum Disorder (ASD). The similarity hypothesis predicts that individuals with ASD will be able to easily retrieve other individuals with ASD from their memory. Participants were carefully selected and read 24 stories, before completing a recognition task. The results showed that ASD individuals demonstrated the same level of accuracy as TD individuals, but memory-retrieval patterns between the two groups were different. 1
Individuals with ASD were able to retrieve ASD-consistent stories more easily than ASD-inconsistent stories. TD individuals were also able to retrieve TD-consistent stories more easily than ASD-protagonist stories. These results are consistent with the similarity hypothesis, suggesting that individuals with ASD characteristics are able to help other ASD individuals due to empathy arising from their similarities. 1
Related TDL Content
The Similar-To-Me Effect
Why do we tend to surround ourselves with people similar to ourselves? While it is normal to get along with people who have similar experiences, like your basketball teammate or a fellow college alumnus, favoring people similar to you becomes a problem when it leads to discrimination.
Why do we feel more strongly about one option after a third one is added?
You might never buy the most expensive option, but do you sometimes buy the second-most expensive option? The decoy effect explains why the addition of a third choice can make us spend more money – even if we don’t opt for the new choice.
- Definition of Rapport . (n.d.). Dictionary by Merriam-Webster. Retrieved October 4, 2021, from https://www.merriam-webster.com/dictionary/rapport
- Rapport Quotes . (n.d.). A-Z Quotes. Retrieved October 4, 2021, from https://www.azquotes.com/quotes/topics/rapport.html
- Mcleod, S. (2020). Humanistic approach . Simply Psychology. https://www.simplypsychology.org/humanistic.html
- Active Listening . (n.d.). Skills You Need. Retrieved October 4, 2021, from https://www.skillsyouneed.com/ips/active-listening.html
- Vollmer, S. (2010, January 6). Transference . Psychology Today. https://www.psychologytoday.com/ca/blog/learning-play/201001/transference
- American Psychological Association. (n.d.). therapeutic alliance . APA Dictionary of Psychology. Retrieved October 4, 2021, from https://dictionary.apa.org/therapeutic-alliance
- The Mind Tools Content Team. (2019). Building Rapport . Mind Tools. https://www.mindtools.com/pages/article/building-rapport.htm
- What is Rapport? Techniques for Relationship Building . (2018, May 17). Exploring Your Mind. https://exploringyourmind.com/what-is-rapport-techniques-for-relationship-building/
- Mcleod, S. (2014). Carl Rogers Theory . Simply Psychology. https://www.simplypsychology.org/carl-rogers.html
- Coan, G. (1984). Rapport: Definitions and Dimensions. Advances in Consumer Research , 11 , 333-336. https://www.acrwebsite.org/volumes/6269/volumes/v11/NA-11
- Tickle-Degnen, L., & Rosenthal, R. (1990). The nature of rapport and its nonverbal correlates. Psychological Inquiry , 1 (4), 285-293. https://doi.org/10.1207/s15327965pli0104_1
- Buskist, W., & Saville, B. K. (2001). Creating Positive Emotional Contexts for Enhancing Teaching and Learning. APS Observer , 12-13. https://www.socialpsychology.org/rapport.htm
- Ardito, R. B., & Rabellino, D. (2011). Therapeutic Alliance and Outcome of Psychotherapy: Historical Excursus, Measurements, and Prospects for Research. Frontiers in Psychology , 2 (270). https://doi.org/10.3389/fpsyg.2011.00270
- Miles, L. K., Nind, L. K., & Macrae, C. N. (2009). The rhythm of rapport: Interpersonal synchrony and social perception. Journal of Experimental Social Psychology , 45 (3), 585-589. https://doi.org/10.1016/j.jesp.2009.02.002
- Drolet, A. L., & Morris, M. W. (2000). Rapport in conflict resolution: Accounting for how face-to-face contact fosters mutual cooperation in mixed-motive conflicts. Journal of Experimental Social Psychology , 36 (1), 26-50. https://doi.org/10.1006/jesp.1999.1395
About the Author

Joshua was a former content creator with a passion for behavioral science. He previously created content for The Decision Lab, and his insights continue to be valuable to our readers.

Functionalism
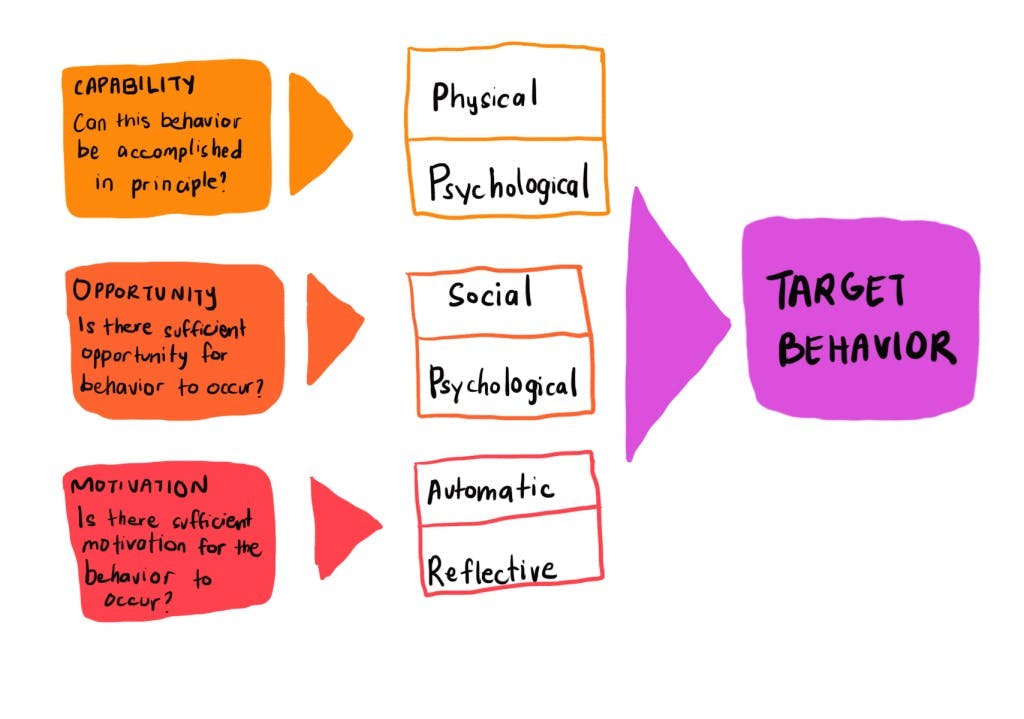
The COM-B Model for Behavior Change

The ABC Model

Behavioral Perspective
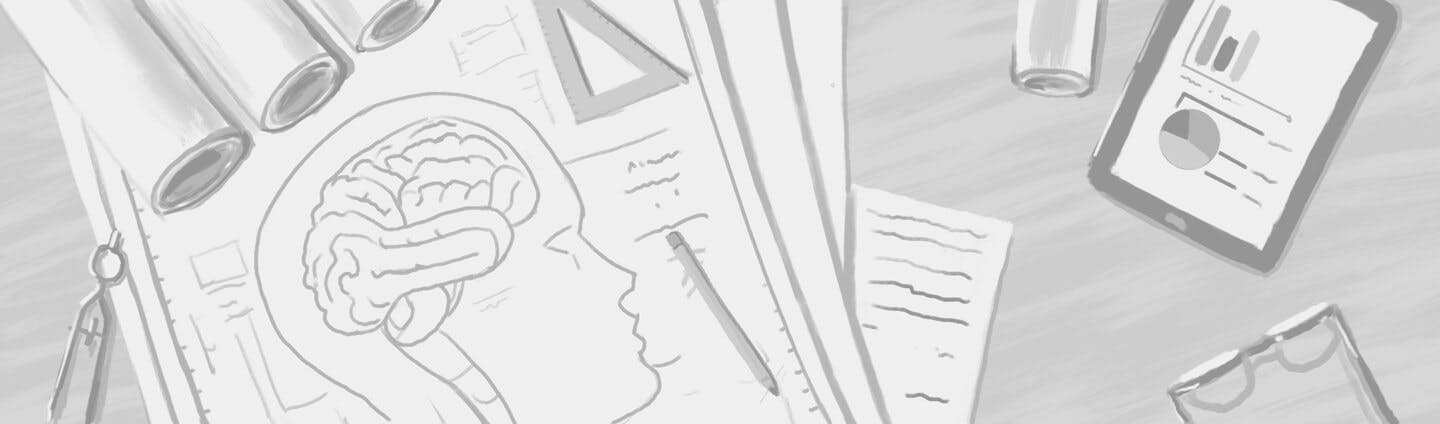
Eager to learn about how behavioral science can help your organization?
Get new behavioral science insights in your inbox every month..
- Search Menu
- Sign in through your institution
- Author Guidelines
- Submission Site
- Self-Archiving Policy
- Why Submit?
- About Journal of Computer-Mediated Communication
- About International Communication Association
- Editorial Board
- Advertising & Corporate Services
- Journals Career Network
- Journals on Oxford Academic
- Books on Oxford Academic
Article Contents
Study 1: the effects of when-similarity, study 2: when-similarity and who-similarity, general discussion, about the authors.
- < Previous
Extending the Similarity-Attraction Effect: The Effects of When-Similarity in Computer-Mediated Communication *
Accepted by previous editor Maria Bakardjieva
- Article contents
- Figures & tables
- Supplementary Data
Maurits Kaptein, Deonne Castaneda, Nicole Fernandez, Clifford Nass, Extending the Similarity-Attraction Effect: The Effects of When-Similarity in Computer-Mediated Communication, Journal of Computer-Mediated Communication , Volume 19, Issue 3, 1 April 2014, Pages 342–357, https://doi.org/10.1111/jcc4.12049
- Permissions Icon Permissions
The feeling of connectedness experienced in computer-mediated relationships can be explained by the similarity-attraction effect (SAE). Though SAE is well established in psychology, the effects of some types of similarity have not yet been explored. In 2 studies, we demonstrate similarity-attraction based on the timing of activities—“when-similarity.” We describe a novel experimental paradigm for manifesting when-similarity while controlling for the activities being performed (what-similarity). Study 1 (N = 24) shows when-similarity attraction in the evaluation of connectedness with others. Study 2 (N = 42) identifies an interaction between who-similarity—similarity in personal backgrounds—and when-similarity. Both studies show that real-time computer-mediated interaction can lead to greater feelings of connectedness between people when there is an opportunity to discover when-similarity.
In their early stages, social networking sites (SNSs) focused primarily on sharing personal information via user profiles, enabling users to discover similarities in demographics, interests, and attitudes. A great deal of empirical evidence indicates that when users discover these types of similarities, even when this discovery is mediated, they become more attracted to each other ( Montoya et al., 2008 )—the so-called similarity-attraction effect ( Byrne, 1971 ). Previous similarity-attraction effect manipulations include personality traits ( Banikiotes & Neimeyer, 1981 ; Bleda, 1974 ), attitudes (Yeong Tan & Singh, 1995 ), ethnic backgrounds ( Hu et al., 2008 ), facial features ( Bailenson et al., 2008 ), and voice features ( Nass & Brave, 2005 ), among others.
Similarity-attraction has been a topic of investigation for more than half a century, but the recent growth of mediated communication inspires new questions regarding similarity and interpersonal attraction. In this paper, we explore a previously unstudied type of interpersonal similarity: similarity in the timing of activities. That is, beyond knowing that someone else holds the same attitudes as oneself, shares a common background, or performs the same activities, is similarity-attraction amplified when two people know that they are doing the same activity at the same time? With the dramatic growth of real-time applications—such as Facebook and Twitter—one can be presented with contemporaneity information without actively interacting with the other person. Does this computer-mediated simultaneity influence people's feelings of connectedness and belonging? Does similarity-attraction extend to similarity in the timing of activities?
Background on The Similarity-Attraction Effect
The empirical evidence for similarity-attraction is so compelling that Byrne and Rhamey ( 1965 ) early on labeled the positive relationship between respondents' similarity and the attraction between respondents the Law of Attraction . After numerous replications in multiple domains using different similarity manipulations, Berger ( 1975 ) proclaimed that similarity-attraction is “one of the most robust relationships in all of the behavioral sciences.”
The explanations for the origin of similarity-attraction are, however, multifold and often disputed. Initially, no explanations for the effect were offered, and similarity-attraction was regarded to be self-evident—as we see even today by the lack of explanations for similarity-attraction in many social psychology textbooks ( Heine et al., 2009 ). When explanations are proposed, one of the most popular views is based on people's innate desire to be consistent with societal norms and values. This explanation assumes that the discovery of interpersonal similarity leads to the validation of one's own characteristics and views by providing consensus support ( Byrne & Clore, 1970 ). The validation then leads to a higher perceived appropriateness of one's current beliefs, attitudes, behaviors, and traits.
Byrne ( 1971 ) and Clore and Byrne ( 1974 ) extended this explanation in their formulation of the reinforcement-affect model. This model is based on the assumptions that: (a) people experience stimuli as rewarding or punishing and seek out those that are rewarding, (b) positive feelings—affect—are associated with rewarding stimuli, and (c) other people are liked or disliked according to their association with rewarding or punishing stimuli. That is, we learn to associate positive feelings with people that are linked to rewards. Instances of interpersonal similarity function as rewarding stimuli, which leads people to associate positive feelings with similar others, which in turn leads people to be more attracted to similar others.
Another popular explanation for similarity-attraction is the emergence of positive feelings stemming from smooth and rewarding interactions, which are more likely to arise when communicating with people who are similar to oneself ( Berscheid & Walster, 1978 ) than when communicating with dissimilar others. While this explanation is similar to the explanation of Clore and Byrne ( 1974 ) in its emphasis on rewards experienced by positive stimuli, Berscheid & Walster ( 1978 ) specifically focus on the smooth interactions that are likely to occur with similar others as opposed to the positive feelings stemming from discovering instances of interpersonal similarity. Through these smooth interactions with others, similarity-attraction partly satisfies a person's “Need to Belong” ( Baumeister, 1995 ).
Other explanations put forward for similarity-attraction include enhanced reciprocal liking towards similar others ( Condon & Crano, 1988 ) or a desire to satisfy implicit egoism ( Jones et al., 2004 ). One final explanation for the similarity-attraction effect inverts these arguments: A person's default state is to like everyone, and dissimilarity leads to repulsion ( Rosenbaum, 1986 ). Rosenbaum supports this view by showing several experiments in which the standard experimental paradigm of the SAE is extended by adding a “no-interaction” control group. His work shows that attraction ratings in the no-interaction and in the similar other groups are comparable, while the ratings in the nonsimilar other group are significantly lower.
Despite the overwhelming empirical evidence supporting the existence of similarity-attraction as well as the numerous plausible mechanisms identified to explain it, several questions have been raised regarding the importance and integrity of the effect. Some authors have discounted the effect as merely resulting from demand characteristics operating in experiments ( Sunnafrank, 1991 ) or other methodological flaws ( Bochner, 1991 ). Morry ( 2007 ) questions the claimed causality of similarity-attraction, and Sunnafrank and Miller ( 1981 ) demonstrate that similarity-attraction is diminished greatly when allowing for initial interactions between participants in laboratory studies. Overall, these results lead critics to conclude that similarity-attraction only exists in a laboratory setting using ad-hoc dyads and is of no practical importance in a real-world setting. Meta-analysis of numerous SAE studies both within and outside of the laboratory indeed shows that while similarity manipulations have strong effects on attraction in laboratory settings, these effects are limited in real long-term relationships ( Montoya et al., 2008 ). In the next section, we will explain why these criticisms do not discredit similarity-attraction as an important psychological effect in real-time computer-mediated interactions.
Types of Similarity in New Media
The most common laboratory paradigm in similarity-attraction research is the phantom-other technique ( Smith, 1957 ; Byrne, 1961 ). In laboratory experiments using the phantom-other technique, the target is often unknown to participants. Participants are then presented with details about the nonpresent target, such as age, personality, judgments, or social status. This phantom-other technique leads to the strongest similarity-attraction effects.
Coincidentally, these laboratory characteristics frequently hold for a wide variety of computer-mediated communications that take place in new media and SNSs: A large number of “friends” in people's social networks are relatively unfamiliar, not physically present, and their profiles present details not commonly discovered in face-to-face interactions. Thus, while similarity-attraction has been hard to replicate outside of the laboratory, the situation is now reversed: Real life, through SNSs and other mediated communication, has replicated the laboratory conditions in which similarity-attraction was first observed. It is thus plausible that the laboratory studies that support the similarity-attraction effect will possess high external validity in these new media contexts.
There are many types of similarity encountered by people when using new media like SNSs. Initially, SNSs enabled people to explore and experience “who-similarity": similarity in demographic features such as ethnic background or religious affiliation. As personal profiles and information streams on SNSs grew, people were also able to discover “what-similarity": similarity in attitudes, activities, and hobbies. The use of social GPS tracking, as is done by applications like Foursquare, has even increased the salience of “where-similarity": similarity in location.
Recent advancements—specifically, real-time social technologies—have created the ability to discover a new type of similarity that has not been previously examined either in the laboratory or in the field: when-similarity. While it was already possible to connect with remote others in real-time since the emergence of chat rooms and instant messaging (see e.g. Baker, 2008 ), experiencing co-occurrence of (remote) activities in time without an explicit conversation or shared activity is relatively novel. In this paper, we create a situation in which co-occurrence of remote activities is experienced without introducing additional confounds that would naturally arise during an explicit conversation.
Real-time services like Facebook and Twitter enable users to experience similarity with others in the timing of activities. With millions of people broadcasting their current activity status in real-time repeatedly during the day, it is highly likely users will experience some form of when-similarity: Users discover that at the point in time that they are carrying out a specific activity, someone in their extended social network is carrying out that same activity, without an active conversation or conscious joint activity of the two parties involved. Due to the growing prominence of real-time services both on the web as well as on other devices such as mobile phones, it is worthwhile to explore whether this new type of similarity also enhances attraction and thus supports the similarity-attraction effect.
Overview of the Studies
In this paper, we describe two studies in which when-similarity (Study 1) and both when- and who-similarity (Study 2) are manipulated. The effects on the participant's perception of a target other are measured. Based on the strong evidence that supports similarity-attraction in settings similar to those experienced by users of real-time services, we hypothesize that similarity-attraction will also hold for this new type of similarity. That is, we expect that users who discover when-similarity with a target through computer-mediated communication channels will feel closer to the target. This expectation is already supported by a number of sociological investigations into the effects of the timing of events such as religious festivities: When a group of individuals perform an activity or ritual at the same time, simultaneity plays a key role in the formation of feelings of connectedness within the social group. ( Durkheim, 1912 / 2008 ; Horton, 1967 ; Lee & Liebenau, 2000 ; Zerubavel, 1982 ).
In both of the presented studies, we use a novel method to manipulate when-similarity while controlling for other types of similarity. We are the first to isolate the effects of the timing of activities from confounds such as the type of activity being performed. The mediated nature of the interactions in the experiment enables us to manipulate the timing of activities while keeping the pattern of activity types constant and while avoiding confounds caused by physical proximity. Our manipulation of when-similarity differs from traditional experimental manipulations in that the two experimental groups—those high and low in when-similarity—are created dynamically based on the behavior of participants. We explain this method in detail.
This study tests the effects of when-similarity—while controlling for other types of similarity—on the participants' evaluations of others. Interpersonal attraction, after a weeklong intervention manipulating when-similarity, was measured using both social connectedness and intimacy scales. When-similarity was manipulated between participants.
Participants
Twenty-four United States college students participated in this balanced, between-participants experiment. Thirteen (54.2%) participants were female, and gender was balanced as much as possible across the conditions. The average age of respondents was 20.1 (S D = 1.61) years. Participants received partial course credit for their participation.
First, participants were asked to complete a brief personal profile, which asked for their gender, age, area(s) of academic study, and favorite pastimes. Participants were then ostensibly partnered with another participant of the same gender. Participants were provided with the name of their partner for the duration of the experiment, and no other information about the partner was disclosed. In reality, participants' partners were not actual participants, and partners' names were selected to provide a perceived gender match with the participant.
Next, participants received six text messages per day on their mobile phones over the course of five days. Each message contained the question: “What are you doing right now?” Participants were instructed to reply with a number from 1 to 5, which represented different behavioral categories: 1: Eating , 2: Studying , 3: Physical Activity , 4: Relaxing , and 5: Working . These categories were pretested to resonate with the participant population and were found exclusive as well as exhaustive: a 2-day pretest showed that for each moment in the day that they were queried, students ( N = 11) were able to pick exclusively one of the five provided categories as their current activity.
A few minutes after responding to the text message, participants received a follow-up message stating the activity their partner was ostensibly performing at that same point in time, and whether their own activities matched their partner's. Participants were not provided with any information about their partner other than these activity messages. After 5 days of text messages, summing to 5 × 6 = 30 messages, participants answered an online questionnaire to evaluate their partner and their perceived relationship with their partner.
We chose text messaging as our medium, as opposed to an SNS, since respondents would have direct access to their devices to be able to report on their activities at the moment the messages were received. Furthermore, the text messages allowed us to fully control the conversation with the ostensible other with less risk of directed online searches to get in contact via other means. Thus, text messaging was chosen mainly for methodological ease. We do not feel our results are restricted to text messaging but rather are representative of a much broader class of mediated real-time interactions.
When-Similarity Manipulation
To manipulate when-similarity while controlling for other types of similarity, half of the participants were assigned to the Similar Timing condition and half were assigned to the Dissimilar Timing condition. In both conditions, to keep constant what the partner was doing, the ostensible partner responded in such a way that after all six text messages had been sent for a specific day, the partner had studied twice and performed each of the other behavioral categories only once. This constraint on the activity pattern of the ostensible partner was imposed to prevent the actual activity from influencing the perceptions of the partner. By providing the same “activity profile” in both of our conditions, we minimize the effect of what-similarity.
The two conditions differed only in when the participants were told their partners were performing these activities. In the Dissimilar Timing condition, the response, when possible, consisted of a different behavioral category than the one performed by the participant at that point in time. For example, if the participant indicated she was “eating” at the time she received a message, the response message would be any (random) behavioral category other than eating—unless all other behavioral categories had already been exhausted that day, leaving “eating” to be the only valid remaining response. In the Similar Timing condition, the response was, when possible, in the same behavioral category. Since no background information about the participants' ostensible partners was presented, this experimental setup also controlled for possible confounds of who- or where-similarity (beyond the matching gender).
Validity of the When-Similarity Manipulation
Something to keep in mind is that ostensible partner responses generated by our algorithm depend on the activities that are performed by our participants. Thus, to evaluate the validity of our when-similarity manipulation, we need to determine whether there would always be a difference between the number of simultaneous activity occurrences for the Similar and Dissimilar Timing groups regardless of whether our participants' overall activity pattern were different. We addressed this by conducting simulations of our response algorithm for different possible participant activity patterns. For N = 20 participants per simulation ( M = 1000), we took six draws from a five-category multinomial—corresponding to the six activity messages sent daily. By changing the initial probabilities of the five activity categories, we were able to test the consistency of our algorithm's responses to different participant activity patterns (i.e., what are the generated responses from the ostensible partner if the participant performs the same activity at all times?).
Figure 1 shows the simulation results for the actual observed activity probabilities in our study (Row 1), a flat activity pattern in which the simulated participants performed each of the activities equally often (Row 2), and a severely skewed distribution of activities in which simulated participants spent far more time studying than performing any of the other activities (row 3). The distribution of responses by the ostensible partner (Column 2) is the same for all simulations due to the restricted daily activity pattern implemented to control for what-similarity (i.e., study twice a day and perform all other categories only once). Column 3 shows the distribution of the percentage of simultaneous activities in the Similar Timing and the Dissimilar Timing conditions—that is, the times in the simulation when the activities of the participant and the ostensible partner would match. In each of the simulated scenarios, including the severely skewed scenario (Row 3, Column 3), the number of matches in activity responses produced by the algorithm differed significantly between the Dissimilar Timing and Similar Timing conditions. Thus, our when-similarity manipulation creates distinct numbers of activity matches between the Similar Timing and the Dissimilar Timing groups while controlling for what-similarity even in situations where the activities of the participant are skewed 1 .

Evaluation by means of simulation of the when-similarity algorithm for different true population distributions of activities. The column on the right shows the distribution of the number of matches over all simulated experiments. Rows show different participant activity patterns: Row 1 shows the actual activity pattern of our participants, Row 2 shows an evenly distributed activity pattern, and Row 3 shows a highly skewed pattern. Even in the last case, the response generated by our matching algorithm led to a significant difference in the number of matches between the two conditions.
In this study, the activity of the ostensible partner matched that of the participant 50.6% of the time in the Similar Timing condition and 3.0% of the time in the Dissimilar Timing condition. Thus, the actual number of instances of experienced when-similarity in the two conditions is in accordance with those produced in the simulations.
Participants evaluated their partner after the one-week manipulation using three rating scales. The first scale was a 6-item, 7-point Social Connectedness Scale (Cronbach's α = 0.94) (Van Bel et al., 2009 ). The endpoints of the items were labeled “(1) Totally disagree” to “(7) Totally agree.” This social connectedness scale consisted of items addressing the feelings of closeness and shared thoughts between the participant and their partner (e.g. “ I often know what my partner feels ” and “ I feel that my partner often knows what I think ”).
The second scale was the Inclusion of the Other in the Self (IOS) Scale. The IOS measures perceived intimacy ( Aron et al., 2006 ) using a single, 7-point pictorial item. Each of the pictures shows two circles labeled “You” and “Your Partner.” In each picture, the circles overlap more and more—from nontouching to almost fully overlapping. Participants are asked which of the pictures most closely represents the relationship with their ostensible partner.
Finally, participants were asked to state how much they agreed with the statement “ I would like to meet my partner ” on a 7-point scale. The end-points of this scale—which was specifically designed for the purposes of this experiment—were labeled “(1) Completely disagree” to “(7) Completely agree.”
A MANOVA with when-similarity as an independent factor and the three attitudinal measures as dependent factors showed a strong multivariate main effect of when-similarity-attraction on the overall partner evaluations, F(3,20) = 10.58, p < 0.001, η 2 = 0.61. Table 1 presents the mean scores of each dependent variable and the outcomes of separate t-tests for the effect of when-similarity. For each of the three dependent measures, participants in the Similar Timing condition scored significantly higher than participants in the Dissimilar Timing condition.
Comparisons of attitudes towards the ostensible partner in the Dissimilar Timing and Similar Timing conditions. N = 24
Study 1 examined the effects of similarity in the timing of activities—when-similarity—on participants' evaluations of others using a method that controlled for other types of similarity. This experimental manipulation of when-similarity produced a large difference in the number of matching simultaneous activities between our experimental conditions while controlling for the patterns of activities performed during the day. The latter constraint controls for what-similarity but also ensures a realistic activity pattern performed by the ostensible partners of participants in this study. The ostensible partner-generated activity patterns are very close to the activity patterns performed by the participants themselves (compare Figure 1 , column 1, row 1 & 2).
Participants in the Similar Timing condition evaluated their partners more positively than those in the Dissimilar Timing condition: Participants that experienced a similar timing of activities felt more connected to their partner, felt more intimate, and were more eager to meet their partner than those who did not experience timing similarity. These findings support our hypothesis that similarity-attraction holds for this previously unstudied type of similarity. The finding extends similarity-attraction to when-similarity in a computer-mediated setting that is a common experience for people using real-time web services.
Besides the practical importance of showing that when-similarity can have positive effects on people's evaluations of others in SNSs, these results and the proposed method can be further used to study the interactions between different types of similarity and even to evaluate possible similarity-attraction explanations. Historically, similarity-attraction explanations were concerned largely with who- or what-similarity. The existence of a positive when-similarity attraction effect in cases for which who- and what-similarity is absent could partially invalidate explanations that are solely or heavily dependent on who people are and/or what they do. To explore this further and test the robustness of the when-similarity effect, Study 2 combines a manipulation of when-similarity with a more traditional manipulation of who-similarity.
In Study 2, we used a similar experimental protocol as detailed in Study 1 to examine the possible interaction between when-similarity and who-similarity. This interaction is of practical importance because these two types of similarity are often experienced together when using SNSs as well as other forms of computer-mediated communication. In addition to their practical importance, interactions between different types of similarity are also theoretically interesting: Hypotheses about these interactions would differ based on which explanation of similarity-attraction to which one subscribes. When following the popular explanation that similarity-attraction is caused by a desire to validate one's own beliefs, when-similarity would have no effect (which is not consistent with Study 1). If, however, an explanation of similarity-attraction is not necessarily tied to this type of similarity and rather encompasses a holistic view in which any type of additional similarity increases attraction, one would expect separate main effects of both when- and who-similarity.
Study 2 examined the effects of both when-similarity and who-similarity simultaneously. A 2 (when-similarity: Similar Timing vs. Dissimilar Timing) × 2 (who-similarity: Similar Profile vs. Dissimilar Profile) between-participants experiment was created to test the effects of both when-similarity and who-similarity on the evaluations of others.
Participants in this study consisted of 17 male (38.6%) and 27 female (61.4%) United States college students with an average age of 20.9 ( SD = 1.5) years. Gender was evenly balanced as much as possible across conditions, and participants again received partial course credit for their participation in this study. None of the participants had participated in Study 1.
The procedure in this study was very similar to the procedure in Study 1 with one minor change: after participants were told that they would be paired with another person—during the introduction questionnaire—they were shown a profile of their ostensible partner. The profile contained information about their partner's gender, age, academic focus, and three favorite pastimes. These variables were chosen to link directly to the types of information presented in profiles that are typically used in SNSs.
The when-similarity manipulation was similar to that described in Study 1. In this study, activities matched temporally 52.2% of the time in the Similar Timing condition and 2.5% of the time in the Dissimilar Timing condition. Again, keeping the ostensible partner's activity pattern constant within each day of the experiment controlled for what-similarity.
Who-Similarity Manipulation
Based on the participant's profile, their partner's profile was dynamically generated as employed in the phantom-other technique ( Smith, 1957 ). The partner's profile was adjusted to implement the two experimental conditions. In the Dissimilar Profile condition, the participant's partner was randomly 3 to 4 years older or younger, pursued a different academic focus, and had at least two different pastimes. In the Similar Profile condition, the partner was randomly one year older or younger, pursued the same academic focus, and had two similar pastimes. Who-similarity was thus manipulated on multiple dimensions. In all cases, the partner's gender matched the participant's gender.
This manipulation of who-similarity in which the difference in age, academic focus, and favorite pastimes are all simultaneously manipulated may seem overstated. However, to examine the robustness of when-similarity, it was important to have a strong manipulation of who-similarity and -dissimilarity. The manipulation was not unrealistic: frequently on SNSs, people are exposed to profiles of other people with whom they have a single or very few commonalities (e.g., a shared alma mater) but who are, in most other respects (e.g., their academic focus, age, and favorite pastimes), different from themselves.
Study 2 used the same dependent variables as Study 1: Connectedness ( Cronbach's α = 0.93), Intimacy, and Willingness to Meet. Different than in Study 1, participants answered these questions both directly after the who-similarity manipulation when they received their partner's profile—prior to the experience of when-similarity—and at the end of the text messaging intervention period—after the experience of when-similarity.
Pre-When-Similarity Manipulation
Participants were asked to rate their initial impressions of their partner directly after reading their profile and thus before the when-similarity manipulation. A MANOVA with who-similarity as an independent factor and the three attitudinal measures (i.e. Connectedness, Intimacy, and Willingness to Meet) as dependent factors showed a strong multivariate main effect of who-similarity on the overall partner evaluations, F(1,42) = 7.02, p < 0.001, η 2 = 0.36. Table 2 shows separate t-tests for each of the dependent measures. Participants in the Similar Profile condition felt more connected to and more intimate with their partner, consistent with previous findings using the phantom-other technique. The who-similarity manipulation failed to influence whether or not participants wanted to meet their partner, although the difference was in the expected direction.
Comparisons of attitudes in the Dissimilar Profile and Similar Profile conditions after reading the profile. N = 44
Post-When-Similarity Manipulation
Participants also rated their impressions of their partner after the weeklong text messaging intervention—the experimental manipulation of when-similarity. In the 2x2 MANOVA, there was a significant multivariate main effect of when-similarity, F (3,38) = 6.61, p < 0.001, η 2 = 0.34, such that participants who worked with ostensible partners who had similar timing of activities were much more attracted to their partners than those participants who had a partner with dissimilar timing. There was no multivariate main effect of who-similarity, F(3,38) = 1.26, p > 0.05, η 2 = 0.09, although the means were in the expected direction.
There was a significant interaction effect between who- and when-similarity, F (3,38) = 7.46, p < 0.001, η 2 = 0.37, presented in Figure 2 . The effect of when-similarity is strong and positive when the partner is initially perceived as dissimilar based on the presented profile information. If, however, attraction is already established based on a similar profile, the additional when-similarity does not increase attraction.

Estimated marginal means and standard errors of the similarity-attraction scores for the four experimental groups.
Table 3 presents separate univariate results to provide a more detailed look at the data. Participants' feelings of Connectedness and Perceived Intimacy with their partners were influenced by the when-similarity manipulations. Again, the Willingness to Meet question—as was the case after the who-similarity manipulation—does not exhibit an effect of the similarity manipulations, although the means were in the expected direction.
Results of both the Who- and When-similarity manipulations
The results of the who-similarity manipulation directly after the presentation of the partner profiles replicate the laboratory-setting finding that who-similarity affects people's evaluations of others positively: Sharing of a common background leads to more attraction when no other information about the partner is provided.
In Study 2, no results were found regarding the willingness to meet the ostensible partner based on both who- and when-similarity, but this item has limited validity and reliability: It queries a behavioral intention of the participants instead of a judgment about the ostensible partner and is a single item. After a week of interactions geared for the discovery of when-similarity, while controlling for who- and what-similarity, the main effect of who-similarity was weakened. This is probably due to the fact that throughout the week, the ostensible partner only matched the participant's activities 50% of the time, leading to a weakening of the initial manipulation of extremely high similarity.
The reinforcement-affect model provides an interesting means for understanding these results. The discovery of similarity or dissimilarity functions as a reward or punishment, which in turn leads to a change in affect towards similar or dissimilar others. A classical finding in the operant conditioning literature shows that rewards or punishments are not additive but rather elicit satiety: the effectiveness of reinforcement reduces once an individual's need for that reinforcement has been satisfied ( see, e.g., Guttman, 1953 ). Under this view, while who-similarity acts as a reward and in turn produces positive affect, any potentially positive effects of additional similarity are limited.
The results of Study 2 do however indicate that a weak attraction to others based on encountering a dissimilar profile in SNSs can be overcome by the synchronous timing of activities. In cases of initial dissimilarity (a dissimilar profile), the reward of discovering when-similarity leads to more positive evaluations.
The opportunity for people to experience when-similarity—without experiencing other types of similarity simultaneously—has emerged due to the recent growth of real-time technology. Services like Twitter enable people to discover what people who are essentially strangers are doing at exactly that moment in time. The mediated nature of these services enables a separation of when-similarity from what-similarity (and physical proximity) that was virtually unimaginable before the existence of real-time mediated interactions. Current communication practices, mediated through computers, mobile phones, and other devices, affect the nature and structure of our encounters with interpersonal similarity—in some respects bringing them closer to the encounters that occurred in past laboratory studies examining similarity-attraction. Our two studies show that when no other information about the other is provided (Study 1) or when the other is regarded as dissimilar based on a number of demographics and interests (Study 2), when-similarity leads to attraction and a more positive evaluation of the other. Greater feelings of connectedness towards the other are reported, and the perceived intimacy between people is increased upon encountering when-similarity.
We introduce a new method to study when-similarity in a setting that has high external validity. The presented manipulation enables control over other types of similarity. In both of our studies, this manipulation of when behaviors occurred while controlling for daily behavioral patterns.
When-Similarity Manipulation and Interactions
The when-similarity manipulation presented in this paper is qualitatively different from most manipulations found in experimental psychology or communication studies. While participants were randomly assigned to a fixed condition, the stimuli they received in these respective conditions were not deterministic. Due to our imposed constraint, which controlled for what-similarity—and thus ensured that participants' partners had the same activity pattern in both conditions—the generated responses differed based on the activity patterns of our participants. However, through simulations including severely skewed activity distributions for participants, we showed that the manipulation consistently led to differing numbers of time-matched activities between the two conditions. We hope that this manipulation can be useful in further research into the effects of similar timing of activities within the similarity-attraction paradigm.
Our when-similarity manipulation also created a clear distinction between different types of similarity that can be experienced by people on SNSs. While within the similarity-attraction literature, numerous moderating variables have been investigated ( Pilkington & Lydon, 1997 ), there is no work detailing interactions between the different types of similarity as introduced in our studies. However, these interactions are of great interest because they enable studying a possible additive effect of increased similarity and can also be used to further examine the explanations offered for similarity-attraction.
Explanations for Similarity-Attraction
As noted in the introduction, a popular explanation for similarity-attraction put forward by Byrne and Clore ( 1970 ) is based on people's need for accuracy: Finding people who share similar attitudes or background corroborates one's own beliefs and as such, positively enforces one's feeling of accuracy. While this explanation is very plausible for what-similarity—similarity based on shared attitudes or beliefs—given that finding similar others indeed reinforces the accuracy of consciously chosen and held beliefs, it is debatable that this explanation holds for every type of similarity. Already for who-similarity, in which characteristics that are not consciously chosen or selected by respondents are manipulated, it is harder to argue that, for example, being of the same gender enforces one's motivation towards accuracy. In Study 2, the who-similarity manipulation included several factors and thus its effectiveness does not necessarily invalidate the motivation-for-accuracy explanation. However, for the when-similarity manipulation employed in this study, it is not very plausible that the need for accuracy is an appropriate explanation for the established effect: One would have to subscribe to the assumption that people strive for accuracy in their timing of relatively trivial activities—such as eating—and that finding someone eating at some other time threatens their own accuracy perception. Future work, through manipulating different similarity types, can test conflicting hypotheses elicited from the different similarity-attraction explanations. In this way, the new types of similarity emerging in computer-mediated communication can aid our understanding of important social phenomena.
Our results are best explained not by a need for accuracy but rather by the predictions one would make based on the reinforcement-affect model. In this model, similarity-attraction is explained by the idea that similarity (or dissimilarity) functions as a reward (or punishment). Rewards are then associated with positive feelings which in turn lead to both positive affect towards similar others as well as a tendency to actively seek out similar others. The literature on operant conditioning however shows that both rewards and punishment are not additive: Once a person's need for a certain reward has been satisfied, the effectiveness of that reward reduces. This explains the interaction between who- and when-similarity observed in Study 2: Both who- and when-similarity can increase people's evaluations of others; however, once one is established, the additional effect of more similarity (or different types of similarity) is reduced.
Future Work
In this article, we presented when-similarity and the idea that this particular type of similarity is emergent in mediated communication. Next, we described a method to study this type of similarity while controlling for other types of similarity and have shown the method's effects on interpersonal evaluations. However, the results presented in this article still require further explanation: Possible conflicting explanations for the origins of the interaction between different types of similarity need to be examined in more detail. In particular, a more in-depth examination of the interactions between other types of similarity (e.g. what-similarity and who-similarity) is clearly called for. A better understanding of the effects demonstrated in this paper would also emerge if the exact nature of the reinforcing process that is likely in play when experiencing when-similarity is clarified: Does similar timing reinforce one's own previous choices? Or does similar timing create a bond through more subtle means identical to the sharing of physical space (e.g. Gibson, 1984 )?
Finally, we believe it is also important for future research to look at the cognitive processes that are activated when experiencing when-similarity: these processes might provide a more developed explanation for the interaction effects observed in Study 2.
Practical Implications
Web services like Twitter and Facebook show the activities of other users at a given moment. Critics of the impact of social real-time technologies have argued that people's urge to post status updates to SNSs can be explained merely by an egocentric urge for self-exhibition. Our two studies of the effects of when-similarity, the type of similarity that is primarily experienced using services like Twitter, suggest that another effect could be in play: people's need to belong ( Baumeister, 1995 ) is partly satisfied by the discovery of when-similarity. Hence, real-time services can serve a very social goal: enhancing connectedness by emphasizing when-similarity .
The exact algorithm to generate the replies is available in [R] (used for the simulation study) or PHP (used for the empirical studies) upon request.
Aron , A. , Aron , E. N. , & Smollan , D. ( 2006 ). Inclusion of other in the self scale and the structure of interpersonal closeness . Journal of Personality and Social Psychology , 63 , 596 – 612 .
Google Scholar
Bailenson , J. N. , Iyengar , S. , Yee , N. , & Collins , N. A. ( 2008 ). Facial similarity between voters and candidates causes influence . Public Opinion Quarterly . 72 ( 5 ), 935 – 961 .
Baker , A. J. ( 2008 ). Down the rabbit hole: The role of place in the initiation and development of online relationships . In A. Barak (Ed.), Psychological aspects of cyberspace: Theory, research, applications . Cambridge, UK : Cambridge University Press .
Google Preview
Banikiotes , P. G. , & Neimeyer , G. J. ( 1981 ). Construct importance and rating similarity as determinants of interpersonal attraction . British Journal of Social Psychology , 20 , 259 – 263 .
Baumeister , R. F. ( 1995 ). The need to belong: Desire for interpersonal attachments as a fundamental human motivation . Psychological bulletin , 3 , 497 – 529 .
Berger , C. R. ( 1975 ). Task performance and attributional communication as determinants of interpersonal attraction . Speech Monographs , 40 , 280 – 286 .
Berscheid , E. , & Walster , E. H. ( 1978 ). Interpersonal attraction . McGraw-Hill College .
Bleda , P. R. ( 1974 ). Toward a clarification of the role of cognitive and affective processes in the similarity-attratction relationship . Journal of Personality and Social Psychology , 29 , 368 – 373 .
Bochner , A. P. ( 1991 ). On the paradigm that would not die . Communication Yearbook , 14 484 – 491 .
Byrne , D. ( 1961 ). Interpersonal attraction and attitude similarity . Journal of Abnormal and Social Psychology , 62 , 713 – 715 .
Byrne , D. ( 1971 ). The attraction paradigm . Academic Press .
Byrne , D. , & Clore , G. L. ( 1970 ). A reinforcement model of evaluative responses . Personality: An International Journal , 1 , 103 – 128 .
Byrne , D. , & Rhamey , R. ( 1965 ). Magnitude of positive and negative reinforcements as a determinant of attraction . Journal of Personality and Social Psychology , 2 , 884 – 889 .
Clore , G. L. , & Byrne , D. A reinforcement-affect model of attraction . In T. L. Huston (Ed.), Foundations of interpersonal attraction . New York : Academic Press , 1974 .
Condon , J. , & Crano , W. D. ( 1988 ). Inferred evaluation and the relation between attitude similarity and interpersonal attraction . Journal of Personality and Social Psychology , 54 , 789 – 797 .
Durkheim , E . ( 1912/2008 ). The elementary forms of religious life . trans. by C. Cosman . New York : Oxford University Press .
Gibson , W. ( 1984 ). Necromancer . New York : Ace .
Guttman , N. ( 1953 ). Operant conditioning, extinction, and periodic reinforcement in relation to concentration of sucrose used as reinforcing agent . Journal of Experimental Psychology , 46 ( 4 ), 213 – 224 .
Heine , S. J. , Foster , JA. B. , & Spina , R. ( 2009 ). Do birds of a feather universally flock together? Cultural variation in the similarity-attraction effect . Asian Journal of Social Psychology , 12 , 247 – 258 .
Horton , J. ( 1967 , April). Time and cool people . Society , 4 ( 5 ), 5 – 12 .
Hu , C. , Thomas , K. M. , & Lance , C. E. ( 2008 ). Intentions to initiate mentoring relationships: Understanding the impact of race, proactivity, feelings of deprivation, and relationship roles . The Journal of Social Psychology , 148 ( 6 ), 727 – 744 .
Jones , J. T ., Pelham, B. W., M., C., & Mirenberg , M. C . ( 2004 ). How do I love thee? let me count the ways: Implicit egotism and interpersonal attraction . Journal of Personality and Social Psychology , 87 , 665 – 683 .
Lee , H. , & Liebenau , J. ( 2000 ). Time and the internet at the turn of the millennium . Time and Society , 9 ( 1 ), 43 – 56 .
Montoya , R. M. , Horton , R. S. , & Kirchner , J. ( 2008 ). Is actual similarity necessary for attraction? A meta-analysis of actual and perceived similarity . Journal of Social and Personal Relationships , 25 ( 6 ), 889 – 922 .
Morry , M. M. ( 2007 ). Relationship satisfaction as a predictor of perceived similarity among cross-sex friends . Journal of Social and Personal Relationships , 24 , 117 – 138 .
Nass , C. & Brave , S. ( 2005 ). Wired for speech: How voice activates and advances the human-computer relationship . Cambridge, MA : MIT Press .
Pilkington , N. W. , & Lydon , J. E. ( 1997 ). The relative effect of attitude similarity and attitude dissimilarity on interpersonal attraction: Investigating the moderating roles of prejudice and group membership . Personality and Social Psychology Bulletin , 23 ( 2 ), 107 – 122 .
Rosenbaum , M. E. ( 1986 ). The repulsion hypothesis: On the nondevelopment of relationships . Journal of Personality and Social Psychology , 51 , 1156 – 1166 .
Smith , A. J. ( 1957 ). Similarity of values and its relation to acceptance and the projection of similarity . Journal of Psychology , 43 , 251 – 260 .
Sunnafrank , M. J. ( 1991 ). Interpersonal attraction and attitude similarity: A communication-based assessment . In Communication yearbook 14 . Sage .
Sunnafrank , M. J. , & Miller , G. R. ( 1981 ). The role of initial conversations in determining attraction to similar and dissimilar strangers . Human Communication Research , 8 , 16 – 25 .
Van Bel , D. T ., Smolders , K. C. H. J ., IJsselsteijn , W. A ., & De Kort , Y. A. W . ( 2009 ). Social connectedness: concept and measurement . In V. Callaghan , A. Kameas , A. Reyes , D. Royo , & M. Weber (Eds.), Proceedings of the 5th international conference on intelligent environments . (p. 67 – 74 ). Amsterdam : IOS Press .
Yeong Tan , D. T. , & Singh , R. ( 1995 ). Attitudes and attraction: A developmental study of the similarity-attraction and dissimilarity-repulsion hypotheses . Personality and Social Psychology Bulletin , 21 ( 9 ), 975 – 986 .
Zerubavel , E. ( 1982 , July). The standardization of time: A sociohistorical perspective . The American Journal of Sociology , 88 ( 1 ), 1 – 23 .
Maurits Kaptein is an assistant professor at the Department of Methodology and Statistics at the Tilburg school of Social and Behavioral Sciences. Maurits is also Chief Scientist at PersuasionAPI.
Address : Department of Methodology and Statistics, Room P1.106. Tilburg University. PO Box 90153, 5000LE Tilburg, the Netherlands.
Deonne Castaneda is a User Experience Designer in California. She received an M.S. in Computer Science and a B.A. in Sociology from Stanford University.
Address : Department of Computer Science, 353 Serra Mall, Stanford, CA 94305
Nicole Fernandez is a User Experience Researcher at Google in New York. She received her Masters in Human-Computer Interaction from Carnegie Mellon University and a B.S. in Symbolic Systems from Stanford University.
Address : Google Inc, 76 Ninth Avenue, 4 th Floor, New York, NY 10011
Clifford Nass is the Thomas M. Storke Professor at Stanford University; he has been a professor at Stanford since 1986. His primary appointment is in Communication, but he also has appointments by courtesy in Computer Science, Education, Law, and Sociology, and is affiliated with the programs in Science, Technology, and Society and Symbolic Systems (cognitive science).
Address : Department of Communication Room 300E, McClatchy Hall Stanford University Stanford, CA. 94305–2050, USA.
Author notes
Email alerts, citing articles via.
- Recommend to Your Librarian
- Advertising and Corporate Services
Affiliations
- Online ISSN 1083-6101
- Copyright © 2024 International Communication Association
- About Oxford Academic
- Publish journals with us
- University press partners
- What we publish
- New features
- Open access
- Institutional account management
- Rights and permissions
- Get help with access
- Accessibility
- Advertising
- Media enquiries
- Oxford University Press
- Oxford Languages
- University of Oxford
Oxford University Press is a department of the University of Oxford. It furthers the University's objective of excellence in research, scholarship, and education by publishing worldwide
- Copyright © 2024 Oxford University Press
- Cookie settings
- Cookie policy
- Privacy policy
- Legal notice
This Feature Is Available To Subscribers Only
Sign In or Create an Account
This PDF is available to Subscribers Only
For full access to this pdf, sign in to an existing account, or purchase an annual subscription.
Empirical studies of the “similarity leads to attraction” hypothesis in workplace interactions: a systematic review
- Open access
- Published: 16 January 2023
- Volume 74 , pages 661–709, ( 2024 )
Cite this article
You have full access to this open access article
- Zoleikha Abbasi ORCID: orcid.org/0000-0002-8803-2239 1 ,
- Jon Billsberry ORCID: orcid.org/0000-0002-3015-4196 1 &
- Mathew Todres ORCID: orcid.org/0000-0001-6496-7809 1
7380 Accesses
2 Citations
Explore all metrics
Although the similarity-attraction hypothesis (SAH) is one of the main theoretical foundations of management and industrial/organizational (I/O) psychology research, systematic reviews of the hypothesis have not been published. An overall review of the existing body of knowledge is therefore warranted as a means of identifying what is known about the hypothesis and also identifying what future studies should investigate. The current study focuses on empirical workplace SAH studies. This systematic review surfaced and analyzed 49 studies located in 45 papers. The results demonstrate that SAH is valid in organizational settings and it is a fundamental force driving employees’ behavior. However, the force is not so strong that it cannot be overridden or moderated by other forces, which includes forces from psychological, organizational, and legal domains. This systematic review highlights a number of methodological issues in tests of SAH relating to the low number of longitudinal studies, which is important given the predictive nature of the hypotheses, and the varying conceptualizations of attraction measurement.
Similar content being viewed by others
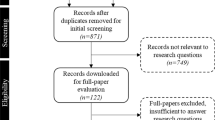
Job flourishing research: A systematic literature review
Construct overlap between employee engagement and job satisfaction: a function of semantic equivalence.
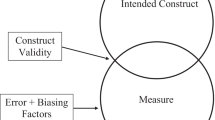
Are Biasing Factors Idiosyncratic to Measures? A Comparison of Interpersonal Conflict, Organizational Constraints, and Workload
Avoid common mistakes on your manuscript.
1 Introduction
Individuals are positively inclined towards people who are similar to themselves. This simple but striking assertion underpins the similarity-attraction hypothesis (SAH), which frames much relationship and interpersonal attraction research (e.g., Byrne 1971 ; Montoya and Horton 2013 ). According to Byrne ( 1971 ), when people perceive themselves to be similar to other people, they experience positive feelings of attraction towards them. These similarities cover a large number of factors typically separated into demographic (e.g., race, gender, ethnicity, socio-economic background, and age) and psychological (e.g., personality, values, interests, religion, education, and occupation) divisions. Many studies have shown that similarities in these various forms lead to friendships and other close relationships (e.g., Graziano and Bruce 2008 ; Kleinbaum et al. 2013 ; McPherson et al. 2001 ; Riordan 2000 ).
In work settings, SAH is a double-edged sword. On the one hand, it is a fundamental human drive that underpins effective social interaction in workplaces (e.g., McPherson et al. 2001 ; Montoya and Horton 2012 ), but on the other hand, it can lead to affinity or similarity bias and exclude those unlike the people making decisions (e.g., Björklund et al. 2012 ; Coates and Carr 2005 ; Hambrick 2007 ; O’Reilly et al. 2014 ; Sacco et al. 2003 ). To combat such ‘natural’ biases, most countries have passed laws to protect employees and potential employees who are dissimilar to those currently employed by organizations and who wield considerable power to decide who can enter organizations, who gets promoted, and how people are treated at work. Hence, within organizational settings, there is an eternal conflict at the heart of this field study; a conflict between natural human processes and natural justice.
In the mid 1990s, two reviews of work-related SAH appeared. An unpublished paper by Alliger et al. ( 1993 ) reviewed SAH in the context of personnel selection decision and work relations. Pierce et al. ( 1996 ) reviewed the hypothesis through the lens of romance in the workplace. There do not appear to be any more recent reviews and no broad-sweep reviews of SAH in the workplace. This paper makes a contribution by conducting such a study with the goal of reviewing extant knowledge on SAH in the workplace. Prior to a detailed account of the methods used for our systematic review, we first contextualize the current study by discussing relevant concepts and their evolution over time, namely the SAH and attraction, respectively. In our findings section, we review the cluster of studies providing empirical support for SAH, draw attention to measurement-design issues, and look at the study of SAH during the distinct organizational phases of recruitment and selection, employment, and organizational exit. In the discussion, we further the contribution of the paper with an examination of the paradox of similarity effects in an age when diversity and inclusion are prime considerations for organizations. We also draw out methodological challenges in the extant SAH in the workplace literature.
2 Similarity-attraction hypothesis
Although scientific focus on SAH gathered steam in the 1950s and 1960s (e.g., Byrne 1961 ; Festinger et al. 1950 ; Newcomb 1961 ; Walster et al. 1966 ), it has been studied for much longer. The relation between similarity and interpersonal attraction was mentioned as early as 1870 by Sir Francis Galton, who observed that illustrious men married illustrious women. Terman ( 1938 ) demonstrated that the greater the similarity between husband and wife, the more successful the marriage.
In the 1950s and 1960s, SAH studies focused on the interpersonal space and the role of attribution in attraction. In one of the first examples, Newcomb ( 1961 ) analyzed the establishment of friendships between new students at a college residence. He recorded students’ demographic information, attitudes, values, and beliefs and then measured their interpersonal attraction to each other. He showed that similarity between the students was the main predictor of attraction amongst them. Taking an experimental approach, Byrne and his colleagues used a “bogus stranger” methodology, in which they varied the similarity of a perceiver’s attitudes to those of a stranger, and then quantified the liking of that stranger. In a series of studies (e.g., Byrne 1971 , 1997 ; Byrne and Blaylock 1963 ; Byrne and Clore 1967 ; Byrne and Nelson 1965 ), they demonstrated that attitude similarity delivered more liking of the target.
Byrne and Clore ( 1967 ) presented a reinforcement model to explain the positive relationship between similarity and attraction, in which similarity presents social validation of one’s views of the self and the world, thus helping to satisfy an individual’s needs. The positive influence that is caused by this need fulfillment becomes correlated with its source, namely the similar individual, and leads to their liking. As an alternative, dissimilarity jeopardizes epistemic needs (i.e., the desire for establishing understanding) as it challenges one’s views about the self and the world, and therefore stimulates negative affect that, in turn, becomes linked with the dissimilar person. Consequently, according to Byrne and Clore ( 1967 ), personal attraction is a conditioned reaction to the positive or negative effect that is created by an unconditioned similarity motivation.
While cognition was recognized as an essential aspect of shaping a judgment of another, the data indicated that the more attitudes individuals held in common with each other, the more attracted they were to the other person (Byrne 1971 , 1997 ). This explains why people are attracted to like-minded individuals. Motivation to find others who are similar may have something to do with keeping a person’s perspective coherent with what they already know; people struggle for guaranteed certainty in dealing with the world around them (Byrne et al. 1966 ). Drawing on Newcomb ( 1956 ), Byrne ( 1997 ) argues that a key reason explaining the repeated support for SAH is due to the interpersonal rewards that follow from attraction: “At its simplest level, […] people like feeling good and dislike feeling bad” (Byrne 1997 : 425). Further, assessments of similarity increase the validation of individuals’ values, which leads to attraction, harmony, and cooperation between individuals (Edwards and Cable 2009 ). Thus, the more similar people perceive themselves to be to each other, the more attractive they will be to each other.
Although most SAH studies have researched similarities in peoples’ attitudes, concluding that individuals are more attracted to people with whom they have many shared attitudes (Byrne 1961 ; Byrne et al. 1970 ; Kaptein et al. 2014 ), studies have found that actual similarity in external characteristics (e.g., age, hairstyle) is more predictive of attraction than similarity in psychological characteristics such as cleverness and confidence (Condon and Crano 1988 ; Duck and Craig 1975 ; Montoya et al. 2008 ). Amongst the demographic attributes most commonly studied are age, education, ethnic background, religious affiliation (Gardiner 2022 ; Grigoryan 2020 ), and occupation (Bond et al. 1968 ; Heine et al. 2009 ; Singh et al. 2008 ). A possible explanation for this is that external abilities can be more easily identified and measured. Nevertheless, there is also support for the SAH from studies of psychological similarity. These studies demonstrate that people are attracted to others on the perceived basis of shared attitudes (Newcomb 1961 ; Tidwell et al. 2013 ), personality traits (Griffitt 1966 ; Klohnen and Luo 2003 ), and values (Cable and DeRue 2002 ).
In addition to studies measuring the impact of actual similarity, scholars have also looked at the impact of perceived similarity. Many of these studies show that perceived similarities are better predictors of attraction than real similarities (Condon and Crano 1988 ; Montoya et al. 2008 ), but the impact of actual similarity–attraction is mainly restricted to interactions with associates or impressions of “bogus strangers” in laboratory settings (Sunnafrank 1992 ). Montoya et al. ( 2008 ) found a significant impact of actual similarity on attraction, while the strength of the attraction is highly connected to the interaction of the participants and targets (e.g., romantic partner, confederate, bogus stranger); these findings are typically interpreted to mean that demographic similarity information is a single source of information and that over time other information becomes more important than similarity-derived information.
The positive association of similarity to attraction can be explained by social cognition theory (Bandura 1991 ). According to this theory, people make sense of the world around them by gathering information in suitable cognitive classes or conceptual memory bins. This theory highlights how the role played by the categories of schemas, prototypes, and stereotypes biases decision-making reducing the accuracy and objectiveness of judgments (Fiske and Taylor 2008 ). For example, when people perceive someone who has grown up in the same neighborhood as themselves (same sport and school), it results in approval of them as they match a well-formed and well-understood stereotype in the person’s mind. The same person might be much less comfortable with someone from a different racial group or who comes from a completely different area since the appropriate cognitive ‘bin’ is still very immature and might even be distorted due to a few random encounters with similar stimuli. According to social cognition theory, people judge others not based on individual qualities, but rather on the stereotype held regarding that individual’s group membership (Kulik and Bainbridge 2006 ). Self-categorization theory builds on social cognition theory. It says that people assign people to ingroup and outgroup membership of prototypes. People are not thought of as unique individuals but rather as expressions of the relevant prototype, which is a process of depersonalization. Self-categorization theory suggests people classify their membership internally for themselves and others based on socially defined qualities (Turner and Chelladurai 2005 ). Individuals identify themselves and others into in-group and out-group (Hogg and Terry 2000 ) according to their preconceived notions of fit based on the following amongst other things: gender, age, race, organizational membership, and status (Turner and Chelladurai 2005 ).
3 Attraction
One of the vaguer elements in SAH is the definition of the word “attraction’. In the 1500s, the word ‘attraction’ was a medical phrase referring to the body’s tendency to absorb fluids or nourishment (Oxford English Dictionary 2013). Over time, the meaning of this word changed to the ability for an object to draw an object to itself, and then to the capability of a person to draw another person to him or her (Montoya and Horton 2014 ). Although there are a few studies that explore different definitions of the word, there is considerable variation in how it is conceptualized in SAH studies. Some studies emphasize the behavioral dimension (i.e., “drawing one to another,” Schachter 1959 ), other scholars highlight the emotion and affection (i.e., feeling positive towards another; e.g., Zajonc 1968 ), while others stress cognitive aspects (i.e., inferring positive attributes; e.g., Singh et al. 2007 ). Despite the variety, these all have positive connotations (Berscheid 1985 ; Huston and Levinger 1978 ). More recently, the literature has focused on the definition of attraction as an attitude, but limited the definition to an individual’s direct and positive emotional and/or behavioral response to a specific person (Montoya and Horton 2014 ). In this approach, the definition of attraction concentrates on the quality of someone’s emotional reaction to another person and a behavioral element that shows an individual’s tendency to act in a specific way to another (i.e., choosing to move closer to them). According to Montoya and Horton ( 2014 : 60), “attraction is a person’s immediate and positive affective and/or behavioral response to a specific individual, a response that is influenced by the person’s cognitive assessments”. According to this point of view, the cognitive component is not considered part of attraction, but rather a process of forecasting an attraction reaction (Montoya et al. 2018 ).
Hence, the attraction literature has evolved different terms to describe the various attraction elements: affective attraction, behavioral attraction, and interpersonal attraction (or liking), Footnote 1 among other terms (Montoya and Horton 2020 ). Montoya et al. ( 2018 ) applied behavioral attraction to refer entirely to a self-reported preference for a certain behavioral reaction by using liking and interpersonal attraction to refer to an undifferentiated positive evaluation that comprises both affective and behavioral attraction. Reinforcing variation in definition of the word ‘attraction’, Montoya and Horton ( 2020 ) define attraction as an emotion that ranges from the professional, to the romantic, to the familial, meaning that attraction can be operationalized as an emotion in a wide range of settings (Montoya and Horton 2020 ).
4 Methodology
The goal of this study (Kuckertz and Block 2021 ) is to provide an overview of research findings on SAH as they relate to the workplace and, in particular, how they relate to interactions between people at work. To do this, we conducted a systematic review of SAH adopting the PRISMA (i.e., Preferred Reporting Items for Systematic reviews and Meta-Analyses) approach (Caulley et al. 2020 ; O’Dea et al. 2021 ). We conducted a systematic review that looked for both empirical and theoretical papers published in refereed journals with JCR impact factors that investigate SAH. We only included papers written in English. As there had been no previous systematic reviews of SAH, no date limits were set.
4.1 Search methodology
4.1.1 inclusion criteria.
The following search terms were used to identify studies on SAH: ‘similarity-attraction’, ‘similarity attraction’, ‘similarity leads to attraction’, ‘similarity predicts attraction’, ‘similarity-interpersonal attraction’, ‘similarity to attraction’, ‘similarity/attraction’, ‘dissimilarity-repulsion’, ‘dissimilarity leads to repulsion’, ‘dissimilarity/repulsion’, ‘dissimilarity repulsion’, ‘Rosenbaum’s repulsion hypothesis’. Given the specific nature of these search terms, logical operators were used to find at least one of these terms in the titles, subjects, or abstracts of papers. For thoroughness, we included the reverse hypothesis, dissimilarity leads to repulsion (Rosenbaum 1986 ), in our search.
4.1.2 Exclusion criteria
As we employed relatively complex search strings such as ‘similarity-attraction’, exclusion criteria were not needed at the subject level.
4.1.3 Databases searched
The following databases were searched for articles on SAH: PsycInfo and PsycArticles, Web of Science, and Business Search Complete.
4.2 Search results
Table 1 presents the results of the initial trawl of the databases and shows how these 880 articles were filtered. Since the search yielded articles published before the onset of journal rankings and some journals have subsequently merged or ceased publishing, there were papers in the database that would have been excluded because of the evolution of the journal rather than due to their own inherent qualities. To avoid this, we decided to include any paper in a journal without a JCR impact factor that had been published before 2000 and had received 100 or more citations on Google Scholar. This resulted in five additional papers that otherwise would have been excluded from the dataset.
The final stage of filtering was to eliminate papers not located in organizational, business, or management settings in which similarity comparisons were based on staff-staff, staff-team members, staff-leaders, staff-managers, or staff-supervisors. This led to the removal of a further 275 studies and 8 more which were not empirical. 45 studies emerged containing four papers that featured two separate studies. The final database for this systematic review is therefore 45 papers containing 49 separate studies. Figure 1 shows the chronological distribution of the 45 workplace SAH articles in the dataset.
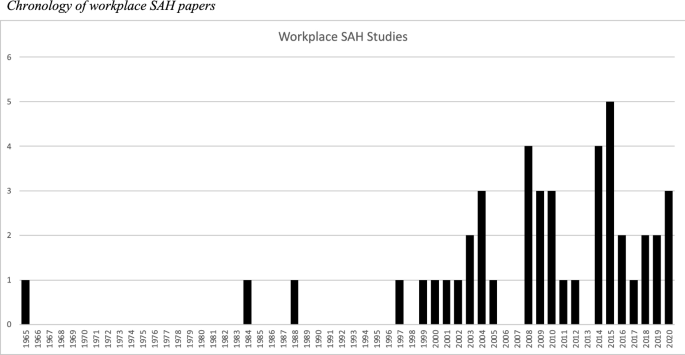
Chronology of workplace SAH papers
Descriptive details and brief summaries of the 49 employee SAH studies surfaced in this systematic review are presented in Tables 3 , 4 , 5 , 6 , 7 and 8 . They are placed in one of three categories. A total of 22 articles explore demographic similarity (Tables 2 and 3 ), 20 studies in 17 papers study psychological similarity (Tables 4 and 5 ), and seven studies in six papers investigate both psychological and demographic similarity (Tables 6 and 7 ). Demographic similarity involves the comparison of surface-level characteristics, such as gender, age, and race. They are permanent, usually observable, and easily measured (Harrison et al. 1998 ; Jackson et al. 1991 ). Psychological similarity involves deep-level attributes such as values, personality, attitudes, and beliefs (e.g., Engle and Lord 1997 ) and tend to be measured through direct assessment of self-reported perceptions.
5.1 Support for SAH
Just over half of the studies in the dataset yield empirical findings broadly in line with SAH predictions. These breakdown as follows: 11 demographic, 11 psychological, and 4 combined studies. In the demographic studies, similarity in gender, race, age, educational level, political affiliation have all been shown repeatedly to (1) positively associate with trust, job satisfaction, affective commitment, and in-role and extra-role performance, and selection decisions, and (2) negatively associate with staff turnover and related exit outcomes. In the psychological similarity studies, a matching pattern of results can be observed when similarity involves personality, emotional intelligence, and leadership and cognitive style. Such studies demonstrate that the SAH applies as much in organizational settings as it does in other settings. Rather, it is the studies that produce contradictory and asymmetric results that provide a more nuanced understanding of how SAH applies in these settings, particularly in studies of demographic similarity.
For example, Geddes and Konrad ( 2003 ) demonstrated a complex set of results in terms of race and performance feedback. They showed that both white and black employees responded more favorably to performance feedback from white managers demonstrating that SAH interacts with effects from other theories; in this case, status characteristics theory (Ridgeway 1991 ; Ridgeway and Balkwell 1997 ; Webster and Hysom 1998 ). Goldberg ( 2005 ) demonstrated a contrarian finding. Both male and female interviewers favored applicants on the opposite sex, which is better explained by social identity theory (Gaertner and Dovidio 2000 ) than SAH. Chatman and O’Reilly ( 2004 ) demonstrated that women reported a greater likelihood of leaving homogenous groups (i.e., groups comprising members of the same gender) than men, suggesting that other factors are in-play such as status conflict (Carli and Eagly 1999 ; Pugh and Wahrman 1983 ). It seems that SAH may be an underlying and natural driver of human behavior, but it is not such a dominant force in organizational settings that it cannot be moderated or eliminated by alternative forces. Although studies have shown that competing forces can influence the emergence of SAH effects, research is needed to understand the causes, circumstances, and conditions that give rise to this submergence. When and why does the SAH not appear in organizational settings?
5.2 What is attraction?
In these studies, there is considerable variation in the ways that attraction has been defined and conceptualized. Examples of the three different definitions of attraction – affective attraction, behavioral attraction, and interpersonal attraction – could be found in the dataset. Very few of the ways in which attraction has been measured might be regarded as direct measurement of attraction. They may be influenced by attractiveness, but most constructs used as interpretations of attraction in these studies are implicit and (at least) one step away from direct and isolated measures of attractiveness. For example, constructs like affective and normative commitment, perceived trustworthiness of managers, reaction to performance feedback, and organizational citizenship behaviors (OCBs) may all be associated with feeling closer (affective attraction), moving closer (behavior attraction), or liking, but many other factors are simultaneously in play and at the very least require some explanation as to the reasons why and how they relate to attraction. Even the more direct conceptualizations like organizational attractiveness or selection outcomes are confounded by other factors such as the scant and managed information typically available during recruitment and selection processes (Billsberry 2007a ; Herriot 1989 ) and other factors influencing attractiveness choices, such as the need to find a job (Billsberry 2007b ). Overall, one of the biggest weaknesses in tests of SAH in organizational settings is the way that attractiveness has been conceived. An analysis of attraction conceptualizations can be found in Table 8 .
Another noteworthy feature of the constructs used to capture attraction is the infrequency of repetition. Other than selection outcomes (of various sorts), perceptions of leadership, and OCBs (which have quite a tenuous association with attraction as there are many and varied reasons why people might engage in extra-role activities), most of the other constructs feature in just one study, occasionally two. This creates a sense that the field is still in an exploratory mode scoping out relevant relationships. Replication studies would provide confidence in these original findings and bring robustness. Further, there is a danger that the inclusiveness of constructs to represent attraction risks reifying the term. A challenge with such reification is that “scholars unknowingly integrate findings from studies with inconsistent construct definitions, which can create serious threats to validity” (Lane et al. 2006 : 835). To avoid this, construct clarity is essential and an important precondition of theory testing (Fisher et al. 2021 ).
5.3 Measurement and design issues
Most work on SAH has been conducted outside of organizations. Our initial searches of these databases generated 880 journal articles of which only 45 were empirical studies in which the participants were workers and data was collected from them or about them. At the fringes of eligibility, we included analyses of the gender composition of top management teams (TMTs) and members of unions. We also included policy capturing studies that involve employees undertaking some type of experiment to find out what they would do in circumstances relevant to their jobs. But we excluded student samples even when they were performing a work-related task as students are too far detached from the reality of work. Our sample was also limited to studies that set out to examine the SAH rather than studies that adopted a SAH to test other relationships, such as value congruence studies (e.g., Cable and DeRue 2002 ). As such, the studies in the present paper typically employed a design in which a similarity independent variable (IV) predicted (or, more commonly, as a result of the dominance of cross-sectional designs, was associated with) an attractiveness dependent variable (DV). This IV → DV relationship was predicted and used to design the empirical study, which was tested with a variation of it (i.e., different types of similarity or attraction variable), typically with moderators or mediators. As such, similarity is viewed as the fundamental driver of effects and there is an absence of studies exploring why similarity is important to people in workplaces.
This dataset is dominated by cross-sectional (13 demographic (59%), 11 psychological (55%), and 4 combined (57%)) studies, which is counter-intuitive given the inherently predictive nature of the SAH (i.e., similarity leads to attraction). Testing the IV and DV at the same time makes the predictive element of the hypothesis unproven. Cross-sectional designs can, at best, show an association of the two constructs and only imply a predictive relationship (Kraemer et al. 2000 ). To get around the confounds in cross-sectional designs, researchers have adopted (1) experimental designs (2 demographic (9%) and 6 psychological (30%)) that capture policy intentions of organizational members, (2) archival studies (6 demographic (27%), 1 psychological (5%), and 1 combined (14%)), which can capture the historical effects on staff turnover and recruitment of similarity, and (3) longitudinal designs (1 demographic similarity, 2 psychological similarity, and 1 combined study). The low number of longitudinal studies in this field clearly presents an opportunity for future research. Such studies could validate the findings of cross-sectional studies, test the predictive nature of the similarity → attraction relationship, and explore the influence of other factors upon it. In addition, longitudinal studies adopting repeated measure methodologies could test for bidirectional effects and look at the strength and duration of the effect; this latter point is important given that Rosenfeld and Jackson ( 1965 ) reported that personality similarity only influenced friendship in the 1st year of acquaintance suggesting that SAH influences the initiation of attraction, but not its long-term survival.
Almost all of the demographic similarity studies in this review operationalize their similarity variables in very stark categorical terms. Gender is male or female; race is skin color and/or ethnographic background. Similarity or difference is calculated as being on one of the predetermined categories. These singular categorizations present a simple view of gender, race, and other demographic similarities. An alternative approach is intersectionality (Crenshaw 1990 ), which argues that different aspects of a person’s identity intersect to influence behavior towards them. For example, to treat Black women like White women ignores many socio-cultural factors disadvantaging them (Wilkins 2012 ). Intersectional analysis could take demographic similarity into a cultural and political space where the purpose of studying demographic SAH is to highlight privilege, disadvantage, and discrimination (Tatli and Özbilgin 2012 ).
5.4 Recruitment and selection
Perhaps the greatest influence of SAH in organizational settings is in explaining recruitment and selection decisions. Various scholars (e.g., Riordan 2000 ; Schneider 1987 ) have argued that applicants are more attracted to, and more expected to choose to work for, an organization whose workforce has features similar to their own. For example, people may be more attracted to a company that recruits a group of employees who are racially like them, predicting that these workers share their values and attitudes (Avery et al. 2004 ). These predictions have been demonstrated to be accurate in several studies in this review. Roth et al. ( 2020 ) showed that candidates’ perceived similarity of their political affiliation influences employment decision-makers, which eventually resulted in liking and organizational citizenship behavior performance (i.e., an employee’s voluntary commitment to the organization beyond his/her contractually obligated tasks). Kacmarek et al. ( 2012 ) found that greater female presence on nominating committees subsequently led to increased female representation on company boards. Chen and Lin ( 2014 ) showed that recruiters’ perceptions of applicants’ similarity to them influenced selection decisions. However, some asymmetric findings have emerged. For example, while Goldberg ( 2005 ) demonstrated race similarity effects, she also noted that male recruiters have a preference for female applicants. But, by and large, these studies align with findings in the general recruitment and selection literature reporting that organizational recruiters favor applicants resembling themselves and this reinforces opportunity in organizations to groups of people who already enjoy employment positions there (Bye et al. 2014 ; Kennedy and Power 2010 ; Noon 2010 ; Persell and Cookson 1985 ; Rivera 2012 ). As a consequence, many countries have passed legislation to protect those disadvantaged by these processes, although such legislation mainly confines itself to surface-level demographic factors such as gender, race, and disability. Hence, the application of SAH to recruitment situations is challenging as it separates demographic and psychological similarity and is located behind a legal mask that probably suppresses its appearance.
5.5 Post-hire
During employment, SAH presents a paradox for organizations. On the one hand, employees like working with people like themselves and are more productive (Bakar and McCann 2014 ; Chatman and O’Reilly 2004 ). But, on the other hand, it creates employee homogeneity, which Schneider ( 1987 ) argues causes organizations to occupy a self-defeating and increasingly narrow ecological niche, and crystalizes disadvantage and discrimination (Dali 2018 ). Empirical studies included in this review demonstrate both sides of this paradox. Interestingly though, looking across the demographic and psychological similarity studies separately, we gained a sense that demographic similarity worked in the initial phases of relationships to bring people together (e.g., Rosenfeld and Jackson 1965 ) and during short engagements (e.g., during recruitment and selection and loan decisions) when demographic similarity aids decision-making when there is impoverished information. Psychological similarity works over more prolonged periods and therefore comes more to the fore in during long-term employment (e.g., Marchiondo et al. 2018 ; Sears and Holmvall, 2010 ).
One noticeable absence in the studies captured in this review are those that explore value congruence (e.g., Adkins et al. 1994 ; Billsberry 2007b ; Chatman 1989 , 1991 ; Meglino et al. 1992 ; Yu and Verma 2019 ). These studies compare the similarity of aspects of people at work, most typically work values, and explore the consequences. Work value congruence has been shown to lead to positive organizational outcomes such as job satisfaction, organizational commitment, decrease employee conflict and negatively related to intentions to leave and organizational exit (Hoffman and Woehr 2006 ; Jehn 1994 ; Jehn et al. 1997 , 1999 ; Kristof-Brown and Guay 2011 ; Subramanian et al. 2022 ; Verquer et al. 2003 ), thereby supporting SAH. Conversely, value incongruence is associated with distancing outcomes such as feelings of not belonging or being unfulfilled, and organizational exit (Edwards and Cable 2009 ; Edwards and Shipp 2007 ; Follmer et al. 2018 ; Kristof-Brown et al. 2005 ; Vogel et al. 2016 ) thereby supporting DRH leading Abbasi et al. ( 2021 ) to suggest that value congruence and value incongruence, and therefore SAH and DRH, are two different forces. The non-appearance of these studies in the current review appears to stem from the way these value congruence studies are theoretically justified. Rather than being direct tests of SAH, they are one step removed and based on ideas of person-environment (PE) fit and person-organization (PO) fit. These theories are themselves grounded in SAH (e.g., Chatman 1989 ; Schneider 1987 ), but the field is sufficiently well developed as a branch of PE and PO fit that it is not necessary to refer back to the conceptual roots of the SAH. This is likely to be the case for many others forms of congruence and incongruence such as political ideology incongruence (e.g., Bermiss and MacDonald 2018 ) or personality congruence (Schneider et al. 1998 ).

5.6 Organizational exit
Perhaps the biggest surprise in this dataset is the almost complete absence of any SAH studies exploring the organizational exit phase of work as voluntary decisions to leave organizations is one of the clearest examples of employees’ behavioral distancing (i.e., moving apart). Furthermore, there is theory arguing (e.g., Schneider 1987 ) that when employees feel dissimilar to others, they leave organizations. As mentioned above, there are many studies in the value congruence, PE fit, and PO fit literatures that explore the effect of dissimilarity on organizational exit, but these are not positioned as direct tests of SAH and so did not appear in this review.
6 Discussion
This systematic review has shown that, by and large, SAH holds true in organizational settings; similarity leads to attraction. There are some contrarian and asymmetrical findings, but these typically occur when other theories interact with SAH. An example comes from Gaertner and Dovidio ( 2000 ) who showed that both male and female interviewers favored applicants of the opposite sex, which is better explained by social identity theory. Further, legal sanctions can influence the appearance of SAH during recruitment, selection, and other episodes. Consequently, SAH can be viewed as a strong underlying force driving employees’ natural behavior, but it is not so strong that it cannot be overcome by other forces.
In organizational settings, paradox surrounds the application of SAH. People have a natural tendency to want to be with people like themselves and, without other influence, will choose to recruit people like themselves. Moreover, they prefer working with people like themselves and are more productive doing so. But such behavior can be exclusionary, discriminatory, and inequitable. Most countries have laws protecting many different categories of people from disadvantageous behavior for this reason. Further, many, perhaps most, organizations have espoused values and adopt policies of equal opportunity and these policies police formal selection processes, performance appraisals, and promotion practices, and informal behavior between employees. Neo-normative organizations go further and espouse values celebrating diversity and inclusivity (Husted 2021 ). In such organizations, employees are encouraged to “just be yourself” (Fleming and Sturdy 2011 : 178), although this does not extend to their natural tendency to want to associate with people like themselves and strict rules exist to ensure compliance (Fleming and Sturdy 2009 ). So, there are many rules and regulations in organizations protecting those who might be disadvantaged from people’s natural tendency to be attracted to people similar to themselves.
Organization-level analysis is missing from the studies included in this systematic review. Instead, all the studies are designed as individual-level studies where data for both IVs and DVs are gathered from or about individuals and the impact of similarity or dissimilarity for them. This aligns with traditions in industrial/organizational psychology (Schneider and Pulakos 2022 ), but although it sheds light on individual differences, it fails to explore the ramifications of similarity and dissimilarity for organizations. This is not an insignificant omission as theoretical work by Schneider ( 1987 ) argues that the similarity-attraction hypothesis is a powerful force creating and reinforcing the cultures of organizations that explains why organizations are different to each other even when in the same industry and location. Further, exploring the impact of individual-level processes at the level of the organization can test assumptions about the importance of individual-level effects for the organization. As Schneider and Pulakos ( 2022 : 386) state, “[t]he problem lies in our tendency to assume that the characteristics that produce high-performing individuals and teams also yield high-performing organizations, without testing this as often as we could or should.” In short, the assumption that effects at the individual-level lead to effects at the organization-level is an ecological fallacy and there is a need to test the organizational impact, if any, of individual-level findings.
Similarly, this systematic review did not include any empirical studies of the dissimilarity-repulsion hypothesis (DRH; Rosenbaum 1986 ). Relevant search terms were included, but no empirical studies of DRH in workplaces were found. One possible explanation accounting for this gap is that researchers may have presumed that since this theory is the polar opposite of similarity-attraction theory, low similarly-attraction means high dissimilarity repulsion, so the testing of both hypotheses may have been viewed as unnecessary. Alternatively, the low number of studies of organizational exit, particularly voluntary turnover, the most natural and powerful repulsion outcome in organizational settings, in this dataset constrains the appearance of the DRH. Organizational exit is a large literature and dissimilarity and misfit are known to be drivers of these actions (e.g., Doblhofer et al. 2019 ; Jackson et al. 1991 ; Kristof-Brown et al. 2005 ), so the suggestion is, like with value congruence, that these studies are grounded on theories other than DRH. Another interesting problem with DRH is the use of the term repulsion, which hints at “abhorrence, loathing, disgust and hatred” (Abbasi et al. 2021 : 9). In times when there is a strong skew towards positive psychology (Kanfer 2005 ), examining the darker side of organizational life is much less common. Nevertheless, these are the moments that have the most impact on people and deserve much greater scholarly attention.
In addition to the above, this systematic review has surfaced several key avenues for future research related to methodological advancement. The first notable weakness in the empirical papers reviewed on this topic is the absence of studies adopting a longitudinal design. This is particularly noteworthy because the similarity leads to attraction hypothesis is inherently predictive in nature. Cross-sectional studies can give a sense of associations between similarity and attraction constructs, but do not convince when applied to predictive hypotheses. Some authors (e.g., Bruns et al. 2008 ; Eagleson et al. 2000 ; Young et al. 1997 ) have circumvented this issue by adopting policy-gathering designs in which respondents give an opinion about what they would do in a particular situation, but the findings of such studies would carry more weight if they were followed up with empirical studies of what people actually do or did in such circumstances. In this literature, there is a tendency for each study to stand separate from the other studies almost as if each study was exploring the hypothesis in a particular aspect of workplaces for the first time. Such exploratory work is commendable, but there is a need for studies to replicate and integrate findings.
The second noticeable methodological weakness in these studies is the manner in which attraction outcomes have been defined. Decisions to join or leave organizations are the most obvious examples of behavioral attraction and repulsion (i.e., ‘moving to’ and ‘moving away’). However, even attraction and repulsion outcomes like these are rarely the exclusive consequence of similarity or dissimilarity, but they have the advantage of being clear movements into or out of organizations. Other behavioral outcomes used in these studies as measures of attraction include absenteeism, TMT homogeneity, diversity levels, advice-seeking, and small business loan decisions, which appear to show decreasing alignment to the notions of attraction or repulsion. The psychological interpretations of attraction are perhaps even further removed from direct definitions of the word. Many constructs have been used as the dependent variables ranging from job satisfaction, perceived PO fit, perceptions of the quality of LMX relationships, commitment to a trade union, reactions to incivility, and employee well-being. These are all important outcomes, but they are not necessarily capturing a sense of feeling closer to others. Only five studies in this sample collected attraction data based on interpersonal liking, relations, or friendship, which might be thought to be the most direct capture of attraction. As a result, the workplace SAH literature gives the impression of actually being a literature that has rigorously explored the impact of many different types of demographic and psychological similarity in organizations, but not necessarily in terms of how it predicts attraction and repulsion outcomes. Hence, there is a strong need for SAH studies that capture more direct measures of attraction and this could prove to be a fruitful avenue for future research.
Another methodological consideration centers on causation. All the studies reviewed in this review employed an IV predicts DV design where the IVs were a form of similarity or dissimilarity and the DVs were a form of attraction or repulsion. As the SAH was being tested, the natural assumption in these studies is that similarity itself is the driver of outcomes. This, of course, triggers questions about why similarity (or dissimilarity) might be driving outcomes. What is it about similarity that drives attraction outcomes? An unkind hypothesis might be that people are inherently racist, sexist, ageist, linguicist, and so forth, in which case management control strategies appear a natural response. A kinder hypothesis is that people feel less threat from people similar to themselves and have a greater sense of belonging amongst people similar to themselves, in which case strategies focused on improving integration appear appropriate. A more neutral hypothesis might be the similarity makes it easier to predict others’ behavior and brings expectations of reciprocity (Newcomb 1956 ), which would raise distributive justice concerns. At present, the SAH in the workplace literature talks little about why similarity drives attraction outcomes and instead tends to focus on whether it does lead to attraction. But as different organizational responses might be expected to follow different causations, it is important to explore the reasons why similarity (and dissimilarity) are having attraction (and repulsion) effects in workplaces.
6.1 Limitations
This systematic review was strictly limited to empirical studies that set out to test SAH (and DRH) in organizational settings. To be included in this systematic review, this intention needed to be stated in the title, abstract, or keywords through the mention of various words and phrases suggesting SAH. This approach means that there are likely to be many studies not included in this systematic review that might measure some form of human similarity and use it to predict some form of attraction. The most obvious examples are the various organizational fit literatures, the value congruence and incongruence literatures, the recruitment, selection, induction, and socialization literatures, and the organizational exit literature. In all these cases, there are theoretical foundations based on SAH or DRH, but these literatures take the SAH and DRH roots ‘as a given’ and have placed their own theoretical frameworks on them (Evertz and Süß 2017 ). For example, an empirical PO fit study, which has similarity assessments at its core, might refer to PE interactions as its theoretical base and not deconstruct this further to the underlying SAH. It seems a common theme in these fields; there is no longer feel the need to justify the study in terms of testing SAH or DRH and therefore these underpinning hypotheses are not mentioned in titles, abstracts, or keywords. Although considerable scholarly advantage would accrue from including these literatures in a systematic review of SAH and DRH, it would be a truly Herculean task as it would require reading literally thousands of papers without any search terms to guide the hunt for similarity IVs and attraction DVs.
7 Conclusion
This paper systematically reviewed empirical studies of the ‘similarity leads to attraction’ (SAH) and ‘dissimilarity leads to repulsion’ (DRH) hypotheses in organizational settings. A total of 49 studies in 45 separate papers were surfaced, which split roughly 50/50 into studies of demographic similarity and psychological similarity. The results of these studies confirm that SAH remains valid in organizational settings and that it is a fundamental force driving employees’ behavior. However, although similarity and dissimilarity drive attraction and repulsion outcomes, the force is not so strong that it cannot be overridden or moderated by other forces, which includes forces from psychological, organizational, and legal domains. This systematic review highlighted a number of methodological issues in tests of SAH relating to the low number of longitudinal studies, which is important given the predictive nature of the hypotheses, and the varying conceptualizations of attraction measurement. This study also demonstrated that paradox is at the heart of SAH in organizational settings.
Data availability
The datasets generated during and/or analysed during the current study are available from the corresponding author on reasonable request.
Code availability
The search terms used to gather data are detailed in the methodology section.
It should be noted that although Montoya and Horton ( 2020 ) categorize ‘liking’ as an element of interpersonal attraction, others have categorized it as an aspect of affective attraction. Differences relate to how ‘liking’ is conceptualized and measured in studies.
Avery DR, Hernandez M, Hebl MR (2004) Who’s watching the race? Racial salience in recruitment advertising. J Appl Soc Psychol 34:146–161. https://doi.org/10.1111/j.1559-1816.2004.tb02541.x
Article Google Scholar
*Bacharach SB, Bamberger PA (2004) Diversity and the union: the effect of demographic dissimilarity on members’ union attachment. Group Org Manag 29:385–418. https://doi.org/10.1177/1059601103257414
*Bagues MF, Esteve-Volart B (2010) Can gender parity break the glass ceiling? Evidence from a repeated randomized experiment. Rev Econ Stud 77:1301–1328. https://doi.org/10.1111/j.1467-937X.2009.00601.x
*Bakar HA, McCann RM (2014) Matters of demographic similarity and dissimilarity in supervisor–subordinate relationships and workplace attitudes. Int J Intercultl Relat 41:1–16. https://doi.org/10.1016/j.ijintrel.2014.04.004
Bandura A (1991) Social cognitive theory of self-regulation. Org Behav Human Decis Process 50:248–287. https://doi.org/10.1016/0749-5978(91)90022-l
*Bechtoldt MN (2015) Wanted: self-doubting employees—Managers scoring positively on impostorism favor insecure employees in task delegation. Pers Individ Differ 86:482–486. https://doi.org/10.1016/j.paid.2015.07.002
Bermiss YS, McDonald R (2018) Ideological misfit? Political affiliation and employee departure in the private-equity industry. Acad Manag J 61:2182–2209. https://doi.org/10.5465/amj.2016.0817
Berscheid ES (1985) Interpersonal attraction. In: Lindzey G, Aronson E (eds) Handbook of social psychology, vol 2. Random House, New York, pp 413–483
Google Scholar
Billsberry J (2007a) Experiencing recruitment and selection. Wiley, Chichester
Billsberry J (2007b) Attracting for values: an empirical study of ASA’s attraction proposition. J Manag Psychol 22:132–149. https://doi.org/10.1108/02683940710726401
Björklund F, Bäckström M, Wolgast S (2012) Company norms affect which traits are preferred in job candidates and may cause employment discrimination. J Psychol 146:579–594. https://doi.org/10.1080/00223980.2012.658459
Bond MH, Byrne D, Diamond MJ (1968) Effect of occupational prestige and attitude similarity on attraction as a function of assumed similarity of attitude. Psychol Rep 23:1167–1172. https://doi.org/10.2466/pr0.1968.23.3f.1167
*Bruns V, Holland DV, Shepherd DA, Woklund J (2008) The role of human capital in loan officers’ decision policies. Entrep Theor Pract 32:485–506. https://doi.org/10.1111/j.1540-6520.2008.00237.x
Bye HH, Horverak JG, Sandal GM, Sam DL, van de Vijver FJR (2014) Cultural fit and ethnic background in the job interview. Int J Cross Cult Manag 14:7–26. https://doi.org/10.1177/1470595813491237
Byrne D (1961) Interpersonal attraction and attitude similarity. J Abnorm Soc Psychol 62:713–715. https://doi.org/10.1037/h0044721
Byrne D (1971) The attraction paradigm. Academic Press, New York
Byrne D (1997) An overview (and underview) of research and theory within the attraction paradigm. J Soc Pers Relat 14:417–431. https://doi.org/10.1177/0265407597143008
Byrne D, Blaylock B (1963) Similarity and assumed similarity among husbands and wives. J Abnorm Soc Psychol 67:636–640. https://doi.org/10.1037/h0045531
Byrne D, Clore GL (1967) Effectance arousal and attraction. J Pers Soc Psychol 6:1–18. https://doi.org/10.1037/h0024829
Byrne D, Nelson D (1965) Attraction as a linear function of proportion of positive reinforcements. J Pers Soc Psychol 1:659–663. https://doi.org/10.1037/h0022073
Byrne D, Clore GL, Worchel P (1966) The effect of economic similarity-dissimilarity on interpersonal attraction. J Pers Soc Psychol 4:220–224. https://doi.org/10.1037/h0023559
Byrne D, Ervin CR, Lamberth J (1970) Continuity between the experimental study of attraction and real-life computing dating. J Pers Soc Psychol 16:157–165. https://doi.org/10.1037/h0029836
Cable DM, DeRue DS (2002) The convergent and discriminant validity of subjective fit perceptions. J Appl Psychol 87:875–884. https://doi.org/10.1037//0021-9010.87.5.875
Carli L, Eagly A (1999) Gender effects on social influence and emergent leadership. In: Powell GN (ed) Handbook of gender and work. Sage, Thousand Oaks, pp 203–222
Chapter Google Scholar
Caulley L, Cheng W, Catalá-López F, Whelan J, Khoury M, Ferraro J, Moher D (2020) Citation impact was highly variable for reporting guidelines of health research: a citation analysis. J Clin Epidemiol 127:96–104. https://doi.org/10.1016/j.jclinepi.2020.07.013
Chatman J (1989) Improving interactional organizational research: a model of person-organization fit. Acad Manag Rev 14:333–349. https://doi.org/10.5465/amr.1989.4279063
Chatman JA (1991) Matching people and organizations: selection and socialization in public accounting firms. Adm Sci Q 36:459–484. https://doi.org/10.2307/2393204
*Chatman JA, O’Reilly CA (2004) Asymmetric reactions to work group sex diversity among men and women. Acad Manag J 47:193–208. https://doi.org/10.5465/20159572
*Chen CC, Lin MM (2014) The effect of applicant impression management tactics on hiring recommendations: cognitive and affective processes. Appl Psychol Int Rev 63:698–724. https://doi.org/10.1111/apps.12013
Coates K, Carr SC (2005) Skilled immigrants and selection bias: a theory-based field study from New Zealand. Int J Intercult Relat 29:577–599. https://doi.org/10.1016/j.ijintrel.2005.05.001
Condon JW, Crano WD (1988) Inferred evaluation and the relation between attitude similarity and interpersonal attraction. J Pers Soc Psychol 54:789–797
Crenshaw K (1990) Mapping the margins: intersectionality, identity politics, and violence against women of color. Stan Law Rev 43:1241–1299. https://doi.org/10.2307/1229039
Dali K (2018) Culture fit as anti-diversity Avoiding Human Resources decisions that disadvantage the brightest. Int J Info Divers Incl 2:1–8. https://doi.org/10.33137/ijidi.v2i4.32199
*Den Hartog DN, De Hoogh AHB, Belschak FD (2020) Toot your own horn? Leader narcissism and the effectiveness of employee self-promotion. J Manag 46:261–286. https://doi.org/10.1177/0149206318785240
*Devendorf SA, Highhouse S (2008) Applicant-employee similarity and attraction to an employer. J Occup Org Psychol 81:607–617. https://doi.org/10.1348/096317907X248842
Doblhofer DS, Hauser A, Kuonath A, Haas K, Agthe M, Frey D (2019) Make the best out of the bad: coping with value incongruence through displaying facades of conformity, positive reframing, and self-disclosure. Eur J Work Org Psychol 28:572–593. https://doi.org/10.1080/1359432X.2019.1567579
*Doms M, zu Knyphausen-Aufseß D (2014) Structure and characteristics of top management teams as antecedents of outside executive appointments: a three-country study. Int J Human Resour Manag 25(22):3060–3085. https://doi.org/10.1080/09585192.2014.914052
Duck SW, Craig G (1975) Effects of type of information upon interpersonal attraction. Soc Behav Pers Int J 3:157–164. https://doi.org/10.2224/sbp.1975.3.2.157
*Eagleson G, Waldersee R, Simmons R (2000) Leadership behaviour similarity as a basis of selection into a management team. Br J Soc Psychol 39:301–308. https://doi.org/10.1348/014466600164480
Edwards JR, Cable DM (2009) The value of value congruence. J Appl Psychol 94:654–677. https://doi.org/10.1037/a0014891
Edwards J, Shipp A (2007) The relationship between person-organization fit and outcomes: An integrative theoretical framework. In: Ostroff C, Judge TA (eds) Perspectives on organizational fit. Jossey-Bass, San Francisco, pp 209–258
Engle EM, Lord RG (1997) Implicit theories, self-schemas, and leader-member exchange. Acad Manag J 40:988–1010. https://doi.org/10.2307/256956
Evertz L, Süß S (2017) The importance of individual differences for applicant attraction: a literature review and avenues for future research. Manag Rev Q 67:141–174. https://doi.org/10.1007/s11301-017-0126-2
Festinger L, Schachter S, Back K (1950) Social pressures in informal groups; a study of human factors in housing. Milbank Memorial Fund Q 30:384–387. https://doi.org/10.2307/3348388
Fisher G, Mayer K, Morris S (2021) From the editors—Phenomenon-based theorizing. Acad Manag Rev 46(4):631–639. https://doi.org/10.5465/amr.2021.0320
Fiske ST, Taylor SE (2008) Social cognition: From brains to culture, 3rd edn. McGraw-Hill, New York
Fleming P, Sturdy A (2009) “Just be yourself!: towards neo-normative control in organizations. Emp Relat 31:569–583. https://doi.org/10.1108/01425450910991730
Fleming P, Sturdy A (2011) ‘Being yourself’ in the electronic sweatshop: new forms of normative control. Human Relat 64:177–200. https://doi.org/10.1177/0018726710375481
Follmer EH, Talbot DL, Kristof-Brown AL, Astrove SL, Billsberry J (2018) Resolution, relief, and resignation: a qualitative study of responses to misfit at work. Acad Manag J 61:440–465. https://doi.org/10.5465/amj.2014.0566
Gaertner SL, Dovidio JF (2000) Reducing intergroup bias: The common ingroup identity model. Psychology Press, Philadelphia
Gardiner E (2022) What’s age got to do with it? The effect of board member age diversity: a systematic review. Manag Rev Q. https://doi.org/10.1007/s11301-022-00294-5
*Geddes D, Konrad AM (2003) Demographic differences and reactions to performance feedback. Human Relat 56:1485–1513. https://doi.org/10.1177/00187267035612003
*Georgiou G (2017) Are oral examinations objective? Evidence from the hiring process for judges in Greece. Eur J Law Econ 44:217–239. https://doi.org/10.1007/s10657-016-9545-0
*Goldberg CB (2005) Relational demography and similarity-attraction in interview assessments and subsequent offer decisions - Are we missing something? Group Org Manag 30:597–624. https://doi.org/10.1177/1059601104267661
*Graves LM, Powell GN (1988) An investigation of sex discrimination in recruiters’ evaluations of actual applicants. J Appl Psychol 73:20–29. https://doi.org/10.1037/0021-9010.73.1.20
Graziano WG, Bruce JW (2008) Attraction and the initiation of relationships: A review of the empirical literature. In: Sprecher S, Wenzel A, Harvey J (eds) Handbook of relationship initiation. Psychology Press, Philadelphia, pp 275–301. https://doi.org/10.4324/9780429020513-24
Griffitt WB (1966) Interpersonal attraction as a function of self-concept and personality similarity-dissimilarity. J Pers Soc Psychol 4:581–584. https://doi.org/10.1037/h0023893
Grigoryan L (2020) Perceived similarity in multiple categorization. Appl Psychol Int Rev 69:1122–1144. https://doi.org/10.1111/apps.12202
Hambrick DC (2007) Upper echelons theory: an update. Acad Manag Rev 32:334–343. https://doi.org/10.5465/amr.2007.24345254
Harrison DA, Price KH, Bell MP (1998) Beyond relational demography: time and the effects of surface- and deep-level diversity on work group cohesion. Acad Manag J 41:96–107. https://doi.org/10.5465/256901
Heine SJ, Foster JAB, Spina R (2009) Do birds of a feather universally flock together? Cultural variation in the similarity-attraction effect. Asian J Soc Psychol 12:247–258. https://doi.org/10.1111/j.1467-839x.2009.01289.x
Herriot P (1989) Selection as a social process. In: Smith M, Robertson IT (eds) Advances in selection and assessment. Wiley, Chichester, pp 171–187
Hoffman B, Woehr D (2006) A quantitative review of the relationship between person–organization fit and behavioral outcomes. J Vocat Behav 68:389–399. https://doi.org/10.1016/j.jvb.2005.08.003
Hogg MA, Terry DJ (2000) Social identity and self-categorization processes in organizational contexts. Acad Manag Rev 25:121–140. https://doi.org/10.5465/amr.2000.2791606
Husted E (2021) Alternative organization and neo-normative control: notes on a British town council. Cult Org 27:132–151. https://doi.org/10.1080/14759551.2020.1775595
Huston TL, Levinger G (1978) Interpersonal attraction and relationships. Annu Rev Psychol 29:115–156. https://doi.org/10.1146/annurev.ps.29.020178.000555
Jackson SE, Brett JF, Sessa VI, Cooper DM, Julin JA, Peyronnin K (1991) Some differences make a difference: individual dissimilarity and group heterogeneity as correlates of recruitment, promotions, and turnover. J Appl Psychol 76:675–689. https://doi.org/10.1037/0021-9010.76.5.675
*Jaiswal A, Dyaram L (2019) Towards well-being: role of diversity and nature of work. Emp Relat 41:158–175. https://doi.org/10.1108/ER-11-2017-0279
Jehn KA (1994) Enhancing effectiveness: an investigation of advantages and disadvantages of value-based intragroup conflict. Int J Confl Manag 5:223–238. https://doi.org/10.1108/eb022744
Jehn KA, Chadwick C, Thatcher SM (1997) To agree or not to agree: The effects of value congruence, individual demographic dissimilarity, and conflict on workgroup outcomes. Int J Confl Manag 8:287–305. https://doi.org/10.1108/eb022799
Jehn KA, Northcraft GB, Neale MA (1999) Why differences make a difference: a field study of diversity, conflict, and performance in workgroups. Adm Sci Q 44:741–763. https://doi.org/10.2307/2667054
*Jiang CX, Chua RYJ, Kotabe M, Murray JY (2011) Effects of cultural ethnicity, firm size, and firm age on senior executives’ trust in their overseas business partners: evidence from China. J Int Bus Stud 42:1150–1173. https://doi.org/10.1057/jibs.2011.35
*Kaczmarek S, Kimino S, Pye A (2012) Antecedents of board composition: the role of nomination committees. Corpor Gov Int Rev 20:474–489. https://doi.org/10.1111/j.1467-8683.2012.00913.x
Kanfer R (2005) Self-regulation research in work and I/O psychology. Appl Psychol Int Rev 54:186–191. https://doi.org/10.1111/j.1464-0597.2005.00203.x
Kaptein M, Castaneda D, Fernandez N, Nass C (2014) Extending the similarity-attraction effect: the effects of when-similarity in computer-mediated communication. J Comput Med Commun 19:342–357. https://doi.org/10.1111/jcc4.12049
Kennedy M, Power MJ (2010) The smokescreen of meritocracy: elite education in Ireland and the reproduction of class privilege. J Crit Educ Policy Stud 8:222–248
*Klein KJ, Lim BC, Saltz JL, Mayer DM (2004) How do they get there? An examination of the antecedents of centrality in team networks. Acad Manag J 47:952–963. https://doi.org/10.5465/20159634
Kleinbaum AM, Stuart TE, Tushman ML (2013) Discretion within constraint: homophily and structure in a formal organization. Org Sci 24:1316–1336. https://doi.org/10.1287/orsc.1120.0804
Klohnen EC, Luo S (2003) Interpersonal attraction and personality: what is attractive-self similarity, ideal similarity, complementarity or attachment security? J Pers Soc Psychol 85:709–722. https://doi.org/10.1037/0022-3514.85.4.709
Kraemer HC, Yesavage JA, Taylor JL, Kupfer D (2000) How can we learn about developmental processes from cross-sectional studies, or can we? Am J Psychiatry 157:163–171. https://doi.org/10.1176/appi.ajp.157.2.163
Kristof-Brown AL, Guay RP (2011) Person-environment fit. In: Zedeck S (ed) APA handbook of industrial and organizational psychology, vol 3. American Psychological Association, Washington DC, pp 3–50. https://doi.org/10.1037/12171-001
Kristof-Brown AL, Zimmerman RD, Johnson EC (2005) Consequences of individuals’ fit at work: a meta-analysis of person-job, person-organization, person-group, and person-supervisor fit. Pers Psychol 58:281–342. https://doi.org/10.1111/j.1744-6570.2005.00672.x
Kuckertz A, Block J (2021) Reviewing systematic literature reviews: ten key questions and criteria for reviewers. Manag Rev Q 71:519–524. https://doi.org/10.1007/s11301-021-00228-7
Kulik CT, Bainbridge HT (2006) HR and the line: the distribution of HR activities in Australian organisations. Asia Pac J Human Resour 44:240–256. https://doi.org/10.1177/1038411106066399
Lane PJ, Pathak KBR, S, (2006) The reification of absorptive capacity: a critical review and rejuvenation of the construct. Acad Manag Rev 31:833–863. https://doi.org/10.5465/amr.2006.22527456
*Lau DC, Lam LW, Salamon SD (2008) The impact of relational demographics on perceived managerial trustworthiness: similarity or norms? J Soc Psychol 148:187–209. https://doi.org/10.3200/socp.148.2.187-209
*Liang HY, Shih HA, Chiang YH (2015) Team diversity and team helping behavior: the mediating roles of team cooperation and team cohesion. Eur Manag J 33:48–59. https://doi.org/10.1016/j.emj.2014.07.002
*Marchiondo LA, Biermeier-Hanson B, Krenn DR, Kabat-Farr D (2018) Target meaning-making of workplace incivility based on perceived personality similarity with perpetrators. J Psychol Interdiscip Appl 152:474–496. https://doi.org/10.1080/00223980.2018.1481819
*Marstand AF, Epitropaki O, Martin R (2018) Cross-lagged relations between perceived leader–employee value congruence and leader identification. J Occup Org Psychol 91:411–420. https://doi.org/10.1111/joop.12192
McPherson M, Smith-Lovin L, Cook JM (2001) Birds of a feather: homophily in social networks. Annu Rev Soc 27:415–444. https://doi.org/10.1146/annurev.soc.27.1.415
Meglino BM, Ravlin EC, Adkins CL (1992) The measurement of work value congruence: a field study comparison. J Manag 18:33–43. https://doi.org/10.1177/014920639201800103
*Mitteness CR, DeJordy R, Ahuja MK, Sudek R (2016) Extending the role of similarity attraction in friendship and advice networks in angel groups. Entrep Theor Pract 40:627–655. https://doi.org/10.1111/etap.12135
Montoya RM, Horton RS (2012) The reciprocity of liking effect. In: Paludi M (ed) The psychology of love. Praeger, Santa Barbara, pp 39–57
Montoya RM, Horton RS (2013) A meta-analytic investigation of the processes underlying the similarity-attraction effect. J Soc Pers Rel 30:64–94. https://doi.org/10.1177/0265407512452989
Montoya RM, Horton RS (2014) A two-dimensional model for the study of interpersonal attraction. Pers Soc Psychol Rev 18:59–86. https://doi.org/10.1177/1088868313501887
Montoya RM, Horton RS (2020) Understanding the attraction process. Soc Pers Psychol Compass 14:e12526. https://doi.org/10.1111/spc3.12526
Montoya RM, Horton RS, Kirchner J (2008) Is actual similarity necessary for attraction? A meta-analysis of actual and perceived similarity. J Soc Pers Relat 25:889–922. https://doi.org/10.1177/0265407508096700
Montoya RM, Kershaw C, Prosser JL (2018) A meta-analytic investigation of the relation between interpersonal attraction and enacted behavior. Psychol Bull 144:673–709. https://doi.org/10.1037/bul0000148
Newcomb TM (1956) The prediction of interpersonal attraction. Am Psychol 11:575–586. https://doi.org/10.1037/h0046141
Newcomb TM (1961) The acquaintanceship process. Holt, New York
Book Google Scholar
*Nielsen S (2009) Why do top management teams look the way they do? A multilevel exploration of the antecedents of TMT heterogeneity. Strateg Org 7:277–305. https://doi.org/10.1177/1476127009340496
Noon M (2010) The shackled runner: time to rethink positive discrimination? Work Employ Soc 24:728–739. https://doi.org/10.1177/0950017010380648
O’Dea RE, Lagisz M, Jennions MD, Koricheva J, Noble DW, Parker TH, Nakagawa S (2021) Preferred reporting items for systematic reviews and meta-analyses in ecology and evolutionary biology: a PRISMA extension. Biol Rev 96:1695–1722. https://doi.org/10.1111/brv.12721
O’Reilly CA III, Caldwell DF, Chatman JA, Doerr B (2014) The promise and problems of organizational culture: CEO personality, culture, and firm performance. Group Org Manag 39:595–625. https://doi.org/10.1177/1059601114550713
*Orpen C (1984) Attitude similarity, attraction, and decision-making in the employment interview. J Psychol 117:111–120. https://doi.org/10.1080/00223980.1984.9923666
*Parent-Rocheleau X, Bentein K, Simard G (2020) Positive together? The effects of leader-follower (dis) similarity in psychological capital. J Bus Res 110:435–444. https://doi.org/10.1016/j.jbusres.2020.02.016
*Perry EL, Kulik CT, Zhou J (1999) A closer look at the effects of subordinate-supervisor age differences. J Org Behav 20:341–357. https://doi.org/10.1002/(sici)1099-1379(199905)20:3%3c341::aid-job915%3e3.0.co;2-d
Persell CH, Cookson P (1985) Chartering and bartering: elite education and social reproduction. Soc Probl 33:114–129. https://doi.org/10.2307/800556
Pierce CA, Byrne D, Aguinis H (1996) Attraction in organizations: a model of workplace romance. J Org Behav 17:5–32. https://doi.org/10.1002/(SICI)1099-1379(199601)17:1%3c5::AID-JOB734%3e3.0.CO;2-E
Pugh M, Wahrman R (1983) Neutralizing sexism in mixed-sex groups: do women have to be better than men? Am J Soc 88:746–762. https://doi.org/10.1086/227731
Ridgeway CL (1991) The social construction of status value: gender and other nominal characteristics. Soc Forces 70:367–386. https://doi.org/10.1093/sf/70.2.367
Ridgeway CL, Balkwell JW (1997) Group processes and the diffusion of status beliefs. Soc Psychol Q 60:14–31. https://doi.org/10.2307/2787009
Riordan CM (2000) Relational demography within groups: Past developments, contradictions, and new directions. In: Ferris GR (ed) Research in personnel and human resources management, vol 19. JAI Press, New York, pp 131–174
Rivera LA (2012) Hiring as cultural matching: the case of elite professional service firms. Am Soc Rev 77:999–1022. https://doi.org/10.1177/0003122412463213
Rosenbaum ME (1986) The repulsion hypothesis. On the nondevelopment of relationships. J Pers Soc Psychol 51:1156–1166. https://doi.org/10.1037/0022-3514.51.6.1156
*Rosenfeld HM, Jackson J (1965) Temporal mediation of the similarity-attraction hypothesis. J Pers 33:649–656. https://doi.org/10.1111/j.1467-6494.1965.tb01410.x
*Roth PL, Thatcher JB, Bobko P, Matthews KD, Ellingson JE, Goldberg CB (2020) Political affiliation and employment screening decisions: the role of similarity and identification processes. J Appl Psychol 105:472–486. https://doi.org/10.1037/apl0000422
*Rupert J, Jehn KA, van Engen ML, de Reuver RS (2010) Commitment of cultural minorities in organizations: effects of leadership and pressure to conform. J Bus Psychol 25:25–37. https://doi.org/10.1007/s10869-009-9131-3
Sacco JM, Scheu CR, Ryan AM, Schmitt N (2003) An investigation of race and sex similarity effects in interviews: a multi-level approach to relational demography. J Appl Psychol 88:852–865. https://doi.org/10.1037/0021-9010.88.5.852
*Salminen M, Henttonen P, Ravaja N (2016) The role of personality in dyadic interaction: a psychophysiological study. Int J Psychophysiol 109:45–50. https://doi.org/10.1016/j.ijpsycho.2016.09.014
Schachter S (1959) The psychology of affiliation. Stanford University Press, Stanford
*Schieman S, McMullen T (2008) Relational demography in the workplace and health: an analysis of gender and the subordinate-superordinate role-set. J Health Soc Behav 49:286–300. https://doi.org/10.1177/002214650804900304
Schneider B (1987) The people make the place. Pers Psychol 40:437–453. https://doi.org/10.1111/j.1744-6570.1987.tb00609.x
Schneider B, Pulakos ED (2022) Expanding the I-O psychology mindset to organizational success. Ind Organ Psychol 15:385–402. https://doi.org/10.1017/iop.2022.27
Schneider B, Smith DB, Taylor S, Fleenor J (1998) Personality and organizations: a test of the homogeneity of personality hypothesis. J Appl Psychol 83:462–470. https://doi.org/10.1037/0021-9010.83.3.462
*Schreurs B, Druart C, Proost K, De Witte K (2009) Symbolic attributes and organizational attractiveness: the moderating effects of applicant personality. Int J Sel Assess 17:35–46. https://doi.org/10.1111/j.1468-2389.2009.00449.x
*Sears GJ, Holmvall CM (2010) The joint influence of supervisor and subordinate emotional intelligence on leader–member exchange. J Bus Psychol 25:593–605. https://doi.org/10.1007/s10869-009-9152-y
Singh R, Ho LJ, Tan HL, Bell PA (2007) Attitudes, personal evaluations, cognitive evaluation, and interpersonal attraction: on the direct, indirect, and reverse-causal effects. Br J Soc Psychol 46:19–42. https://doi.org/10.1348/014466606x104417
Singh R, Lin PKF, Tan HL, Ho LJ (2008) Evaluations, attitude similarity, and interpersonal attraction: testing the hypothesis of weighting interference across responses. Basic Appl Soc Psychol 30:241–252. https://doi.org/10.1080/01973530802375052
*Stark E, Poppler P (2009) Leadership, performance evaluations, and all the usual suspects. Pers Rev 38:320–338. https://doi.org/10.1108/00483480910943368
Subramanian S, Billsberry J, Barrett M (2022) A bibliometric analysis of person-organization fit research: significant features and contemporary trends. Manag Rev Q. https://doi.org/10.1007/s11301-022-00290-9
Sunnafrank M (1992) On debunking the attitude similarity myth. Commun Monogr 59:164–179. https://doi.org/10.1080/03637759209376259
Tatli A, Özbilgin MF (2012) An emic approach to intersectional study of diversity at work: a Bourdieuan framing. Int J Manag Rev 14:180–200. https://doi.org/10.1111/j.1468-2370.2011.00326.x
Terman LM (1938) Psychological factors in marital happiness. McGraw-Hill, New York
Tidwell ND, Eastwick PW, Finkel EJ (2013) Perceived, not actual, similarity predicts initial attraction in a live romantic context: evidence from the speed-dating paradigm. Pers Relat 20:199–215. https://doi.org/10.1111/j.1475-6811.2012.01405.x
*Tsui AS, Porter LW, Egan TD (2002) When both similarities and dissimilarities matter: extending the concept of relational demography. Human Relat 55:899–929. https://doi.org/10.1177/0018726702055008176
Turner BA, Chelladurai P (2005) Organizational and occupational commitment, intention to leave, and perceived performance of intercollegiate coaches. J Sport Manag 19:193–211. https://doi.org/10.1123/jsm.19.2.193
*Van Hoye G, Turban DB (2015) Applicant–employee fit in personality: testing predictions from similarity-attraction theory and trait activation theory. Int J Sel Assess 23:210–223. https://doi.org/10.1111/ijsa.12109
Verquer ML, Beehr TA, Wagner SH (2003) A meta-analysis of relations between person–organization fit and work attitudes. J Vocat Behav 63:473–489. https://doi.org/10.1016/S0001-8791(02)00036-2
Vogel RM, Rodell JB, Lynch JW (2016) Engaged and productive misfits: how job crafting and leisure activity mitigate the negative effects of value incongruence. Acad Manag J 59:1561–1584. https://doi.org/10.5465/amj.2014.0850
Walster E, Aronson V, Abrahams D, Rottman L (1966) Importance of physical attractiveness in dating behavior. J Pers Soc Psychol 4:508–516. https://doi.org/10.1037/h0021188
Webster M Jr, Hysom SJ (1998) Creating status characteristics. Am Soc Rev. https://doi.org/10.2307/2657554
Wilkins AC (2012) Becoming black women: intimate stories and intersectional identities. Soc Psychol Q 75:173–196. https://doi.org/10.1177/0190272512440106
*Xu J, Yun K, Yan F, Jang P, Kim J, Pang C (2019) A study on the effect of TMT characteristics and vertical dyad similarity on enterprise achievements. Sustain 11:1–16. https://doi.org/10.3390/su11102913
*Young IP, Place AW, Rinehart JS, Jury JC, Baits DF (1997) Teacher recruitment: a test of the similarity-attraction hypothesis for race and sex. Educ Adm Q 33:86–106. https://doi.org/10.1177/0013161X97033001005
Yu KYT, Verma K (2019) Investigating the role of goal orientation in job seekers’ experience of value congruence. Appl Psychol Int Rev 68:83–125. https://doi.org/10.1111/apps.12152
Zajonc RB (1968) Attitudinal effects of mere exposure. J Pers Soc Psychol Monogr 9:1–27. https://doi.org/10.1037/h0025848
*Zatzick CD, Elvira MM, Cohen LE (2003) When is more better? The effects of racial composition on voluntary turnover. Org Sci 14:483–496. https://doi.org/10.1287/orsc.14.5.483.16768
*Zhu DH, Chen G (2015) Narcissism, director selection, and risk-taking spending. Strateg Manag J 36:2075–2098. https://doi.org/10.1002/smj.2322
* Included in systematic review
Abbasi Z, Billsberry J, Todres M (2021) An integrative conceptual two-factor model of workplace value congruence and incongruence. Manag Res Rev. https://doi.org/10.1108/MRR-03-2021-0211
Adkins CL, Russell CJ, Werbel JD (1994) Judgments of fit in the selection process: the role of work value congruence. Pers Psychol 47:605–623. https://doi.org/10.1111/j.1744-6570.1994.tb01740.x
Alliger GM, Janak EA, Streeter D, Byrne D, Turban D (1993) Psychological similarity effects in personnel selection decisions and work relations: A meta-analysis. In: Annual conference of the society for industrial and organizational psychology, San Francisco CA, USA.
*Allinson CW, Armstrong SJ, Hayes J (2001) The effects of cognitive style on leader-member exchange: a study of manager-subordinate dyads. J Occup Org Psychol 74:201–220. https://doi.org/10.1348/096317901167316
*Almeida S, Fernando M, Hannif Z, Dharmage SC (2015) Fitting the mould: the role of employer perceptions in immigrant recruitment decision-making. Int J Human Resour Manag 26:2811–2832. https://doi.org/10.1080/09585192.2014.1003087
*Aronson ZH, Dominick PG, Wang M (2014) Exhibiting leadership and facilitation behaviors in NPD project-based work: does team personal style composition matter? Eng Manag J 26:25–35. https://doi.org/10.1080/10429247.2014.11432017
Download references
Acknowledgements
We would like to thank the editor, Professor Joern Block, and the anonymous reviewer for his or her valuable comments.
Open Access funding enabled and organized by CAUL and its Member Institutions.
Author information
Authors and affiliations.
University of Wollongong, Northfield Avenue, Wollongong, NSW, 2522, Australia
Zoleikha Abbasi, Jon Billsberry & Mathew Todres
You can also search for this author in PubMed Google Scholar
Corresponding author
Correspondence to Jon Billsberry .
Ethics declarations
Conflict of interest.
The authors declare that they have no conflicts of interest.
Consent to participate
The authors consent to participate.
Consent for publication
The authors consent for publication.
Additional information
Publisher's note.
Springer Nature remains neutral with regard to jurisdictional claims in published maps and institutional affiliations.
Rights and permissions
Open Access This article is licensed under a Creative Commons Attribution 4.0 International License, which permits use, sharing, adaptation, distribution and reproduction in any medium or format, as long as you give appropriate credit to the original author(s) and the source, provide a link to the Creative Commons licence, and indicate if changes were made. The images or other third party material in this article are included in the article's Creative Commons licence, unless indicated otherwise in a credit line to the material. If material is not included in the article's Creative Commons licence and your intended use is not permitted by statutory regulation or exceeds the permitted use, you will need to obtain permission directly from the copyright holder. To view a copy of this licence, visit http://creativecommons.org/licenses/by/4.0/ .
Reprints and permissions
About this article
Abbasi, Z., Billsberry, J. & Todres, M. Empirical studies of the “similarity leads to attraction” hypothesis in workplace interactions: a systematic review. Manag Rev Q 74 , 661–709 (2024). https://doi.org/10.1007/s11301-022-00313-5
Download citation
Received : 29 January 2022
Accepted : 11 December 2022
Published : 16 January 2023
Issue Date : June 2024
DOI : https://doi.org/10.1007/s11301-022-00313-5
Share this article
Anyone you share the following link with will be able to read this content:
Sorry, a shareable link is not currently available for this article.
Provided by the Springer Nature SharedIt content-sharing initiative
- Similarity-attraction hypothesis
- Value congruence
- Systematic review
- Recruitment
- Dissimilarity-repulsion hypothesis
JEL Classification
- Find a journal
- Publish with us
- Track your research
Thank you for visiting nature.com. You are using a browser version with limited support for CSS. To obtain the best experience, we recommend you use a more up to date browser (or turn off compatibility mode in Internet Explorer). In the meantime, to ensure continued support, we are displaying the site without styles and JavaScript.
- View all journals
- My Account Login
- Explore content
- About the journal
- Publish with us
- Sign up for alerts
- Open access
- Published: 16 March 2023
Partner choice, confounding and trait convergence all contribute to phenotypic partner similarity
- Jennifer Sjaarda 1 , 2 , 3 &
- Zoltán Kutalik ORCID: orcid.org/0000-0001-8285-7523 1 , 2 , 3
Nature Human Behaviour volume 7 , pages 776–789 ( 2023 ) Cite this article
7310 Accesses
3 Citations
29 Altmetric
Metrics details
- Behavioural genetics
- Population genetics
Partners are often similar in terms of their physical and behavioural traits, such as their education, political affiliation and height. However, it is currently unclear what exactly causes this similarity—partner choice, partner influence increasing similarity over time or confounding factors such as shared environment or indirect assortment. Here, we applied Mendelian randomization to the data of 51,664 couples in the UK Biobank and investigated partner similarity in 118 traits. We found evidence of partner choice for 64 traits, 40 of which had larger phenotypic correlation than causal effect. This suggests that confounders contribute to trait similarity, among which household income, overall health rating and education accounted for 29.8, 14.1 and 11.6% of correlations between partners, respectively. Finally, mediation analysis revealed that most causal associations between different traits in the two partners are indirect. In summary, our results show the mechanisms through which indirect assortment increases the observed partner similarity.
Similar content being viewed by others
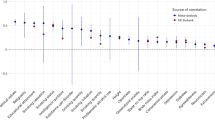
Evidence of correlations between human partners based on systematic reviews and meta-analyses of 22 traits and UK Biobank analysis of 133 traits
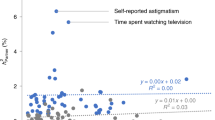
Evidence of horizontal indirect genetic effects in humans
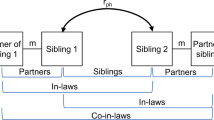
Modeling assortative mating and genetic similarities between partners, siblings, and in-laws
People in partnerships are more similar to one another than randomly sampled pairs. Partners tend to be similar with respect to traits such as various anthropometric measures (body mass index (BMI) and height), socioeconomic factors, behaviours (religious views 1 and social attitudes 2 ), lifestyle (diet, smoking habits and hobbies) and even disease risk 3 , 4 , 5 , 6 , 7 , 8 , 9 , 10 .
Several different causes can explain this phenotypic similarity. First, people actively look for partners who are similar to them 11 , 12 , a phenomenon known as assortative mating (AM). Second, phenotypic similarity can reflect trait convergence during the partnership. In this case, traits become more similar over time because partners share a household or they influence each other’s behaviour 13 , 14 , 15 . Third, partner similarity may be caused by confounders at the time of partner choice (or later), such as shared sociocultural environment and geographical barriers 16 , 17 , 18 (Fig. 1 ). Indirect assortment is a special case of the latter, where the confounder is the correlated trait which people use in direct partner selection 19 .
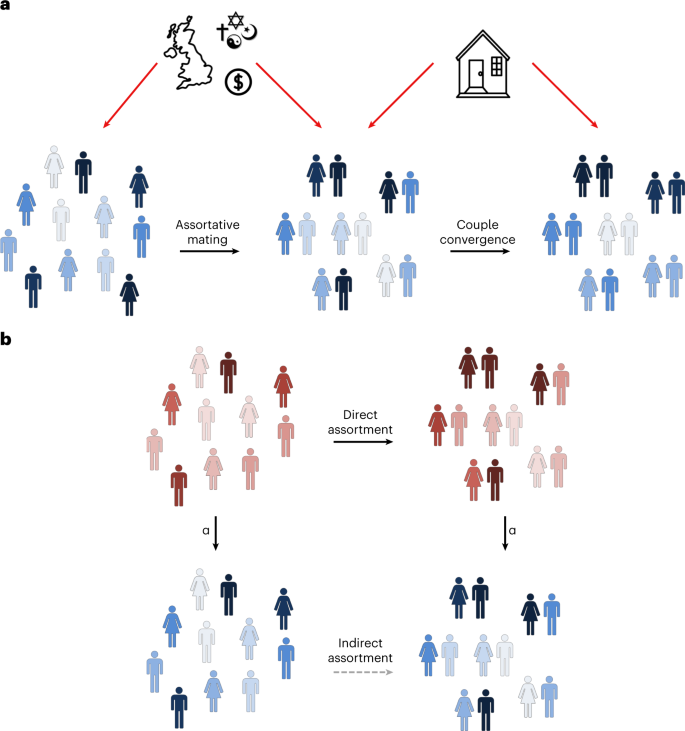
a , Illustrates a trait (given by the colour blue) which shows increased similarity between partners, either directly (through mate choice) or due to confounding factors such as shared geography, cultural or religious status or socioeconomic measures. Subsequently, this trait may also undergo postmating convergence, which could be due to direct causal influence from one partner on the other (that is, through imitation or influence) or due to confounding factors such as shared environment. b , Illustrates a trait which shows increased similarity among couples (given by the blue trait); however, this assortment is only observed because of a causal effect (α) that exists between another trait (shown in red) acting on the blue trait. For example, if direct assortment occurs under a trait such as BMI (that is, couples intentionally select partners of similar BMI as themselves), phenotypic correlation will also be observed at all traits which have a causal effect on BMI, such as blood pressure, fasting glucose and so on.
In couples, one person’s genome correlates with certain traits of their partner 20 , and one study found evidence of direct genetic associations between the genome of an individual and the phenotypes of their partner. This finding suggests that partner heritability of a trait cannot be explained by between-trait correlation alone 21 . Overall, causes and consequences of phenotypic assortment remain unresolved.
The causes of partner similarity matter for the fields such as behavioural science, population genetics and public health. For instance, high phenotypic similarity could imply genetic similarity. In this case, otherwise independent gene variants would become correlated and would ultimately increase genotype homozygocity 22 . Partner similarity also affects the studies of genetic associations; it increases the estimates of heritability 23 and genetic correlation 24 , even in ACE (additive, common environment and unique environment) models 25 . Moreover, partner similarity can introduce collider bias in within-spouse association models 26 .
Similar to classical epidemiological studies where it is difficult to discern causal factors from confounders, mere phenotypic similarity within couples poses interpretational challenges. Mendelian randomization (MR) is a special case of instrumental variable (IV) analysis, whereby genetic markers are used as instruments to infer causal relationship. Because of the random allocation of genetic variants at birth, MR can infer causality between an exposure and an outcome 27 , avoiding reverse causality and confounding.
In this work, we used MR to study causality in couples where the exposure and outcome traits belong to different people (Fig. 2a ). This concept is different from classical MR designs within individuals, such as the study of BMI causally affecting the risk for coronary artery disease 28 . The authors of ref. 29 used an approach similar to ours to study partner similarity in alcohol use. They showed that phenotypic correlation in couples does not increase with age and there was a difference between correlation and the direct causal effect 29 .
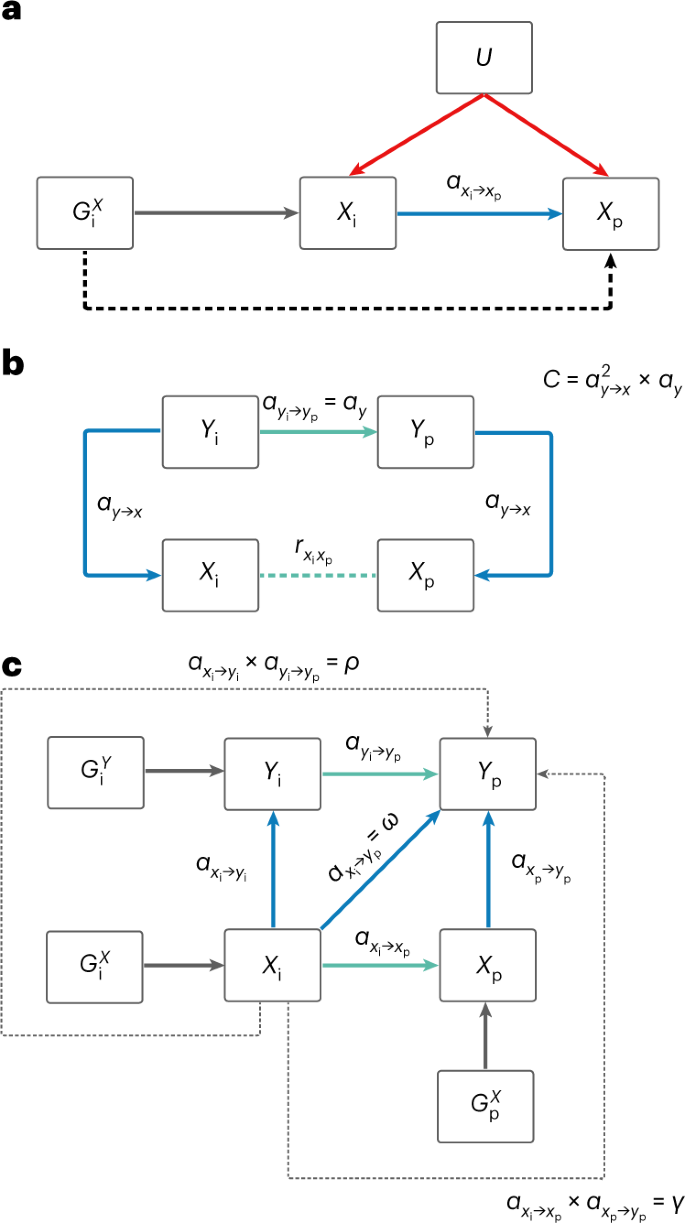
a , Illustrates the causal effect among couples with a single trait \((\alpha _{x_{\mathrm{i}} \to x_{\mathrm{p}}})\) , where G represents genetic variant(s), X represents a single trait (in an index individual ( X i ) and a partner ( X p )) and U represents confounding factors that are not associated with genetic variance owing to the random distribution of alleles at conception. Throughout the paper subscript i and p refer to the index and the partner, respectively. b , Directed acyclic graph illustrates the impact a confounder (trait Y ) could have on the phenotypic correlation between partners for a given trait X ( \(r_{x_{\mathrm{i}}x_{\mathrm{p}}}\) ). Correlation due to confounding can be calculated as \(C = \alpha _{y \to x}^2 \times \alpha _y\) . c , Represents the expanded causal network involving two traits and the various estimated causal paths from trait X of an index case ( \(X_{\mathrm{i}}\) ) to a phenotype Y in the partner ( \(Y_{\mathrm{p}}\) ) given by ω , γ and ρ . Cross-trait causal effects from \(X_{\mathrm{i}}\) to \(Y_{\mathrm{p}}\) ( ω ) can be summarized by three possible (non-independent) scenarios: (1) \(X_{\mathrm{i}}\) could exert a causal effect on \(X_{\mathrm{p}}\) , followed by \(X_{\mathrm{p}}\) having a causal effect on \(Y_{\mathrm{p}}\) in the partner alone ( γ ); (2) the reverse could occur whereby \(X_{\mathrm{i}}\) has a causal effect on \(Y_{\mathrm{i}}\) in the index alone, followed by a causal effect of \(Y_{\mathrm{i}}\) case on \(Y_{\mathrm{p}}\) ( ρ ); or (3) there could be other mechanisms, either acting directly or through other unmeasured or unconsidered variables. To quantify ρ , we first estimated the causal effect of \(Y_{\mathrm{i}}\) on \(Y_{\mathrm{p}}\) in MVMR (not illustrated) to exclude any residual effect of X on phenotype Y from index to partner. These three scenarios could also act in some combination. Therefore, the ω estimate would capture the paths of γ , ρ and other mechanisms combined. In both a and c , cross-partner causal effects are given by blue arrows and same-person causal effects are given by green arrows.
Here, we applied MR to estimate the causal effects between partners for 118 traits. We studied direct effects on trait similarity, the impact of time couples have lived together and the role of confounders. Finally, we explored how cross-trait partner similarity emerges by dissecting them to direct and indirect parts.
This study analysed data from the UK Biobank (UKBB) cohort, a prospective population-based study with over 500,000 adult participants. Among them, 51,664 couples were identified and selected according to a procedure described in the Methods and Supplementary Fig. 1 . Starting from 1,278 available phenotypes, we selected those with between-partner (Pearson) correlation larger than 0.1 and having at least five valid instruments. Pearson and Spearman correlations led to very consistent estimates (Supplementary Fig. 2 ). These were further filtered ( Methods and Supplementary Fig. 3 ) to yield 118 traits to analyse.
Effect of sex, age and time spent together
Among the 118 phenotypes tested, we identified 64 significant ( P < 0.05/66) causal effects in partners after adjusting for the effective number of tests (66) (Supplementary Table 1 ). We also examined the Cochran’s heterogeneity Q statistic to identify traits with high heterogeneity and found no evidence of heterogeneity in the MR estimates (all P > 0.05/66). We assessed the 64 significant results for sex differences but did not identify any after adjusting for the effective number of tests among the remaining traits based on their pairwise correlation matrix ( P < 0.05/29).
To identify if partner traits converge over time, we explored the impact of age and time spent together (proxied by the amount of time at the same address) among the 64 significant traits in both men and women separately and both sexes combined. Using linear regression of MR estimates versus the median of the five age and/or time spent together bins, we detected no significant results in the sex-combined results after adjustment for the number of effective tests ( P < 0.05/66). We also examined the Pearson phenotypic correlation within the different bins and assessed for the presence of a trend using linear models (phenotypic correlation versus median bin) for all 118 phenotypes. Two traits showed a significant ( P < 0.05/66) trend across the bins according to time spent together, namely body fat percentage (slope = −0.0018, P = 1.96 × 10 −5 ) and forced expiratory volume in 1 second (slope = −0.0043, P = 2.98 × 10 −4 ). In both cases, the correlation decreased as time spent together increased. We found two other traits that showed a significant trend across the bins by median age, namely previous smoking status (slope = 0.0011, P = 6.9 × 10 −4 ) and aspirin use (slope = 0.0015, P = 1.8 × 10 −4 ). In this case, for both phenotypes, the slope increased as median age increased (Fig. 3 and Supplementary Table 1 ). Using Spearman correlation yielded consistent results (all P < 0.05/66).
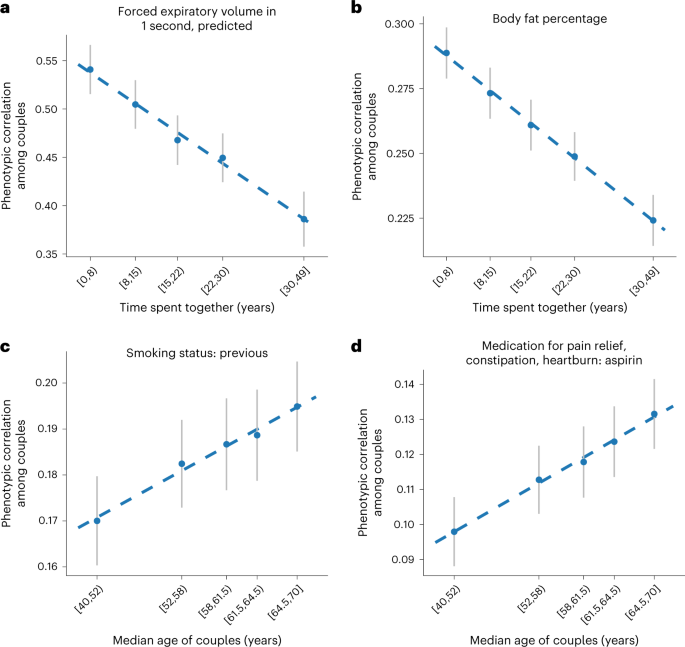
a – d , Scatterplots show the phenotypic correlation for four selected traits among couples within different bins. Couples were binned by time spent together (proxied by the time lived at the same household) ( a , b ) and median age ( c , d ). The four panels show correlations for four different traits: forced expiratory volume ( a ), body fat percentage ( b ), previous smoker ( c ) and aspirin use ( d ).
Causal effects versus raw phenotypic correlations
To better understand the nature of phenotypic assortment, we assessed whether there were any discrepancies between the causal effect estimates within couples ( 𝛼 ̂) and observational correlations ( 𝑟 ̂). Using MR, the causal effects between partners (within couples) were estimated for 118 phenotypes. These traits were selected based on their elevated correlation between partners and sufficient (more than five) valid IVs, making them suitable for MR analysis. Using a two-tailed Z test to gauge the statistical significance of the difference between the estimates (with test P -value denoted by P diff ), we compared (standardized) causal MR effects to the raw phenotypic correlation among couples to identify any traits where the correlation was different than the MR estimate. After adjusting for the effective number of tested traits ( P < 0.05/66), we identified 43 traits which showed different phenotypic correlation compared to MR estimate (Fig. 4a and Supplementary Table 1 ). Of these, three had a larger MR estimate compared to correlation (time spent watching television, comparative height size at age 10 and overall health rating), while the remaining 40 traits had a larger (absolute) correlation compared to MR estimate. These included place of birth, north coordinate (NC; \({\hat{r}} = 0.58\) versus \({\hat{\alpha}} = 0.33\) , P diff = 2.47 × 10 −18 ), systolic blood pressure ( \({\hat{r}} = 0.16\) versus \({\hat{\alpha}} = 0.05\) , P diff = 4.89 × 10 −9 ), height ( \({\hat{r}} = 0.25\) versus \({\hat{\alpha}} = 0.21\) , P diff = 6.63 × 10 −6 ), forced vital capacity ( \({\hat{r}} = 0.25\) versus \({\hat{\alpha}} = 0.13\) , P diff = 5.62 × 10 −16 ), basal metabolic rate ( \({\hat{r}} = 0.21\) versus \({\hat{\alpha}} = 0.16\) , P diff = 9.78 × 10 −7 ) and basophil count ( \({\hat{r}} = 0.47\) versus \({\hat{\alpha}} = 0\) , P diff = 1.76 × 10 −38 ) (Fig. 4a ).
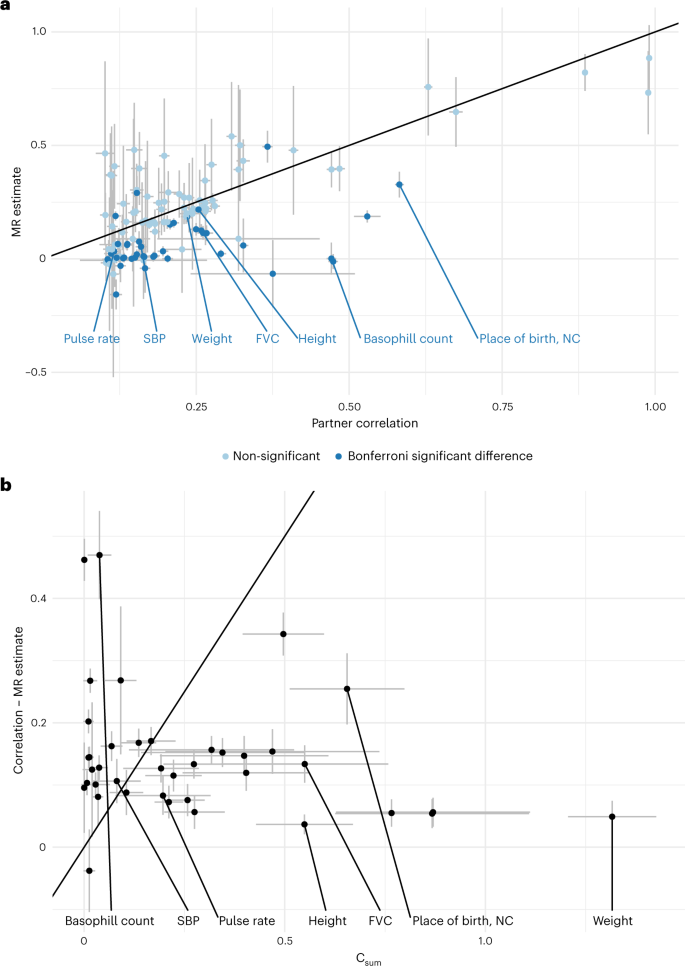
a , Scatterplot shows the within-couple standardized MR estimates ( \(\alpha _{x_{\mathrm{i}} \to x_{\mathrm{p}}}\) ) versus the phenotypic correlation among couples \((r_{x_{\mathrm{i}}x_{\mathrm{p}}})\) . The centre of the confidence interval (CI) is the estimate for the corresponding parameter and error bars represent 95% CIs. A two-tailed Z test was used to test for a significant difference between the estimates. After adjusting for the number of effective tests ( P < 0.05/66), 43 significant differences were identified (shown in dark blue), where 3 traits showed larger MR estimates compared to correlation and 40 traits showed larger correlation compared to MR estimates. The identity line is shown in black. Labelled pairs are discussed in the main text. b , Scatterplot shows the difference in phenotypic correlation and MR estimate versus the C sum value (estimating the correlation induced by measured (uncorrelated) confounders) for each trait where the phenotypic correlation was greater than the MR estimate (number of traits = 39); error bars represent 95% CIs. The identity line is shown in black. FVC, forced vital capacity; NC, north coordinate; SBP, systolic blood pressure.
Significant differences could be indicative of the presence of confounders (either negative or positive) driving the observed phenotypic correlation. Thus, for traits where couple correlation was significantly different than MR causal estimates, we sought to identify potential confounders which may, in part, explain the discrepant estimates. For the three traits where correlation was less than the MR estimate, we searched for negative confounders (that is, negative \({\upalpha}_{y_{\mathrm{i}} \to y_{\mathrm{p}}}\) ) but did not identify any.
Conversely, for traits where the correlation was greater than the MR estimate, we searched for positive confounders and found many potential positive confounders. Namely, the mean number of potential confounders from our set of 117 candidates was 22.56, with a maximum of 39; there was only one trait for which we did not identify any potential confounders (Supplementary Table 2 ). For instance, for systolic blood pressure, we identified 29 (correlated) potential confounders, which may explain the larger phenotypic correlation ( \({\hat{r}} = 0.16\) ) as compared to MR effect ( \({\hat{\alpha}} = 0.05\) ). These potential confounders included physical activity, BMI, lung fitness measures and overall health rating. For weight ( \({\hat{r}} = 0.23\) versus \({\hat{\alpha}} = 0.19\) ), we found 30 potential confounders, including anthropometric traits (such as leg, trunk and arm fat mass) and various behavioural traits which are reflective of exercise patterns, such as time spent watching television, walking pace and phone use, among many others (Supplementary Table 2 ). Many of the 40 traits with larger phenotypic correlation compared to MR estimates included blood cell counts and/or percentages (such as white blood cell (leucocyte) count, neutrophil count, monocyte count and percentage and reticulocyte percentage and count). The potential confounders for these traits were highly overlapping, including physical activity level, anthropometric traits, smoking and health rating (Supplementary Table 2 ). Other notable confounders included measures of physical activity for forced vital capacity; smoking status and fitness measures for basal metabolic rate; and measures of body size for hand grip strength.
Finally, for each confounder we calculated the correlation due to confounding ( C ) as described above ( Methods and Figs. 1b and 2b ) and summed up these C values for all uncorrelated confounders. We then, for each trait, compared the difference in estimates (that is, \({\hat{r}} - {\hat{\alpha}}\) ) to the estimated value C sum (Fig. 4b ). One can observe some traits (for example, systolic blood pressure) where the difference between partner correlation and causal effects can be well explained by the tested confounders, but for the majority of the traits, the observed confounders are not sufficient to account for the discrepancy (for example, basophil count has strong positive confounders missing).
Major confounders of trait correlations
Next, we assessed the impact of potential confounders on trait correlation in couples by calculating the ratio of correlation due to confounding over the raw phenotypic correlation among couples averaged across all traits tested (Supplementary Table 3 ). While geographical location (using place of birth north/east coordinates) was found to have a negligible impact on phenotypic correlations (mean confounding ratio 1%), household income (mean confounding ratio 29.8%), age completed full-time education (mean confounding ratio 11.6%) and physical activity levels (measured using the variable ‘leisure/social activities: sport club or gym’; mean confounding ratio 17.1%) had an important confounding impact on raw phenotypic correlation among couples (Fig. 5 ).
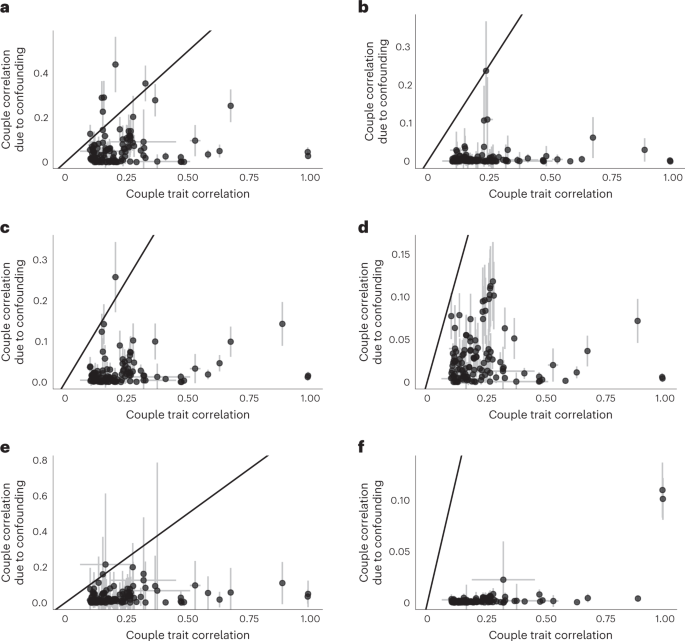
a – f , Scatterplots of couple correlation due to confounding versus the phenotypic trait correlation among couples for selected potential confounder traits ( Z ). The couple correlation due to confounding for each trait X was calculated for each confounder Y as \(C = \alpha _{y \to x}^2 \times \alpha _{y_{\mathrm{i}} \to y_{\mathrm{p}}}\) . In the case of birthplace coordinates, C values were summed across the two (independent) north and east coordinates. The centre of the CI is the estimator value and the error bars represent the 95% CI. These confidence intervals for the correlations shown on the x axis are based on the number of couples shown in the ‘n_pairs’ column of Supplementary Table 2 . The CI for confounder-induced correlation was computed as 1.96 times the s.e. of the estimator \({\hat{\alpha}} _{y \to x}^2 \times {\hat{\alpha}} _{y_{\mathrm{i}} \to y_{\mathrm{p}}}\) , the computation of which is described in the Methods . For each trait in the pipeline, we tested how the contribution of six confounder traits (average total household income before tax ( a ), current tobacco smoking ( b ), age completed full-time education ( c ), overall health rating ( d ), sports club or gym user ( e ) and place of birth coordinates ( f )) could impact the phenotypic couple correlation. The identity line is shown in black.
Cross-trait assortment
We sought to identify the mechanisms underlying partner similarity by comparing three estimated paths from a phenotype in the index case ( \(X_{\mathrm{i}}\) ) to another phenotype in its partner ( \(Y_{\mathrm{p}}\) ) as illustrated in Fig. 2c . The total causal effect between \(X_{\mathrm{i}}\) and \(Y_{\mathrm{p}}\) (denoted by ω ) can be split up into three components: (1) AM through X (that is, \(X_{\mathrm{i}} \to X_{\mathrm{p}}\) ) followed by a causal effect between X and Y in the partner (that is, \(X_{\mathrm{p}} \to Y_{\mathrm{p}}\) ), their product being denoted by γ ; (2) causal effect between X and Y in the index individual (that is, \(X_{\mathrm{i}} \to Y_{\mathrm{i}}\) ), followed by AM through Y (that is, \(Y_{\mathrm{i}} \to Y_{\mathrm{p}}\) ), their product being denoted by ρ ; and (3) any remaining effect of \(X_{\mathrm{i}}\) on \(Y_{\mathrm{p}}\) .
We computed within-couple cross-trait causal effect estimates \(X_{\mathrm{i}} \to X_{\mathrm{p}}\) (that is, \({\hat{\omega}}\) ) for all combinations of trait pairs ( \(X,Y\) ). Of these, we identified 1,327 significant MR effects ( \(p_{\hat{\omega}}\) < 0.05/[66 2 ]) among couples, which were reduced to 1,088 pairs after removing pairs with phenotypic correlation >0.8 (a summary of a set of pruned traits can be found in Supplementary Table 4 ). Several relationships were almost completely dominated by ρ (AM through the outcome) and others dominated by γ (AM through the exposure). Specifically, we found 326 relationships which were significantly different between ρ and γ , of which 89 (27.3%) showed larger effects through ρ and the other 237 (72.7%) showed larger effects through γ. For instance, we found causal relationships between partners for leg fat percentage on the time spent watching television and BMI on overall health rating, all dominated by ρ .
On the other hand, we found some causal relationships between partners which were primarily dominated by γ (AM through the exposure), including comparative height at age 10 (that is ‘When you were 10 years old, compared to average would you describe yourself as shorter, taller, average’), forced vital capacity and standing height on hand grip strength. Finally, we found other pairs where neither ρ nor γ captured the relationship (that is, \({\hat{\omega}}\) was significantly larger than both estimates), including BMI effect on partner’s systolic and/or diastolic blood pressure.
Finally, we estimated the contribution of the first two components ( \({\hat{\gamma}}\) and \({\hat{\rho}}\) ) contributing to these significant cross-trait effects and compared their contribution to the total effect using standard linear regression (Fig. 6 and Supplementary Table 5 ). Paired t test comparing \({\hat{\gamma}}\) and \({\hat{\rho}}\) effect estimates revealed that \({\hat{\gamma}}\) (AM through X ) is stronger ( P = 1.1 × 10 −5 ) in general compared to \({\hat{\rho}}\) (AM through Y ). When we summed up the effects of \({\hat{\gamma}}\) and \({\hat{\rho}}\) , we found that the sum was significantly larger than \({\hat{\omega}}\) . However, these two effects seemed to be correlated, carrying potentially shared signals. Hence, we first residualized \({\hat{\rho}}\) for the effects of \({\hat{\gamma}}\) ( \(\widehat {\rho _{{\mathrm{resid}}}}\) ) to ensure independence between the two estimates and then added \(\widehat {\rho _{{\mathrm{resid}}}}\) to \({\hat{\gamma}}\) ( \(\widehat {\rho _{{\mathrm{resid}}}} + {\hat{\gamma}}\) ). We found no significant difference between \({\hat{\omega}}\) and the sum of \(\widehat {\rho _{{\mathrm{resid}}}} + {\hat{\gamma}}\) in this analysis and with data points in general falling near the identity line, suggesting that \({\hat{\omega}}\) was capturing the paths given by \({\hat{\gamma}}\) and \({\hat{\rho}}\) . Indeed, linear regression results revealed that 76% of the total effect ( \({\hat{\omega}}\) ) can be explained by the two paths ( \(\widehat {\rho _{{\mathrm{resid}}}} + {\hat{\gamma}}\) ) and that the \(\widehat {\rho _{{\mathrm{resid}}}} + {\hat{\gamma}}\) is on average very close to the total effect.
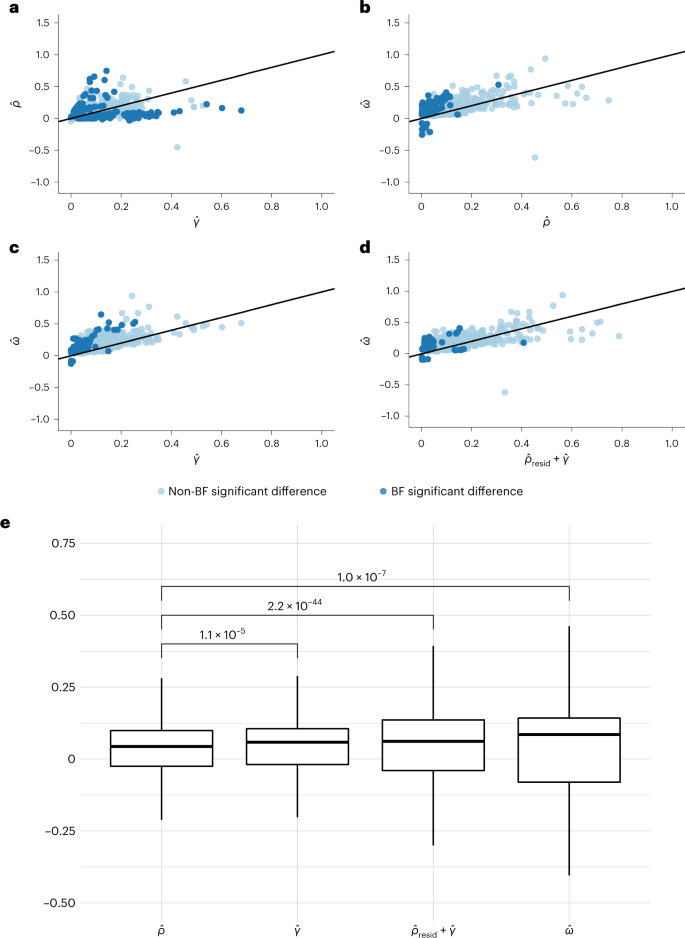
a – d , We estimated various causal effect paths (ρ, γ and ω, see Fig. 2c ) from a phenotype of the index case ( \(X_{\mathrm{i}}\) ) to another phenotype of its partner ( \(Y_{\mathrm{p}}\) ) for the 1,088 trait pairs with significant MR effects among couples ( \(p_{\hat{\omega}}\) < 0.05/[66 2 ]) and trait pair correlation <0.8. Panel ( a ) provides a scatter plot for 𝜌 ̂ against 𝛾 ̂ ; panel ( b ) for 𝜔 ̂ against 𝜌 ̂; panel ( c ) for 𝜔 ̂ against 𝛾 ̂ and panel ( d ) for 𝜔 ̂ against \(\widehat {\rho _{\mathrm{resid}}} + {\hat{\gamma}}\) . The solid black line represents the linear regression fit. Dark blue dots indicate trait pairs with significant (after Bonferroni, BF, correction) difference between the respective parameters shown in the scatter plot, while light blue one mark the remaining traits. To calculate \(\widehat {\rho _{{\mathrm{resid}}}} + {\hat{\gamma}}\) , we residualized \({\hat{\rho}}\) for the effects of \({\hat{\gamma}}\) ( \(\widehat {\rho _{{\mathrm{resid}}}}\) ) to ensure complete independence between the estimates and then added \(\widehat {\rho _{{\mathrm{resid}}}}\) to \({\hat{\gamma}}\) ( \(\widehat {\rho _{{\mathrm{resid}}}} + {\hat{\gamma}}\) ). e , A box plot comparing the coefficients of the estimates among the trait pairs after removing 19 trait pairs where the sign did not match between any combination of the four coefficients. In the box plots, the lower and upper hinges correspond to the first and third quartiles and the middle bar corresponds to the median; the upper whisker is the largest point smaller than 1.5 times the interquartile range above the third quartile; the lower whisker is defined analogously. We used a two-sided paired t test to compare the presented estimates ( \({\hat{\rho}} ,{\hat{\gamma}} ,{\widehat{{\rho} _{{\mathrm{resid}}}}} + {\hat{\gamma}}\) and \({\hat{\omega}}\) ).
The extent of bias in the MR estimates
In the Methods , we formulated a general model that accommodates parental effects and direct, indirect assortment (Fig. 7 ). We then, assuming this model, derived the analytical formula for the bias of cross-sample MR estimates, which shows its exact dependence on each model parameter. Here, we explored the extent of the bias under realistic ranges of model parameters and visualized it in Fig. 8 . First, we observed (Fig. 8a ) that \(r_{\mathrm{G}}\) (the correlation of the genotype between partners) has little impact on the bias (since it is limited by the heritability and the genetic correlation) compared to direct environmental assortment ( \(r_{\mathrm{E}}\) ), and they contribute additively. A similar relationship can be observed (Fig. 8b ) for the parental genetic- ( \(s_{\mathrm{G}}\) ) and parental trait effects ( \(s_{\mathrm{X}}\) ) on the offspring’s environment; the impact of the former is dwarfed by the latter for the same reason. Unsurprisingly, the largest bias emerges when both the \(r_{\mathrm{E}}\) and \(s_{\mathrm{X}}\) are the largest (Fig. 8c ). This can be complemented by \(r_{\mathrm{E}}\) combined with parental genetic effects on the offspring’s environment ( \(s_{\mathrm{G}}\) ) (Fig. 8d ).
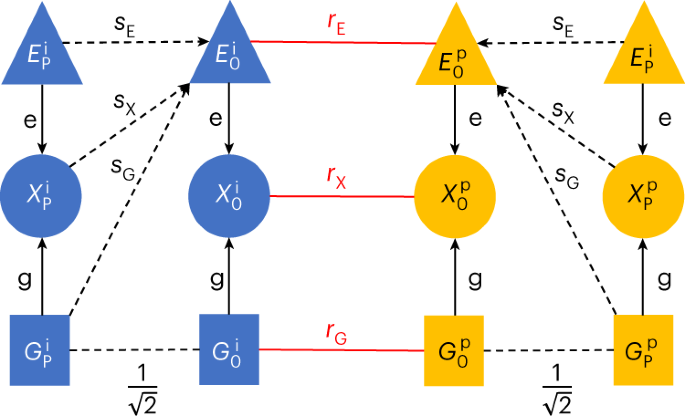
The diagram represents the underlying joint model of parental effects and assortment. A focal trait (X) has genetic (G) and envionmental (E) components, with effect size g and e , respectively. Their subscripts can be O, F or M, referring to the offspring, the father or the mother, respectively. The superscripts can be either i or p , indicating the index individual or its partner. We allowed parental genetics, parental environment and the parental trait each to influence the offspring’s environment, with corresponding direct effect strengths s G , s E and s X , respectively. Finally, couples are formed under direct assortments acting on G, E and X, leading to correlations r G , r E and r X , respectively.
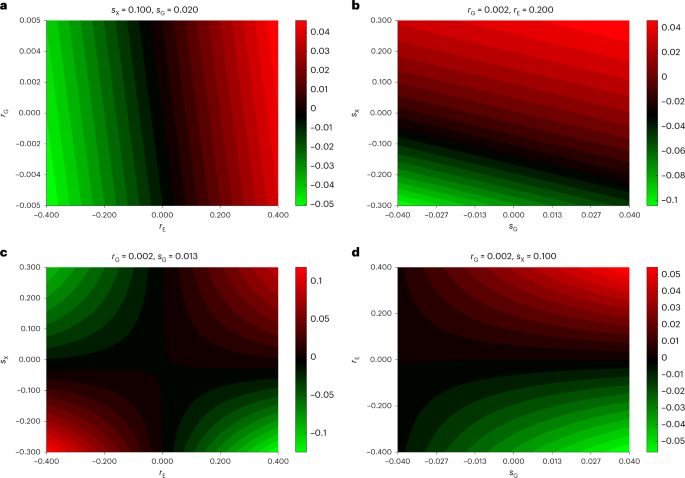
We plotted the bias of the cross-sample MR estimates as a function of the proposed model parameters. Parameter \(r_{\mathrm{G}}\) refers to direct genetic assortment, \(r_{\mathrm{E}}\) to direct environmental assortment, \(s_{\mathrm{G}}\) to direct parental genetic effect and \(s_{\mathrm{X}}\) to direct parental trait effect. The different panels show the extent of bias when different pairs of parameters were covaried: \(r_{\mathrm{G}},r_{\mathrm{E}}\) ( a ), \(s_{\mathrm{G}},s_{\mathrm{X}}\) ( b ), \(r_{\mathrm{E}},s_{\mathrm{X}}\) ( c ) and \(s_{\mathrm{G}},r_{\mathrm{E}}\) ( d ).
Overall, when direct environmental or genetic assortment is moderate, parental effects lead to negligible bias in the causal effect estimate between \(X_{\mathrm{O}}^{\mathrm{i}}\) and \(X_{\mathrm{O}}^{\mathrm{p}}\) . This is why we focused our real data analysis efforts on identifying such X -associated (confounding) factors for which direct assortment may occur, so that \(r_{\mathrm{E}}\) (and hence, the MR bias) could be limited.
In this article, we studied causal relationships behind trait similarity within couples by applying MR to the UKBB data. We analysed 118 traits, representing a wide range of anthropometric-, behavioural- and disease-related traits. Among the 118 phenotypes tested, we found widespread evidence of causal effects among partners. In particular, we identified 64 same-trait causal effects within partners (of 118 traits) and no evidence of heterogeneity among same-trait couple MR estimates ( \(\alpha _{x_{\rm{i}} \to x_{\rm{p}}}\) ). This suggests that associations between a person’s genotype and their partner’s phenotype are primarily acting indirectly through the cross-partner causal relationship between the trait(s) associated with the genotype, rather than the presence of a direct effect for index genotype to the partner’s phenotype.
Our results suggest that fitness and anthropometric measures are important initially (at the time of mate choice), but their correlation decreases with time; the longer partners stay together, the less important it gets to remain similar. On the other hand, we found that couples become more similar with respect to smoking cessation and aspirin use as their age increases. As age and time spent together are highly correlated, it is difficult to distinguish whether this is an effect of convergence or of age-dependent partner choice. We did not find any significant trends of causal MR effects on time spent together or by age. While this could be because of limitations such as statistical power, this is consistent with previous reports which suggest that initial partner choice is more important than convergence 9 , 30 , 31 , 32 .
Deriving an analytical formula for the bias in the cross-partner MR estimation allowed a detailed analysis of model parameters that contribute most to such potential bias. Our analysis revealed that the largest contribution to the bias is direct environmental assortment combined with strong parental effects. This conclusion prompted us to explore potential confounders for each examined trait.
When investigating the impact of common confounders on our entire panel of phenotypes (that is, fixing a confounder and assessing its widespread impact on all single-trait AM), we found that household income, age completed education and participant of a sport club or gym are important confounders, explaining on average 29.8, 11.6 and 17.1% of the phenotypic couple correlations among traits tested, respectively. These results also suggest that phenotypic correlations in couples are significantly confounded and point to a relatively few key traits which are driving observed partner similarity. These confounder traits are strongly intertwined and hence correlated, therefore elucidating that the key driver is not feasible with the data at hand. Overall, we noticed that the tested confounders are not sufficient to account for the gap between couple correlations and causal effects or the latter being incorrectly estimated. Of note, phenotypic correlations in couples are impacted differently by measurement noise than causal effect estimates. While the former estimates are attenuated by a factor of \(\frac{{{\mathrm{Var}}(y)}}{{{\mathrm{Var}}\left(y \right) + s^2}}\) in case the true phenotype y is measured with a noise with variance s 2 , the causal effect estimates do not change noticeably because the exposure and outcome effects are equally diluted. This could lead to an underestimation of confounding effects in our results and may explain why for three traits we observed larger causal effect than correlation.
Our findings investigating cross-trait assortment suggest that causal effects from \(X_{\mathrm{i}}\) to \(Y_{\mathrm{p}}\) are primarily driven by AM through X (that is, \(X_{\rm{i}} \to X_{\rm{p}}\) ) followed by a causal effect within the partner from X to Y (that is, \(X_{\mathrm{p}} \to Y_{\mathrm{p}}\) ). In contrast, a less likely path would be the inverse, whereby the presence of a causal effect from X to Y in an index case is then followed by Y being passed directly from index to partner. These results were expected, as it is more reasonable for couples to influence each other at the exposure level rather than the outcome level, especially since often outcome traits (such as diseases) appear much later than mate choice.
We found 1,088 significant cross-trait causal effects within couples ( ω ), which can be summarized by three categories: (1) driven by assortment on the exposure ( \(\omega = \gamma\) ), (2) driven by assortment on the outcome ( \(\omega = \rho\) ) and (3) not explained by either (that is, ω being greater than both ρ and γ ). Of note, there were fewer cases in category 3, where the causal effect from \(X_{\mathrm{i}}\) to \(Y_{\mathrm{p}}\) was not captured by γ or ρ , suggestive of either a direct effect \(X_{\mathrm{i}}\) to \(Y_{\mathrm{p}}\) or indirect effects through variables we have not explored. An example from the first category involves a positive causal effect of time spent watching television on BMI driven by the fact that partners causally influence each other with respect to time spent watching television, which, in turn, has an impact on BMI at the individual level. On the other hand, an example of the second category includes a positive causal relationship from height to education, with a stronger path through ρ , whereby height (a proxy for ‘dynastic’ wealth) increases educational attainment (found previously 33 ) within a single individual and AM subsequently occurs via education level. Finally, as an example for category 3, we found a negative causal effect of never having smoked on leucocyte count within partners, such that leucocyte count was higher among individuals with partners who smoked. While we also identified a significant effect through γ (AM through smoking), the effect was much stronger through ω . These findings suggest that there could be a direct effect from index partner by way of secondhand smoke. These results are consistent with previous work showing higher white blood cell count in smokers 34 , which might already be achieved by secondhand smoking.
This study has limitations which should be acknowledged. First, to increase statistical power and robustness, we focused on traits available in the UKBB with significant correlation among couples and more than five valid IVs. As a result, anthropometric traits constituted a larger proportion of our traits under study and represent a large percentage of our significant findings. Other phenotypes, such as behavioural and lifestyle traits, were included but had less statistical power due to lower couple correlation and fewer IVs.
Second, using our data, we could not find strong evidence for couple convergence over time. This can be due to these effects being weak or the available data being suboptimal. Indeed, we used data on both age and time together (proxied by time at the same address) to answer this question, but these are poor proxies of the relevant traits. To properly disentangle the relationship between AM and convergence, additional longitudinal data including phenotypes at the time of partner selection would be necessary. A complementary approach could be to contrast genetic and phenotypic correlations; however, it is hard to tell whether differences reflect postmating effects or different (genetic and environmental) correlation to traits under primary assortment 20 .
Third, we have not explored indirect causal paths from \(X_{\mathrm{i}}\) to \(X_{\mathrm{p}}\) through another variable ( Z ) measured in either individual. Given the limited evidence of direct cross-trait, cross-partner effects, the most likely such path would be \(X_{\mathrm{i}} \to Z_{\mathrm{i}} \to Z_{\mathrm{p}} \to X_{\mathrm{p}}\) , leading to an indirect effect of \(\alpha _{X \to Z}\alpha _{Z \to X}\alpha _{Z_{\mathrm{i}} \to Z_{\mathrm{p}}}\) . This implies a bidirectional causal effect between X and Z (within the same individual), but their product is expected to negligible 35 . Also, while assortment/convergence through the exposure ( γ ) and the outcome ( ρ ) represents independent paths from X i to Y p , our results suggest that the computed effects using MR estimates are not perfectly independent. This could potentially be because of the overlap in genetic instruments or bidirectional causal effect between them or because both estimates depend on the causal effect from X to Y . To the best of our ability, we tried to mitigate this bias by (1) using a multivariable Mendelian randomization (MVMR) approach to remove effects of X on Y in the calculation of ρ and (2) first residualizing γ for effects of ρ to ensure independence before summation of the effects. Finally, we were limited to the available traits and white British samples in the UKBB. AM and partner selection are often population specific 36 . Therefore, our findings may not generalize to other populations, and more diverse biobanks are needed to systematically explore the heterogeneity in assortative behaviour.
In summary, we have surveyed 118 complex traits with significant couple correlation in the UKBB and explored the major contributors to the observed couple similarity: partner selection, couple convergence and confounding. We found that cross-trait assortment can largely be explained by single-trait assortments between either trait and substantial causal effects between these traits. Our findings provide insights into possible mechanisms underlying observed partner similarity patterns at an unprecedented scale and resolution.
All analyses were run using the R software (v.3.6.3).
Sample selection and couple definition
This study used the UKBB cohort, a prospective population-based study with over 500,000 adult participants. UKBB has approval from the North West Multi-Centre Research Ethics Committee as a Research Tissue Bank approval. This approval means that researchers do not require separate ethical clearance and can operate under the Research Tissue Bank approval. UKBB also possesses a Human Tissue Authority licence, so a separate Human Tissue Authority licence is not required by researchers who receive samples from the resource.
Couples were identified and selected according to the following procedure. The initial UKBB sample comprised 502,616 individuals. First, participants were filtered to only genotyped, white, unrelated individuals according to the genetic quality control (QC) file. Redacted samples and participants who removed consent were also excluded. After filtering, 337,138 participants remained. Within this sample, we retained individuals coming from households with exactly two unrelated, opposite sex individuals, leaving 108,898 participants. Finally, using the data at data field 6,141, ‘How are people in household related to participant’ pairs were filtered to only include couples who had both responded ‘Husband, wife, or partner’, leaving 103,328 participants or 51,664 couples for downstream analyses (Supplementary Fig. 1 ). Note that some studies have used more stringent criteria for couple definition. For example, Yengo et al. 22 additionally requested couples to have been recruited in the same centre, living in the same location, for the same amount of time, living with the same number of people with the same household income, same Townsend deprivation, same number of smokers and so on. Howe et al. 29 used a very similar definition to ours (resulting in 10% fewer couples than us); however, they restricted their analyses to only couples who reported to live at the same address for the same amount of time. We believe that discrepancies in self-reported data can occur frequently (due to misunderstanding, misreporting) and hence, decided to use a more liberal couple definition to increase sample size at the cost of minor misclassification.
MR uses genetic variants as IVs to assess the presence of a causal relationship. The random distribution of genetic variants at birth reduces the possibility of confounding or reverse causation as explanations for the link between the exposure and outcome in the same way that the random allocation of a therapy in a randomized controlled trial minimizes this risk. MR relies on three core assumptions for the genetic variants. First, IVs must be associated with the exposure of interest (the relevance assumption). Second, IVs must not be associated with any confounder in the exposure–outcome relationship (the exchangeability assumption). Third, IVs must not affect the outcome except through the exposure (the exclusion restriction assumption). There are several methods to estimate the causal effect using MR, the simplest being the Wald method, whereby a ratio is taken between the variant–outcome association and the variant–risk factor association. A natural extension of this approach, known as the inverse-variance weighted (IVW) method, combines multiple IVs, applied in this report 37 . The causal effect of exposure X on the outcome Y , using k genetic variants, is given by
with the corresponding variance \({\mathrm{Var}}\left( {\hat{\alpha} } \right) = \frac{1}{{\mathop {\sum}\limits_k {(\beta _k^X)^2(\sigma _k^Y)^{ - 2}} }}\) , where \(\beta _k^X\) and \(\beta _k^Y\) represent the estimated effects of genetic variant k on X and Y , respectively, and \(\sigma _k^Y\) represents the standard error of \(\beta _k^Y\) . Here, we extended the framework of MR to situations where the exposure and the outcome are measured in different individuals. More specifically, our exposure was a trait of an index individual, and the outcome was the same (or another) trait in its partner.
Phenotype selection and processing
We used an agnostic, phenome-wide approach for selecting phenotypes. Specifically, we first selected phenotypes which were analysed by the Neale group and which had men, women and joint summary statistics available ( http://www.nealelab.is/uk-biobank/ ). This list was intersected with our internal database (application number 16389), leaving 1,278 phenotypes available for analysis. Phenotypes were processed in the filtered QC dataset ( n = 337,138) according to a slightly modified version of the PHEnome Scan ANalysis Tool (PHESANT) pipeline to accommodate the phenotypes that we had available in our database 38 . Continuous variables were transformed to a normal distribution using a rank-preserving inverse normal quantile transformation, while ordinal and binary traits were recategorized according to PHESANT documentation (for example, categories with fewer than ten participants were removed). We then filtered these phenotypes as follows. First, to focus on traits with some indication of assortment, we computed the raw phenotypic correlation ( \(r_{x_{\mathrm{i}}x_{\mathrm{p}}}\) , where x refers to trait X , i represents the index person and p is its partner) among couples and removed phenotypes with a Pearson correlation of <0.1. To ensure that inverse normal quantile transformation was not significantly impacting the correlations of each trait, we also calculated the correlation between partners for each trait using the nonparametric Spearman correlation and found consistent estimates (Supplementary Fig. 2 ). Second, we removed phenotypes that had fewer than five valid IVs for MR. IVs were defined based on an association P < 5 × 10 −8 in the joint Neale summary statistics, after pruning for independence (based on a clumping procedure performed in PLINK 39 with the options –clump-kb 10000 and –clump-r2 0.001 using the 1000 Genomes European samples as a reference). Third, using the sex-specific summary statistics, the IV heterogeneity between sexes was calculated. IVs that showed (Bonferroni-corrected) significant evidence of heterogeneity between sexes were excluded ( P < 0.05/[number of IVs]). After this procedure, phenotypes were again filtered to those with at least five valid IVs remaining. Fourth, dietary phenotypes were removed due to high correlation among these phenotypes (due to the shared household), insufficient power, problems with reverse causation and difficult interpretation 40 . Finally, we manually removed several duplicated and redundant phenotypes. Specifically, (1) left-side body traits (highly correlated with right side) were removed; (2) we retained only one of the duplicated phenotypes for BMI and weight (retaining UKBB data fields 21001 and 21002, respectively); and (3) all ‘qualifications’ data were removed (corresponding to UKBB field 6,138) due to the availability of finer-scale correlated variables, such as ‘age completed full-time education’ (data field 845). This ad hoc procedure was meant to capture only major redundancies. After this process, 118 phenotypes remained for analysis (Supplementary Fig. 3 ).
Estimation of single-trait causal effects in couples
To investigate the causal effect of a trait in one individual on the same trait of their partner, we performed couple-specific MR analyses. Specifically, the trait in the index case was used as the exposure and the same trait in the partner was used as the outcome trait. The effect of genetic variants on the exposure was obtained from the Neale summary statistics using the full UKBB sample. Instruments for each trait were selected as described above: that is, being both genome-wide significant ( P < 5 × 10 −8 ) and pruned for independence. Next, we estimated the effects of single-nucleotide polymorphisms (SNPs) on the outcomes of interest by testing the association between each genetic instrument measured in the index individual with the phenotype measured in the partner using the UKBB partner dataset described above. In other words, for each phenotype, the corresponding genetic data for the IVs were obtained from the index case, while the phenotypes (dependent variable) were taken from the corresponding partner. All SNP trait estimates were estimated in men and women separately (that is, using the sex-specific Neale summary statistics or two separate models in the couple data), adjusting for age and the first 40 genetic principal components of both the index and partner. To mimic the Neale models, we performed linear regression of SNP effects on phenotypes, regardless of data type (including binary). Continuous phenotypes were scaled to have mean of zero and s.d. of one before regression, while ordinal and binary phenotypes were left as processed by PHESANT.
To estimate the causal effect of a trait from an index case to a partner ( \(\alpha _{x_{\mathrm{i}} \to x_{\mathrm{p}}}\) , we combined the effects of genetic instruments on the exposure (from Neale) with effects on the outcomes (measured among couples) in an MR framework using the IVW method (Fig. 2a ) 37 . To estimate the causal effects in both sexes combined, SNP effects were first meta-analysed across sexes using fixed effects models before performing MR (rather than meta-analysing the MR estimates directly) to minimize weak instrument bias 41 . Effects of the genetic estimates on both the exposure and outcome were first standardized (such that the squared effect size represents the explained variance) to allow for seamless comparison across traits and to the raw phenotype correlation. Significance was determined by adjusting for the number of effective tests based on the correlation matrix of phenotypes tested 42 , resulting in 66 independent tests. The significance threshold was adapted accordingly as P < 0.05/66.
After estimating single-trait causal effects in couples, we used a two-tailed Z test to identify traits with a significant difference between the MR estimate and the phenotypic correlation in couples. For each trait with discrepant estimates, we tested the causal effect of each of the remaining 117 phenotypes in our pipeline ( \(Y_1, \ldots ,Y_{117}\) ) on the focal trait of interest ( X ) using MR ( \(\alpha _{y \to x}\) ). These same-person MR estimates were calculated using meta-analysed sex-specific Neale estimates for both the SNP exposure and SNP outcome effects using the IVW method. Before performing each same-person MR, genetic variants were first filtered for evidence of reverse causality at a threshold of P < 0.001 (Steiger filter) 43 , whereby SNPs were removed if the standardized SNP effect on the outcome was stronger than the effect on the exposure based on a one-tailed t test at a significance level of P < 0.001. SNP effects were standardized before calculating MR effects.
We then explored those potential confounders, \(Y_1, Y_2, \dots, Y_{117}\) , with a significant impact on X ( P < 0.05/66). As the confounding impact of each \(Y_k\) involves a within-couple effect ( \({\upalpha}_{y_{\mathrm{i}} \to y_{\mathrm{p}}}\) ), as illustrated in Fig. 2b , we further filtered the remaining \(Y_k\) traits to those with a significant within-couple MR effect ( P < 0.05/[number of remaining \(Y_k\) ]). The couple correlation induced by confounder Y can be expressed as \(\alpha _{y \to x}^2 \times \alpha _{y_{\mathrm{i}} \to y_{\mathrm{p}}}\) . We restricted confounders to only those that had a correlation with X less than 0.8 to avoid using meaninglessly similar traits to X . Since potential confounders may be correlated, one could use MVMR to assess the joint contribution of these confounders on the couple correlation for trait X . This, however, led to numerical instability, and we decided to rather prune confounders ( \(r^2 < 0.1\) , prioritizing for larger \({\hat{\alpha}}_{y \to x}^2 {\hat{\alpha}}_{y_{\mathrm{i}} \to y_{\mathrm{p}}}\) values) and obtain \({\hat{\alpha}} _{y \to x}\) estimated from univariable MR, where IVs were pruned for independence ( \(r^2 < 0.001\) ). Finally, we plugged in the obtained causal effect estimates for the m remaining confounder traits ( \(Y_1,Y_2, \ldots ,Y_m\) ) on X , ( \({\hat{\alpha}} _{Y_1 \to {{X}}},{\hat{\alpha}} _{Y_2 \to {{X}}}, \ldots ,{\hat{\alpha}} _{Y_m \to {{X}}}\) ), into the estimator of the correlation induced by these confounders to get the estimate of total confounding \(C = \mathop {\sum }\limits_{{j} = 1}^{{{\mathrm{m}}}} {\hat{\alpha}}_{\left({Y_j} \right)_{{{\mathrm{i}}}} \to \left({Y_j} \right)_{{{\mathrm{p}}}}} ({\hat{\alpha}}_{Y_j \to {{X}}})^2\) . The variance of such a sum was estimated as the sum of the variances of the individual terms (since they are uncorrelated). The variance of \(({\hat{\alpha}}_{Y_j \to {{X}}})^2\) was approximated by assuming \({\hat{\alpha}}_{Y_j \to {{X}}}\) following a normal distribution and used the general formula of \({\mathrm{Var}}\left({X^2} \right) = 4\mu ^2\sigma ^2 + 2\sigma ^4\) for \(X\approx N(\mu ,\sigma ^2)\) . The variance of the product of \({\hat{\alpha}}_{\left( {Y_j} \right)_{{{\mathrm{i}}}} \to \left( {Y_j} \right)_{{{\mathrm{p}}}}}\) and \(({\hat{\alpha}}_{Y_j \to {{X}}})^2\) was estimated based on the formula for the variance of the product of independent random variables: \({\mathrm{Var}}\left( {XY} \right) = {\mathrm{Var}}\left( X \right){\mathrm{Var}}\left( Y \right) + {\mathrm{Var}}\left( X \right)E^2\left( Y \right) + {\mathrm{Var}}(Y)E^2\left( X \right)\) .
The role of confounders on trait correlation in couples
We sought to explore the impact of potential confounders on mate choice by calculating the trait correlations between partners that are due to confounding. We considered the impact of the following confounders ( Y ) on the partner correlations of the remaining 117 traits selected by our pipeline: average household income, age completed full-time education, sports club or gym user, current tobacco smoking, overall health rating, and north and east birth place coordinates (UKBB data fields 738; 845; 6160; 1239; 2178; 129; and 130, respectively). The choice of these traits was somewhat ad hoc, mostly driven by previous evidence for driving trait similarities and themselves showing strong couple correlation in the UKBB. Using the single-trait causal effects in couples and the same-person MR estimates, correlation due to founding was calculated for each pair \((Y,X)\) as \(C = \alpha _{y \to x}^2 \alpha _{y_{\mathrm{i}} \to y_{\mathrm{p}}}\) (Fig. 2b ). These confounding estimates were finally contrasted to the couple correlation values to explore the extent that each Y may confound couple correlations by examining the ratio between the two estimates (that is, \(\frac{C}{{\mathrm{cor}}(X_{\mathrm{i}},X_{\mathrm{p}})}\) ). Birthplace coordinates (east–west and north–south) were considered together and their invoked trait correlations were summed up, as they are orthogonal by definition.
Trait convergence over time
Trait similarity in couples can be driven by both mate choice and/or trait convergence over time spent together. To tease out the contribution of these different sources, we explored whether the cross-partner causal effects change as a function of the length of the relationship and age. The length of relationship was proxied by the minimum value of the ‘length of time at current address’ (data field 699) for the two partners. To estimate the effect of age, we took the median age of couples. For each of the two derived variables, we split the couples into five roughly equal-sized bins (using the ‘smart_cut’ function from the cutr R package). We first estimated the phenotypic correlation of each trait within couples of each bin. Next, for each single-trait MR described above, analyses were run in the full sample as well as in the different bins. Of the significant results identified in the sex-combined analysis above, we tested to see if there was any significant difference in MR estimates among the bins. Binned MR estimates were computed using the SNP outcome effect estimated in each bin separately, and the SNP outcome effects used the same SNP exposure effects from Neale. Analyses were run in each sex separately and combined (meta-analysed at the SNP level). As above, SNP effects were standardized before calculating MR estimates. To assess for the presence of a trend across bins, we tested the significance of the slope of a linear model of bin-specific correlations and bin-specific MR estimates, inversely weighted by the SE, versus the bin centre (that is, the median age or time spent together for the given bin). Multiple testing was, as described above, adapted based on the effective number of tests but restricted to traits which showed significant causal effects in the joint (both sexes combined), nonbinned MR (resulting in a threshold of P < 0.05/29).
Estimation of cross-trait causal effects in couples
Using the same process as in the AM analysis involving a single trait, we also sought to investigate causal effects within couples involving two traits ( \(\alpha _{x_{\mathrm{i}} \to y_{\mathrm{p}}}\) ). In other words, two different traits were used as exposure and outcome to determine the causal effects of trait X (in the index individual) on trait Y (in the partner). Here, we only considered trait combinations with phenotypic correlation of <0.8 (estimated in the entire UKBB, n = 337,138) to avoid too closely related traits. The same set of SNPs was used as in the same-person MR (that is, first filtered for the presence of reverse causality). As in the single-trait MR, SNP exposure effects were obtained from the Neale summary statistics and SNP outcome effects were estimated in the couple-derived dataset. MR models were run in both sexes separately and jointly (meta-analysing the SNP effects before performing MR analyses). Significance was determined based on the squared effective number of tests ( P < 0.05/[66 2 ]).
Comparison of paths from index to partner
There are several independent paths through which a trait in an index case could exert a causal effect on another trait in the partner. We wanted to explore if one path was more dominant, in general, and if there was evidence for the presence of direct effects (or indirect effects with additional traits involved). Restricting to Bonferroni-significant trait pairs (with phenotypic correlation of <0.8) from the couple MR, we sought to explore the various paths through which a phenotype X in an index case ( X i ) could causally impact a phenotype Y in the partner ( Y p ) as illustrated in Fig. 2c . With the exception of exposure traits that directly alter the environment of their partner, such as smoking creating the presence of secondhand smoke, X i is unlikely to have a direct effect on another Y p . Alternatively, X i might indirectly impact Y p by inducing changes in X p , which in turn, impacts Y p . For instance, increased BMI in an index case is not expected to directly increase cardiovascular disease risk in their partner but rather, to modify the partner’s risk through first increasing their BMI. To explore this intuition, we dissected the causal effect from X i to Y p ( ω ) into three possible (nonindependent) mechanisms. First, X i could exert a causal effect on X p , followed by X p having a causal effect on Y p in the partner alone ( γ ). Second, the reverse could occur, whereby \(X_i\) has a causal effect on Y i in the index alone, followed by a causal effect of Y i case on Y p ( ρ ). Third, there could be other mechanisms, either acting directly or through other unmeasured/considered variables. These three scenarios could also act in some combination. In this way, the ω estimate would capture the paths of γ , ρ and other mechanisms combined.
Using the same-person MR estimates that were calculated as described above, we estimated γ and ρ representing the various paths from X i to Y p and contrasted them to the total cross-trait cross-partner effect ω . To quantify γ , the single-trait couple causal effect estimate (that is, from the regression \(X_{\mathrm{p}} \sim X_{\mathrm{i}}\) ) was multiplied by the same-individual causal estimate (that is, \(\alpha _{x \to y}\) from \(Y \sim X\) ). To quantify ρ , we first estimated the causal effect of Y i on Y p in MVMR to exclude any residual effect of X on phenotype Y from index to partner. Specifically, Y p was used as the independent variable with both Y i and X i as independent variables (that is, the MVMR was \(Y_{\mathrm{p}} \sim Y_{\mathrm{i}} + X_{\mathrm{i}}\) ). We included both IVs from X and Y , pruned for independence (performed in PLINK with the options –clump-kb 10000 and –clump-r2 0.001 using the 1000 Genomes European samples as a reference). We took the coefficient of Y i as the direct causal effect from Y i to Y p ( \(\alpha _{y_{\mathrm{i}} \to y_{\mathrm{p}}}\) ) and multiplied this by the same-individual causal estimate ( \(\alpha _{x \to y}\) ). Finally, we estimated ω directly from our cross-trait couple MR framework ( \(\alpha _{x_{\mathrm{i}} \to y_{\mathrm{p}}}\) ). We compared the estimates of γ , ρ and ω using a Z test to assess their difference and using linear regression with the intercept forced through the origin to determine their relationship. Finally, we quantified the proportion of ω that could not be explained by the paths quantified by γ and ρ . As γ and ρ are not perfectly independent, potentially due to correlation between X and Y or pleiotropic limitations of MR, we estimated the extent of dependence via the correlation between ρ and γ across the different trait pairs. To account for the duplicate signals due to this correlation, we removed the effects of γ from ρ by keeping the residuals from the linear regression \(\rho \sim \gamma\) . We then estimated the proportion of variance explained ( \(R^2\) ) of ω jointly by γ and the residualised ρ .
Biases in the causal effect estimation
Violations of the MR assumptions are different in applications like ours, where the exposure and outcome are the same trait but measured in different individuals. Hartwig et al. 44 explored the impact of AM on MR but for the classical setting of testing different traits in the same individual. Therefore, we set up a model (Fig. 7 ) specific to causal effect estimations where exposures and outcomes are the same traits but in different individuals (couples) to examine the potential issues. We have allowed for a more complex set of MR violations, which we describe below.
The model focuses on trait X in the offspring (O) ( \(X_{\mathrm{O}}^{\mathrm{i}}\) ) and that in the partner ( \(X_{\mathrm{O}}^{\mathrm{p}}\) ). Analogously, we denoted the same trait in the offspring’s mother and father with subscripts M and F. These traits are influenced by direct genetic effects ( \(G_{\mathrm{O}}^{\mathrm{i}}\) ) and direct (non-genetic) environmental effects ( \(E_{\mathrm{O}}^{\mathrm{i}}\) ), with effect sizes g and e, respectively. Everything derived below works the same way even if \(G_{\mathrm{O}}^{\mathrm{i}}\) represents a single SNP (and hence, \(E_{\mathrm{O}}^{\mathrm{i}}\) is heritable). The offspring environment is influenced by the parental environment ( \(E_{\mathrm{P}}^{\mathrm{i}}\) ), the parental genes ( \(G_{\mathrm{P}}^{\rm{i}}\) ) and the parental trait ( \(X_{\mathrm{P}}^{\mathrm{i}}\) ). Parental characteristics ( \(G,E,X\) ) are simply defined as a rescaled average of the maternal and paternal traits: that is, \(X_{\mathrm{P}}^{\mathrm{i}}\) = \((X_{\mathrm{M}}^{\mathrm{i}} + X_{\mathrm{F}}^{\mathrm{i}})/\sqrt {2 \left({1 + {\mathrm{cor}}(X_{\mathrm{M}}^{\mathrm{i}},X_{\mathrm{F}}^{\mathrm{i}})} \right.}\) to ensure that the trait variance is equal to 1, i.e. \({\mathrm{Var}}(X_{\mathrm{P}}^{\rm{i}}) = 1\) . Note that this simplification assumes that paternal and maternal effects are identical, which holds in general 32 . The direct causal effects of \(G_{\mathrm{P}}^{\mathrm{i}},E_{\mathrm{P}}^{\mathrm{i}}\) and \(X_{\mathrm{P}}^{\mathrm{i}}\) on \(E_{\mathrm{O}}^{\mathrm{i}}\) are denoted by \(s_{\mathrm{G}},s_{\mathrm{E}}\) and \(s_{\mathrm{X}}\) , respectively. By definition, the correlation between mean parental genotype and offspring genotype is \(1/\sqrt 2\) . Parental traits ( \(E_{\mathrm{P}}^{\mathrm{i}},X_{\mathrm{P}}^{\mathrm{i}}\) ) cannot modify offspring genotype; thus, they can influence \(X_{\mathrm{O}}^{\mathrm{i}}\) only via its environmental component ( \(E_{\mathrm{O}}^{\mathrm{i}}\) ). The same description holds for all corresponding variables of the partner. Finally, \(X_{\mathrm{O}}^{\mathrm{i}}\) and \(X_{\mathrm{O}}^{\mathrm{p}}\) are paired such that predefined correlations between \(G,E\) and \(X\) are satisfied. For this, direct assortment coefficients \(r_{\mathrm{G}},r_{\mathrm{E}}\) and \(r_{\mathrm{X}}\) are defined between \(G,E\) and X , respectively.
As can be seen from Fig. 7 , parental effects induce a correlation of \(\left( {\frac{1}{{\sqrt 2 }}} \right) (s_{\mathrm{G}} + s_{\mathrm{X}}\times g)\) between the offspring genotype ( \(G_{\mathrm{O}}^{\mathrm{i}}\) ) and the offspring environment ( \(E_{\mathrm{O}}^{\mathrm{i}}\) ). Also, one can note that the total couple correlation for X can arise from three independent sources: direct X assortment ( \(r_{\mathrm{X}}\) ), through direct assortment for \(E\) ( \(r_{\mathrm{E}} \times e^2\) ) and through direct assortment through \(G\) ( \(r_{\mathrm{G}} \times g^2\) ). Thus, the expected correlation between \(X_{\mathrm{O}}^{\mathrm{i}}\) and \(X_{\mathrm{O}}^{\mathrm{p}}\) is \(r_{\mathrm{X}} + r_{\mathrm{E}} \times e^2 + r_{\mathrm{G}} \times g^2\) . Similarly, the expected correlation between \(E_{\mathrm{O}}^{\mathrm{i}}\) and \(E_{\mathrm{O}}^{\mathrm{p}}\) is \(r_{\mathrm{E}} + r_{\mathrm{X}} \times e^2 + r_{\mathrm{G}} \left( {\left( {\frac{1}{{\sqrt 2 }}} \right) (s_{\mathrm{G}} + g \times s_{\mathrm{X}})} \right)^2\) and between \(G_{\mathrm{O}}^{\mathrm{i}}\) and \(G_{\mathrm{O}}^{\mathrm{p}}\) is \(r_{\mathrm{G}} + r_{\mathrm{X}} \times g^2 + r_{\mathrm{E}} \left( {\left( {\frac{1}{{\sqrt 2 }}} \right) (s_{\mathrm{G}} + g \times s_{\mathrm{X}})} \right)^2\) .
Under this model, the expected effect of the index genotype on the index trait is
while the expected effect of the index genotype on its partner’s trait is
Therefore, the expectation of the estimated causal effect \(X_{\mathrm{O}}^{\mathrm{i}} \to X_{\mathrm{O}}^{\mathrm{p}}\) can be written as
Several parameters in this model can lead to the violation of MR assumptions
Violation 1 ( \({{{\boldsymbol{s}}}}_{{{\bf{{{G}}}}}} \ne 0\) ): this violation implies that parental genetics directly impact offspring environment (not through E or X ). Such violation would most likely happen if \(G_{\mathrm{P}}^{\mathrm{i}}\) impacts another parental phenotype \(Y_{\mathrm{P}}^{\mathrm{i}}\) which has an impact on \(E_{\mathrm{O}}^{\mathrm{i}}:\) that is, \(s_{\mathrm{G}} = g \cdot r_{\mathrm{G}}\left( {X,Y} \right) s_Y\) . Here, \(r_{\mathrm{G}}\left( {X,Y} \right)\) refers to the genetic correlation between traits X and Y . The formula assumes that the effect of \(G_{\mathrm{P}}^{\mathrm{i}}\) to a secondary trait ( Y ) is expected to be its genetic effect on the primary trait ( X ) multiplied by their genetic correlation.
Violation 2 ( \({{{\boldsymbol{s}}}}_{{{\bf{{{X}}}}}} \ne 0\) ): this violation is the classical parental rearing (or in other terms, dynasty) effect.
Violation 3 ( \({{{\boldsymbol{s}}}}_{{\bf{E}}} \ne 0\) ): this violation does not lead to MR bias since there is no path from the offspring genotype to the offspring environment through the parental environment.
Violation 4 \(\left({{{\boldsymbol{r}}}}_{{\bf{G}}} \ne 0\right):\) this violation allows direct assortment based on index and partner genetics, which most likely happens due to assortment for another trait ( Y ). Hence, its typical size is \(r_{\mathrm{G}} = (g \times r_{\mathrm{G}}\left( {X,Y} \right))^2 r_Y\) . Here, we made a similar assumption as for Violation 1. One (toy) example for such scenario could be educational attainment ( X ), whose genetic component is strongly associated with intelligence, for which additional assortment occurs.
Violation 5 ( \({{{\boldsymbol{r}}}}_{{{\bf{{{E}}}}}} \ne 0\) ): this violation allows direct assortment based on the environment of the index and partner. A simplified example for this scenario is trait X being BMI and E representing ‘going to the gym’. In such case, beyond AM for BMI, people are more likely to choose their partners from the gym (selecting for fitter individuals); hence; the environment has an additional direct effect of the partner’s BMI.
To gauge the extent of the MR bias in realistic parameter settings, we have visualized the bias for a wide range of parameters. We set \(r_{\mathrm{X}} = 0.2\) , the heritability to 20% (hence, \(g = \sqrt {0.2}\) and \(e = \sqrt {1 - 0.2}\) ). Then, two of the remaining four crucial parameters ( \(s_{\mathrm{G}},s_{\mathrm{X}};r_{\mathrm{E}},r_{\mathrm{G}}\) ) were fixed and the other two were varied. For example, the chosen range for \(r_{\mathrm{G}}\) assumed a value of \(r_{\mathrm{G}}\left( {X,Y} \right) = 0.3\) and \(r_Y\) range of [−0.3, 0.3] using the abovementioned formula of \(r_{\mathrm{G}} = (g \times r_{\mathrm{G}}\left( {X,Y} \right))^2 r_Y\) . Similarly, we used the formula \(s_{\mathrm{G}} = g \times r_{\mathrm{G}}\left( {X,Y} \right) s_Y\) to justify the explored range for \(s_{\mathrm{G}}\) , with \(r_{\mathrm{G}}\left( {X,Y} \right) = 0.3\) and \(s_Y\) between −0.3 and 0.3.
Reporting summary
Further information on research design is available in the Nature Portfolio Reporting Summary linked to this article.
Data availability
The UK Biobank data are available through the standard UK Biobank application procedure ( https://www.ukbiobank.ac.uk/enable-your-research/apply-for-access ). The household information can be separately requested from the UK Biobank access team, as it is not part of the variables listed on the showcase ( https://biobank.ndph.ox.ac.uk/showcase/index.cgi ). The 1000 Genomes European genetic data can be downloaded from http://ftp.1000genomes.ebi.ac.uk/vol1/ftp/ .
Code availability
Custom code is available at https://github.com/jennysjaarda/proxyMR .
Willoughby, E. A. et al. Parent contributions to the development of political attitudes in adoptive and biological families. Psychol. Sci. 32 , 2023–2034 (2021).
Article PubMed PubMed Central Google Scholar
Kandler, C., Bleidorn, W. & Riemann, R. Left or right? Sources of political orientation: the roles of genetic factors, cultural transmission, assortative mating, and personality. J. Pers. Soc. Psychol. 102 , 633–645 (2012).
Article PubMed Google Scholar
Silventoinen, K., Kaprio, J., Lahelma, E., Viken, R. J. & Rose, R. J. Assortative mating by body height and BMI: Finnish twins and their spouses. Am. J. Hum. Biol. 15 , 620–627 (2003).
Maes, H. H., Neale, M. C. & Eaves, L. J. Genetic and environmental factors in relative body weight and human adiposity. Behav. Genet. 27 , 325–351 (1997).
Article CAS PubMed Google Scholar
Keller, M.C. The genetic correlation between height and IQ: shared genes or assortative mating? PLoS Genet. 9 , e1003451 (2013).
Article CAS PubMed PubMed Central Google Scholar
Mare, R. D. Five decades of educational assortative mating. Am. Sociological Rev. 56 , 15–32 (1991).
Article Google Scholar
Agrawal, A. et al. Assortative mating for cigarette smoking and for alcohol consumption in female Australian twins and their spouses. Behav. Genet. 36 , 553–566 (2006).
Buss, D. M. Marry someone who is similar to us in almost every variable. Am. Sci. 73 , 47–51 (1985).
Google Scholar
Watson, D. et al. Match makers and deal breakers: analyses of assortative mating in newlywed couples. J. Personal. 72 , 1029–1068 (2004).
Hippisley-Cox, J. Married couples’ risk of same disease: cross sectional study. Brit. Med. J. 325 , 636–636 (2002).
Buss, D. M. et al. International preferences in selecting mates. J. Cross-Cultural Psychol. 21 , 5–47 (1990).
Buss, D. M. & Barnes, M. Preferences in human mate selection. J. Personal. Soc. Psychol. 50 , 559–570 (1986).
Anderson, C., Keltner, D. & John, O. P. Emotional convergence between people over time. J. Personal. Soc. Psychol. 84 , 1054–1068 (2003).
Gonzaga, G. C., Campos, B. & Bradbury, T. Similarity, convergence, and relationship satisfaction in dating and married couples. J. Personal. Soc. Psychol. 93 , 34–48 (2007).
Humbad, M. N., Donnellan, M. B., Iacono, W. G., McGue, M. & Burt, S. A. Is spousal similarity for personality a matter of convergence or selection? Personal. Individ. Differen. 49 , 827–830 (2010).
Risch, N. Ancestry-related assortative mating in Latino populations. Genome Biology 10 , R132 (2009).
Sebro, R., Peloso, G. M., Dupuis, J. & Risch, N. J. Structured mating: patterns and implications. PLoS Genet. 13 , e1006655 (2017).
Abdellaoui, A. et al. Genetic correlates of social stratification in Great Britain. Nat. Hum. Behav. 3 , 1332–1342 (2019).
Rawlik, K., Canela-Xandri, O. & Tenesa, A. Indirect assortative mating for human disease and longevity. Heredity 123 , 106–116 (2019).
Robinson, M. R. et al. Genetic evidence of assortative mating in humans. Nat. Hum. Behav. 1 , 0016 (2017).
Xia, C., Canela-Xandri, O., Rawlik, K. & Tenesa, A. Evidence of horizontal indirect genetic effects in humans. Nat. Hum. Behav. 5 , 399–406 (2020).
Yengo, L. et al. Imprint of assortative mating on the human genome. Nat. Hum. Behav. 2 , 948–954 (2018).
Border, R. Assortative mating biases marker-based heritability estimators. Nat. Commun. 13 , 660 (2022).
Border, R. et al. Cross-trait assortative mating is widespread and inflates genetic correlation estimates. Science 378 , 754–761 (2022).
Beauchamp, J. P., Cesarini, D., Johannesson, M., Lindqvist, E. & Apicella, C. On the sources of the height–intelligence correlation: new insights from a bivariate ACE model with assortative mating. Behav. Genet 41 , 242–252 (2011).
Howe, L. J. et al. Assortative mating and within-spouse pair comparisons. PLoS Genet. 17 , e1009883 (2021).
Lawlor, D. A. et al. Mendelian randomization: using genes as instruments for making causal inferences in epidemiology. Stat. Med. 27 , 1133–1163 (2008).
Holmes, M. V. et al. Causal effects of body mass index on cardiometabolic traits and events: a Mendelian randomization analysis. Am. J. Hum. Genet 94 , 198–208 (2014).
Howe, L.J. Genetic evidence for assortative mating on alcohol consumption in the UK Biobank. Nat. Commun. 10 , 5039 (2019).
Mascie-Taylor, C. G. N. Spouse similarity for IQ and personality and convergence. Behav. Genet. 19 , 223–227 (1989).
Caspi, A., Herbener, E. S. & Ozer, D. J. Shared experiences and the similarity of personalities: a longitudinal study of married couples. J. Personal. Soc. Psychol. 62 , 281–291 (1992).
Article CAS Google Scholar
Yengo, L. et al. No evidence for social genetic effects or genetic similarity among friends beyond that due to population stratification: a reappraisal of Domingue et al (2018). Behav. Genet. 50 , 67–71 (2019).
Tyrrell, J. Height, body mass index, and socioeconomic status: Mendelian randomisation study in UK Biobank. BMJ 352 , i582 (2016).
Pedersen, K. M. et al. Smoking and increased white and red blood cells: a Mendelian randomization approach in the Copenhagen General Population Study. Arter. Thromb. Vasc. Biol. 39 , 965–977 (2019).
Darrous, L., Mounier, N. & Kutalik, Z. Simultaneous estimation of bi-directional causal effects and heritable confounding from GWAS summary statistics. Nat. Commun. 12 , 7274 (2021).
Stulp, G., Simons, M.J., Grasman, S. & Pollet, T.V. Assortative mating for human height: a meta-analysis. Am. J. Hum. Biol. 29 , e22917 (2017).
Burgess, S., Butterworth, A. & Thompson, S. G. Mendelian randomization analysis with multiple genetic variants using summarized data. Genet. Epidemiol. 37 , 658–665 (2013).
Millard, L. A. C., Davies, N. M., Gaunt, T. R., Smith, G. D. & Tilling, K. Software application profile: PHESANT: a tool for performing automated phenome scans in UK Biobank. Int. J. Epidemiol. 47 , 29–35 (2018).
Purcell, S. et al. PLINK: a tool set for whole-genome association and population-based linkage analyses. Am. J. Hum. Genet. https://doi.org/10.1086/519795 (2007).
Pirastu, N. et al. Using genetic variation to disentangle the complex relationship between food intake and health outcomes. PLoS Genet. 18 , e1010162 (2022).
Burgess, S., Small, D. S. & Thompson, S. G. A review of instrumental variable estimators for Mendelian randomization. Stat. Methods Med. Res. 26 , 2333–2355 (2017).
Gao, X., Starmer, J. & Martin, E. R. A multiple testing correction method for genetic association studies using correlated single nucleotide polymorphisms. Genet. Epidemiol. 32 , 361–369 (2008).
Hemani, G., Tilling, K. & Davey Smith, G. Orienting the causal relationship between imprecisely measured traits using GWAS summary data. PLoS Genet. 13 , e1007081 (2017).
Hartwig, F. P., Davies, N. M. & Davey Smith, G. Bias in Mendelian randomization due to assortative mating. Genet Epidemiol. 42 , 608–620 (2018).
Download references
Acknowledgements
For computations, we used the CHUV HPC cluster. We thank C. Auwerx, M. Sadler and A. van der Graaf for their valuable feedback on the manuscript. This research has been conducted using the UK Biobank Resource under Application Number 16389. Z.K. was funded by the Swiss National Science Foundation (310030-189147). The funders had no role in study design, data collection and analysis, decision to publish or preparation of the manuscript.
Open access funding provided by University of Lausanne.
Author information
Authors and affiliations.
University Center for Primary Care and Public Health, Lausanne, Switzerland
Jennifer Sjaarda & Zoltán Kutalik
Department of Computational Biology, University of Lausanne, Lausanne, Switzerland
Swiss Institute of Bioinformatics, Lausanne, Switzerland
You can also search for this author in PubMed Google Scholar
Contributions
Z.K. devised and directed the project. J.S. and Z.K. contributed to the mathematical derivations, design and implementation of the research; to the analysis of the results; and to the writing of the manuscript.
Corresponding author
Correspondence to Zoltán Kutalik .
Ethics declarations
Competing interests.
The authors declare no competing interests.
Peer review
Peer review information.
Nature Human Behaviour thanks Laurence Howe, Emily Willoughby and the other, anonymous, reviewer(s) for their contribution to the peer review of this work. Peer reviewer reports are available.
Additional information
Publisher’s note Springer Nature remains neutral with regard to jurisdictional claims in published maps and institutional affiliations.
Supplementary information
Supplementary information.
Supplementary Figs. 1–3.
Reporting Summary
Peer review file, supplementary table 1.
Summary of all age- and sex-specific results for all 118 traits. Phenotypic correlation among couples (pheno_couple_r2, pheno_couple_r, pheno_couple_r_se), MR estimates (n_exposure, n_outcome, IVW_beta, IVW_se, IVW_pval), differences between phenotypic estimates and MR estimates (couple_r_versus_IVW_diff_pval), sex-specific MR estimates and differences (sex_het_pval, IVW_beta_male, IVW_se_male, IVW_pval_male and female), and model estimates to assess the trend across bins by both age and time spent together for (1) MR estimates (weighted by SE), (2) Pearson correlations and (3) Spearman correlations (column names are given as bin_slope_beta, bin_slope_se, bin_slope_pval, with suffixes MR_wt, pearson and spearman to denote the different models and age and time_together to denote the different binning category). Note that n_pairs refers to the number of couples who each have data for the specified phenotype to compute couple correlations (couple_r2, couple_r and so on), n_exposure refers to the number of participants in the Neale models (sample size summed across men and women models) and n_outcome refers to the number of participants in the models estimating the effect of SNPs on the outcome of interest. Specifically, the model estimated the association between each genetic instrument measured in the index individual with the phenotype measured in the partner using the UKBB partner data set described. As these models were performed in males and females separately and do not require phenotype information for each partner, the result n_outcome is larger than n_pairs. All P values presented in this table come from two-sided P values.
Supplementary Table 2
Summary of the identified potential confounders. Of the 40 traits showing phenotypic correlations significantly larger than MR estimates, we were able to identify at least one potential confounder for all but one trait. Potential confounders were pruned to only those that had correlation with any other potential confounder ( \(Y_{m}\) ) <0.1, and the resulting C statistics were summed (C-sum).
Supplementary Table 3
Summary of the global confounding impact of six selected traits on phenotypic couple correlations. The confounding ratio corresponds to the ratio of correlation due to confounding over phenotypic correlation in couples (that is, \({\mathrm{C}}/{\mathrm{cor}}({\mathrm{X}}_{{\mathrm{i}}},{\mathrm{X}}_{{\mathrm{p}}})\) , where \({\mathrm{C}} = {\upalpha}_{{\mathrm{y}} \to {\mathrm{x}}}^2 \times {\upalpha}_{{\mathrm{y}}_{{\mathrm{i}}} \to {\mathrm{y}}_{{\mathrm{p}}}}\) ).
Supplementary Table 4
Summary of the 1,088 significant two-trait MR associations. Results are pruned such that pair A–B and C–D and if max(corr(A,C)corr(B,D),corr(A,D) × corr(B,C)) > 0.8, then one pair is dropped, prioritized by lower \(p_{{\hat{\omega}}}\) . P values were computed based on a Z test (estimate difference divided by the s.e. of the parameter estimate differences).
Supplementary Table 5
Summary of the linear regression results comparing different mediated and total effects between traits across partners. P values come from a normal linear regression of this model of the form V ≈ ω + 0.
Rights and permissions
Open Access This article is licensed under a Creative Commons Attribution 4.0 International License, which permits use, sharing, adaptation, distribution and reproduction in any medium or format, as long as you give appropriate credit to the original author(s) and the source, provide a link to the Creative Commons license, and indicate if changes were made. The images or other third party material in this article are included in the article’s Creative Commons license, unless indicated otherwise in a credit line to the material. If material is not included in the article’s Creative Commons license and your intended use is not permitted by statutory regulation or exceeds the permitted use, you will need to obtain permission directly from the copyright holder. To view a copy of this license, visit http://creativecommons.org/licenses/by/4.0/ .
Reprints and permissions
About this article
Cite this article.
Sjaarda, J., Kutalik, Z. Partner choice, confounding and trait convergence all contribute to phenotypic partner similarity. Nat Hum Behav 7 , 776–789 (2023). https://doi.org/10.1038/s41562-022-01500-w
Download citation
Received : 20 April 2022
Accepted : 17 November 2022
Published : 16 March 2023
Issue Date : May 2023
DOI : https://doi.org/10.1038/s41562-022-01500-w
Share this article
Anyone you share the following link with will be able to read this content:
Sorry, a shareable link is not currently available for this article.
Provided by the Springer Nature SharedIt content-sharing initiative
This article is cited by
Genetic similarity between relatives provides evidence on the presence and history of assortative mating.
- Hans Fredrik Sunde
- Nikolai Haahjem Eftedal
- Fartein Ask Torvik
Nature Communications (2024)
Participation bias in the UK Biobank distorts genetic associations and downstream analyses
- Tabea Schoeler
- Zoltán Kutalik
Nature Human Behaviour (2023)
Quick links
- Explore articles by subject
- Guide to authors
- Editorial policies
Sign up for the Nature Briefing newsletter — what matters in science, free to your inbox daily.


COMMENTS
The similarity thesis (that people are attracted to people like themselves) is based on which kinds of similarities between partners? ... Learning more about what is desired in a partner; Learning not to jump into a relationship too quickly; ALL THE ABOVE. Terminating a relationship can be a learning experience. Some of the positive things ...
The similarity thesis states that similarities form the basis of relationships. Complementarity is a factor, as differences strengthen a relationship when they are complementary so that each partner's characteristics satisfy the other's needs. Some relationships are based on the economic model, called Social Exchange Theory, that we seek out ...
It is also known as similarity thesis. The similarity thesis basically states that we are attracted to and tend to form relationships with others who are similar to us. 7 There are three reasons why similarity thesis works: validation, predictability, and affiliation. First, it is validating to know that someone likes the same things that we do.
The similarity hypothesis suggests that we tend to be drawn towards those who are similar to ourselves. Similarities can refer to shared attitudes and values, as well as political opinions, cultural background, or even minute details like posture. 1. The experience of interacting with similar individuals jumpstarts cognitive processing, like ...
In this research area, some studies explore the similarity between partners on a variety of relationship issues (Weigel, 2008) and the perception of this similarity. The findings on the first aspect are often inconsistent as, while some studies have found that spousal similarity is associated with marital satisfaction and stability ( Gaunt ...
For decades, social scientists have observed that people greatly desire a partner who is similar to themselves. Less is known, however, about whether particular similarity domains (e.g., music preferences) may uniquely influence relationship formation. We address this gap by examining people's preferences for 18 similarity domains in three types of relationships: friendships, casual/short ...
2004). Similar individuals are assumed to be attractive because they validate our beliefs about the world and ourselves and reduce the risk of conflicts (e.g., Morry and Gaines, 2005). Not surprisingly therefore, similarity between partners contributes to relationship satisfaction (e.g., Lutz-Zois, Bradley, Mihalik, and Moorman-Eavers, 2006).
Similarity helps separate kin from nonkin. With resemblances on par with distant cousins, friends qualify as near-kin, individuals whose similarities signal preference for the same phenotypically compatible environments (2). Yet friends differ from kin in one key respect: Friends are voluntary affiliates (3).
thus unable to fully disentangle the average similarities between partners from the similarities in how they change across time. In the current research, we test the nature and the extent of individual-difference convergence in 171 mixed-gender couples from four annual waves of the New Zealand Attitudes and Values Survey (NZAVS).
Research on trust development has generally focused on how similarities between people influence trust allocation. However, similarity in interests and beliefs, which underpins trust development and may be critical to relationship success, is seldom apparent upon initial interaction and thus may not be a primary predictor of initial trust decisions. Here we ask how mimicry, a visible social ...
The similarity thesis is based on which similarities between partners? race. all of these are correct. educational standing. economic class. likes the same things you like. Here's the best way to solve it. Share Share. The similarity thesis is a concept in social psych...
In the 2x2 MANOVA, there was a significant multivariate main effect of when-similarity, F(3,38) = 6.61, p < 0.001, η 2 = 0.34, such that participants who worked with ostensible partners who had similar timing of activities were much more attracted to their partners than those participants who had a partner with dissimilar timing.
Our expert help has broken down your problem into an easy-to-learn solution you can count on. Question: With regard to interpersonal attraction, the similarity thesis is based upon which similarities between partners? a. age and education b. race and ethnic background c. socioenomic status d. all of the above. With regard to interpersonal ...
Romantic partners are similar. People are more romantically attracted to others who share their beliefs (Berscheid, 1985; Montoya et al., 2008), and both committed and casual relationships are characterized by feelings of shared goals, power, and interests (Columbus et al., 2020).Similarity between the self and others is a signal of kinship (Park & Schaller, 2005) and trust (Ziegler & Golbeck ...
Although the similarity-attraction hypothesis (SAH) is one of the main theoretical foundations of management and industrial/organizational (I/O) psychology research, systematic reviews of the hypothesis have not been published. An overall review of the existing body of knowledge is therefore warranted as a means of identifying what is known about the hypothesis and also identifying what future ...
Mark Knapp's 10 Stage Model of Relationship. Initiating, experimenting, intensifying, integrating, bonding. Differentiating, circumscribing, stagnating, avoiding, terminating. Study with Quizlet and memorize flashcards containing terms like Why We Form Relationships, Similarity Thesis, Reasons for Similarity Thesis and more.
The similarity thesis suggests that people form relationships with others who share interests, values, personality traits, and social status, supporting the concept of homophily, which is the tendency to associate with similar individuals. Explanation: The similarity thesis is based on the premise that we are attracted to people who are similar ...
More specifically, according to Kerckhoff and Davis (1962) initial attraction between partners is based on social and cultural similarities, such as corresponding social status, education or religious background, which yield a field of eligibles. From this field of eligibles individuals choose each other on the basis of complementarity of needs.
Such similarities contribute to relationship stability, ease communication, and facilitate understanding amongst the partners. Also, they act as a platform for negotiation and compromise during conflicts. Explanation: The similarity thesis is based on the concept that partners in a relationship often share common attributes. These similarities ...
Partners are often similar in traits, such as their education and political views, but it is unclear what exactly causes this similarity. Using Mendelian randomization and data on 50,000 partner ...