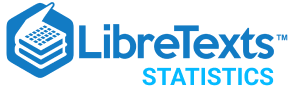
- school Campus Bookshelves
- menu_book Bookshelves
- perm_media Learning Objects
- login Login
- how_to_reg Request Instructor Account
- hub Instructor Commons

Margin Size
- Download Page (PDF)
- Download Full Book (PDF)
- Periodic Table
- Physics Constants
- Scientific Calculator
- Reference & Cite
- Tools expand_more
- Readability
selected template will load here
This action is not available.
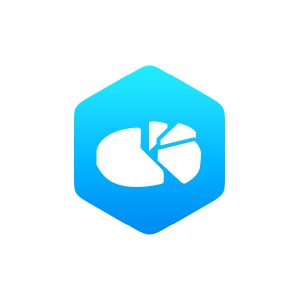
8.1: Samples, Populations and Sampling
- Last updated
- Save as PDF
- Page ID 36115
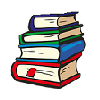
- Danielle Navarro
- University of New South Wales
\( \newcommand{\vecs}[1]{\overset { \scriptstyle \rightharpoonup} {\mathbf{#1}} } \)
\( \newcommand{\vecd}[1]{\overset{-\!-\!\rightharpoonup}{\vphantom{a}\smash {#1}}} \)
\( \newcommand{\id}{\mathrm{id}}\) \( \newcommand{\Span}{\mathrm{span}}\)
( \newcommand{\kernel}{\mathrm{null}\,}\) \( \newcommand{\range}{\mathrm{range}\,}\)
\( \newcommand{\RealPart}{\mathrm{Re}}\) \( \newcommand{\ImaginaryPart}{\mathrm{Im}}\)
\( \newcommand{\Argument}{\mathrm{Arg}}\) \( \newcommand{\norm}[1]{\| #1 \|}\)
\( \newcommand{\inner}[2]{\langle #1, #2 \rangle}\)
\( \newcommand{\Span}{\mathrm{span}}\)
\( \newcommand{\id}{\mathrm{id}}\)
\( \newcommand{\kernel}{\mathrm{null}\,}\)
\( \newcommand{\range}{\mathrm{range}\,}\)
\( \newcommand{\RealPart}{\mathrm{Re}}\)
\( \newcommand{\ImaginaryPart}{\mathrm{Im}}\)
\( \newcommand{\Argument}{\mathrm{Arg}}\)
\( \newcommand{\norm}[1]{\| #1 \|}\)
\( \newcommand{\Span}{\mathrm{span}}\) \( \newcommand{\AA}{\unicode[.8,0]{x212B}}\)
\( \newcommand{\vectorA}[1]{\vec{#1}} % arrow\)
\( \newcommand{\vectorAt}[1]{\vec{\text{#1}}} % arrow\)
\( \newcommand{\vectorB}[1]{\overset { \scriptstyle \rightharpoonup} {\mathbf{#1}} } \)
\( \newcommand{\vectorC}[1]{\textbf{#1}} \)
\( \newcommand{\vectorD}[1]{\overrightarrow{#1}} \)
\( \newcommand{\vectorDt}[1]{\overrightarrow{\text{#1}}} \)
\( \newcommand{\vectE}[1]{\overset{-\!-\!\rightharpoonup}{\vphantom{a}\smash{\mathbf {#1}}}} \)
In the prelude to Part I discussed the riddle of induction, and highlighted the fact that all learning requires you to make assumptions. Accepting that this is true, our first task to come up with some fairly general assumptions about data that make sense. This is where sampling theory comes in. If probability theory is the foundations upon which all statistical theory builds, sampling theory is the frame around which you can build the rest of the house. Sampling theory plays a huge role in specifying the assumptions upon which your statistical inferences rely. And in order to talk about “making inferences” the way statisticians think about it, we need to be a bit more explicit about what it is that we’re drawing inferences from (the sample) and what it is that we’re drawing inferences about (the population).
In almost every situation of interest, what we have available to us as researchers is a sample of data. We might have run experiment with some number of participants; a polling company might have phoned some number of people to ask questions about voting intentions; etc. Regardless: the data set available to us is finite, and incomplete. We can’t possibly get every person in the world to do our experiment; a polling company doesn’t have the time or the money to ring up every voter in the country etc. In our earlier discussion of descriptive statistics (Chapter 5, this sample was the only thing we were interested in. Our only goal was to find ways of describing, summarising and graphing that sample. This is about to change.
Defining a population
A sample is a concrete thing. You can open up a data file, and there’s the data from your sample. A population , on the other hand, is a more abstract idea. It refers to the set of all possible people, or all possible observations, that you want to draw conclusions about, and is generally much bigger than the sample. In an ideal world, the researcher would begin the study with a clear idea of what the population of interest is, since the process of designing a study and testing hypotheses about the data that it produces does depend on the population about which you want to make statements. However, that doesn’t always happen in practice: usually the researcher has a fairly vague idea of what the population is and designs the study as best he/she can on that basis.
Sometimes it’s easy to state the population of interest. For instance, in the “polling company” example that opened the chapter, the population consisted of all voters enrolled at the a time of the study – millions of people. The sample was a set of 1000 people who all belong to that population. In most situations the situation is much less simple. In a typical a psychological experiment, determining the population of interest is a bit more complicated. Suppose I run an experiment using 100 undergraduate students as my participants. My goal, as a cognitive scientist, is to try to learn something about how the mind works. So, which of the following would count as “the population”:
- All of the undergraduate psychology students at the University of Adelaide?
- Undergraduate psychology students in general, anywhere in the world?
- Australians currently living?
- Australians of similar ages to my sample?
- Anyone currently alive?
- Any human being, past, present or future?
- Any biological organism with a sufficient degree of intelligence operating in a terrestrial environment?
- Any intelligent being?
Each of these defines a real group of mind-possessing entities, all of which might be of interest to me as a cognitive scientist, and it’s not at all clear which one ought to be the true population of interest. As another example, consider the Wellesley-Croker game that we discussed in the prelude. The sample here is a specific sequence of 12 wins and 0 losses for Wellesley. What is the population?
- All outcomes until Wellesley and Croker arrived at their destination?
- All outcomes if Wellesley and Croker had played the game for the rest of their lives?
- All outcomes if Wellseley and Croker lived forever and played the game until the world ran out of hills?
- All outcomes if we created an infinite set of parallel universes and the Wellesely/Croker pair made guesses about the same 12 hills in each universe?
Again, it’s not obvious what the population is.
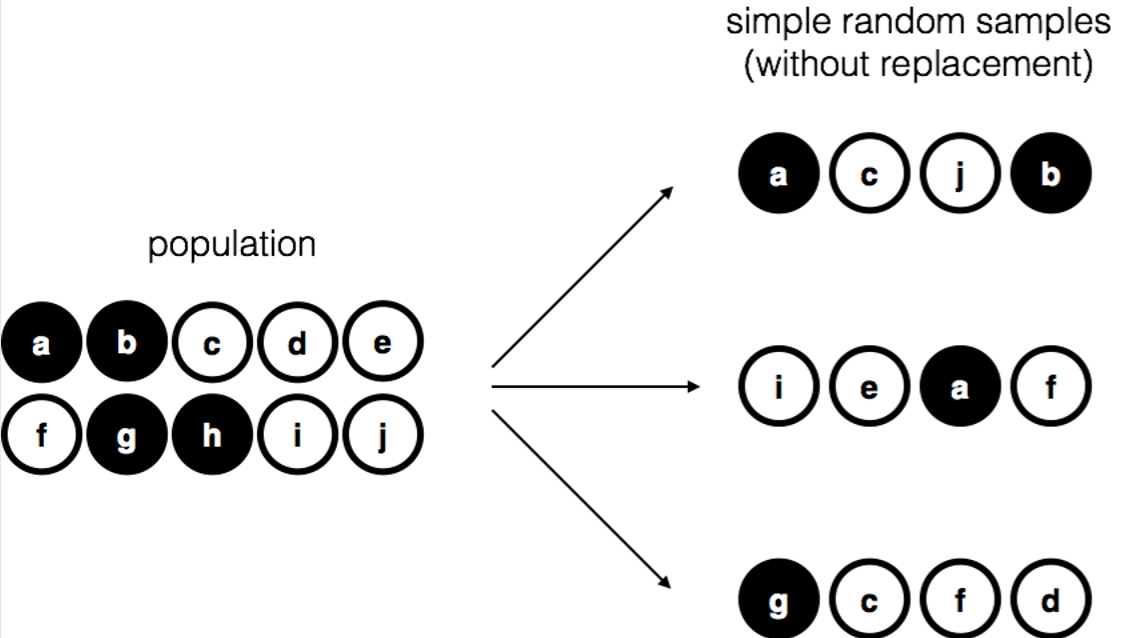
Irrespective of how I define the population, the critical point is that the sample is a subset of the population, and our goal is to use our knowledge of the sample to draw inferences about the properties of the population. The relationship between the two depends on the procedure by which the sample was selected. This procedure is referred to as a sampling method , and it is important to understand why it matters.
To keep things simple, let’s imagine that we have a bag containing 10 chips. Each chip has a unique letter printed on it, so we can distinguish between the 10 chips. The chips come in two colours, black and white. This set of chips is the population of interest, and it is depicted graphically on the left of Figure 10.1. As you can see from looking at the picture, there are 4 black chips and 6 white chips, but of course in real life we wouldn’t know that unless we looked in the bag. Now imagine you run the following “experiment”: you shake up the bag, close your eyes, and pull out 4 chips without putting any of them back into the bag. First out comes the a chip (black), then the c chip (white), then j (white) and then finally b (black). If you wanted, you could then put all the chips back in the bag and repeat the experiment, as depicted on the right hand side of Figure 10.1. Each time you get different results, but the procedure is identical in each case. The fact that the same procedure can lead to different results each time, we refer to it as a random process. 147 However, because we shook the bag before pulling any chips out, it seems reasonable to think that every chip has the same chance of being selected. A procedure in which every member of the population has the same chance of being selected is called a simple random sample . The fact that we did not put the chips back in the bag after pulling them out means that you can’t observe the same thing twice, and in such cases the observations are said to have been sampled without replacement .
To help make sure you understand the importance of the sampling procedure, consider an alternative way in which the experiment could have been run. Suppose that my 5-year old son had opened the bag, and decided to pull out four black chips without putting any of them back in the bag. This biased sampling scheme is depicted in Figure 10.2. Now consider the evidentiary value of seeing 4 black chips and 0 white chips. Clearly, it depends on the sampling scheme, does it not? If you know that the sampling scheme is biased to select only black chips, then a sample that consists of only black chips doesn’t tell you very much about the population! For this reason, statisticians really like it when a data set can be considered a simple random sample, because it makes the data analysis much easier.
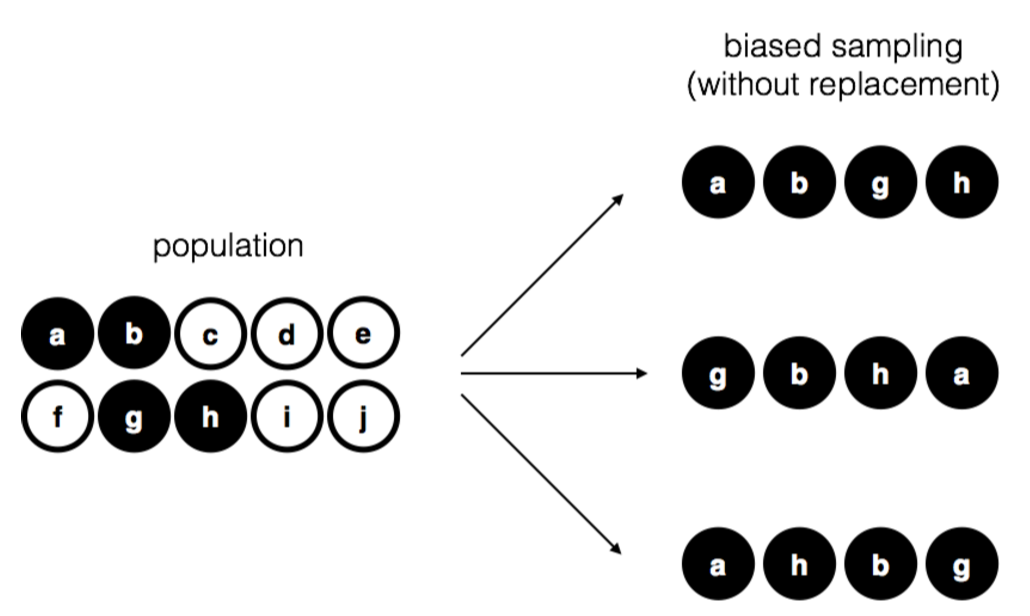
A third procedure is worth mentioning. This time around we close our eyes, shake the bag, and pull out a chip. This time, however, we record the observation and then put the chip back in the bag. Again we close our eyes, shake the bag, and pull out a chip. We then repeat this procedure until we have 4 chips. Data sets generated in this way are still simple random samples, but because we put the chips back in the bag immediately after drawing them it is referred to as a sample with replacement . The difference between this situation and the first one is that it is possible to observe the same population member multiple times, as illustrated in Figure 10.3.
In my experience, most psychology experiments tend to be sampling without replacement, because the same person is not allowed to participate in the experiment twice. However, most statistical theory is based on the assumption that the data arise from a simple random sample with replacement. In real life, this very rarely matters. If the population of interest is large (e.g., has more than 10 entities!) the difference between sampling with- and without- replacement is too small to be concerned with. The difference between simple random samples and biased samples, on the other hand, is not such an easy thing to dismiss.
Most samples are not simple random samples
As you can see from looking at the list of possible populations that I showed above, it is almost impossible to obtain a simple random sample from most populations of interest. When I run experiments, I’d consider it a minor miracle if my participants turned out to be a random sampling of the undergraduate psychology students at Adelaide university, even though this is by far the narrowest population that I might want to generalise to. A thorough discussion of other types of sampling schemes is beyond the scope of this book, but to give you a sense of what’s out there I’ll list a few of the more important ones:
- Stratified sampling . Suppose your population is (or can be) divided into several different subpopulations, or strata . Perhaps you’re running a study at several different sites, for example. Instead of trying to sample randomly from the population as a whole, you instead try to collect a separate random sample from each of the strata. Stratified sampling is sometimes easier to do than simple random sampling, especially when the population is already divided into the distinct strata. It can also be more efficient that simple random sampling, especially when some of the subpopulations are rare. For instance, when studying schizophrenia it would be much better to divide the population into two 148 strata (schizophrenic and not-schizophrenic), and then sample an equal number of people from each group. If you selected people randomly, you would get so few schizophrenic people in the sample that your study would be useless. This specific kind of of stratified sampling is referred to as oversampling because it makes a deliberate attempt to over-represent rare groups.
- Snowball sampling is a technique that is especially useful when sampling from a “hidden” or hard to access population, and is especially common in social sciences. For instance, suppose the researchers want to conduct an opinion poll among transgender people. The research team might only have contact details for a few trans folks, so the survey starts by asking them to participate (stage 1). At the end of the survey, the participants are asked to provide contact details for other people who might want to participate. In stage 2, those new contacts are surveyed. The process continues until the researchers have sufficient data. The big advantage to snowball sampling is that it gets you data in situations that might otherwise be impossible to get any. On the statistical side, the main disadvantage is that the sample is highly non-random, and non-random in ways that are difficult to address. On the real life side, the disadvantage is that the procedure can be unethical if not handled well, because hidden populations are often hidden for a reason. I chose transgender people as an example here to highlight this: if you weren’t careful you might end up outing people who don’t want to be outed (very, very bad form), and even if you don’t make that mistake it can still be intrusive to use people’s social networks to study them. It’s certainly very hard to get people’s informed consent before contacting them, yet in many cases the simple act of contacting them and saying “hey we want to study you” can be hurtful. Social networks are complex things, and just because you can use them to get data doesn’t always mean you should.
- Convenience sampling is more or less what it sounds like. The samples are chosen in a way that is convenient to the researcher, and not selected at random from the population of interest. Snowball sampling is one type of convenience sampling, but there are many others. A common example in psychology are studies that rely on undergraduate psychology students. These samples are generally non-random in two respects: firstly, reliance on undergraduate psychology students automatically means that your data are restricted to a single subpopulation. Secondly, the students usually get to pick which studies they participate in, so the sample is a self selected subset of psychology students not a randomly selected subset. In real life, most studies are convenience samples of one form or another. This is sometimes a severe limitation, but not always.
much does it matter if you don’t have a simple random sample?
Okay, so real world data collection tends not to involve nice simple random samples. Does that matter? A little thought should make it clear to you that it can matter if your data are not a simple random sample: just think about the difference between Figures 10.1 and 10.2. However, it’s not quite as bad as it sounds. Some types of biased samples are entirely unproblematic. For instance, when using a stratified sampling technique you actually know what the bias is because you created it deliberately, often to increase the effectiveness of your study, and there are statistical techniques that you can use to adjust for the biases you’ve introduced (not covered in this book!). So in those situations it’s not a problem.
More generally though, it’s important to remember that random sampling is a means to an end, not the end in itself. Let’s assume you’ve relied on a convenience sample, and as such you can assume it’s biased. A bias in your sampling method is only a problem if it causes you to draw the wrong conclusions. When viewed from that perspective, I’d argue that we don’t need the sample to be randomly generated in every respect: we only need it to be random with respect to the psychologically-relevant phenomenon of interest. Suppose I’m doing a study looking at working memory capacity. In study 1, I actually have the ability to sample randomly from all human beings currently alive, with one exception: I can only sample people born on a Monday. In study 2, I am able to sample randomly from the Australian population. I want to generalise my results to the population of all living humans. Which study is better? The answer, obviously, is study 1. Why? Because we have no reason to think that being “born on a Monday” has any interesting relationship to working memory capacity. In contrast, I can think of several reasons why “being Australian” might matter. Australia is a wealthy, industrialised country with a very well-developed education system. People growing up in that system will have had life experiences much more similar to the experiences of the people who designed the tests for working memory capacity. This shared experience might easily translate into similar beliefs about how to “take a test”, a shared assumption about how psychological experimentation works, and so on. These things might actually matter. For instance, “test taking” style might have taught the Australian participants how to direct their attention exclusively on fairly abstract test materials relative to people that haven’t grown up in a similar environment; leading to a misleading picture of what working memory capacity is.
There are two points hidden in this discussion. Firstly, when designing your own studies, it’s important to think about what population you care about, and try hard to sample in a way that is appropriate to that population. In practice, you’re usually forced to put up with a “sample of convenience” (e.g., psychology lecturers sample psychology students because that’s the least expensive way to collect data, and our coffers aren’t exactly overflowing with gold), but if so you should at least spend some time thinking about what the dangers of this practice might be.
Secondly, if you’re going to criticise someone else’s study because they’ve used a sample of convenience rather than laboriously sampling randomly from the entire human population, at least have the courtesy to offer a specific theory as to how this might have distorted the results. Remember, everyone in science is aware of this issue, and does what they can to alleviate it. Merely pointing out that “the study only included people from group BLAH” is entirely unhelpful, and borders on being insulting to the researchers, who are of course aware of the issue. They just don’t happen to be in possession of the infinite supply of time and money required to construct the perfect sample. In short, if you want to offer a responsible critique of the sampling process, then be helpful . Rehashing the blindingly obvious truisms that I’ve been rambling on about in this section isn’t helpful.
Population parameters and sample statistics
Okay. Setting aside the thorny methodological issues associated with obtaining a random sample and my rather unfortunate tendency to rant about lazy methodological criticism, let’s consider a slightly different issue. Up to this point we have been talking about populations the way a scientist might. To a psychologist, a population might be a group of people. To an ecologist, a population might be a group of bears. In most cases the populations that scientists care about are concrete things that actually exist in the real world. Statisticians, however, are a funny lot. On the one hand, they are interested in real world data and real science in the same way that scientists are. On the other hand, they also operate in the realm of pure abstraction in the way that mathematicians do. As a consequence, statistical theory tends to be a bit abstract in how a population is defined. In much the same way that psychological researchers operationalise our abstract theoretical ideas in terms of concrete measurements (Section 2.1, statisticians operationalise the concept of a “population” in terms of mathematical objects that they know how to work with. You’ve already come across these objects in Chapter 9: they’re called probability distributions.
The idea is quite simple. Let’s say we’re talking about IQ scores. To a psychologist, the population of interest is a group of actual humans who have IQ scores. A statistician “simplifies” this by operationally defining the population as the probability distribution depicted in Figure ?? . IQ tests are designed so that the average IQ is 100, the standard deviation of IQ scores is 15, and the distribution of IQ scores is normal. These values are referred to as the population parameters because they are characteristics of the entire population. That is, we say that the population mean μ is 100, and the population standard deviation σ is 15.

Now suppose I run an experiment. I select 100 people at random and administer an IQ test, giving me a simple random sample from the population. My sample would consist of a collection of numbers like this:
Each of these IQ scores is sampled from a normal distribution with mean 100 and standard deviation 15. So if I plot a histogram of the sample, I get something like the one shown in Figure 10.4b. As you can see, the histogram is roughly the right shape, but it’s a very crude approximation to the true population distribution shown in Figure 10.4a. When I calculate the mean of my sample, I get a number that is fairly close to the population mean 100 but not identical. In this case, it turns out that the people in my sample have a mean IQ of 98.5, and the standard deviation of their IQ scores is 15.9. These sample statistics are properties of my data set, and although they are fairly similar to the true population values, they are not the same. In general, sample statistics are the things you can calculate from your data set, and the population parameters are the things you want to learn about. Later on in this chapter I’ll talk about how you can estimate population parameters using your sample statistics (Section 10.4 and how to work out how confident you are in your estimates (Section 10.5 but before we get to that there’s a few more ideas in sampling theory that you need to know about.
Introduction to Research Methods
7 samples and populations.
So you’ve developed your research question, figured out how you’re going to measure whatever you want to study, and have your survey or interviews ready to go. Now all your need is other people to become your data.
You might say ‘easy!’, there’s people all around you. You have a big family tree and surely them and their friends would have happy to take your survey. And then there’s your friends and people you’re in class with. Finding people is way easier than writing the interview questions or developing the survey. That reaction might be a strawman, maybe you’ve come to the conclusion none of this is easy. For your data to be valuable, you not only have to ask the right questions, you have to ask the right people. The “right people” aren’t the best or the smartest people, the right people are driven by what your study is trying to answer and the method you’re using to answer it.
Remember way back in chapter 2 when we looked at this chart and discussed the differences between qualitative and quantitative data.
One of the biggest differences between quantitative and qualitative data was whether we wanted to be able to explain something for a lot of people (what percentage of residents in Oklahoma support legalizing marijuana?) versus explaining the reasons for those opinions (why do some people support legalizing marijuana and others not?). The underlying differences there is whether our goal is explain something about everyone, or whether we’re content to explain it about just our respondents.
‘Everyone’ is called the population . The population in research is whatever group the research is trying to answer questions about. The population could be everyone on planet Earth, everyone in the United States, everyone in rural counties of Iowa, everyone at your university, and on and on. It is simply everyone within the unit you are intending to study.
In order to study the population, we typically take a sample or a subset. A sample is simply a smaller number of people from the population that are studied, which we can use to then understand the characteristics of the population based on that subset. That’s why a poll of 1300 likely voters can be used to guess at who will win your states Governor race. It isn’t perfect, and we’ll talk about the math behind all of it in a later chapter, but for now we’ll just focus on the different types of samples you might use to study a population with a survey.
If correctly sampled, we can use the sample to generalize information we get to the population. Generalizability , which we defined earlier, means we can assume the responses of people to our study match the responses everyone would have given us. We can only do that if the sample is representative of the population, meaning that they are alike on important characteristics such as race, gender, age, education. If something makes a large difference in people’s views on a topic in your research and your sample is not balanced, you’ll get inaccurate results.
Generalizability is more of a concern with surveys than with interviews. The goal of a survey is to explain something about people beyond the sample you get responses from. You’ll never see a news headline saying that “53% of 1250 Americans that responded to a poll approve of the President”. It’s only worth asking those 1250 people if we can assume the rest of the United States feels the same way overall. With interviews though we’re looking for depth from their responses, and so we are less hopefully that the 15 people we talk to will exactly match the American population. That doesn’t mean the data we collect from interviews doesn’t have value, it just has different uses.
There are two broad types of samples, with several different techniques clustered below those. Probability sampling is associated with surveys, and non-probability sampling is often used when conducting interviews. We’ll first describe probability samples, before discussing the non-probability options.
The type of sampling you’ll use will be based on the type of research you’re intending to do. There’s no sample that’s right or wrong, they can just be more or less appropriate for the question you’re trying to answer. And if you use a less appropriate sampling strategy, the answer you get through your research is less likely to be accurate.
7.1 Types of Probability Samples
So we just hinted at the idea that depending on the sample you use, you can generalize the data you collect from the sample to the population. That will depend though on whether your sample represents the population. To ensure that your sample is representative of the population, you will want to use a probability sample. A representative sample refers to whether the characteristics (race, age, income, education, etc) of the sample are the same as the population. Probability sampling is a sampling technique in which every individual in the population has an equal chance of being selected as a subject for the research.
There are several different types of probability samples you can use, depending on the resources you have available.
Let’s start with a simple random sample . In order to use a simple random sample all you have to do is take everyone in your population, throw them in a hat (not literally, you can just throw their names in a hat), and choose the number of names you want to use for your sample. By drawing blindly, you can eliminate human bias in constructing the sample and your sample should represent the population from which it is being taken.
However, a simple random sample isn’t quite that easy to build. The biggest issue is that you have to know who everyone is in order to randomly select them. What that requires is a sampling frame , a list of all residents in the population. But we don’t always have that. There is no list of residents of New York City (or any other city). Organizations that do have such a list wont just give it away. Try to ask your university for a list and contact information of everyone at your school so you can do a survey? They wont give it to you, for privacy reasons. It’s actually harder to think of popultions you could easily develop a sample frame for than those you can’t. If you can get or build a sampling frame, the work of a simple random sample is fairly simple, but that’s the biggest challenge.
Most of the time a true sampling frame is impossible to acquire, so researcher have to settle for something approximating a complete list. Earlier generations of researchers could use the random dial method to contact a random sample of Americans, because every household had a single phone. To use it you just pick up the phone and dial random numbers. Assuming the numbers are actually random, anyone might be called. That method actually worked somewhat well, until people stopped having home phone numbers and eventually stopped answering the phone. It’s a fun mental exercise to think about how you would go about creating a sampling frame for different groups though; think through where you would look to find a list of everyone in these groups:
Plumbers Recent first-time fathers Members of gyms
The best way to get an actual sampling frame is likely to purchase one from a private company that buys data on people from all the different websites we use.
Let’s say you do have a sampling frame though. For instance, you might be hired to do a survey of members of the Republican Party in the state of Utah to understand their political priorities this year, and the organization could give you a list of their members because they’ve hired you to do the reserach. One method of constructing a simple random sample would be to assign each name on the list a number, and then produce a list of random numbers. Once you’ve matched the random numbers to the list, you’ve got your sample. See the example using the list of 20 names below

and the list of 5 random numbers.

Systematic sampling is similar to simple random sampling in that it begins with a list of the population, but instead of choosing random numbers one would select every kth name on the list. What the heck is a kth? K just refers to how far apart the names are on the list you’re selecting. So if you want to sample one-tenth of the population, you’d select every tenth name. In order to know the k for your study you need to know your sample size (say 1000) and the size of the population (75000). You can divide the size of the population by the sample (75000/1000), which will produce your k (750). As long as the list does not contain any hidden order, this sampling method is as good as the random sampling method, but its only advantage over the random sampling technique is simplicity. If we used the same list as above and wanted to survey 1/5th of the population, we’d include 4 of the names on the list. It’s important with systematic samples to randomize the starting point in the list, otherwise people with A names will be oversampled. If we started with the 3rd name, we’d select Annabelle Frye, Cristobal Padilla, Jennie Vang, and Virginia Guzman, as shown below. So in order to use a systematic sample, we need three things, the population size (denoted as N ), the sample size we want ( n ) and k , which we calculate by dividing the population by the sample).
N= 20 (Population Size) n= 4 (Sample Size) k= 5 {20/4 (kth element) selection interval}

We can also use a stratified sample , but that requires knowing more about the population than just their names. A stratified sample divides the study population into relevant subgroups, and then draws a sample from each subgroup. Stratified sampling can be used if you’re very concerned about ensuring balance in the sample or there may be a problem of underrepresentation among certain groups when responses are received. Not everyone in your sample is equally likely to answer a survey. Say for instance we’re trying to predict who will win an election in a county with three cities. In city A there are 1 million college students, in city B there are 2 million families, and in City C there are 3 million retirees. You know that retirees are more likely than busy college students or parents to respond to a poll. So you break the sample into three parts, ensuring that you get 100 responses from City A, 200 from City B, and 300 from City C, so the three cities would match the population. A stratified sample provides the researcher control over the subgroups that are included in the sample, whereas simple random sampling does not guarantee that any one type of person will be included in the final sample. A disadvantage is that it is more complex to organize and analyze the results compared to simple random sampling.
Cluster sampling is an approach that begins by sampling groups (or clusters) of population elements and then selects elements from within those groups. A researcher would use cluster sampling if getting access to elements in an entrie population is too challenging. For instance, a study on students in schools would probably benefit from randomly selecting from all students at the 36 elementary schools in a fictional city. But getting contact information for all students would be very difficult. So the researcher might work with principals at several schools and survey those students. The researcher would need to ensure that the students surveyed at the schools are similar to students throughout the entire city, and greater access and participation within each cluster may make that possible.
The image below shows how this can work, although the example is oversimplified. Say we have 12 students that are in 6 classrooms. The school is in total 1/4th green (3/12), 1/4th yellow (3/12), and half blue (6/12). By selecting the right clusters from within the school our sample can be representative of the entire school, assuming these colors are the only significant difference between the students. In the real world, you’d want to match the clusters and population based on race, gender, age, income, etc. And I should point out that this is an overly simplified example. What if 5/12s of the school was yellow and 1/12th was green, how would I get the right proportions? I couldn’t, but you’d do the best you could. You still wouldn’t want 4 yellows in the sample, you’d just try to approximiate the population characteristics as best you can.

7.2 Actually Doing a Survey
All of that probably sounds pretty complicated. Identifying your population shouldn’t be too difficult, but how would you ever get a sampling frame? And then actually identifying who to include… It’s probably a bit overwhelming and makes doing a good survey sound impossible.
Researchers using surveys aren’t superhuman though. Often times, they use a little help. Because surveys are really valuable, and because researchers rely on them pretty often, there has been substantial growth in companies that can help to get one’s survey to its intended audience.
One popular resource is Amazon’s Mechanical Turk (more commonly known as MTurk). MTurk is at its most basic a website where workers look for jobs (called hits) to be listed by employers, and choose whether to do the task or not for a set reward. MTurk has grown over the last decade to be a common source of survey participants in the social sciences, in part because hiring workers costs very little (you can get some surveys completed for penny’s). That means you can get your survey completed with a small grant ($1-2k at the low end) and get the data back in a few hours. Really, it’s a quick and easy way to run a survey.
However, the workers aren’t perfectly representative of the average American. For instance, researchers have found that MTurk respondents are younger, better educated, and earn less than the average American.
One way to get around that issue, which can be used with MTurk or any survey, is to weight the responses. Because with MTurk you’ll get fewer responses from older, less educated, and richer Americans, those responses you do give you want to count for more to make your sample more representative of the population. Oversimplified example incoming!
Imagine you’re setting up a pizza party for your class. There are 9 people in your class, 4 men and 5 women. You only got 4 responses from the men, and 3 from the women. All 4 men wanted peperoni pizza, while the 3 women want a combination. Pepperoni wins right, 4 to 3? Not if you assume that the people that didn’t respond are the same as the ones that did. If you weight the responses to match the population (the full class of 9), a combination pizza is the winner.

Because you know the population of women is 5, you can weight the 3 responses from women by 5/3 = 1.6667. If we weight (or multiply) each vote we did receive from a woman by 1.6667, each vote for a combination now equals 1.6667, meaning that the 3 votes for combination total 5. Because we received a vote from every man in the class, we just weight their votes by 1. The big assumption we have to make is that the people we didn’t hear from (the 2 women that didn’t vote) are similar to the ones we did hear from. And if we don’t get any responses from a group we don’t have anything to infer their preferences or views from.
Let’s go through a slightly more complex example, still just considering one quality about people in the class. Let’s say your class actually has 100 students, but you only received votes from 50. And, what type of pizza people voted for is mixed, but men still prefer peperoni overall, and women still prefer combination. The class is 60% female and 40% male.
We received 21 votes from women out of the 60, so we can weight their responses by 60/21 to represent the population. We got 29 votes out of the 40 for men, so their responses can be weighted by 40/29. See the math below.

53.8 votes for combination? That might seem a little odd, but weighting isn’t a perfect science. We can’t identify what a non-respondent would have said exactly, all we can do is use the responses of other similar people to make a good guess. That issue often comes up in polling, where pollsters have to guess who is going to vote in a given election in order to project who will win. And we can weight on any characteristic of a person we think will be important, alone or in combination. Modern polls weight on age, gender, voting habits, education, and more to make the results as generalizable as possible.
There’s an appendix later in this book where I walk through the actual steps of creating weights for a sample in R, if anyone actually does a survey. I intended this section to show that doing a good survey might be simpler than it seemed, but now it might sound even more difficult. A good lesson to take though is that there’s always another door to go through, another hurdle to improve your methods. Being good at research just means being constantly prepared to be given a new challenge, and being able to find another solution.
7.3 Non-Probability Sampling
Qualitative researchers’ main objective is to gain an in-depth understanding on the subject matter they are studying, rather than attempting to generalize results to the population. As such, non-probability sampling is more common because of the researchers desire to gain information not from random elements of the population, but rather from specific individuals.
Random selection is not used in nonprobability sampling. Instead, the personal judgment of the researcher determines who will be included in the sample. Typically, researchers may base their selection on availability, quotas, or other criteria. However, not all members of the population are given an equal chance to be included in the sample. This nonrandom approach results in not knowing whether the sample represents the entire population. Consequently, researchers are not able to make valid generalizations about the population.
As with probability sampling, there are several types of non-probability samples. Convenience sampling , also known as accidental or opportunity sampling, is a process of choosing a sample that is easily accessible and readily available to the researcher. Researchers tend to collect samples from convenient locations such as their place of employment, a location, school, or other close affiliation. Although this technique allows for quick and easy access to available participants, a large part of the population is excluded from the sample.
For example, researchers (particularly in psychology) often rely on research subjects that are at their universities. That is highly convenient, students are cheap to hire and readily available on campuses. However, it means the results of the study may have limited ability to predict motivations or behaviors of people that aren’t included in the sample, i.e., people outside the age of 18-22 that are going to college.
If I ask you to get find out whether people approve of the mayor or not, and tell you I want 500 people’s opinions, should you go stand in front of the local grocery store? That would be convinient, and the people coming will be random, right? Not really. If you stand outside a rural Piggly Wiggly or an urban Whole Foods, do you think you’ll see the same people? Probably not, people’s chracteristics make the more or less likely to be in those locations. This technique runs the high risk of over- or under-representation, biased results, as well as an inability to make generalizations about the larger population. As the name implies though, it is convenient.
Purposive sampling , also known as judgmental or selective sampling, refers to a method in which the researcher decides who will be selected for the sample based on who or what is relevant to the study’s purpose. The researcher must first identify a specific characteristic of the population that can best help answer the research question. Then, they can deliberately select a sample that meets that particular criterion. Typically, the sample is small with very specific experiences and perspectives. For instance, if I wanted to understand the experiences of prominent foreign-born politicians in the United States, I would purposefully build a sample of… prominent foreign-born politicians in the United States. That would exclude anyone that was born in the United States or and that wasn’t a politician, and I’d have to define what I meant by prominent. Purposive sampling is susceptible to errors in judgment by the researcher and selection bias due to a lack of random sampling, but when attempting to research small communities it can be effective.
When dealing with small and difficult to reach communities researchers sometimes use snowball samples , also known as chain referral sampling. Snowball sampling is a process in which the researcher selects an initial participant for the sample, then asks that participant to recruit or refer additional participants who have similar traits as them. The cycle continues until the needed sample size is obtained.
This technique is used when the study calls for participants who are hard to find because of a unique or rare quality or when a participant does not want to be found because they are part of a stigmatized group or behavior. Examples may include people with rare diseases, sex workers, or a child sex offenders. It would be impossible to find an accurate list of sex workers anywhere, and surveying the general population about whether that is their job will produce false responses as people will be unwilling to identify themselves. As such, a common method is to gain the trust of one individual within the community, who can then introduce you to others. It is important that the researcher builds rapport and gains trust so that participants can be comfortable contributing to the study, but that must also be balanced by mainting objectivity in the research.
Snowball sampling is a useful method for locating hard to reach populations but cannot guarantee a representative sample because each contact will be based upon your last. For instance, let’s say you’re studying illegal fight clubs in your state. Some fight clubs allow weapons in the fights, while others completely ban them; those two types of clubs never interreact because of their disagreement about whether weapons should be allowed, and there’s no overlap between them (no members in both type of club). If your initial contact is with a club that uses weapons, all of your subsequent contacts will be within that community and so you’ll never understand the differences. If you didn’t know there were two types of clubs when you started, you’ll never even know you’re only researching half of the community. As such, snowball sampling can be a necessary technique when there are no other options, but it does have limitations.
Quota Sampling is a process in which the researcher must first divide a population into mutually exclusive subgroups, similar to stratified sampling. Depending on what is relevant to the study, subgroups can be based on a known characteristic such as age, race, gender, etc. Secondly, the researcher must select a sample from each subgroup to fit their predefined quotas. Quota sampling is used for the same reason as stratified sampling, to ensure that your sample has representation of certain groups. For instance, let’s say that you’re studying sexual harassment in the workplace, and men are much more willing to discuss their experiences than women. You might choose to decide that half of your final sample will be women, and stop requesting interviews with men once you fill your quota. The core difference is that while stratified sampling chooses randomly from within the different groups, quota sampling does not. A quota sample can either be proportional or non-proportional . Proportional quota sampling refers to ensuring that the quotas in the sample match the population (if 35% of the company is female, 35% of the sample should be female). Non-proportional sampling allows you to select your own quota sizes. If you think the experiences of females with sexual harassment are more important to your research, you can include whatever percentage of females you desire.
7.4 Dangers in sampling
Now that we’ve described all the different ways that one could create a sample, we can talk more about the pitfalls of sampling. Ensuring a quality sample means asking yourself some basic questions:
- Who is in the sample?
- How were they sampled?
- Why were they sampled?
A meal is often only as good as the ingredients you use, and your data will only be as good as the sample. If you collect data from the wrong people, you’ll get the wrong answer. You’ll still get an answer, it’ll just be inaccurate. And I want to reemphasize here wrong people just refers to inappropriate for your study. If I want to study bullying in middle schools, but I only talk to people that live in a retirement home, how accurate or relevant will the information I gather be? Sure, they might have grandchildren in middle school, and they may remember their experiences. But wouldn’t my information be more relevant if I talked to students in middle school, or perhaps a mix of teachers, parents, and students? I’ll get an answer from retirees, but it wont be the one I need. The sample has to be appropriate to the research question.
Is a bigger sample always better? Not necessarily. A larger sample can be useful, but a more representative one of the population is better. That was made painfully clear when the magazine Literary Digest ran a poll to predict who would win the 1936 presidential election between Alf Landon and incumbent Franklin Roosevelt. Literary Digest had run the poll since 1916, and had been correct in predicting the outcome every time. It was the largest poll ever, and they received responses for 2.27 million people. They essentially received responses from 1 percent of the American population, while many modern polls use only 1000 responses for a much more populous country. What did they predict? They showed that Alf Landon would be the overwhelming winner, yet when the election was held Roosevelt won every state except Maine and Vermont. It was one of the most decisive victories in Presidential history.
So what went wrong for the Literary Digest? Their poll was large (gigantic!), but it wasn’t representative of likely voters. They polled their own readership, which tended to be more educated and wealthy on average, along with people on a list of those with registered automobiles and telephone users (both of which tended to be owned by the wealthy at that time). Thus, the poll largely ignored the majority of Americans, who ended up voting for Roosevelt. The Literary Digest poll is famous for being wrong, but led to significant improvements in the science of polling to avoid similar mistakes in the future. Researchers have learned a lot in the century since that mistake, even if polling and surveys still aren’t (and can’t be) perfect.
What kind of sampling strategy did Literary Digest use? Convenience, they relied on lists they had available, rather than try to ensure every American was included on their list. A representative poll of 2 million people will give you more accurate results than a representative poll of 2 thousand, but I’ll take the smaller more representative poll than a larger one that uses convenience sampling any day.
7.5 Summary
Picking the right type of sample is critical to getting an accurate answer to your reserach question. There are a lot of differnet options in how you can select the people to participate in your research, but typically only one that is both correct and possible depending on the research you’re doing. In the next chapter we’ll talk about a few other methods for conducting reseach, some that don’t include any sampling by you.
- USC Libraries
- Research Guides
Organizing Your Social Sciences Research Paper
- 9. The Conclusion
- Purpose of Guide
- Design Flaws to Avoid
- Independent and Dependent Variables
- Glossary of Research Terms
- Reading Research Effectively
- Narrowing a Topic Idea
- Broadening a Topic Idea
- Extending the Timeliness of a Topic Idea
- Academic Writing Style
- Applying Critical Thinking
- Choosing a Title
- Making an Outline
- Paragraph Development
- Research Process Video Series
- Executive Summary
- The C.A.R.S. Model
- Background Information
- The Research Problem/Question
- Theoretical Framework
- Citation Tracking
- Content Alert Services
- Evaluating Sources
- Primary Sources
- Secondary Sources
- Tiertiary Sources
- Scholarly vs. Popular Publications
- Qualitative Methods
- Quantitative Methods
- Insiderness
- Using Non-Textual Elements
- Limitations of the Study
- Common Grammar Mistakes
- Writing Concisely
- Avoiding Plagiarism
- Footnotes or Endnotes?
- Further Readings
- Generative AI and Writing
- USC Libraries Tutorials and Other Guides
- Bibliography
The conclusion is intended to help the reader understand why your research should matter to them after they have finished reading the paper. A conclusion is not merely a summary of the main topics covered or a re-statement of your research problem, but a synthesis of key points derived from the findings of your study and, if applicable, where you recommend new areas for future research. For most college-level research papers, two or three well-developed paragraphs is sufficient for a conclusion, although in some cases, more paragraphs may be required in describing the key findings and their significance.
Conclusions. The Writing Center. University of North Carolina; Conclusions. The Writing Lab and The OWL. Purdue University.
Importance of a Good Conclusion
A well-written conclusion provides you with important opportunities to demonstrate to the reader your understanding of the research problem. These include:
- Presenting the last word on the issues you raised in your paper . Just as the introduction gives a first impression to your reader, the conclusion offers a chance to leave a lasting impression. Do this, for example, by highlighting key findings in your analysis that advance new understanding about the research problem, that are unusual or unexpected, or that have important implications applied to practice.
- Summarizing your thoughts and conveying the larger significance of your study . The conclusion is an opportunity to succinctly re-emphasize your answer to the "So What?" question by placing the study within the context of how your research advances past research about the topic.
- Identifying how a gap in the literature has been addressed . The conclusion can be where you describe how a previously identified gap in the literature [first identified in your literature review section] has been addressed by your research and why this contribution is significant.
- Demonstrating the importance of your ideas . Don't be shy. The conclusion offers an opportunity to elaborate on the impact and significance of your findings. This is particularly important if your study approached examining the research problem from an unusual or innovative perspective.
- Introducing possible new or expanded ways of thinking about the research problem . This does not refer to introducing new information [which should be avoided], but to offer new insight and creative approaches for framing or contextualizing the research problem based on the results of your study.
Bunton, David. “The Structure of PhD Conclusion Chapters.” Journal of English for Academic Purposes 4 (July 2005): 207–224; Conclusions. The Writing Center. University of North Carolina; Kretchmer, Paul. Twelve Steps to Writing an Effective Conclusion. San Francisco Edit, 2003-2008; Conclusions. The Writing Lab and The OWL. Purdue University; Assan, Joseph. "Writing the Conclusion Chapter: The Good, the Bad and the Missing." Liverpool: Development Studies Association (2009): 1-8.
Structure and Writing Style
I. General Rules
The general function of your paper's conclusion is to restate the main argument . It reminds the reader of the strengths of your main argument(s) and reiterates the most important evidence supporting those argument(s). Do this by clearly summarizing the context, background, and necessity of pursuing the research problem you investigated in relation to an issue, controversy, or a gap found in the literature. However, make sure that your conclusion is not simply a repetitive summary of the findings. This reduces the impact of the argument(s) you have developed in your paper.
When writing the conclusion to your paper, follow these general rules:
- Present your conclusions in clear, concise language. Re-state the purpose of your study, then describe how your findings differ or support those of other studies and why [i.e., what were the unique, new, or crucial contributions your study made to the overall research about your topic?].
- Do not simply reiterate your findings or the discussion of your results. Provide a synthesis of arguments presented in the paper to show how these converge to address the research problem and the overall objectives of your study.
- Indicate opportunities for future research if you haven't already done so in the discussion section of your paper. Highlighting the need for further research provides the reader with evidence that you have an in-depth awareness of the research problem but that further investigations should take place beyond the scope of your investigation.
Consider the following points to help ensure your conclusion is presented well:
- If the argument or purpose of your paper is complex, you may need to summarize the argument for your reader.
- If, prior to your conclusion, you have not yet explained the significance of your findings or if you are proceeding inductively, use the end of your paper to describe your main points and explain their significance.
- Move from a detailed to a general level of consideration that returns the topic to the context provided by the introduction or within a new context that emerges from the data [this is opposite of the introduction, which begins with general discussion of the context and ends with a detailed description of the research problem].
The conclusion also provides a place for you to persuasively and succinctly restate the research problem, given that the reader has now been presented with all the information about the topic . Depending on the discipline you are writing in, the concluding paragraph may contain your reflections on the evidence presented. However, the nature of being introspective about the research you have conducted will depend on the topic and whether your professor wants you to express your observations in this way. If asked to think introspectively about the topics, do not delve into idle speculation. Being introspective means looking within yourself as an author to try and understand an issue more deeply, not to guess at possible outcomes or make up scenarios not supported by the evidence.
II. Developing a Compelling Conclusion
Although an effective conclusion needs to be clear and succinct, it does not need to be written passively or lack a compelling narrative. Strategies to help you move beyond merely summarizing the key points of your research paper may include any of the following:
- If your essay deals with a critical, contemporary problem, warn readers of the possible consequences of not attending to the problem proactively.
- Recommend a specific course or courses of action that, if adopted, could address a specific problem in practice or in the development of new knowledge leading to positive change.
- Cite a relevant quotation or expert opinion already noted in your paper in order to lend authority and support to the conclusion(s) you have reached [a good source would be from your literature review].
- Explain the consequences of your research in a way that elicits action or demonstrates urgency in seeking change.
- Restate a key statistic, fact, or visual image to emphasize the most important finding of your paper.
- If your discipline encourages personal reflection, illustrate your concluding point by drawing from your own life experiences.
- Return to an anecdote, an example, or a quotation that you presented in your introduction, but add further insight derived from the findings of your study; use your interpretation of results from your study to recast it in new or important ways.
- Provide a "take-home" message in the form of a succinct, declarative statement that you want the reader to remember about your study.
III. Problems to Avoid
Failure to be concise Your conclusion section should be concise and to the point. Conclusions that are too lengthy often have unnecessary information in them. The conclusion is not the place for details about your methodology or results. Although you should give a summary of what was learned from your research, this summary should be relatively brief, since the emphasis in the conclusion is on the implications, evaluations, insights, and other forms of analysis that you make. Strategies for writing concisely can be found here .
Failure to comment on larger, more significant issues In the introduction, your task was to move from the general [the field of study] to the specific [the research problem]. However, in the conclusion, your task is to move from a specific discussion [your research problem] back to a general discussion framed around the implications and significance of your findings [i.e., how your research contributes new understanding or fills an important gap in the literature]. In short, the conclusion is where you should place your research within a larger context [visualize your paper as an hourglass--start with a broad introduction and review of the literature, move to the specific analysis and discussion, conclude with a broad summary of the study's implications and significance].
Failure to reveal problems and negative results Negative aspects of the research process should never be ignored. These are problems, deficiencies, or challenges encountered during your study. They should be summarized as a way of qualifying your overall conclusions. If you encountered negative or unintended results [i.e., findings that are validated outside the research context in which they were generated], you must report them in the results section and discuss their implications in the discussion section of your paper. In the conclusion, use negative results as an opportunity to explain their possible significance and/or how they may form the basis for future research.
Failure to provide a clear summary of what was learned In order to be able to discuss how your research fits within your field of study [and possibly the world at large], you need to summarize briefly and succinctly how it contributes to new knowledge or a new understanding about the research problem. This element of your conclusion may be only a few sentences long.
Failure to match the objectives of your research Often research objectives in the social and behavioral sciences change while the research is being carried out. This is not a problem unless you forget to go back and refine the original objectives in your introduction. As these changes emerge they must be documented so that they accurately reflect what you were trying to accomplish in your research [not what you thought you might accomplish when you began].
Resist the urge to apologize If you've immersed yourself in studying the research problem, you presumably should know a good deal about it [perhaps even more than your professor!]. Nevertheless, by the time you have finished writing, you may be having some doubts about what you have produced. Repress those doubts! Don't undermine your authority as a researcher by saying something like, "This is just one approach to examining this problem; there may be other, much better approaches that...." The overall tone of your conclusion should convey confidence to the reader about the study's validity and realiability.
Assan, Joseph. "Writing the Conclusion Chapter: The Good, the Bad and the Missing." Liverpool: Development Studies Association (2009): 1-8; Concluding Paragraphs. College Writing Center at Meramec. St. Louis Community College; Conclusions. The Writing Center. University of North Carolina; Conclusions. The Writing Lab and The OWL. Purdue University; Freedman, Leora and Jerry Plotnick. Introductions and Conclusions. The Lab Report. University College Writing Centre. University of Toronto; Leibensperger, Summer. Draft Your Conclusion. Academic Center, the University of Houston-Victoria, 2003; Make Your Last Words Count. The Writer’s Handbook. Writing Center. University of Wisconsin Madison; Miquel, Fuster-Marquez and Carmen Gregori-Signes. “Chapter Six: ‘Last but Not Least:’ Writing the Conclusion of Your Paper.” In Writing an Applied Linguistics Thesis or Dissertation: A Guide to Presenting Empirical Research . John Bitchener, editor. (Basingstoke,UK: Palgrave Macmillan, 2010), pp. 93-105; Tips for Writing a Good Conclusion. Writing@CSU. Colorado State University; Kretchmer, Paul. Twelve Steps to Writing an Effective Conclusion. San Francisco Edit, 2003-2008; Writing Conclusions. Writing Tutorial Services, Center for Innovative Teaching and Learning. Indiana University; Writing: Considering Structure and Organization. Institute for Writing Rhetoric. Dartmouth College.
Writing Tip
Don't Belabor the Obvious!
Avoid phrases like "in conclusion...," "in summary...," or "in closing...." These phrases can be useful, even welcome, in oral presentations. But readers can see by the tell-tale section heading and number of pages remaining that they are reaching the end of your paper. You'll irritate your readers if you belabor the obvious.
Assan, Joseph. "Writing the Conclusion Chapter: The Good, the Bad and the Missing." Liverpool: Development Studies Association (2009): 1-8.
Another Writing Tip
New Insight, Not New Information!
Don't surprise the reader with new information in your conclusion that was never referenced anywhere else in the paper. This why the conclusion rarely has citations to sources. If you have new information to present, add it to the discussion or other appropriate section of the paper. Note that, although no new information is introduced, the conclusion, along with the discussion section, is where you offer your most "original" contributions in the paper; the conclusion is where you describe the value of your research, demonstrate that you understand the material that you’ve presented, and position your findings within the larger context of scholarship on the topic, including describing how your research contributes new insights to that scholarship.
Assan, Joseph. "Writing the Conclusion Chapter: The Good, the Bad and the Missing." Liverpool: Development Studies Association (2009): 1-8; Conclusions. The Writing Center. University of North Carolina.
- << Previous: Limitations of the Study
- Next: Appendices >>
- Last Updated: May 18, 2024 11:38 AM
- URL: https://libguides.usc.edu/writingguide
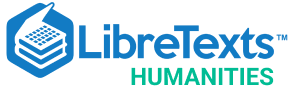
- school Campus Bookshelves
- menu_book Bookshelves
- perm_media Learning Objects
- login Login
- how_to_reg Request Instructor Account
- hub Instructor Commons
Margin Size
- Download Page (PDF)
- Download Full Book (PDF)
- Periodic Table
- Physics Constants
- Scientific Calculator
- Reference & Cite
- Tools expand_more
- Readability
selected template will load here
This action is not available.
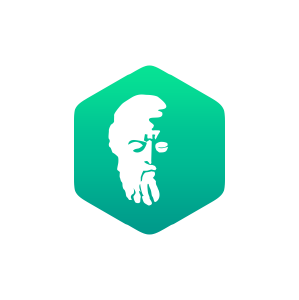
15.2: Inferences from Samples to Populations
- Last updated
- Save as PDF
- Page ID 95149
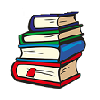
- Jason Southworth & Chris Swoyer
- Fort Hays State & University University of Oklahoma
\( \newcommand{\vecs}[1]{\overset { \scriptstyle \rightharpoonup} {\mathbf{#1}} } \)
\( \newcommand{\vecd}[1]{\overset{-\!-\!\rightharpoonup}{\vphantom{a}\smash {#1}}} \)
\( \newcommand{\id}{\mathrm{id}}\) \( \newcommand{\Span}{\mathrm{span}}\)
( \newcommand{\kernel}{\mathrm{null}\,}\) \( \newcommand{\range}{\mathrm{range}\,}\)
\( \newcommand{\RealPart}{\mathrm{Re}}\) \( \newcommand{\ImaginaryPart}{\mathrm{Im}}\)
\( \newcommand{\Argument}{\mathrm{Arg}}\) \( \newcommand{\norm}[1]{\| #1 \|}\)
\( \newcommand{\inner}[2]{\langle #1, #2 \rangle}\)
\( \newcommand{\Span}{\mathrm{span}}\)
\( \newcommand{\id}{\mathrm{id}}\)
\( \newcommand{\kernel}{\mathrm{null}\,}\)
\( \newcommand{\range}{\mathrm{range}\,}\)
\( \newcommand{\RealPart}{\mathrm{Re}}\)
\( \newcommand{\ImaginaryPart}{\mathrm{Im}}\)
\( \newcommand{\Argument}{\mathrm{Arg}}\)
\( \newcommand{\norm}[1]{\| #1 \|}\)
\( \newcommand{\Span}{\mathrm{span}}\) \( \newcommand{\AA}{\unicode[.8,0]{x212B}}\)
\( \newcommand{\vectorA}[1]{\vec{#1}} % arrow\)
\( \newcommand{\vectorAt}[1]{\vec{\text{#1}}} % arrow\)
\( \newcommand{\vectorB}[1]{\overset { \scriptstyle \rightharpoonup} {\mathbf{#1}} } \)
\( \newcommand{\vectorC}[1]{\textbf{#1}} \)
\( \newcommand{\vectorD}[1]{\overrightarrow{#1}} \)
\( \newcommand{\vectorDt}[1]{\overrightarrow{\text{#1}}} \)
\( \newcommand{\vectE}[1]{\overset{-\!-\!\rightharpoonup}{\vphantom{a}\smash{\mathbf {#1}}}} \)
We frequently use sample statistics to draw inductive inferences about population parameters. When a newspaper conducts a poll to see how many people think President Trump should be impeached, they check with a sample, say 2,000 adults across the U. S. and draw a conclusion about what American adults in general think. Their results would be more accurate if they checked with everyone, but when a population is large, it simply isn’t practical to examine all its members. We have no choice but to rely on a sample from the population and make an inference based on it. When scientists engage in such inferences, they are said to be using inferential statistics . But all of us draw inferences from samples to populations many times every day.
Sampling in Everyday Life
Inferences based on samples are common in medical research, the social sciences, and polling. In these settings, scientists use what are called inferential statistics to move from claims about samples to conclusions about populations.
But we all draw similar inferences many times each day. You are driving through Belleville, KS for the first time and trying to decide where to eat. You have had good experiences at McDonalds restaurants in the past (the set of McDonald’s restaurants where you have eaten in the past at constitutes your sample). So, you might conclude that all McDonald’s restaurants (the population) are likely to be good and decide to sample the culinary delights of the one in Belleville. Or suppose you know six people (this is your sample) who have dated Wilbur, and all of them found him boring. You may well conclude that almost everyone (this is the population) would find him boring.
Whenever you make a generalization based on several (but not all) of the cases, you used sampling. You are drawing a conclusion about some larger group based on what you’ve observed about one of its subgroups.
Most learning from experience involves drawing inferences about unobserved cases (populations) from information about a limited number of cases that we have observed (our samples). You know what strategies worked in the cases you have experienced (your sample) for getting a date, quitting smoking, getting your car running when the battery seems dead, or doing well on an exam. And you then draw conclusions about the relevant populations based on this knowledge.
An examination is really a sampling procedure to determine how much you have learned. When your calculus professor makes up an examination, they hope to sample items from the population of things you have learned in the course and to use your grade as an indicator of how much information you have acquired.
Samples and Inference
We often infer a conclusion about a population from a description of a sample that was drawn from it. When we do:
- Our premises are claims about the sample .
- Our conclusion is a claim about the population .
.png?revision=1&size=bestfit&width=264&height=268)
For example, we might draw a conclusion about the divorce rate of people living in Florida from premises describing the divorce rates among 800 couples that our school’s Human Relations Department sampled.
In such a case, our inference is not deductively valid. It involves an inductive leap. The conclusion goes beyond the information in the argument’s premises, because it contains information about the entire population , while the premises only contain information about the sample. But if we are careful, our inference can still be inductively strong. This means that if we begin with true premises (which in this case means a correct description of the sample), we are likely to arrive at a true conclusion (about the entire population).
Good Samples
A good inductive inference from a sample to a population requires:
- A large enough sample.
- A representative (unbiased) sample.
We would need to delve more deeply into probability to say exactly how large is large enough, but we won’t worry about that here. The important point is that in everyday life we very often rely on samples that are clearly too small.
We also need a sample that is as representative of the entire population as possible. A sample that is not representative is said to be biased. An unbiased sample is typical of the population. By contrast, in a biased sample, some portions of the population are overrepresented and others are underrepresented .
The problem with a very small sample is that it is not likely to be representative. Other things being equal, a bigger sample will be more representative. But there are costs to gathering information—a price in time, dollars, and energy—so it is rarely feasible to get huge samples.
We can never be certain that a sample is unbiased, but we can strive to avoid any non-trivial biases we can discover. With some thought, the worst biases are often obvious. Suppose, for example, that we want to know what the U.S. adult public (our population) thinks about the consumption of alcohol. We would clearly get a biased sample if we distributed questionnaires only at a pool hall (we would have an overrepresentation of drinkers and an underrepresentation of those favoring temperance), or only at the local meetings of MADD (here, the biases would be reversed).
A classic example of a biased sample occurred in 1936, when a magazine, The Literary Digest , conducted a poll using names in telephone directories and on car registration lists. Most of the people they sampled favored Alf Landon over Franklin Roosevelt in that year’s Presidential election, but when election day rolled around, Roosevelt won in a landslide. What went wrong? News organizations now use telephone polling routinely, but in 1936, a relatively small percentage of people had telephones and cars, and most of them were affluent. These were the people most likely to vote for the Republican candidate, Landon, and so the sample was not representative of all voters.
There are other cases where bias is likely, even though it won’t be this blatant. For example, any time members of the sample volunteer, e.g., by returning a questionnaire in the mail, we are likely to have a biased sample. People willing to return a questionnaire are likely to differ in various ways from people who are not. Or, to take an example closer to home, tests that focus on only some of the material covered in class are likely to elicit a biased, unrepresentative sample of what you have learned. They aren’t a fair sample of what you know. Unfortunately, in some cases biases may be difficult to detect, and it may require a good deal of expertise to find it at all.
Random Sampling
The best way to obtain an unbiased sample is to use random sampling. A random sample is a method of sampling in which each member of the population has an equally good chance of being chosen for the sample. Random sampling does not guarantee a representative sample—nothing short of checking the entire population can guarantee that—but it does make it more likely. Random sampling avoids the biases involved with many other methods of sampling.
We can rarely get a truly random sample, but under some conditions, typically in carefully conducted studies and surveys, we can come reasonably close. But even in daily life we can use samples that are much less biased than those we often rely on.
Random Digit Dialing (RDD)
Modern technology now allows national polling organizations with large resources to approach the ideal of random sampling. Polls like the New York Times/CBS News poll use what is called Random Digit Dialing (RDD). The goal here is to give every residential phone number an equal chance of being called for an interview. Nowadays, almost all major polls use some form of RDD. For example, the New York Times/CBS News poll uses the GENESYS system, which employs a database of over 42,000 residential telephone numbers throughout the U.S. that is updated every few months. The system also employs software that draws a random sample of phone numbers from this database and then randomly makes up the last four digits of the number to be called. Of course, some sorts of people are harder to reach on the phone than others, and some sorts are more willing to volunteer information over the phone (the elderly tend to be more available, more willing to share, and more likely to answer an unknown number, for instance). But RDD constitutes an impressive step in the direction of randomization.
Stratified Random Sampling
Scientists sometimes go a step further and use a stratified random sample . Here, the aim is to ensure that there is a certain percentage of members of various subpopulations in our sample (e.g., an equal number of men and of women). They separate the population into relevant categories or “strata” before sampling (e.g., into the categories or subpopulations of men and of women). Then they sample randomly within each category. The thinking here is that results will be more accurate than mere random sampling because random sampling might accidentally over-weigh some group (because they were sampled at a higher rate through variance). So, rather than doing a random statewide poll about who is favored in the gubernatorial election, a poll using stratified random polling would make sure to poll all counties in proportion to turnout expectations (they might also stratify further based on gender, race, education, etc.).
A growing practical problem in recent years has been the decline in the public’s participation in polls. Pollsters are getting more and more “nonresponses.” Some of these result from the difficulty in contacting people by phone (people are at work, unwillingness to answer unknown numbers, etc.). But those contacted are also less willing to participate than they were in the past. The reasons for this aren’t completely clear, but growing disillusionment with politics and lack of patience for unsolicited calls resulting from the increase in telemarketing may be part of the reason.
The use of push polling also leads to a wariness about polls. Push polling is not really polling at all. Instead, an organization, e.g., the campaign organization of a candidate running for Senate, calls thousands of homes. The caller says they are conducting a poll, but in fact no results are collected and instead a damaging— and often false—claim about the other side is implanted in what is presented as a neutral question. For example, the caller might ask, “Do you agree with Candidate X’s goal to cut social security payments over the next six years?” Such deceptive uses of polling are likely to make the public more cynical about polls.
Sampling Variability
It is unlikely that any sample that is substantially smaller than the parent population will be perfectly representative of the population. Suppose that we drew many samples of the same size from the same population. For example, if we drew many samples of 1,000 each from the entire population of Oklahoma voters. The set of all these samples in called a sampling distribution .
The samples in our sampling distribution will vary from one to another. This just means that if we draw many samples from the same population, we are likely to get somewhat different results each time. For example, if we examine twenty samples, each with 1,000 Oklahoma voters, we are likely to find different percentages of Republicans in each of our samples.
This variation among samples is called sampling variability or sampling error (though it is not really an error or mistake). Suppose that we just take one sample of 1,000 Oklahoma voters and discover that 60% of them prefer the Republican candidate for Governor. Because of sampling variability, we know that if we had drawn a different sample of the same size, we would probably have gotten a somewhat different percentage of people favoring the Republican. So, we won’t be able to conclude that exactly 60% of all Oklahoma voters favor the Republican from the fact that 60% of the voters in our single sample do.
As our samples become larger, it becomes less likely that the sample mean will be the same as the mean of the parent population. But it becomes more likely that the mean of the sample will be close to the mean of the population. Thus, if 60% of the voters in a sample of 10 favor the Republican candidate, we can’t be very confident in predicting that about 60% of voters in general do. If 60% of a sample of 100 do, we can be more confident, and if 60% of a sample of 1,000 do, we can be more confident still.
Statisticians overcome the problem of sampling variability by calculating a margin of error . This is a number that tells us how close the result of a poll should usually be to the population parameter in question. For example, our claim might be that 60% of the population, plus or minus three percent , will vote Republican this coming year. The smaller the sample, the larger the margin of error.
But there are often large costs in obtaining a large sample, so we must compromise between what’s feasible and the margin of error. Here you do get what you pay for. It is surprising, but the size of the sample does not need to be a large percentage of the population for a poll or survey to be a good one. What is important is that the sample not be biased, and that it be large enough; once this is achieved, it is the absolute number of things in the sample (rather than the proportion of the population that the sample makes up) that is relevant for taking a reliable poll. In our daily life, we can’t hope for random samples, but with a little care we can avoid flagrant biases in our samples, and this can improve our reasoning dramatically.
Bad Sampling and Bad Reasoning
Many of the reasoning errors we will study in later chapters result from the use of small or biased samples. Drawing conclusions about a general population based on a sample from it is often called generalization. And drawing such a conclusion from a sample that is too small is sometimes called the fallacy of hasty generalization or, in everyday language, jumping to a conclusion .
Our samples are also often biased. For example, a person in a job interview may do unusually well (they are striving to create a good first impression) or unusually poorly (they may be very nervous). If so, their actions constitute a biased sample, and they will not be an accurate predictor of the candidate’s future job performance. In coming chapters, we will find many examples of bad reasoning that result from the use of samples that are too small, highly biased, or both.
Example: The Two Hospitals
There are two hospitals in Smudsville. About 50 babies are born every day in the larger one, and about 14 babies are born every day in the smaller one down the street. On average, 50% of the births in both hospitals are girls and 50% are boys, but the number bounces around some from one day to the next in both hospitals.
- Why would the percentage of boys vary from day to day?
- Which hospital, if either, is more likely to have more days per year when over 65% of the babies born are boys?
We all know that bigger samples are likely to be more representative of their parent populations. But we often fail to realize that we are dealing with a problem that involves this principle; we don’t “code” it as a problem involving sample size. Since about half of all births are boys and about half are girls, the true percentages in the general population are about half and half. Since a smaller sample will be less likely to reflect these true proportions, the smaller hospital is more likely to have more days per year when over 65% of the births are boys. The births at the smaller hospital constitutes a smaller sample.
This will seem more intuitive if you think about the following example. If you flipped a fair coin four times, you wouldn’t be all that surprised if you got four heads. The sample (four flips) is small, so this wouldn’t be too surprising; the probability is 116. But if you flipped the same coin one hundred times, you would be very surprised to get all heads. A sample this large is very unlikely to deviate so much from the population of all possible flips of the coin.
- What is the probability of getting all heads when you flip a fair coin (a) four times, (b) ten times, (c) twenty times, (d) one hundred times?
In 2–7 say (a) what the relevant population is, (b) what the sample is (i.e., what is in the sample), (c) whether the sample seems to be biased; then (d) evaluate the inference.
- We can’t afford to carefully check all the computer chips that come off the assembly line in our factory. But we check one out of every 300, using a randomizing device to pick the ones we examine. If we find a couple of bad ones, we check out the whole bunch.
- We can’t afford to carefully check all the computer chips coming off the assembly line. But we check the first few from the beginning of each day’s run. If we find a couple of bad ones, we check out the whole bunch.
- There are more than a hundred nuclear power plants operating in the United States and Western Europe today. Each of them has operated for years without killing anybody. So, nuclear power plants don’t seem to pose much of a danger to human life.
- Joining the Weight Away program is a good way to lose weight. Your friend Millie and uncle Wilbur both lost all the weight they wanted to lose, and they’ve kept if off for six months now.
- Joining the Weight Away program is a good way to lose weight. Consumer Reports did a pretty exhaustive study, surveying hundreds of people, and found that it worked better than any alternative method for losing weight. [This is a fictitious example; I don’t know the success rates of various weight-reduction programs.]
- Alfred has a good track record as a source of information about the social lives of people you know, so you conclude that he’s a reliable source of gossip in general.
- Pollsters are getting more and more “nonresponses.” Some of these result from the difficulty in contacting people by phone (people are at work, screening unknown numbers, etc.). But those contacted are also less willing to participate than they were in the past. Does this automatically mean that recent polls are more likely to be biased? How could we determine whether there are? In which ways might they be biased (defend your answer)?
- We began the chapter with the story of cats that had fallen from windows. What could account for these finding?
- Several groups of studies have shown that the survival rates of patients after surgery is actually lower in better hospitals than in hospitals that aren’t as good. More people die in the better hospitals. What might account for this?
- The psychologist Robyn Dawes recounts a story about his involvement with a committee that was trying to set guidelines for professional psychologists. They were trying to decide what the rules should be for reporting a client (and thus breaching confidentiality) who admitted having sexually abused children, when the abuse occurred in the distant past. Several people on the committee said that they should be required to report it, even when it occurred in the distant past, because the one sure thing about child abusers is that they never stop on their own without professional help. Dawes asked the others how they knew this. They replied, quite sincerely, that as counselors they had extensive contact with child abusers. Does this give us good reason to think that child abusers rarely stop on their own? What problems, if any, are involved in the group’s reasoning?
- A poll with a 4% margin of error finds that 47% of voters surveyed plan to vote for Smith for Sheriff and 51% plan to vote for her opponent, Jones. What information would you like to know in evaluating these results? Assuming sound polling procedures were used, what can we conclude about who will win the election?
- Suppose that a polling organization was worried that a telephone poll would result in a biased sample. So instead, they pick addresses randomly and visit the residences in person. They have limited resources, however, and so they can only make one visit to each residence, and if no one is home they just go on to the next place on their list. How representative is this sample likely to be? What are its flaws? Could they be corrected? At what cost?
- Suppose that you are an excellent chess player and that Wilbur is good, but not as good as you. Would you be more likely to beat him in a best of three series or in a best of seven series (or would the number of games make any difference)? Defend your answer.
- A study was conducted by the Centers for Disease Control and Prevention was reported in the Journal of the American Medical Association (JAMA). The study was based on a survey of 9,215 adult patients who were members of Kaiser Permanente health maintenance organization (an HMO). The study focused on eight childhood traumas, including psychological, physical and sexual abuse, having a mother who was abused, parents who are separated or divorced, and living with those were substance abusers, mentally ill, or who had been imprisoned. It was found that more instances of trauma seemed to increase the probability of smoking. For example, people who experienced five or more instances of trauma were 5.4 times more likely to start smoking by the age of 14 than were people who reported no childhood trauma. Analyze this study. What was the sample and the population? What are possible strong points and weak points?
In 16 and 17 explain what (if anything) is wrong in each of the following examples. When reasoning goes wrong, it often goes very wrong, so it’s quite possible for even a short argument to be flawed in more than one way.
- Suppose that the NRA (National Rifle Association) recently conducted a poll of its members and found that they were overwhelmingly opposed to any further gun control measures.
- Suppose that a random sample of 500 students at our college showed that an overwhelming majority do not think that the school needs to budget more money for making the campus more accessible to people with disabilities.
- A TV psychologist recently noted that the average marriage lasts 7 years, a fact that she sought to explain by pointing to evidence that life goes in 7 year cycles (so that it was not surprising that marriages should last 7 years). How may she have misunderstood the fact that she was explaining?
10. The actual cause, though you could only hypothesize it based on the information in this problem, turns out to be that high-risk patients, those needing the most dangerous types of surgery, often go to better hospitals (which are more likely to provide such surgery, or at least more likely to provide it at a lower risk).
14. Hint: think about the hospital example above.
Get science-backed answers as you write with Paperpal's Research feature
How to Write a Conclusion for Research Papers (with Examples)
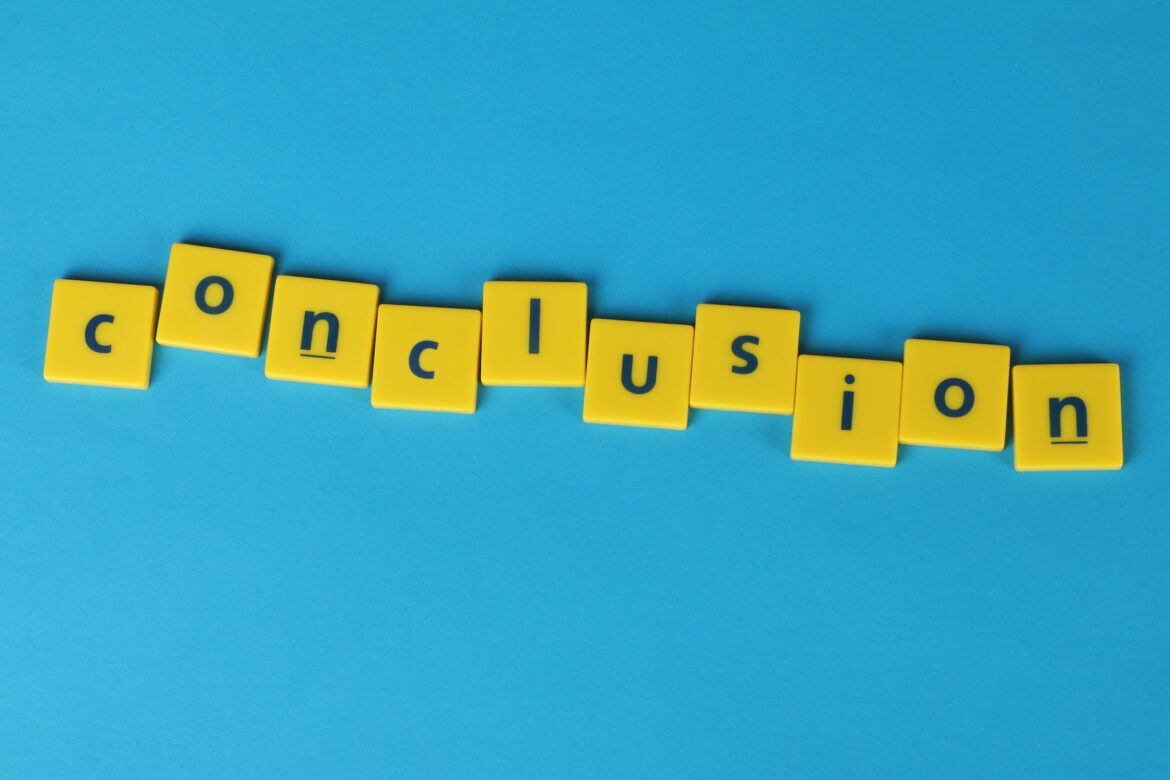
The conclusion of a research paper is a crucial section that plays a significant role in the overall impact and effectiveness of your research paper. However, this is also the section that typically receives less attention compared to the introduction and the body of the paper. The conclusion serves to provide a concise summary of the key findings, their significance, their implications, and a sense of closure to the study. Discussing how can the findings be applied in real-world scenarios or inform policy, practice, or decision-making is especially valuable to practitioners and policymakers. The research paper conclusion also provides researchers with clear insights and valuable information for their own work, which they can then build on and contribute to the advancement of knowledge in the field.
The research paper conclusion should explain the significance of your findings within the broader context of your field. It restates how your results contribute to the existing body of knowledge and whether they confirm or challenge existing theories or hypotheses. Also, by identifying unanswered questions or areas requiring further investigation, your awareness of the broader research landscape can be demonstrated.
Remember to tailor the research paper conclusion to the specific needs and interests of your intended audience, which may include researchers, practitioners, policymakers, or a combination of these.
Table of Contents
What is a conclusion in a research paper, summarizing conclusion, editorial conclusion, externalizing conclusion, importance of a good research paper conclusion, how to write a conclusion for your research paper, research paper conclusion examples.
- How to write a research paper conclusion with Paperpal?
Frequently Asked Questions
A conclusion in a research paper is the final section where you summarize and wrap up your research, presenting the key findings and insights derived from your study. The research paper conclusion is not the place to introduce new information or data that was not discussed in the main body of the paper. When working on how to conclude a research paper, remember to stick to summarizing and interpreting existing content. The research paper conclusion serves the following purposes: 1
- Warn readers of the possible consequences of not attending to the problem.
- Recommend specific course(s) of action.
- Restate key ideas to drive home the ultimate point of your research paper.
- Provide a “take-home” message that you want the readers to remember about your study.
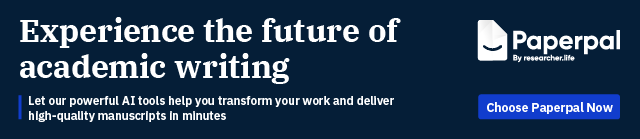
Types of conclusions for research papers
In research papers, the conclusion provides closure to the reader. The type of research paper conclusion you choose depends on the nature of your study, your goals, and your target audience. I provide you with three common types of conclusions:
A summarizing conclusion is the most common type of conclusion in research papers. It involves summarizing the main points, reiterating the research question, and restating the significance of the findings. This common type of research paper conclusion is used across different disciplines.
An editorial conclusion is less common but can be used in research papers that are focused on proposing or advocating for a particular viewpoint or policy. It involves presenting a strong editorial or opinion based on the research findings and offering recommendations or calls to action.
An externalizing conclusion is a type of conclusion that extends the research beyond the scope of the paper by suggesting potential future research directions or discussing the broader implications of the findings. This type of conclusion is often used in more theoretical or exploratory research papers.
Align your conclusion’s tone with the rest of your research paper. Start Writing with Paperpal Now!
The conclusion in a research paper serves several important purposes:
- Offers Implications and Recommendations : Your research paper conclusion is an excellent place to discuss the broader implications of your research and suggest potential areas for further study. It’s also an opportunity to offer practical recommendations based on your findings.
- Provides Closure : A good research paper conclusion provides a sense of closure to your paper. It should leave the reader with a feeling that they have reached the end of a well-structured and thought-provoking research project.
- Leaves a Lasting Impression : Writing a well-crafted research paper conclusion leaves a lasting impression on your readers. It’s your final opportunity to leave them with a new idea, a call to action, or a memorable quote.
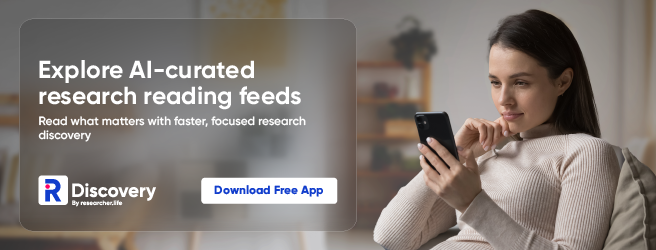
Writing a strong conclusion for your research paper is essential to leave a lasting impression on your readers. Here’s a step-by-step process to help you create and know what to put in the conclusion of a research paper: 2
- Research Statement : Begin your research paper conclusion by restating your research statement. This reminds the reader of the main point you’ve been trying to prove throughout your paper. Keep it concise and clear.
- Key Points : Summarize the main arguments and key points you’ve made in your paper. Avoid introducing new information in the research paper conclusion. Instead, provide a concise overview of what you’ve discussed in the body of your paper.
- Address the Research Questions : If your research paper is based on specific research questions or hypotheses, briefly address whether you’ve answered them or achieved your research goals. Discuss the significance of your findings in this context.
- Significance : Highlight the importance of your research and its relevance in the broader context. Explain why your findings matter and how they contribute to the existing knowledge in your field.
- Implications : Explore the practical or theoretical implications of your research. How might your findings impact future research, policy, or real-world applications? Consider the “so what?” question.
- Future Research : Offer suggestions for future research in your area. What questions or aspects remain unanswered or warrant further investigation? This shows that your work opens the door for future exploration.
- Closing Thought : Conclude your research paper conclusion with a thought-provoking or memorable statement. This can leave a lasting impression on your readers and wrap up your paper effectively. Avoid introducing new information or arguments here.
- Proofread and Revise : Carefully proofread your conclusion for grammar, spelling, and clarity. Ensure that your ideas flow smoothly and that your conclusion is coherent and well-structured.
Write your research paper conclusion 2x faster with Paperpal. Try it now!
Remember that a well-crafted research paper conclusion is a reflection of the strength of your research and your ability to communicate its significance effectively. It should leave a lasting impression on your readers and tie together all the threads of your paper. Now you know how to start the conclusion of a research paper and what elements to include to make it impactful, let’s look at a research paper conclusion sample.
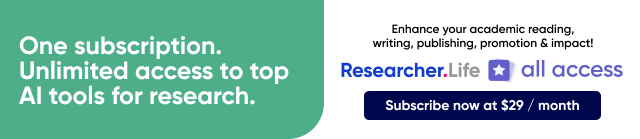
How to write a research paper conclusion with Paperpal?
A research paper conclusion is not just a summary of your study, but a synthesis of the key findings that ties the research together and places it in a broader context. A research paper conclusion should be concise, typically around one paragraph in length. However, some complex topics may require a longer conclusion to ensure the reader is left with a clear understanding of the study’s significance. Paperpal, an AI writing assistant trusted by over 800,000 academics globally, can help you write a well-structured conclusion for your research paper.
- Sign Up or Log In: Create a new Paperpal account or login with your details.
- Navigate to Features : Once logged in, head over to the features’ side navigation pane. Click on Templates and you’ll find a suite of generative AI features to help you write better, faster.
- Generate an outline: Under Templates, select ‘Outlines’. Choose ‘Research article’ as your document type.
- Select your section: Since you’re focusing on the conclusion, select this section when prompted.
- Choose your field of study: Identifying your field of study allows Paperpal to provide more targeted suggestions, ensuring the relevance of your conclusion to your specific area of research.
- Provide a brief description of your study: Enter details about your research topic and findings. This information helps Paperpal generate a tailored outline that aligns with your paper’s content.
- Generate the conclusion outline: After entering all necessary details, click on ‘generate’. Paperpal will then create a structured outline for your conclusion, to help you start writing and build upon the outline.
- Write your conclusion: Use the generated outline to build your conclusion. The outline serves as a guide, ensuring you cover all critical aspects of a strong conclusion, from summarizing key findings to highlighting the research’s implications.
- Refine and enhance: Paperpal’s ‘Make Academic’ feature can be particularly useful in the final stages. Select any paragraph of your conclusion and use this feature to elevate the academic tone, ensuring your writing is aligned to the academic journal standards.
By following these steps, Paperpal not only simplifies the process of writing a research paper conclusion but also ensures it is impactful, concise, and aligned with academic standards. Sign up with Paperpal today and write your research paper conclusion 2x faster .
The research paper conclusion is a crucial part of your paper as it provides the final opportunity to leave a strong impression on your readers. In the research paper conclusion, summarize the main points of your research paper by restating your research statement, highlighting the most important findings, addressing the research questions or objectives, explaining the broader context of the study, discussing the significance of your findings, providing recommendations if applicable, and emphasizing the takeaway message. The main purpose of the conclusion is to remind the reader of the main point or argument of your paper and to provide a clear and concise summary of the key findings and their implications. All these elements should feature on your list of what to put in the conclusion of a research paper to create a strong final statement for your work.
A strong conclusion is a critical component of a research paper, as it provides an opportunity to wrap up your arguments, reiterate your main points, and leave a lasting impression on your readers. Here are the key elements of a strong research paper conclusion: 1. Conciseness : A research paper conclusion should be concise and to the point. It should not introduce new information or ideas that were not discussed in the body of the paper. 2. Summarization : The research paper conclusion should be comprehensive enough to give the reader a clear understanding of the research’s main contributions. 3 . Relevance : Ensure that the information included in the research paper conclusion is directly relevant to the research paper’s main topic and objectives; avoid unnecessary details. 4 . Connection to the Introduction : A well-structured research paper conclusion often revisits the key points made in the introduction and shows how the research has addressed the initial questions or objectives. 5. Emphasis : Highlight the significance and implications of your research. Why is your study important? What are the broader implications or applications of your findings? 6 . Call to Action : Include a call to action or a recommendation for future research or action based on your findings.
The length of a research paper conclusion can vary depending on several factors, including the overall length of the paper, the complexity of the research, and the specific journal requirements. While there is no strict rule for the length of a conclusion, but it’s generally advisable to keep it relatively short. A typical research paper conclusion might be around 5-10% of the paper’s total length. For example, if your paper is 10 pages long, the conclusion might be roughly half a page to one page in length.
In general, you do not need to include citations in the research paper conclusion. Citations are typically reserved for the body of the paper to support your arguments and provide evidence for your claims. However, there may be some exceptions to this rule: 1. If you are drawing a direct quote or paraphrasing a specific source in your research paper conclusion, you should include a citation to give proper credit to the original author. 2. If your conclusion refers to or discusses specific research, data, or sources that are crucial to the overall argument, citations can be included to reinforce your conclusion’s validity.
The conclusion of a research paper serves several important purposes: 1. Summarize the Key Points 2. Reinforce the Main Argument 3. Provide Closure 4. Offer Insights or Implications 5. Engage the Reader. 6. Reflect on Limitations
Remember that the primary purpose of the research paper conclusion is to leave a lasting impression on the reader, reinforcing the key points and providing closure to your research. It’s often the last part of the paper that the reader will see, so it should be strong and well-crafted.
- Makar, G., Foltz, C., Lendner, M., & Vaccaro, A. R. (2018). How to write effective discussion and conclusion sections. Clinical spine surgery, 31(8), 345-346.
- Bunton, D. (2005). The structure of PhD conclusion chapters. Journal of English for academic purposes , 4 (3), 207-224.
Paperpal is a comprehensive AI writing toolkit that helps students and researchers achieve 2x the writing in half the time. It leverages 21+ years of STM experience and insights from millions of research articles to provide in-depth academic writing, language editing, and submission readiness support to help you write better, faster.
Get accurate academic translations, rewriting support, grammar checks, vocabulary suggestions, and generative AI assistance that delivers human precision at machine speed. Try for free or upgrade to Paperpal Prime starting at US$19 a month to access premium features, including consistency, plagiarism, and 30+ submission readiness checks to help you succeed.
Experience the future of academic writing – Sign up to Paperpal and start writing for free!
Related Reads:
- 5 Reasons for Rejection After Peer Review
- Ethical Research Practices For Research with Human Subjects
7 Ways to Improve Your Academic Writing Process
- Paraphrasing in Academic Writing: Answering Top Author Queries
Preflight For Editorial Desk: The Perfect Hybrid (AI + Human) Assistance Against Compromised Manuscripts
You may also like, how to write a high-quality conference paper, academic editing: how to self-edit academic text with..., measuring academic success: definition & strategies for excellence, phd qualifying exam: tips for success , ai in education: it’s time to change the..., is it ethical to use ai-generated abstracts without..., what are journal guidelines on using generative ai..., quillbot review: features, pricing, and free alternatives, what is an academic paper types and elements , should you use ai tools like chatgpt for....
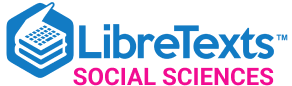
- school Campus Bookshelves
- menu_book Bookshelves
- perm_media Learning Objects
- login Login
- how_to_reg Request Instructor Account
- hub Instructor Commons
Margin Size
- Download Page (PDF)
- Download Full Book (PDF)
- Periodic Table
- Physics Constants
- Scientific Calculator
- Reference & Cite
- Tools expand_more
- Readability
selected template will load here
This action is not available.
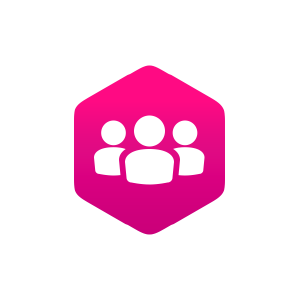
5.15: Drawing Conclusions from Statistics
- Last updated
- Save as PDF
- Page ID 59855
\( \newcommand{\vecs}[1]{\overset { \scriptstyle \rightharpoonup} {\mathbf{#1}} } \)
\( \newcommand{\vecd}[1]{\overset{-\!-\!\rightharpoonup}{\vphantom{a}\smash {#1}}} \)
\( \newcommand{\id}{\mathrm{id}}\) \( \newcommand{\Span}{\mathrm{span}}\)
( \newcommand{\kernel}{\mathrm{null}\,}\) \( \newcommand{\range}{\mathrm{range}\,}\)
\( \newcommand{\RealPart}{\mathrm{Re}}\) \( \newcommand{\ImaginaryPart}{\mathrm{Im}}\)
\( \newcommand{\Argument}{\mathrm{Arg}}\) \( \newcommand{\norm}[1]{\| #1 \|}\)
\( \newcommand{\inner}[2]{\langle #1, #2 \rangle}\)
\( \newcommand{\Span}{\mathrm{span}}\)
\( \newcommand{\id}{\mathrm{id}}\)
\( \newcommand{\kernel}{\mathrm{null}\,}\)
\( \newcommand{\range}{\mathrm{range}\,}\)
\( \newcommand{\RealPart}{\mathrm{Re}}\)
\( \newcommand{\ImaginaryPart}{\mathrm{Im}}\)
\( \newcommand{\Argument}{\mathrm{Arg}}\)
\( \newcommand{\norm}[1]{\| #1 \|}\)
\( \newcommand{\Span}{\mathrm{span}}\) \( \newcommand{\AA}{\unicode[.8,0]{x212B}}\)
\( \newcommand{\vectorA}[1]{\vec{#1}} % arrow\)
\( \newcommand{\vectorAt}[1]{\vec{\text{#1}}} % arrow\)
\( \newcommand{\vectorB}[1]{\overset { \scriptstyle \rightharpoonup} {\mathbf{#1}} } \)
\( \newcommand{\vectorC}[1]{\textbf{#1}} \)
\( \newcommand{\vectorD}[1]{\overrightarrow{#1}} \)
\( \newcommand{\vectorDt}[1]{\overrightarrow{\text{#1}}} \)
\( \newcommand{\vectE}[1]{\overset{-\!-\!\rightharpoonup}{\vphantom{a}\smash{\mathbf {#1}}}} \)
Learning Objectives
- Describe the role of random sampling and random assignment in drawing cause-and-effect conclusions
Generalizability
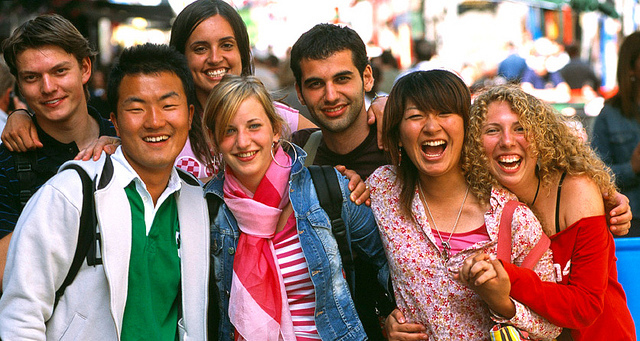
One limitation to the study mentioned previously about the babies choosing the “helper” toy is that the conclusion only applies to the 16 infants in the study. We don’t know much about how those 16 infants were selected. Suppose we want to select a subset of individuals (a sample ) from a much larger group of individuals (the population ) in such a way that conclusions from the sample can be generalized to the larger population. This is the question faced by pollsters every day.
Example 1 : The General Social Survey (GSS) is a survey on societal trends conducted every other year in the United States. Based on a sample of about 2,000 adult Americans, researchers make claims about what percentage of the U.S. population consider themselves to be “liberal,” what percentage consider themselves “happy,” what percentage feel “rushed” in their daily lives, and many other issues. The key to making these claims about the larger population of all American adults lies in how the sample is selected. The goal is to select a sample that is representative of the population, and a common way to achieve this goal is to select a random sample that gives every member of the population an equal chance of being selected for the sample. In its simplest form, random sampling involves numbering every member of the population and then using a computer to randomly select the subset to be surveyed. Most polls don’t operate exactly like this, but they do use probability-based sampling methods to select individuals from nationally representative panels.
In 2004, the GSS reported that 817 of 977 respondents (or 83.6%) indicated that they always or sometimes feel rushed. This is a clear majority, but we again need to consider variation due to random sampling . Fortunately, we can use the same probability model we did in the previous example to investigate the probable size of this error. (Note, we can use the coin-tossing model when the actual population size is much, much larger than the sample size, as then we can still consider the probability to be the same for every individual in the sample.) This probability model predicts that the sample result will be within 3 percentage points of the population value (roughly 1 over the square root of the sample size, the margin of error ). A statistician would conclude, with 95% confidence, that between 80.6% and 86.6% of all adult Americans in 2004 would have responded that they sometimes or always feel rushed.
The key to the margin of error is that when we use a probability sampling method, we can make claims about how often (in the long run, with repeated random sampling) the sample result would fall within a certain distance from the unknown population value by chance (meaning by random sampling variation) alone. Conversely, non-random samples are often suspect to bias, meaning the sampling method systematically over-represents some segments of the population and under-represents others. We also still need to consider other sources of bias, such as individuals not responding honestly. These sources of error are not measured by the margin of error.
Query \(\PageIndex{1}\)
Query \(\PageIndex{2}\)
Cause and Effect
In many research studies, the primary question of interest concerns differences between groups. Then the question becomes how were the groups formed (e.g., selecting people who already drink coffee vs. those who don’t). In some studies, the researchers actively form the groups themselves. But then we have a similar question—could any differences we observe in the groups be an artifact of that group-formation process? Or maybe the difference we observe in the groups is so large that we can discount a “fluke” in the group-formation process as a reasonable explanation for what we find?
Example 2 : A psychology study investigated whether people tend to display more creativity when they are thinking about intrinsic (internal) or extrinsic (external) motivations (Ramsey & Schafer, 2002, based on a study by Amabile, 1985). The subjects were 47 people with extensive experience with creative writing. Subjects began by answering survey questions about either intrinsic motivations for writing (such as the pleasure of self-expression) or extrinsic motivations (such as public recognition). Then all subjects were instructed to write a haiku, and those poems were evaluated for creativity by a panel of judges. The researchers conjectured beforehand that subjects who were thinking about intrinsic motivations would display more creativity than subjects who were thinking about extrinsic motivations. The creativity scores from the 47 subjects in this study are displayed in Figure 2, where higher scores indicate more creativity.
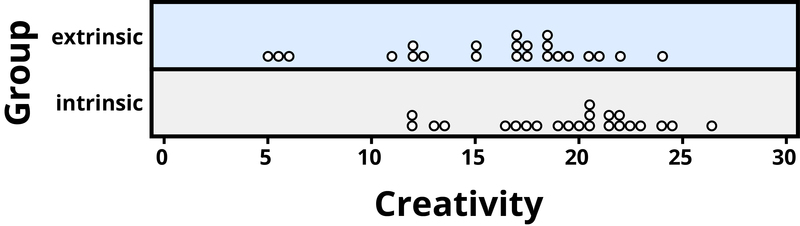
In this example, the key question is whether the type of motivation affects creativity scores. In particular, do subjects who were asked about intrinsic motivations tend to have higher creativity scores than subjects who were asked about extrinsic motivations?
Figure 2 reveals that both motivation groups saw considerable variability in creativity scores, and these scores have considerable overlap between the groups. In other words, it’s certainly not always the case that those with extrinsic motivations have higher creativity than those with intrinsic motivations, but there may still be a statistical tendency in this direction. (Psychologist Keith Stanovich (2013) refers to people’s difficulties with thinking about such probabilistic tendencies as “the Achilles heel of human cognition.”)
The mean creativity score is 19.88 for the intrinsic group, compared to 15.74 for the extrinsic group, which supports the researchers’ conjecture. Yet comparing only the means of the two groups fails to consider the variability of creativity scores in the groups. We can measure variability with statistics using, for instance, the standard deviation: 5.25 for the extrinsic group and 4.40 for the intrinsic group. The standard deviations tell us that most of the creativity scores are within about 5 points of the mean score in each group. We see that the mean score for the intrinsic group lies within one standard deviation of the mean score for extrinsic group. So, although there is a tendency for the creativity scores to be higher in the intrinsic group, on average, the difference is not extremely large.
We again want to consider possible explanations for this difference. The study only involved individuals with extensive creative writing experience. Although this limits the population to which we can generalize, it does not explain why the mean creativity score was a bit larger for the intrinsic group than for the extrinsic group. Maybe women tend to receive higher creativity scores? Here is where we need to focus on how the individuals were assigned to the motivation groups. If only women were in the intrinsic motivation group and only men in the extrinsic group, then this would present a problem because we wouldn’t know if the intrinsic group did better because of the different type of motivation or because they were women. However, the researchers guarded against such a problem by randomly assigning the individuals to the motivation groups. Like flipping a coin, each individual was just as likely to be assigned to either type of motivation. Why is this helpful? Because this random assignment tends to balance out all the variables related to creativity we can think of, and even those we don’t think of in advance, between the two groups. So we should have a similar male/female split between the two groups; we should have a similar age distribution between the two groups; we should have a similar distribution of educational background between the two groups; and so on. Random assignment should produce groups that are as similar as possible except for the type of motivation, which presumably eliminates all those other variables as possible explanations for the observed tendency for higher scores in the intrinsic group.
But does this always work? No, so by “luck of the draw” the groups may be a little different prior to answering the motivation survey. So then the question is, is it possible that an unlucky random assignment is responsible for the observed difference in creativity scores between the groups? In other words, suppose each individual’s poem was going to get the same creativity score no matter which group they were assigned to, that the type of motivation in no way impacted their score. Then how often would the random-assignment process alone lead to a difference in mean creativity scores as large (or larger) than 19.88 – 15.74 = 4.14 points?
We again want to apply to a probability model to approximate a p-value , but this time the model will be a bit different. Think of writing everyone’s creativity scores on an index card, shuffling up the index cards, and then dealing out 23 to the extrinsic motivation group and 24 to the intrinsic motivation group, and finding the difference in the group means. We (better yet, the computer) can repeat this process over and over to see how often, when the scores don’t change, random assignment leads to a difference in means at least as large as 4.41. Figure 3 shows the results from 1,000 such hypothetical random assignments for these scores.
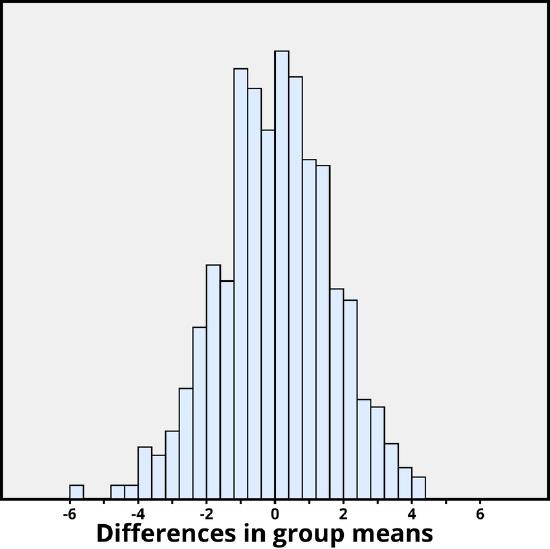
Only 2 of the 1,000 simulated random assignments produced a difference in group means of 4.41 or larger. In other words, the approximate p-value is 2/1000 = 0.002. This small p-value indicates that it would be very surprising for the random assignment process alone to produce such a large difference in group means. Therefore, as with Example 2, we have strong evidence that focusing on intrinsic motivations tends to increase creativity scores, as compared to thinking about extrinsic motivations.
Notice that the previous statement implies a cause-and-effect relationship between motivation and creativity score; is such a strong conclusion justified? Yes, because of the random assignment used in the study. That should have balanced out any other variables between the two groups, so now that the small p-value convinces us that the higher mean in the intrinsic group wasn’t just a coincidence, the only reasonable explanation left is the difference in the type of motivation. Can we generalize this conclusion to everyone? Not necessarily—we could cautiously generalize this conclusion to individuals with extensive experience in creative writing similar the individuals in this study, but we would still want to know more about how these individuals were selected to participate.
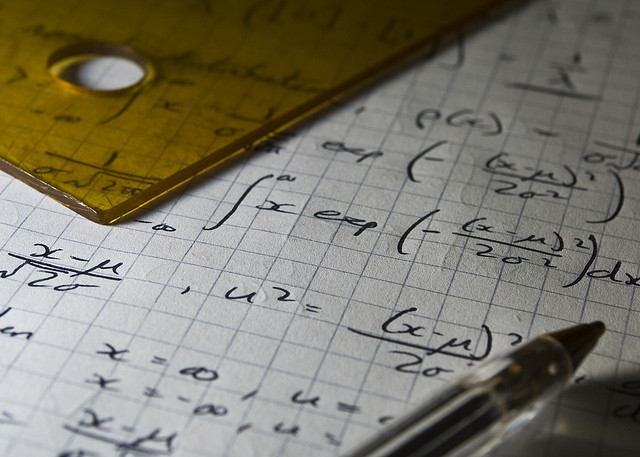
Statistical thinking involves the careful design of a study to collect meaningful data to answer a focused research question, detailed analysis of patterns in the data, and drawing conclusions that go beyond the observed data. Random sampling is paramount to generalizing results from our sample to a larger population, and random assignment is key to drawing cause-and-effect conclusions. With both kinds of randomness, probability models help us assess how much random variation we can expect in our results, in order to determine whether our results could happen by chance alone and to estimate a margin of error.
So where does this leave us with regard to the coffee study mentioned previously (the Freedman, Park, Abnet, Hollenbeck, & Sinha, 2012 found that men who drank at least six cups of coffee a day had a 10% lower chance of dying (women 15% lower) than those who drank none)? We can answer many of the questions:
- This was a 14-year study conducted by researchers at the National Cancer Institute.
- The results were published in the June issue of the New England Journal of Medicine , a respected, peer-reviewed journal.
- The study reviewed coffee habits of more than 402,000 people ages 50 to 71 from six states and two metropolitan areas. Those with cancer, heart disease, and stroke were excluded at the start of the study. Coffee consumption was assessed once at the start of the study.
- About 52,000 people died during the course of the study.
- People who drank between two and five cups of coffee daily showed a lower risk as well, but the amount of reduction increased for those drinking six or more cups.
- The sample sizes were fairly large and so the p-values are quite small, even though percent reduction in risk was not extremely large (dropping from a 12% chance to about 10%–11%).
- Whether coffee was caffeinated or decaffeinated did not appear to affect the results.
- This was an observational study, so no cause-and-effect conclusions can be drawn between coffee drinking and increased longevity, contrary to the impression conveyed by many news headlines about this study. In particular, it’s possible that those with chronic diseases don’t tend to drink coffee.
This study needs to be reviewed in the larger context of similar studies and consistency of results across studies, with the constant caution that this was not a randomized experiment. Whereas a statistical analysis can still “adjust” for other potential confounding variables, we are not yet convinced that researchers have identified them all or completely isolated why this decrease in death risk is evident. Researchers can now take the findings of this study and develop more focused studies that address new questions.
Explore these outside resources to learn more about applied statistics:
- Video about p-values: P-Value Extravaganza
- Interactive web applets for teaching and learning statistics
- Inter-university Consortium for Political and Social Research where you can find and analyze data.
- The Consortium for the Advancement of Undergraduate Statistics
Think It Over
- Find a recent research article in your field and answer the following: What was the primary research question? How were individuals selected to participate in the study? Were summary results provided? How strong is the evidence presented in favor or against the research question? Was random assignment used? Summarize the main conclusions from the study, addressing the issues of statistical significance, statistical confidence, generalizability, and cause and effect. Do you agree with the conclusions drawn from this study, based on the study design and the results presented?
- Is it reasonable to use a random sample of 1,000 individuals to draw conclusions about all U.S. adults? Explain why or why not.
cause-and-effect: related to whether we say one variable is causing changes in the other variable, versus other variables that may be related to these two variables.
generalizability : related to whether the results from the sample can be generalized to a larger population.
margin of error : the expected amount of random variation in a statistic; often defined for 95% confidence level.
population : a larger collection of individuals that we would like to generalize our results to.
p-value : the probability of observing a particular outcome in a sample, or more extreme, under a conjecture about the larger population or process.
random assignment : using a probability-based method to divide a sample into treatment groups.
random sampling : using a probability-based method to select a subset of individuals for the sample from the population.
sample : the collection of individuals on which we collect data.
Licenses and Attributions
CC licensed content, Original
- Modification, adaptation, and original content. Authored by : Pat Carroll and Lumen Learning. Provided by : Lumen Learning. License : CC BY: Attribution
- Statistical Thinking. Authored by : Beth Chance and Allan Rossman, California Polytechnic State University, San Luis Obispo. Provided by : Noba. Located at : http://nobaproject.com/modules/statistical-thinking . License : CC BY-NC-SA: Attribution-NonCommercial-ShareAlike
- The Replication Crisis. Authored by : Colin Thomas William. Provided by : Ivy Tech Community College. License : CC BY: Attribution
If you're seeing this message, it means we're having trouble loading external resources on our website.
If you're behind a web filter, please make sure that the domains *.kastatic.org and *.kasandbox.org are unblocked.
To log in and use all the features of Khan Academy, please enable JavaScript in your browser.
AP®︎/College Statistics
Course: ap®︎/college statistics > unit 6, identifying a sample and population.
- Identify the population and sample
- Generalizability of survey results example
- Generalizability of results
- Types of studies
- Worked example identifying observational study
- Invalid conclusions from studies example
Want to join the conversation?
- Upvote Button navigates to signup page
- Downvote Button navigates to signup page
- Flag Button navigates to signup page

Video transcript
Generalizability and Transferability
In this chapter, we discuss generalizabililty, transferability, and the interrelationship between the two. We also explain how these two aspects of research operate in different methodologies, demonstrating how researchers may apply these concepts throughout the research process.
Generalizability Overview
Generalizability is applied by researchers in an academic setting. It can be defined as the extension of research findings and conclusions from a study conducted on a sample population to the population at large. While the dependability of this extension is not absolute, it is statistically probable. Because sound generalizability requires data on large populations, quantitative research -- experimental for instance -- provides the best foundation for producing broad generalizability. The larger the sample population, the more one can generalize the results. For example, a comprehensive study of the role computers play in the writing process might reveal that it is statistically probable that students who do most of their composing on a computer will move chunks of text around more than students who do not compose on a computer.
Transferability Overview
Transferability is applied by the readers of research. Although generalizability usually applies only to certain types of quantitative methods, transferability can apply in varying degrees to most types of research . Unlike generalizability, transferability does not involve broad claims, but invites readers of research to make connections between elements of a study and their own experience. For instance, teachers at the high school level might selectively apply to their own classrooms results from a study demonstrating that heuristic writing exercises help students at the college level.
Interrelationships
Generalizability and transferability are important elements of any research methodology, but they are not mutually exclusive: generalizability, to varying degrees, rests on the transferability of research findings. It is important for researchers to understand the implications of these twin aspects of research before designing a study. Researchers who intend to make a generalizable claim must carefully examine the variables involved in the study. Among these are the sample of the population used and the mechanisms behind formulating a causal model. Furthermore, if researchers desire to make the results of their study transferable to another context, they must keep a detailed account of the environment surrounding their research, and include a rich description of that environment in their final report. Armed with the knowledge that the sample population was large and varied, as well as with detailed information about the study itself, readers of research can more confidently generalize and transfer the findings to other situations.
Generalizability
Generalizability is not only common to research, but to everyday life as well. In this section, we establish a practical working definition of generalizability as it is applied within and outside of academic research. We also define and consider three different types of generalizability and some of their probable applications. Finally, we discuss some of the possible shortcomings and limitations of generalizability that researchers must be aware of when constructing a study they hope will yield potentially generalizable results.
In many ways, generalizability amounts to nothing more than making predictions based on a recurring experience. If something occurs frequently, we expect that it will continue to do so in the future. Researchers use the same type of reasoning when generalizing about the findings of their studies. Once researchers have collected sufficient data to support a hypothesis, a premise regarding the behavior of that data can be formulated, making it generalizable to similar circumstances. Because of its foundation in probability, however, such a generalization cannot be regarded as conclusive or exhaustive.
While generalizability can occur in informal, nonacademic settings, it is usually applied only to certain research methods in academic studies. Quantitative methods allow some generalizability. Experimental research, for example, often produces generalizable results. However, such experimentation must be rigorous in order for generalizable results to be found.
An example of generalizability in everyday life involves driving. Operating an automobile in traffic requires that drivers make assumptions about the likely outcome of certain actions. When approaching an intersection where one driver is preparing to turn left, the driver going straight through the intersection assumes that the left-turning driver will yield the right of way before turning. The driver passing through the intersection applies this assumption cautiously, recognizing the possibility that the other driver might turn prematurely.
American drivers also generalize that everyone will drive on the right hand side of the road. Yet if we try to generalize this assumption to other settings, such as England, we will be making a potentially disastrous mistake. Thus, it is obvious that generalizing is necessary for forming coherent interpretations in many different situations, but we do not expect our generalizations to operate the same way in every circumstance. With enough evidence we can make predictions about human behavior, yet we must simultaneously recognize that our assumptions are based on statistical probability.
Consider this example of generalizable research in the field of English studies. A study on undergraduate instructor evaluations of composition instructors might reveal that there is a strong correlation between the grade students are expecting to earn in a course and whether they give their instructor high marks. The study might discover that 95% of students who expect to receive a "C" or lower in their class give their instructor a rating of "average" or below. Therefore, there would be a high probability that future students expecting a "C" or lower would not give their instructor high marks. However, the results would not necessarily be conclusive. Some students might defy the trend. In addition, a number of different variables could also influence students' evaluations of an instructor, including instructor experience, class size, and relative interest in a particular subject. These variables -- and others -- would have to be addressed in order for the study to yield potentially valid results. However, even if virtually all variables were isolated, results of the study would not be 100% conclusive. At best, researchers can make educated predictions of future events or behaviors, not guarantee the prediction in every case. Thus, before generalizing, findings must be tested through rigorous experimentation, which enables researchers to confirm or reject the premises governing their data set.
Considerations
There are three types of generalizability that interact to produce probabilistic models. All of them involve generalizing a treatment or measurement to a population outside of the original study. Researchers who wish to generalize their claims should try to apply all three forms to their research, or the strength of their claims will be weakened (Runkel & McGrath, 1972).
In one type of generalizability, researchers determine whether a specific treatment will produce the same results in different circumstances. To do this, they must decide if an aspect within the original environment, a factor beyond the treatment, generated the particular result. This will establish how flexibly the treatment adapts to new situations. Higher adaptability means that the treatment is generalizable to a greater variety of situations. For example, imagine that a new set of heuristic prewriting questions designed to encourage freshman college students to consider audience more fully works so well that the students write thoroughly developed rhetorical analyses of their target audiences. To responsibly generalize that this heuristic is effective, a researcher would need to test the same prewriting exercise in a variety of educational settings at the college level, using different teachers, students, and environments. If the same positive results are produced, the treatment is generalizable.
A second form of generalizability focuses on measurements rather than treatments. For a result to be considered generalizable outside of the test group, it must produce the same results with different forms of measurement. In terms of the heuristic example above, the findings will be more generalizable if the same results are obtained when assessed "with questions having a slightly different wording, or when we use a six-point scale instead of a nine-point scale" (Runkel & McGrath, 1972, p.46).
A third type of generalizability concerns the subjects of the test situation. Although the results of an experiment may be internally valid, that is, applicable to the group tested, in many situations the results cannot be generalized beyond that particular group. Researchers who hope to generalize their results to a larger population should ensure that their test group is relatively large and randomly chosen. However, researchers should consider the fact that test populations of over 10,000 subjects do not significantly increase generalizability (Firestone,1993).
Potential Limitations
No matter how carefully these three forms of generalizability are applied, there is no absolute guarantee that the results obtained in a study will occur in every situation outside the study. In order to determine causal relationships in a test environment, precision is of utmost importance. Yet if researchers wish to generalize their findings, scope and variance must be emphasized over precision. Therefore, it becomes difficult to test for precision and generalizability simultaneously, since a focus on one reduces the reliability of the other. One solution to this problem is to perform a greater number of observations, which has a dual effect: first, it increases the sample population, which heightens generalizability; second, precision can be reasonably maintained because the random errors between observations will average out (Runkel and McGrath, 1972).
Transferability
Transferability describes the process of applying the results of research in one situation to other similar situations. In this section, we establish a practical working definition of transferability as it's applied within and outside of academic research. We also outline important considerations researchers must be aware of in order to make their results potentially transferable, as well as the critical role the reader plays in this process. Finally, we discuss possible shortcomings and limitations of transferability that researchers must be aware of when planning and conducting a study that will yield potentially transferable results.
Transferability is a process performed by readers of research. Readers note the specifics of the research situation and compare them to the specifics of an environment or situation with which they are familiar. If there are enough similarities between the two situations, readers may be able to infer that the results of the research would be the same or similar in their own situation. In other words, they "transfer" the results of a study to another context. To do this effectively, readers need to know as much as possible about the original research situation in order to determine whether it is similar to their own. Therefore, researchers must supply a highly detailed description of their research situation and methods.
Results of any type of research method can be applied to other situations, but transferability is most relevant to qualitative research methods such as ethnography and case studies. Reports based on these research methods are detailed and specific. However, because they often consider only one subject or one group, researchers who conduct such studies seldom generalize the results to other populations. The detailed nature of the results, however, makes them ideal for transferability.
Transferability is easy to understand when you consider that we are constantly applying this concept to aspects of our daily lives. If, for example, you are an inexperienced composition instructor and you read a study in which a veteran writing instructor discovered that extensive prewriting exercises helped students in her classes come up with much more narrowly defined paper topics, you could ask yourself how much the instructor's classroom resembled your own. If there were many similarities, you might try to draw conclusions about how increasing the amount of prewriting your students do would impact their ability to arrive at sufficiently narrow paper topics. In doing so, you would be attempting to transfer the composition researcher's techniques to your own classroom.
An example of transferable research in the field of English studies is Berkenkotter, Huckin, and Ackerman's (1988) study of a graduate student in a rhetoric Ph.D. program. In this case study, the researchers describe in detail a graduate student's entrance into the language community of his academic program, and particularly his struggle learning the writing conventions of this community. They make conclusions as to why certain things might have affected the graduate student, "Nate," in certain ways, but they are unable to generalize their findings to all graduate students in rhetoric Ph.D. programs. It is simply one study of one person in one program. However, from the level of detail the researchers provide, readers can take certain aspects of Nate's experience and apply them to other contexts and situations. This is transferability. First-year graduate students who read the Berkenhotter, Huckin, and Ackerman study may recognize similarities in their own situation while professors may recognize difficulties their students are having and understand these difficulties a bit better. The researchers do not claim that their results apply to other situations. Instead, they report their findings and make suggestions about possible causes for Nate's difficulties and eventual success. Readers then look at their own situation and decide if these causes may or may not be relevant.
When designing a study researchers have to consider their goals: Do they want to provide limited information about a broad group in order to indicate trends or patterns? Or do they want to provide detailed information about one person or small group that might suggest reasons for a particular behavior? The method they choose will determine the extent to which their results can be transferred since transferability is more applicable to certain kinds of research methods than others.
Thick Description: When writing up the results of a study, it is important that the researcher provide specific information about and a detailed description of her subject(s), location, methods, role in the study, etc. This is commonly referred to as "thick description" of methods and findings; it is important because it allows readers to make an informed judgment about whether they can transfer the findings to their own situation. For example, if an educator conducts an ethnography of her writing classroom, and finds that her students' writing improved dramatically after a series of student-teacher writing conferences, she must describe in detail the classroom setting, the students she observed, and her own participation. If the researcher does not provide enough detail, it will be difficult for readers to try the same strategy in their own classrooms. If the researcher fails to mention that she conducted this research in a small, upper-class private school, readers may transfer the results to a large, inner-city public school expecting a similar outcome.
The Reader's Role: The role of readers in transferability is to apply the methods or results of a study to their own situation. In doing so, readers must take into account differences between the situation outlined by the researcher and their own. If readers of the Berkenhotter, Huckin, and Ackerman study are aware that the research was conducted in a small, upper-class private school, but decide to test the method in a large inner-city public school, they must make adjustments for the different setting and be prepared for different results.
Likewise, readers may decide that the results of a study are not transferable to their own situation. For example, if a study found that watching more than 30 hours of television a week resulted in a worse GPA for graduate students in physics, graduate students in broadcast journalism may conclude that these results do not apply to them.
Readers may also transfer only certain aspects of the study and not the entire conclusion. For example, in the Berkenhotter, Huckin, and Ackerman study, the researchers suggest a variety of reasons for why the graduate student studied experienced difficulties adjusting to his Ph.D. program. Although composition instructors cannot compare "Nate" to first-year college students in their composition class, they could ask some of the same questions about their own class, offering them insight into some of the writing difficulties the first-year undergraduates are experiencing. It is up to readers to decide what findings are important and which may apply to their own situation; if researchers fulfill their responsibility to provide "thick description," this decision is much easier to make.
Understanding research results can help us understand why and how something happens. However, many researchers believe that such understanding is difficult to achieve in relation to human behaviors which they contend are too difficult to understand and often impossible to predict. "Because of the many and varied ways in which individuals differ from each other and because these differences change over time, comprehensive and definitive experiments in the social sciences are not possible...the most we can ever realistically hope to achieve in educational research is not prediction and control but rather only temporary understanding" (Cziko, 1993, p. 10).
Cziko's point is important because transferability allows for "temporary understanding." Instead of applying research results to every situation that may occur in the future, we can apply a similar method to another, similar situation, observe the new results, apply a modified version to another situation, and so on. Transferability takes into account the fact that there are no absolute answers to given situations; rather, every individual must determine their own best practices. Transferring the results of research performed by others can help us develop and modify these practices. However, it is important for readers of research to be aware that results cannot always be transferred; a result that occurs in one situation will not necessarily occur in a similar situation. Therefore, it is critical to take into account differences between situations and modify the research process accordingly.
Although transferability seems to be an obvious, natural, and important method for applying research results and conclusions, it is not perceived as a valid research approach in some academic circles. Perhaps partly in response to critics, in many modern research articles, researchers refer to their results as generalizable or externally valid. Therefore, it seems as though they are not talking about transferability. However, in many cases those same researchers provide direction about what points readers may want to consider, but hesitate to make any broad conclusions or statements. These are characteristics of transferable results.
Generalizability is actually, as we have seen, quite different from transferability. Unfortunately, confusion surrounding these two terms can lead to misinterpretation of research results. Emphasis on the value of transferable results -- as well as a clear understanding among researchers in the field of English of critical differences between the conditions under which research can be generalized, transferred, or, in some cases, both generalized and transferred -- could help qualitative researchers avoid some of the criticisms launched by skeptics who question the value of qualitative research methods.
Generalizability and Transferability: Synthesis
Generalizability allows us to form coherent interpretations in any situation, and to act purposefully and effectively in daily life. Transferability gives us the opportunity to sort through given methods and conclusions to decide what to apply to our own circumstances. In essence, then, both generalizability and transferability allow us to make comparisons between situations. For example, we can generalize that most people in the United States will drive on the right side of the road, but we cannot transfer this conclusion to England or Australia without finding ourselves in a treacherous situation. It is important, therefore, to always consider context when generalizing or transferring results.
Whether a study emphasizes transferability or generalizability is closely related to the goals of the researcher and the needs of the audience. Studies done for a magazine such as Time or a daily newspaper tend towards generalizability, since the publishers want to provide information relevant to a large portion of the population. A research project pointed toward a small group of specialists studying a similar problem may emphasize transferability, since specialists in the field have the ability to transfer aspects of the study results to their own situations without overt generalizations provided by the researcher. Ultimately, the researcher's subject, audience, and goals will determine the method the researcher uses to perform a study, which will then determine the transferability or generalizability of the results.
A Comparison of Generalizability and Transferability
Although generalizability has been a preferred method of research for quite some time, transferability is relatively a new idea. In theory, however, it has always accompanied research issues. It is important to note that generalizability and transferability are not necessarily mutually exclusive; they can overlap.
From an experimental study to a case study, readers transfer the methods, results, and ideas from the research to their own context. Therefore, a generalizable study can also be transferable. For example, a researcher may generalize the results of a survey of 350 people in a university to the university population as a whole; readers of the results may apply, or transfer, the results to their own situation. They will ask themselves, basically, if they fall into the majority or not. However, a transferable study is not always generalizable. For example, in case studies , transferability allows readers the option of applying results to outside contexts, whereas generalizability is basically impossible because one person or a small group of people is not necessarily representative of the larger population.
Controversy, Worth, and Function
Research in the natural sciences has a long tradition of valuing empirical studies; experimental investigation has been considered "the" way to perform research. As social scientists adapted the methods of natural science research to their own needs, they adopted this preference for empirical research. Therefore, studies that are generalizable have long been thought to be more worthwhile; the value of research was often determined by whether a study was generalizable to a population as a whole. However, more and more social scientists are realizing the value of using a variety of methods of inquiry, and the value of transferability is being recognized.
It is important to recognize that generalizability and transferability do not alone determine a study's worth. They perform different functions in research, depending on the topic and goals of the researcher. Where generalizable studies often indicate phenomena that apply to broad categories such as gender or age, transferability can provide some of the how and why behind these results.
However, there are weaknesses that must be considered. Researchers can study a small group that is representative of a larger group and claim that it is likely that their results are applicable to the larger group, but it is impossible for them to test every single person in the larger group. Their conclusions, therefore, are only valid in relation to their own studies. Another problem is that a non-representative group can lead to a faulty generalization. For example, a study of composition students'; revision capabilities which compared students' progress made during a semester in a computer classroom with progress exhibited by students in a traditional classroom might show that computers do aid students in the overall composing process. However, if it were discovered later that an unusually high number of students in the traditional classrooms suffered from substance abuse problems outside of the classroom, the population studied would not be considered representative of the student population as a whole. Therefore, it would be problematic to generalize the results of the study to a larger student population.
In the case of transferability, readers need to know as much detail as possible about a research situation in order to accurately transfer the results to their own. However, it is impossible to provide an absolutely complete description of a situation, and missing details may lead a reader to transfer results to a situation that is not entirely similar to the original one.
Applications to Research Methods
The degree to which generalizability and transferability are applicable differs from methodology to methodology as well as from study to study. Researchers need to be aware of these degrees so that results are not undermined by over-generalizations, and readers need to ensure that they do not read researched results in such a way that the results are misapplied or misinterpreted.
Applications of Transferability and Generalizability: Case Study
Research Design Case studies examine individuals or small groups within a specific context. Research is typically gathered through qualitative means: interviews, observations, etc. Data is usually analyzed either holistically or by coding methods.
Assumptions In research involving case studies, a researcher typically assumes that the results will be transferable. Generalizing is difficult or impossible because one person or small group cannot represent all similar groups or situations. For example, one group of beginning writing students in a particular classroom cannot represent all beginning student writers. Also, conclusions drawn in case studies are only about the participants being observed. With rare exceptions, case studies are not meant to establish cause/effect relationships between variables. The results of a case study are transferable in that researchers "suggest further questions, hypotheses, and future implications," and present the results as "directions and questions" (Lauer & Asher 32).
Example In order to illustrate the writing skills of beginning college writers, a researcher completing a case study might single out one or more students in a composition classroom and set about talking to them about how they judge their own writing as well as reading actual papers, setting up criteria for judgment, and reviewing paper grades/teacher interpretation.
Results of a Study In presenting the results of the previous example, a researcher should define the criteria that were established in order to determine what the researcher meant by "writing skills," provide noteworthy quotes from student interviews, provide other information depending on the kinds of research methods used (e.g., surveys, classroom observation, collected writing samples), and include possibilities for furthering this type of research. Readers are then able to assess for themselves how the researcher's observations might be transferable to other writing classrooms.
Applications of Transferability and Generalizability: Ethnography
Research Design Ethnographies study groups and/or cultures over a period of time. The goal of this type of research is to comprehend the particular group/culture through observer immersion into the culture or group. Research is completed through various methods, which are similar to those of case studies, but since the researcher is immersed within the group for an extended period of time, more detailed information is usually collected during the research. (Jonathon Kozol's "There Are No Children Here" is a good example of this.)
Assumptions As with case studies, findings of ethnographies are also considered to be transferable. The main goals of an ethnography are to "identify, operationally define, and interrelate variables" within a particular context, which ultimately produce detailed accounts or "thick descriptions" (Lauer & Asher 39). Unlike a case study, the researcher here discovers many more details. Results of ethnographies should "suggest variables for further investigation" and not generalize beyond the participants of a study (Lauer & Asher 43). Also, since analysts completing this type of research tend to rely on multiple methods to collect information (a practice also referred to as triangulation), their results typically help create a detailed description of human behavior within a particular environment.
Example The Iowa Writing Program has a widespread reputation for producing excellent writers. In order to begin to understand their training, an ethnographer might observe students throughout their degree program. During this time, the ethnographer could examine the curriculum, follow the writing processes of individual writers, and become acquainted with the writers and their work. By the end of a two year study, the researcher would have a much deeper understanding of the unique and effective features of the program.
Results of a Study Obviously, the Iowa Writing Program is unique, so generalizing any results to another writing program would be problematic. However, an ethnography would provide readers with insights into the program. Readers could ask questions such as: what qualities make it strong and what is unique about the writers who are trained within the program? At this point, readers could attempt to "transfer" applicable knowledge and observations to other writing environments.
Applications of Transferability and Generalizability: Experimental Research
Research Design A researcher working within this methodology creates an environment in which to observe and interpret the results of a research question. A key element in experimental research is that participants in a study are randomly assigned to groups. In an attempt to create a causal model (i.e., to discover the causal origin of a particular phenomenon), groups are treated differently and measurements are conducted to determine if different treatments appear to lead to different effects.
Assumptions Experimental research is usually thought to be generalizable. This methodology explores cause/effect relationships through comparisons among groups (Lauer & Asher 152). Since participants are randomly assigned to groups, and since most experiments involve enough individuals to reasonably approximate the populations from which individual participants are drawn, generalization is justified because "over a large number of allocations, all the groups of subjects will be expected to be identical on all variables" (155).
Example A simplified example: Six composition classrooms are randomly chosen (as are the students and instructors) in which three instructors incorporate the use of electronic mail as a class activity and three do not. When students in the first three classes begin discussing their papers through e-mail and, as a result, make better revisions to their papers than students in the other three classes, a researcher is likely to conclude that incorporating e-mail within a writing classroom improves the quality of students' writing.
Results of a Study Although experimental research is based on cause/effect relationships, "certainty" can never be obtained, but rather results are "probabilistic" (Lauer and Asher 161). Depending on how the researcher has presented the results, they are generalizable in that the students were selected randomly. Since the quality of writing improved with the use of e-mail within all three classrooms, it is probable that e-mail is the cause of the improvement. Readers of this study would transfer the results when they sorted out the details: Are these students representative of a group of students with which the reader is familiar? What types of previous writing experiences have these students had? What kind of writing was expected from these students? The researcher must have provided these details in order for the results to be transferable.
Applications of Transferability and Generalizability: Survey
Research Design The goal of a survey is to gain specific information about either a specific group or a representative sample of a particular group. Survey respondents are asked to respond to one or more of the following kinds of items: open-ended questions, true-false questions, agree-disagree (or Likert) questions, rankings, ratings, and so on. Results are typically used to understand the attitudes, beliefs, or knowledge of a particular group.
Assumptions Assuming that care has been taken in the development of the survey items and selection of the survey sample and that adequate response rates have been achieved, surveys results are generalizable. Note, however, that results from surveys should be generalized only to the population from which the survey results were drawn.
Example For instance, a survey of Colorado State University English graduate students undertaken to determine how well French philosopher/critic Jacques Derrida is understood before and after students take a course in critical literary theory might inform professors that, overall, Derrida's concepts are understood and that CSU's literary theory class, E615, has helped students grasp Derrida's ideas.
Results of a Study The generalizability of surveys depends on several factors. Whether distributed to a mass of people or a select few, surveys are of a "personal nature and subject to distortion." Survey respondents may or may not understand the questions being asked of them. Depending on whether or not the survey designer is nearby, respondents may or may not have the opportunity to clarify their misunderstandings.
It is also important to keep in mind that errors can occur at the development and processing levels. A researcher may inadequately pose questions (that is, not ask the right questions for the information being sought), disrupt the data collection (surveying certain people and not others), and distort the results during the processing (misreading responses and not being able to question the participant, etc.). One way to avoid these kinds of errors is for researchers to examine other studies of a similar nature and compare their results with results that have been obtained in previous studies. This way, any large discrepancies will be exposed. Depending on how large those discrepancies are and what the context of the survey is, the results may or may not be generalizable. For example, if an improved understanding of Derrida is apparent after students complete E615, it can be theorized that E615 effectively teaches students the concepts of Derrida. Issues of transferability might be visible in the actual survey questions themselves; that is, they could provide critical background information readers might need to know in order to transfer the results to another context.
The Qualitative versus Quantitative Debate
In Miles and Huberman's 1994 book Qualitative Data Analysis , quantitative researcher Fred Kerlinger is quoted as saying, "There's no such thing as qualitative data. Everything is either 1 or 0" (p. 40). To this another researcher, D. T. Campbell, asserts "all research ultimately has a qualitative grounding" (p. 40). This back and forth banter among qualitative and quantitative researchers is "essentially unproductive" according to Miles and Huberman. They and many other researchers agree that these two research methods need each other more often than not. However, because typically qualitative data involves words and quantitative data involves numbers, there are some researchers who feel that one is better (or more scientific) than the other. Another major difference between the two is that qualitative research is inductive and quantitative research is deductive. In qualitative research, a hypothesis is not needed to begin research. However, all quantitative research requires a hypothesis before research can begin.
Another major difference between qualitative and quantitative research is the underlying assumptions about the role of the researcher. In quantitative research, the researcher is ideally an objective observer that neither participates in nor influences what is being studied. In qualitative research, however, it is thought that the researcher can learn the most about a situation by participating and/or being immersed in it. These basic underlying assumptions of both methodologies guide and sequence the types of data collection methods employed.
Although there are clear differences between qualitative and quantitative approaches, some researchers maintain that the choice between using qualitative or quantitative approaches actually has less to do with methodologies than it does with positioning oneself within a particular discipline or research tradition. The difficulty of choosing a method is compounded by the fact that research is often affiliated with universities and other institutions. The findings of research projects often guide important decisions about specific practices and policies. The choice of which approach to use may reflect the interests of those conducting or benefitting from the research and the purposes for which the findings will be applied. Decisions about which kind of research method to use may also be based on the researcher's own experience and preference, the population being researched, the proposed audience for findings, time, money, and other resources available (Hathaway, 1995).
Some researchers believe that qualitative and quantitative methodologies cannot be combined because the assumptions underlying each tradition are so vastly different. Other researchers think they can be used in combination only by alternating between methods: qualitative research is appropriate to answer certain kinds of questions in certain conditions and quantitative is right for others. And some researchers think that both qualitative and quantitative methods can be used simultaneously to answer a research question.
To a certain extent, researchers on all sides of the debate are correct: each approach has its drawbacks. Quantitative research often "forces" responses or people into categories that might not "fit" in order to make meaning. Qualitative research, on the other hand, sometimes focuses too closely on individual results and fails to make connections to larger situations or possible causes of the results. Rather than discounting either approach for its drawbacks, though, researchers should find the most effective ways to incorporate elements of both to ensure that their studies are as accurate and thorough as possible.
It is important for researchers to realize that qualitative and quantitative methods can be used in conjunction with each other. In a study of computer-assisted writing classrooms, Snyder (1995) employed both qualitative and quantitative approaches. The study was constructed according to guidelines for quantitative studies: the computer classroom was the "treatment" group and the traditional pen and paper classroom was the "control" group. Both classes contained subjects with the same characteristics from the population sampled. Both classes followed the same lesson plan and were taught by the same teacher in the same semester. The only variable used was the computers. Although Snyder set this study up as an "experiment," she used many qualitative approaches to supplement her findings. She observed both classrooms on a regular basis as a participant-observer and conducted several interviews with the teacher both during and after the semester. However, there were several problems in using this approach: the strict adherence to the same syllabus and lesson plans for both classes and the restricted access of the control group to the computers may have put some students at a disadvantage. Snyder also notes that in retrospect she should have used case studies of the students to further develop her findings. Although her study had certain flaws, Snyder insists that researchers can simultaneously employ qualitative and quantitative methods if studies are planned carefully and carried out conscientiously.
Annotated Bibliography
Babbie, Earl R. (1979). The practice of social research . Belmont: Wadsworth Publishing Company, Inc.
A comprehensive review of social scientific research, including techniques for research. The logic behind social scientific research is discussed.
Berkenkotter, C., Huckin, T.N., & Ackerman, J. (1988). Conventions, conversations, and the writer: Case study of a student in a rhetoric Ph.D. program. Research in the Teaching of English 22 (1), 9-44.
Describes a case study of a beginning student in a Ph.D. program. Looks at the process of his entry into an academic discourse community.
Black, Susan. (1996). Redefining the teacher's role. Executive Educator,18 (8), 23-26.
Discusses the value of well-trained teacher-researchers performing research in their classrooms. Notes that teacher-research focuses on the particular; it does not look for broad, generalizable principles.
Blank, Steven C. (1984). Practical business research methods . Westport: AVI Publishing Company, Inc.
A comprehensive book of how to set up a research project, collect data, and reach and report conclusions.
Bridges, David. (1993). Transferable Skills: A Philosophical Perspective. Studies in Higher Education 18 (1), 43-51.
Transferability of skills in learning is discussed, focusing on the notions of cross-disciplinary, generic, core, and transferable skills and their role in the college curriculum.
Brookhart, Susan M. & Rusnak, Timothy G. (1993). A pedagogy of enrichment, not poverty: Successful lessons of exemplary urban teachers. Journal of Teacher Education, 44 (1), 17-27.
Reports the results of a study that explored the characteristics of effective urban teachers in Pittsburgh. Suggests that the results may be transferable to urban educators in other contexts.
Bryman, Alan. (1988). Quantity and quality in social research . Boston: Unwin Hyman Ltd.
Butcher, Jude. (1994, July). Cohort and case study components in teacher education research. Paper presented at the annual conference of the Australian Teacher Education Association, Brisbane, Queensland, Australia.
Argues that studies of teacher development will be more generalizable if a broad set of methods are used to collect data, if the data collected is both extensive and intensive, and if the methods used take into account the differences in people and situations being studied.
Carter, Duncan. (1993). Critical thinking for writers: Transferable skills or discipline-specific strategies? Composition Studies/Freshman English News, 21 (1), 86-93.
Questions the context-dependency of critical thinking, and whether critical thinking skills are transferable to writing tasks.
Carter, Kathy. (1993). The place of story in the study of teaching and teacher education. Educational Researcher, 22 (1), 5-12.
Discusses the advantages of story-telling in teaching and teacher education, but cautions instructors, who are currently unfamiliar with story-telling in current pedagogical structures, to be careful in implementing this method in their teaching.
Clonts, Jean G. (1992, January). The concept of reliability as it pertains to data from qualitative studies. Paper presented at the annual meeting of the Southwest Educational Research Association, Houston, TX.
Presents a review of literature on reliability in qualitative studies and defines reliability as the extent to which studies can be replicated by using the same methods and getting the same results. Strategies to enhance reliability through study design, data collection, and data analysis are suggested. Generalizability as an estimate of reliability is also explored.
Connelly, Michael F. & Clandinin D. Jean. (1990). Stories of experience and narrative inquiry. Educational Researcher, 19. (5), 2-14.
Describes narrative as a site of inquiry and a qualitative research methodology in which experiences of observer and observed interact. This form of research necessitates the development of new criteria, which may include apparency, verisimilitude, and transferability (7).
Crocker, Linda & Algina, James. (1986). Introduction to classical & modern test theory. New York: Holt, Rinehart and Winston.
Discusses test theory and its application to psychometrics. Chapters range from general overview of major issues to statistical methods and application.
Cronbach, Lee J. et al. (1967). The dependability of behavioral measurements: multifaceted studies of generalizability. Stanford: Stanford UP.
A technical research report that includes statistical methodology in order to contrast multifaceted generalizability with classical reliability.
Cziko, Gary A. (1992). Purposeful behavior as the control of perception: implications for educational research. Educational Researcher, 21 (9), 10-18. El-Hassan, Karma. (1995). Students' Rating of Instruction: Generalizability of Findings. Studies in Educational Research 21 (4), 411-29.
Issues of dimensionality, validity, reliability, and generalizability of students' ratings of instruction are discussed in relation to a study in which 610 college students who evaluated their instructors on the Teacher Effectiveness Scale.
Feingold, Alan. (1994). Gender differences in variability in intellectual abilities: a cross-cultural perspective. Sex Roles: A Journal of Research 20 (1-2), 81-93.
Feingold conducts a cross-cultural quantitative review of contemporary findings of gender differences in variability in verbal, mathematical, and spatial abilities to assess the generalizability of U.S. findings that males are more variable than females in mathematical and spatial abilities, and the sexes are equally variable in verbal ability.
Firestone,William A. (1993). Alternative arguments for generalizing from data as applied to qualitative research. Educational Researcher, 22 (4), 16-22.
Focuses on generalization in three areas of qualitative research: sample to population extrapolation, analytic generalization, and case-to-case transfer (16). Explains underlying principles, related theories, and criteria for each approach.
Fyans, Leslie J. (Ed.). (1983). Generalizability theory: Inferences and practical applications. In New Directions for Testing and Measurement: Vol. 18. San Francisco: Jossey-Bass.
A collection of articles on generalizability theory. The goal of the book is to present different aspects and applications of generalizability theory in a way that allows the reader to apply the theory.
Hammersley, Martyn. (Ed.). (1993). Social research: Philosophy, politics and practice. Newbury Park, CA: Sage Publications.
A collection of articles that provide an overview of positivism; includes an article on increasing the generalizability of qualitative research by Janet Ward Schofield.
Hathaway, R. (1995). Assumptions underlying quantitative and qualitative research: Implications for institutional research. Research in higher education, 36 (5), 535-562.
Hathaway says that the choice between using qualitative or quantitative approaches is less about methodology and more about aligning oneself with particular theoretical and academic traditions. He concluded that the two approaches address questions in very different ways, each one having its own advantages and drawbacks.
Heck, Ronald H., Marcoulides, George A. (1996). . Research in the Teaching of English 22 (1), 9-44.
Hipps, Jerome A. (1993). Trustworthiness and authenticity: Alternate ways to judge authentic assessments. Paper presented at the annual meeting of the American Educational Research Association, Atlanta, GA.
Contrasts the foundational assumptions of the constructivist approach to traditional research and the positivist approach to authentic assessment in relation to generalizability and other research issues.
Howe, Kenneth & Eisenhart, Margaret. (1990). Standards for qualitative (and quantitative) research: A prolegomenon. Educational Researcher, 19 (4), 2-9.
Huang, Chi-yu, et al. (1995, April). A generalizability theory approach to examining teaching evaluation instruments completed by students. Paper presented at the annual meeting of the American Educational Research Association, San Francisco, CA.
Presents the results of a study that used generalizability theory to investigate the reasons for variability in a teacher and course evaluation mechanism.
Hungerford, Harold R. et al. (1992). Investigating and Evaluating Environmental Issues and Actions: Skill Development Modules .
A guide designed to teach students how to investigate and evaluate environmental issues and actions. The guide is presented in six modules including information collecting and surveys, questionnaires, and opinionnaires.
Jackson, Philip W. (1990). The functions of educational research. Educational Researcher 19 (7), 3-9. Johnson, Randell G. (1993, April). A validity generalization study of the multiple assessment and program services test. Paper presented at the annual meeting of the American Educational Research Association, Atlanta, GA.
Presents results of study of validity reports of the Multiple Assessment and Program Services Test using quantitative analysis to determine the generalizability of the results.
Jones, Elizabeth A & Ratcliff, Gary. (1993). Critical thinking skills for college students. (National Center on Postsecondary Teaching, Learning, and Asessment). University Park, PA.
Reviews research literature exploring the nature of critical thinking; discusses the extent to which critical thinking is generalizable across disciplines.
Karpinski, Jakub. (1990). Causality in Sociological Research . Boston: Kluwer Academic Publishers.
Discusses causality and causal analysis in terms of sociological research. Provides equations and explanations.
Kirsch, Irwin S. & Jungeblut, Ann. (1995). Using large-scale assessment results to identify and evaluate generalizable indicators of literacy. (National Center on Adult Literacy, Publication No. TR94-19). Philadelphia, PA.
Reports analysis of data collected during an extensive literacy survey in order to help understand the different variables involved in literacy proficiency. Finds that literacy skills can be predicted across large, heterogeneous populations, but not as effectively across homogeneous populations.
Lauer, Janice M. & Asher, J. William. (1988). Composition research: empirical designs. New York: Oxford Press.
Explains the selection of subjects, formulation of hypotheses or questions, data collection, data analysis, and variable identification through discussion of each design.
LeCompte, Margaret & Goetz, Judith Preissle. (1982). Problems of reliability and validity in ethnographic research. Review of Educational Research, 52 (1), 31-60.
Concentrates on educational research and ethnography and shows how to better take reliability and validity into account when doing ethnographic research.
Marcoulides, George; Simkin, Mark G. (1991). Evaluating student papers: the case for peer review. Journal of Education for Business 67 (2), 80-83.
A preprinted evaluation form and generalizability theory are used to judge the reliability of student grading of their papers.
Maxwell, Joseph A. (1992). Understanding and validity in qualitative research. Harvard Educational Review, 62 (3), 279-300.
Explores the five types of validity used in qualitative research, including generalizable validity, and examines possible threats to research validity.
McCarthy, Christine L. (1996, Spring). What is "critical thinking"? Is it generalizable? Educational Theory, 46 217-239.
Reviews, compares and contrasts a selection of essays from Stephen P. Norris' book The Generalizability of Critical Thinking: Multiple Perspectives on an Education Ideal in order to explore the diversity of the topic of critical thinking.
Miles, Matthew B. & Huberman, A. Michael. (1994). Qualitative data analysis. Thousand Oaks: Sage Publications.
A comprehensive review of data analysis. Subjects range from collecting data to producing an actual report.
Minium, Edward W. & King, M. Bruce, & Bear, Gordon. (1993). Statistical reasoning in psychology and education . New York: John Wiley & Sons, Inc.
A textbook designed to teach students about statistical data and theory.
Moss, Pamela A. (1992). Shifting conceptions of validity in educational measurement: Implications for performance assessment. Review of Educational Research, 62 (3), 229-258. Nachmias, David & Nachmias, Chava . (1981). Research methods in the social sciences. New York: St. Martin's Press.
Discusses the foundations of empirical research, data collection, data processing and analysis, inferential methods, and the ethics of social science research.
Nagy, Philip; Jarchow, Elaine McNally. (1981). Estimating variance components of essay ratings in a complex design. Speech/Conference Paper .
This paper discusses variables influencing written composition quality and how they can be best controlled to improve the reliability assessment of writing ability.
Nagy, William E., Herman, Patricia A., & Anderson, Richard C. (1985). Learning word meanings from context: How broadly generalizable? (University of Illinois at Urbana-Champaign. Center for the Study of Reading, Technical Report No. 347). Cambridge, MA: Bolt, Beranek and Newman.
Reports the results of a study that investigated how students learn word meanings while reading from context. Claims that the study was designed to be generalized.
Naizer, Gilbert. (1992, January). Basic concepts in generalizability theory: A more powerful approach to evaluating reliability. Presented at the annual meeting of the Southwest Educational Research Association, Houston, TX.
Discusses how a measurement approach called generalizability theory (G-theory) is an important alternative to the more classical measurement theory that yields less useful coefficients. G-theory is about the dependability of behavioral measurements that allows the simultaneous estimation of multiple sources of error variance.
Newman, Isadore & Macdonald, Suzanne. (1993, May). Interpreting qualitative data: A methodological inquiry. Paper presented at the annual meeting of the Ohio Academy of Science, Youngstown, OH.
Issues of consistency, triangulation, and generalizability are discussed in relation to a qualitative study involving graduate student participants. The authors refute Polkinghorne's views of the generalizability of qualitative research, arguing that quantitative research is more suitable for generalizability.
Norris, Stephen P. (Ed.). (1992). The generalizability of critical thinking: multiple perspectives on an education ideal. New York: Teachers College Press. A set of essays from a variety of disciplines presenting different perspectives on the topic of the generalizability of critical thinking. The authors refer and respond to each other. Peshkin, Alan. (1993). The goodness of qualitative research. Educational Researcher, 22 (2), 23-29.
Discusses how effective qualitative research can be in obtaining desired results and concludes that it is an important tool scholars can use in their explorations. The four categories of qualitative research--description, interpretation, verification, and evaluation--are examined.
Rafilson, Fred. (1991, July). The case for validity generalization.
Describes generalization as a quantitative process. Briefly discusses theory, method, examples, and applications of validity generalization, emphasizing unseen local methodological problems.
Rhodebeck, Laurie A. The structure of men's and women's feminist orientations: feminist identity and feminist opinion. Gender & Society 10 (4), 386-404.
This study considers two problems: the extent to which feminist opinions are distinct from feminist identity and the generalizability of these separate constructs across gender and time.
Runkel, Philip J. & McGrath, E. Joseph. (1972). Research on human behavior: A systematic guide to method. New York: Holt, Rinehart and Winston, Inc.
Discusses how researchers can utilize their experiences of human behavior and apply them to research in a systematic and explicit fashion.
Salomon, Gavriel. (1991). Transcending the qualitative-quantitative debate: The analytic and systemic approaches to educational research. Educational Researcher, 20 (6), 10-18.
Examines the complex issues/variables involved in studies. Two types of approaches are explored: an Analytic Approach, which assumes internal and external issues, and a Systematic Approach, in which each component affects the whole. Also discusses how a study can never fully measure how much x affects y because there are so many inter-relations. Knowledge is applied differently within each approach.
Schrag, Francis. (1992). In defense of positivist research paradigms. Educational Researcher, 21 (5), 5-8.
Positivist critics Elliot Eisner, Fredrick Erikson, Henry Giroux, and Thomas Popkewitz are logically committed to propositions that can be tested only by means of positivist research paradigms. A definition of positivism is gathered through example. Overall, it is concluded that educational research need not aspire to be practical.
Sekaran, Uma. (1984). Research methods for managers: A skill-building approach. New York: John Wiley and Sons.
Discusses managerial approaches to conducting research in organizations. Provides understandable definitions and explanations of such methods as sampling and data analysis and interpretation.
Shadish, William R. (1995). The logic of generalization: five principles common to experiments and ethnographies. American Journal of Community Psychology 23 (3), 419-29.
Both experiments and ethnographies are highly localized, so they are often criticized for lack of generalizability. This article describes a logic of generalization that may help solve such problems.
Shavelson, Richard J. & Webb, Noreen M. (1991). Generalizability theory: A primer. Newbury Park, CA: Sage Publications.
Snyder, I. (1995). Multiple perspectives in literacy research: Integrating the quantitative and qualitative. Language and Education, 9 (1), 45-59.
This article explains a study in which the author employed quantitative and qualitative methods simultaneously to compare computer composition classrooms and traditional classrooms. Although there were some problems with integrating both approaches, Snyder says they can be used together if researchers plan carefully and use their methods thoughtfully.
Stallings, William M. (1995). Confessions of a quantitative educational researcher trying to teach qualitative research. Educational Researcher, 24 (3), 31-32.
Discusses the trials and tribulations of teaching a qualitative research course to graduate students. The author describes the successes and failings he encounters and asks colleagues for suggestions of readings for his syllabus.
Wagner, Ellen D. (1993, January). Evaluating distance learning projects: An approach for cross-project comparisons. Paper presented at the annual meeting of the Association for educational Communication and Technology, New Orleans, LA.
Describes a methodology developed to evaluate distance learning projects in a way that takes into account specific institutional issues while producing generalizable, valid and reliable results that allow for discussion among different institutions.
Yin, Robert K. (1989). Case Study Research: Design and Methods. London: Sage Publications.
A small section on the application of generalizability in regards to case studies.
Citation Information
Jeffrey Barnes, Kerri Conrad, Christof Demont-Heinrich, Mary Graziano, Dawn Kowalski, Jamie Neufeld, Jen Zamora, and Mike Palmquist. (1994-2024). Generalizability and Transferability. The WAC Clearinghouse. Colorado State University. Available at https://wac.colostate.edu/repository/writing/guides/.
Copyright Information
Copyright © 1994-2024 Colorado State University and/or this site's authors, developers, and contributors . Some material displayed on this site is used with permission.
When you choose to publish with PLOS, your research makes an impact. Make your work accessible to all, without restrictions, and accelerate scientific discovery with options like preprints and published peer review that make your work more Open.
- PLOS Biology
- PLOS Climate
- PLOS Complex Systems
- PLOS Computational Biology
- PLOS Digital Health
- PLOS Genetics
- PLOS Global Public Health
- PLOS Medicine
- PLOS Mental Health
- PLOS Neglected Tropical Diseases
- PLOS Pathogens
- PLOS Sustainability and Transformation
- PLOS Collections
- How to Write Discussions and Conclusions

The discussion section contains the results and outcomes of a study. An effective discussion informs readers what can be learned from your experiment and provides context for the results.
What makes an effective discussion?
When you’re ready to write your discussion, you’ve already introduced the purpose of your study and provided an in-depth description of the methodology. The discussion informs readers about the larger implications of your study based on the results. Highlighting these implications while not overstating the findings can be challenging, especially when you’re submitting to a journal that selects articles based on novelty or potential impact. Regardless of what journal you are submitting to, the discussion section always serves the same purpose: concluding what your study results actually mean.
A successful discussion section puts your findings in context. It should include:
- the results of your research,
- a discussion of related research, and
- a comparison between your results and initial hypothesis.
Tip: Not all journals share the same naming conventions.
You can apply the advice in this article to the conclusion, results or discussion sections of your manuscript.
Our Early Career Researcher community tells us that the conclusion is often considered the most difficult aspect of a manuscript to write. To help, this guide provides questions to ask yourself, a basic structure to model your discussion off of and examples from published manuscripts.

Questions to ask yourself:
- Was my hypothesis correct?
- If my hypothesis is partially correct or entirely different, what can be learned from the results?
- How do the conclusions reshape or add onto the existing knowledge in the field? What does previous research say about the topic?
- Why are the results important or relevant to your audience? Do they add further evidence to a scientific consensus or disprove prior studies?
- How can future research build on these observations? What are the key experiments that must be done?
- What is the “take-home” message you want your reader to leave with?
How to structure a discussion
Trying to fit a complete discussion into a single paragraph can add unnecessary stress to the writing process. If possible, you’ll want to give yourself two or three paragraphs to give the reader a comprehensive understanding of your study as a whole. Here’s one way to structure an effective discussion:
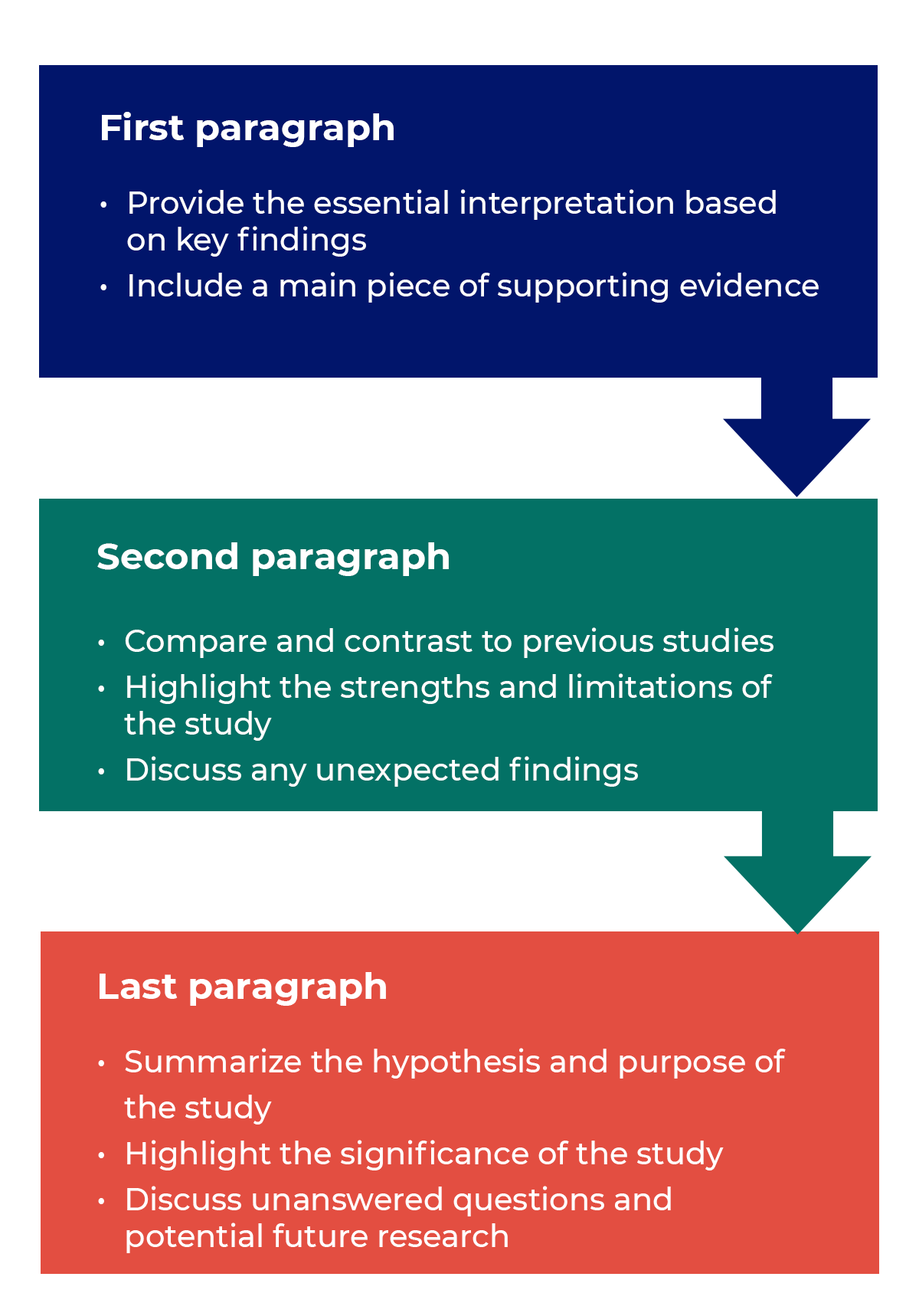
Writing Tips
While the above sections can help you brainstorm and structure your discussion, there are many common mistakes that writers revert to when having difficulties with their paper. Writing a discussion can be a delicate balance between summarizing your results, providing proper context for your research and avoiding introducing new information. Remember that your paper should be both confident and honest about the results!

- Read the journal’s guidelines on the discussion and conclusion sections. If possible, learn about the guidelines before writing the discussion to ensure you’re writing to meet their expectations.
- Begin with a clear statement of the principal findings. This will reinforce the main take-away for the reader and set up the rest of the discussion.
- Explain why the outcomes of your study are important to the reader. Discuss the implications of your findings realistically based on previous literature, highlighting both the strengths and limitations of the research.
- State whether the results prove or disprove your hypothesis. If your hypothesis was disproved, what might be the reasons?
- Introduce new or expanded ways to think about the research question. Indicate what next steps can be taken to further pursue any unresolved questions.
- If dealing with a contemporary or ongoing problem, such as climate change, discuss possible consequences if the problem is avoided.
- Be concise. Adding unnecessary detail can distract from the main findings.
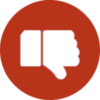
Don’t
- Rewrite your abstract. Statements with “we investigated” or “we studied” generally do not belong in the discussion.
- Include new arguments or evidence not previously discussed. Necessary information and evidence should be introduced in the main body of the paper.
- Apologize. Even if your research contains significant limitations, don’t undermine your authority by including statements that doubt your methodology or execution.
- Shy away from speaking on limitations or negative results. Including limitations and negative results will give readers a complete understanding of the presented research. Potential limitations include sources of potential bias, threats to internal or external validity, barriers to implementing an intervention and other issues inherent to the study design.
- Overstate the importance of your findings. Making grand statements about how a study will fully resolve large questions can lead readers to doubt the success of the research.
Snippets of Effective Discussions:
Consumer-based actions to reduce plastic pollution in rivers: A multi-criteria decision analysis approach
Identifying reliable indicators of fitness in polar bears
- How to Write a Great Title
- How to Write an Abstract
- How to Write Your Methods
- How to Report Statistics
- How to Edit Your Work
The contents of the Peer Review Center are also available as a live, interactive training session, complete with slides, talking points, and activities. …
The contents of the Writing Center are also available as a live, interactive training session, complete with slides, talking points, and activities. …
There’s a lot to consider when deciding where to submit your work. Learn how to choose a journal that will help your study reach its audience, while reflecting your values as a researcher…
Throughout this module, we have explored characteristics about the statistics that can be recorded to summarize a sample. We've discussed relevant statistics that we can record, depending on:
- the type of variable
- the number of populations
- the characteristics and structure of the data
We've also demonstrated that the specific values of a statistic will vary from sample to sample. Sampling distributions help us to understand all of the values that a statistic could take from different samples. In some instances, we can determine the theoretical sampling distribution for some statistics (those based on sample means $\bar{x}s$ and sample proportions $\hat{p}$s). On the other hand, we can rely on simulation to determine the sampling distribution for many statistics, and we can do so using either the population data if we have it or using the sample data to approximate the sampling distribution. We specifically examined how properties of the sampling distribution vary depending on characteristics, including:
- the number of repetitions (samples)
- the sample size
- the type of statistic recorded.
The most important characteristics of sampling distributions that we observed were the:
- variability (or spread)
Once we have a sampling distribution, we can then use it to calculate probabilities associated with the statistic.
Often, we don't actually know the true population, and so often we have to rely on our sample data to help us estimate the sampling distribution. Although this is an approximation that relies on having appropriate data, this approximation does allow us to evaluate the uncertainty associated with statistics measured from samples.

An official website of the United States government
The .gov means it’s official. Federal government websites often end in .gov or .mil. Before sharing sensitive information, make sure you’re on a federal government site.
The site is secure. The https:// ensures that you are connecting to the official website and that any information you provide is encrypted and transmitted securely.
- Publications
- Account settings
Preview improvements coming to the PMC website in October 2024. Learn More or Try it out now .
- Advanced Search
- Journal List
- J Clin Diagn Res
- v.11(5); 2017 May
Critical Appraisal of Clinical Research
Azzam al-jundi.
1 Professor, Department of Orthodontics, King Saud bin Abdul Aziz University for Health Sciences-College of Dentistry, Riyadh, Kingdom of Saudi Arabia.
Salah Sakka
2 Associate Professor, Department of Oral and Maxillofacial Surgery, Al Farabi Dental College, Riyadh, KSA.
Evidence-based practice is the integration of individual clinical expertise with the best available external clinical evidence from systematic research and patient’s values and expectations into the decision making process for patient care. It is a fundamental skill to be able to identify and appraise the best available evidence in order to integrate it with your own clinical experience and patients values. The aim of this article is to provide a robust and simple process for assessing the credibility of articles and their value to your clinical practice.
Introduction
Decisions related to patient value and care is carefully made following an essential process of integration of the best existing evidence, clinical experience and patient preference. Critical appraisal is the course of action for watchfully and systematically examining research to assess its reliability, value and relevance in order to direct professionals in their vital clinical decision making [ 1 ].
Critical appraisal is essential to:
- Combat information overload;
- Identify papers that are clinically relevant;
- Continuing Professional Development (CPD).
Carrying out Critical Appraisal:
Assessing the research methods used in the study is a prime step in its critical appraisal. This is done using checklists which are specific to the study design.
Standard Common Questions:
- What is the research question?
- What is the study type (design)?
- Selection issues.
- What are the outcome factors and how are they measured?
- What are the study factors and how are they measured?
- What important potential confounders are considered?
- What is the statistical method used in the study?
- Statistical results.
- What conclusions did the authors reach about the research question?
- Are ethical issues considered?
The Critical Appraisal starts by double checking the following main sections:
I. Overview of the paper:
- The publishing journal and the year
- The article title: Does it state key trial objectives?
- The author (s) and their institution (s)
The presence of a peer review process in journal acceptance protocols also adds robustness to the assessment criteria for research papers and hence would indicate a reduced likelihood of publication of poor quality research. Other areas to consider may include authors’ declarations of interest and potential market bias. Attention should be paid to any declared funding or the issue of a research grant, in order to check for a conflict of interest [ 2 ].
II. ABSTRACT: Reading the abstract is a quick way of getting to know the article and its purpose, major procedures and methods, main findings, and conclusions.
- Aim of the study: It should be well and clearly written.
- Materials and Methods: The study design and type of groups, type of randomization process, sample size, gender, age, and procedure rendered to each group and measuring tool(s) should be evidently mentioned.
- Results: The measured variables with their statistical analysis and significance.
- Conclusion: It must clearly answer the question of interest.
III. Introduction/Background section:
An excellent introduction will thoroughly include references to earlier work related to the area under discussion and express the importance and limitations of what is previously acknowledged [ 2 ].
-Why this study is considered necessary? What is the purpose of this study? Was the purpose identified before the study or a chance result revealed as part of ‘data searching?’
-What has been already achieved and how does this study be at variance?
-Does the scientific approach outline the advantages along with possible drawbacks associated with the intervention or observations?
IV. Methods and Materials section : Full details on how the study was actually carried out should be mentioned. Precise information is given on the study design, the population, the sample size and the interventions presented. All measurements approaches should be clearly stated [ 3 ].
V. Results section : This section should clearly reveal what actually occur to the subjects. The results might contain raw data and explain the statistical analysis. These can be shown in related tables, diagrams and graphs.
VI. Discussion section : This section should include an absolute comparison of what is already identified in the topic of interest and the clinical relevance of what has been newly established. A discussion on a possible related limitations and necessitation for further studies should also be indicated.
Does it summarize the main findings of the study and relate them to any deficiencies in the study design or problems in the conduct of the study? (This is called intention to treat analysis).
- Does it address any source of potential bias?
- Are interpretations consistent with the results?
- How are null findings interpreted?
- Does it mention how do the findings of this study relate to previous work in the area?
- Can they be generalized (external validity)?
- Does it mention their clinical implications/applicability?
- What are the results/outcomes/findings applicable to and will they affect a clinical practice?
- Does the conclusion answer the study question?
- -Is the conclusion convincing?
- -Does the paper indicate ethics approval?
- -Can you identify potential ethical issues?
- -Do the results apply to the population in which you are interested?
- -Will you use the results of the study?
Once you have answered the preliminary and key questions and identified the research method used, you can incorporate specific questions related to each method into your appraisal process or checklist.
1-What is the research question?
For a study to gain value, it should address a significant problem within the healthcare and provide new or meaningful results. Useful structure for assessing the problem addressed in the article is the Problem Intervention Comparison Outcome (PICO) method [ 3 ].
P = Patient or problem: Patient/Problem/Population:
It involves identifying if the research has a focused question. What is the chief complaint?
E.g.,: Disease status, previous ailments, current medications etc.,
I = Intervention: Appropriately and clearly stated management strategy e.g.,: new diagnostic test, treatment, adjunctive therapy etc.,
C= Comparison: A suitable control or alternative
E.g.,: specific and limited to one alternative choice.
O= Outcomes: The desired results or patient related consequences have to be identified. e.g.,: eliminating symptoms, improving function, esthetics etc.,
The clinical question determines which study designs are appropriate. There are five broad categories of clinical questions, as shown in [ Table/Fig-1 ].
[Table/Fig-1]:
Categories of clinical questions and the related study designs.
2- What is the study type (design)?
The study design of the research is fundamental to the usefulness of the study.
In a clinical paper the methodology employed to generate the results is fully explained. In general, all questions about the related clinical query, the study design, the subjects and the correlated measures to reduce bias and confounding should be adequately and thoroughly explored and answered.
Participants/Sample Population:
Researchers identify the target population they are interested in. A sample population is therefore taken and results from this sample are then generalized to the target population.
The sample should be representative of the target population from which it came. Knowing the baseline characteristics of the sample population is important because this allows researchers to see how closely the subjects match their own patients [ 4 ].
Sample size calculation (Power calculation): A trial should be large enough to have a high chance of detecting a worthwhile effect if it exists. Statisticians can work out before the trial begins how large the sample size should be in order to have a good chance of detecting a true difference between the intervention and control groups [ 5 ].
- Is the sample defined? Human, Animals (type); what population does it represent?
- Does it mention eligibility criteria with reasons?
- Does it mention where and how the sample were recruited, selected and assessed?
- Does it mention where was the study carried out?
- Is the sample size justified? Rightly calculated? Is it adequate to detect statistical and clinical significant results?
- Does it mention a suitable study design/type?
- Is the study type appropriate to the research question?
- Is the study adequately controlled? Does it mention type of randomization process? Does it mention the presence of control group or explain lack of it?
- Are the samples similar at baseline? Is sample attrition mentioned?
- All studies report the number of participants/specimens at the start of a study, together with details of how many of them completed the study and reasons for incomplete follow up if there is any.
- Does it mention who was blinded? Are the assessors and participants blind to the interventions received?
- Is it mentioned how was the data analysed?
- Are any measurements taken likely to be valid?
Researchers use measuring techniques and instruments that have been shown to be valid and reliable.
Validity refers to the extent to which a test measures what it is supposed to measure.
(the extent to which the value obtained represents the object of interest.)
- -Soundness, effectiveness of the measuring instrument;
- -What does the test measure?
- -Does it measure, what it is supposed to be measured?
- -How well, how accurately does it measure?
Reliability: In research, the term reliability means “repeatability” or “consistency”
Reliability refers to how consistent a test is on repeated measurements. It is important especially if assessments are made on different occasions and or by different examiners. Studies should state the method for assessing the reliability of any measurements taken and what the intra –examiner reliability was [ 6 ].
3-Selection issues:
The following questions should be raised:
- - How were subjects chosen or recruited? If not random, are they representative of the population?
- - Types of Blinding (Masking) Single, Double, Triple?
- - Is there a control group? How was it chosen?
- - How are patients followed up? Who are the dropouts? Why and how many are there?
- - Are the independent (predictor) and dependent (outcome) variables in the study clearly identified, defined, and measured?
- - Is there a statement about sample size issues or statistical power (especially important in negative studies)?
- - If a multicenter study, what quality assurance measures were employed to obtain consistency across sites?
- - Are there selection biases?
- • In a case-control study, if exercise habits to be compared:
- - Are the controls appropriate?
- - Were records of cases and controls reviewed blindly?
- - How were possible selection biases controlled (Prevalence bias, Admission Rate bias, Volunteer bias, Recall bias, Lead Time bias, Detection bias, etc.,)?
- • Cross Sectional Studies:
- - Was the sample selected in an appropriate manner (random, convenience, etc.,)?
- - Were efforts made to ensure a good response rate or to minimize the occurrence of missing data?
- - Were reliability (reproducibility) and validity reported?
- • In an intervention study, how were subjects recruited and assigned to groups?
- • In a cohort study, how many reached final follow-up?
- - Are the subject’s representatives of the population to which the findings are applied?
- - Is there evidence of volunteer bias? Was there adequate follow-up time?
- - What was the drop-out rate?
- - Any shortcoming in the methodology can lead to results that do not reflect the truth. If clinical practice is changed on the basis of these results, patients could be harmed.
Researchers employ a variety of techniques to make the methodology more robust, such as matching, restriction, randomization, and blinding [ 7 ].
Bias is the term used to describe an error at any stage of the study that was not due to chance. Bias leads to results in which there are a systematic deviation from the truth. As bias cannot be measured, researchers need to rely on good research design to minimize bias [ 8 ]. To minimize any bias within a study the sample population should be representative of the population. It is also imperative to consider the sample size in the study and identify if the study is adequately powered to produce statistically significant results, i.e., p-values quoted are <0.05 [ 9 ].
4-What are the outcome factors and how are they measured?
- -Are all relevant outcomes assessed?
- -Is measurement error an important source of bias?
5-What are the study factors and how are they measured?
- -Are all the relevant study factors included in the study?
- -Have the factors been measured using appropriate tools?
Data Analysis and Results:
- Were the tests appropriate for the data?
- Are confidence intervals or p-values given?
- How strong is the association between intervention and outcome?
- How precise is the estimate of the risk?
- Does it clearly mention the main finding(s) and does the data support them?
- Does it mention the clinical significance of the result?
- Is adverse event or lack of it mentioned?
- Are all relevant outcomes assessed?
- Was the sample size adequate to detect a clinically/socially significant result?
- Are the results presented in a way to help in health policy decisions?
- Is there measurement error?
- Is measurement error an important source of bias?
Confounding Factors:
A confounder has a triangular relationship with both the exposure and the outcome. However, it is not on the causal pathway. It makes it appear as if there is a direct relationship between the exposure and the outcome or it might even mask an association that would otherwise have been present [ 9 ].
6- What important potential confounders are considered?
- -Are potential confounders examined and controlled for?
- -Is confounding an important source of bias?
7- What is the statistical method in the study?
- -Are the statistical methods described appropriate to compare participants for primary and secondary outcomes?
- -Are statistical methods specified insufficient detail (If I had access to the raw data, could I reproduce the analysis)?
- -Were the tests appropriate for the data?
- -Are confidence intervals or p-values given?
- -Are results presented as absolute risk reduction as well as relative risk reduction?
Interpretation of p-value:
The p-value refers to the probability that any particular outcome would have arisen by chance. A p-value of less than 1 in 20 (p<0.05) is statistically significant.
- When p-value is less than significance level, which is usually 0.05, we often reject the null hypothesis and the result is considered to be statistically significant. Conversely, when p-value is greater than 0.05, we conclude that the result is not statistically significant and the null hypothesis is accepted.
Confidence interval:
Multiple repetition of the same trial would not yield the exact same results every time. However, on average the results would be within a certain range. A 95% confidence interval means that there is a 95% chance that the true size of effect will lie within this range.
8- Statistical results:
- -Do statistical tests answer the research question?
Are statistical tests performed and comparisons made (data searching)?
Correct statistical analysis of results is crucial to the reliability of the conclusions drawn from the research paper. Depending on the study design and sample selection method employed, observational or inferential statistical analysis may be carried out on the results of the study.
It is important to identify if this is appropriate for the study [ 9 ].
- -Was the sample size adequate to detect a clinically/socially significant result?
- -Are the results presented in a way to help in health policy decisions?
Clinical significance:
Statistical significance as shown by p-value is not the same as clinical significance. Statistical significance judges whether treatment effects are explicable as chance findings, whereas clinical significance assesses whether treatment effects are worthwhile in real life. Small improvements that are statistically significant might not result in any meaningful improvement clinically. The following questions should always be on mind:
- -If the results are statistically significant, do they also have clinical significance?
- -If the results are not statistically significant, was the sample size sufficiently large to detect a meaningful difference or effect?
9- What conclusions did the authors reach about the study question?
Conclusions should ensure that recommendations stated are suitable for the results attained within the capacity of the study. The authors should also concentrate on the limitations in the study and their effects on the outcomes and the proposed suggestions for future studies [ 10 ].
- -Are the questions posed in the study adequately addressed?
- -Are the conclusions justified by the data?
- -Do the authors extrapolate beyond the data?
- -Are shortcomings of the study addressed and constructive suggestions given for future research?
- -Bibliography/References:
Do the citations follow one of the Council of Biological Editors’ (CBE) standard formats?
10- Are ethical issues considered?
If a study involves human subjects, human tissues, or animals, was approval from appropriate institutional or governmental entities obtained? [ 10 , 11 ].
Critical appraisal of RCTs: Factors to look for:
- Allocation (randomization, stratification, confounders).
- Follow up of participants (intention to treat).
- Data collection (bias).
- Sample size (power calculation).
- Presentation of results (clear, precise).
- Applicability to local population.
[ Table/Fig-2 ] summarizes the guidelines for Consolidated Standards of Reporting Trials CONSORT [ 12 ].
[Table/Fig-2]:
Summary of the CONSORT guidelines.
Critical appraisal of systematic reviews: provide an overview of all primary studies on a topic and try to obtain an overall picture of the results.
In a systematic review, all the primary studies identified are critically appraised and only the best ones are selected. A meta-analysis (i.e., a statistical analysis) of the results from selected studies may be included. Factors to look for:
- Literature search (did it include published and unpublished materials as well as non-English language studies? Was personal contact with experts sought?).
- Quality-control of studies included (type of study; scoring system used to rate studies; analysis performed by at least two experts).
- Homogeneity of studies.
[ Table/Fig-3 ] summarizes the guidelines for Preferred Reporting Items for Systematic reviews and Meta-Analyses PRISMA [ 13 ].
[Table/Fig-3]:
Summary of PRISMA guidelines.
Critical appraisal is a fundamental skill in modern practice for assessing the value of clinical researches and providing an indication of their relevance to the profession. It is a skills-set developed throughout a professional career that facilitates this and, through integration with clinical experience and patient preference, permits the practice of evidence based medicine and dentistry. By following a systematic approach, such evidence can be considered and applied to clinical practice.
Financial or other Competing Interests
- Privacy Policy

Home » Research Paper Conclusion – Writing Guide and Examples
Research Paper Conclusion – Writing Guide and Examples
Table of Contents

Research Paper Conclusion
Definition:
A research paper conclusion is the final section of a research paper that summarizes the key findings, significance, and implications of the research. It is the writer’s opportunity to synthesize the information presented in the paper, draw conclusions, and make recommendations for future research or actions.
The conclusion should provide a clear and concise summary of the research paper, reiterating the research question or problem, the main results, and the significance of the findings. It should also discuss the limitations of the study and suggest areas for further research.

Parts of Research Paper Conclusion
The parts of a research paper conclusion typically include:
Restatement of the Thesis
The conclusion should begin by restating the thesis statement from the introduction in a different way. This helps to remind the reader of the main argument or purpose of the research.
Summary of Key Findings
The conclusion should summarize the main findings of the research, highlighting the most important results and conclusions. This section should be brief and to the point.
Implications and Significance
In this section, the researcher should explain the implications and significance of the research findings. This may include discussing the potential impact on the field or industry, highlighting new insights or knowledge gained, or pointing out areas for future research.
Limitations and Recommendations
It is important to acknowledge any limitations or weaknesses of the research and to make recommendations for how these could be addressed in future studies. This shows that the researcher is aware of the potential limitations of their work and is committed to improving the quality of research in their field.
Concluding Statement
The conclusion should end with a strong concluding statement that leaves a lasting impression on the reader. This could be a call to action, a recommendation for further research, or a final thought on the topic.
How to Write Research Paper Conclusion
Here are some steps you can follow to write an effective research paper conclusion:
- Restate the research problem or question: Begin by restating the research problem or question that you aimed to answer in your research. This will remind the reader of the purpose of your study.
- Summarize the main points: Summarize the key findings and results of your research. This can be done by highlighting the most important aspects of your research and the evidence that supports them.
- Discuss the implications: Discuss the implications of your findings for the research area and any potential applications of your research. You should also mention any limitations of your research that may affect the interpretation of your findings.
- Provide a conclusion : Provide a concise conclusion that summarizes the main points of your paper and emphasizes the significance of your research. This should be a strong and clear statement that leaves a lasting impression on the reader.
- Offer suggestions for future research: Lastly, offer suggestions for future research that could build on your findings and contribute to further advancements in the field.
Remember that the conclusion should be brief and to the point, while still effectively summarizing the key findings and implications of your research.
Example of Research Paper Conclusion
Here’s an example of a research paper conclusion:
Conclusion :
In conclusion, our study aimed to investigate the relationship between social media use and mental health among college students. Our findings suggest that there is a significant association between social media use and increased levels of anxiety and depression among college students. This highlights the need for increased awareness and education about the potential negative effects of social media use on mental health, particularly among college students.
Despite the limitations of our study, such as the small sample size and self-reported data, our findings have important implications for future research and practice. Future studies should aim to replicate our findings in larger, more diverse samples, and investigate the potential mechanisms underlying the association between social media use and mental health. In addition, interventions should be developed to promote healthy social media use among college students, such as mindfulness-based approaches and social media detox programs.
Overall, our study contributes to the growing body of research on the impact of social media on mental health, and highlights the importance of addressing this issue in the context of higher education. By raising awareness and promoting healthy social media use among college students, we can help to reduce the negative impact of social media on mental health and improve the well-being of young adults.
Purpose of Research Paper Conclusion
The purpose of a research paper conclusion is to provide a summary and synthesis of the key findings, significance, and implications of the research presented in the paper. The conclusion serves as the final opportunity for the writer to convey their message and leave a lasting impression on the reader.
The conclusion should restate the research problem or question, summarize the main results of the research, and explain their significance. It should also acknowledge the limitations of the study and suggest areas for future research or action.
Overall, the purpose of the conclusion is to provide a sense of closure to the research paper and to emphasize the importance of the research and its potential impact. It should leave the reader with a clear understanding of the main findings and why they matter. The conclusion serves as the writer’s opportunity to showcase their contribution to the field and to inspire further research and action.
When to Write Research Paper Conclusion
The conclusion of a research paper should be written after the body of the paper has been completed. It should not be written until the writer has thoroughly analyzed and interpreted their findings and has written a complete and cohesive discussion of the research.
Before writing the conclusion, the writer should review their research paper and consider the key points that they want to convey to the reader. They should also review the research question, hypotheses, and methodology to ensure that they have addressed all of the necessary components of the research.
Once the writer has a clear understanding of the main findings and their significance, they can begin writing the conclusion. The conclusion should be written in a clear and concise manner, and should reiterate the main points of the research while also providing insights and recommendations for future research or action.
Characteristics of Research Paper Conclusion
The characteristics of a research paper conclusion include:
- Clear and concise: The conclusion should be written in a clear and concise manner, summarizing the key findings and their significance.
- Comprehensive: The conclusion should address all of the main points of the research paper, including the research question or problem, the methodology, the main results, and their implications.
- Future-oriented : The conclusion should provide insights and recommendations for future research or action, based on the findings of the research.
- Impressive : The conclusion should leave a lasting impression on the reader, emphasizing the importance of the research and its potential impact.
- Objective : The conclusion should be based on the evidence presented in the research paper, and should avoid personal biases or opinions.
- Unique : The conclusion should be unique to the research paper and should not simply repeat information from the introduction or body of the paper.
Advantages of Research Paper Conclusion
The advantages of a research paper conclusion include:
- Summarizing the key findings : The conclusion provides a summary of the main findings of the research, making it easier for the reader to understand the key points of the study.
- Emphasizing the significance of the research: The conclusion emphasizes the importance of the research and its potential impact, making it more likely that readers will take the research seriously and consider its implications.
- Providing recommendations for future research or action : The conclusion suggests practical recommendations for future research or action, based on the findings of the study.
- Providing closure to the research paper : The conclusion provides a sense of closure to the research paper, tying together the different sections of the paper and leaving a lasting impression on the reader.
- Demonstrating the writer’s contribution to the field : The conclusion provides the writer with an opportunity to showcase their contribution to the field and to inspire further research and action.
Limitations of Research Paper Conclusion
While the conclusion of a research paper has many advantages, it also has some limitations that should be considered, including:
- I nability to address all aspects of the research: Due to the limited space available in the conclusion, it may not be possible to address all aspects of the research in detail.
- Subjectivity : While the conclusion should be objective, it may be influenced by the writer’s personal biases or opinions.
- Lack of new information: The conclusion should not introduce new information that has not been discussed in the body of the research paper.
- Lack of generalizability: The conclusions drawn from the research may not be applicable to other contexts or populations, limiting the generalizability of the study.
- Misinterpretation by the reader: The reader may misinterpret the conclusions drawn from the research, leading to a misunderstanding of the findings.
About the author
Muhammad Hassan
Researcher, Academic Writer, Web developer
You may also like

How to Cite Research Paper – All Formats and...

Data Collection – Methods Types and Examples

Delimitations in Research – Types, Examples and...

Research Paper Format – Types, Examples and...

Research Process – Steps, Examples and Tips

Research Design – Types, Methods and Examples

Building a Foundation for Sound Environmental Decisions (1997)
Chapter: 5 summary, conclusions, and recommendations, 5 summary, conclusions, and recommendations.
Pressures on the environment will continue to increase. Global population increase, rising incomes, and agricultural and industrial expansion will inevitably produce unanticipated and potentially deleterious ecological, economic, and human health consequences. Environmental research has proven its value in helping to respond to and prevent many environmental problems, and it continues to be a wise and necessary investment.
The charge to this committee was to provide an overview of significant emerging environmental issues; identify and prioritize research themes and projects that are most relevant to understanding and resolving these issues; and consider the role of EPA's research program in addressing these issues, in the context of research being conducted or sponsored by other organizations. After careful deliberation, the committee decided not to simply present a limited list of "emerging" issues with specific research projects to address them. Such an exercise would provide a mere snapshot in time, based on the insights of one particular collection of individuals. Instead—and hopefully more valuably—this report provides an overview of important environmental issues and presents a framework for organizing environmental research. The report also describes major research themes and programs of relevance to EPA; suggests criteria that can be used to identify and prioritize among important research areas; recommends actions EPA should take to build its scientific capacity; and provides illustrations of the kinds of research projects that EPA should consider.
CONCLUSIONS
As a key environmental agency, EPA needs to support and maintain a strong research program. An evolving understanding of the complexity, magnitude,
and inter-relatedness of environmental problems leads us to conclude that a new balance of research programs may be helpful. This report describes a framework for conducting research in a way that will help alleviate the problems of the moment while providing a basis for solving tomorrow's problems.
In the past, pressing environmental issues have been addressed primarily through focused research efforts directed toward solving particular problems. Although this approach to environmental research can be effective, has often been necessary, and will surely continue, it also has limitations. In order to address the abundance of established, emerging, and as-yet-unknown environmental issues, an expanded understanding of the scientific principles underlying environmental systems is needed. Achieving this understanding will require innovative, interdisciplinary approaches.
To develop the knowledge needed to address current and emerging environmental issues, EPA should undertake both problem-driven research and core research . Problem-driven research is targeted at understanding and solving identified environmental problems, while core research aims to provide broader, more generic information that will help improve understanding of many problems now and in the future. Core research includes three components: (1) understanding the processes that drive and connect environmental systems; (2) development of innovative tools and methods for understanding and managing environmental problems; and (3) long-term collection and dissemination of accurate environmental data.
Research activities within problem-driven and core research programs may often overlap. Fundamental discoveries can be made during the search for a solution to a narrowly defined problem; likewise, as illustrated earlier in this report, breakthroughs in problem-solving often occur as a result of core research efforts. Both kinds of investigations are needed, and feedback between them will greatly enhance the overall environmental research endeavor (see Figure 5-1 ).
Because EPA's task of protecting the environment and human health is so vast and difficult, and because resources to undertake the necessary research are very limited, choices will have to be made among many worthwhile projects. The approaches for making these choices will be different in the core and problem-driven portions of the research program. The former should seek better understanding of fundamental phenomena and generate broadly relevant research tools and information. The latter will be more responsive to regulatory activities and other immediate needs and should be guided by the paradigm of risk reduction. Because there are so many specific issues of importance to the public, the Congress, and EPA's own program and regional offices, there is a temptation to include many problems for attention. It is important to resist this trend: it will inevitably lead either to the dilution of efforts to solve the most pressing problems or to the reduction of funding available for critical core research needs.
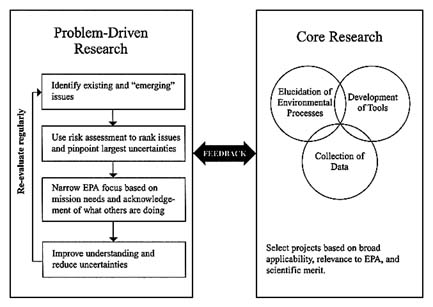
FIGURE 5-1 A framework for environmental research at EPA.
Interactions among the natural environment, plants, animals, and the evergrowing human population are highly complex and inherently unpredictable. Although this report provides a broad overview of current and emerging environmental issues, it is important to note that this is merely a snapshot in time. Identification of issues requiring attention is a dynamic, continuous process.
With its limited budget, staff, and mandate, it is not possible or reasonable for EPA to act alone in understanding and addressing all environmental problems. Many other federal agencies, state agencies, other organizations (including utilities), universities, and private companies have played and will continue to play important roles in environmental research. Cooperation with others will be particularly needed in the area of environmental monitoring, a complex and costly undertaking, and in the investigation of global-scale issues.
Another factor to consider in determining EPA's research role on a particular environmental issue is whether the private sector has any incentive to study or develop better solutions, or whether the primary research must originate from the public sector to serve the public good. Examples of areas of "public good" that might deserve EPA attention include municipal wastewater and drinking water treatment, nonpoint-source pollution control, restoration of degraded ecosystems, and large-scale regional and global air pollution problems.
RECOMMENDATIONS
To enhance the productivity and effectiveness of EPA's research efforts, the committee makes recommendations in three areas: a general approach to research, core research themes, and problem-driven research themes.
Approach to Research
EPA should establish a balance between problem-driven and core research. Although there is currently an emphasis on problem-driven research projects in EPA, the core component of EPA's research program should be developed to be approximately equal in magnitude.
EPA should develop an internal mechanism for continually identifying emerging issues and then applying a risk assessment evaluation to these issues to determine the highest priorities and areas of greatest uncertainty. One important method for identifying emerging issues is to review and synthesize new findings from the core research program. EPA research personnel should be fully engaged in the issue identification and research planning process.
EPA should cooperate closely with agencies, organizations, municipalities, universities, and industries involved in environmental research. In addition to providing research support, mechanisms for cooperation might include participation of EPA management in interagency coordination efforts, participation of staff in scientific meetings and conferences, and incentives and rewards for individuals who seek out and work with their counterparts in other organizations. Collaboration should be maintained in research endeavors, environmental monitoring, data archiving, and environmental policy formulation and evaluation. EPA should continue to act as a coordinator in bringing various environmental researchers together to exchange information and ideas, possibly in the form of interdisciplinary workshops on particular environmental topics. This would also help in ''scanning the horizon" to identify new environmental trends and emerging problems. Through these meetings, EPA can discuss the relative risks as well as solutions and policies and can determine which areas require more research.
EPA should compile, publish, and disseminate an annual summary of all research being conducted or funded by the agency in order to facilitate both better cooperation with others and better internal planning. The report should be organized into broad strategic categories, with sub-categories describing program areas. Publications and other output should be listed and made available upon request.
Core Research Themes
The core component of EPA's research program should include three basic objectives:
Acquisition of systematic understanding about underlying environmental processes (such as those displayed in Table 2.2 );
Development of broadly applicable research tools, including better techniques for measuring physical, chemical, biological, social, and economic variables of interest; more accurate models of complex systems and their interactions; and new methods for analyzing, displaying, and using environmental information for science-based decision making;
Design, implementation, and maintenance of appropriate environmental monitoring programs, with evaluation, analysis, synthesis and dissemination of the data and results to improve understanding of the status of and changes in environmental resources over time and to confirm that environmental policies are having the desired effect.
Core research projects should be selected based on their relevance to EPA's mission, whether such research is already being sponsored by other agencies, and the quality of the work proposed, as determined by a peer-review process. Cross-cutting, interdisciplinary studies that take advantage of advances in many different fields will be particularly valuable.
As part of its core research efforts, EPA should conduct retrospective evaluations of the effectiveness of environmental policies and decisions. Retrospective evaluations are critical to ensuring that environmental policies are achieving their intended goals without creating unpredicted, undesirable side-effects.
EPA should make a long-term financial and intellectual commitment to core research projects. Progress in core research generally does not come quickly; therefore it is important that the agency provide adequate long-term support to this kind of knowledge development, allowing it to follow its often unpredictable course. Tool development and data collection must be ongoing endeavors in order to be fully effective.
Problem-Driven Research Themes
EPA should maintain a focused, problem-driven research program. The problem-driven and core research areas will be complementary and result in the interaction of ideas and results.
Evaluation of problem-driven research areas should focus on reducing the risks and uncertainties associated with each problem. EPA should retain its emphasis on risk assessment to prioritize among problem-driven research areas. Using criteria such as timing, novelty, scope, severity, and probability satisfies this requirement, as does the more detailed risk assessment framework described in the EPA strategic plan for ORD. Although risk assessment and
TABLE 5-1 Recommended Actions for EPA
management provide a good framework for choosing among issues, the methodology must be refined to achieve more accurate assessments.
EPA should concentrate efforts in areas where the private sector has little incentive to conduct research or develop better solutions to environmental problems.
Problem-driven research should be re-evaluated and re-focused on a regular basis to ensure that the most important problems are being addressed. Unlike core research priorities, which may not change much over time, in the problem-driven area EPA must develop adaptive feedback capabilities to allow it to change directions when new issues arise and old issues are "solved" or judged to pose less risk than expected.
This committee was not asked to, and did not, address issues concerning EPA's research infrastructure, the appropriate balance between internal and external research, mechanisms for peer review, and other research management issues. Recommendations in these areas will be made by the Committee on Research and Peer Review at EPA (see Chapter 1 ). Table 5-1 summarizes recommended
actions that are intended to provide EPA with the knowledge needed to address current and emerging environmental issues.
Good science is essential for sound environmental decision-making. By implementing the recommendations contained in this report, EPA can increase the effectiveness of its research program and thus continue to play an important role in efforts to protect the environment and human health into the next century.
Over the past decades, environmental problems have attracted enormous attention and public concern. Many actions have been taken by the U.S. Environmental Protection Agency and others to protect human health and ecosystems from particular threats. Despite some successes, many problems remain unsolved and new ones are emerging. Increasing population and related pressures, combined with a realization of the interconnectedness and complexity of environmental systems, present new challenges to policymakers and regulators.
Scientific research has played, and will continue to play, an essential part in solving environmental problems. Decisions based on incorrect or incomplete understanding of environmental systems will not achieve the greatest reduction of risk at the lowest cost.
This volume describes a framework for acquiring the knowledge needed both to solve current recognized problems and to be prepared for the kinds of problems likely to emerge in the future. Many case examples are included to illustrate why some environmental control strategies have succeeded where others have fallen short and how we can do better in the future.
READ FREE ONLINE
Welcome to OpenBook!
You're looking at OpenBook, NAP.edu's online reading room since 1999. Based on feedback from you, our users, we've made some improvements that make it easier than ever to read thousands of publications on our website.
Do you want to take a quick tour of the OpenBook's features?
Show this book's table of contents , where you can jump to any chapter by name.
...or use these buttons to go back to the previous chapter or skip to the next one.
Jump up to the previous page or down to the next one. Also, you can type in a page number and press Enter to go directly to that page in the book.
Switch between the Original Pages , where you can read the report as it appeared in print, and Text Pages for the web version, where you can highlight and search the text.
To search the entire text of this book, type in your search term here and press Enter .
Share a link to this book page on your preferred social network or via email.
View our suggested citation for this chapter.
Ready to take your reading offline? Click here to buy this book in print or download it as a free PDF, if available.
Get Email Updates
Do you enjoy reading reports from the Academies online for free ? Sign up for email notifications and we'll let you know about new publications in your areas of interest when they're released.
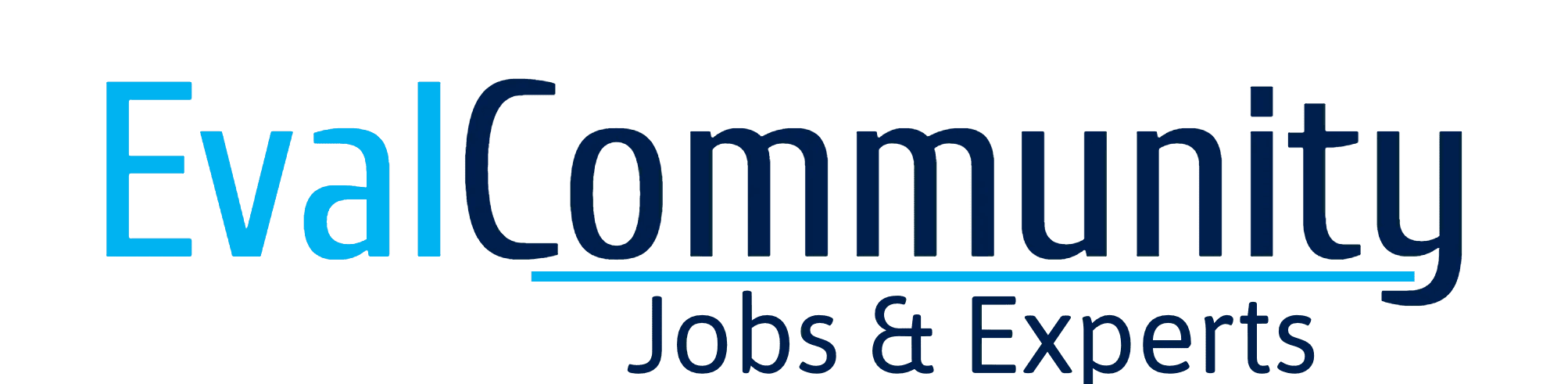
- Differences between a finding, a conclusion, and a recommendation: examples
- Learning Center
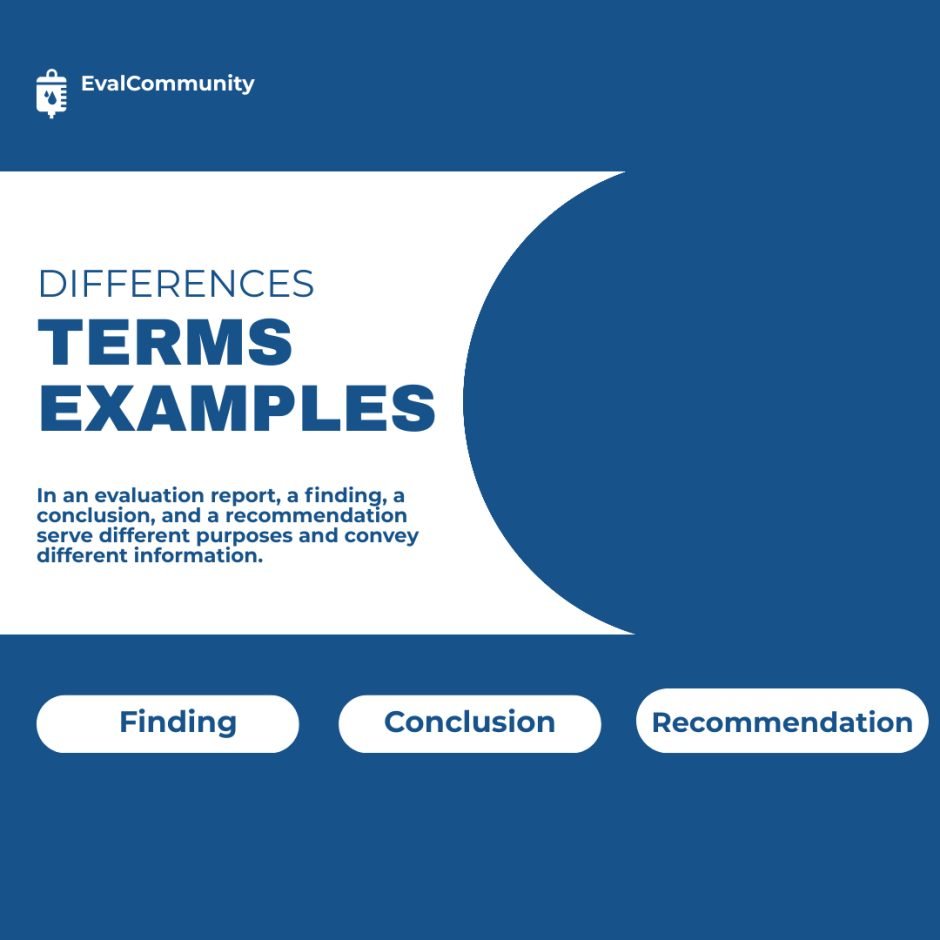
Table of Contents
- Defining the Terms: What Is a Finding, a Conclusion, and a Recommendation in M&E?
- Why It Matters: Understanding the Importance of Differentiating between Findings, Conclusions, and Recommendations in M&E
- How to Identify and Distinguish between Findings, Conclusions, and Recommendations in M&E
- How to Communicate Findings, Conclusions, and Recommendations Effectively in M&E Reports
- The Benefits of Clear and Accurate Reporting of Findings, Conclusions, and Recommendations in M&E
1. Defining the Terms: What Is a Finding, a Conclusion, and a Recommendation in M&E?
Monitoring and Evaluation (M&E) is a critical process for assessing the effectiveness of development programs and policies. During the M&E process, evaluators collect and analyze data to draw conclusions and make recommendations for program improvement. In M&E, it is essential to differentiate between findings, conclusions, and recommendations to ensure that the evaluation report accurately reflects the program’s strengths, weaknesses, and potential areas for improvement.
In an evaluation report, a finding, a conclusion, and a recommendation serve different purposes and convey different information. Here are the differences between these three elements:
1.1 Finding
A finding is a factual statement that is based on evidence collected during the evaluation . It describes what was observed, heard, or experienced during the evaluation process. A finding should be objective, unbiased, and supported by data. Findings are typically presented in the form of a summary or a list of key points, and they provide the basis for the evaluation’s conclusions and recommendations.
Findings are an important part of the evaluation process, as they provide objective and unbiased information about what was observed, heard, or experienced during the evaluation. Findings are based on the evidence collected during the evaluation, and they should be supported by data and other relevant information. They are typically presented in a summary or list format, and they serve as the basis for the evaluation’s conclusions and recommendations. By presenting clear and accurate findings, evaluators can help stakeholders understand the strengths and weaknesses of the program or initiative being evaluated, and identify opportunities for improvement.
Catch HR’s eye instantly?
- Resume Review
- Resume Writing
- Resume Optimization
Premier global development resume service since 2012
Stand Out with a Pro Resume
1.2 Examples of Finding
Here are some examples of findings in M&E:
- “Program participants reported a high level of satisfaction with the quality of training provided, with 85% rating it as good or excellent.”
- “The program was successful in increasing the number of girls enrolled in secondary school, with a 25% increase observed in the target communities.”
- “Program beneficiaries reported improved access to healthcare services, with a 40% increase in the number of individuals accessing healthcare facilities in the program area.”
- “The program’s training curriculum was found to be outdated and ineffective, with only 30% of participants reporting that the training was useful.”
- “The program’s monitoring and evaluation system was found to be inadequate, with data quality issues and insufficient capacity among staff to carry out effective monitoring and evaluation activities.”
These findings represent objective, measurable results of the data collected during the M&E process, and can be used to inform program design and implementation, as well as to draw conclusions and make recommendations for improvement.
1.3 Conclusion
A conclusion is a judgment or interpretation of the findings based on the evidence collected during the evaluation. It is typically expressed in terms of what the findings mean or what can be inferred from them. Conclusions should be logical, evidence-based, and free from personal bias or opinion.
Conclusions often answer the evaluation questions or objectives, and they provide insights into the effectiveness or impact of the program, project, or intervention being evaluated. By synthesizing the findings into a cohesive narrative, evaluators can provide stakeholders with a clear and actionable understanding of the program or initiative being evaluated. Conclusions can also inform future planning and decision-making, by identifying areas for improvement and highlighting successful strategies or interventions. Overall, conclusions are a crucial component of the evaluation process, as they help stakeholders make informed decisions about the programs and initiatives they are involved in.
1.4 Examples of Conclusion
Here are some examples of conclusions in M&E:
- Based on the data collected, it can be concluded that the program was successful in achieving its objective of increasing access to clean water in the target communities.”
- “The data indicates that the program’s training curriculum is ineffective and in need of revision in order to better meet the needs of participants.”
- “It can be concluded that the program’s community mobilization efforts were successful in increasing community participation and ownership of the program.”
- “Based on the data collected, it is concluded that the program’s impact on improving maternal and child health outcomes is limited and further efforts are needed to address the underlying health system and infrastructure issues.”
- “The data collected indicates that the program’s impact on reducing poverty in the target area is modest, but still significant, and further investment in complementary programs may be needed to achieve more substantial reductions in poverty rates.”
- These conclusions are based on the evidence presented in the findings and represent the interpretation or explanation of the meaning of the findings. They help to provide insight into the impact and effectiveness of the program and can be used to make recommendations for improvement.
1.5 Recommendation
A recommendation is a specific action or set of actions proposed based on the findings and conclusions of the evaluation. Recommendations should be practical, feasible, and tailored to the needs of the stakeholders who will be implementing them. They should be supported by evidence and aligned with the goals of the program, project, or intervention being evaluated.
Recommendations often provide guidance on how to improve the effectiveness or efficiency of the program, project, or intervention, and they can help to inform decision-making and resource allocation. By presenting clear and actionable recommendations, evaluators can help stakeholders identify and prioritize areas for improvement, and develop strategies to address identified issues. Recommendations can also serve as a roadmap for future planning and implementation and can help to ensure that the program or initiative continues to achieve its intended outcomes over time.
Overall, recommendations are an essential component of the evaluation process, as they help to bridge the gap between evaluation findings and programmatic action. By proposing specific and evidence-based actions, evaluators can help to ensure that evaluation results are translated into meaningful improvements in program design, implementation, and outcomes.
1.6 Examples of Recommendation
Here are some examples of recommendations in M&E:
- “To improve the effectiveness of the program’s training, the curriculum should be revised to better meet the needs of participants, with a focus on practical, hands-on learning activities.”
- “To address the data quality issues identified in the monitoring and evaluation system, staff should receive additional training on data collection and management, and the system should be revised to incorporate additional quality control measures.”
- “To build on the success of the program’s community mobilization efforts, further investments should be made in strengthening community-based organizations and networks, and in promoting greater community participation in program planning and decision-making.”
- “To improve the program’s impact on maternal and child health outcomes, efforts should be made to address underlying health system and infrastructure issues, such as improving access to health facilities and training health workers.”
- “To achieve more substantial reductions in poverty rates in the target area, complementary programs should be implemented to address issues such as economic development, education, and social protection.”
These recommendations are specific actions that can be taken based on the findings and conclusions of the M&E process. They should be practical, feasible, and based on the evidence presented in the evaluation report. By implementing these recommendations, development practitioners can improve program effectiveness and impact, and better meet the needs of the target population.
2. Why It Matters: Understanding the Importance of Differentiating between Findings, Conclusions, and Recommendations in M&E
Differentiating between findings, conclusions, and recommendations is crucial in M&E for several reasons. First, it ensures accuracy and clarity in the evaluation report. Findings, conclusions, and recommendations are distinct components of an evaluation report, and they serve different purposes. By clearly defining and differentiating these components, evaluators can ensure that the report accurately reflects the program’s strengths and weaknesses, potential areas for improvement, and the evidence supporting the evaluation’s conclusions.
Second, differentiating between findings, conclusions, and recommendations helps to facilitate evidence-based decision-making. By clearly presenting the evidence supporting the evaluation’s findings and conclusions, and making recommendations based on that evidence, evaluators can help program managers and policymakers make informed decisions about program design, implementation, and resource allocation.
Finally, differentiating between findings, conclusions, and recommendations can help to increase the credibility and trustworthiness of the evaluation report. Clear and accurate reporting of findings, conclusions, and recommendations helps to ensure that stakeholders understand the evaluation’s results and recommendations, and can have confidence in the evaluation’s rigor and objectivity.
In summary, differentiating between findings, conclusions, and recommendations is essential in M&E to ensure accuracy and clarity in the evaluation report, facilitate evidence-based decision-making, and increase the credibility and trustworthiness of the evaluation.
3. How to Identify and Distinguish between Findings, Conclusions, and Recommendations in M&E
Identifying and distinguishing between findings, conclusions, and recommendations in M&E requires careful consideration of the evidence and the purpose of each component. Here are some tips for identifying and distinguishing between findings, conclusions, and recommendations in M&E:
- Findings: Findings are the results of the data analysis and should be objective and evidence-based. To identify findings, look for statements that summarize the data collected and analyzed during the evaluation. Findings should be specific, measurable, and clearly stated.
- Conclusions: Conclusions are interpretations of the findings and should be supported by the evidence. To distinguish conclusions from findings, look for statements that interpret or explain the meaning of the findings. Conclusions should be logical and clearly explained, and should take into account any limitations of the data or analysis.
- Recommendations: Recommendations are specific actions that can be taken based on the findings and conclusions. To distinguish recommendations from conclusions, look for statements that propose actions to address the issues identified in the evaluation. Recommendations should be practical, feasible, and clearly explained, and should be based on the evidence presented in the findings and conclusions.
It is also important to ensure that each component is clearly labeled and presented in a logical order in the evaluation report. Findings should be presented first, followed by conclusions and then recommendations.
In summary, identifying and distinguishing between findings, conclusions, and recommendations in M&E requires careful consideration of the evidence and the purpose of each component. By ensuring that each component is clearly labeled and presented in a logical order, evaluators can help to ensure that the evaluation report accurately reflects the program’s strengths, weaknesses, and potential areas for improvement, and facilitates evidence-based decision-making.
4. How to Communicate Findings, Conclusions, and Recommendations Effectively in M&E Reports
Communicating findings, conclusions, and recommendations effectively in M&E reports is critical to ensuring that stakeholders understand the evaluation’s results and recommendations and can use them to inform decision-making. Here are some tips for communicating findings, conclusions, and recommendations effectively in M&E reports:
- Use clear and concise language: Use clear, simple language to explain the findings, conclusions, and recommendations. Avoid technical jargon and use examples to illustrate key points.
- Present data visually: Use tables, graphs, and charts to present data visually, making it easier for stakeholders to understand and interpret the findings.
- Provide context: Provide context for the findings, conclusions, and recommendations by explaining the evaluation’s purpose, methodology, and limitations. This helps stakeholders understand the scope and significance of the evaluation’s results and recommendations.
- Highlight key points: Use headings, bullet points, and other formatting techniques to highlight key points, making it easier for stakeholders to identify and remember the most important findings, conclusions, and recommendations.
- Be objective: Present the findings, conclusions, and recommendations objectively and avoid bias. This helps to ensure that stakeholders have confidence in the evaluation’s rigor and objectivity.
- Tailor the report to the audience: Tailor the report to the audience by using language and examples that are relevant to their interests and needs. This helps to ensure that the report is accessible and useful to stakeholders.
In summary, communicating findings, conclusions, and recommendations effectively in M&E reports requires clear and concise language, visual presentation of data, contextualization, highlighting of key points, objectivity, and audience-tailoring. By following these tips, evaluators can help to ensure that stakeholders understand the evaluation’s results and recommendations and can use them to inform decision-making.
5. The Benefits of Clear and Accurate Reporting of Findings, Conclusions, and Recommendations in M&E
Clear and accurate reporting of M&E findings, conclusions, and recommendations has many benefits for development programs and policies. One of the most significant benefits is improved program design and implementation. By clearly identifying areas for improvement, program designers and implementers can make adjustments that lead to more effective and efficient programs that better meet the needs of the target population.
Another important benefit is evidence-based decision-making. When M&E findings, conclusions, and recommendations are reported accurately and clearly, decision-makers have access to reliable information on which to base their decisions. This can lead to more informed decisions about program design, implementation, and resource allocation.
Clear and accurate reporting of M&E findings, conclusions, and recommendations also supports accountability. By reporting transparently on program performance, development practitioners can build trust and support among stakeholders, including program beneficiaries, donors, and the general public.
M&E findings, conclusions, and recommendations also support continuous learning and improvement. By identifying best practices, lessons learned, and areas for improvement, development practitioners can use this information to improve future programming.
Finally, clear and accurate reporting of M&E findings, conclusions, and recommendations can increase program impact. By identifying areas for improvement and supporting evidence-based decision-making, development programs can have a greater positive impact on the communities they serve.
In summary, clear and accurate reporting of M&E findings, conclusions, and recommendations is critical for improving program design and implementation, supporting evidence-based decision-making, ensuring accountability, supporting continuous learning and improvement, and increasing program impact. By prioritizing clear and accurate reporting, development practitioners can ensure that their programs are effective, efficient, and have a positive impact on the communities they serve.
Very interesting reading which clearly explain the M&E finding, recommendation and conclusion, which sometimes the terms can be confusing
Leave a Comment Cancel Reply
Your email address will not be published.
How strong is my Resume?
Only 2% of resumes land interviews.
Land a better, higher-paying career
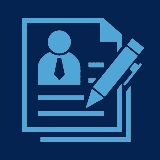
Jobs for You
Business development associate.
- United States
Director of Finance and Administration
- Bosnia and Herzegovina
Request for Information – Collecting Information on Potential Partners for Local Works Evaluation
- Washington, USA
Principal Field Monitors
Technical expert (health, wash, nutrition, education, child protection, hiv/aids, supplies), survey expert, data analyst, team leader, usaid-bha performance evaluation consultant.
- International Rescue Committee
Manager II, Institutional Support Program Implementation
Senior human resources associate, energy and environment analyst – usaid bureau for latin america and the caribbean, intern- international project and proposal support, ispi, deputy chief of party, senior accounting associate, services you might be interested in, useful guides ....
How to Create a Strong Resume
Monitoring And Evaluation Specialist Resume
Resume Length for the International Development Sector
Types of Evaluation
Monitoring, Evaluation, Accountability, and Learning (MEAL)
LAND A JOB REFERRAL IN 2 WEEKS (NO ONLINE APPS!)
Sign Up & To Get My Free Referral Toolkit Now:
Thank you for visiting nature.com. You are using a browser version with limited support for CSS. To obtain the best experience, we recommend you use a more up to date browser (or turn off compatibility mode in Internet Explorer). In the meantime, to ensure continued support, we are displaying the site without styles and JavaScript.
- View all journals
- My Account Login
- Explore content
- About the journal
- Publish with us
- Sign up for alerts
- Open access
- Published: 17 May 2024
Association of major and minor ECG abnormalities with traditional cardiovascular risk factors in the general population: a large scale study
- Toktam Sahranavard 1 ,
- Rasoul Alimi 2 na1 ,
- Javad Arabkhazaei 1 na1 ,
- Mohamad Nasrabadi 3 ,
- Seyyed Mohammad Matin Alavi Dana 3 ,
- Yazdan Gholami 3 ,
- Azadeh Izadi-Moud 4 ,
- Habiobollah Esmaily 5 ,
- Mahmoud Ebrahimi 6 ,
- Gordon A. Ferns 7 ,
- Mohsen Moohebati 4 , 8 ,
- Sara Saffar Soflaei 1 &
- Majid Ghayour Mobarhan 1
Scientific Reports volume 14 , Article number: 11289 ( 2024 ) Cite this article
Metrics details
- Medical research
Cardiovascular disease (CVD) can be determined and quantified using the electrocardiogram (ECG) analysis. Identification of the risk factors associated with ECG abnormalities may advise prevention approaches to decrease CVD burden. In this study we aimed to investigate the association between CVD risk factors and minor and major ECG abnormalities in a general Iranian adult population. This study was conducted in 2010 and covered a population of 9035 males and females aged 35 to 65 years recruiting from the phase I of Mashhad Stroke and Heart Atherosclerotic Disorder (MASHAD) cohort study. The participants were drawn by a stratified cluster random sampling technique. The Bivariate and multinomial logistic regression analysis were conducted considering gender stratification to explore the association of ECG abnormalities with traditional cardiovascular risk factors. There was a significant association between minor and major ECG abnormalities and hypertension (HTN), type 2 diabetes (T2DM), smoking, and physical activity ( p < 0.005). There was a significant trend, in both genders, for increasing major abnormalities as the number of CVD risk factors increased. But, only in women, the minor abnormalities increase in frequency as the number of CVD risk factors increased. The results of multinomial logistic regression showed that men with HTN [ARRR = 1.25, 95% CI 0.99, 1.57] and T2DM [ARRR = 1.31, 95% CI 0.99, 1.74] had the highest likelihood to have major abnormalities, although these are not statistically significant. For women, those with HTN had the highest likelihood to have major [ARRR = 1.36, 95% CI 1.13, 1.63] and minor [ARRR = 1.35, 95% CI 1.15, 1.58] abnormalities. Also, women aged > 60 years were more likely to have major [ARRR = 2.01, 95% CI 1.49, 2.74] and minor [ARRR = 1.59, 95% CI 1.20, 2.10] abnormalities compared to women aged < 45 years. Age and HTN were significantly associated with major and minor ECG abnormalities in women, and, on the other hand, HTN and T2DM were associated with major abnormalities in men. Taken together, these findings suggest that healthcare providers should advise preventive approaches to the asymptomatic adults with both major and minor electrocardiographic abnormalities that may predict cardiovascular risk.
Similar content being viewed by others
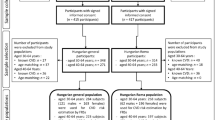
Comparative risk assessment for the development of cardiovascular diseases in the Hungarian general and Roma population
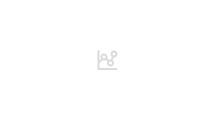
Prevalence and associated factors of electrocardiographic left ventricular hypertrophy in a rural community, central Thailand
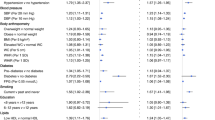
Sex differences in risk factors for coronary heart disease events: a prospective cohort study in Iran
Introduction.
Cardiovascular diseases (CVDs) represent the most widespread non-communicable diseases and hold the position of being the primary cause of mortality on a global scale 1 . According to a report by the World Health Organization, CVDs accounted for 32% of all recorded deaths 2 . The organization also reported an increasing trend in the mortality rate of CVDs, with an expected rise from 16.7 million deaths in 2010 to a forecasted 23.3 million deaths by the year 2030 3 . It is believed that over half of cardiovascular death can be attributed to the presence of following prominent risk factors: aging, hypercholesterolemia, hypertension (HTN), type 2 diabetes (T2DM), obesity, and smoking 4 . The prevalence of many risk factors of CVDs is increasing, particularly in developing regions 5 .
Despite the continuous progression of new technologies for the diagnostic evaluation of patients with CVD, the electrocardiogram (ECG) remains an essential tool 6 . Several studies have demonstrated promising prospects of utilizing the Minnesota ECG Code Classification System for the diagnosis of clinical types of coronary artery disease and heart failure, in addition to prognosticating CVD mortality 7 , 8 , 9 .
The ECG is a good candidate to use for risk stratification of apparently healthy participants due to its safety, wide availability, and low cost. Whereas routine ECG performance among healthy adults is not supported by present evidence 10 . If current CVD risk assessment tools could be enhanced, treatment might be improved, thereby maximizing the advantages of and minimizing the disadvantages of screening 11 .
Some studies reported the association of traditional CVD risk factors with ECG abnormalities 12 , 13 . However, it is not clear whether and to what extent these factors are associated with abnormal ECG changes in Iranian population. Identifying the groups at increased risk for ECG abnormalities may advise prevention approaches focused on modifiable risk factors to decrease the ECG abnormalities burden, which may in turn develop CVD prevention. This study was designed to explore the association of traditional CVD risk factors and major and minor ECG abnormalities on a large community in Iran.
Study population
This cross‐sectional study was carried out on the population of the first phase of Mashhad stroke and heart atherosclerotic disorder (MASHAD) cohort study. The MASHAD cohort study was initiated in 2010, consisting of 9704 participants aged 35 to 65 years. The population study were drawn from three regions in Mashhad by a stratified cluster random sampling technique. Details of the study design and sampling methods have been published previously 14 . Among the 9704 subjects, the ECGs of 9035 subjects were available for final analysis including 3615 (40.0%) males and 5420 (60.0%) females.
Anthropometric assessments
Height, weight, and body mass index (BMI) were assessed regarding the standardized protocols 15 in all subjects. Height (cm) was recorded to the nearest millimetre using a tape measure. Weight (kg) was measured to the nearest 0.1 kg using electronic scales. The BMI was evaluated by dividing weight (kg) to height squared (m 2 ) 15 . Obesity was considered when BMI ≥ 30 kg/m 2 16 .
Laboratory evaluation
Blood samples of all subjects were gathered after a 14 h overnight fasting. Serum total cholesterol (TC) and fasting blood glucose (FBG) were estimated using enzymatic methods on an automated analyser. Hypercholesterolemia was defined as TC ≥ 240 mg/dl 17 . T2DM was described as FBG ≥ 126 mg/dl or previously diagnosed Type 2 T2DM 18 .
Blood pressure assessment
Systolic blood pressure (SBP) and diastolic blood pressure (DBP) were measured by standard mercury sphygmomanometers. HTN was defined as a SBP ≥ 140 mmHg or a DBP ≥ 90 mmHg or a history of HTN 19 .
Assessment of physical activity
The questionnaire utilized for evaluating physical activity was regarding the James and Schofield human energy requirements equations and was filled out by each participant. Questions were classified into three sections including the activities in work hours, off time, and in bed 20 . Individuals with 1–1.39 physical activity level were classified in inactive group and those who had PAL more than 1.4 were categorized as active ones 21 .
Assessment of other variables
Demographic and socioeconomic features (e.g. age, gender, education, and occupation status) and smoking (current smoker, ever smoker and never smoker) for all subjects were recorded by health care professionals and a nurse interview.
Electrocardiographic measures
A standard resting 12-lead ECG at a 25 mm/s paper speed and at 10 mm/mV was digitally recorded. All ECGs were inspected visually to detect technical errors, missing leads, and inadequate quality, and such records were rejected from electrocardiographic data files.
The readable ECGs (n = 9035) were subsequently examined and recorded by trained senior medical students regarding the Minnesota coding (MC) system 22 , 23 . ECG abnormalities were divided as major and minor abnormalities based on the standards of the MC for ECG classification 22 . Major ECG abnormalities were defined as any of the following: major Q wave abnormalities (MC 1-2, 1-2), Minor Q wave abnormalities Plus ST-T abnormalities (MC 4-1 or 4-2, or 5-1 or 5-2), Major isolated ST-T abnormalities (MC 4-1 or 4-2 or 5-1 or 5-2), complete or intermittent left bundle branch block (LBBB) (MC 7-1), right bundle branch block (RBBB) (MC 7-2), nonspecific intraventricular block (MC 7-4), RBBB with left anterior hemiblock (MC 7-8), Brugada pattern (MC 7-9), left ventricular hypertrophy plus ST-T abnormalities (MC 3-1 plus MC 4-1 or 4-2), Major QT index ≥ 116%, where QT Index = [QT interval × (heart rate + 100)/656] 24 , atrial fibrillation or flutter (MC 8-3), Third-degree AV conduction (AVB3) (MC 6-1), second-degree AV block (AVB2) (MC 6-2), WPW (MC 6-4), artificial pacemaker (MC 6-8), ventricular fibrillation or ventricular asystole (MC 8-2), supraventricular tachycardia (SVT) (MC 8-4-2 or MC 8-4-1 with Heart rate > 140). Minor abnormalities included: minor isolated Q/QS waves (MC 1-3), minor ST/T abnormalities (MC 4-3, 4-4, 5-3, 5-4), high R wave (left ventricular) (MC 3-1, 3-3, 3-4), high R wave (right ventricular) (MC 3-2), ST segment elevation (MC 9-2), incomplete RBBB (MC 7-3), incomplete LBBB (MC 7-6, 7-7), minor QT prolongation (QT index ≥ 112%), short PR interval (MC 6-5), long PR interval (MC 6-3), left axis deviation (MC 2-1), right axis deviation (MC 2-2), premature supraventricular beats (MC 8-1-1),premature ventricular beats (MC 8-1-2), premature combined beats (MC 8-1-3, 8-1-5), wandering atrial pacemaker (MC 8-1-4), sinus tachycardia (MC 8-7), sinus bradycardia (MC 8-8), supraventricular rhythm persistent (MC 8-4-1), low voltage QRS (MC 9-1), high amplitude P wave (MC 9-3), left atrial enlargement (LAE) (MC 9-6-), fragmented QRS (MC 7-1-0). Participants with both major and minor abnormalities were classified as having major abnormalities. Participants without minor or major ECG abnormalities were classified as having marginal or no abnormalities and their ECG was considered normal 10 .
Statistical analysis
In this study, both descriptive, bivariate and multinomial logistic regression analysis were conducted considering gender stratification. The quantitative results were stated as mean and standard deviation and the qualitative results as frequency and percentage. Independent t-test and chi-square tests were used to compare the variables by sex. Bonferroni adjustments was used for multiple testing. Additionally, Multinomial logistic regression model was employed because the dependent variable had three outcomes (normal, minor and major). The results for the multinomial logistic regression analyses were presented as relative risk ratios (RRR) with their respective 95% confidence intervals (CIs) signifying precision. Also, to eliminate the effect of confounders, adjusted relative risk ratios (ARRR) along with their respective 95% confidence intervals (CIs) were used. All the analyses were done with SPSS version 26.
Ethical approval and consent of participant
The Human Research Ethics Committee of Mashhad University of Medical Sciences (MUMS) reviewed and approved the study (IR.MUMS.MEDICAL.REC.1399.783). All subjects provided written informed consent. For illiterate participants, their literate spouse or children read and sign the form. All study procedures were conducted according to the World Medical Association Declaration of Helsinki ethical standards for medical research 25 .
Baseline characteristics of the participants by gender is showed in Table 1 . Average (± SD) of the age was 48.91 ± 8.40 years in men and 47.60 ± 8.09 years in women, respectively.
The relationship between abnormal ECG changes and cardiovascular risk factors is presented in Table 2 . There was a considerable association between minor and major abnormalities and HTN, T2DM, smoking, and physical activity.
The comparison of the major and minor ECG abnormalities by CVD risk status or disease in men and women is shown in Tables 3 and 4 . There was a significant trend, in both men and women, for increasing major abnormalities as the number of CVD risk factors increased. But, only for women, minor abnormalities increased as the number of CVD risk factors increased. The results showed that men with more than 3 CVD risk factors were more likely [RRR = 1.39, 95% CI 1.01, 1.93] to have major abnormalities compared to those with no CVD risk factor. Also, women with 1 and 2 risk factor and more than 3 CVD risk factors were more likely [RRR = 1.21, 95% CI 1.01, 1.45] and [RRR = 1.41, 95% CI 1.10, 1.81] to have major abnormalities compared to those with no CVD risk factor, respectively. Minor abnormalities increased about 50 percent [RRR = 1.50, 95% CI 1.21, 1.85] for women with more than 3 CVD risk factors compared to those with no CVD risk factor.
Tables 5 and 6 present the results on the multinomial logistic regression analysis on ECG abnormalities among men and women.
With no abnormalities (normal) as the base outcome, the results showed that men with HTN had the highest likelihood [by 25%] to have major abnormalities, although it is not statistically significant. Also, those with T2DM had the highest likelihood [by 31%] to have major abnormalities, although it is not statistically significant.
Women aged 45–60 and > 60 years were more likely [by 34%] and [About 2 times] to have higher risk of major abnormalities compared to those aged < 45, respectively.
Also, those with HTN had the highest likelihood [by 36%] to have major abnormalities.
Women aged 45–60 and > 60 years were more likely [by 24%] and [by 59%] to have higher risk of minor abnormalities compared to those aged < 45, respectively. Women illiterate and primary education were more likely [by 45%] and [by 52%] to have minor abnormalities compared to those with diploma an above, respectively. Also, those with HTN had the highest likelihood [by 35%] to have minor abnormalities.
The main findings of the Tables 5 and 6 are shown in Fig. 1 . Figure 1 showed the adjusted relative risk ratios and confidence intervals for minor and major ECG abnormalities in male and female.
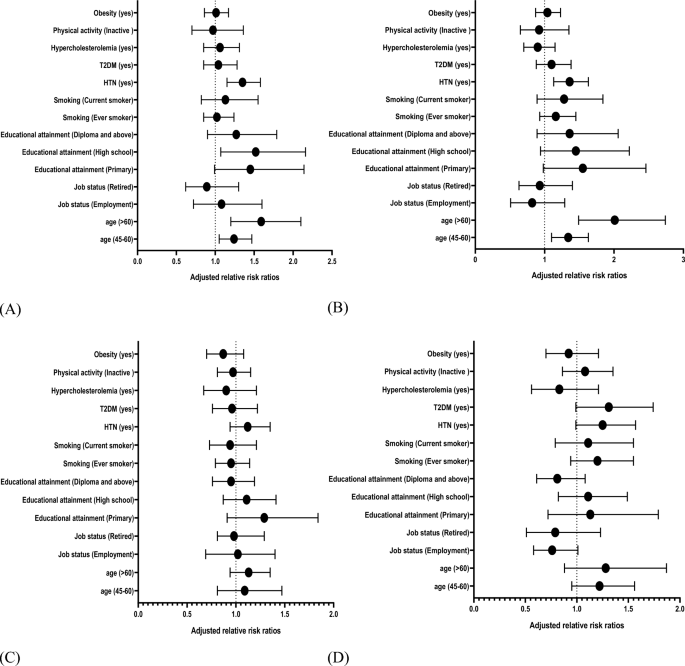
Adjusted relative risk ratios and confidence intervals of predictor variables for ECG abnormalities by gender. ( A ) Minor ECG abnormalities in female. ( B ) Major ECG abnormalities in female. ( C ) Minor ECG abnormalities in male. ( D ) Major ECG abnormalities in male.
Our results showed that in a population-based study of men and women, minor and major ECG abnormalities were associated with age, history of HTN, and T2DM. An interesting finding of our study was that the major ECG abnormalities increased as the number of CVD risk factors increased in both men and women. But, only for women, minor abnormalities increased as the number of CVD risk factors increased.
After multinomial logistic regression analysis, only age and HTN remained significantly associated with major and minor ECG abnormalities in females. In males, age, HTN and T2DM were associated with major abnormalities. Although these associations were not statistically significant, they are clinically important.
The risk of CVD differs between men and women due to a protective impact of sex steroid hormones in women, particularly estrogen 26 . Therefore, we did all analysis in both genders separately.
This study showed that minor and major ECG abnormalities increased with aging in women. In line with our research, In both genders, the odds of presenting major ECG abnormalities surge with a rise in age 27 , 28 . The etiology of the observed ECG variations between age groups are not completely identified. These ECG differences between age groups may be contributed to developmental, electrical, and structural conduction system alternations in the heart throughout life 29 . Moreover, differences in the atherosclerosis and other CVDs risks, comorbidities, medications, and lifestyle parameters can also affect ECG components at different ages 28 , 30 .
HTN is one of the main risk factors for CVD. In our investigation, it was shown that HTN has a remarkable association with both minor and major ECG abnormalities in women. A similar study indicated that the ECG abnormalities were more commonly observed in the hypertensive group rather than the normotensive group 31 . In addition, a review study reported a significant association between HTN and ECG abnormalities including QTc prolongation and QRS wave fragmentation 32 . Our finding supports that evidence declaring that hypertensive condition can results in cardiac structure and electrical abnormalities 33 .
T2DM is another risk factor of CVD that is associated with major ECG abnormalities in men. In consistent with our results, Sahil et al. investigated the prevalence of ECG abnormalities in asymptomatic diabetic patients. Twenty-six percent of diabetic patients had ECG abnormalities whereas in the control group, no ECG abnormalities were reported 34 . In another research, minor and major ECG abnormalities were common in all diabetics (29.1%), including patients with no CVD history (24%) 35 . A small study in Sub-Sahara Africa mentioned conduction defects (11.9%) and arrhythmias (16.2%) as the two most common ECG abnormalities among diabetic patients 36 . The high prevalence of these two abnormalities might be attributed to missed ischemic heart daises and/or contractile disorders as consequence of disturbed Ca 2+ handing triggered by T2DM, a suggested mechanism of diabetic cardiomyopathy 37 .
Physical activity levels were associated with minor and major abnormalities in this study. Commonly, physical activity is advised for the prevention of CVD. However, some electrical and structural changes occur in the heart of athletes secondary to intense exercise including sinus bradycardia, early repolarization, prolongation of QT interval, and first-degree atrioventricular block 38 , 39 . In our study, smoking was not significantly associated with major and minor ECG abnormalities. A study by Michelle et al. showed that ECG abnormalities are more common in cigarette smokers compared to the healthy individuals 40 . In another investigation, the ECG of 532 smokers recorded in the baseline and after 3 years. Major ECG abnormalities significantly were related to higher pack-years. Almost 43 percent of participants quit smoking after three years 41 . The reason for this discrepancy is not clear but it may be due to the number of pack-years in participants and the duration of being smoker. Further studies, which take these variables into account, will need to be undertaken.
We didn’t find any association between minor and major ECG abnormalities with obesity and hypercholesterolemia. In line with our study, two other studies also denied any association between left ventricular hypertrophy patterns in ECG and obesity 42 , 43 . However, it is previously identified that obese cases may have ECG changes including, low QRS voltage, left ventricular hypertrophy, and leftward shifts of the P wave QRS and T wave axes 44 . An analysis of 302 women aged 50 to 69 years showed that abdominal obesity and hyperlipidemia had no association with ventricular repolarization 45 .
In spite of our findings, obesity was reported to be associated with the prolongation of PR interval and the widening of QRS 46 . This disparity may be due to the heterogeneity of our data or confounding variables. In addition, there are different types of obesity phenotypes including metabolic healthy and metabolic unhealthy obesity which didn’t separate in this research. Researchers showed that poor metabolic health is more important than BMI and fat mass for the development of CVD 47 . According to current evidence, the metabolic unhealthy obesity phenotype is more predisposed to cardiovascular events 48 . Thus, we suggest future studies to investigate the association of ECG abnormalities with obesity phenotypes.
In another part of this research, the association between specific minor and major ECG abnormalities and the number of CVD risk factors was also analyzed separately. We observed that in men, as the number of CVD risk factors increases, the frequency of the presence of minor isolated Q/QS waves, high R waves (left ventricular), and major abnormalities increase as well. In women, the frequency of minor abnormalities, minor isolated Q/QS waves, minor isolated Q/QS waves, long PR interval, sinus tachycardia, left atrial enlargement, major abnormalities, complete or intermittent RBBB, and nonspecific intraventricular block were associated with the higher number of CVD risk factors. A similar study among 16 415 Hispanic/Latinos reported there was a significant trend for increasing prevalence of minor isolated ST-T abnormalities, left ventricular hypertrophy plus major ST-T abnormality, major Q waves, major isolated ST-T abnormalities, and ventricular conduction defects with the number of CVD risk factors and prevalent CVD in men. In women, minor isolated ST-T abnormalities, minor isolated Q waves, tall R waves, major Q waves, major isolated ST-T abnormalities, left ventricular hypertrophy plus major ST-T abnormality, and major QT prolongation index with the number of CVD risk factors and prevalent CVD 49 .
The key strength of the study is that an in-depth analysis was undertaken to investigate the relationship between CVD risk factors and ECG abnormalities in a large sample size. However, a number of limitations need to be noted regarding the present study. It is a cross-sectional study, so the causal relationship cannot be determined. Also, the individuals over 65 years and/or lower than 35 years were not included in this study. Another limitation is the fact that only one ECG was obtained at the beginning of the study, ECG criteria can be dynamic and could be more useful if multiple ECGs were obtained at various time points.
This study showed that age and HTN significantly associated with major and minor ECG abnormalities in women, and HTN and DM were associated with major abnormalities in men. Therefore, pursuing therapeutic lifestyle modification to decrease blood pressure and blood glucose are realistic and testable approaches to lower the risk of some prognostically important ECG abnormalities.
Data availability
The authors confirm that the data supporting the findings of this study are available from the corresponding author on request.
Banatvala, N. & Bovet, P. Noncommunicable diseases: A compendium (Taylor & Francis, 2023).
Book Google Scholar
(WHO). W. H. O. Cardiovascular diseases (CVDs) (2021).
Soltani, S. et al. Community-based cardiovascular disease prevention programmes and cardiovascular risk factors: A systematic review and meta-analysis. Public Health 200 , 59–70. https://doi.org/10.1016/j.puhe.2021.09.006 (2021).
Article CAS PubMed Google Scholar
Patel, S. A., Winkel, M., Ali, M. K., Narayan, K. M. & Mehta, N. K. Cardiovascular mortality associated with 5 leading risk factors: National and state preventable fractions estimated from survey data. Ann. Intern. Med. 163 , 245–253. https://doi.org/10.7326/m14-1753 (2015).
Article PubMed Google Scholar
Krittayaphong, R. et al. Electrocardiographic predictors of cardiovascular events in patients at high cardiovascular risk: A multicenter study. J. Geriatr. Cardiol. JGC 16 , 630–638. https://doi.org/10.11909/j.issn.1671-5411.2019.08.004 (2019).
Rafie, N., Kashou, A. H. & Noseworthy, P. A. ECG interpretation: Clinical relevance, challenges, and advances. Hearts 2 , 505–513 (2021).
Article Google Scholar
Soliman, E. Z. et al. Usefulness of maintaining a normal electrocardiogram over time for predicting cardiovascular health. Am. J. Cardiol. 119 , 249–255 (2017).
Denes, P., Larson, J. C., Lloyd-Jones, D. M., Prineas, R. J. & Greenland, P. Major and minor ECG abnormalities in asymptomatic women and risk of cardiovascular events and mortality. Jama 297 , 978–985 (2007).
Tamosiunas, A. et al. Trends in electrocardiographic abnormalities and risk of cardiovascular mortality in Lithuania, 1986–2015. BMC Cardiovasc. Disord. 19 , 1–9 (2019).
Auer, R. et al. Association of major and minor ECG abnormalities with coronary heart disease events. Jama 307 , 1497–1505 (2012).
Article CAS PubMed PubMed Central Google Scholar
Curry, S. J. et al. Risk assessment for cardiovascular disease with nontraditional risk factors: US preventive services task force recommendation statement. Jama 320 , 272–280 (2018).
Healy, C. F. & Lloyd-Jones, D. M. Association of traditional cardiovascular risk factors with development of major and minor electrocardiographic abnormalities: A systematic review. Cardiol. Rev. 24 , 163–169. https://doi.org/10.1097/crd.0000000000000109 (2016).
Niu, J. et al. The association and predictive ability of ECG abnormalities with cardiovascular diseases: A prospective analysis. Glob. Heart 15 , 59. https://doi.org/10.5334/gh.790 (2020).
Article PubMed PubMed Central Google Scholar
Ghayour-Mobarhan, M. et al. Mashhad stroke and heart atherosclerotic disorder (MASHAD) study: Design, baseline characteristics and 10-year cardiovascular risk estimation. Int. J. Public Health 60 , 561–572 (2015).
Norton, K. Measurement techniques in anthropometry. Antropometrica (1996).
Purnell, J. Q. Definitions, classification, and epidemiology of obesity. Endotext (2023).
Shrestha, B., Shrestha, S. & Dhital, B. M. Lipid abnormalities in patients suffering from migraine. J. Chitwan Med. Coll. 13 , 49–52 (2023).
World Health Organization. Noncommunicable diseases global monitoring framework: Indicator definitions and specifications (2014).
Giles, T. D., Materson, B. J., Cohn, J. N. & Kostis, J. B. Definition and classification of hypertension: An update. J. Clin. Hypertens. 11 , 611–614 (2009).
Gerrior, S., Juan, W. & Peter, B. An easy approach to calculating estimated energy requirements. Prev. Chronic Dis. 3 , A129 (2006).
PubMed PubMed Central Google Scholar
James, W. P. T. & Schofield, E. C. Human energy requirements. A manual for planners and nutritionists (Oxford University Press, 1990).
Google Scholar
Prineas, R. J., Crow, R. S. & Zhang, Z.-M. The Minnesota code manual of electrocardiographic findings (Springer, 2009).
Saffar Soflaei, S. et al. A large population-based study on the prevalence of electrocardiographic abnormalities: A result of Mashhad stroke and heart atherosclerotic disorder cohort study. Ann. Noninvasive Electrocardiol. 28 , e13086 (2023).
Rautaharju, P. M., Warren, J. W. & Calhoun, H. P. Estimation of QT prolongation: A persistent, avoidable error in computer electrocardiography. J. Electrocardiol. 23 , 111–117 (1990).
World Medical Association. World Medical Association Declaration of Helsinki: Ethical principles for medical research involving human subjects. JAMA 310 , 2191–2194 (2013).
Peters, S. A., Muntner, P. & Woodward, M. Sex differences in the prevalence of, and trends in, cardiovascular risk factors, treatment, and control in the United States, 2001 to 2016. Circulation 139 , 1025–1035 (2019).
Khane, R. S., Surdi, A. D. & Bhatkar, R. S. Changes in ECG pattern with advancing age. J. Basic Clin. Physiol. Pharmacol. 22 , 97–101. https://doi.org/10.1515/JBCPP.2011.017 (2011).
Ahmadi, P. et al. Age and gender differences of basic electrocardiographic values and abnormalities in the general adult population; Tehran Cohort Study. BMC Cardiovasc. Disord. 23 , 1–13 (2023).
Olgar, Y. et al. Aging related functional and structural changes in the heart and aorta: MitoTEMPO improves aged-cardiovascular performance. Exp. Gerontol. 110 , 172–181. https://doi.org/10.1016/j.exger.2018.06.012 (2018).
North, B. J. & Sinclair, D. A. The intersection between aging and cardiovascular disease. Circ. Res. 110 , 1097–1108. https://doi.org/10.1161/circresaha.111.246876 (2012).
Lehtonen, A. O. et al. Prevalence and prognosis of ECG abnormalities in normotensive and hypertensive individuals. J. Hypertens. 34 , 959–966. https://doi.org/10.1097/hjh.0000000000000882 (2016).
Bird, K. et al. Assessment of hypertension using clinical electrocardiogram features: A first-ever review. Front. Med. 7 , 583331 (2020).
Conceição-Vertamatti, A. G. et al. Electrocardiographic abnormalities in hypertension models. Int. J. Cardiovasc. Sci. 33 , 321–328 (2020).
Gupta, S., Gupta, R. K., KulShReStha, M. & ChaudhaRy, R. R. Evaluation of ECG abnormalities in patients with asymptomatic type 2 diabetes mellitus. J. Clin. Diagn. Res. JCDR 11 , OC39 (2017).
PubMed Google Scholar
Harms, P. P. et al. Prevalence of ECG abnormalities in people with type 2 diabetes: The Hoorn diabetes care system cohort. J. Diabetes Complicat. 35 , 107810 (2021).
DZuDiE, A. et al. Prevalence and determinants of electrocardiographic abnormalities in sub-Saharan African individuals with type 2 diabetes: Cardiovascular topic. Cardiovasc. J. Afr. 23 , 533–537 (2012).
Bugger, H. & Abel, E. D. Molecular mechanisms of diabetic cardiomyopathy. Diabetologia 57 , 660–671 (2014).
Mirahmadizadeh, A. et al. The relationship between demographic features, anthropometric parameters, sleep duration, and physical activity with ECG parameters in Fasa Persian cohort study. BMC Cardiovasc. Disord. 21 , 1–11 (2021).
Abela, M. & Sharma, S. Abnormal ECG findings in athletes: clinical evaluation and considerations. Curr. Treat. Options Cardiovasc. Med. 21 , 1–17 (2019).
Ip, M. et al. Tobacco and electronic cigarettes adversely impact ECG indexes of ventricular repolarization: Implication for sudden death risk. Am. J. Physiol. Heart Circ. Physiol. 318 , H1176–H1184 (2020).
Gepner, A. D. et al. Electrocardiographic changes associated with smoking and smoking cessation: Outcomes from a randomized controlled trial. PLoS One 8 , e62311. https://doi.org/10.1371/journal.pone.0062311 (2013).
Article ADS CAS PubMed PubMed Central Google Scholar
Lin, Y.-K. et al. Obesity phenotypes and electrocardiographic characteristics in physically active males: CHIEF study. Front. Cardiovasc. Med. 8 , 738575 (2021).
Muiesan, M. L. et al. Obesity and ECG left ventricular hypertrophy. J. Hypertens. 35 , 162–169 (2017).
Fraley, M., Birchem, J., Senkottaiyan, N. & Alpert, M. Obesity and the electrocardiogram. Obes. Rev. 6 , 275–281 (2005).
Pshenichnikov, I. et al. Association between ventricular repolarization and main cardiovascular risk factors. Scand. Cardiovasc. J. SCJ 45 , 33–40. https://doi.org/10.3109/14017431.2010.532232 (2011).
Sun, G. Z. et al. Association between obesity and ECG variables in children and adolescents: A cross-sectional study. Exp. Ther. Med. 6 , 1455–1462 (2013).
Dobson, R. et al. Metabolically healthy and unhealthy obesity: Differential effects on myocardial function according to metabolic syndrome, rather than obesity. Int. J. Obes. 40 , 153–161. https://doi.org/10.1038/ijo.2015.151 (2016).
Article CAS Google Scholar
Eckel, N. et al. Transition from metabolic healthy to unhealthy phenotypes and association with cardiovascular disease risk across BMI categories in 90 257 women (the Nurses’ Health Study): 30 year follow-up from a prospective cohort study. Lancet Diabetes Endocrinol. 6 , 714–724 (2018).
Denes, P. et al. Major and minor electrocardiographic abnormalities and their association with underlying cardiovascular disease and risk factors in Hispanics/Latinos (from the Hispanic Community Health Study/Study of Latinos). Am. J. Cardiol. 112 , 1667–1675 (2013).
Download references
Acknowledgements
We would like to thank Mashhad University of Medical Sciences for supporting this research.
The collecting of clinical data was financially supported by Mashhad University of Medical Sciences.
Author information
These authors contributed equally: Rasoul Alimi and Javad Arabkhazaei.
Authors and Affiliations
International UNESCO Center for Health-Related Basic Sciences and Human Nutrition, Mashhad University of Medical Sciences, Mashhad, 99199-91766, Iran
Toktam Sahranavard, Javad Arabkhazaei, Sara Saffar Soflaei & Majid Ghayour Mobarhan
Department of Epidemiology and Biostatistics, School of Health, Torbat Heydariyeh University of Medical Sciences, Torbat Heydariyeh, Iran
Rasoul Alimi
Faculty of Medicine, Mashhad University of Medical Sciences, Mashhad, Iran
Mohamad Nasrabadi, Seyyed Mohammad Matin Alavi Dana & Yazdan Gholami
Department of Cardiovascular Diseases, Faculty of Medicine, Mashhad University of Medical Sciences, Mashhad, Iran
Azadeh Izadi-Moud & Mohsen Moohebati
Department of Biostatistics, School of Health, Mashhad University of Medical Sciences, Mashhad, Iran
Habiobollah Esmaily
Vascular and Endovascular Research Center, Faculty of Medicine, Mashhad University of Medical Sciences, Mashhad, Iran
Mahmoud Ebrahimi
Division of Medical Education, Brighton & Sussex Medical School, Falmer, Brighton, Sussex, UK
Gordon A. Ferns
Heart and Vascular Research Center, Mashhad University of Medical Sciences, Mashhad, Iran
Mohsen Moohebati
You can also search for this author in PubMed Google Scholar
Contributions
All authors have read and approved the manuscript. Study concept and design: TS and SSS; data collection: JA, MN, AI, ME; Analysis and interpretation of data: RA and HE; Drafting of the manuscript: TS, SMAD, YG; Critical revision of the manuscript for important intellectual content: GF, MG, SSS, and MM.
Corresponding authors
Correspondence to Mohsen Moohebati , Sara Saffar Soflaei or Majid Ghayour Mobarhan .
Ethics declarations
Competing interests.
The authors declare no competing interests.
Additional information
Publisher's note.
Springer Nature remains neutral with regard to jurisdictional claims in published maps and institutional affiliations.
Rights and permissions
Open Access This article is licensed under a Creative Commons Attribution 4.0 International License, which permits use, sharing, adaptation, distribution and reproduction in any medium or format, as long as you give appropriate credit to the original author(s) and the source, provide a link to the Creative Commons licence, and indicate if changes were made. The images or other third party material in this article are included in the article's Creative Commons licence, unless indicated otherwise in a credit line to the material. If material is not included in the article's Creative Commons licence and your intended use is not permitted by statutory regulation or exceeds the permitted use, you will need to obtain permission directly from the copyright holder. To view a copy of this licence, visit http://creativecommons.org/licenses/by/4.0/ .
Reprints and permissions
About this article
Cite this article.
Sahranavard, T., Alimi, R., Arabkhazaei, J. et al. Association of major and minor ECG abnormalities with traditional cardiovascular risk factors in the general population: a large scale study. Sci Rep 14 , 11289 (2024). https://doi.org/10.1038/s41598-024-62142-8
Download citation
Received : 06 November 2023
Accepted : 14 May 2024
Published : 17 May 2024
DOI : https://doi.org/10.1038/s41598-024-62142-8
Share this article
Anyone you share the following link with will be able to read this content:
Sorry, a shareable link is not currently available for this article.
Provided by the Springer Nature SharedIt content-sharing initiative
By submitting a comment you agree to abide by our Terms and Community Guidelines . If you find something abusive or that does not comply with our terms or guidelines please flag it as inappropriate.
Quick links
- Explore articles by subject
- Guide to authors
- Editorial policies
Sign up for the Nature Briefing newsletter — what matters in science, free to your inbox daily.

Epidemiology of consumer product–related ocular injuries in the geriatric population
Article sidebar, main article content.
Introduction We investigate consumer product-related ocular injuries among the geriatric population presenting to the emergency department (ED) in the United States. Methods The National Electronic Injury Surveillance System (NEISS) is an injury surveillance system based on a nationally representative probability sample of hospitals. Patients ages 65 years and older with ocular injury recorded in the NEISS between 1/1/2010 and 12/31/2021 were included. Patients were divided into four age groups: 65-74 years old (G1), 75-84 (G2), 85-94 (G3), and 95 or older (G4). Sex, race, type of injury, location where injury occurred, year of injury, product category involved, and disposition were compared through Pearson χ 2 . Results A total of 113,408 ED visits for G1, 43,180 for G2, 10,896 for G3, and 1,381 for G4 were included. Statistically greater proportion of ED visits involved female patients in G3 and G4 compared to G1 and G2 (55%, 36%, resp., P < 0.00001). The proportion of White/Non-Hispanic patients declined from 57% in G1 to 53% in G2 and G3 to 41% in G4 ( P < 0.00001). Household items/furnishing and construction tools were responsible for the majority of injuries (60%) and proportion increased with age (58% in G1, 61% in G2, 71% in G3, and 81% in G4). 5% of ED visits in G1 and G2 required admission, transfer, or being held for observation, versus 17% in G3 and G4 ( P < 0.00001). The injury occurred in the home setting for the majority of the cases (65%) in the geriatric population (range, 50%-69%). Contusion, abrasion, and foreign body injuries accounted for 59% of cases (range, 45%-63%). Conclusions Our findings suggest that trends exist in epidemiology of consumer product- related ocular injury within the geriatric population, and may assist public health initiatives targeting reduction of ocular injury.
Article Details
- American Medical Association

This work is licensed under a Creative Commons Attribution-NonCommercial-NoDerivatives 4.0 International License .
- Share full article
Advertisement
Supported by
At Last, Detroit Sees Population Grow, New Estimates Show
New census estimates find moderate post-pandemic rebounds for big cities in the Midwest and Northeast.

By Julie Bosman
After decades of painful decline, Detroit’s population grew in 2023, according to new estimates released on Thursday by the Census Bureau.
The increase — to 633,218 from 631,366 residents — was slight, lifting Detroit to slightly below levels of 2021. But the symbolism was meaningful in a city that had hollowed out , year after year, since the days when more than 1.8 million people lived there. City leaders have long promised to reverse Detroit’s long decline in residents brought on by the shrinking of the auto industry, flight to the suburbs and municipal bankruptcy.
The new census estimates showed similar, moderate population rebounds for many big cities in the Midwest and Northeast after previous pandemic-era declines.
In the Northeast, cities with populations of 50,000 or more grew by an average of 0.2 percent after declining an average of 0.3 percent in 2022. In the Midwest, cities of that size grew 0.1 percent in 2023 after declining an average of 0.2 percent the year before.
Some major cities that saw population dips in 2021 — including New York City, Los Angeles and Chicago — largely returned to prepandemic trends, with increased rates of growth and smaller population declines than during the pandemic.
“We know that people moved out of bigger cities and into smaller cities during the pandemic,” said Andrew Beveridge, the president of Social Explorer, a demographic firm. “That’s abated somewhat.”
Robust growth in cities in the South and Sun Belt continued in 2023, with Southern cities growing an average of 1 percent.
“Thirteen of the 15 fastest-growing cities were in the South, with eight in Texas alone,” Crystal Delbé, a statistician in the Census Bureau’s population division, said in a statement.
The largest 15 cities in 2023 stayed the same as in 2022, though some moved up or down on the list: Jacksonville, Fla., climbed higher than Austin, Texas; and Fort Worth, Texas, grew larger than San Jose, Calif.
Midsize cities, with populations between 10,000 and 50,000 people, saw growth in every region but the Northeast.
Mayor Mike Duggan of Detroit said that the new population estimate for his city was the first time since 1957 that the Census Bureau has not found the city to have lost population. According to census estimates, Detroit is now the 26th most populous city in the nation, up from the 29th in 2022. Decades ago, the city was the nation’s fourth most populous.
The administration of Mr. Duggan, who was elected mayor in 2013 and is serving his third term, has in the past raised questions about the Census Bureau’s population counts in Detroit, claiming that city residents were undercounted. For Mr. Duggan, the new population estimate fulfills a campaign promise he made before his first term to get the city growing again.
“I have said all along, A mayor ought to be judged by whether the population is going up or going down,” he said in an interview. “It took me longer to get there than I hoped.”
Mr. Duggan said that while Detroit is still facing some of the same challenges — like faltering demand for office space — as many larger cities are, the population growth is the result of years of steady, difficult work.
In the last decade, more than 10,000 houses have been renovated, property values have gone up, and a new auto plant to produce Jeeps was built in the city.
Julie Bosman is the Chicago bureau chief for The Times, writing and reporting stories from around the Midwest. More about Julie Bosman

IMAGES
VIDEO
COMMENTS
Defining a population. A sample is a concrete thing. You can open up a data file, and there's the data from your sample. A population, on the other hand, is a more abstract idea.It refers to the set of all possible people, or all possible observations, that you want to draw conclusions about, and is generally much bigger than the sample. In an ideal world, the researcher would begin the ...
If similar findings are observed across multiple studies, this increases confidence in the applicability of the results to the broader population. In conclusion, understanding the concept of population and its implications for scientific research is crucial for ensuring the validity, reliability, and generalizability of study findings.
So if you want to sample one-tenth of the population, you'd select every tenth name. In order to know the k for your study you need to know your sample size (say 1000) and the size of the population (75000). You can divide the size of the population by the sample (75000/1000), which will produce your k (750).
A population is the entire group that you want to draw conclusions about.. A sample is the specific group that you will collect data from. The size of the sample is always less than the total size of the population. In research, a population doesn't always refer to people. It can mean a group containing elements of anything you want to study, such as objects, events, organizations, countries ...
The conclusion is intended to help the reader understand why your research should matter to them after they have finished reading the paper. A conclusion is not merely a summary of the main topics covered or a re-statement of your research problem, but a synthesis of key points derived from the findings of your study and, if applicable, where you recommend new areas for future research.
Our conclusion is a claim about the population. Figure 15.2.1 15.2. 1: Inference from Sample to Population. For example, we might draw a conclusion about the divorce rate of people living in Florida from premises describing the divorce rates among 800 couples that our school's Human Relations Department sampled.
The conclusion in a research paper is the final section, where you need to summarize your research, presenting the key findings and insights derived from your study. ... between social media and mental health to develop effective interventions and support systems for this vulnerable population. Editorial Conclusion: Environmental impact of ...
The study reviewed coffee habits of more than 402,000 people ages 50 to 71 from six states and two metropolitan areas. Those with cancer, heart disease, and stroke were excluded at the start of the study. Coffee consumption was assessed once at the start of the study. About 52,000 people died during the course of the study.
Here are a few best practices: Your results should always be written in the past tense. While the length of this section depends on how much data you collected and analyzed, it should be written as concisely as possible. Only include results that are directly relevant to answering your research questions.
Table of contents. Step 1: Restate the problem. Step 2: Sum up the paper. Step 3: Discuss the implications. Research paper conclusion examples. Frequently asked questions about research paper conclusions.
The population is the ENTIRE group we are interested in, and as phrased that means all the out-state-traffic on the whole bridge. ... To draw valid conclusions, statistical analysis requires careful planning from the very start of the research process. You need to specify your hypotheses and make decisions about your research design, sample ...
It can be defined as the extension of research findings and conclusions from a study conducted on a sample population to the population at large. While the dependability of this extension is not absolute, it is statistically probable. Because sound generalizability requires data on large populations, quantitative research -- experimental for ...
Conclusion: This section provides a summary of the key findings and the main conclusions of the study. ... Research findings are often intended to be generalizable to a larger population or context beyond the specific study. This means that the findings can be applied to other situations or populations with similar characteristics.
Begin with a clear statement of the principal findings. This will reinforce the main take-away for the reader and set up the rest of the discussion. Explain why the outcomes of your study are important to the reader. Discuss the implications of your findings realistically based on previous literature, highlighting both the strengths and ...
a subset of the populat ion, known as the sample, to represent the entire population and draw conclusions from their findings. Sample in Research . In research, a sample refers to a subset of the population that is selected for study purposes. The sample represents a smaller, manageable group that researchers use to make inferences and draw ...
On the other hand, we can rely on simulation to determine the sampling distribution for many statistics, and we can do so using either the population data if we have it or using the sample data to approximate the sampling distribution. We specifically examined how properties of the sampling distribution vary depending on characteristics, including:
A sample population is therefore taken and results from this sample are then generalized to the target population. The sample should be representative of the target population from which it came. Knowing the baseline characteristics of the sample population is important because this allows researchers to see how closely the subjects match their ...
6 Conciseness. Above all, every research paper conclusion should be written with conciseness. In general, conclusions should be short, so keep an eye on your word count as you write and aim to be as succinct as possible. You can expound on your topic in the body of your paper, but the conclusion is more for summarizing and recapping.
Here are some steps you can follow to write an effective research paper conclusion: Restate the research problem or question: Begin by restating the research problem or question that you aimed to answer in your research. This will remind the reader of the purpose of your study. Summarize the main points: Summarize the key findings and results ...
Increasing population and related pressures, combined with a realization of the interconnectedness and complexity of environmental systems, present new challenges to policymakers and regulators. Scientific research has played, and will continue to play, an essential part in solving environmental problems.
The target population consists of all the individuals in the world, or in the United States, with the same characteristics as the sample to which we would like to apply the conclusions of a study. Because it is unrealistic to perform research on all individuals on earth or in the United States or in one state, we settle on a subset, or a sample ...
In research, a finding is an empirical fact, based on data collected, that does not just rely on opinion (even if it is that of an expert); a conclusion synthesizes and interprets the finding and ...
Increased global temperature is associated with a wide range of health risks, which are disproportionately high for certain populations. Individual- and population-level measures can reduce risks.
1.3 Conclusion. A conclusion is a judgment or interpretation of the findings based on the evidence collected during the evaluation. It is typically expressed in terms of what the findings mean or what can be inferred from them. Conclusions should be logical, evidence-based, and free from personal bias or opinion.
Cardiovascular disease (CVD) can be determined and quantified using the electrocardiogram (ECG) analysis. Identification of the risk factors associated with ECG abnormalities may advise prevention ...
IntroductionWe investigate consumer product-related ocular injuries among the geriatric population presenting to the emergency department (ED) in the United States.MethodsThe National Electronic Injury Surveillance System (NEISS) is an injury surveillance system based on a nationally representative probability sample of hospitals. Patients ages 65 years and older with ocular injury recorded in ...
MAY 16, 2024 — Large cities in the Northeast and Midwest grew in 2023, reversing earlier population declines, according to Vintage 2023 Population Estimates released today by the U.S. Census Bureau. Cities with populations of 50,000 or more grew by an average of 0.2% in the Northeast and 0.1% in the Midwest after declining an average of 0.3% and 0.2%, respectively, in 2022.
Conclusions: Targeted prevention interventions aimed at population groups with shared early-life characteristics could have an impact on obesity-hypertension prevalence which are known risk factors for further morbidity including cardiovascular disease. ### Competing Interest Statement R.O. is a member of the National Institute for Health and ...
May 16, 2024. After decades of painful decline, Detroit's population grew in 2023, according to new estimates released on Thursday by the Census Bureau. The increase — to 633,218 from 631,366 ...
Fort Worth's population grew by 2.2 percent, the largest increase in the state. Exclusively Available to Subscribers Try it now for $1. According to another map based on overall population data ...