Ohio State nav bar
The Ohio State University
- BuckeyeLink
- Find People
- Search Ohio State

Research Questions & Hypotheses
Generally, in quantitative studies, reviewers expect hypotheses rather than research questions. However, both research questions and hypotheses serve different purposes and can be beneficial when used together.
Research Questions
Clarify the research’s aim (farrugia et al., 2010).
- Research often begins with an interest in a topic, but a deep understanding of the subject is crucial to formulate an appropriate research question.
- Descriptive: “What factors most influence the academic achievement of senior high school students?”
- Comparative: “What is the performance difference between teaching methods A and B?”
- Relationship-based: “What is the relationship between self-efficacy and academic achievement?”
- Increasing knowledge about a subject can be achieved through systematic literature reviews, in-depth interviews with patients (and proxies), focus groups, and consultations with field experts.
- Some funding bodies, like the Canadian Institute for Health Research, recommend conducting a systematic review or a pilot study before seeking grants for full trials.
- The presence of multiple research questions in a study can complicate the design, statistical analysis, and feasibility.
- It’s advisable to focus on a single primary research question for the study.
- The primary question, clearly stated at the end of a grant proposal’s introduction, usually specifies the study population, intervention, and other relevant factors.
- The FINER criteria underscore aspects that can enhance the chances of a successful research project, including specifying the population of interest, aligning with scientific and public interest, clinical relevance, and contribution to the field, while complying with ethical and national research standards.
- The P ICOT approach is crucial in developing the study’s framework and protocol, influencing inclusion and exclusion criteria and identifying patient groups for inclusion.
- Defining the specific population, intervention, comparator, and outcome helps in selecting the right outcome measurement tool.
- The more precise the population definition and stricter the inclusion and exclusion criteria, the more significant the impact on the interpretation, applicability, and generalizability of the research findings.
- A restricted study population enhances internal validity but may limit the study’s external validity and generalizability to clinical practice.
- A broadly defined study population may better reflect clinical practice but could increase bias and reduce internal validity.
- An inadequately formulated research question can negatively impact study design, potentially leading to ineffective outcomes and affecting publication prospects.
Checklist: Good research questions for social science projects (Panke, 2018)
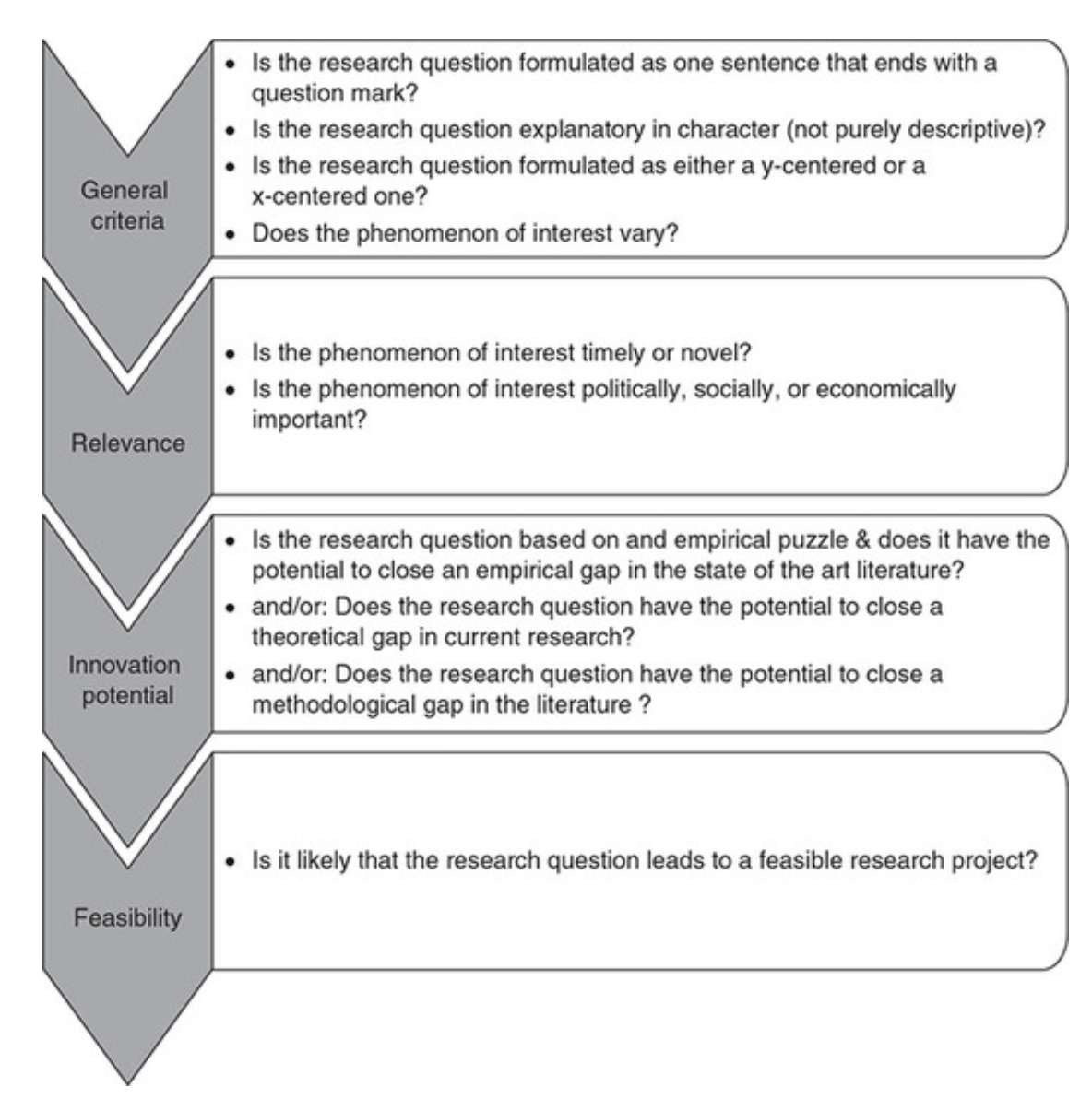
Research Hypotheses
Present the researcher’s predictions based on specific statements.
- These statements define the research problem or issue and indicate the direction of the researcher’s predictions.
- Formulating the research question and hypothesis from existing data (e.g., a database) can lead to multiple statistical comparisons and potentially spurious findings due to chance.
- The research or clinical hypothesis, derived from the research question, shapes the study’s key elements: sampling strategy, intervention, comparison, and outcome variables.
- Hypotheses can express a single outcome or multiple outcomes.
- After statistical testing, the null hypothesis is either rejected or not rejected based on whether the study’s findings are statistically significant.
- Hypothesis testing helps determine if observed findings are due to true differences and not chance.
- Hypotheses can be 1-sided (specific direction of difference) or 2-sided (presence of a difference without specifying direction).
- 2-sided hypotheses are generally preferred unless there’s a strong justification for a 1-sided hypothesis.
- A solid research hypothesis, informed by a good research question, influences the research design and paves the way for defining clear research objectives.
Types of Research Hypothesis
- In a Y-centered research design, the focus is on the dependent variable (DV) which is specified in the research question. Theories are then used to identify independent variables (IV) and explain their causal relationship with the DV.
- Example: “An increase in teacher-led instructional time (IV) is likely to improve student reading comprehension scores (DV), because extensive guided practice under expert supervision enhances learning retention and skill mastery.”
- Hypothesis Explanation: The dependent variable (student reading comprehension scores) is the focus, and the hypothesis explores how changes in the independent variable (teacher-led instructional time) affect it.
- In X-centered research designs, the independent variable is specified in the research question. Theories are used to determine potential dependent variables and the causal mechanisms at play.
- Example: “Implementing technology-based learning tools (IV) is likely to enhance student engagement in the classroom (DV), because interactive and multimedia content increases student interest and participation.”
- Hypothesis Explanation: The independent variable (technology-based learning tools) is the focus, with the hypothesis exploring its impact on a potential dependent variable (student engagement).
- Probabilistic hypotheses suggest that changes in the independent variable are likely to lead to changes in the dependent variable in a predictable manner, but not with absolute certainty.
- Example: “The more teachers engage in professional development programs (IV), the more their teaching effectiveness (DV) is likely to improve, because continuous training updates pedagogical skills and knowledge.”
- Hypothesis Explanation: This hypothesis implies a probable relationship between the extent of professional development (IV) and teaching effectiveness (DV).
- Deterministic hypotheses state that a specific change in the independent variable will lead to a specific change in the dependent variable, implying a more direct and certain relationship.
- Example: “If the school curriculum changes from traditional lecture-based methods to project-based learning (IV), then student collaboration skills (DV) are expected to improve because project-based learning inherently requires teamwork and peer interaction.”
- Hypothesis Explanation: This hypothesis presumes a direct and definite outcome (improvement in collaboration skills) resulting from a specific change in the teaching method.
- Example : “Students who identify as visual learners will score higher on tests that are presented in a visually rich format compared to tests presented in a text-only format.”
- Explanation : This hypothesis aims to describe the potential difference in test scores between visual learners taking visually rich tests and text-only tests, without implying a direct cause-and-effect relationship.
- Example : “Teaching method A will improve student performance more than method B.”
- Explanation : This hypothesis compares the effectiveness of two different teaching methods, suggesting that one will lead to better student performance than the other. It implies a direct comparison but does not necessarily establish a causal mechanism.
- Example : “Students with higher self-efficacy will show higher levels of academic achievement.”
- Explanation : This hypothesis predicts a relationship between the variable of self-efficacy and academic achievement. Unlike a causal hypothesis, it does not necessarily suggest that one variable causes changes in the other, but rather that they are related in some way.
Tips for developing research questions and hypotheses for research studies
- Perform a systematic literature review (if one has not been done) to increase knowledge and familiarity with the topic and to assist with research development.
- Learn about current trends and technological advances on the topic.
- Seek careful input from experts, mentors, colleagues, and collaborators to refine your research question as this will aid in developing the research question and guide the research study.
- Use the FINER criteria in the development of the research question.
- Ensure that the research question follows PICOT format.
- Develop a research hypothesis from the research question.
- Ensure that the research question and objectives are answerable, feasible, and clinically relevant.
If your research hypotheses are derived from your research questions, particularly when multiple hypotheses address a single question, it’s recommended to use both research questions and hypotheses. However, if this isn’t the case, using hypotheses over research questions is advised. It’s important to note these are general guidelines, not strict rules. If you opt not to use hypotheses, consult with your supervisor for the best approach.
Farrugia, P., Petrisor, B. A., Farrokhyar, F., & Bhandari, M. (2010). Practical tips for surgical research: Research questions, hypotheses and objectives. Canadian journal of surgery. Journal canadien de chirurgie , 53 (4), 278–281.
Hulley, S. B., Cummings, S. R., Browner, W. S., Grady, D., & Newman, T. B. (2007). Designing clinical research. Philadelphia.
Panke, D. (2018). Research design & method selection: Making good choices in the social sciences. Research Design & Method Selection , 1-368.
Globalstats Academic
Statistic consultant for academic research.

Quantitative Research Hypothesis Examples
In general, a researcher arranges hypotheses based on the formulation of problems and theoretical studies. For quantitative research, the hypothesis used is a statistical hypothesis, meaning that the hypothesis must be tested using statistical rules. Whereas for qualitative research does not need to use statistical rules. In a quantitative study, the formulated statistical hypothesis has two forms, the null hypothesis (Ho) and the alternative hypothesis (Ha). In general, hypotheses for quantitative research have three types: Descriptive Hypothesis, Comparative Hypothesis, and Associative Hypothesis.
Descriptive Hypothesis
Descriptive hypotheses are temporary conjectures about the value of a variable, not expressing relationships or comparisons. Remember, only about the value of a variable. Statistics used to test descriptive hypotheses are sample mean tests or standard deviation tests. A researcher formulates hypothesis based on the problem formulation and theoretical study. Following are some examples of problem formulations (PF), hypotheses (H). PF: What is the percentage of junior high school mathematics mastery in the subject matter of the set? H: Junior high school mathematics teacher mastery in the subject matter reaches 70%.
PF: How good is the grade XI mastery of class XI material? H: mastery of class X material by class XI students reaches 75%.
Comparative Hypothesis
The comparative hypothesis is a temporary construct that compares the values of two variables. That is, in the comparative hypothesis, we do not determine with certainty the value of the variables we examine, but compare. Means, there are two variables that are the same, but different samples. The statistics used to test this comparative hypothesis are (assuming normality is met) using a t-test. But before that, the normality and homogeneity must be tested first. Following are some examples of problem formulations (PF), hypotheses (H). PF: Is there a difference in the problem-solving abilities of students who got X learning better than students who got Y learning? H: the problem solving ability of students who get learning X is better than students who get learning Y.
PF: Are there differences in the critical thinking skills of students who study during the day are better than students who study in the morning? H: there is no difference in the critical thinking skills of students who study in the afternoon with students who study in the morning.
The two hypothetical examples above are slightly different. In the first hypothesis, we claim that the problem solving ability of students who get learning X is better than students who get learning Y. While in the second hypothesis, there is no one-sided claim that the critical thinking skills of students who learn during the day are better or worse. We only state that there are differences. Which problem is better, it does not concern this hypothesis. The first hypothesis is a one-party test hypothesis, while the second hypothesis is called a two-party test hypothesis.
Associative Quantitative Hypothesis
The associative hypothesis is a relationship between the relationship between two variables, the dependent variable and the independent variable. The statistics are used to test this comparative hypothesis are (assuming normality is met) using Product Moment Correlation, Double Correlation, or Partial Correlation. The following are examples of problem formulations (PF), hypotheses (H). PF: Is there a relationship between student achievement and the level of student anxiety? H: there is a negative relationship between student achievement with the level of student anxiety.
PF: Is there a relationship between student learning outcomes and seating arrangements? H: there is a positive relationship between student achievement with the level of student anxiety. In the first hypothesis there are the words ‘negative relationship’. Negative relationship means inversely proportional. That is if the level of student anxiety is high, then student achievement is low. Whereas in the second hypothesis there are the words ‘positive relationship’. Positive relationship means directly proportional. It means if the seating arrangement is good, the student learning outcomes are high.
Have a language expert improve your writing
Run a free plagiarism check in 10 minutes, automatically generate references for free.
- Knowledge Base
- Methodology
- How to Write a Strong Hypothesis | Guide & Examples
How to Write a Strong Hypothesis | Guide & Examples
Published on 6 May 2022 by Shona McCombes .
A hypothesis is a statement that can be tested by scientific research. If you want to test a relationship between two or more variables, you need to write hypotheses before you start your experiment or data collection.
Table of contents
What is a hypothesis, developing a hypothesis (with example), hypothesis examples, frequently asked questions about writing hypotheses.
A hypothesis states your predictions about what your research will find. It is a tentative answer to your research question that has not yet been tested. For some research projects, you might have to write several hypotheses that address different aspects of your research question.
A hypothesis is not just a guess – it should be based on existing theories and knowledge. It also has to be testable, which means you can support or refute it through scientific research methods (such as experiments, observations, and statistical analysis of data).
Variables in hypotheses
Hypotheses propose a relationship between two or more variables . An independent variable is something the researcher changes or controls. A dependent variable is something the researcher observes and measures.
In this example, the independent variable is exposure to the sun – the assumed cause . The dependent variable is the level of happiness – the assumed effect .
Prevent plagiarism, run a free check.
Step 1: ask a question.
Writing a hypothesis begins with a research question that you want to answer. The question should be focused, specific, and researchable within the constraints of your project.
Step 2: Do some preliminary research
Your initial answer to the question should be based on what is already known about the topic. Look for theories and previous studies to help you form educated assumptions about what your research will find.
At this stage, you might construct a conceptual framework to identify which variables you will study and what you think the relationships are between them. Sometimes, you’ll have to operationalise more complex constructs.
Step 3: Formulate your hypothesis
Now you should have some idea of what you expect to find. Write your initial answer to the question in a clear, concise sentence.
Step 4: Refine your hypothesis
You need to make sure your hypothesis is specific and testable. There are various ways of phrasing a hypothesis, but all the terms you use should have clear definitions, and the hypothesis should contain:
- The relevant variables
- The specific group being studied
- The predicted outcome of the experiment or analysis
Step 5: Phrase your hypothesis in three ways
To identify the variables, you can write a simple prediction in if … then form. The first part of the sentence states the independent variable and the second part states the dependent variable.
In academic research, hypotheses are more commonly phrased in terms of correlations or effects, where you directly state the predicted relationship between variables.
If you are comparing two groups, the hypothesis can state what difference you expect to find between them.
Step 6. Write a null hypothesis
If your research involves statistical hypothesis testing , you will also have to write a null hypothesis. The null hypothesis is the default position that there is no association between the variables. The null hypothesis is written as H 0 , while the alternative hypothesis is H 1 or H a .
Hypothesis testing is a formal procedure for investigating our ideas about the world using statistics. It is used by scientists to test specific predictions, called hypotheses , by calculating how likely it is that a pattern or relationship between variables could have arisen by chance.
A hypothesis is not just a guess. It should be based on existing theories and knowledge. It also has to be testable, which means you can support or refute it through scientific research methods (such as experiments, observations, and statistical analysis of data).
A research hypothesis is your proposed answer to your research question. The research hypothesis usually includes an explanation (‘ x affects y because …’).
A statistical hypothesis, on the other hand, is a mathematical statement about a population parameter. Statistical hypotheses always come in pairs: the null and alternative hypotheses. In a well-designed study , the statistical hypotheses correspond logically to the research hypothesis.
Cite this Scribbr article
If you want to cite this source, you can copy and paste the citation or click the ‘Cite this Scribbr article’ button to automatically add the citation to our free Reference Generator.
McCombes, S. (2022, May 06). How to Write a Strong Hypothesis | Guide & Examples. Scribbr. Retrieved 31 May 2024, from https://www.scribbr.co.uk/research-methods/hypothesis-writing/
Is this article helpful?
Shona McCombes
Other students also liked, operationalisation | a guide with examples, pros & cons, what is a conceptual framework | tips & examples, a quick guide to experimental design | 5 steps & examples.

An official website of the United States government
The .gov means it’s official. Federal government websites often end in .gov or .mil. Before sharing sensitive information, make sure you’re on a federal government site.
The site is secure. The https:// ensures that you are connecting to the official website and that any information you provide is encrypted and transmitted securely.
- Publications
- Account settings
Preview improvements coming to the PMC website in October 2024. Learn More or Try it out now .
- Advanced Search
- Journal List
- J Korean Med Sci
- v.37(16); 2022 Apr 25

A Practical Guide to Writing Quantitative and Qualitative Research Questions and Hypotheses in Scholarly Articles
Edward barroga.
1 Department of General Education, Graduate School of Nursing Science, St. Luke’s International University, Tokyo, Japan.
Glafera Janet Matanguihan
2 Department of Biological Sciences, Messiah University, Mechanicsburg, PA, USA.
The development of research questions and the subsequent hypotheses are prerequisites to defining the main research purpose and specific objectives of a study. Consequently, these objectives determine the study design and research outcome. The development of research questions is a process based on knowledge of current trends, cutting-edge studies, and technological advances in the research field. Excellent research questions are focused and require a comprehensive literature search and in-depth understanding of the problem being investigated. Initially, research questions may be written as descriptive questions which could be developed into inferential questions. These questions must be specific and concise to provide a clear foundation for developing hypotheses. Hypotheses are more formal predictions about the research outcomes. These specify the possible results that may or may not be expected regarding the relationship between groups. Thus, research questions and hypotheses clarify the main purpose and specific objectives of the study, which in turn dictate the design of the study, its direction, and outcome. Studies developed from good research questions and hypotheses will have trustworthy outcomes with wide-ranging social and health implications.
INTRODUCTION
Scientific research is usually initiated by posing evidenced-based research questions which are then explicitly restated as hypotheses. 1 , 2 The hypotheses provide directions to guide the study, solutions, explanations, and expected results. 3 , 4 Both research questions and hypotheses are essentially formulated based on conventional theories and real-world processes, which allow the inception of novel studies and the ethical testing of ideas. 5 , 6
It is crucial to have knowledge of both quantitative and qualitative research 2 as both types of research involve writing research questions and hypotheses. 7 However, these crucial elements of research are sometimes overlooked; if not overlooked, then framed without the forethought and meticulous attention it needs. Planning and careful consideration are needed when developing quantitative or qualitative research, particularly when conceptualizing research questions and hypotheses. 4
There is a continuing need to support researchers in the creation of innovative research questions and hypotheses, as well as for journal articles that carefully review these elements. 1 When research questions and hypotheses are not carefully thought of, unethical studies and poor outcomes usually ensue. Carefully formulated research questions and hypotheses define well-founded objectives, which in turn determine the appropriate design, course, and outcome of the study. This article then aims to discuss in detail the various aspects of crafting research questions and hypotheses, with the goal of guiding researchers as they develop their own. Examples from the authors and peer-reviewed scientific articles in the healthcare field are provided to illustrate key points.
DEFINITIONS AND RELATIONSHIP OF RESEARCH QUESTIONS AND HYPOTHESES
A research question is what a study aims to answer after data analysis and interpretation. The answer is written in length in the discussion section of the paper. Thus, the research question gives a preview of the different parts and variables of the study meant to address the problem posed in the research question. 1 An excellent research question clarifies the research writing while facilitating understanding of the research topic, objective, scope, and limitations of the study. 5
On the other hand, a research hypothesis is an educated statement of an expected outcome. This statement is based on background research and current knowledge. 8 , 9 The research hypothesis makes a specific prediction about a new phenomenon 10 or a formal statement on the expected relationship between an independent variable and a dependent variable. 3 , 11 It provides a tentative answer to the research question to be tested or explored. 4
Hypotheses employ reasoning to predict a theory-based outcome. 10 These can also be developed from theories by focusing on components of theories that have not yet been observed. 10 The validity of hypotheses is often based on the testability of the prediction made in a reproducible experiment. 8
Conversely, hypotheses can also be rephrased as research questions. Several hypotheses based on existing theories and knowledge may be needed to answer a research question. Developing ethical research questions and hypotheses creates a research design that has logical relationships among variables. These relationships serve as a solid foundation for the conduct of the study. 4 , 11 Haphazardly constructed research questions can result in poorly formulated hypotheses and improper study designs, leading to unreliable results. Thus, the formulations of relevant research questions and verifiable hypotheses are crucial when beginning research. 12
CHARACTERISTICS OF GOOD RESEARCH QUESTIONS AND HYPOTHESES
Excellent research questions are specific and focused. These integrate collective data and observations to confirm or refute the subsequent hypotheses. Well-constructed hypotheses are based on previous reports and verify the research context. These are realistic, in-depth, sufficiently complex, and reproducible. More importantly, these hypotheses can be addressed and tested. 13
There are several characteristics of well-developed hypotheses. Good hypotheses are 1) empirically testable 7 , 10 , 11 , 13 ; 2) backed by preliminary evidence 9 ; 3) testable by ethical research 7 , 9 ; 4) based on original ideas 9 ; 5) have evidenced-based logical reasoning 10 ; and 6) can be predicted. 11 Good hypotheses can infer ethical and positive implications, indicating the presence of a relationship or effect relevant to the research theme. 7 , 11 These are initially developed from a general theory and branch into specific hypotheses by deductive reasoning. In the absence of a theory to base the hypotheses, inductive reasoning based on specific observations or findings form more general hypotheses. 10
TYPES OF RESEARCH QUESTIONS AND HYPOTHESES
Research questions and hypotheses are developed according to the type of research, which can be broadly classified into quantitative and qualitative research. We provide a summary of the types of research questions and hypotheses under quantitative and qualitative research categories in Table 1 .
Research questions in quantitative research
In quantitative research, research questions inquire about the relationships among variables being investigated and are usually framed at the start of the study. These are precise and typically linked to the subject population, dependent and independent variables, and research design. 1 Research questions may also attempt to describe the behavior of a population in relation to one or more variables, or describe the characteristics of variables to be measured ( descriptive research questions ). 1 , 5 , 14 These questions may also aim to discover differences between groups within the context of an outcome variable ( comparative research questions ), 1 , 5 , 14 or elucidate trends and interactions among variables ( relationship research questions ). 1 , 5 We provide examples of descriptive, comparative, and relationship research questions in quantitative research in Table 2 .
Hypotheses in quantitative research
In quantitative research, hypotheses predict the expected relationships among variables. 15 Relationships among variables that can be predicted include 1) between a single dependent variable and a single independent variable ( simple hypothesis ) or 2) between two or more independent and dependent variables ( complex hypothesis ). 4 , 11 Hypotheses may also specify the expected direction to be followed and imply an intellectual commitment to a particular outcome ( directional hypothesis ) 4 . On the other hand, hypotheses may not predict the exact direction and are used in the absence of a theory, or when findings contradict previous studies ( non-directional hypothesis ). 4 In addition, hypotheses can 1) define interdependency between variables ( associative hypothesis ), 4 2) propose an effect on the dependent variable from manipulation of the independent variable ( causal hypothesis ), 4 3) state a negative relationship between two variables ( null hypothesis ), 4 , 11 , 15 4) replace the working hypothesis if rejected ( alternative hypothesis ), 15 explain the relationship of phenomena to possibly generate a theory ( working hypothesis ), 11 5) involve quantifiable variables that can be tested statistically ( statistical hypothesis ), 11 6) or express a relationship whose interlinks can be verified logically ( logical hypothesis ). 11 We provide examples of simple, complex, directional, non-directional, associative, causal, null, alternative, working, statistical, and logical hypotheses in quantitative research, as well as the definition of quantitative hypothesis-testing research in Table 3 .
Research questions in qualitative research
Unlike research questions in quantitative research, research questions in qualitative research are usually continuously reviewed and reformulated. The central question and associated subquestions are stated more than the hypotheses. 15 The central question broadly explores a complex set of factors surrounding the central phenomenon, aiming to present the varied perspectives of participants. 15
There are varied goals for which qualitative research questions are developed. These questions can function in several ways, such as to 1) identify and describe existing conditions ( contextual research question s); 2) describe a phenomenon ( descriptive research questions ); 3) assess the effectiveness of existing methods, protocols, theories, or procedures ( evaluation research questions ); 4) examine a phenomenon or analyze the reasons or relationships between subjects or phenomena ( explanatory research questions ); or 5) focus on unknown aspects of a particular topic ( exploratory research questions ). 5 In addition, some qualitative research questions provide new ideas for the development of theories and actions ( generative research questions ) or advance specific ideologies of a position ( ideological research questions ). 1 Other qualitative research questions may build on a body of existing literature and become working guidelines ( ethnographic research questions ). Research questions may also be broadly stated without specific reference to the existing literature or a typology of questions ( phenomenological research questions ), may be directed towards generating a theory of some process ( grounded theory questions ), or may address a description of the case and the emerging themes ( qualitative case study questions ). 15 We provide examples of contextual, descriptive, evaluation, explanatory, exploratory, generative, ideological, ethnographic, phenomenological, grounded theory, and qualitative case study research questions in qualitative research in Table 4 , and the definition of qualitative hypothesis-generating research in Table 5 .
Qualitative studies usually pose at least one central research question and several subquestions starting with How or What . These research questions use exploratory verbs such as explore or describe . These also focus on one central phenomenon of interest, and may mention the participants and research site. 15
Hypotheses in qualitative research
Hypotheses in qualitative research are stated in the form of a clear statement concerning the problem to be investigated. Unlike in quantitative research where hypotheses are usually developed to be tested, qualitative research can lead to both hypothesis-testing and hypothesis-generating outcomes. 2 When studies require both quantitative and qualitative research questions, this suggests an integrative process between both research methods wherein a single mixed-methods research question can be developed. 1
FRAMEWORKS FOR DEVELOPING RESEARCH QUESTIONS AND HYPOTHESES
Research questions followed by hypotheses should be developed before the start of the study. 1 , 12 , 14 It is crucial to develop feasible research questions on a topic that is interesting to both the researcher and the scientific community. This can be achieved by a meticulous review of previous and current studies to establish a novel topic. Specific areas are subsequently focused on to generate ethical research questions. The relevance of the research questions is evaluated in terms of clarity of the resulting data, specificity of the methodology, objectivity of the outcome, depth of the research, and impact of the study. 1 , 5 These aspects constitute the FINER criteria (i.e., Feasible, Interesting, Novel, Ethical, and Relevant). 1 Clarity and effectiveness are achieved if research questions meet the FINER criteria. In addition to the FINER criteria, Ratan et al. described focus, complexity, novelty, feasibility, and measurability for evaluating the effectiveness of research questions. 14
The PICOT and PEO frameworks are also used when developing research questions. 1 The following elements are addressed in these frameworks, PICOT: P-population/patients/problem, I-intervention or indicator being studied, C-comparison group, O-outcome of interest, and T-timeframe of the study; PEO: P-population being studied, E-exposure to preexisting conditions, and O-outcome of interest. 1 Research questions are also considered good if these meet the “FINERMAPS” framework: Feasible, Interesting, Novel, Ethical, Relevant, Manageable, Appropriate, Potential value/publishable, and Systematic. 14
As we indicated earlier, research questions and hypotheses that are not carefully formulated result in unethical studies or poor outcomes. To illustrate this, we provide some examples of ambiguous research question and hypotheses that result in unclear and weak research objectives in quantitative research ( Table 6 ) 16 and qualitative research ( Table 7 ) 17 , and how to transform these ambiguous research question(s) and hypothesis(es) into clear and good statements.
a These statements were composed for comparison and illustrative purposes only.
b These statements are direct quotes from Higashihara and Horiuchi. 16
a This statement is a direct quote from Shimoda et al. 17
The other statements were composed for comparison and illustrative purposes only.
CONSTRUCTING RESEARCH QUESTIONS AND HYPOTHESES
To construct effective research questions and hypotheses, it is very important to 1) clarify the background and 2) identify the research problem at the outset of the research, within a specific timeframe. 9 Then, 3) review or conduct preliminary research to collect all available knowledge about the possible research questions by studying theories and previous studies. 18 Afterwards, 4) construct research questions to investigate the research problem. Identify variables to be accessed from the research questions 4 and make operational definitions of constructs from the research problem and questions. Thereafter, 5) construct specific deductive or inductive predictions in the form of hypotheses. 4 Finally, 6) state the study aims . This general flow for constructing effective research questions and hypotheses prior to conducting research is shown in Fig. 1 .

Research questions are used more frequently in qualitative research than objectives or hypotheses. 3 These questions seek to discover, understand, explore or describe experiences by asking “What” or “How.” The questions are open-ended to elicit a description rather than to relate variables or compare groups. The questions are continually reviewed, reformulated, and changed during the qualitative study. 3 Research questions are also used more frequently in survey projects than hypotheses in experiments in quantitative research to compare variables and their relationships.
Hypotheses are constructed based on the variables identified and as an if-then statement, following the template, ‘If a specific action is taken, then a certain outcome is expected.’ At this stage, some ideas regarding expectations from the research to be conducted must be drawn. 18 Then, the variables to be manipulated (independent) and influenced (dependent) are defined. 4 Thereafter, the hypothesis is stated and refined, and reproducible data tailored to the hypothesis are identified, collected, and analyzed. 4 The hypotheses must be testable and specific, 18 and should describe the variables and their relationships, the specific group being studied, and the predicted research outcome. 18 Hypotheses construction involves a testable proposition to be deduced from theory, and independent and dependent variables to be separated and measured separately. 3 Therefore, good hypotheses must be based on good research questions constructed at the start of a study or trial. 12
In summary, research questions are constructed after establishing the background of the study. Hypotheses are then developed based on the research questions. Thus, it is crucial to have excellent research questions to generate superior hypotheses. In turn, these would determine the research objectives and the design of the study, and ultimately, the outcome of the research. 12 Algorithms for building research questions and hypotheses are shown in Fig. 2 for quantitative research and in Fig. 3 for qualitative research.

EXAMPLES OF RESEARCH QUESTIONS FROM PUBLISHED ARTICLES
- EXAMPLE 1. Descriptive research question (quantitative research)
- - Presents research variables to be assessed (distinct phenotypes and subphenotypes)
- “BACKGROUND: Since COVID-19 was identified, its clinical and biological heterogeneity has been recognized. Identifying COVID-19 phenotypes might help guide basic, clinical, and translational research efforts.
- RESEARCH QUESTION: Does the clinical spectrum of patients with COVID-19 contain distinct phenotypes and subphenotypes? ” 19
- EXAMPLE 2. Relationship research question (quantitative research)
- - Shows interactions between dependent variable (static postural control) and independent variable (peripheral visual field loss)
- “Background: Integration of visual, vestibular, and proprioceptive sensations contributes to postural control. People with peripheral visual field loss have serious postural instability. However, the directional specificity of postural stability and sensory reweighting caused by gradual peripheral visual field loss remain unclear.
- Research question: What are the effects of peripheral visual field loss on static postural control ?” 20
- EXAMPLE 3. Comparative research question (quantitative research)
- - Clarifies the difference among groups with an outcome variable (patients enrolled in COMPERA with moderate PH or severe PH in COPD) and another group without the outcome variable (patients with idiopathic pulmonary arterial hypertension (IPAH))
- “BACKGROUND: Pulmonary hypertension (PH) in COPD is a poorly investigated clinical condition.
- RESEARCH QUESTION: Which factors determine the outcome of PH in COPD?
- STUDY DESIGN AND METHODS: We analyzed the characteristics and outcome of patients enrolled in the Comparative, Prospective Registry of Newly Initiated Therapies for Pulmonary Hypertension (COMPERA) with moderate or severe PH in COPD as defined during the 6th PH World Symposium who received medical therapy for PH and compared them with patients with idiopathic pulmonary arterial hypertension (IPAH) .” 21
- EXAMPLE 4. Exploratory research question (qualitative research)
- - Explores areas that have not been fully investigated (perspectives of families and children who receive care in clinic-based child obesity treatment) to have a deeper understanding of the research problem
- “Problem: Interventions for children with obesity lead to only modest improvements in BMI and long-term outcomes, and data are limited on the perspectives of families of children with obesity in clinic-based treatment. This scoping review seeks to answer the question: What is known about the perspectives of families and children who receive care in clinic-based child obesity treatment? This review aims to explore the scope of perspectives reported by families of children with obesity who have received individualized outpatient clinic-based obesity treatment.” 22
- EXAMPLE 5. Relationship research question (quantitative research)
- - Defines interactions between dependent variable (use of ankle strategies) and independent variable (changes in muscle tone)
- “Background: To maintain an upright standing posture against external disturbances, the human body mainly employs two types of postural control strategies: “ankle strategy” and “hip strategy.” While it has been reported that the magnitude of the disturbance alters the use of postural control strategies, it has not been elucidated how the level of muscle tone, one of the crucial parameters of bodily function, determines the use of each strategy. We have previously confirmed using forward dynamics simulations of human musculoskeletal models that an increased muscle tone promotes the use of ankle strategies. The objective of the present study was to experimentally evaluate a hypothesis: an increased muscle tone promotes the use of ankle strategies. Research question: Do changes in the muscle tone affect the use of ankle strategies ?” 23
EXAMPLES OF HYPOTHESES IN PUBLISHED ARTICLES
- EXAMPLE 1. Working hypothesis (quantitative research)
- - A hypothesis that is initially accepted for further research to produce a feasible theory
- “As fever may have benefit in shortening the duration of viral illness, it is plausible to hypothesize that the antipyretic efficacy of ibuprofen may be hindering the benefits of a fever response when taken during the early stages of COVID-19 illness .” 24
- “In conclusion, it is plausible to hypothesize that the antipyretic efficacy of ibuprofen may be hindering the benefits of a fever response . The difference in perceived safety of these agents in COVID-19 illness could be related to the more potent efficacy to reduce fever with ibuprofen compared to acetaminophen. Compelling data on the benefit of fever warrant further research and review to determine when to treat or withhold ibuprofen for early stage fever for COVID-19 and other related viral illnesses .” 24
- EXAMPLE 2. Exploratory hypothesis (qualitative research)
- - Explores particular areas deeper to clarify subjective experience and develop a formal hypothesis potentially testable in a future quantitative approach
- “We hypothesized that when thinking about a past experience of help-seeking, a self distancing prompt would cause increased help-seeking intentions and more favorable help-seeking outcome expectations .” 25
- “Conclusion
- Although a priori hypotheses were not supported, further research is warranted as results indicate the potential for using self-distancing approaches to increasing help-seeking among some people with depressive symptomatology.” 25
- EXAMPLE 3. Hypothesis-generating research to establish a framework for hypothesis testing (qualitative research)
- “We hypothesize that compassionate care is beneficial for patients (better outcomes), healthcare systems and payers (lower costs), and healthcare providers (lower burnout). ” 26
- Compassionomics is the branch of knowledge and scientific study of the effects of compassionate healthcare. Our main hypotheses are that compassionate healthcare is beneficial for (1) patients, by improving clinical outcomes, (2) healthcare systems and payers, by supporting financial sustainability, and (3) HCPs, by lowering burnout and promoting resilience and well-being. The purpose of this paper is to establish a scientific framework for testing the hypotheses above . If these hypotheses are confirmed through rigorous research, compassionomics will belong in the science of evidence-based medicine, with major implications for all healthcare domains.” 26
- EXAMPLE 4. Statistical hypothesis (quantitative research)
- - An assumption is made about the relationship among several population characteristics ( gender differences in sociodemographic and clinical characteristics of adults with ADHD ). Validity is tested by statistical experiment or analysis ( chi-square test, Students t-test, and logistic regression analysis)
- “Our research investigated gender differences in sociodemographic and clinical characteristics of adults with ADHD in a Japanese clinical sample. Due to unique Japanese cultural ideals and expectations of women's behavior that are in opposition to ADHD symptoms, we hypothesized that women with ADHD experience more difficulties and present more dysfunctions than men . We tested the following hypotheses: first, women with ADHD have more comorbidities than men with ADHD; second, women with ADHD experience more social hardships than men, such as having less full-time employment and being more likely to be divorced.” 27
- “Statistical Analysis
- ( text omitted ) Between-gender comparisons were made using the chi-squared test for categorical variables and Students t-test for continuous variables…( text omitted ). A logistic regression analysis was performed for employment status, marital status, and comorbidity to evaluate the independent effects of gender on these dependent variables.” 27
EXAMPLES OF HYPOTHESIS AS WRITTEN IN PUBLISHED ARTICLES IN RELATION TO OTHER PARTS
- EXAMPLE 1. Background, hypotheses, and aims are provided
- “Pregnant women need skilled care during pregnancy and childbirth, but that skilled care is often delayed in some countries …( text omitted ). The focused antenatal care (FANC) model of WHO recommends that nurses provide information or counseling to all pregnant women …( text omitted ). Job aids are visual support materials that provide the right kind of information using graphics and words in a simple and yet effective manner. When nurses are not highly trained or have many work details to attend to, these job aids can serve as a content reminder for the nurses and can be used for educating their patients (Jennings, Yebadokpo, Affo, & Agbogbe, 2010) ( text omitted ). Importantly, additional evidence is needed to confirm how job aids can further improve the quality of ANC counseling by health workers in maternal care …( text omitted )” 28
- “ This has led us to hypothesize that the quality of ANC counseling would be better if supported by job aids. Consequently, a better quality of ANC counseling is expected to produce higher levels of awareness concerning the danger signs of pregnancy and a more favorable impression of the caring behavior of nurses .” 28
- “This study aimed to examine the differences in the responses of pregnant women to a job aid-supported intervention during ANC visit in terms of 1) their understanding of the danger signs of pregnancy and 2) their impression of the caring behaviors of nurses to pregnant women in rural Tanzania.” 28
- EXAMPLE 2. Background, hypotheses, and aims are provided
- “We conducted a two-arm randomized controlled trial (RCT) to evaluate and compare changes in salivary cortisol and oxytocin levels of first-time pregnant women between experimental and control groups. The women in the experimental group touched and held an infant for 30 min (experimental intervention protocol), whereas those in the control group watched a DVD movie of an infant (control intervention protocol). The primary outcome was salivary cortisol level and the secondary outcome was salivary oxytocin level.” 29
- “ We hypothesize that at 30 min after touching and holding an infant, the salivary cortisol level will significantly decrease and the salivary oxytocin level will increase in the experimental group compared with the control group .” 29
- EXAMPLE 3. Background, aim, and hypothesis are provided
- “In countries where the maternal mortality ratio remains high, antenatal education to increase Birth Preparedness and Complication Readiness (BPCR) is considered one of the top priorities [1]. BPCR includes birth plans during the antenatal period, such as the birthplace, birth attendant, transportation, health facility for complications, expenses, and birth materials, as well as family coordination to achieve such birth plans. In Tanzania, although increasing, only about half of all pregnant women attend an antenatal clinic more than four times [4]. Moreover, the information provided during antenatal care (ANC) is insufficient. In the resource-poor settings, antenatal group education is a potential approach because of the limited time for individual counseling at antenatal clinics.” 30
- “This study aimed to evaluate an antenatal group education program among pregnant women and their families with respect to birth-preparedness and maternal and infant outcomes in rural villages of Tanzania.” 30
- “ The study hypothesis was if Tanzanian pregnant women and their families received a family-oriented antenatal group education, they would (1) have a higher level of BPCR, (2) attend antenatal clinic four or more times, (3) give birth in a health facility, (4) have less complications of women at birth, and (5) have less complications and deaths of infants than those who did not receive the education .” 30
Research questions and hypotheses are crucial components to any type of research, whether quantitative or qualitative. These questions should be developed at the very beginning of the study. Excellent research questions lead to superior hypotheses, which, like a compass, set the direction of research, and can often determine the successful conduct of the study. Many research studies have floundered because the development of research questions and subsequent hypotheses was not given the thought and meticulous attention needed. The development of research questions and hypotheses is an iterative process based on extensive knowledge of the literature and insightful grasp of the knowledge gap. Focused, concise, and specific research questions provide a strong foundation for constructing hypotheses which serve as formal predictions about the research outcomes. Research questions and hypotheses are crucial elements of research that should not be overlooked. They should be carefully thought of and constructed when planning research. This avoids unethical studies and poor outcomes by defining well-founded objectives that determine the design, course, and outcome of the study.
Disclosure: The authors have no potential conflicts of interest to disclose.
Author Contributions:
- Conceptualization: Barroga E, Matanguihan GJ.
- Methodology: Barroga E, Matanguihan GJ.
- Writing - original draft: Barroga E, Matanguihan GJ.
- Writing - review & editing: Barroga E, Matanguihan GJ.
- Bipolar Disorder
- Therapy Center
- When To See a Therapist
- Types of Therapy
- Best Online Therapy
- Best Couples Therapy
- Best Family Therapy
- Managing Stress
- Sleep and Dreaming
- Understanding Emotions
- Self-Improvement
- Healthy Relationships
- Student Resources
- Personality Types
- Guided Meditations
- Verywell Mind Insights
- 2024 Verywell Mind 25
- Mental Health in the Classroom
- Editorial Process
- Meet Our Review Board
- Crisis Support
How to Write a Great Hypothesis
Hypothesis Definition, Format, Examples, and Tips
Kendra Cherry, MS, is a psychosocial rehabilitation specialist, psychology educator, and author of the "Everything Psychology Book."
:max_bytes(150000):strip_icc():format(webp)/IMG_9791-89504ab694d54b66bbd72cb84ffb860e.jpg)
Amy Morin, LCSW, is a psychotherapist and international bestselling author. Her books, including "13 Things Mentally Strong People Don't Do," have been translated into more than 40 languages. Her TEDx talk, "The Secret of Becoming Mentally Strong," is one of the most viewed talks of all time.
:max_bytes(150000):strip_icc():format(webp)/VW-MIND-Amy-2b338105f1ee493f94d7e333e410fa76.jpg)
Verywell / Alex Dos Diaz
- The Scientific Method
Hypothesis Format
Falsifiability of a hypothesis.
- Operationalization
Hypothesis Types
Hypotheses examples.
- Collecting Data
A hypothesis is a tentative statement about the relationship between two or more variables. It is a specific, testable prediction about what you expect to happen in a study. It is a preliminary answer to your question that helps guide the research process.
Consider a study designed to examine the relationship between sleep deprivation and test performance. The hypothesis might be: "This study is designed to assess the hypothesis that sleep-deprived people will perform worse on a test than individuals who are not sleep-deprived."
At a Glance
A hypothesis is crucial to scientific research because it offers a clear direction for what the researchers are looking to find. This allows them to design experiments to test their predictions and add to our scientific knowledge about the world. This article explores how a hypothesis is used in psychology research, how to write a good hypothesis, and the different types of hypotheses you might use.
The Hypothesis in the Scientific Method
In the scientific method , whether it involves research in psychology, biology, or some other area, a hypothesis represents what the researchers think will happen in an experiment. The scientific method involves the following steps:
- Forming a question
- Performing background research
- Creating a hypothesis
- Designing an experiment
- Collecting data
- Analyzing the results
- Drawing conclusions
- Communicating the results
The hypothesis is a prediction, but it involves more than a guess. Most of the time, the hypothesis begins with a question which is then explored through background research. At this point, researchers then begin to develop a testable hypothesis.
Unless you are creating an exploratory study, your hypothesis should always explain what you expect to happen.
In a study exploring the effects of a particular drug, the hypothesis might be that researchers expect the drug to have some type of effect on the symptoms of a specific illness. In psychology, the hypothesis might focus on how a certain aspect of the environment might influence a particular behavior.
Remember, a hypothesis does not have to be correct. While the hypothesis predicts what the researchers expect to see, the goal of the research is to determine whether this guess is right or wrong. When conducting an experiment, researchers might explore numerous factors to determine which ones might contribute to the ultimate outcome.
In many cases, researchers may find that the results of an experiment do not support the original hypothesis. When writing up these results, the researchers might suggest other options that should be explored in future studies.
In many cases, researchers might draw a hypothesis from a specific theory or build on previous research. For example, prior research has shown that stress can impact the immune system. So a researcher might hypothesize: "People with high-stress levels will be more likely to contract a common cold after being exposed to the virus than people who have low-stress levels."
In other instances, researchers might look at commonly held beliefs or folk wisdom. "Birds of a feather flock together" is one example of folk adage that a psychologist might try to investigate. The researcher might pose a specific hypothesis that "People tend to select romantic partners who are similar to them in interests and educational level."
Elements of a Good Hypothesis
So how do you write a good hypothesis? When trying to come up with a hypothesis for your research or experiments, ask yourself the following questions:
- Is your hypothesis based on your research on a topic?
- Can your hypothesis be tested?
- Does your hypothesis include independent and dependent variables?
Before you come up with a specific hypothesis, spend some time doing background research. Once you have completed a literature review, start thinking about potential questions you still have. Pay attention to the discussion section in the journal articles you read . Many authors will suggest questions that still need to be explored.
How to Formulate a Good Hypothesis
To form a hypothesis, you should take these steps:
- Collect as many observations about a topic or problem as you can.
- Evaluate these observations and look for possible causes of the problem.
- Create a list of possible explanations that you might want to explore.
- After you have developed some possible hypotheses, think of ways that you could confirm or disprove each hypothesis through experimentation. This is known as falsifiability.
In the scientific method , falsifiability is an important part of any valid hypothesis. In order to test a claim scientifically, it must be possible that the claim could be proven false.
Students sometimes confuse the idea of falsifiability with the idea that it means that something is false, which is not the case. What falsifiability means is that if something was false, then it is possible to demonstrate that it is false.
One of the hallmarks of pseudoscience is that it makes claims that cannot be refuted or proven false.
The Importance of Operational Definitions
A variable is a factor or element that can be changed and manipulated in ways that are observable and measurable. However, the researcher must also define how the variable will be manipulated and measured in the study.
Operational definitions are specific definitions for all relevant factors in a study. This process helps make vague or ambiguous concepts detailed and measurable.
For example, a researcher might operationally define the variable " test anxiety " as the results of a self-report measure of anxiety experienced during an exam. A "study habits" variable might be defined by the amount of studying that actually occurs as measured by time.
These precise descriptions are important because many things can be measured in various ways. Clearly defining these variables and how they are measured helps ensure that other researchers can replicate your results.
Replicability
One of the basic principles of any type of scientific research is that the results must be replicable.
Replication means repeating an experiment in the same way to produce the same results. By clearly detailing the specifics of how the variables were measured and manipulated, other researchers can better understand the results and repeat the study if needed.
Some variables are more difficult than others to define. For example, how would you operationally define a variable such as aggression ? For obvious ethical reasons, researchers cannot create a situation in which a person behaves aggressively toward others.
To measure this variable, the researcher must devise a measurement that assesses aggressive behavior without harming others. The researcher might utilize a simulated task to measure aggressiveness in this situation.
Hypothesis Checklist
- Does your hypothesis focus on something that you can actually test?
- Does your hypothesis include both an independent and dependent variable?
- Can you manipulate the variables?
- Can your hypothesis be tested without violating ethical standards?
The hypothesis you use will depend on what you are investigating and hoping to find. Some of the main types of hypotheses that you might use include:
- Simple hypothesis : This type of hypothesis suggests there is a relationship between one independent variable and one dependent variable.
- Complex hypothesis : This type suggests a relationship between three or more variables, such as two independent and dependent variables.
- Null hypothesis : This hypothesis suggests no relationship exists between two or more variables.
- Alternative hypothesis : This hypothesis states the opposite of the null hypothesis.
- Statistical hypothesis : This hypothesis uses statistical analysis to evaluate a representative population sample and then generalizes the findings to the larger group.
- Logical hypothesis : This hypothesis assumes a relationship between variables without collecting data or evidence.
A hypothesis often follows a basic format of "If {this happens} then {this will happen}." One way to structure your hypothesis is to describe what will happen to the dependent variable if you change the independent variable .
The basic format might be: "If {these changes are made to a certain independent variable}, then we will observe {a change in a specific dependent variable}."
A few examples of simple hypotheses:
- "Students who eat breakfast will perform better on a math exam than students who do not eat breakfast."
- "Students who experience test anxiety before an English exam will get lower scores than students who do not experience test anxiety."
- "Motorists who talk on the phone while driving will be more likely to make errors on a driving course than those who do not talk on the phone."
- "Children who receive a new reading intervention will have higher reading scores than students who do not receive the intervention."
Examples of a complex hypothesis include:
- "People with high-sugar diets and sedentary activity levels are more likely to develop depression."
- "Younger people who are regularly exposed to green, outdoor areas have better subjective well-being than older adults who have limited exposure to green spaces."
Examples of a null hypothesis include:
- "There is no difference in anxiety levels between people who take St. John's wort supplements and those who do not."
- "There is no difference in scores on a memory recall task between children and adults."
- "There is no difference in aggression levels between children who play first-person shooter games and those who do not."
Examples of an alternative hypothesis:
- "People who take St. John's wort supplements will have less anxiety than those who do not."
- "Adults will perform better on a memory task than children."
- "Children who play first-person shooter games will show higher levels of aggression than children who do not."
Collecting Data on Your Hypothesis
Once a researcher has formed a testable hypothesis, the next step is to select a research design and start collecting data. The research method depends largely on exactly what they are studying. There are two basic types of research methods: descriptive research and experimental research.
Descriptive Research Methods
Descriptive research such as case studies , naturalistic observations , and surveys are often used when conducting an experiment is difficult or impossible. These methods are best used to describe different aspects of a behavior or psychological phenomenon.
Once a researcher has collected data using descriptive methods, a correlational study can examine how the variables are related. This research method might be used to investigate a hypothesis that is difficult to test experimentally.
Experimental Research Methods
Experimental methods are used to demonstrate causal relationships between variables. In an experiment, the researcher systematically manipulates a variable of interest (known as the independent variable) and measures the effect on another variable (known as the dependent variable).
Unlike correlational studies, which can only be used to determine if there is a relationship between two variables, experimental methods can be used to determine the actual nature of the relationship—whether changes in one variable actually cause another to change.
The hypothesis is a critical part of any scientific exploration. It represents what researchers expect to find in a study or experiment. In situations where the hypothesis is unsupported by the research, the research still has value. Such research helps us better understand how different aspects of the natural world relate to one another. It also helps us develop new hypotheses that can then be tested in the future.
Thompson WH, Skau S. On the scope of scientific hypotheses . R Soc Open Sci . 2023;10(8):230607. doi:10.1098/rsos.230607
Taran S, Adhikari NKJ, Fan E. Falsifiability in medicine: what clinicians can learn from Karl Popper [published correction appears in Intensive Care Med. 2021 Jun 17;:]. Intensive Care Med . 2021;47(9):1054-1056. doi:10.1007/s00134-021-06432-z
Eyler AA. Research Methods for Public Health . 1st ed. Springer Publishing Company; 2020. doi:10.1891/9780826182067.0004
Nosek BA, Errington TM. What is replication ? PLoS Biol . 2020;18(3):e3000691. doi:10.1371/journal.pbio.3000691
Aggarwal R, Ranganathan P. Study designs: Part 2 - Descriptive studies . Perspect Clin Res . 2019;10(1):34-36. doi:10.4103/picr.PICR_154_18
Nevid J. Psychology: Concepts and Applications. Wadworth, 2013.
By Kendra Cherry, MSEd Kendra Cherry, MS, is a psychosocial rehabilitation specialist, psychology educator, and author of the "Everything Psychology Book."
User Preferences
Content preview.
Arcu felis bibendum ut tristique et egestas quis:
- Ut enim ad minim veniam, quis nostrud exercitation ullamco laboris
- Duis aute irure dolor in reprehenderit in voluptate
- Excepteur sint occaecat cupidatat non proident
Keyboard Shortcuts
5.2 - writing hypotheses.
The first step in conducting a hypothesis test is to write the hypothesis statements that are going to be tested. For each test you will have a null hypothesis (\(H_0\)) and an alternative hypothesis (\(H_a\)).
When writing hypotheses there are three things that we need to know: (1) the parameter that we are testing (2) the direction of the test (non-directional, right-tailed or left-tailed), and (3) the value of the hypothesized parameter.
- At this point we can write hypotheses for a single mean (\(\mu\)), paired means(\(\mu_d\)), a single proportion (\(p\)), the difference between two independent means (\(\mu_1-\mu_2\)), the difference between two proportions (\(p_1-p_2\)), a simple linear regression slope (\(\beta\)), and a correlation (\(\rho\)).
- The research question will give us the information necessary to determine if the test is two-tailed (e.g., "different from," "not equal to"), right-tailed (e.g., "greater than," "more than"), or left-tailed (e.g., "less than," "fewer than").
- The research question will also give us the hypothesized parameter value. This is the number that goes in the hypothesis statements (i.e., \(\mu_0\) and \(p_0\)). For the difference between two groups, regression, and correlation, this value is typically 0.
Hypotheses are always written in terms of population parameters (e.g., \(p\) and \(\mu\)). The tables below display all of the possible hypotheses for the parameters that we have learned thus far. Note that the null hypothesis always includes the equality (i.e., =).
Quantitative Research
- Reference work entry
- First Online: 13 January 2019
- Cite this reference work entry
- Leigh A. Wilson 2 , 3
4455 Accesses
4 Citations
Quantitative research methods are concerned with the planning, design, and implementation of strategies to collect and analyze data. Descartes, the seventeenth-century philosopher, suggested that how the results are achieved is often more important than the results themselves, as the journey taken along the research path is a journey of discovery. High-quality quantitative research is characterized by the attention given to the methods and the reliability of the tools used to collect the data. The ability to critique research in a systematic way is an essential component of a health professional’s role in order to deliver high quality, evidence-based healthcare. This chapter is intended to provide a simple overview of the way new researchers and health practitioners can understand and employ quantitative methods. The chapter offers practical, realistic guidance in a learner-friendly way and uses a logical sequence to understand the process of hypothesis development, study design, data collection and handling, and finally data analysis and interpretation.
This is a preview of subscription content, log in via an institution to check access.
Access this chapter
- Available as PDF
- Read on any device
- Instant download
- Own it forever
- Available as EPUB and PDF
- Durable hardcover edition
- Dispatched in 3 to 5 business days
- Free shipping worldwide - see info
Tax calculation will be finalised at checkout
Purchases are for personal use only
Institutional subscriptions
Babbie ER. The practice of social research. 14th ed. Belmont: Wadsworth Cengage; 2016.
Google Scholar
Descartes. Cited in Halverston, W. (1976). In: A concise introduction to philosophy, 3rd ed. New York: Random House; 1637.
Doll R, Hill AB. The mortality of doctors in relation to their smoking habits. BMJ. 1954;328(7455):1529–33. https://doi.org/10.1136/bmj.328.7455.1529 .
Article Google Scholar
Liamputtong P. Research methods in health: foundations for evidence-based practice. 3rd ed. Melbourne: Oxford University Press; 2017.
McNabb DE. Research methods in public administration and nonprofit management: quantitative and qualitative approaches. 2nd ed. New York: Armonk; 2007.
Merriam-Webster. Dictionary. http://www.merriam-webster.com . Accessed 20th December 2017.
Olesen Larsen P, von Ins M. The rate of growth in scientific publication and the decline in coverage provided by Science Citation Index. Scientometrics. 2010;84(3):575–603.
Pannucci CJ, Wilkins EG. Identifying and avoiding bias in research. Plast Reconstr Surg. 2010;126(2):619–25. https://doi.org/10.1097/PRS.0b013e3181de24bc .
Petrie A, Sabin C. Medical statistics at a glance. 2nd ed. London: Blackwell Publishing; 2005.
Portney LG, Watkins MP. Foundations of clinical research: applications to practice. 3rd ed. New Jersey: Pearson Publishing; 2009.
Sheehan J. Aspects of research methodology. Nurse Educ Today. 1986;6:193–203.
Wilson LA, Black DA. Health, science research and research methods. Sydney: McGraw Hill; 2013.
Download references
Author information
Authors and affiliations.
School of Science and Health, Western Sydney University, Penrith, NSW, Australia
Leigh A. Wilson
Faculty of Health Science, Discipline of Behavioural and Social Sciences in Health, University of Sydney, Lidcombe, NSW, Australia
You can also search for this author in PubMed Google Scholar
Corresponding author
Correspondence to Leigh A. Wilson .
Editor information
Editors and affiliations.
Pranee Liamputtong
Rights and permissions
Reprints and permissions
Copyright information
© 2019 Springer Nature Singapore Pte Ltd.

About this entry
Cite this entry.
Wilson, L.A. (2019). Quantitative Research. In: Liamputtong, P. (eds) Handbook of Research Methods in Health Social Sciences. Springer, Singapore. https://doi.org/10.1007/978-981-10-5251-4_54
Download citation
DOI : https://doi.org/10.1007/978-981-10-5251-4_54
Published : 13 January 2019
Publisher Name : Springer, Singapore
Print ISBN : 978-981-10-5250-7
Online ISBN : 978-981-10-5251-4
eBook Packages : Social Sciences Reference Module Humanities and Social Sciences Reference Module Business, Economics and Social Sciences
Share this entry
Anyone you share the following link with will be able to read this content:
Sorry, a shareable link is not currently available for this article.
Provided by the Springer Nature SharedIt content-sharing initiative
- Publish with us
Policies and ethics
- Find a journal
- Track your research

Quantitative Research Methods
- Introduction
- Descriptive and Inferential Statistics
- Hypothesis Testing
- Regression and Correlation
- Time Series
- Meta-Analysis
- Mixed Methods
- Additional Resources
- Get Research Help
Hypothesis Tests
A hypothesis test is exactly what it sounds like: You make a hypothesis about the parameters of a population, and the test determines whether your hypothesis is consistent with your sample data.
- Hypothesis Testing Penn State University tutorial
- Hypothesis Testing Wolfram MathWorld overview
- Hypothesis Testing Minitab Blog entry
- List of Statistical Tests A list of commonly used hypothesis tests and the circumstances under which they're used.
The p-value of a hypothesis test is the probability that your sample data would have occurred if you hypothesis were not correct. Traditionally, researchers have used a p-value of 0.05 (a 5% probability that your sample data would have occurred if your hypothesis was wrong) as the threshold for declaring that a hypothesis is true. But there is a long history of debate and controversy over p-values and significance levels.
Nonparametric Tests
Many of the most commonly used hypothesis tests rely on assumptions about your sample data—for instance, that it is continuous, and that its parameters follow a Normal distribution. Nonparametric hypothesis tests don't make any assumptions about the distribution of the data, and many can be used on categorical data.
- Nonparametric Tests at Boston University A lesson covering four common nonparametric tests.
- Nonparametric Tests at Penn State Tutorial covering the theory behind nonparametric tests as well as several commonly used tests.
- << Previous: Descriptive and Inferential Statistics
- Next: Regression and Correlation >>
- Last Updated: Aug 18, 2023 11:55 AM
- URL: https://guides.library.duq.edu/quant-methods

Want to create or adapt books like this? Learn more about how Pressbooks supports open publishing practices.
11 Hypothesis Testing with One Sample
Student learning outcomes.
By the end of this chapter, the student should be able to:
- Be able to identify and develop the null and alternative hypothesis
- Identify the consequences of Type I and Type II error.
- Be able to perform an one-tailed and two-tailed hypothesis test using the critical value method
- Be able to perform a hypothesis test using the p-value method
- Be able to write conclusions based on hypothesis tests.
Introduction
Now we are down to the bread and butter work of the statistician: developing and testing hypotheses. It is important to put this material in a broader context so that the method by which a hypothesis is formed is understood completely. Using textbook examples often clouds the real source of statistical hypotheses.
Statistical testing is part of a much larger process known as the scientific method. This method was developed more than two centuries ago as the accepted way that new knowledge could be created. Until then, and unfortunately even today, among some, “knowledge” could be created simply by some authority saying something was so, ipso dicta . Superstition and conspiracy theories were (are?) accepted uncritically.
The scientific method, briefly, states that only by following a careful and specific process can some assertion be included in the accepted body of knowledge. This process begins with a set of assumptions upon which a theory, sometimes called a model, is built. This theory, if it has any validity, will lead to predictions; what we call hypotheses.
As an example, in Microeconomics the theory of consumer choice begins with certain assumption concerning human behavior. From these assumptions a theory of how consumers make choices using indifference curves and the budget line. This theory gave rise to a very important prediction, namely, that there was an inverse relationship between price and quantity demanded. This relationship was known as the demand curve. The negative slope of the demand curve is really just a prediction, or a hypothesis, that can be tested with statistical tools.
Unless hundreds and hundreds of statistical tests of this hypothesis had not confirmed this relationship, the so-called Law of Demand would have been discarded years ago. This is the role of statistics, to test the hypotheses of various theories to determine if they should be admitted into the accepted body of knowledge; how we understand our world. Once admitted, however, they may be later discarded if new theories come along that make better predictions.
Not long ago two scientists claimed that they could get more energy out of a process than was put in. This caused a tremendous stir for obvious reasons. They were on the cover of Time and were offered extravagant sums to bring their research work to private industry and any number of universities. It was not long until their work was subjected to the rigorous tests of the scientific method and found to be a failure. No other lab could replicate their findings. Consequently they have sunk into obscurity and their theory discarded. It may surface again when someone can pass the tests of the hypotheses required by the scientific method, but until then it is just a curiosity. Many pure frauds have been attempted over time, but most have been found out by applying the process of the scientific method.
This discussion is meant to show just where in this process statistics falls. Statistics and statisticians are not necessarily in the business of developing theories, but in the business of testing others’ theories. Hypotheses come from these theories based upon an explicit set of assumptions and sound logic. The hypothesis comes first, before any data are gathered. Data do not create hypotheses; they are used to test them. If we bear this in mind as we study this section the process of forming and testing hypotheses will make more sense.
One job of a statistician is to make statistical inferences about populations based on samples taken from the population. Confidence intervals are one way to estimate a population parameter. Another way to make a statistical inference is to make a decision about the value of a specific parameter. For instance, a car dealer advertises that its new small truck gets 35 miles per gallon, on average. A tutoring service claims that its method of tutoring helps 90% of its students get an A or a B. A company says that women managers in their company earn an average of $60,000 per year.
A statistician will make a decision about these claims. This process is called ” hypothesis testing .” A hypothesis test involves collecting data from a sample and evaluating the data. Then, the statistician makes a decision as to whether or not there is sufficient evidence, based upon analyses of the data, to reject the null hypothesis.
In this chapter, you will conduct hypothesis tests on single means and single proportions. You will also learn about the errors associated with these tests.
Null and Alternative Hypotheses
The actual test begins by considering two hypotheses . They are called the null hypothesis and the alternative hypothesis . These hypotheses contain opposing viewpoints.

Since the null and alternative hypotheses are contradictory, you must examine evidence to decide if you have enough evidence to reject the null hypothesis or not. The evidence is in the form of sample data.
Table 1 presents the various hypotheses in the relevant pairs. For example, if the null hypothesis is equal to some value, the alternative has to be not equal to that value.
NOTE
We want to test whether the mean GPA of students in American colleges is different from 2.0 (out of 4.0). The null and alternative hypotheses are:

We want to test if college students take less than five years to graduate from college, on the average. The null and alternative hypotheses are:
Outcomes and the Type I and Type II Errors
The four possible outcomes in the table are:
Each of the errors occurs with a particular probability. The Greek letters α and β represent the probabilities.

By way of example, the American judicial system begins with the concept that a defendant is “presumed innocent”. This is the status quo and is the null hypothesis. The judge will tell the jury that they can not find the defendant guilty unless the evidence indicates guilt beyond a “reasonable doubt” which is usually defined in criminal cases as 95% certainty of guilt. If the jury cannot accept the null, innocent, then action will be taken, jail time. The burden of proof always lies with the alternative hypothesis. (In civil cases, the jury needs only to be more than 50% certain of wrongdoing to find culpability, called “a preponderance of the evidence”).
The example above was for a test of a mean, but the same logic applies to tests of hypotheses for all statistical parameters one may wish to test.
The following are examples of Type I and Type II errors.
Type I error : Frank thinks that his rock climbing equipment may not be safe when, in fact, it really is safe.
Type II error : Frank thinks that his rock climbing equipment may be safe when, in fact, it is not safe.
Notice that, in this case, the error with the greater consequence is the Type II error. (If Frank thinks his rock climbing equipment is safe, he will go ahead and use it.)
This is a situation described as “accepting a false null”.
Type I error : The emergency crew thinks that the victim is dead when, in fact, the victim is alive. Type II error : The emergency crew does not know if the victim is alive when, in fact, the victim is dead.
The error with the greater consequence is the Type I error. (If the emergency crew thinks the victim is dead, they will not treat him.)
Distribution Needed for Hypothesis Testing
Particular distributions are associated with hypothesis testing.We will perform hypotheses tests of a population mean using a normal distribution or a Student’s t -distribution. (Remember, use a Student’s t -distribution when the population standard deviation is unknown and the sample size is small, where small is considered to be less than 30 observations.) We perform tests of a population proportion using a normal distribution when we can assume that the distribution is normally distributed. We consider this to be true if the sample proportion, p ‘ , times the sample size is greater than 5 and 1- p ‘ times the sample size is also greater then 5. This is the same rule of thumb we used when developing the formula for the confidence interval for a population proportion.
Hypothesis Test for the Mean
Going back to the standardizing formula we can derive the test statistic for testing hypotheses concerning means.

This gives us the decision rule for testing a hypothesis for a two-tailed test:
P-Value Approach
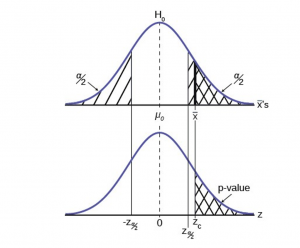
Both decision rules will result in the same decision and it is a matter of preference which one is used.
One and Two-tailed Tests

The claim would be in the alternative hypothesis. The burden of proof in hypothesis testing is carried in the alternative. This is because failing to reject the null, the status quo, must be accomplished with 90 or 95 percent significance that it cannot be maintained. Said another way, we want to have only a 5 or 10 percent probability of making a Type I error, rejecting a good null; overthrowing the status quo.
Figure 5 shows the two possible cases and the form of the null and alternative hypothesis that give rise to them.
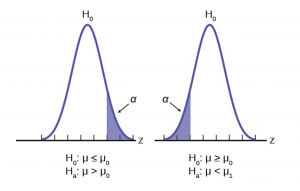
Effects of Sample Size on Test Statistic

Table 3 summarizes test statistics for varying sample sizes and population standard deviation known and unknown.
A Systematic Approach for Testing A Hypothesis
A systematic approach to hypothesis testing follows the following steps and in this order. This template will work for all hypotheses that you will ever test.
- Set up the null and alternative hypothesis. This is typically the hardest part of the process. Here the question being asked is reviewed. What parameter is being tested, a mean, a proportion, differences in means, etc. Is this a one-tailed test or two-tailed test? Remember, if someone is making a claim it will always be a one-tailed test.
- Decide the level of significance required for this particular case and determine the critical value. These can be found in the appropriate statistical table. The levels of confidence typical for the social sciences are 90, 95 and 99. However, the level of significance is a policy decision and should be based upon the risk of making a Type I error, rejecting a good null. Consider the consequences of making a Type I error.
- Take a sample(s) and calculate the relevant parameters: sample mean, standard deviation, or proportion. Using the formula for the test statistic from above in step 2, now calculate the test statistic for this particular case using the parameters you have just calculated.
- Compare the calculated test statistic and the critical value. Marking these on the graph will give a good visual picture of the situation. There are now only two situations:
a. The test statistic is in the tail: Cannot Accept the null, the probability that this sample mean (proportion) came from the hypothesized distribution is too small to believe that it is the real home of these sample data.
b. The test statistic is not in the tail: Cannot Reject the null, the sample data are compatible with the hypothesized population parameter.
- Reach a conclusion. It is best to articulate the conclusion two different ways. First a formal statistical conclusion such as “With a 95 % level of significance we cannot accept the null hypotheses that the population mean is equal to XX (units of measurement)”. The second statement of the conclusion is less formal and states the action, or lack of action, required. If the formal conclusion was that above, then the informal one might be, “The machine is broken and we need to shut it down and call for repairs”.
All hypotheses tested will go through this same process. The only changes are the relevant formulas and those are determined by the hypothesis required to answer the original question.
Full Hypothesis Test Examples
Tests on means.
Jeffrey, as an eight-year old, established a mean time of 16.43 seconds for swimming the 25-yard freestyle, with a standard deviation of 0.8 seconds . His dad, Frank, thought that Jeffrey could swim the 25-yard freestyle faster using goggles. Frank bought Jeffrey a new pair of expensive goggles and timed Jeffrey for 15 25-yard freestyle swims . For the 15 swims, Jeffrey’s mean time was 16 seconds. Frank thought that the goggles helped Jeffrey to swim faster than the 16.43 seconds. Conduct a hypothesis test using a preset α = 0.05.
Solution – Example 6
Set up the Hypothesis Test:
Since the problem is about a mean, this is a test of a single population mean . Set the null and alternative hypothesis:
In this case there is an implied challenge or claim. This is that the goggles will reduce the swimming time. The effect of this is to set the hypothesis as a one-tailed test. The claim will always be in the alternative hypothesis because the burden of proof always lies with the alternative. Remember that the status quo must be defeated with a high degree of confidence, in this case 95 % confidence. The null and alternative hypotheses are thus:
For Jeffrey to swim faster, his time will be less than 16.43 seconds. The “<” tells you this is left-tailed. Determine the distribution needed:
Distribution for the test statistic:
The sample size is less than 30 and we do not know the population standard deviation so this is a t-test and the proper formula is:

Our step 2, setting the level of significance, has already been determined by the problem, .05 for a 95 % significance level. It is worth thinking about the meaning of this choice. The Type I error is to conclude that Jeffrey swims the 25-yard freestyle, on average, in less than 16.43 seconds when, in fact, he actually swims the 25-yard freestyle, on average, in 16.43 seconds. (Reject the null hypothesis when the null hypothesis is true.) For this case the only concern with a Type I error would seem to be that Jeffery’s dad may fail to bet on his son’s victory because he does not have appropriate confidence in the effect of the goggles.
To find the critical value we need to select the appropriate test statistic. We have concluded that this is a t-test on the basis of the sample size and that we are interested in a population mean. We can now draw the graph of the t-distribution and mark the critical value (Figure 6). For this problem the degrees of freedom are n-1, or 14. Looking up 14 degrees of freedom at the 0.05 column of the t-table we find 1.761. This is the critical value and we can put this on our graph.
Step 3 is the calculation of the test statistic using the formula we have selected.

We find that the calculated test statistic is 2.08, meaning that the sample mean is 2.08 standard deviations away from the hypothesized mean of 16.43.
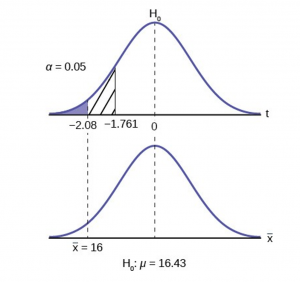
Step 4 has us compare the test statistic and the critical value and mark these on the graph. We see that the test statistic is in the tail and thus we move to step 4 and reach a conclusion. The probability that an average time of 16 minutes could come from a distribution with a population mean of 16.43 minutes is too unlikely for us to accept the null hypothesis. We cannot accept the null.
Step 5 has us state our conclusions first formally and then less formally. A formal conclusion would be stated as: “With a 95% level of significance we cannot accept the null hypothesis that the swimming time with goggles comes from a distribution with a population mean time of 16.43 minutes.” Less formally, “With 95% significance we believe that the goggles improves swimming speed”
If we wished to use the p-value system of reaching a conclusion we would calculate the statistic and take the additional step to find the probability of being 2.08 standard deviations from the mean on a t-distribution. This value is .0187. Comparing this to the α-level of .05 we see that we cannot accept the null. The p-value has been put on the graph as the shaded area beyond -2.08 and it shows that it is smaller than the hatched area which is the alpha level of 0.05. Both methods reach the same conclusion that we cannot accept the null hypothesis.
Jane has just begun her new job as on the sales force of a very competitive company. In a sample of 16 sales calls it was found that she closed the contract for an average value of $108 with a standard deviation of 12 dollars. Test at 5% significance that the population mean is at least $100 against the alternative that it is less than 100 dollars. Company policy requires that new members of the sales force must exceed an average of $100 per contract during the trial employment period. Can we conclude that Jane has met this requirement at the significance level of 95%?
Solution – Example 7
STEP 1 : Set the Null and Alternative Hypothesis.
STEP 2 : Decide the level of significance and draw the graph (Figure 7) showing the critical value.

STEP 3 : Calculate sample parameters and the test statistic.
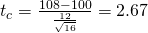
STEP 4 : Compare test statistic and the critical values
STEP 5 : Reach a Conclusion
The test statistic is a Student’s t because the sample size is below 30; therefore, we cannot use the normal distribution. Comparing the calculated value of the test statistic and the critical value of t ( t a ) at a 5% significance level, we see that the calculated value is in the tail of the distribution. Thus, we conclude that 108 dollars per contract is significantly larger than the hypothesized value of 100 and thus we cannot accept the null hypothesis. There is evidence that supports Jane’s performance meets company standards.

Again we will follow the steps in our analysis of this problem.
Solution – Example 8
STEP 1 : Set the Null and Alternative Hypothesis. The random variable is the quantity of fluid placed in the bottles. This is a continuous random variable and the parameter we are interested in is the mean. Our hypothesis therefore is about the mean. In this case we are concerned that the machine is not filling properly. From what we are told it does not matter if the machine is over-filling or under-filling, both seem to be an equally bad error. This tells us that this is a two-tailed test: if the machine is malfunctioning it will be shutdown regardless if it is from over-filling or under-filling. The null and alternative hypotheses are thus:
STEP 2 : Decide the level of significance and draw the graph showing the critical value.
This problem has already set the level of significance at 99%. The decision seems an appropriate one and shows the thought process when setting the significance level. Management wants to be very certain, as certain as probability will allow, that they are not shutting down a machine that is not in need of repair. To draw the distribution and the critical value, we need to know which distribution to use. Because this is a continuous random variable and we are interested in the mean, and the sample size is greater than 30, the appropriate distribution is the normal distribution and the relevant critical value is 2.575 from the normal table or the t-table at 0.005 column and infinite degrees of freedom. We draw the graph and mark these points (Figure 8).
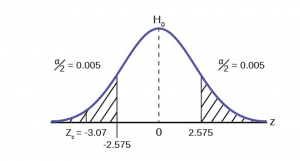
STEP 3 : Calculate sample parameters and the test statistic. The sample parameters are provided, the sample mean is 7.91 and the sample variance is .03 and the sample size is 35. We need to note that the sample variance was provided not the sample standard deviation, which is what we need for the formula. Remembering that the standard deviation is simply the square root of the variance, we therefore know the sample standard deviation, s, is 0.173. With this information we calculate the test statistic as -3.07, and mark it on the graph.

STEP 4 : Compare test statistic and the critical values Now we compare the test statistic and the critical value by placing the test statistic on the graph. We see that the test statistic is in the tail, decidedly greater than the critical value of 2.575. We note that even the very small difference between the hypothesized value and the sample value is still a large number of standard deviations. The sample mean is only 0.08 ounces different from the required level of 8 ounces, but it is 3 plus standard deviations away and thus we cannot accept the null hypothesis.
Three standard deviations of a test statistic will guarantee that the test will fail. The probability that anything is within three standard deviations is almost zero. Actually it is 0.0026 on the normal distribution, which is certainly almost zero in a practical sense. Our formal conclusion would be “ At a 99% level of significance we cannot accept the hypothesis that the sample mean came from a distribution with a mean of 8 ounces” Or less formally, and getting to the point, “At a 99% level of significance we conclude that the machine is under filling the bottles and is in need of repair”.
Media Attributions
- Type1Type2Error
- HypTestFig2
- HypTestFig3
- HypTestPValue
- OneTailTestFig5
- HypTestExam7
- HypTestExam8
Quantitative Analysis for Business Copyright © by Margo Bergman is licensed under a Creative Commons Attribution-NonCommercial-ShareAlike 4.0 International License , except where otherwise noted.
Share This Book
- Skip to main content
- Skip to primary sidebar
- Skip to footer
- QuestionPro

- Solutions Industries Gaming Automotive Sports and events Education Government Travel & Hospitality Financial Services Healthcare Cannabis Technology Use Case NPS+ Communities Audience Contactless surveys Mobile LivePolls Member Experience GDPR Positive People Science 360 Feedback Surveys
- Resources Blog eBooks Survey Templates Case Studies Training Help center

Home Market Research
Quantitative Research: What It Is, Practices & Methods
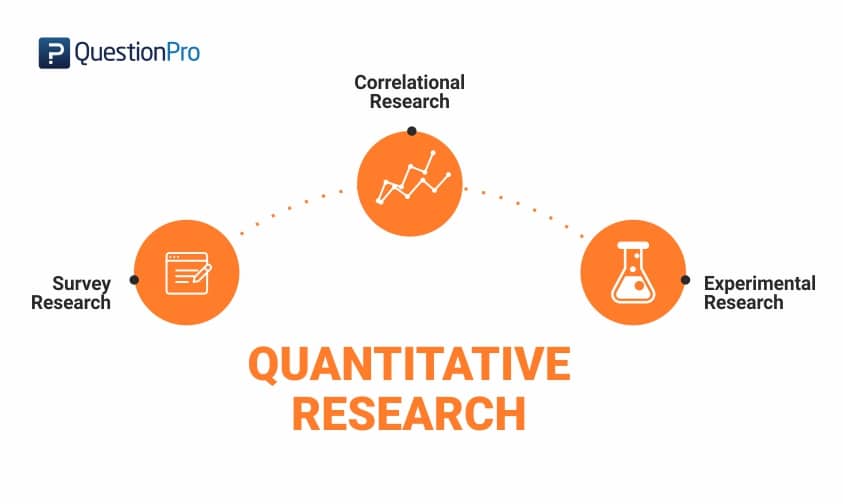
Quantitative research involves analyzing and gathering numerical data to uncover trends, calculate averages, evaluate relationships, and derive overarching insights. It’s used in various fields, including the natural and social sciences. Quantitative data analysis employs statistical techniques for processing and interpreting numeric data.
Research designs in the quantitative realm outline how data will be collected and analyzed with methods like experiments and surveys. Qualitative methods complement quantitative research by focusing on non-numerical data, adding depth to understanding. Data collection methods can be qualitative or quantitative, depending on research goals. Researchers often use a combination of both approaches to gain a comprehensive understanding of phenomena.
What is Quantitative Research?
Quantitative research is a systematic investigation of phenomena by gathering quantifiable data and performing statistical, mathematical, or computational techniques. Quantitative research collects statistically significant information from existing and potential customers using sampling methods and sending out online surveys , online polls , and questionnaires , for example.
One of the main characteristics of this type of research is that the results can be depicted in numerical form. After carefully collecting structured observations and understanding these numbers, it’s possible to predict the future of a product or service, establish causal relationships or Causal Research , and make changes accordingly. Quantitative research primarily centers on the analysis of numerical data and utilizes inferential statistics to derive conclusions that can be extrapolated to the broader population.
An example of a quantitative research study is the survey conducted to understand how long a doctor takes to tend to a patient when the patient walks into the hospital. A patient satisfaction survey can be administered to ask questions like how long a doctor takes to see a patient, how often a patient walks into a hospital, and other such questions, which are dependent variables in the research. This kind of research method is often employed in the social sciences, and it involves using mathematical frameworks and theories to effectively present data, ensuring that the results are logical, statistically sound, and unbiased.
Data collection in quantitative research uses a structured method and is typically conducted on larger samples representing the entire population. Researchers use quantitative methods to collect numerical data, which is then subjected to statistical analysis to determine statistically significant findings. This approach is valuable in both experimental research and social research, as it helps in making informed decisions and drawing reliable conclusions based on quantitative data.
Quantitative Research Characteristics
Quantitative research has several unique characteristics that make it well-suited for specific projects. Let’s explore the most crucial of these characteristics so that you can consider them when planning your next research project:
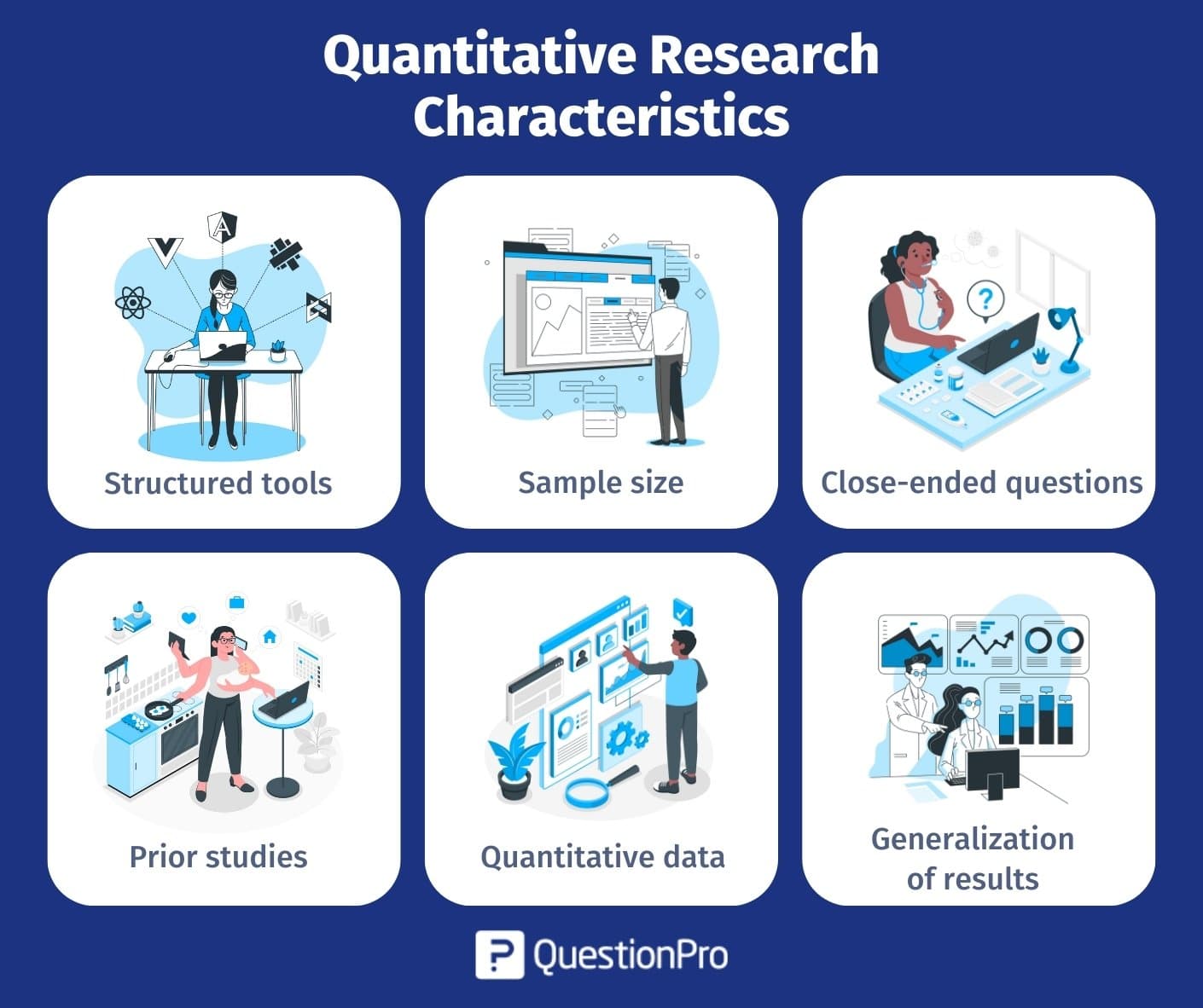
- Structured tools: Quantitative research relies on structured tools such as surveys, polls, or questionnaires to gather quantitative data . Using such structured methods helps collect in-depth and actionable numerical data from the survey respondents, making it easier to perform data analysis.
- Sample size: Quantitative research is conducted on a significant sample size representing the target market . Appropriate Survey Sampling methods, a fundamental aspect of quantitative research methods, must be employed when deriving the sample to fortify the research objective and ensure the reliability of the results.
- Close-ended questions: Closed-ended questions , specifically designed to align with the research objectives, are a cornerstone of quantitative research. These questions facilitate the collection of quantitative data and are extensively used in data collection processes.
- Prior studies: Before collecting feedback from respondents, researchers often delve into previous studies related to the research topic. This preliminary research helps frame the study effectively and ensures the data collection process is well-informed.
- Quantitative data: Typically, quantitative data is represented using tables, charts, graphs, or other numerical forms. This visual representation aids in understanding the collected data and is essential for rigorous data analysis, a key component of quantitative research methods.
- Generalization of results: One of the strengths of quantitative research is its ability to generalize results to the entire population. It means that the findings derived from a sample can be extrapolated to make informed decisions and take appropriate actions for improvement based on numerical data analysis.
Quantitative Research Methods
Quantitative research methods are systematic approaches used to gather and analyze numerical data to understand and draw conclusions about a phenomenon or population. Here are the quantitative research methods:
- Primary quantitative research methods
- Secondary quantitative research methods
Primary Quantitative Research Methods
Primary quantitative research is the most widely used method of conducting market research. The distinct feature of primary research is that the researcher focuses on collecting data directly rather than depending on data collected from previously done research. Primary quantitative research design can be broken down into three further distinctive tracks and the process flow. They are:
A. Techniques and Types of Studies
There are multiple types of primary quantitative research. They can be distinguished into the four following distinctive methods, which are:
01. Survey Research
Survey Research is fundamental for all quantitative outcome research methodologies and studies. Surveys are used to ask questions to a sample of respondents, using various types such as online polls, online surveys, paper questionnaires, web-intercept surveys , etc. Every small and big organization intends to understand what their customers think about their products and services, how well new features are faring in the market, and other such details.
By conducting survey research, an organization can ask multiple survey questions , collect data from a pool of customers, and analyze this collected data to produce numerical results. It is the first step towards collecting data for any research. You can use single ease questions . A single-ease question is a straightforward query that elicits a concise and uncomplicated response.
This type of research can be conducted with a specific target audience group and also can be conducted across multiple groups along with comparative analysis . A prerequisite for this type of research is that the sample of respondents must have randomly selected members. This way, a researcher can easily maintain the accuracy of the obtained results as a huge variety of respondents will be addressed using random selection.
Traditionally, survey research was conducted face-to-face or via phone calls. Still, with the progress made by online mediums such as email or social media, survey research has also spread to online mediums.There are two types of surveys , either of which can be chosen based on the time in hand and the kind of data required:
Cross-sectional surveys: Cross-sectional surveys are observational surveys conducted in situations where the researcher intends to collect data from a sample of the target population at a given point in time. Researchers can evaluate various variables at a particular time. Data gathered using this type of survey is from people who depict similarity in all variables except the variables which are considered for research . Throughout the survey, this one variable will stay constant.
- Cross-sectional surveys are popular with retail, SMEs, and healthcare industries. Information is garnered without modifying any parameters in the variable ecosystem.
- Multiple samples can be analyzed and compared using a cross-sectional survey research method.
- Multiple variables can be evaluated using this type of survey research.
- The only disadvantage of cross-sectional surveys is that the cause-effect relationship of variables cannot be established as it usually evaluates variables at a particular time and not across a continuous time frame.
Longitudinal surveys: Longitudinal surveys are also observational surveys , but unlike cross-sectional surveys, longitudinal surveys are conducted across various time durations to observe a change in respondent behavior and thought processes. This time can be days, months, years, or even decades. For instance, a researcher planning to analyze the change in buying habits of teenagers over 5 years will conduct longitudinal surveys.
- In cross-sectional surveys, the same variables were evaluated at a given time, and in longitudinal surveys, different variables can be analyzed at different intervals.
- Longitudinal surveys are extensively used in the field of medicine and applied sciences. Apart from these two fields, they are also used to observe a change in the market trend analysis , analyze customer satisfaction, or gain feedback on products/services.
- In situations where the sequence of events is highly essential, longitudinal surveys are used.
- Researchers say that when research subjects need to be thoroughly inspected before concluding, they rely on longitudinal surveys.
02. Correlational Research
A comparison between two entities is invariable. Correlation research is conducted to establish a relationship between two closely-knit entities and how one impacts the other, and what changes are eventually observed. This research method is carried out to give value to naturally occurring relationships, and a minimum of two different groups are required to conduct this quantitative research method successfully. Without assuming various aspects, a relationship between two groups or entities must be established.
Researchers use this quantitative research design to correlate two or more variables using mathematical analysis methods. Patterns, relationships, and trends between variables are concluded as they exist in their original setup. The impact of one of these variables on the other is observed, along with how it changes the relationship between the two variables. Researchers tend to manipulate one of the variables to attain the desired results.
Ideally, it is advised not to make conclusions merely based on correlational research. This is because it is not mandatory that if two variables are in sync that they are interrelated.
Example of Correlational Research Questions :
- The relationship between stress and depression.
- The equation between fame and money.
- The relation between activities in a third-grade class and its students.
03. Causal-comparative Research
This research method mainly depends on the factor of comparison. Also called quasi-experimental research , this quantitative research method is used by researchers to conclude the cause-effect equation between two or more variables, where one variable is dependent on the other independent variable. The independent variable is established but not manipulated, and its impact on the dependent variable is observed. These variables or groups must be formed as they exist in the natural setup. As the dependent and independent variables will always exist in a group, it is advised that the conclusions are carefully established by keeping all the factors in mind.
Causal-comparative research is not restricted to the statistical analysis of two variables but extends to analyzing how various variables or groups change under the influence of the same changes. This research is conducted irrespective of the type of relationship that exists between two or more variables. Statistical analysis plan is used to present the outcome using this quantitative research method.
Example of Causal-Comparative Research Questions:
- The impact of drugs on a teenager. The effect of good education on a freshman. The effect of substantial food provision in the villages of Africa.
04. Experimental Research
Also known as true experimentation, this research method relies on a theory. As the name suggests, experimental research is usually based on one or more theories. This theory has yet to be proven before and is merely a supposition. In experimental research, an analysis is done around proving or disproving the statement. This research method is used in natural sciences. Traditional research methods are more effective than modern techniques.
There can be multiple theories in experimental research. A theory is a statement that can be verified or refuted.
After establishing the statement, efforts are made to understand whether it is valid or invalid. This quantitative research method is mainly used in natural or social sciences as various statements must be proved right or wrong.
- Traditional research methods are more effective than modern techniques.
- Systematic teaching schedules help children who struggle to cope with the course.
- It is a boon to have responsible nursing staff for ailing parents.
B. Data Collection Methodologies
The second major step in primary quantitative research is data collection. Data collection can be divided into sampling methods and data collection using surveys and polls.
01. Data Collection Methodologies: Sampling Methods
There are two main sampling methods for quantitative research: Probability and Non-probability sampling .
Probability sampling: A theory of probability is used to filter individuals from a population and create samples in probability sampling . Participants of a sample are chosen by random selection processes. Each target audience member has an equal opportunity to be selected in the sample.
There are four main types of probability sampling:
- Simple random sampling: As the name indicates, simple random sampling is nothing but a random selection of elements for a sample. This sampling technique is implemented where the target population is considerably large.
- Stratified random sampling: In the stratified random sampling method , a large population is divided into groups (strata), and members of a sample are chosen randomly from these strata. The various segregated strata should ideally not overlap one another.
- Cluster sampling: Cluster sampling is a probability sampling method using which the main segment is divided into clusters, usually using geographic segmentation and demographic segmentation parameters.
- Systematic sampling: Systematic sampling is a technique where the starting point of the sample is chosen randomly, and all the other elements are chosen using a fixed interval. This interval is calculated by dividing the population size by the target sample size.
Non-probability sampling: Non-probability sampling is where the researcher’s knowledge and experience are used to create samples. Because of the researcher’s involvement, not all the target population members have an equal probability of being selected to be a part of a sample.
There are five non-probability sampling models:
- Convenience sampling: In convenience sampling , elements of a sample are chosen only due to one prime reason: their proximity to the researcher. These samples are quick and easy to implement as there is no other parameter of selection involved.
- Consecutive sampling: Consecutive sampling is quite similar to convenience sampling, except for the fact that researchers can choose a single element or a group of samples and conduct research consecutively over a significant period and then perform the same process with other samples.
- Quota sampling: Using quota sampling , researchers can select elements using their knowledge of target traits and personalities to form strata. Members of various strata can then be chosen to be a part of the sample as per the researcher’s understanding.
- Snowball sampling: Snowball sampling is conducted with target audiences who are difficult to contact and get information. It is popular in cases where the target audience for analysis research is rare to put together.
- Judgmental sampling: Judgmental sampling is a non-probability sampling method where samples are created only based on the researcher’s experience and research skill .
02. Data collection methodologies: Using surveys & polls
Once the sample is determined, then either surveys or polls can be distributed to collect the data for quantitative research.
Using surveys for primary quantitative research
A survey is defined as a research method used for collecting data from a pre-defined group of respondents to gain information and insights on various topics of interest. The ease of survey distribution and the wide number of people it can reach depending on the research time and objective makes it one of the most important aspects of conducting quantitative research.
Fundamental levels of measurement – nominal, ordinal, interval, and ratio scales
Four measurement scales are fundamental to creating a multiple-choice question in a survey. They are nominal, ordinal, interval, and ratio measurement scales without the fundamentals of which no multiple-choice questions can be created. Hence, it is crucial to understand these measurement levels to develop a robust survey.
Use of different question types
To conduct quantitative research, close-ended questions must be used in a survey. They can be a mix of multiple question types, including multiple-choice questions like semantic differential scale questions , rating scale questions , etc.
Survey Distribution and Survey Data Collection
In the above, we have seen the process of building a survey along with the research design to conduct primary quantitative research. Survey distribution to collect data is the other important aspect of the survey process. There are different ways of survey distribution. Some of the most commonly used methods are:
- Email: Sending a survey via email is the most widely used and effective survey distribution method. This method’s response rate is high because the respondents know your brand. You can use the QuestionPro email management feature to send out and collect survey responses.
- Buy respondents: Another effective way to distribute a survey and conduct primary quantitative research is to use a sample. Since the respondents are knowledgeable and are on the panel by their own will, responses are much higher.
- Embed survey on a website: Embedding a survey on a website increases a high number of responses as the respondent is already in close proximity to the brand when the survey pops up.
- Social distribution: Using social media to distribute the survey aids in collecting a higher number of responses from the people that are aware of the brand.
- QR code: QuestionPro QR codes store the URL for the survey. You can print/publish this code in magazines, signs, business cards, or on just about any object/medium.
- SMS survey: The SMS survey is a quick and time-effective way to collect a high number of responses.
- Offline Survey App: The QuestionPro App allows users to circulate surveys quickly, and the responses can be collected both online and offline.
Survey example
An example of a survey is a short customer satisfaction (CSAT) survey that can quickly be built and deployed to collect feedback about what the customer thinks about a brand and how satisfied and referenceable the brand is.
Using polls for primary quantitative research
Polls are a method to collect feedback using close-ended questions from a sample. The most commonly used types of polls are election polls and exit polls . Both of these are used to collect data from a large sample size but using basic question types like multiple-choice questions.
C. Data Analysis Techniques
The third aspect of primary quantitative research design is data analysis . After collecting raw data, there must be an analysis of this data to derive statistical inferences from this research. It is important to relate the results to the research objective and establish the statistical relevance of the results.
Remember to consider aspects of research that were not considered for the data collection process and report the difference between what was planned vs. what was actually executed.
It is then required to select precise Statistical Analysis Methods , such as SWOT, Conjoint, Cross-tabulation, etc., to analyze the quantitative data.
- SWOT analysis: SWOT Analysis stands for the acronym of Strengths, Weaknesses, Opportunities, and Threat analysis. Organizations use this statistical analysis technique to evaluate their performance internally and externally to develop effective strategies for improvement.
- Conjoint Analysis: Conjoint Analysis is a market analysis method to learn how individuals make complicated purchasing decisions. Trade-offs are involved in an individual’s daily activities, and these reflect their ability to decide from a complex list of product/service options.
- Cross-tabulation: Cross-tabulation is one of the preliminary statistical market analysis methods which establishes relationships, patterns, and trends within the various parameters of the research study.
- TURF Analysis: TURF Analysis , an acronym for Totally Unduplicated Reach and Frequency Analysis, is executed in situations where the reach of a favorable communication source is to be analyzed along with the frequency of this communication. It is used for understanding the potential of a target market.
Inferential statistics methods such as confidence interval, the margin of error, etc., can then be used to provide results.
Secondary Quantitative Research Methods
Secondary quantitative research or desk research is a research method that involves using already existing data or secondary data. Existing data is summarized and collated to increase the overall effectiveness of the research.
This research method involves collecting quantitative data from existing data sources like the internet, government resources, libraries, research reports, etc. Secondary quantitative research helps to validate the data collected from primary quantitative research and aid in strengthening or proving, or disproving previously collected data.
The following are five popularly used secondary quantitative research methods:
- Data available on the internet: With the high penetration of the internet and mobile devices, it has become increasingly easy to conduct quantitative research using the internet. Information about most research topics is available online, and this aids in boosting the validity of primary quantitative data.
- Government and non-government sources: Secondary quantitative research can also be conducted with the help of government and non-government sources that deal with market research reports. This data is highly reliable and in-depth and hence, can be used to increase the validity of quantitative research design.
- Public libraries: Now a sparingly used method of conducting quantitative research, it is still a reliable source of information, though. Public libraries have copies of important research that was conducted earlier. They are a storehouse of valuable information and documents from which information can be extracted.
- Educational institutions: Educational institutions conduct in-depth research on multiple topics, and hence, the reports that they publish are an important source of validation in quantitative research.
- Commercial information sources: Local newspapers, journals, magazines, radio, and TV stations are great sources to obtain data for secondary quantitative research. These commercial information sources have in-depth, first-hand information on market research, demographic segmentation, and similar subjects.
Quantitative Research Examples
Some examples of quantitative research are:
- A customer satisfaction template can be used if any organization would like to conduct a customer satisfaction (CSAT) survey . Through this kind of survey, an organization can collect quantitative data and metrics on the goodwill of the brand or organization in the customer’s mind based on multiple parameters such as product quality, pricing, customer experience, etc. This data can be collected by asking a net promoter score (NPS) question , matrix table questions, etc. that provide data in the form of numbers that can be analyzed and worked upon.
- Another example of quantitative research is an organization that conducts an event, collecting feedback from attendees about the value they see from the event. By using an event survey , the organization can collect actionable feedback about the satisfaction levels of customers during various phases of the event such as the sales, pre and post-event, the likelihood of recommending the organization to their friends and colleagues, hotel preferences for the future events and other such questions.
What are the Advantages of Quantitative Research?
There are many advantages to quantitative research. Some of the major advantages of why researchers use this method in market research are:
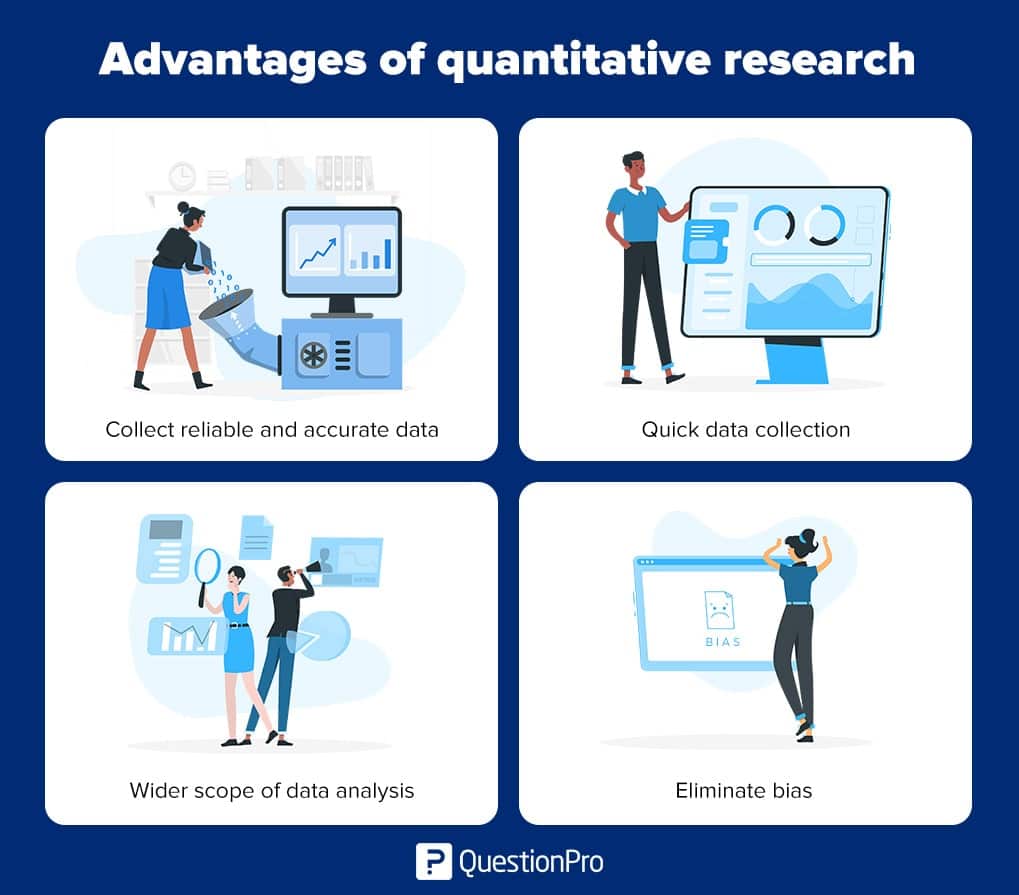
Collect Reliable and Accurate Data:
Quantitative research is a powerful method for collecting reliable and accurate quantitative data. Since data is collected, analyzed, and presented in numbers, the results obtained are incredibly reliable and objective. Numbers do not lie and offer an honest and precise picture of the conducted research without discrepancies. In situations where a researcher aims to eliminate bias and predict potential conflicts, quantitative research is the method of choice.
Quick Data Collection:
Quantitative research involves studying a group of people representing a larger population. Researchers use a survey or another quantitative research method to efficiently gather information from these participants, making the process of analyzing the data and identifying patterns faster and more manageable through the use of statistical analysis. This advantage makes quantitative research an attractive option for projects with time constraints.
Wider Scope of Data Analysis:
Quantitative research, thanks to its utilization of statistical methods, offers an extensive range of data collection and analysis. Researchers can delve into a broader spectrum of variables and relationships within the data, enabling a more thorough comprehension of the subject under investigation. This expanded scope is precious when dealing with complex research questions that require in-depth numerical analysis.
Eliminate Bias:
One of the significant advantages of quantitative research is its ability to eliminate bias. This research method leaves no room for personal comments or the biasing of results, as the findings are presented in numerical form. This objectivity makes the results fair and reliable in most cases, reducing the potential for researcher bias or subjectivity.
In summary, quantitative research involves collecting, analyzing, and presenting quantitative data using statistical analysis. It offers numerous advantages, including the collection of reliable and accurate data, quick data collection, a broader scope of data analysis, and the elimination of bias, making it a valuable approach in the field of research. When considering the benefits of quantitative research, it’s essential to recognize its strengths in contrast to qualitative methods and its role in collecting and analyzing numerical data for a more comprehensive understanding of research topics.
Best Practices to Conduct Quantitative Research
Here are some best practices for conducting quantitative research:
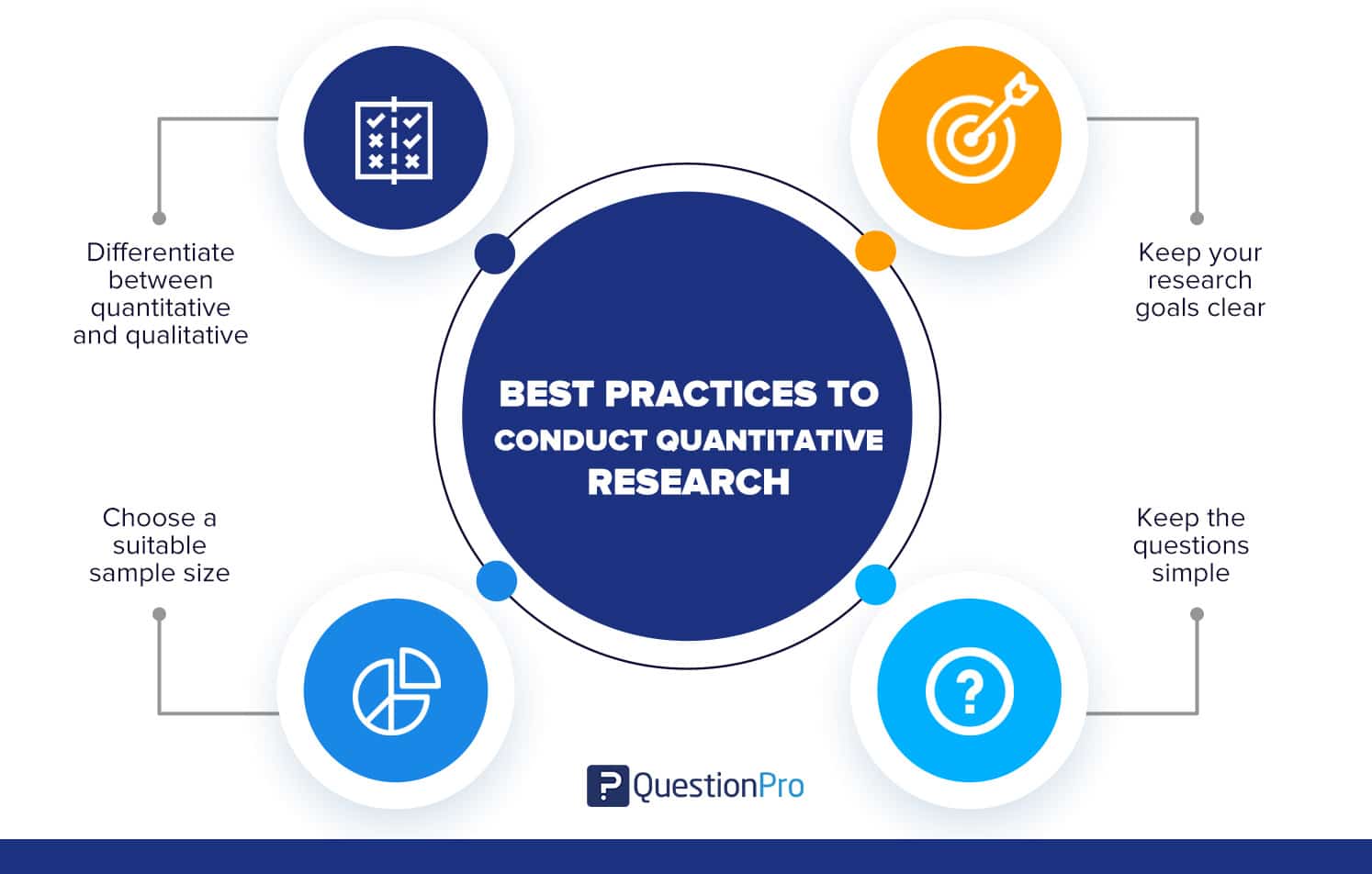
- Differentiate between quantitative and qualitative: Understand the difference between the two methodologies and apply the one that suits your needs best.
- Choose a suitable sample size: Ensure that you have a sample representative of your population and large enough to be statistically weighty.
- Keep your research goals clear and concise: Know your research goals before you begin data collection to ensure you collect the right amount and the right quantity of data.
- Keep the questions simple: Remember that you will be reaching out to a demographically wide audience. Pose simple questions for your respondents to understand easily.
Quantitative Research vs Qualitative Research
Quantitative research and qualitative research are two distinct approaches to conducting research, each with its own set of methods and objectives. Here’s a comparison of the two:
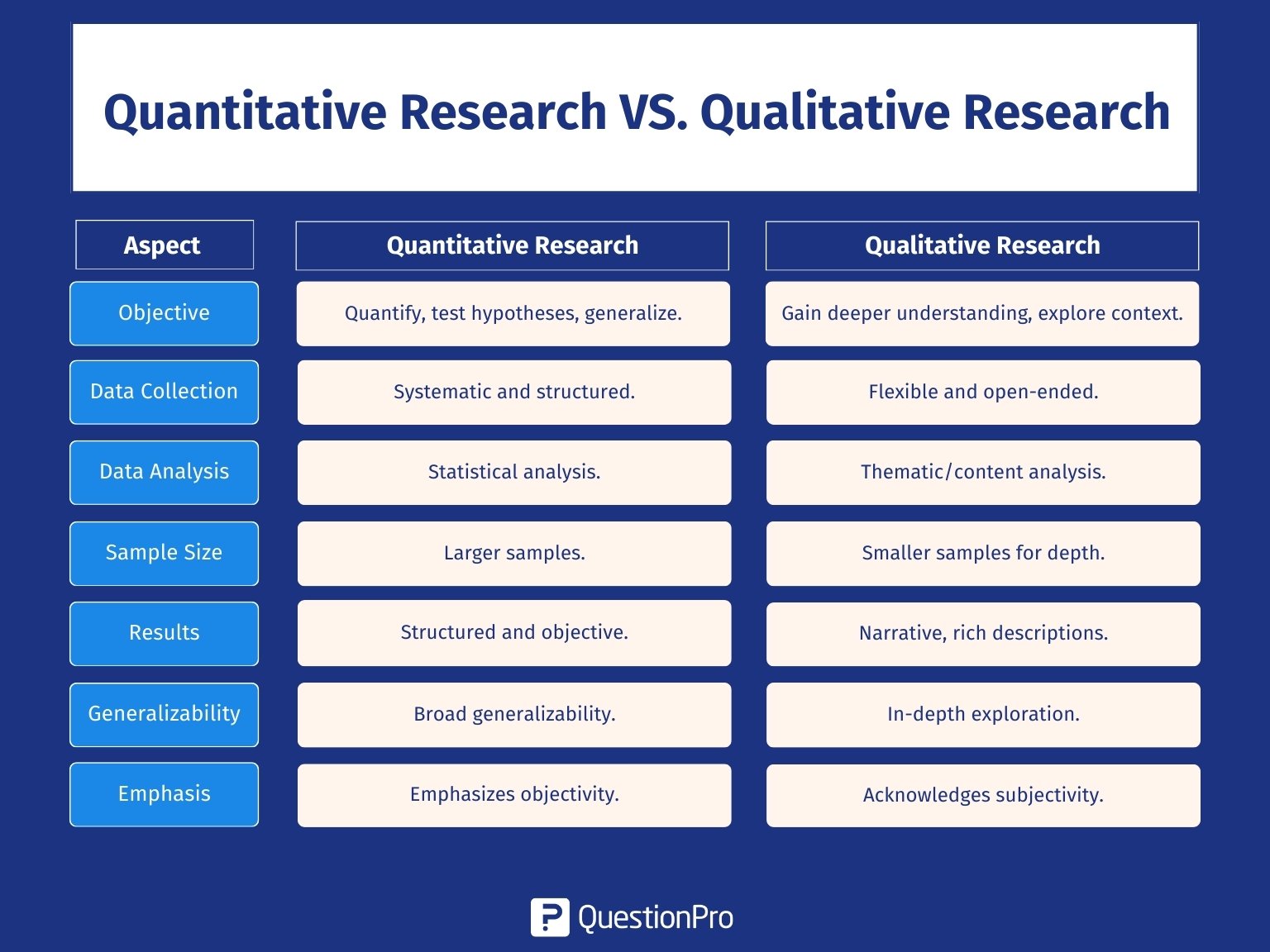
Quantitative Research
- Objective: The primary goal of quantitative research is to quantify and measure phenomena by collecting numerical data. It aims to test hypotheses, establish patterns, and generalize findings to a larger population.
- Data Collection: Quantitative research employs systematic and standardized approaches for data collection, including techniques like surveys, experiments, and observations that involve predefined variables. It is often collected from a large and representative sample.
- Data Analysis: Data is analyzed using statistical techniques, such as descriptive statistics, inferential statistics, and mathematical modeling. Researchers use statistical tests to draw conclusions and make generalizations based on numerical data.
- Sample Size: Quantitative research often involves larger sample sizes to ensure statistical significance and generalizability.
- Results: The results are typically presented in tables, charts, and statistical summaries, making them highly structured and objective.
- Generalizability: Researchers intentionally structure quantitative research to generate outcomes that can be helpful to a larger population, and they frequently seek to establish causative connections.
- Emphasis on Objectivity: Researchers aim to minimize bias and subjectivity, focusing on replicable and objective findings.
Qualitative Research
- Objective: Qualitative research seeks to gain a deeper understanding of the underlying motivations, behaviors, and experiences of individuals or groups. It explores the context and meaning of phenomena.
- Data Collection: Qualitative research employs adaptable and open-ended techniques for data collection, including methods like interviews, focus groups, observations, and content analysis. It allows participants to express their perspectives in their own words.
- Data Analysis: Data is analyzed through thematic analysis, content analysis, or grounded theory. Researchers focus on identifying patterns, themes, and insights in the data.
- Sample Size: Qualitative research typically involves smaller sample sizes due to the in-depth nature of data collection and analysis.
- Results: Findings are presented in narrative form, often in the participants’ own words. Results are subjective, context-dependent, and provide rich, detailed descriptions.
- Generalizability: Qualitative research does not aim for broad generalizability but focuses on in-depth exploration within a specific context. It provides a detailed understanding of a particular group or situation.
- Emphasis on Subjectivity: Researchers acknowledge the role of subjectivity and the researcher’s influence on the Research Process . Participant perspectives and experiences are central to the findings.
Researchers choose between quantitative and qualitative research methods based on their research objectives and the nature of the research question. Each approach has its advantages and drawbacks, and the decision between them hinges on the particular research objectives and the data needed to address research inquiries effectively.
Quantitative research is a structured way of collecting and analyzing data from various sources. Its purpose is to quantify the problem and understand its extent, seeking results that someone can project to a larger population.
Companies that use quantitative rather than qualitative research typically aim to measure magnitudes and seek objectively interpreted statistical results. So if you want to obtain quantitative data that helps you define the structured cause-and-effect relationship between the research problem and the factors, you should opt for this type of research.
At QuestionPro , we have various Best Data Collection Tools and features to conduct investigations of this type. You can create questionnaires and distribute them through our various methods. We also have sample services or various questions to guarantee the success of your study and the quality of the collected data.
Quantitative research is a systematic and structured approach to studying phenomena that involves the collection of measurable data and the application of statistical, mathematical, or computational techniques for analysis.
Quantitative research is characterized by structured tools like surveys, substantial sample sizes, closed-ended questions, reliance on prior studies, data presented numerically, and the ability to generalize findings to the broader population.
The two main methods of quantitative research are Primary quantitative research methods, involving data collection directly from sources, and Secondary quantitative research methods, which utilize existing data for analysis.
1.Surveying to measure employee engagement with numerical rating scales. 2.Analyzing sales data to identify trends in product demand and market share. 4.Examining test scores to assess the impact of a new teaching method on student performance. 4.Using website analytics to track user behavior and conversion rates for an online store.
1.Differentiate between quantitative and qualitative approaches. 2.Choose a representative sample size. 3.Define clear research goals before data collection. 4.Use simple and easily understandable survey questions.
MORE LIKE THIS
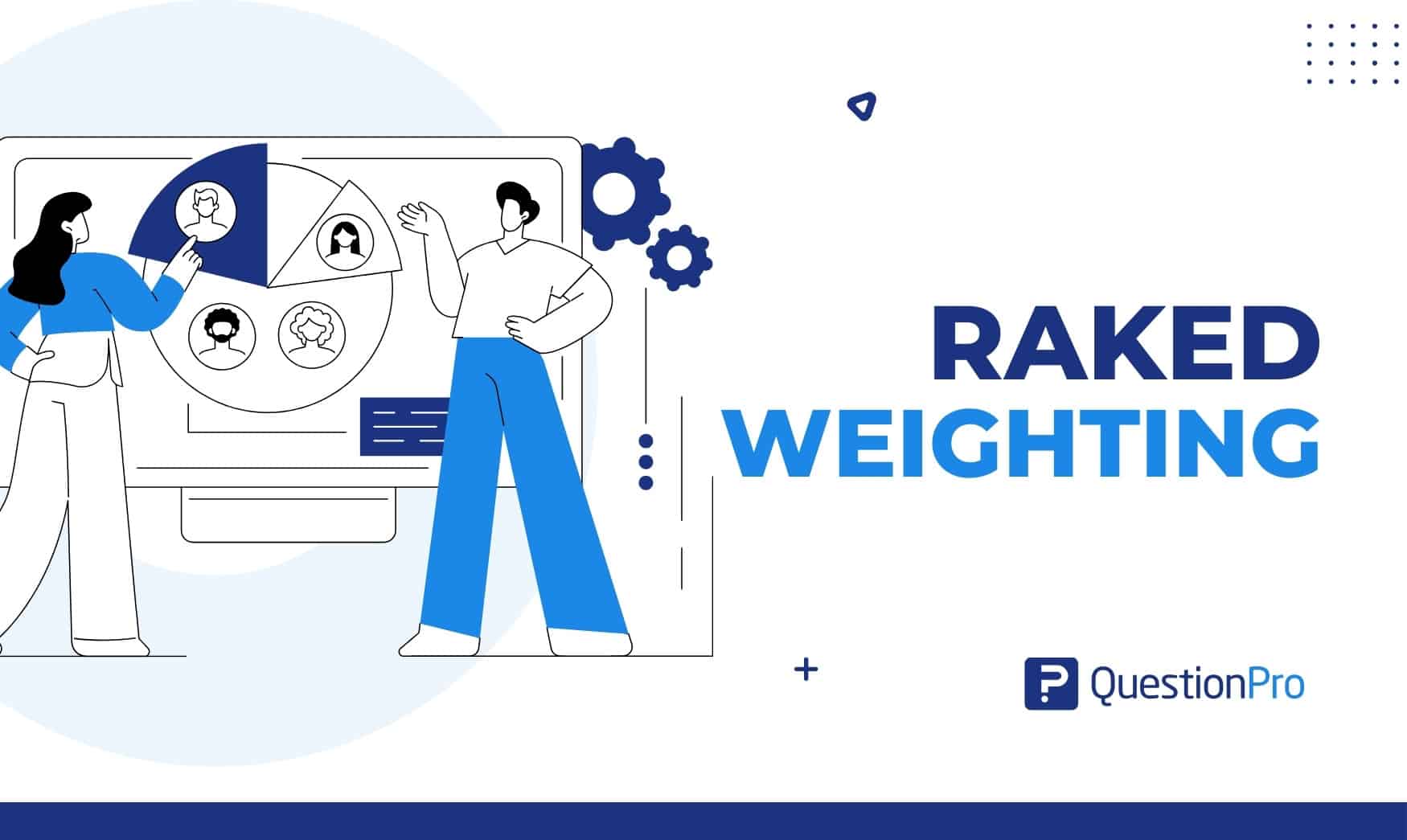
Raked Weighting: A Key Tool for Accurate Survey Results
May 31, 2024
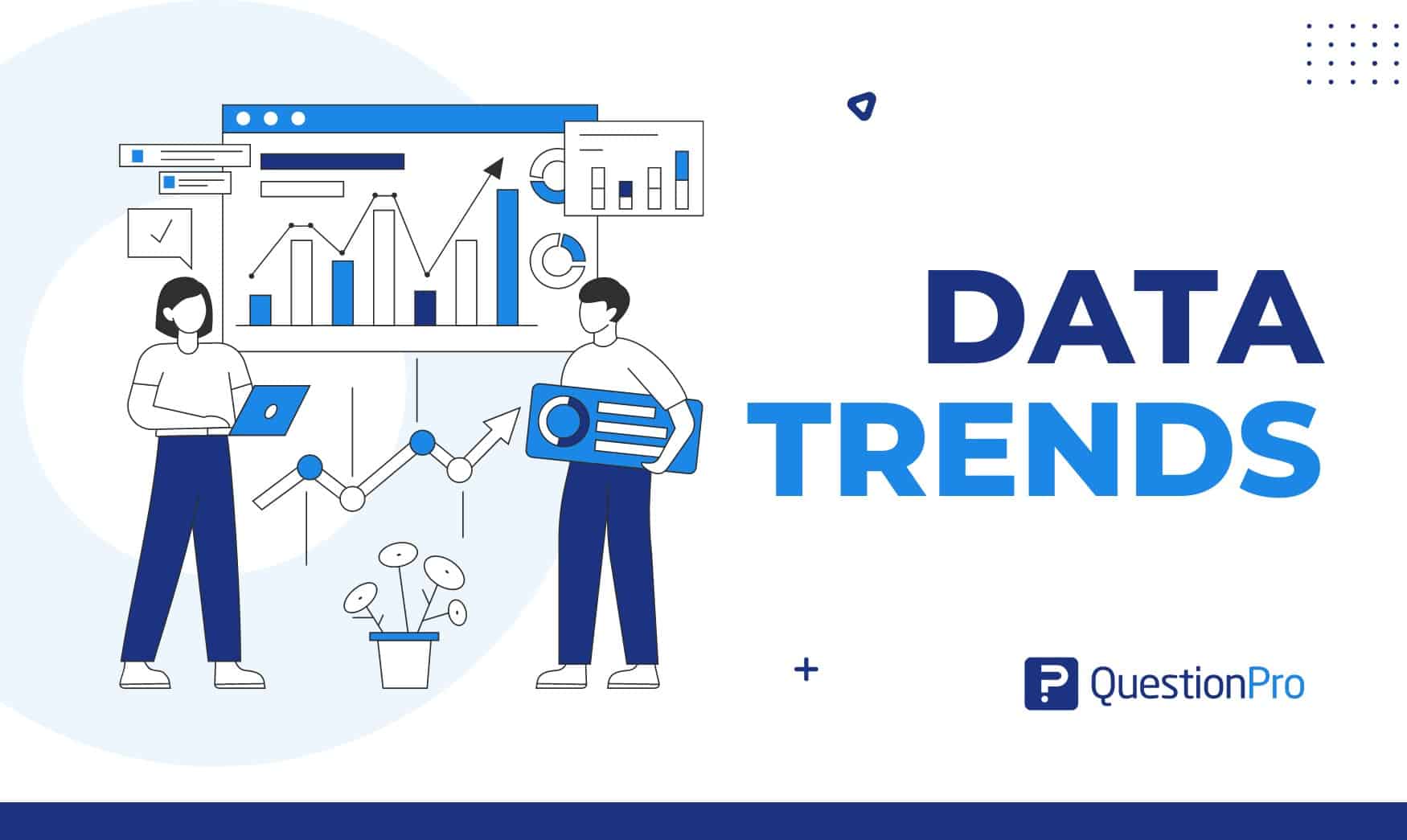
Top 8 Data Trends to Understand the Future of Data
May 30, 2024
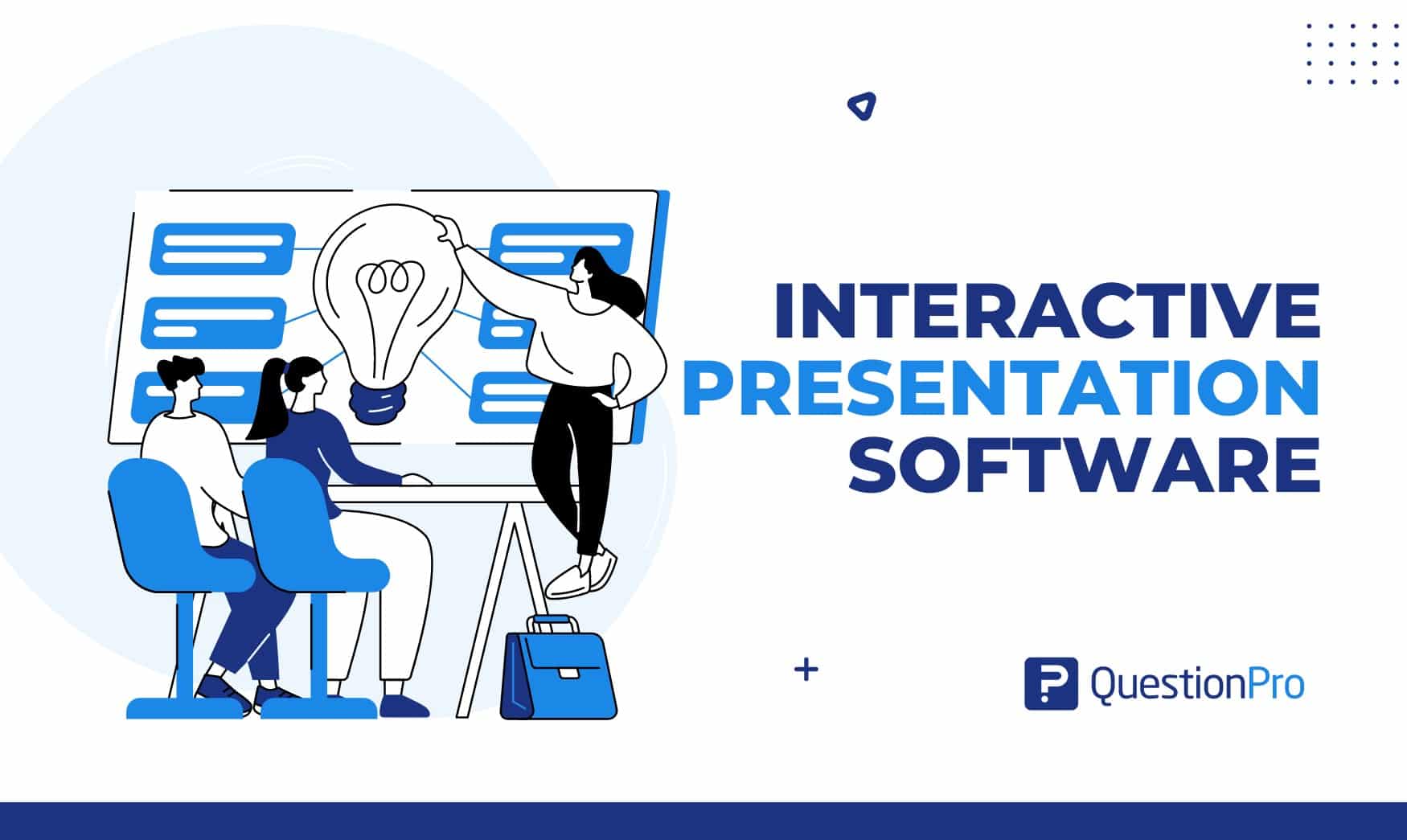
Top 12 Interactive Presentation Software to Engage Your User
May 29, 2024
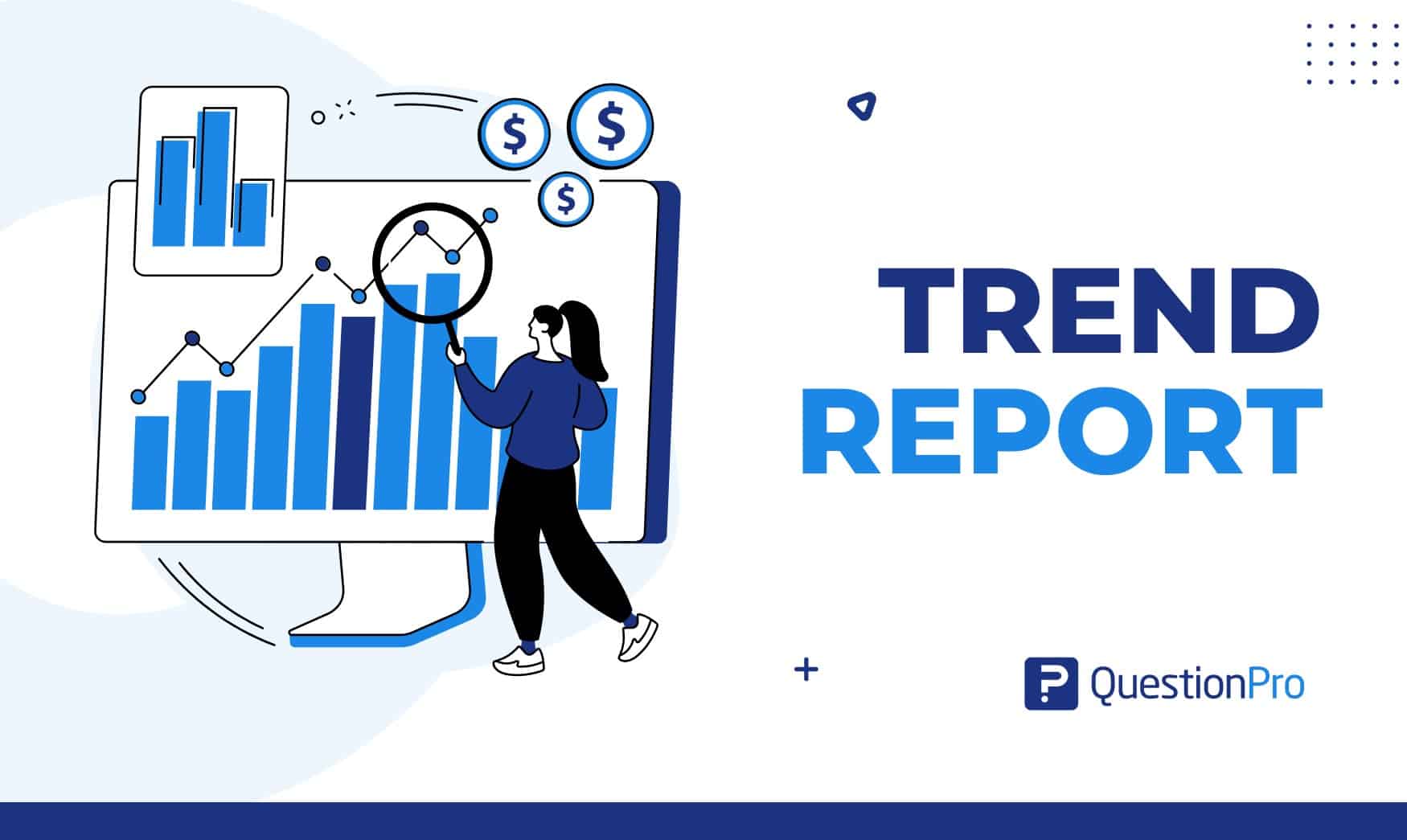
Trend Report: Guide for Market Dynamics & Strategic Analysis
Other categories.
- Academic Research
- Artificial Intelligence
- Assessments
- Brand Awareness
- Case Studies
- Communities
- Consumer Insights
- Customer effort score
- Customer Engagement
- Customer Experience
- Customer Loyalty
- Customer Research
- Customer Satisfaction
- Employee Benefits
- Employee Engagement
- Employee Retention
- Friday Five
- General Data Protection Regulation
- Insights Hub
- Life@QuestionPro
- Market Research
- Mobile diaries
- Mobile Surveys
- New Features
- Online Communities
- Question Types
- Questionnaire
- QuestionPro Products
- Release Notes
- Research Tools and Apps
- Revenue at Risk
- Survey Templates
- Training Tips
- Uncategorized
- Video Learning Series
- What’s Coming Up
- Workforce Intelligence
Click through the PLOS taxonomy to find articles in your field.
For more information about PLOS Subject Areas, click here .
Loading metrics
Open Access
Peer-reviewed
Research Article
Quantitative evidence for dimorphism suggests sexual selection in the maxillary caniniform process of Placerias hesternus
Roles Conceptualization, Data curation, Formal analysis, Investigation, Methodology, Project administration, Software, Visualization, Writing – original draft, Writing – review & editing
* E-mail: [email protected] (JLP); [email protected] (DVL)
Affiliation Department of Integrative Biology and Museum of Paleontology, University of California, Berkeley, California, United States of America

Roles Conceptualization, Project administration, Supervision, Writing – original draft, Writing – review & editing
Roles Conceptualization, Investigation, Project administration, Supervision, Visualization, Writing – original draft, Writing – review & editing
Affiliation Department of Geosciences, Virginia Tech, Blacksburg, Virginia, United States of America
Roles Conceptualization, Formal analysis, Investigation, Methodology, Software, Visualization, Writing – original draft, Writing – review & editing
- James L. Pinto,
- Charles R. Marshall,
- Sterling J. Nesbitt,
- Daniel Varajão de Latorre
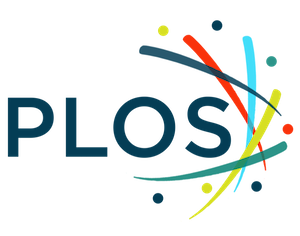
- Published: May 31, 2024
- https://doi.org/10.1371/journal.pone.0297894
- Peer Review
- Reader Comments
Placerias hesternus , a Late Triassic dicynodont, is one of the last megafaunal synapsids of the Mesozoic. The species has a tusk-like projection on its maxillary bone, known as the caniniform process. This process has been hypothesized to be sexually dimorphic since the 1950s, however this claim has not been thoroughly investigated quantitatively. Here, we examined maxillae, premaxillae, quadrates, and fibulae from a single population from the Placerias Quarry in the Blue Mesa Member of the Chinle Formation, near St. Johns, Arizona, USA to determine if the caniniform process is dimorphic. We made a total of 25 measurements from the four bones and used a maximum likelihood framework to compare the fit of unimodal versus bimodal distributions for each set of measurements. Our results from complete maxillae reveal that the caniniform process has two distinct morphs, with a shorter and longer form. This interpretation is substantiated both by strong statistical support for bimodal distribution of caniniform lengths, and by clustering analysis that clearly distinguishes two morphs for the maxillae. Clustering analysis also shows support for potential dimorphism in the shape of the quadrate. However, no measurements from elements other than the maxilla have a strong likelihood of bimodal distribution. These results support the long-standing hypothesis that the caniniform in Placerias was dimorphic. Alternative explanations to sexual dimorphism that could account for the dimorphism among these fossils include the presence of juveniles in the sample or time-averaged sampling of a chronospecies, but both have been previously rejected for the Placerias Quarry population. The lack of strong dimorphism in non-maxilla elements and increased variation in caniniform length of the large-caniniform morph suggest that the caniniform is a secondary sexual trait, possibly used in intraspecific competition.
Citation: Pinto JL, Marshall CR, Nesbitt SJ, Varajão de Latorre D (2024) Quantitative evidence for dimorphism suggests sexual selection in the maxillary caniniform process of Placerias hesternus . PLoS ONE 19(5): e0297894. https://doi.org/10.1371/journal.pone.0297894
Editor: Jun Liu, Chinese Academy of Sciences, CHINA
Received: August 18, 2023; Accepted: January 16, 2024; Published: May 31, 2024
Copyright: © 2024 Pinto et al. This is an open access article distributed under the terms of the Creative Commons Attribution License , which permits unrestricted use, distribution, and reproduction in any medium, provided the original author and source are credited.
Data Availability: All relevant data are within the manuscript and its Supporting information files.
Funding: Amended Funding Statement: CRM was partially supported by the Philip Sandford Boone Chair in Paleontology at the University of California, Berkeley. There was no additional external funding received for this study.
Competing interests: The authors have declared that no competing interests exist.
Introduction
Sexual dimorphism in dicynodonts.
Sexual dimorphism is notoriously difficult to establish in the fossil record [but see 1 , 2 ], especially in vertebrates, unless there are massive sample sizes [ 3 ]. The detection of sexual size dimorphism (SSD) has been particularly challenging because the upper and lower extremes of each sex’s size distribution often overlap, making it hard to distinguish the two even for extant taxa known to be sexually dimorphic, particularly if a priori knowledge of sex is removed [ 4 ]. In contrast, discontinuous secondary sexual traits, such as sexually dimorphic weaponry or ornamentation used for intraspecific combat or competition through visual display, are typically clearly dimorphic, either being significantly enlarged in, or entirely unique to, one sex [ 5 ]. Many living synapsids have features that fall into this second category, like the presence or size of a tusk or horn [ 6 ], and as a result the sex of an individual can be distinguished even from isolated skeletal elements. These structures sometimes continue to grow past sexual maturity in the competitive sex [ 7 ], usually males in living synapsids [ 8 ], which can lead to a positively allometric relationship between body size and secondary sexual structure size.
Tusks have evolved to become sexually dimorphic multiple times in synapsid groups, including in elephants, walruses, and muntjacs. The oldest known occurrence of this adaptation is in dicynodonts, a clade of non-mammalian therapsid synapsids. Genera like the Late Permian Diictodon have been demonstrated to have a sexually dimorphic presence or absence of tusks [ 9 ]. Many other dicynodonts have been argued to have sexually dimorphic weaponry or ornamentation, ranging from tusks to nasal bosses to varying robustness of facial bone structures such as the maxillary caniniform process. Sexual dimorphism in dicynodonts was first suggested in the 19th century in Lystrosaurus murrayi [ 10 ], and variation in cranial elements based on sex was proposed soon after [ 11 ]. Arguments for dimorphism have sometimes been quantitative, for example in Aulacephalodon [ 12 ] and Lystrosaurus [ 13 ], but have often been based on just qualitative differences among a handful of specimens, and thus need more data or analysis to be statistically supported. Examples include Dinodontosaurus [ 14 ], Tetragonias [ 15 ], Stahleckeria , Ischigualastia [ 16 ], Pelanomodon [ 17 ], and Wadiasaurus [ 18 ]. Wadiasaurus has also been speculated to have formed nursery herds of females and juveniles, similarly to modern elephants, based on the presence of an assemblage site with only tuskless and juvenile individuals, though remains from this taxon are mostly fragmentary, making these claims difficult to assess.
Dicynodonts in the Late Triassic
One of the earliest radiations of herbivorous megafauna following the end-Permian mass extinction was the kannemeyeriiform dicynodonts, a clade that ranged from the Induan in the earliest Triassic [ 19 ] to the Late Norian or Early Rhaetian [ 20 , 21 ] near the end of the Triassic. The last and largest members of this group all fall within the Stahleckeriidae [ 22 ], and are the latest Mesozoic occurrences of synapsid megafauna, with later synapsids (in the form of mammals) not reaching similar sizes until more than 140 million years later [ 23 ]. Stahleckeriidae has been viewed as a “relict taxon” of the Kannemeyeriformes, which were prolific throughout the Triassic [ 22 ], though the Stahleckeriidae was still taxonomically diverse and distributed across at least four continents in the Late Triassic [ 20 , 24 – 26 ]. However, most species are only known from a few individuals, most assemblages where they occur only bear one species [ 22 ], and they are less abundant than other contemporaneous groups such as the archosaurs. The only valid kannemeyeriiforms described from North America are the placeriine stahleckeriid Placerias hesternus [ 24 ], the only species in the genus Placerias , Eubrachiosaurus browni (a stahleckeriine stahleckeriid) [ 22 , 27 ], and Argodicynodon boreni (a placeriine stahleckeriid) [ 28 ], all of which are extremely rare in their respective known areas. The first of these taxa to be described, and by far the most well-known, is Placerias hesternus .
Placerias hesternus (hereafter referred to simply as Placerias ) is one of the largest known species of dicynodonts, and among the most massive herbivores known from the Chinle Formation, reaching three meters in length and possibly weighing over one tonne [ 29 ]. Placerias has a farily broad geographic distribution, having been found in the Chinle Formation in Arizona [ 30 ] and New Mexico [ 31 ], the Pekin Formation in North Carolina [ 32 ], and possibly the Dockum Group in Texas [ 33 ], but it is mostly known from fragmentary remains. The one exception is the Placerias Quarry, a small death assemblage site in the Blue Mesa Member of the Chinle Formation, near the boundaries of Petrified Forest National Park, southwest of the town of St. Johns, Arizona, USA, which has produced over 1700 elements from at least 41 individuals of Placerias [ 34 ]. As a result, Placerias provides a unique opportunity to observe individual variation in a single population of large Late Triassic dicynodonts, not offered by the other, rarer stahleckeriids.
The initial description of Placerias was over a century ago [ 24 ], but it was only described from a humerus until a comprehensive monograph of its anatomy was published based on material from Placerias Quarry [ 29 ]. The site, though extremely dense in bone material, is almost entirely composed of disarticulated bones, including separated skull bones. Camp and Welles’ monograph included a composite reconstruction of the skull of Placerias , which was physically constructed from bones of at least nine different individuals [ 29 ], with gaps filled in using plaster. The composite skull was created with the largest left and right maxillae from the collection (UCMP 24935 and PEFO 2369, respectively), and was meant to represent an individual among the largest in the population. The reconstructed skull was modified in a later publication by Cox [ 14 ], with revisions including replacing the maxillae with slightly smaller ones (UCMP 25317 and UCMP 25318), and changing their angle to improve articulation with the premaxilla ( Fig 1 ). An articulated partial skull of Placerias (MNA.V.8464) was later described from a different locality and supports Cox’s interpretation of the position of the maxilla, but it is poorly preserved and crushed, making anatomical analysis difficult [ 22 , 31 ].
- PPT PowerPoint slide
- PNG larger image
- TIFF original image
(A) photograph in left lateral view of a plaster cast based on the original composite reconstruction from Camp and Welles [ 29 ]; (B) the current version of the composite as revised in Cox [ 14 ]. Primary differences include shifting the maxilla and quadrate posteriorly in B, and the replacement of the maxillae in A with smaller elements. Abbreviations : mx , maxilla; pmx , premaxilla; q , quadrate. Arrow denotes anterior direction. Scale bar equals 10 cm.
https://doi.org/10.1371/journal.pone.0297894.g001
The composite skull and measurements from multiple specimens for each cranial element [ 29 ] show that Placerias had an extremely robust skull, with very large squamosals and jugals connected to rugose maxillae and premaxillae that were likely keratinized, suggesting a herbivorous diet of tough plant remains such as roots and tubers [ 35 ]. Like most dicynodonts, Placerias has only two teeth: a set of true tusks. While tusks evolved independently in multiple dicynodont groups [ 36 ], those found on Placerias and other kannemeyeriiforms lack enamel and have a cone-in-cone growth arrangement [ 29 , 37 ], much like the tusks of living mammalian synapsids such as walruses and elephants. Placerias , like all dicynodonts [ 38 ], has a projection of rugose bone on the maxilla lateral to the tusk, known as the “caniniform process”. The size and shape of the caniniform and the nearby tusk vary widely across the clade, with some species lacking tusks entirely. In Placerias the caniniform process forms a blade-like edge, particularly on the anterior surface ventral to the premaxillary suture. The posterior surface of the maxilla is wider mediolaterally and lacks this edge. The edge meets with the ventral edge of the premaxilla, creating a single continuous “beak”, similar to what is seen in most other stahleckeriids [ 16 ]. Unique to Placerias , however, is the extreme length of the caniniform, the tip of which lies ventral to the closed mandible in some individuals.
In their description of the Placerias Quarry material, Camp and Welles [ 29 ] identified two potential morphs among isolated maxillae, one with a much larger caniniform than the other, occurring in roughly equal numbers. From this observation, they hypothesized that Placerias was sexually dimorphic and suggested that the large-caniniform morph consisted of males while the small-caniniform morph represented females. However, this suggestion was based on a qualitative assessment only, and they did not provide a justification for the attribution of sexes to the morphs. Here, we statistically test for the presence of two morphs in the maxillae in the population from Placerias Quarry. Additionally, we ask whether there is any quantitative indication for the presence of two morphs in three other well-represented bones (the fibula, quadrate, and premaxilla) in the fossil assemblage. Tusks in dicynodonts have been speculated to be used for feeding, digging, and display [ 36 ]. In Placerias , we surveyed the presence and morphology of tusks in maxillae, and the morphology of dissociated tusks, to test the hypothesis that the caniniform replaced the tusks for these functions, and discuss evidence that the tusk is not under strong selection or essential for individual survival.
Materials and methods
Specimen assessment.
To quantitatively test Camp and Welles’ [ 29 ] hypothesis of dimorphism in the maxilla of Placerias , we analyzed a total of 36 isolated maxillae from the Placerias Quarry population housed in the UCMP and PEFO. Furthermore, to test if other skeletal elements of Placerias show evidence of dimorphism, we measured 29 premaxillae, 44 quadrates, and 17 fibulae (Full lists of specimen numbers are available in S1 Table ). No permits were required for the described study, which complied with all relevant regulations. We selected these elements because they have the largest sample sizes of bones complete enough to have identifiable homologous landmarks for making linear measurements. While both the premaxilla and the quadrate are cranial bones, the former articulates directly with the maxilla, and the latter does not. Hence, if there is dimorphism in the maxilla and it affects bones in its immediate vicinity, this would more likely be observed for the premaxilla than for the quadrates. Fibulae were included as the largest sample of a postcranial long bone, particularly because long-bone cross-sectional diameters are useful for estimating body size [ 39 ], and could reveal size dimorphism in Placerias .
Our sample of 36 maxillae (20 left maxillae, 16 right maxillae) has some overlap with the specimens analyzed by Camp and Welles [ 29 ]. However, the level of overlap is difficult to ascertain, because while they mention a total count of 39 maxillae (21 lefts and 18 rights) only 17 of those were referred to by a specimen number. Most of these were included in a table of 15 specimens, where they provided measurements of four traits. Two of those 15 specimens had specimen numbers that do not match any in the UCMP collection or database and thus are likely typos in their table. Under this assumption, the specimens they identify as UCMP 27319 and 28398 may well be UCMP 25319 and 28389, respectively. In addition to our sample of 36 maxillae, we found five unlabeled fragmentary maxillae, two of which had been sectioned. An additional 12 maxillae are referred to in the UCMP collections database, five of which have been reported as missing from the collection for decades, and we were not able to find any of these specimens in the collection. One maxilla (UCMP 27370) was figured by Camp and Welles [ 29 ], but is not in the UCMP database and was not located in the collection. Other than UCMP 27370 and the two potentially misreferred specimens, all maxillae mentioned by specimen number by Camp and Welles [ 29 ] are used in this study. Another maxilla (PEFO 2639) was relocated to Petrified Forest National Park for display purposes, but has a field number consistent with the Placerias Quarry specimens, and was evidently part of the original composite skull before being removed by Cox [ 14 ] in his modifications, based on its similarity to the maxilla in the cast of the original composite and the presence of a cut iron rod in the specimen that had been used to attach it to the rest of the skull. All of these labeling discrepancies complicate tracking the exact number of found maxillae, but in total, 49 maxillae collected by the UCMP from Placerias Quarry have been referred to in the past or otherwise theoretically exist, from at least 21 individuals, which falls well within the 41 minimum number of individuals reported from the site [ 34 ], based on left postorbitals, which though common are often fragmentary. Our work is based on a sample of at minimum 20 individuals, based on left maxillae. A list of specimen numbers of all specimens used is available in the S1 Table .
Within this sample, the quality of preservation is very uneven. Particularly, material from the site has suffered from diagenetic fracturing and from excavation or preparation related breakage (e.g, “marks of discovery” and other toolmarks) [ 34 ]. As a result, some of the maxillae are not fully intact, but they were included in the analysis if they were complete with respect to the specific measurement being taken. Extremely fragmentary specimens were considered only for the evaluation of presence/absence of tooth sockets. Determining which left and right elements come from the same individual is extremely difficult due to the disarticulated nature of the Placerias Quarry material. Camp and Welles [ 29 ] stated that some maxillae can be putatively paired, but there is no evidence for the proposed pairs beyond their roughly similar size and shape. Though some association between elements like osteoderms has been found in aetosaurs from Placerias Quarry [ 40 ], based on plotting their locations in positional grid squares, only one pair of maxillae (both numbered UCMP 27553) were found in the same grid square. These are of a similar size, and thus likely associated, but were not actually found connected, and so cannot be confirmed as a pair.
Similarly to the maxillae, many of the 29 premaxillae (24 fused pairs, four right fragments, one left fragment) in the UCMP collection were fragmentary, and only 9 were usable for complete length measurements. The 44 quadrates (23 lefts, 21 rights) also had varying levels of intactness, with 26 being complete enough for all length measurements, and 40 having an intact medial mandibular condyle, the proportions of which can be used as a rough proxy for quadrate size. Eight of the 17 fibulae were complete enough for all length measurements, and a further six had the proximal condyles present, the dorsoventral lengths of which can be used as a proxy for length of the overall fibulae.
Measurements
Maxillae were measured along the dorsoventral length from the dorsal tip of the jugal suture to the ventral tip of the caniniform process, or jugal-caniniform process dorsoventral (jcp DV) length, mediolateral width from the lateral tip of the jugal suture to the furthest medial point just beneath the pterygoid pit, or jugal-pterygoid pit mediolateral (jpp ML) width, and proximal anteroposterior length from the anterior tip of the premaxillary suture to the posterior tip of the jugal suture, or jugal-premaxillary anteroposterior (jpm AP) length. Tooth diameter (td) was also measured if the tooth was present, along with the depth of the large cavity proximal to the alveolus, or alveolar cavity depth (ad), as well as distance from the tooth socket (if present) to the caniniform tip (ttc), giving a total of 6 length measurements. The cavity proximal to the alveolus referred to here is a smooth conical depression on the posterior portion of the dorsal face of the maxilla, in the position of the dental chamber in other kannemeyeriiforms, and likely served a similar function. In some other kannemeyeriiforms (e.g. Kannemeyeria ), the alveolus and proximal end of the tooth is commonly exposed in the dental chamber, however in Placerias this has been reported to be much less common among individuals [ 29 ]. To assess this claim, we also recorded three nominal measurements relating to the tooth that were observed to vary among specimens: presence or absence of the tooth, presence or absence of tooth socket, and eruption of the tooth from the proximal end of alveolus ( Fig 2 ). Seven disassociated tusks were observed for presence of features including longitudinal grooves or variation in diameter across the root, to assess potential variation within the population.
(A) Medial view of a left large-caniniform morph maxilla (UCMP 24935); (B) Medial view of a left small-caniniform morph maxilla (UCMP 27552); (C) Dorsal view of UCMP 24935; (D) Dorsal view of UCMP 27552. Abbreviations : js , jugal suture; ps , premaxillary suture; ts , tooth socket; t , tooth; cp , caniniform process; ac , alveolar cavity; jcp DV, jugal-caniniform process dorsoventral, jpp ML, jugal-pterygoid pit mediolateral; jpm AP, jugal-premaxillary anteroposterior. Arrows denote anterior direction. Scale bars equals 2 cm.
https://doi.org/10.1371/journal.pone.0297894.g002
For the premaxillae, the following eight measurements were recorded: anterior beak tip to posterior nasal process tip (at to np), anterior tip to anterior end of maxillary suture tip (at to mxs), mediolateral length of palatal groove (pg ML), anterior end of maxillary suture tip to nasal process tip (mxs to np), anteroposterior length (AP), mediolateral length between maxillary sutures (mxs ML), depth of palatal groove (pg depth), and nasal process mediolateral length (np ML), ( Fig 3 ).
UCMP 25316. (A) Left lateral view; (B) Ventral view; Abbreviations : at , anterior tip; np , nasal process; mxs , maxillary suture; pg , palatal groove; ML , mediolateral; AP , anteroposterior. Arrows denote anterior direction. Scale bar equals 5 cm.
https://doi.org/10.1371/journal.pone.0297894.g003
The quadrates of Placerias have two condyles, the medial mandibular condyle (MMC) and the lateral mandibular condyle (LMC). For each condyle three measurements were recorded: the dorsoventral (DV), mediolateral (ML), and anteroposterior (AP) lengths, totaling six measurements ( Fig 4 ).
UCMP 24664, a right quadrate. (A) Posterior view; (B) Ventral view. Abbreviations : mmc , medial mandibular condyle; lmc , lateral mandibular condyle; DV , dorsoventral; ML , mediolateral; AP , anteroposterior. Scale bar equals 2 cm.
https://doi.org/10.1371/journal.pone.0297894.g004
For the fibulae, the following five measurements were recorded: distance from the tibial condyle to the femoral condyle (tc to fc), length of the distal condyle from tip to tip (dc tip to tip), distance from the tibial condyle to the distal condyle (tc to dc), the minimum dorsoventral midshaft diameter (ms DV), and the dorsoventral length of the proximal condyle (pc DV) ( Fig 5 ).
UCMP 24884, a right fibula. (A) Posterior view; (B) Lateral view. Abbreviations : tc , tibial condyle; fc , femoral condyle; dc , distal condyle; pc , proximal condyle; ms , midshaft; DV , dorsoventral; ML , mediolateral. Scale bar equals 5 cm.
https://doi.org/10.1371/journal.pone.0297894.g005
All measurements were taken to the nearest millimeter using 15cm long calipers or, for longer lengths, 1m long measuring tape, (measurement error of 1 mm), and were log 10 transformed prior to analysis.
Data analysis
We used two approaches to quantitatively test the hypothesis of dimorphism in Placerias for each of the four elements we measured. First, we used a maximum likelihood approach to estimate parameters that best describe linear measurements by comparing the fit of a unimodal and a bimodal distribution with Akaike’s Information Criterion corrected for small samples (AICc). We used a normal (Gaussian) distribution for the unimodal distribution, which requires the estimation of two parameters (mean and variance). For the bimodal distribution, we used a mixture of two normal distributions (Normal 1 and Normal 2 ), with a total of five parameters, the mean and variance of each normal (μ 1 ,σ 1 2 , μ 2 , σ 2 2 ) and a mixture parameter “ a ” that determines the relative contribution of each of the two normal distributions when they are combined. The parameter “ a ” is bound to vary between 0 and 1, where Normal 1 is multiplied by “ a ” and Normal 2 multiplied by “1- a ”. If “ a ” equals 0.5, then the data are best explained by two equal distributions, as would be expected from a 1:1 ratio of values from each distribution. This model fitting approach was repeated for each length measurement (a total of 25) on the four bones we sampled.
Second, we performed a principal component analysis (PCA) for each bone, with the variance of each measurement scaled so that measurements with larger values did not overly affect the results, to determine the relative position of each specimen in principal component space. We used the new PCA coordinates of each specimen as input for the clustering analysis k -means, which finds the best way to separate the data into two groups, a number preselected in the clustering analysis. We used scatter plots to visually inspect the two groups detected by k -means and determine if they lack overlap, indicating they are likely separate morphotypes, or if they overlap, indicating that they cannot be clearly separated. All statistical calculations were performed in R, and scripts are available as supplementary material ( S2 Table and S1 File ).
For the measurements that were better explained by the bimodal distribution, graphs were used to visualize which specimens contributed most to the positions of Normal 1 and Normal 2 . Note that the two distributions in the mixture function can sometimes have significant overlap, and that specimens in that region cannot be assigned to one group or the other. The bimodal model fitting and k-means clustering allowed for separation of specimens into groups (morphs). With this a priori knowledge we examined the potential correlation between sets of lengths, including those that were not distinct enough on their own to separate individuals into groups, by plotting them against each other.
Institutional Abbreviations: MNA, Museum of Northern Arizona, Flagstaff, AZ, USA; PEFO, Petrified Forest National Park, AZ, USA; UCMP, University of California Museum of Paleontology, Berkeley, CA, USA.
We found stronger support for a bimodal distribution than for a unimodal distribution in the 16 maxillae fully intact for jugal-caniniform process dorsoventral (jcp DV) length ( Fig 6A , Table 1 ). This measurement best captures the length of the caniniform process, and as such this result corroborates Camp & Welles’ [ 29 ] hypothesis of dimorphism for this trait in Placerias . The parameters that describe the bimodal distribution indicate an equal proportion of specimens contributing to each of the two distributions (parameter a = 0.5). The smaller distribution has a mean of 130.6mm (2.1159 in log 10 ), and the larger has a mean of 237.66mm (2.37596 in log 10 ). The standard deviations also differ, with the large-caniniform morph’s being much greater (large-caniniform morph SD = 0.064, small-caniniform morph SD = 0.034), and the coefficient of variation in the large-caniniform morph distribution is about 1.7 times that of the small-caniniform morph (large-caniniform morph CV = 0.0274, small-caniniform morph CV = 0.0159), indicating a greater variance in the jcp DV lengths of large-caniniform morphs even after taking into account their larger size. Other measurements of the maxillae show mixed results. Similar to the caniniform process length, the jugal-pterygoid pit mediolateral (jpp ML) width of the maxillae was better described by a bimodal than a unimodal distribution for the 28 maxillae complete for this measurement ( Fig 6B , Table 1 ). However, in the 23 maxillae with fully intact jugal-premaxillary anteroposterior (jpm AP) lengths, there is strong statistical support for a unimodal distribution ( Fig 6C , Table 1 ).
(A) Jugal-caniniform process dorsoventral (jcp DV) lengths of 16 maxillae fully intact along that length, with a curve showing supported bimodal distribution. (B) Jugal-pterygoid pit mediolateral (jpp ML) widths of 28 maxillae fully intact along that width, with a curve showing supported bimodal distribution. (C) Jugal-premaxillary anteroposterior (jpm AP) length of 23 maxillae fully intact along that length, with a supported unimodal distribution.
https://doi.org/10.1371/journal.pone.0297894.g006
See text and figures for measurement acronyms. ‘n’ is the number of specimens measured; dAICc is AICc difference for the model with the lowest AICc.
https://doi.org/10.1371/journal.pone.0297894.t001
The clustering analysis k-means also detects two distinct groups of specimens, which can be seen when the jcp DV, jpp ML, and jpm PAP lengths are plotted in a PCA ( Fig 7 ). The distribution of specimens between the two clusters corresponds exactly with their classification based on jcp DV length, and they are mostly separated along the second principal component (Dim2), indicating this length alone is sufficient alone to determine whether a specimen is a small-caniniform (hereafter referred to as “small”) or large-caniniform (hereafter referred to as “large”) morph.
Clusters from k-means align with assignment of large and small morphs based on dorsoventral length.
https://doi.org/10.1371/journal.pone.0297894.g007
In the 16 specimens with both the jcp DV length and jpp ML widths intact, the small and large morph are clearly separated along both of these lengths ( Fig 8A ). There is also a strong positive correlation between jcp DV length and jpp ML width in the large morph ( r 2 = 0.771, p = 0.002, n = 8), but not in the small one ( r 2 = -0.133, p = 0.685, n = 7). This likely reflects the caniniform becoming rounder in cross section in the large morph with increasing jcp DV length. This further distinguishes the large morph from the small one, which is more wedge-shaped in cross section, and further substantiates the differences between the two morphs. For the jcp DV and jpm AP lengths in the 14 specimens with both of these lengths intact, the distributions of jpm AP length in each morph differ, but overlap ( Fig 8B ). There is also a positive correlation between these lengths in the large morph ( r 2 = 0.505, p = 0.029, n = 8), but not in the small one ( r 2 = 0.28, p = 0.1275, n = 7).
(A) jugal-caniniform process dorsoventral (jcp DV) length and jugal-pterygoid pit mediolateral (jpp ML) width; (B) jcp DV and jugal-premaxillary anteroposterior (jpm AP) lengths. The r 2 values show the strength of correlation between lengths in the large morph; in the small morph, neither pair of lengths was significantly correlated.
https://doi.org/10.1371/journal.pone.0297894.g008
The small morph maxilla of Placerias is relatively consistent in proportion between individuals ( Fig 9A ). Generally, jcp DV and jpm PAP length are much closer to equal than in the large morph, with the jpp ML width being larger relative to jpm AP length. This results in an overall “slimmer” form in the small morph, with a single anterior edge, and a posterior “wall” with medial and lateral edges, leading to the wedge-like dorsoventral cross-sectional shape. The large morph maxilla of Placerias is less consistent in proportion between individuals, but is still distinct from the small morph ( Fig 9B ). The jcp DV length is always longer than jpm AP length, in some individuals by over 2 times. As jcp DV length and jpp ML width increase, the anterior surface of the maxilla, particularly the portion ventral to the tooth, becomes less of an edge, becoming round in cross section. The caniniform in the larger morph extends ventrally beyond the rest of the skull ( Fig 10 ).
Note the greater variance within the large morph. Classification of morphs is based on the difference in proportion between jugal-caniniform process dorsoventral (jcp DV) length and jugal-pterygoid pit mediolateral (jpp ML) width, compared to jugal-premaxillary anteroposterior (jpm AP) length. Abbreviations : ts , tooth socket; t , tooth; cp , caniniform process; Arrow denotes anterior direction. Scale bar equals 5 cm.
https://doi.org/10.1371/journal.pone.0297894.g009
Differing gray values show the different morphs of maxillae placed in the context of the rest of the skull. Note that the skull is scaled to the large morph, and the small morph likely was associated with a slightly smaller absolute skull size. Arrow denotes anterior direction. Scale bar equals 5 cm.
https://doi.org/10.1371/journal.pone.0297894.g010
In large-caniniform morphs, the tooth is distant from the ventral edge of the maxilla due to the increased caniniform length, but relative to other features, such as the anterior pit and premaxillary and jugal sutures, it is in roughly the same location as in the small-caniniform morph. There is a proportional increase in jcp DV length in the large morph across the entire maxilla, not just in the area between the caniniform and the tooth socket, but it appears that most of the difference in jcp DV length between morphs is ventral to the tooth socket. This is also indicated by the strong positive correlation between longer tooth socket-to-caniniform tip length and overall jcp DV length across both morphs ( r 2 = 0.905, p = 1.058 * 10 −7 , n = 14). Taking the morphs by themselves, this correlation is also present in the large morph ( r 2 = 0.870, p = 0.0004, n = 8), but not the small morph ( r 2 = -0.196, p = 0.619, n = 6). Given this and the supported bimodality in jcp DV length, it would be expected for the distribution of lengths from tooth socket to caniniform tip taken by itself to be bimodal. However, model comparison indicated that this length is better described by a single unimodal distribution than a bimodal one (ttc in Table 1 ).
There is more relative variation in ttc length than in jcp DV length, but the lack of support for bimodality may result from the amount of ttc length variation within each morph being close to the amount of variation between them. While the small-caniniform morph maxillae all have much smaller ttc lengths than any large-caniniform morph maxillae, the variance within each morph, particularly the large-caniniform morph, significantly impacts the overall distribution. The relative size of gaps in the expected range of ttc length within each morph are as large as the gap between the ranges of the small and large morphs, which results in the distribution having more than two clear clusters, and not being recognized as bimodal. These gaps in the expected ranges are likely the result of the relatively small sample size. With this small of a sample, the AIC comparison favors the simpler unimodal model with fewer parameters, and potential dimorphism has not been recognized.
The depth of the proximal alveolar cavity (extending dorsally towards the inside of the skull) in the maxillae depends on the morph, with large morph maxillae having greater cavity depth. There is a weak but statistically significant ( p < 0.05) correlation between alveolar cavity depth and both jcp DV length ( r 2 = 0.3025) and jpp ML width ( r 2 = 0.5342), traits shown to be dimorphic, but not proximal anteroposterior length, which lacks strong evidence for dimorphism. While alveolar depth itself was not shown to have a high log-likelihood of bimodal distribution in the 20 maxillae with the cavity intact (ad in Table 1 ), it correlates more strongly with the dimorphic caniniform traits than with absolute maxilla size.
Tooth characters
Out of 32 maxilla which were intact enough to determine presence or absence of a tooth socket, four (UCMP 28391, 27547, 27538, PEFO 2639) lack any sign of tooth eruption or socket ( Fig 11A ). The other 28 maxillae all have a visible tooth socket present, indicating that the tooth erupted, though only 20 have the tooth present in the socket, with the teeth of the other 8 likely having been dissociated from their sockets post-mortem. In four different individuals (UCMP 25319, 27552, 27555, 32389) the tooth erupts out through both the distal and proximal end of the alveolus, projecting into the alveolar cavity of the skull ( Fig 11B ). Putative pathologies were also observed. Of particular note is specimen UCMP 27550, in which the tooth erupts out near the anterior edge of the maxilla instead of the posterior, and is surrounded by bone that appears to be abscessed ( Fig 11C ).
(A) Medial view of a right small morph maxilla (UCMP 28391), with no sign of tooth eruption or tooth socket; (B) Medial view of a left large morph maxilla (UCMP 27550) with the tooth erupting near the anterior side of the maxilla instead of the typical eruption site near the posterior, and with abcessed surrounding bone. (C) Dorsal views of two right small morph maxillae (from left to right: UCMP 28391, UCMP 27552) with UCMP 27552 showing the uncommon condition of the tooth (circled) erupting through the proximal end of the alveolus; (D) Three disassociated teeth (from left to right: UCMP 32443, UCMP 32444, UCMP 24915) showing variance in the shape and surface texture of the root and crown, including differing extents of circumferential diameter oscillation (“ribbing”) (left), of longitudinal grooves (right) running along the length of the root, and both of these features in combination (middle), along with more conical (right) and obliquely sheared (left) crown shapes. Abbreviations : t , tooth. Arrows denote anterior direction. Scale bars equal 5 cm.
https://doi.org/10.1371/journal.pone.0297894.g011
Disassociated teeth, which were found separated from maxillae and have fully exposed roots, have highly variable root morphology. In seven teeth, the tooth root shafts show variability in two main traits: the presence of longitudinal grooves running down the shaft, and the repeated oscillation of tooth diameter along the shaft ( Fig 11D ). Some teeth only show the oscillations (e.g. UCMP 32443), some show only the grooves (e.g. UCMP 24915), and some show both (e.g. UCMP 32444) ( Fig 11D ). These patterns suggest differences in growth between individuals, possibly related to differing rates of growth over the course of ontogeny. Irregular growth in dicynodont teeth has also been linked to developmental stress from sources like drought conditions [ 41 ]. The crown shape also varies by individual, with some (e.g. UCMP 24915) having a more pointed, cone-like tip, and others (e.g. UCMP 32444) having a single worn down edge, suggesting different levels of tooth wear between individuals. Overall, the differences in growth patterns and presence of pathologies in individuals that all reached adult sizes provides evidence that the tooth being present or in a particular position was not necessary for individual survival. This combined with the proportionately small size of the tooth, provide evidence that it likely evolved neutrally, with little pressure to maintain functionality, and was essentially vestigial.
Though Camp and Welles [ 29 ] speculated that tooth diameter and caniniform morphotype are correlated, we found no evidence for this. In the 19 measured maxillae with intact teeth, there is roughly equal support for a unimodal and a bimodal distribution (td in Table 1 ) of tooth diameter, with AICc weights of 0.53 and 0.47, respectively. However, tooth diameter does not correspond to the caniniform dimorphism, as some specimens with larger caniniforms have smaller tusks, and vice-versa. Further, the distributions are not distinct enough to separate teeth into unambiguous morphs as can be done for the caniniform, and tooth size is not correlated to dorsoventral or mediolateral length. Whether or not teeth are in fact dimorphic, evidence presented here for the tooth’s lack of functionality and the relatively small range of their diameters (13–19mm) imply that any possible dimorphism had little functional significance.
Premaxillae
In the nine premaxillae measured, there was no strong evidence of dimorphism in proportions. Of the eight lengths measured, none had strongly bimodal distributions. The small sample size makes it difficult to confidently assess the log-likelihoods of bimodality, but two morphs of premaxillae are also not apparent visually. The distributions of two traits, the distance from the anterior tip to the nasal process and the anteroposterior length, were only narrowly supported as normal ( Table 1 ), with the AICc difference between distribution models in these traits not being sufficient to confidently support either a bimodal or unimodal distribution ( Fig 12A ), but all other measurements were strongly supported as having unimodal distributions.
(A) Distribution of the length from the anterior tip to nasal process, not showing high log-likelihood of either bimodality or unimodality, but having two peaks; (B) PCA plot of all measured premaxilla lengths grouped into 2 k-means clusters with high overlap, showing poor evidence for dimorphism. (C) PCA plot of measured premaxillae lengths excluding the palatal groove measurements, showing more distinct k-means clusters.
https://doi.org/10.1371/journal.pone.0297894.g012
Clustering the data on a PCA using k-means shows the lack of dimorphism in premaxillae when all measured traits are included ( Fig 12B ). However, removing the mediolateral width and the depth of the palatal groove (pg ML and pg depth in Table 1 ) from the PCA leads to two somewhat distinct k-means clusters ( Fig 12C ). This clustering is consistent with a statement made by Camp and Welles [ 29 ] that the “narrowest” premaxillae might belong to the small morph, and the “broadest” to the large morph. While our results do not explicitly support this interpretation, they do hint that the variance in the shape of the premaxilla may be in some way related to the morph of the maxilla, but it is also possible the differences are driven simply by variation in skull size, rather than sexual dimorphism.
In the 44 quadrates measured, none of the six measured lengths had distributions that were strongly bimodal ( Table 1 ). This is particularly important given the relatively large sample size for this element. However, while not statistically strongly supported as bimodal, distributions of three of the six lengths measured, all associated with the medial mandibular condyle (MMC), show visual signs of bimodality ( Fig 13A–13C ). This is reflected in the histograms of these traits, all of which are correlated to each other, having two visually apparent peaks. The MMC traits also separate into two distinct clusters based on k-means ( Fig 13E ). This points to the possibility that variance in MMC shape is best explained by two overlapping normal distributions that, taken by themselves, are not distinct enough to delineate morphs or statistically support a bimodal distribution. It is worth noting that the bimodal model had nearly equal likelihood than the unimodal model for the MMC dorsoventral (DV) length, despite that model receiving less AICc support after considering that it has higher number fitted parameters.
(A) Distribution of MMC dorsoventral length, without a high log-likelihood of either bimodality or unimodality; (B) Distribution of MMC mediolateral length, showing possible visual bimodality, but with a high log-likelihood of unimodality; (C) Distribution of MMC anteroposterior length, also with a high log-likelihood of unimodality; (D) PCA plot of all measured quadrate lengths from the MMC and lateral mandibular condyle (LMC) grouped into 2 k-means clusters with high overlap, showing poor evidence for dimorphism; (E) PCA plot of measured quadrate MMC lengths, grouped into 2 k-means clusters with little overlap, showing potential evidence for dimorphism.
https://doi.org/10.1371/journal.pone.0297894.g013
The distributions of the other lengths measured, namely those of the lateral mandibular condyle (LMC), were all supported as unimodal, and when added to the MMC PCA make the k-means clusters no longer distinct ( Fig 13D ). This suggests that there are two modules for the quadrate, the LMC and the MMC. While the data showed weak suggestions of bimodality on the MMC, which could be correlated with bimodality in the maxilla, the LMC does not show any indication of bimodality. The LMC pattern prevails when all quadrate measurements were analyzed together in one PCA and k-means analysis. Thus, the potential dimorphism in the quadrate could be in the relative size of the MMC, rather than overall absolute size of the quadrate. However, the MMC was also represented in more specimens than the LMC, so this difference in apparent dimorphism could be the result of the different sample sizes for these portions of the quadrate. While there is no significant statistical support for dimorphism in the quadrate in Placerias , our data raise the possibility that the relative size of the MMC in the quadrate might be correlated to caniniform morph if associated with the maxilla.
In the 17 fibulae measured, there is not strong evidence for proportional dimorphism. None of the five traits measured had strong support for a bimodal distribution ( Table 1 ). Two traits, the length from the tibial condyle to the distal condyle (tc to dc in Table 1 ) and the dorsoventral midshaft diameter (ms DV in Table 1 ), have distributions with two visually apparent peaks ( Fig 14A and 14B ), but these lengths are represented by particularly low sample sizes; just eight fibulae were complete enough to measure tibial condyle to distal condyle length, and just 12 were complete enough to measure dorsoventral midshaft diameter. In both of these measurements, two fibulae are separated from the rest, these being the largest fibulae for all other measured lengths. These two fibulae also form a separate cluster based on k-means ( Fig 14C ), however when the tibial condyle to distal condyle length is removed the clusters are no longer distinct ( Fig 14D ). Given this, and the fact that measured lengths are all generally positively correlated with each other, it is possible that this difference in proportion stems from a positively allometric relationship between diaphyseal size and epiphyseal size of the fibula.
(A) Distribution of TC to DC lengths, with 2 larger fibulae visually separate from the rest of the sample, but statistically overwhelmingly supported as unimodal; (B) Distribution of dorsoventral midshaft diameters, with two visually apparent peaks, also supported as unimodal. (C) PCA plot of all measured traits, with k-means clusters showing separation of UCMP 24875 and 32446 from the rest of the sample; (D) PCA plot of all measured traits except for TC to DC length, showing relatively poor separation into distinct clusters through k-means.
https://doi.org/10.1371/journal.pone.0297894.g014
In the 2 larger fibulae, tibial condyle to distal condyle length and dorsoventral midshaft diameter, lengths related to the size of the diaphysis, are proportionately larger compared to the other measurements, which are related to the size of the epiphyses, than in the smaller fibulae. This would imply that diaphysis size increases faster than epiphysis size as overall body size increases. This may better explain the variation in fibula proportion than sexual dimorphism, and it may be more sensible to interpret the distribution of tibial condyle to distal condyle length ( Fig 14A ), which is the main driver of separation of clusters based on k-means ( Fig 14C ), as a normal distribution with two outlier values, as is supported statistically, rather than a bimodal distribution. While proportional diaphysis size could potentially be correlated with caniniform morph, with the individuals that have the proportionately largest diaphyses being from the large morph, the individual variation within fibulae from the Placerias Quarry population does not show strong evidence of dimorphism.
Reassessment of MNA.V.8464
Currently the best preserved articulated skull of Placerias comes from a site outside of the Placerias Quarry, in another Chinle locality near Cameron, AZ [ 31 ]. The specimen consists of a fully articulated right half of a skull, including the maxilla. However, the specimen is not nearly as well preserved as the Placerias Quarry fossils, having been substantially laterally compressed, and having few discernible suture lines. This has led to difficulty in interpreting it, including in its initial description [ 31 ], which was literally “turned upside down” in a later publication [ 22 ], with the ventral and dorsal sides reversed. Kammerer et al., [ 22 ] argue that the fossil “confirms the accuracy of the Camp and Welles/Cox reconstruction”, but no further statements were made. Since that publication, the skull has undergone additional preparatory work, from ~2019 to 2021, making its morphology clearer. Though the premaxilla is not intact, the maxilla and its suture with the jugal are clear enough to be compared to the Placerias Quarry material.
The proportions of the maxilla from MNA.V.8464 fit well within the bounds of those of the small morphs from the Placerias Quarry population, with the jcp DV and jpm AP lengths being approximately equal. This, combined with the fact that other elements of the skull, such as the quadrate, are closer in size to the smaller end of individuals from Placerias Quarry, provides evidence that MNA.V.8464 likely represents a small morph individual of Placerias . This gives us a useful reference for the relative proportions of skull elements in the small morph, and can potentially be used for comparative purposes with the composite, as that reconstruction consistently uses some of the largest individual elements of the Placerias Quarry population, which more likely come from large morph individuals ( Fig 15 ). This supports the interpretation that other than the significant elongation of the caniniform, the relative proportions of skull bones in Placerias remain similar between morphs, but that the two morphs may have differing distributions in overall size. Though there are some other proportional differences between MNA.V.8464 and the Cox [ 14 ] reconstruction (UCMP 137369), such as the anteroposteriorly shortened squamosal region and smaller pterygoid region in MNA.V.8464, these differences are not dramatic enough to be sure that they are not a product of deformation in MNA.V.8464, or inaccuracies in the reconstruction of UCMP 137369, or both.
(A) Photo of right lateral view; (B), Interpretive drawing with maxilla highlighted in gray; (C) Reconstruction with anterior portion of premaxilla added in dotted line. Abbreviations : mx , maxilla. Scale bar equals 5 cm. Image courtesy of Museum of Northern Arizona.
https://doi.org/10.1371/journal.pone.0297894.g015
In addition to sexual dimorphism, bimodality in size or proportion of bones being observed in a fossil assemblage can also result from changes through ontogeny, or anagenetic evolutionary change if the assemblage is time averaged. With this in mind, it is important to establish that the Placerias Quarry assemblage represents a population of Placerias that lived in the same place at the same time, and the individuals were approximately the same age, when trying to establish that observed conspecific differences constitute sexual dimorphism.
The Placerias Quarry is unusual compared to many sites from the Chinle Formation, both in the fauna represented and in the taphonomy of material preserved there. The most obvious distinction is the atypical abundance of Placerias itself, as well as a much smaller presence of amphibious and aquatic macrovertebrates relative to other Chinle Formation localities. The current understanding is that the Placerias Quarry was a seasonal floodplain, that the assemblage resulted from relatively rapid burial, and that the mechanism of death in Placerias was likely driven by seasonal drought and not extensive predation [ 34 ]. The lower level of Placerias Quarry, where the Placerias fossils are found, has been dated at 219.39 +/-0.12 Ma, based on U-Pb detrital zircon geochronology [ 42 ], placing it within the middle of the Norian. Low taphonomic grade across the specimens indicates they were exposed for short periods of time before burial, and very similar taphonomic grade across specimens is consistent with modern bone assemblages known to have formed on the order of decades [ 34 ]. Therefore the assemblage was likely composed of coeval animals, and though it is unknown if the Placerias lived in a group or herd, the preservation time of the assemblage was almost certainly insufficient for anagenesis to have occurred in the population; there was little to no time averaging.
Individual postcranial remains of Placerias from the site do vary in size ( Fig 16 ), however the entire population appears to be composed entirely of either sub-adults or adults. This observation is supported by previous histological work performed on sampled limbs from the population [ 43 ], which concluded that there were no juveniles in the assemblage. Green et al. [ 43 ] further noted that even though there are bones of multiple sizes in their sample, the smallest having a midshaft diameter 57% the size of that of the largest individual, all bones sampled have extensive secondary remodeling, indicating maturity. Histological evidence shows that Placerias had a similar growth pattern to other Triassic dicynodonts, such as Lystrosaurus [ 43 ] and the placeriine Moghreberia [ 44 ], where periodic rapid osteogenesis was followed by extensive secondary remodeling and reduced growth of parallel-fibered bone later in ontogeny. In Placerias , some specimens that lack peripheral parallel-fibered bone are larger than others that have it, indicating later ontogeny in limb elements which substantially differ in size. This in combination with an inconsistent observed relationship between amount of lines of arrested growth, amount of remodeling, and limb midshaft diameter, suggests that Placerias varied in size to some degree as adults.
(A) UCMP 24884 and (B) UCMP 24875 show a high degree of variance in individual sizes of elements from the Placerias Quarry population, despite histology providing evidence against young juveniles being in the population. Abbreviations : tc , tibial condyle; fc , femoral condyle. Scale bar equals 5 cm.
https://doi.org/10.1371/journal.pone.0297894.g016
The size variation in adult Placerias is proposed by Green et al. [ 43 ] as being a possible result of either sexual dimorphism, developmental plasticity, or of multiple taxa being present in the sample. The possibility of multiple, very similar taxa being present at Placerias Quarry is difficult to disprove, however based on the lack of definable apomorphies that would otherwise separate Placerias into multiple taxa, the roughly 1:1 ratio of large morph to small morph individuals, the extremely sparse record of other North American dicynodont taxa, and the limited time involved in the formation of the fossil assemblage, the discrepancy in body sizes is much more easily explained by individual variation in a single taxon. Developmental plasticity could be at play, particularly due to the assumed stressor of drought conditions on the population based on the taphonomy of the site. That is, perhaps there was some time averaging of populations of subtly different ages, where the body size and ontogeny of some individuals was affected by drought, or some other factor. However, even if present, this does not rule out the likelihood that some portion of the observed differences was due to sexual size dimorphism (SSD).
Though the distribution of sizes in elements other than the maxillae are not strictly bimodal, elements with high sample sizes like the quadrate appear to have two overlapping normal distributions, which could possibly be separated into morphs when associated with a secondary sexual trait, as reflected in the correlation between jpm AP and jcp DV lengths in maxillae. Based on the statistical support for sexual dimorphism presented here, the most likely explanation for the range of adult sizes in Placerias is SSD, consistent with some other synapsid megafauna. Considering the variation seen in the other measured elements, it is possible that cranial and postcranial element proportion varies with sex, but not to the extent that the morphs can be separated based on non-maxillary material alone. Though measuring other elements could show additional variation within the Placerias Quarry population, all of the other elements present (e.g. humeri, femora, etc.) are largely represented by incomplete fragments, and none have large enough complete sample sizes for stronger statistical analysis than those measured here.
The caniniform as a secondary sexual trait
Based on the development of tusks, horns, and other sexually dimorphic weaponry and ornaments, along with differences in body size and robustness of cranial features in extant synapsids (i.e., mammals) [ 8 ], it seems likely that the small morph maxillae represent female individuals and the large morph males. This would also be consistent with the higher prevalence of intrasexual competition among males of mammals, and males being more frequently the sex that invests less in offspring than females. However, this cannot be determined for certain, and in any case not relevant to the claim that dimorphism is present in the population.
Though the most obvious point of difference between the large and small morph caniniform is their dorsoventral length, the variation in mediolateral and proximal anteroposterior lengths of the maxillae indicates there are other differences in the shape of the caniniform. There is strong statistical support for bimodality in mediolateral length of maxillae, and while there is overlap in the two distributions, as might be expected [ 4 ], which confounds unequivocal assignment of all specimens to one of the two distributions, there is complete agreement in the individuals grouped by caniniform length and the mediolateral length. Proximal anteroposterior length is less consistent with morph or dorsoventral length, but is still correlated. The maxilla connects to the rest of the skull along this length, making it a reasonable proxy for overall skull size in individuals. The unimodal distribution for this length indicates that although there is variation in absolute skull size among individuals, the distribution of this variation is different from that of the sexually dimorphic caniniform.
Sexual dimorphism in the caniniform has not been quantitatively reported in other dicynodonts. Other taxa speculated to have sexual dimorphic cranial features, such as Aulocephalodon and Pelanomodon , are placed in morphs based on the thickness of nasal, premaxillary, and prefrontal bosses, and rugose bone around these areas [ 12 , 17 ]. Placerias , in contrast, has relatively thin, smooth nasals and prefrontals. These elements also show very little variation in the Placerias Quarry population, though their relatively small sample sizes could be partially responsible for this. These conditions, and the relative lack of bimodality seen in the premaxillae, imply the lack of a highly developed or dimorphic rostral boss in this species. Given that none of the elements that we measured other than the maxilla have strong statistical support for a bimodal distribution (dimorphism), it appears that the caniniform does indeed represent a secondary sexual feature; a trait that was selected for in a single sex for a function related to sexual competition.
In Placerias , though the tooth is still present in most individuals, it appears to have been functionally replaced by the caniniform process. In some other Stahleckeriids, the tooth is entirely missing (at least in all known individuals) [ 16 ], also likely replaced in function by the caniniform. The teeth of Placerias are underdeveloped, measuring only about 1.5 centimeters in diameter on average in a skull over half a meter in length, and are hidden underneath the caniniforms, if present at all. The tooth, when present, is near the posterior and ventral edges of the maxilla, and depending on the individual may or may not extend past its medial edge, meaning it typically did not occlude with the dentary during jaw closure. The differential growth patterns and variable presence of the tooth observed in the Placerias Quarry sample suggests the dismantling of the developmental pathway for the tooth in this population in the absence of selection for function. The caniniform in Placerias represents a particularly unusual example of functional replacement, as it not only appears to have taken over the feeding-related functions of the tooth, but also the display functions, given that it appears to have been sexually dimorphic analogously to some other tusked dicynodonts [ 9 ] and modern mammals [ 45 ].
Speculations on behavior
Rowe [ 46 ] postulated that Placerias may have used their caniniforms in intraspecific combat, likening them to the sexually dimorphic horns and antlers seen in many modern ungulates. However, this interpretation was based on the version of the composite skull published in Camp and Welles [ 29 ], and did not take into account the more ventral orientation of the caniniforms suggested by Cox [ 14 ]. It also does not take into account the individual variation within large morphs, essentially only using the largest known example of the caniniform in the population to draw conclusions for the entire species. Here, our analysis suggests that the caniniform in the large morphs of Placerias may indeed constitute a form of sexually dimorphic weaponry, defined by Emlen [ 47 ] as a sexually selected structure that is used in physical interaction for intraspecific competition, and/or constitute sexually dimorphic ornamentation, defined by McCullough et al. [ 48 ] as being used for sexual display, without physical interaction between competing individuals. The rugose caniniforms would likely have been covered in keratin in life, and thus do not show any patterns of wear that help distinguish between these two possibilities.
Additional information can be found via comparison with living sexually dimorphic megafauna with similar features and their inferred ecological roles. Many living mammal megafauna, such as hippopotami, elephants, and walruses, have dimorphic tusks and other facial features that can be classified as weaponry [ 49 , 50 ], which are similar to the caniniforms of Placerias . Some of these taxa (e.g., elephants) also represent species with both SSD and proportional differences in features used as weaponry, while others only differ substantially in proportional size of dimorphic weaponry and not in body size (e.g. male hippos have proportionately larger mandibles than females, and use their lower canines for intraspecific combat despite having similar body sizes) [ 50 ]. Within the large Placerias morph, the greater range of size in caniniforms may represent a combination of difference in ages of individuals with heterochronic indeterminate caniniform growth and individual variation based on sexual selection. This is also analogous to weaponry in modern mammal megafauna, which show the same general kind of relationship between size of the weaponry and absolute body size as is indicated by the relationship between caniniform dorsoventral length and other maxillary proportions in Placerias . Thus, even though none of the bones from the Placerias Quarry population show evidence of pre-mortem injury that could have been the result from interaction with other caniniforms [ 34 ], which is weakly suggestive of a role as ornaments rather than weapons, it seems likely that they were used as weapons irrespective of the role as ornaments.
Kinematic work on the jaw of Placerias has posited that it, along with other Late Triassic dicynodonts, was specialized for vertical head movement, based on the distances between occipital muscle attachment points, which correlate to proportions of neck muscles in life [ 51 ]. This inferred vertical plane of movement correlates well with multiple speculated behaviors in Placerias , such as rooting using the caniniforms, but also, for large morphs, aiming their ventrally projecting caniniforms anteriorly towards other individuals while holding up their heads, in a similar manner to walruses [ 49 ]. Some of the largest Placerias maxillae diverge laterally, a phenomenon also observed in male walruses. While there has not been much work exploring the advantage of tusk divergence in male walruses, it may be related to the greater use of tusks as social organs in males than in females, both to enhance visual threat displays by increasing apparent tusk size [ 49 ], and potentially by increasing physical range of the tips of their tusks in combat with other males. These functions may also apply to the caniniforms in Placerias , both for threat displays and physical confrontations, using the cone shaped tips of the caniniforms to stab at opponents. The majority of analogous living synapsid megafaunal species that have cranial secondary sexual structures use them as weaponry. This, in combination with the similarity in robustness and shape in many of these structures to the caniniform in large morph Placerias , suggests that it used its caniniforms as a form of dimorphic weaponry, perhaps in addition to visual display. Assuming that sexually dimorphic tusks in other dicynodonts served a similar combat related purpose, this function may have also been taken over by the caniniform. This would make the caniniform process one of the oldest examples of sexually dimorphic weaponry in a megafaunal taxon currently known, and represent a unique form of social organ for intraspecific competition within dicynodonts.
Supporting information
S1 table. measurements of maxillae, premaxillae, quadrates, and fibulae..
https://doi.org/10.1371/journal.pone.0297894.s001
S2 Table. Parameters of measurement distributions used for likelihood calculations.
https://doi.org/10.1371/journal.pone.0297894.s002
S1 File. R script for comparing log-likelihood of unimodal and bimodal models.
https://doi.org/10.1371/journal.pone.0297894.s003
S2 File. R script for generating PCAs and k-means clusters.
https://doi.org/10.1371/journal.pone.0297894.s004
Acknowledgments
We thank Pat Holroyd (University of California Museum of Paleontology), Matt Smith, Bill Parker and Adam Marsh (all affiliated with Petrified Forest National Park) and Janet Gillette (Museum of Northern Arizona) for access to their museum collections, and for providing historical information on their institutions’ relationships with the Placerias Quarry. We thank Paulo I. Prado for the R function that describes the mixture distribution. We also thank Christian Kammerer, Adam Huttenlocker, and Kenneth Angielcyzk for information on Late Triassic dicynodonts, and members of the Marshall Lab and Leah Kahn for feedback on the manuscript.
- View Article
- Google Scholar
- PubMed/NCBI
- 35. King GM (1990) The dicynodonts: A study in paleobiology. London: Chapman and Hall. 233 p.
- 37. Green JL (2009) Enamel-Reduction and Orthodentine in Dicynodontia (Therapsida) and Xenarthra (Mammalia): An Evaluation of the Potential Ecological Signal Revealed by Dental Microwear. Ph.D. dissertation, North Carolina State University, Raleigh.

COMMENTS
Developing a hypothesis (with example) Step 1. Ask a question. Writing a hypothesis begins with a research question that you want to answer. The question should be focused, specific, and researchable within the constraints of your project. Example: Research question.
The following are examples of qualita tive research questions drawn from several types of strategies. 131 Example 7.1 A Qualitative Central Question From an Ethnography ... a script for a quantitative null hypothesis might be as follows: There is no significant difference between _____ (the control and experimental groups on the independent ...
The primary research question should originate from the hypothesis, not the data, and be established before starting the study. Formulating the research question and hypothesis from existing data (e.g., a database) can lead to multiple statistical comparisons and potentially spurious findings due to chance.
Quantitative Research Hypothesis Examples. 03 Apr June 23, 2021 By global. In general, a researcher arranges hypotheses based on the formulation of problems and theoretical studies. For quantitative research, the hypothesis used is a statistical hypothesis, meaning that the hypothesis must be tested using statistical rules. Whereas for ...
Step 5: Phrase your hypothesis in three ways. To identify the variables, you can write a simple prediction in if … then form. The first part of the sentence states the independent variable and the second part states the dependent variable. If a first-year student starts attending more lectures, then their exam scores will improve.
In quantitative research, hypotheses predict the expected relationships among variables.15 Relationships among variables that can be predicted include 1) between a single dependent variable and a single independent variable (simple hypothesis) or 2) between two or more independent and dependent variables (complex hypothesis).4,11 Hypotheses may ...
Hypotheses are the testable statements linked to your research question. Hypotheses bridge the gap from the general question you intend to investigate (i.e., the research question) to concise statements of what you hypothesize the connection between your variables to be. For example, if we were studying the influence of mentoring relationships ...
Here are some good research hypothesis examples: "The use of a specific type of therapy will lead to a reduction in symptoms of depression in individuals with a history of major depressive disorder.". "Providing educational interventions on healthy eating habits will result in weight loss in overweight individuals.".
3. Simple hypothesis. A simple hypothesis is a statement made to reflect the relation between exactly two variables. One independent and one dependent. Consider the example, "Smoking is a prominent cause of lung cancer." The dependent variable, lung cancer, is dependent on the independent variable, smoking. 4.
A hypothesis is a tentative statement about the relationship between two or more variables. It is a specific, testable prediction about what you expect to happen in a study. It is a preliminary answer to your question that helps guide the research process. Consider a study designed to examine the relationship between sleep deprivation and test ...
"A hypothesis is a conjectural statement of the relation between two or more variables". (Kerlinger, 1956) "Hypothesis is a formal statement that presents the expected relationship between an independent and dependent variable."(Creswell, 1994) "A research question is essentially a hypothesis asked in the form of a question."
5.2 - Writing Hypotheses. The first step in conducting a hypothesis test is to write the hypothesis statements that are going to be tested. For each test you will have a null hypothesis (\ (H_0\)) and an alternative hypothesis (\ (H_a\)). Null Hypothesis. The statement that there is not a difference in the population (s), denoted as \ (H_0\)
Hypothesis testing (sometimes called significance testing) in quantitative research is conducted on either the "null" or the "alternative" hypothesis. 8.1 The Null Hypothesis. The null hypothesis is a statement that assumes the problem being investigated is absent or has no effect (i.e., the difference between means equals zero).
P-Values. The p-value of a hypothesis test is the probability that your sample data would have occurred if you hypothesis were not correct. Traditionally, researchers have used a p-value of 0.05 (a 5% probability that your sample data would have occurred if your hypothesis was wrong) as the threshold for declaring that a hypothesis is true.
Quantitative research is used to validate or test a hypothesis through the collection and analysis of data. (Image by Freepik) If you're wondering what is quantitative research and whether this methodology works for your research study, you're not alone. If you want a simple quantitative research definition, then it's enough to say that this is a method undertaken by researchers based on ...
A systematic approach to hypothesis testing follows the following steps and in this order. This template will work for all hypotheses that you will ever test. Set up the null and alternative hypothesis. This is typically the hardest part of the process. Here the question being asked is reviewed.
Quantitative Research Examples. Some examples of quantitative research are: A customer satisfaction template can be used if any organization would like to conduct a customer satisfaction (CSAT) survey. Through this kind of survey, an organization can collect quantitative data and metrics on the goodwill of the brand or organization in the ...
Sexual dimorphism in dicynodonts. Sexual dimorphism is notoriously difficult to establish in the fossil record [but see 1, 2], especially in vertebrates, unless there are massive sample sizes [].The detection of sexual size dimorphism (SSD) has been particularly challenging because the upper and lower extremes of each sex's size distribution often overlap, making it hard to distinguish the ...
The sample is the specific group of individuals that you will collect data from. ... It is mainly used in quantitative research. If you want to produce results that are representative of the whole population, probability sampling techniques are the most valid choice. ... In these types of research, the aim is not to test a hypothesis about a ...