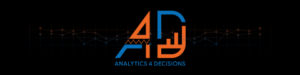

11 Tips For Writing a Dissertation Data Analysis
Since the evolution of the fourth industrial revolution – the Digital World; lots of data have surrounded us. There are terabytes of data around us or in data centers that need to be processed and used. The data needs to be appropriately analyzed to process it, and Dissertation data analysis forms its basis. If data analysis is valid and free from errors, the research outcomes will be reliable and lead to a successful dissertation.
Considering the complexity of many data analysis projects, it becomes challenging to get precise results if analysts are not familiar with data analysis tools and tests properly. The analysis is a time-taking process that starts with collecting valid and relevant data and ends with the demonstration of error-free results.
So, in today’s topic, we will cover the need to analyze data, dissertation data analysis, and mainly the tips for writing an outstanding data analysis dissertation. If you are a doctoral student and plan to perform dissertation data analysis on your data, make sure that you give this article a thorough read for the best tips!
What is Data Analysis in Dissertation?
Dissertation Data Analysis is the process of understanding, gathering, compiling, and processing a large amount of data. Then identifying common patterns in responses and critically examining facts and figures to find the rationale behind those outcomes.
Even f you have the data collected and compiled in the form of facts and figures, it is not enough for proving your research outcomes. There is still a need to apply dissertation data analysis on your data; to use it in the dissertation. It provides scientific support to the thesis and conclusion of the research.
Data Analysis Tools
There are plenty of indicative tests used to analyze data and infer relevant results for the discussion part. Following are some tests used to perform analysis of data leading to a scientific conclusion:
11 Most Useful Tips for Dissertation Data Analysis
Doctoral students need to perform dissertation data analysis and then dissertation to receive their degree. Many Ph.D. students find it hard to do dissertation data analysis because they are not trained in it.
1. Dissertation Data Analysis Services
The first tip applies to those students who can afford to look for help with their dissertation data analysis work. It’s a viable option, and it can help with time management and with building the other elements of the dissertation with much detail.
Dissertation Analysis services are professional services that help doctoral students with all the basics of their dissertation work, from planning, research and clarification, methodology, dissertation data analysis and review, literature review, and final powerpoint presentation.
One great reference for dissertation data analysis professional services is Statistics Solutions , they’ve been around for over 22 years helping students succeed in their dissertation work. You can find the link to their website here .
For a proper dissertation data analysis, the student should have a clear understanding and statistical knowledge. Through this knowledge and experience, a student can perform dissertation analysis on their own.
Following are some helpful tips for writing a splendid dissertation data analysis:
2. Relevance of Collected Data
If the data is irrelevant and not appropriate, you might get distracted from the point of focus. To show the reader that you can critically solve the problem, make sure that you write a theoretical proposition regarding the selection and analysis of data.
3. Data Analysis
For analysis, it is crucial to use such methods that fit best with the types of data collected and the research objectives. Elaborate on these methods and the ones that justify your data collection methods thoroughly. Make sure to make the reader believe that you did not choose your method randomly. Instead, you arrived at it after critical analysis and prolonged research.
On the other hand, quantitative analysis refers to the analysis and interpretation of facts and figures – to build reasoning behind the advent of primary findings. An assessment of the main results and the literature review plays a pivotal role in qualitative and quantitative analysis.
The overall objective of data analysis is to detect patterns and inclinations in data and then present the outcomes implicitly. It helps in providing a solid foundation for critical conclusions and assisting the researcher to complete the dissertation proposal.
4. Qualitative Data Analysis
Qualitative data refers to data that does not involve numbers. You are required to carry out an analysis of the data collected through experiments, focus groups, and interviews. This can be a time-taking process because it requires iterative examination and sometimes demanding the application of hermeneutics. Note that using qualitative technique doesn’t only mean generating good outcomes but to unveil more profound knowledge that can be transferrable.
Presenting qualitative data analysis in a dissertation can also be a challenging task. It contains longer and more detailed responses. Placing such comprehensive data coherently in one chapter of the dissertation can be difficult due to two reasons. Firstly, we cannot figure out clearly which data to include and which one to exclude. Secondly, unlike quantitative data, it becomes problematic to present data in figures and tables. Making information condensed into a visual representation is not possible. As a writer, it is of essence to address both of these challenges.
Qualitative Data Analysis Methods
Following are the methods used to perform quantitative data analysis.
- Deductive Method
This method involves analyzing qualitative data based on an argument that a researcher already defines. It’s a comparatively easy approach to analyze data. It is suitable for the researcher with a fair idea about the responses they are likely to receive from the questionnaires.
- Inductive Method
In this method, the researcher analyzes the data not based on any predefined rules. It is a time-taking process used by students who have very little knowledge of the research phenomenon.
5. Quantitative Data Analysis
Quantitative data contains facts and figures obtained from scientific research and requires extensive statistical analysis. After collection and analysis, you will be able to conclude. Generic outcomes can be accepted beyond the sample by assuming that it is representative – one of the preliminary checkpoints to carry out in your analysis to a larger group. This method is also referred to as the “scientific method”, gaining its roots from natural sciences.
The Presentation of quantitative data depends on the domain to which it is being presented. It is beneficial to consider your audience while writing your findings. Quantitative data for hard sciences might require numeric inputs and statistics. As for natural sciences , such comprehensive analysis is not required.
Quantitative Analysis Methods
Following are some of the methods used to perform quantitative data analysis.
- Trend analysis: This corresponds to a statistical analysis approach to look at the trend of quantitative data collected over a considerable period.
- Cross-tabulation: This method uses a tabula way to draw readings among data sets in research.
- Conjoint analysis : Quantitative data analysis method that can collect and analyze advanced measures. These measures provide a thorough vision about purchasing decisions and the most importantly, marked parameters.
- TURF analysis: This approach assesses the total market reach of a service or product or a mix of both.
- Gap analysis: It utilizes the side-by-side matrix to portray quantitative data, which captures the difference between the actual and expected performance.
- Text analysis: In this method, innovative tools enumerate open-ended data into easily understandable data.
6. Data Presentation Tools
Since large volumes of data need to be represented, it becomes a difficult task to present such an amount of data in coherent ways. To resolve this issue, consider all the available choices you have, such as tables, charts, diagrams, and graphs.
Tables help in presenting both qualitative and quantitative data concisely. While presenting data, always keep your reader in mind. Anything clear to you may not be apparent to your reader. So, constantly rethink whether your data presentation method is understandable to someone less conversant with your research and findings. If the answer is “No”, you may need to rethink your Presentation.
7. Include Appendix or Addendum
After presenting a large amount of data, your dissertation analysis part might get messy and look disorganized. Also, you would not be cutting down or excluding the data you spent days and months collecting. To avoid this, you should include an appendix part.
The data you find hard to arrange within the text, include that in the appendix part of a dissertation . And place questionnaires, copies of focus groups and interviews, and data sheets in the appendix. On the other hand, one must put the statistical analysis and sayings quoted by interviewees within the dissertation.
8. Thoroughness of Data
It is a common misconception that the data presented is self-explanatory. Most of the students provide the data and quotes and think that it is enough and explaining everything. It is not sufficient. Rather than just quoting everything, you should analyze and identify which data you will use to approve or disapprove your standpoints.
Thoroughly demonstrate the ideas and critically analyze each perspective taking care of the points where errors can occur. Always make sure to discuss the anomalies and strengths of your data to add credibility to your research.
9. Discussing Data
Discussion of data involves elaborating the dimensions to classify patterns, themes, and trends in presented data. In addition, to balancing, also take theoretical interpretations into account. Discuss the reliability of your data by assessing their effect and significance. Do not hide the anomalies. While using interviews to discuss the data, make sure you use relevant quotes to develop a strong rationale.
It also involves answering what you are trying to do with the data and how you have structured your findings. Once you have presented the results, the reader will be looking for interpretation. Hence, it is essential to deliver the understanding as soon as you have submitted your data.
10. Findings and Results
Findings refer to the facts derived after the analysis of collected data. These outcomes should be stated; clearly, their statements should tightly support your objective and provide logical reasoning and scientific backing to your point. This part comprises of majority part of the dissertation.
In the finding part, you should tell the reader what they are looking for. There should be no suspense for the reader as it would divert their attention. State your findings clearly and concisely so that they can get the idea of what is more to come in your dissertation.
11. Connection with Literature Review
At the ending of your data analysis in the dissertation, make sure to compare your data with other published research. In this way, you can identify the points of differences and agreements. Check the consistency of your findings if they meet your expectations—lookup for bottleneck position. Analyze and discuss the reasons behind it. Identify the key themes, gaps, and the relation of your findings with the literature review. In short, you should link your data with your research question, and the questions should form a basis for literature.
The Role of Data Analytics at The Senior Management Level
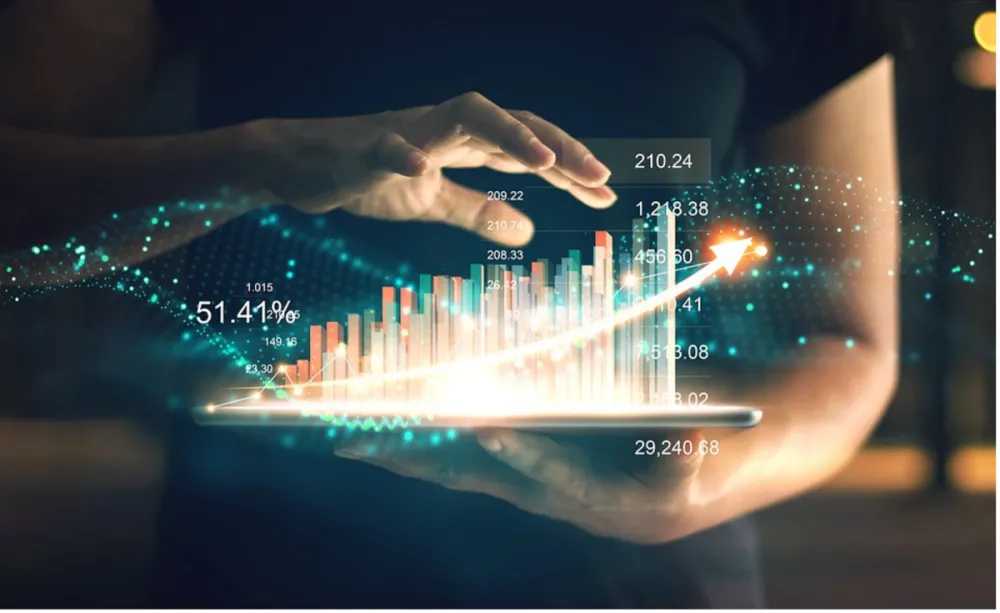
From small and medium-sized businesses to Fortune 500 conglomerates, the success of a modern business is now increasingly tied to how the company implements its data infrastructure and data-based decision-making. According
The Decision-Making Model Explained (In Plain Terms)

Any form of the systematic decision-making process is better enhanced with data. But making sense of big data or even small data analysis when venturing into a decision-making process might
13 Reasons Why Data Is Important in Decision Making
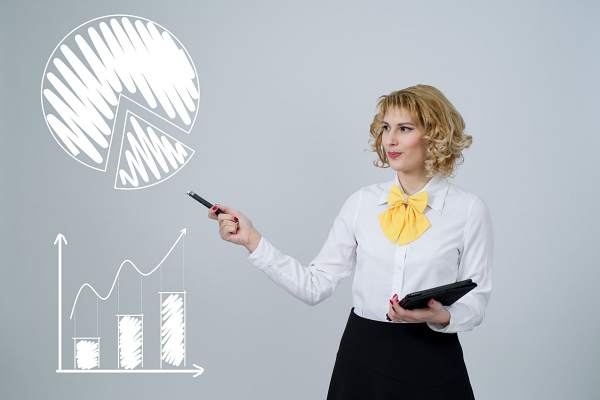
Wrapping Up
Writing data analysis in the dissertation involves dedication, and its implementations demand sound knowledge and proper planning. Choosing your topic, gathering relevant data, analyzing it, presenting your data and findings correctly, discussing the results, connecting with the literature and conclusions are milestones in it. Among these checkpoints, the Data analysis stage is most important and requires a lot of keenness.
In this article, we thoroughly looked at the tips that prove valuable for writing a data analysis in a dissertation. Make sure to give this article a thorough read before you write data analysis in the dissertation leading to the successful future of your research.
Oxbridge Essays. Top 10 Tips for Writing a Dissertation Data Analysis.
Emidio Amadebai
As an IT Engineer, who is passionate about learning and sharing. I have worked and learned quite a bit from Data Engineers, Data Analysts, Business Analysts, and Key Decision Makers almost for the past 5 years. Interested in learning more about Data Science and How to leverage it for better decision-making in my business and hopefully help you do the same in yours.
Recent Posts
Causal vs Evidential Decision-making (How to Make Businesses More Effective)
In today’s fast-paced business landscape, it is crucial to make informed decisions to stay in the competition which makes it important to understand the concept of the different characteristics and...
Bootstrapping vs. Boosting
Over the past decade, the field of machine learning has witnessed remarkable advancements in predictive techniques and ensemble learning methods. Ensemble techniques are very popular in machine...

- Cookies & Privacy
- GETTING STARTED
- Introduction
- FUNDAMENTALS

Getting to the main article
Choosing your route
Setting research questions/ hypotheses
Assessment point
Building the theoretical case
Setting your research strategy
Data collection
Data analysis
Data analysis techniques
In STAGE NINE: Data analysis , we discuss the data you will have collected during STAGE EIGHT: Data collection . However, before you collect your data, having followed the research strategy you set out in this STAGE SIX , it is useful to think about the data analysis techniques you may apply to your data when it is collected.
The statistical tests that are appropriate for your dissertation will depend on (a) the research questions/hypotheses you have set, (b) the research design you are using, and (c) the nature of your data. You should already been clear about your research questions/hypotheses from STAGE THREE: Setting research questions and/or hypotheses , as well as knowing the goal of your research design from STEP TWO: Research design in this STAGE SIX: Setting your research strategy . These two pieces of information - your research questions/hypotheses and research design - will let you know, in principle , the statistical tests that may be appropriate to run on your data in order to answer your research questions.
We highlight the words in principle and may because the most appropriate statistical test to run on your data not only depend on your research questions/hypotheses and research design, but also the nature of your data . As you should have identified in STEP THREE: Research methods , and in the article, Types of variables , in the Fundamentals part of Lærd Dissertation, (a) not all data is the same, and (b) not all variables are measured in the same way (i.e., variables can be dichotomous, ordinal or continuous). In addition, not all data is normal , nor is the data when comparing groups necessarily equal , terms we explain in the Data Analysis section in the Fundamentals part of Lærd Dissertation. As a result, you might think that running a particular statistical test is correct at this point of setting your research strategy (e.g., a statistical test called a dependent t-test ), based on the research questions/hypotheses you have set, but when you collect your data (i.e., during STAGE EIGHT: Data collection ), the data may fail certain assumptions that are important to such a statistical test (i.e., normality and homogeneity of variance ). As a result, you have to run another statistical test (e.g., a Wilcoxon signed-rank test instead of a dependent t-test ).
At this stage in the dissertation process, it is important, or at the very least, useful to think about the data analysis techniques you may apply to your data when it is collected. We suggest that you do this for two reasons:
REASON A Supervisors sometimes expect you to know what statistical analysis you will perform at this stage of the dissertation process
This is not always the case, but if you have had to write a Dissertation Proposal or Ethics Proposal , there is sometimes an expectation that you explain the type of data analysis that you plan to carry out. An understanding of the data analysis that you will carry out on your data can also be an expected component of the Research Strategy chapter of your dissertation write-up (i.e., usually Chapter Three: Research Strategy ). Therefore, it is a good time to think about the data analysis process if you plan to start writing up this chapter at this stage.
REASON B It takes time to get your head around data analysis
When you come to analyse your data in STAGE NINE: Data analysis , you will need to think about (a) selecting the correct statistical tests to perform on your data, (b) running these tests on your data using a statistics package such as SPSS, and (c) learning how to interpret the output from such statistical tests so that you can answer your research questions or hypotheses. Whilst we show you how to do this for a wide range of scenarios in the in the Data Analysis section in the Fundamentals part of Lærd Dissertation, it can be a time consuming process. Unless you took an advanced statistics module/option as part of your degree (i.e., not just an introductory course to statistics, which are often taught in undergraduate and master?s degrees), it can take time to get your head around data analysis. Starting this process at this stage (i.e., STAGE SIX: Research strategy ), rather than waiting until you finish collecting your data (i.e., STAGE EIGHT: Data collection ) is a sensible approach.
Final thoughts...
Setting the research strategy for your dissertation required you to describe, explain and justify the research paradigm, quantitative research design, research method(s), sampling strategy, and approach towards research ethics and data analysis that you plan to follow, as well as determine how you will ensure the research quality of your findings so that you can effectively answer your research questions/hypotheses. However, from a practical perspective, just remember that the main goal of STAGE SIX: Research strategy is to have a clear research strategy that you can implement (i.e., operationalize ). After all, if you are unable to clearly follow your plan and carry out your research in the field, you will struggle to answer your research questions/hypotheses. Once you are sure that you have a clear plan, it is a good idea to take a step back, speak with your supervisor, and assess where you are before moving on to collect data. Therefore, when you are ready, proceed to STAGE SEVEN: Assessment point .
- +44 7897 053596
- [email protected]
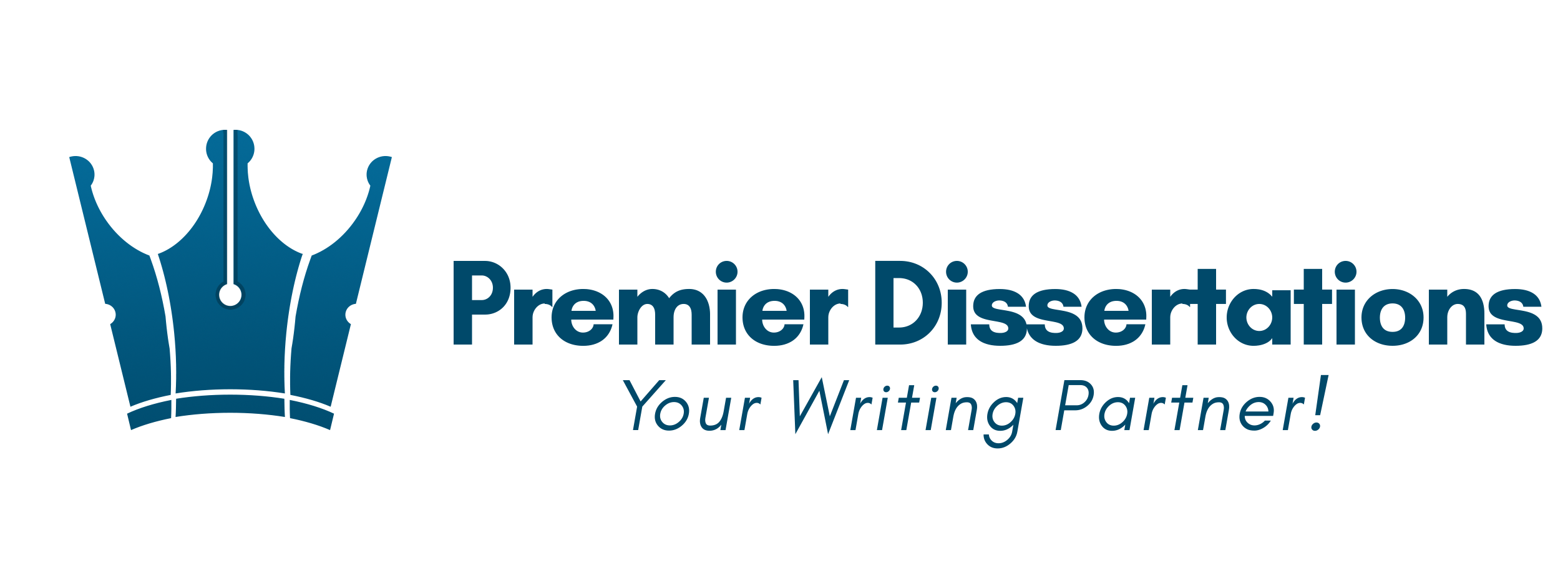
Get an experienced writer start working
Review our examples before placing an order, learn how to draft academic papers, a step-by-step guide to dissertation data analysis.
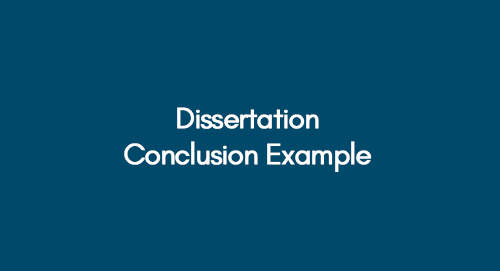
How to Write a Dissertation Conclusion? | Tips & Examples
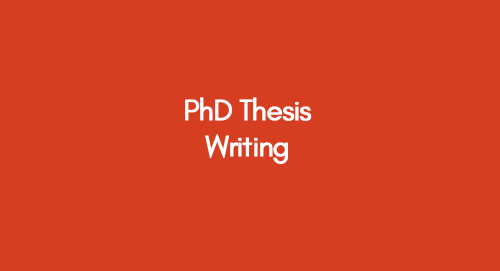
What is PhD Thesis Writing? | Beginner’s Guide
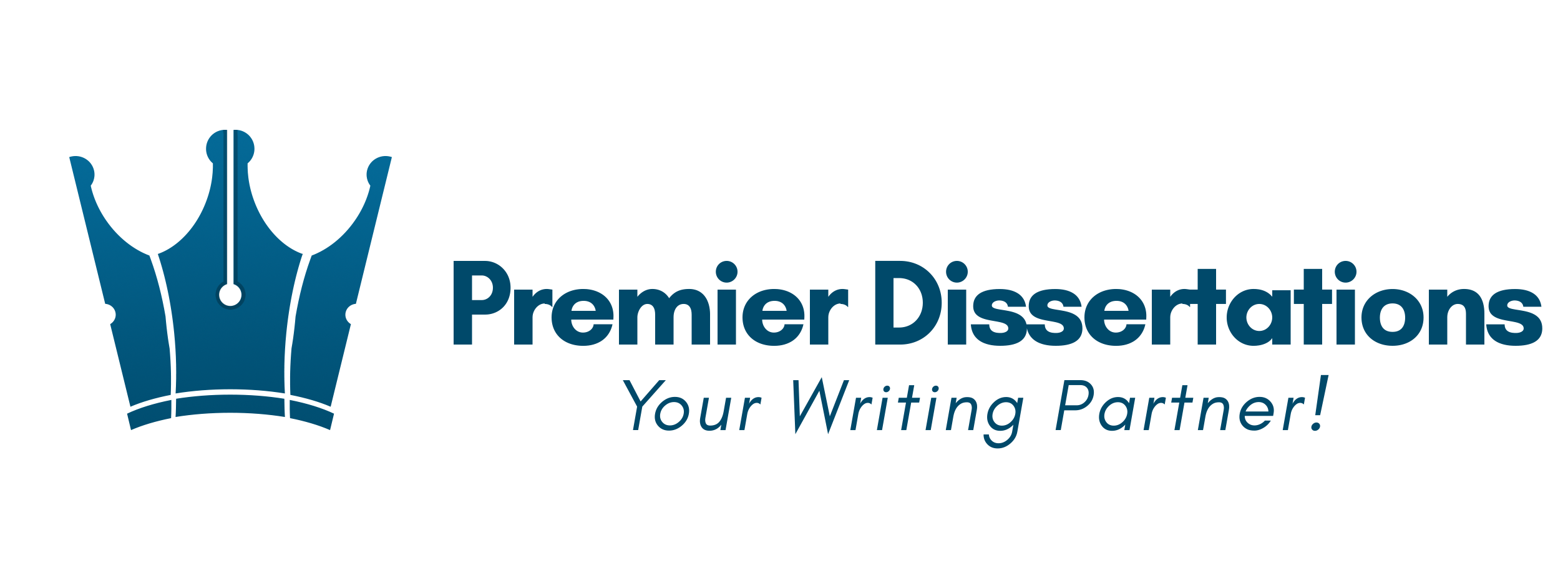
A data analysis dissertation is a complex and challenging project requiring significant time, effort, and expertise. Fortunately, it is possible to successfully complete a data analysis dissertation with careful planning and execution.
As a student, you must know how important it is to have a strong and well-written dissertation, especially regarding data analysis. Proper data analysis is crucial to the success of your research and can often make or break your dissertation.
To get a better understanding, you may review the data analysis dissertation examples listed below;
- Impact of Leadership Style on the Job Satisfaction of Nurses
- Effect of Brand Love on Consumer Buying Behaviour in Dietary Supplement Sector
- An Insight Into Alternative Dispute Resolution
- An Investigation of Cyberbullying and its Impact on Adolescent Mental Health in UK
3-Step Dissertation Process!

Get 3+ Topics

Dissertation Proposal

Get Final Dissertation
Types of data analysis for dissertation.
The various types of data Analysis in a Dissertation are as follows;
1. Qualitative Data Analysis
Qualitative data analysis is a type of data analysis that involves analyzing data that cannot be measured numerically. This data type includes interviews, focus groups, and open-ended surveys. Qualitative data analysis can be used to identify patterns and themes in the data.
2. Quantitative Data Analysis
Quantitative data analysis is a type of data analysis that involves analyzing data that can be measured numerically. This data type includes test scores, income levels, and crime rates. Quantitative data analysis can be used to test hypotheses and to look for relationships between variables.
3. Descriptive Data Analysis
Descriptive data analysis is a type of data analysis that involves describing the characteristics of a dataset. This type of data analysis summarizes the main features of a dataset.
4. Inferential Data Analysis
Inferential data analysis is a type of data analysis that involves making predictions based on a dataset. This type of data analysis can be used to test hypotheses and make predictions about future events.
5. Exploratory Data Analysis
Exploratory data analysis is a type of data analysis that involves exploring a data set to understand it better. This type of data analysis can identify patterns and relationships in the data.
Time Period to Plan and Complete a Data Analysis Dissertation?
When planning dissertation data analysis, it is important to consider the dissertation methodology structure and time series analysis as they will give you an understanding of how long each stage will take. For example, using a qualitative research method, your data analysis will involve coding and categorizing your data.
This can be time-consuming, so allowing enough time in your schedule is important. Once you have coded and categorized your data, you will need to write up your findings. Again, this can take some time, so factor this into your schedule.
Finally, you will need to proofread and edit your dissertation before submitting it. All told, a data analysis dissertation can take anywhere from several weeks to several months to complete, depending on the project’s complexity. Therefore, starting planning early and allowing enough time in your schedule to complete the task is important.
Essential Strategies for Data Analysis Dissertation
A. Planning
The first step in any dissertation is planning. You must decide what you want to write about and how you want to structure your argument. This planning will involve deciding what data you want to analyze and what methods you will use for a data analysis dissertation.
B. Prototyping
Once you have a plan for your dissertation, it’s time to start writing. However, creating a prototype is important before diving head-first into writing your dissertation. A prototype is a rough draft of your argument that allows you to get feedback from your advisor and committee members. This feedback will help you fine-tune your argument before you start writing the final version of your dissertation.
C. Executing
After you have created a plan and prototype for your data analysis dissertation, it’s time to start writing the final version. This process will involve collecting and analyzing data and writing up your results. You will also need to create a conclusion section that ties everything together.
D. Presenting
The final step in acing your data analysis dissertation is presenting it to your committee. This presentation should be well-organized and professionally presented. During the presentation, you’ll also need to be ready to respond to questions concerning your dissertation.
Data Analysis Tools
Numerous suggestive tools are employed to assess the data and deduce pertinent findings for the discussion section. The tools used to analyze data and get a scientific conclusion are as follows:
a. Excel
Excel is a spreadsheet program part of the Microsoft Office productivity software suite. Excel is a powerful tool that can be used for various data analysis tasks, such as creating charts and graphs, performing mathematical calculations, and sorting and filtering data.
b. Google Sheets
Google Sheets is a free online spreadsheet application that is part of the Google Drive suite of productivity software. Google Sheets is similar to Excel in terms of functionality, but it also has some unique features, such as the ability to collaborate with other users in real-time.
c. SPSS
SPSS is a statistical analysis software program commonly used in the social sciences. SPSS can be used for various data analysis tasks, such as hypothesis testing, factor analysis, and regression analysis.
d. STATA
STATA is a statistical analysis software program commonly used in the sciences and economics. STATA can be used for data management, statistical modelling, descriptive statistics analysis, and data visualization tasks.
SAS is a commercial statistical analysis software program used by businesses and organizations worldwide. SAS can be used for predictive modelling, market research, and fraud detection.
R is a free, open-source statistical programming language popular among statisticians and data scientists. R can be used for tasks such as data wrangling, machine learning, and creating complex visualizations.
g. Python
A variety of applications may be used using the distinctive programming language Python, including web development, scientific computing, and artificial intelligence. Python also has a number of modules and libraries that can be used for data analysis tasks, such as numerical computing, statistical modelling, and data visualization.
Testimonials
Very satisfied students
This is our reason for working. We want to make all students happy, every day. Review us on Sitejabber
Tips to Compose a Successful Data Analysis Dissertation
a. Choose a Topic You’re Passionate About
The first step to writing a successful data analysis dissertation is to choose a topic you’re passionate about. Not only will this make the research and writing process more enjoyable, but it will also ensure that you produce a high-quality paper.
Choose a topic that is particular enough to be covered in your paper’s scope but not so specific that it will be challenging to obtain enough evidence to substantiate your arguments.
b. Do Your Research
data analysis in research is an important part of academic writing. Once you’ve selected a topic, it’s time to begin your research. Be sure to consult with your advisor or supervisor frequently during this stage to ensure that you are on the right track. In addition to secondary sources such as books, journal articles, and reports, you should also consider conducting primary research through surveys or interviews. This will give you first-hand insights into your topic that can be invaluable when writing your paper.
c. Develop a Strong Thesis Statement
After you’ve done your research, it’s time to start developing your thesis statement. It is arguably the most crucial part of your entire paper, so take care to craft a clear and concise statement that encapsulates the main argument of your paper.
Remember that your thesis statement should be arguable—that is, it should be capable of being disputed by someone who disagrees with your point of view. If your thesis statement is not arguable, it will be difficult to write a convincing paper.
d. Write a Detailed Outline
Once you have developed a strong thesis statement, the next step is to write a detailed outline of your paper. This will offer you a direction to write in and guarantee that your paper makes sense from beginning to end.
Your outline should include an introduction, in which you state your thesis statement; several body paragraphs, each devoted to a different aspect of your argument; and a conclusion, in which you restate your thesis and summarize the main points of your paper.
e. Write Your First Draft
With your outline in hand, it’s finally time to start writing your first draft. At this stage, don’t worry about perfecting your grammar or making sure every sentence is exactly right—focus on getting all of your ideas down on paper (or onto the screen). Once you have completed your first draft, you can revise it for style and clarity.
And there you have it! Following these simple tips can increase your chances of success when writing your data analysis dissertation. Just remember to start early, give yourself plenty of time to research and revise, and consult with your supervisor frequently throughout the process.
How Does It Work ?
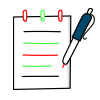
Fill the Form
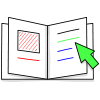
Writer Starts Working
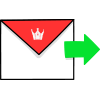
3+ Topics Emailed!
Studying the above examples gives you valuable insight into the structure and content that should be included in your own data analysis dissertation. You can also learn how to effectively analyze and present your data and make a lasting impact on your readers.
In addition to being a useful resource for completing your dissertation, these examples can also serve as a valuable reference for future academic writing projects. By following these examples and understanding their principles, you can improve your data analysis skills and increase your chances of success in your academic career.
You may also contact Premier Dissertations to develop your data analysis dissertation.
For further assistance, some other resources in the dissertation writing section are shared below;
How Do You Select the Right Data Analysis
How to Write Data Analysis For A Dissertation?
How to Develop a Conceptual Framework in Dissertation?
What is a Hypothesis in a Dissertation?
Get an Immediate Response
Discuss your requirments with our writers
WhatsApp Us Email Us Chat with Us
Get 3+ Free Dissertation Topics within 24 hours?
Your Number
Academic Level Select Academic Level Undergraduate Masters PhD
Area of Research
admin farhan
Related posts.
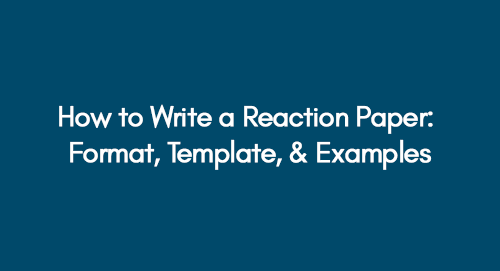
How to Write a Reaction Paper: Format, Template, & Examples
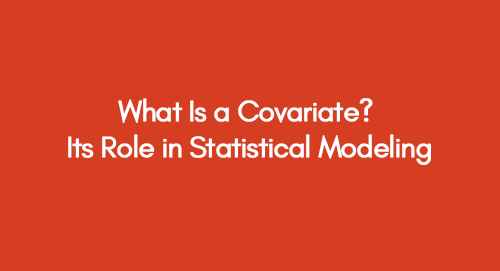
What Is a Covariate? Its Role in Statistical Modeling
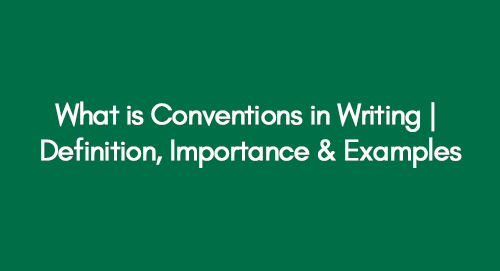
What is Conventions in Writing | Definition, Importance & Examples
Comments are closed.
- Link to facebook
- Link to linkedin
- Link to twitter
- Link to youtube
- Writing Tips
5 Tips for Handling your Thesis Data Analysis
3-minute read
- 23rd June 2015
When writing your thesis, the process of analyzing data and working with statistics can be pretty hard at first. This is true whether you’re using specialized data analysis software, like SPSS, or a more descriptive approach. But there are a few guidelines you can follow to make things simpler.
1. Choose the Best Analytical Method for Your Project
The sheer variety of techniques available for data analysis can be confusing! If you are writing a thesis on internet marketing, for instance, your approach to analysis will be very different to someone writing about biochemistry. As such it is important to adopt an approach appropriate to your research.
2. Double Check Your Methodology
If you are working with quantitative data, it is important to make sure that your analytical techniques are compatible with the methods used to gather your data. Having a clear understanding of what you have done so far will ensure that you achieve accurate results.
For instance, when performing statistical analysis, you may have to choose between parametric and non-parametric testing. If your data is sampled from a population with a broadly Gaussian (i.e., normal) distribution, you will almost always want to use some form of non-parametric testing.
But if you can’t remember or aren’t sure how you selected your sample, you won’t necessarily know the best test to use!
3. Familiarize Yourself with Statistical Analysis and Analytical Software
Thanks to various clever computer programs, you no longer have to be a math genius to conduct top-grade statistical analysis. Nevertheless, learning the basics will help you make informed choices when designing your research and prevent you from making basic mistakes.
Find this useful?
Subscribe to our newsletter and get writing tips from our editors straight to your inbox.
Likewise, trying out different software packages will allow you to pick the one best suited to your needs on your current project.
4. Present Your Data Clearly and Consistently
This is possibly one of the most important parts of writing up your results. Even if your data and statistics are perfect, failure to present your analysis clearly will make it difficult for your reader to follow.
Ask yourself how your analysis would look to someone unfamiliar with your project. If they would be able to understand your analysis, you’re on the right track!
5. Make It Relevant!
Finally, remember that data analysis is about more than just presenting your data. You should also relate your analysis back to your research objectives, discussing its relevance and justifying your interpretations.
This will ensure that your work is easy to follow and demonstrate your understanding of the methods used. So no matter what you are writing about, the analysis is a great time to show off how clever you are!
Share this article:
Post A New Comment
Got content that needs a quick turnaround? Let us polish your work. Explore our editorial business services.
9-minute read
How to Use Infographics to Boost Your Presentation
Is your content getting noticed? Capturing and maintaining an audience’s attention is a challenge when...
8-minute read
Why Interactive PDFs Are Better for Engagement
Are you looking to enhance engagement and captivate your audience through your professional documents? Interactive...
7-minute read
Seven Key Strategies for Voice Search Optimization
Voice search optimization is rapidly shaping the digital landscape, requiring content professionals to adapt their...
4-minute read
Five Creative Ways to Showcase Your Digital Portfolio
Are you a creative freelancer looking to make a lasting impression on potential clients or...
How to Ace Slack Messaging for Contractors and Freelancers
Effective professional communication is an important skill for contractors and freelancers navigating remote work environments....
How to Insert a Text Box in a Google Doc
Google Docs is a powerful collaborative tool, and mastering its features can significantly enhance your...
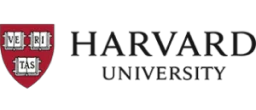
Make sure your writing is the best it can be with our expert English proofreading and editing.
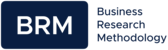
Data Analysis
Methodology chapter of your dissertation should include discussions about the methods of data analysis. You have to explain in a brief manner how you are going to analyze the primary data you will collect employing the methods explained in this chapter.
There are differences between qualitative data analysis and quantitative data analysis . In qualitative researches using interviews, focus groups, experiments etc. data analysis is going to involve identifying common patterns within the responses and critically analyzing them in order to achieve research aims and objectives.
Data analysis for quantitative studies, on the other hand, involves critical analysis and interpretation of figures and numbers, and attempts to find rationale behind the emergence of main findings. Comparisons of primary research findings to the findings of the literature review are critically important for both types of studies – qualitative and quantitative.
Data analysis methods in the absence of primary data collection can involve discussing common patterns, as well as, controversies within secondary data directly related to the research area.
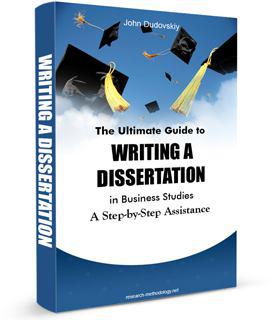
John Dudovskiy

Ace Your Data Analysis
Get hands-on help analysing your data from a friendly Grad Coach. It’s like having a professor in your pocket.

Students Helped
Client pass rate, trustpilot score, facebook rating, how we help you .
Whether you’ve just started collecting your data, are in the thick of analysing it, or you’ve already written a draft chapter – we’re here to help.
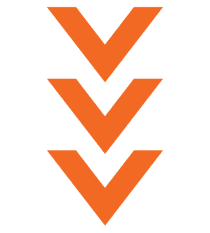
Make sense of the data
If you’ve collected your data, but are feeling confused about what to do and how to make sense of it all, we can help. One of our friendly coaches will hold your hand through each step and help you interpret your dataset .
Alternatively, if you’re still planning your data collection and analysis strategy, we can help you craft a rock-solid methodology that sets you up for success.
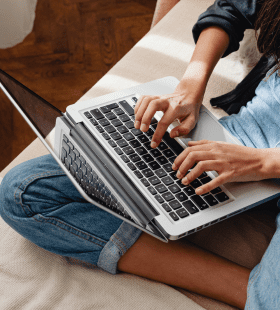
Get your thinking onto paper
If you’ve analysed your data, but are struggling to get your thoughts onto paper, one of our friendly Grad Coaches can help you structure your results and/or discussion chapter to kickstart your writing.
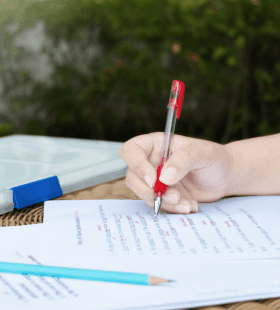
Refine your writing
If you’ve already written up your results but need a second set of eyes, our popular Content Review service can help you identify and address key issues within your writing, before you submit it for grading .
Why Grad Coach ?
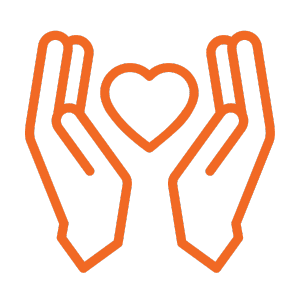
It's all about you
We take the time to understand your unique challenges and work with you to achieve your specific academic goals . Whether you're aiming to earn top marks or just need to cross the finish line, we're here to help.
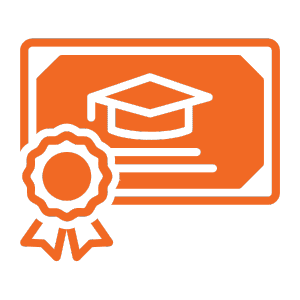
An insider advantage
Our award-winning Dissertation Coaches all hold doctoral-level degrees and share 100+ years of combined academic experience. Having worked on "the inside", we know exactly what markers want .
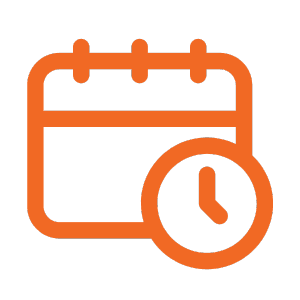
Any time, anywhere
Getting help from your dedicated Dissertation Coach is simple. Book a live video /voice call, chat via email or send your document to us for an in-depth review and critique . We're here when you need us.
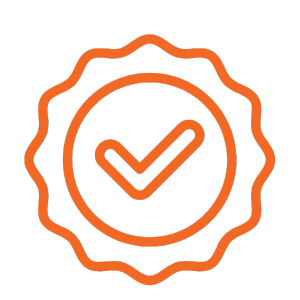
A track record you can trust
Over 10 million students have enjoyed our online lessons and courses, while 3000+ students have benefited from 1:1 Private Coaching. The plethora of glowing reviews reflects our commitment.
Chat With A Friendly Coach, Today
Prefer email? No problem - you c an email us here .

Have a question ?
Below we address some of the most popular questions we receive regarding our data analysis support, but feel free to get in touch if you have any other questions.
Dissertation Coaching
I have no idea where to start. can you help.
Absolutely. We regularly work with students who are completely new to data analysis (both qualitative and quantitative) and need step-by-step guidance to understand and interpret their data.
Can you analyse my data for me?
The short answer – no.
The longer answer:
If you’re undertaking qualitative research , we can fast-track your project with our Qualitative Coding Service. With this service, we take care of the initial coding of your dataset (e.g., interview transcripts), providing a firm foundation on which you can build your qualitative analysis (e.g., thematic analysis, content analysis, etc.).
If you’re undertaking quantitative research , we can fast-track your project with our Statistical Testing Service . With this service, we run the relevant statistical tests using SPSS or R, and provide you with the raw outputs. You can then use these outputs/reports to interpret your results and develop your analysis.
Importantly, in both cases, we are not analysing the data for you or providing an interpretation or write-up for you. If you’d like coaching-based support with that aspect of the project, we can certainly assist you with this (i.e., provide guidance and feedback, review your writing, etc.). But it’s important to understand that you, as the researcher, need to engage with the data and write up your own findings.
Can you help me choose the right data analysis methods?
Yes, we can assist you in selecting appropriate data analysis methods, based on your research aims and research questions, as well as the characteristics of your data.
Which data analysis methods can you assist with?
We can assist with most qualitative and quantitative analysis methods that are commonplace within the social sciences.
Qualitative methods:
- Qualitative content analysis
- Thematic analysis
- Discourse analysis
- Narrative analysis
- Grounded theory
Quantitative methods:
- Descriptive statistics
- Inferential statistics
Can you provide data sets for me to analyse?
If you are undertaking secondary research , we can potentially assist you in finding suitable data sets for your analysis.
If you are undertaking primary research , we can help you plan and develop data collection instruments (e.g., surveys, questionnaires, etc.), but we cannot source the data on your behalf.
Can you write the analysis/results/discussion chapter/section for me?
No. We can provide you with hands-on guidance through each step of the analysis process, but the writing needs to be your own. Writing anything for you would constitute academic misconduct .
Can you help me organise and structure my results/discussion chapter/section?
Yes, we can assist in structuring your chapter to ensure that you have a clear, logical structure and flow that delivers a clear and convincing narrative.
Can you review my writing and give me feedback?
Absolutely. Our Content Review service is designed exactly for this purpose and is one of the most popular services here at Grad Coach. In a Content Review, we carefully read through your research methodology chapter (or any other chapter) and provide detailed comments regarding the key issues/problem areas, why they’re problematic and what you can do to resolve the issues. You can learn more about Content Review here .
Do you provide software support (e.g., SPSS, R, etc.)?
It depends on the software package you’re planning to use, as well as the analysis techniques/tests you plan to undertake. We can typically provide support for the more popular analysis packages, but it’s best to discuss this in an initial consultation.
Can you help me with other aspects of my research project?
Yes. Data analysis support is only one aspect of our offering at Grad Coach, and we typically assist students throughout their entire dissertation/thesis/research project. You can learn more about our full service offering here .

Can I get a coach that specialises in my topic area?
It’s important to clarify that our expertise lies in the research process itself , rather than specific research areas/topics (e.g., psychology, management, etc.).
In other words, the support we provide is topic-agnostic, which allows us to support students across a very broad range of research topics. That said, if there is a coach on our team who has experience in your area of research, as well as your chosen methodology, we can allocate them to your project (dependent on their availability, of course).
If you’re unsure about whether we’re the right fit, feel free to drop us an email or book a free initial consultation.
What qualifications do your coaches have?
All of our coaches hold a doctoral-level degree (for example, a PhD, DBA, etc.). Moreover, they all have experience working within academia, in many cases as dissertation/thesis supervisors. In other words, they understand what markers are looking for when reviewing a student’s work.
Is my data/topic/study kept confidential?
Yes, we prioritise confidentiality and data security. Your written work and personal information are treated as strictly confidential. We can also sign a non-disclosure agreement, should you wish.
I still have questions…
No problem. Feel free to email us or book an initial consultation to discuss.
What our clients say
We've worked 1:1 with 3000+ students . Here's what some of them have to say:
David's depth of knowledge in research methodology was truly impressive. He demonstrated a profound understanding of the nuances and complexities of my research area, offering insights that I hadn't even considered. His ability to synthesize information, identify key research gaps, and suggest research topics was truly inspiring. I felt like I had a true expert by my side, guiding me through the complexities of the proposal.
Cyntia Sacani (US)
I had been struggling with the first 3 chapters of my dissertation for over a year. I finally decided to give GradCoach a try and it made a huge difference. Alexandra provided helpful suggestions along with edits that transformed my paper. My advisor was very impressed.
Tracy Shelton (US)
Working with Kerryn has been brilliant. She has guided me through that pesky academic language that makes us all scratch our heads. I can't recommend Grad Coach highly enough; they are very professional, humble, and fun to work with. If like me, you know your subject matter but you're getting lost in the academic language, look no further, give them a go.
Tony Fogarty (UK)
So helpful! Amy assisted me with an outline for my literature review and with organizing the results for my MBA applied research project. Having a road map helped enormously and saved a lot of time. Definitely worth it.
Jennifer Hagedorn (Canada)
Everything about my experience was great, from Dr. Shaeffer’s expertise, to her patience and flexibility. I reached out to GradCoach after receiving a 78 on a midterm paper. Not only did I get a 100 on my final paper in the same class, but I haven’t received a mark less than A+ since. I recommend GradCoach for everyone who needs help with academic research.
Antonia Singleton (Qatar)
I started using Grad Coach for my dissertation and I can honestly say that if it wasn’t for them, I would have really struggled. I would strongly recommend them – worth every penny!
Richard Egenreider (South Africa)
Fast-Track Your Data Analysis, Today
Enter your details below, pop us an email, or book an introductory consultation .
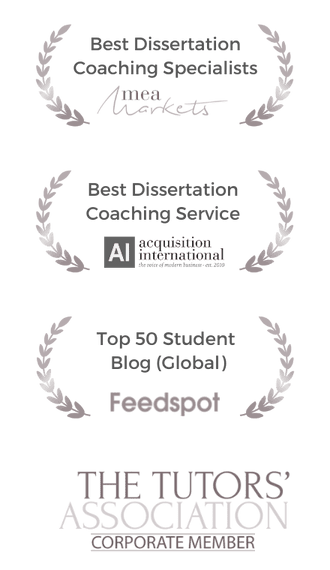
- Deutschland
- United Kingdom
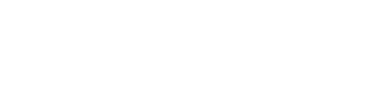
- PhD Dissertations
- Master’s Dissertations
- Bachelor’s Dissertations
- Scientific Dissertations
- Medical Dissertations
- Bioscience Dissertations
- Social Sciences Dissertations
- Psychology Dissertations
- Humanities Dissertations
- Engineering Dissertations
- Economics Dissertations
- Service Overview
- Revisión en inglés
- Relecture en anglais
- Revisão em inglês
Manuscript Editing
- Research Paper Editing
- Lektorat Doktorarbeit
- Dissertation Proofreading
- Englisches Lektorat
- Journal Manuscript Editing
- Scientific Manuscript Editing Services
- Book Manuscript Editing
- PhD Thesis Proofreading Services
- Wissenschaftslektorat
- Korektura anglického textu
- Akademisches Lektorat
- Journal Article Editing
- Manuscript Editing Services
PhD Thesis Editing
- Medical Editing Sciences
- Proofreading Rates UK
- Medical Proofreading
- PhD Proofreading
- Academic Proofreading
- PhD Proofreaders
- Best Dissertation Proofreaders
- Masters Dissertation Proofreading
- Proofreading PhD Thesis Price
- PhD Dissertation Editing
- Lektorat Englisch Preise
- Lektorieren Englisch
- Wissenschaftliches Lektorat
- Thesis Proofreading Services
- PhD Thesis Proofreading
- Proofreading Thesis Cost
- Proofreading Thesis
- Thesis Editing Services
- Professional Thesis Editing
- PhD Thesis Editing Services
- Thesis Editing Cost
- Dissertation Proofreading Services
- Proofreading Dissertation
PhD Dissertation Proofreading
- Dissertation Proofreading Cost
- Dissertation Proofreader
- Correção de Artigos Científicos
- Correção de Trabalhos Academicos
- Serviços de Correção de Inglês
- Correção de Dissertação
- Correção de Textos Precos
- Revision en Ingles
- Revision de Textos en Ingles
- Revision de Tesis
- Revision Medica en Ingles
- Revision de Tesis Precio
- Revisão de Artigos Científicos
- Revisão de Trabalhos Academicos
- Serviços de Revisão de Inglês
- Revisão de Dissertação
- Revisão de Textos Precos
- Corrección de Textos en Ingles
- Corrección de Tesis
- Corrección de Tesis Precio
- Corrección Medica en Ingles
- Corrector ingles
- Choosing the right Journal
- Journal Editor’s Feedback
- Dealing with Rejection
- Quantitative Research Examples
- Number of scientific papers published per year
- Acknowledgements Example
- ISO, ANSI, CFR & Other
- Types of Peer Review
- Withdrawing a Paper
- What is a good h-index
- Appendix paper
- Cover Letter Templates
- Writing an Article
- How To Write the Findings
- Abbreviations: ‘Ibid.’ & ‘Id.’
- Sample letter to editor for publication
- Tables and figures in research paper
- Journal Metrics
- Revision Process of Journal Publishing
- JOURNAL GUIDELINES
Select Page
Writing the Data Analysis Chapter(s): Results and Evidence
Posted by Rene Tetzner | Oct 19, 2021 | PhD Success | 0 |
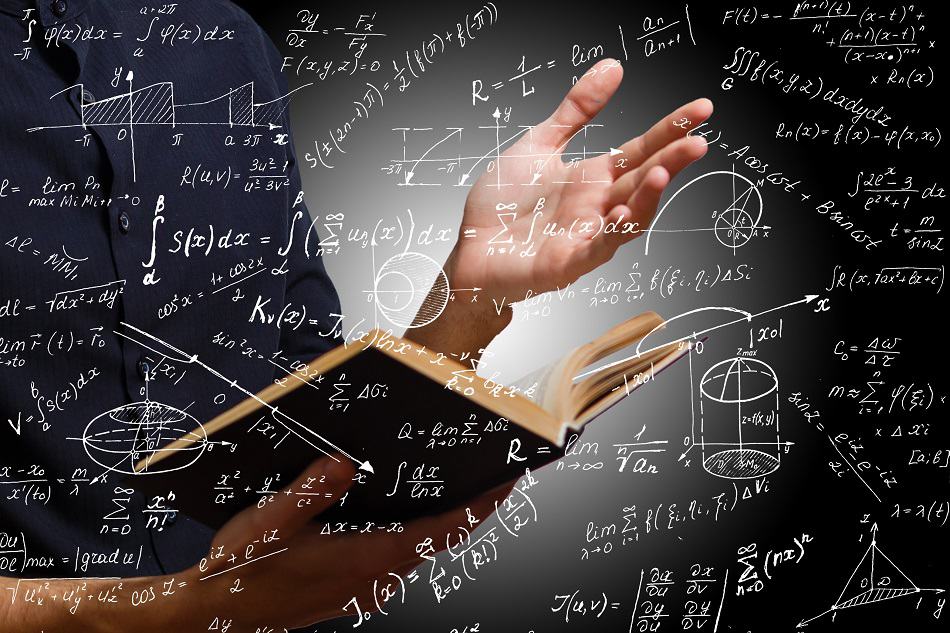
4.4 Writing the Data Analysis Chapter(s): Results and Evidence
Unlike the introduction, literature review and methodology chapter(s), your results chapter(s) will need to be written for the first time as you draft your thesis even if you submitted a proposal, though this part of your thesis will certainly build upon the preceding chapters. You should have carefully recorded and collected the data (test results, participant responses, computer print outs, observations, transcriptions, notes of various kinds etc.) from your research as you conducted it, so now is the time to review, organise and analyse the data. If your study is quantitative in nature, make sure that you know what all the numbers mean and that you consider them in direct relation to the topic, problem or phenomenon you are investigating, and especially in relation to your research questions and hypotheses. You may find that you require the services of a statistician to help make sense of the data, in which case, obtaining that help sooner rather than later is advisable, because you need to understand your results thoroughly before you can write about them. If, on the other hand, your study is qualitative, you will need to read through the data you have collected several times to become familiar with them both as a whole and in detail so that you can establish important themes, patterns and categories. Remember that ‘qualitative analysis is a creative process and requires thoughtful judgments about what is significant and meaningful in the data’ (Roberts, 2010, p.174; see also Miles & Huberman, 1994) – judgements that often need to be made before the findings can be effectively analysed and presented. If you are combining methodologies in your research, you will also need to consider relationships between the results obtained from the different methods, integrating all the data you have obtained and discovering how the results of one approach support or correlate with the results of another. Ideally, you will have taken careful notes recording your initial thoughts and analyses about the sources you consulted and the results and evidence provided by particular methods and instruments as you put them into practice (as suggested in Sections 2.1.2 and 2.1.4), as these will prove helpful while you consider how best to present your results in your thesis.
Although the ways in which to present and organise the results of doctoral research differ markedly depending on the nature of the study and its findings, as on author and committee preferences and university and department guidelines, there are several basic principles that apply to virtually all theses. First and foremost is the need to present the results of your research both clearly and concisely, and in as objective and factual a manner as possible. There will be time and space to elaborate and interpret your results and speculate on their significance and implications in the final discussion chapter(s) of your thesis, but, generally speaking, such reflection on the meaning of the results should be entirely separate from the factual report of your research findings. There are exceptions, of course, and some candidates, supervisors and departments may prefer the factual presentation and interpretive discussion of results to be blended, just as some thesis topics may demand such treatment, but this is rare and best avoided unless there are persuasive reasons to avoid separating the facts from your thoughts about them. If you do find that you need to blend facts and interpretation in reporting your results, make sure that your language leaves no doubt about the line between the two: words such as ‘seems,’ ‘appears,’ ‘may,’ ‘might,’ probably’ and the like will effectively distinguish analytical speculation from more factual reporting (see also Section 4.5).

You need not dedicate much space in this part of the thesis to the methods you used to arrive at your results because these have already been described in your methodology chapter(s), but they can certainly be revisited briefly to clarify or lend structure to your report. Results are most often presented in a straightforward narrative form which is often supplemented by tables and perhaps by figures such as graphs, charts and maps. An effective approach is to decide immediately which information would be best included in tables and figures, and then to prepare those tables and figures before you begin writing the text for the chapter (see Section 4.4.1 on designing effective tables and figures). Arranging your data into the visually immediate formats provided by tables and figures can, for one, produce interesting surprises by enabling you to see trends and details that you may not have noticed previously, and writing the report of your results will prove easier when you have the tables and figures to work with just as your readers ultimately will. In addition, while the text of the results chapter(s) should certainly highlight the most notable data included in tables and figures, it is essential not to repeat information unnecessarily, so writing with the tables and figures already constructed will help you keep repetition to a minimum. Finally, writing about the tables and figures you create will help you test their clarity and effectiveness for your readers, and you can make any necessary adjustments to the tables and figures as you work. Be sure to refer to each table and figure by number in your text and to make it absolutely clear what you want your readers to see or understand in the table or figure (e.g., ‘see Table 1 for the scores’ and ‘Figure 2 shows this relationship’).

Beyond combining textual narration with the data presented in tables and figures, you will need to organise your report of the results in a manner best suited to the material. You may choose to arrange the presentation of your results chronologically or in a hierarchical order that represents their importance; you might subdivide your results into sections (or separate chapters if there is a great deal of information to accommodate) focussing on the findings of different kinds of methodology (quantitative versus qualitative, for instance) or of different tests, trials, surveys, reviews, case studies and so on; or you may want to create sections (or chapters) focussing on specific themes, patterns or categories or on your research questions and/or hypotheses. The last approach allows you to cluster results that relate to a particular question or hypothesis into a single section and can be particularly useful because it provides cohesion for the thesis as a whole and forces you to focus closely on the issues central to the topic, problem or phenomenon you are investigating. You will, for instance, be able to refer back to the questions and hypotheses presented in your introduction (see Section 3.1), to answer the questions and confirm or dismiss the hypotheses and to anticipate in relation to those questions and hypotheses the discussion and interpretation of your findings that will appear in the next part of the thesis (see Section 4.5). Less effective is an approach that organises the presentation of results according to the items of a survey or questionnaire, because these lend the structure of the instrument used to the results instead of connecting those results directly to the aims, themes and argument of your thesis, but such an organisation can certainly be an important early step in your analysis of the findings and might even be valid for the final thesis if, for instance, your work focuses on developing the instrument involved.

The results generated by doctoral research are unique, and this book cannot hope to outline all the possible approaches for presenting the data and analyses that constitute research results, but it is essential that you devote considerable thought and special care to the way in which you structure the report of your results (Section 6.1 on headings may prove helpful). Whatever structure you choose should accurately reflect the nature of your results and highlight their most important and interesting trends, and it should also effectively allow you (in the next part of the thesis) to discuss and speculate upon your findings in ways that will test the premises of your study, work well in the overall argument of your thesis and lead to significant implications for your research. Regardless of how you organise the main body of your results chapter(s), however, you should include a final paragraph (or more than one paragraph if necessary) that briefly summarises and explains the key results and also guides the reader on to the discussion and interpretation of those results in the following chapter(s).
Why PhD Success?
To Graduate Successfully
This article is part of a book called "PhD Success" which focuses on the writing process of a phd thesis, with its aim being to provide sound practices and principles for reporting and formatting in text the methods, results and discussion of even the most innovative and unique research in ways that are clear, correct, professional and persuasive.
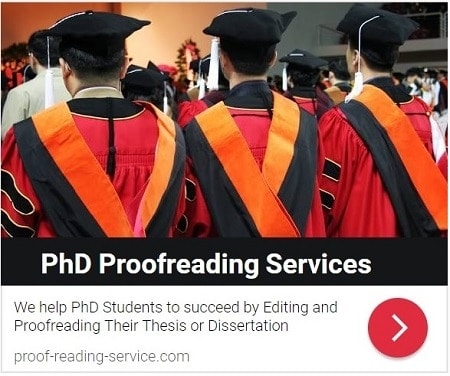
The assumption of the book is that the doctoral candidate reading it is both eager to write and more than capable of doing so, but nonetheless requires information and guidance on exactly what he or she should be writing and how best to approach the task. The basic components of a doctoral thesis are outlined and described, as are the elements of complete and accurate scholarly references, and detailed descriptions of writing practices are clarified through the use of numerous examples.
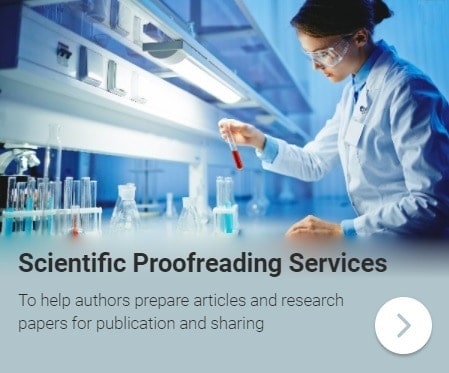
The basic components of a doctoral thesis are outlined and described, as are the elements of complete and accurate scholarly references, and detailed descriptions of writing practices are clarified through the use of numerous examples. PhD Success provides guidance for students familiar with English and the procedures of English universities, but it also acknowledges that many theses in the English language are now written by candidates whose first language is not English, so it carefully explains the scholarly styles, conventions and standards expected of a successful doctoral thesis in the English language.
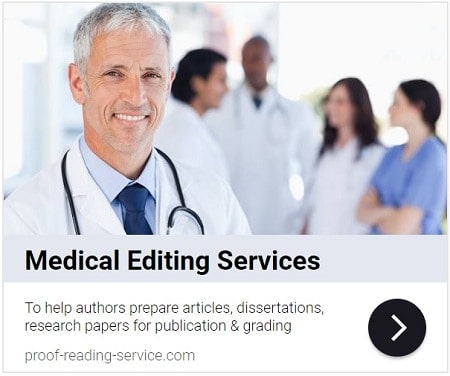
Individual chapters of this book address reflective and critical writing early in the thesis process; working successfully with thesis supervisors and benefiting from commentary and criticism; drafting and revising effective thesis chapters and developing an academic or scientific argument; writing and formatting a thesis in clear and correct scholarly English; citing, quoting and documenting sources thoroughly and accurately; and preparing for and excelling in thesis meetings and examinations.
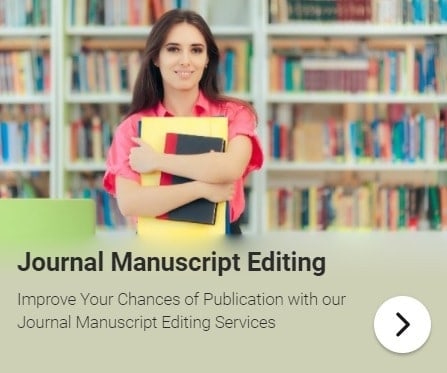
Completing a doctoral thesis successfully requires long and penetrating thought, intellectual rigour and creativity, original research and sound methods (whether established or innovative), precision in recording detail and a wide-ranging thoroughness, as much perseverance and mental toughness as insight and brilliance, and, no matter how many helpful writing guides are consulted, a great deal of hard work over a significant period of time. Writing a thesis can be an enjoyable as well as a challenging experience, however, and even if it is not always so, the personal and professional rewards of achieving such an enormous goal are considerable, as all doctoral candidates no doubt realise, and will last a great deal longer than any problems that may be encountered during the process.
Interested in Proofreading your PhD Thesis? Get in Touch with us
If you are interested in proofreading your PhD thesis or dissertation, please explore our expert dissertation proofreading services.

Rene Tetzner
Rene Tetzner's blog posts dedicated to academic writing. Although the focus is on How To Write a Doctoral Thesis, many other important aspects of research-based writing, editing and publishing are addressed in helpful detail.
Related Posts
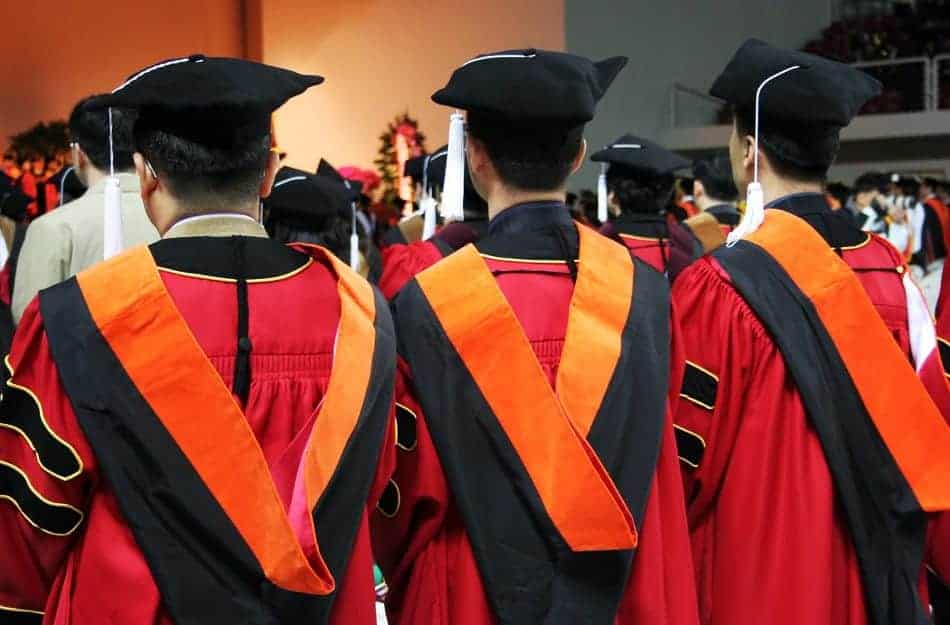
PhD Success – How To Write a Doctoral Thesis
October 1, 2021
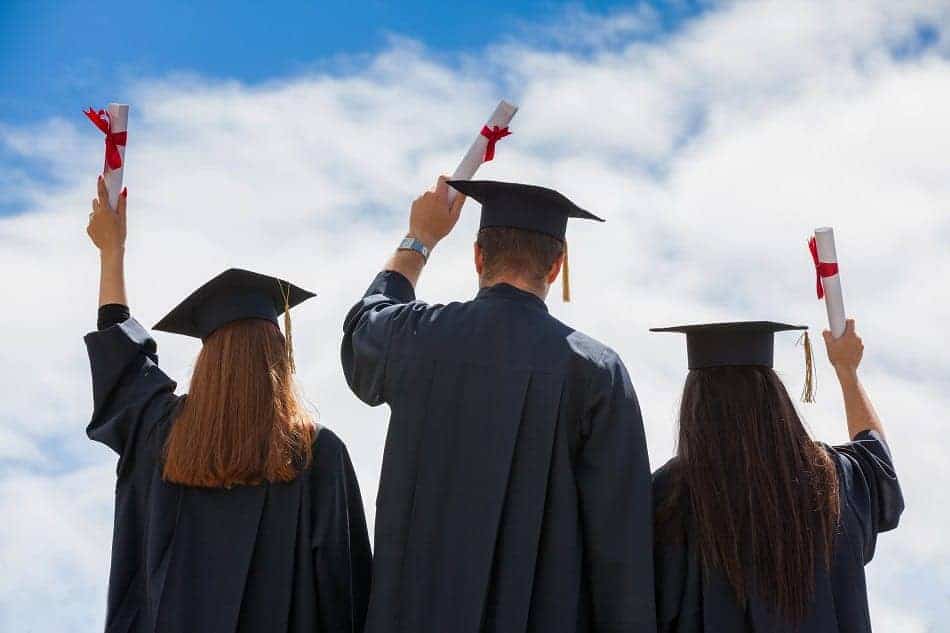
Table of Contents – PhD Success
October 2, 2021
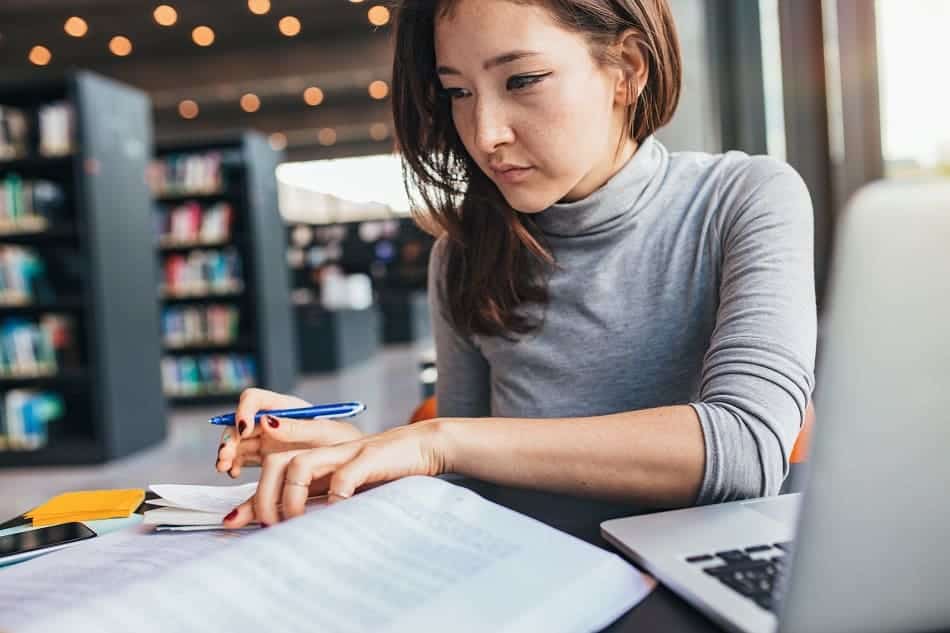
The Essential – Preliminary Matter
October 3, 2021
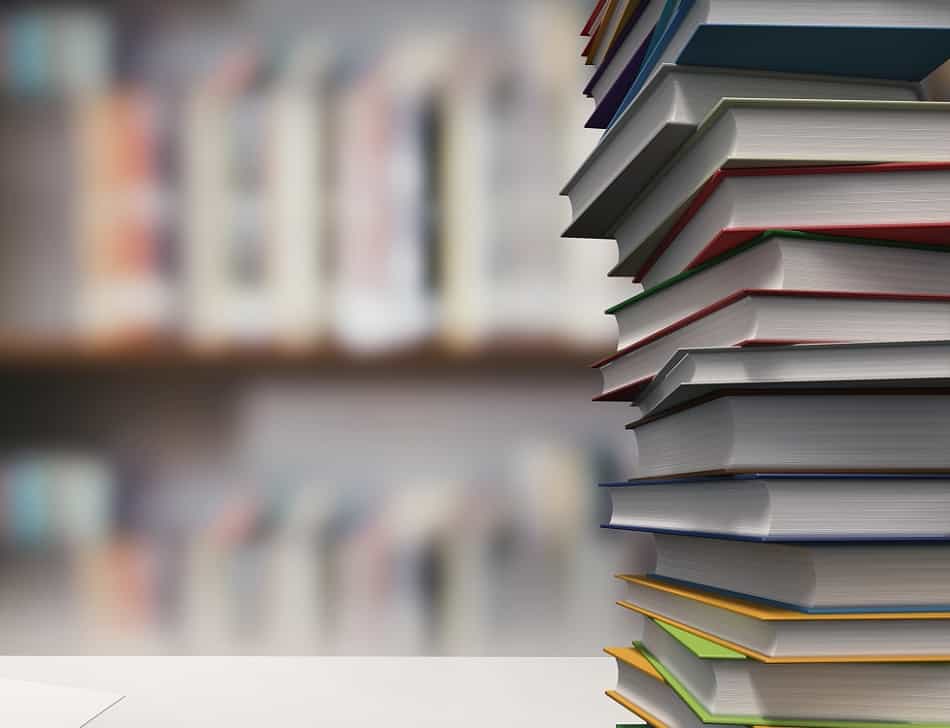
The Main Body of the Thesis
October 4, 2021
How do I make a data analysis for my bachelor, master or PhD thesis?
A data analysis is an evaluation of formal data to gain knowledge for the bachelor’s, master’s or doctoral thesis. The aim is to identify patterns in the data, i.e. regularities, irregularities or at least anomalies.
Data can come in many forms, from numbers to the extensive descriptions of objects. As a rule, this data is always in numerical form such as time series or numerical sequences or statistics of all kinds. However, statistics are already processed data.
Data analysis requires some creativity because the solution is usually not obvious. After all, no one has conducted an analysis like this before, or at least you haven't found anything about it in the literature.
The results of a data analysis are answers to initial questions and detailed questions. The answers are numbers and graphics and the interpretation of these numbers and graphics.
What are the advantages of data analysis compared to other methods?
- Numbers are universal
- The data is tangible.
- There are algorithms for calculations and it is easier than a text evaluation.
- The addressees quickly understand the results.
- You can really do magic and impress the addressees.
- It’s easier to visualize the results.
What are the disadvantages of data analysis?
- Garbage in, garbage out. If the quality of the data is poor, it’s impossible to obtain reliable results.
- The dependency in data retrieval can be quite annoying. Here are some tips for attracting participants for a survey.
- You have to know or learn methods or find someone who can help you.
- Mistakes can be devastating.
- Missing substance can be detected quickly.
- Pictures say more than a thousand words. Therefore, if you can’t fill the pages with words, at least throw in graphics. However, usually only the words count.
Under what conditions can or should I conduct a data analysis?
- If I have to.
- You must be able to get the right data.
- If I can perform the calculations myself or at least understand, explain and repeat the calculated evaluations of others.
- You want a clear personal contribution right from the start.
How do I create the evaluation design for the data analysis?
The most important thing is to ask the right questions, enough questions and also clearly formulated questions. Here are some techniques for asking the right questions:
Good formulation: What is the relationship between Alpha and Beta?
Poor formulation: How are Alpha and Beta related?
Now it’s time for the methods for the calculation. There are dozens of statistical methods, but as always, most calculations can be done with only a handful of statistical methods.
- Which detailed questions can be formulated as the research question?
- What data is available? In what format? How is the data prepared?
- Which key figures allow statements?
- What methods are available to calculate such indicators? Do my details match? By type (scales), by size (number of records).
- Do I not need to have a lot of data for a data analysis?
It depends on the media, the questions and the methods I want to use.
A fixed rule is that I need at least 30 data sets for a statistical analysis in order to be able to make representative statements about the population. So statistically it doesn't matter if I have 30 or 30 million records. That's why statistics were invented...
What mistakes do I need to watch out for?
- Don't do the analysis at the last minute.
- Formulate questions and hypotheses for evaluation BEFORE data collection!
- Stay persistent, keep going.
- Leave the results for a while then revise them.
- You have to combine theory and the state of research with your results.
- You must have the time under control
Which tools can I use?
You can use programs of all kinds for calculations. But asking questions is your most powerful aide.
Who can legally help me with a data analysis?
The great intellectual challenge is to develop the research design, to obtain the data and to interpret the results in the end.
Am I allowed to let others perform the calculations?
That's a thing. In the end, every program is useful. If someone else is operating a program, then they can simply be seen as an extension of the program. But this is a comfortable view... Of course, it’s better if you do your own calculations.
A good compromise is to find some help, do a practical calculation then follow the calculation steps meticulously so next time you can do the math yourself. Basically, this functions as a permitted training. One can then justify each step of the calculation in the defense.
What's the best place to start?
Clearly with the detailed questions and hypotheses. These two guide the entire data analysis. So formulate as many detailed questions as possible to answer your main question or research question. You can find detailed instructions and examples for the formulation of these so-called detailed questions in the Thesis Guide.
How does the Aristolo Guide help with data evaluation for the bachelor’s or master’s thesis or dissertation?
The Thesis Guide or Dissertation Guide has instructions for data collection, data preparation, data analysis and interpretation. The guide can also teach you how to formulate questions and answer them with data to create your own experiment. We also have many templates for questionnaires and analyses of all kinds. Good luck writing your text! Silvio and the Aristolo Team PS: Check out the Thesis-ABC and the Thesis Guide for writing a bachelor or master thesis in 31 days.
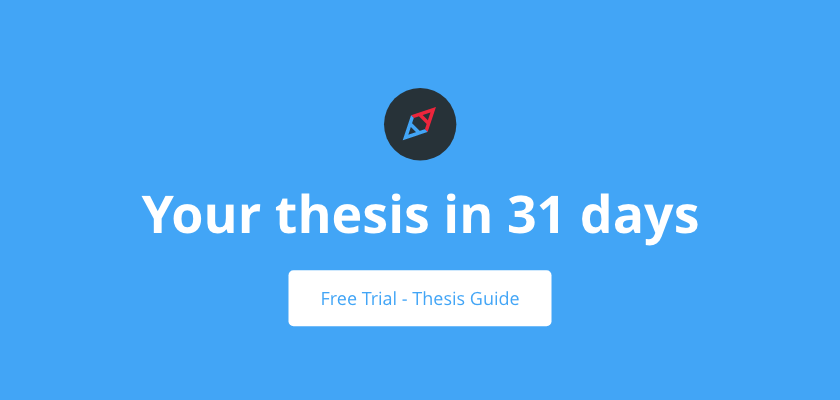
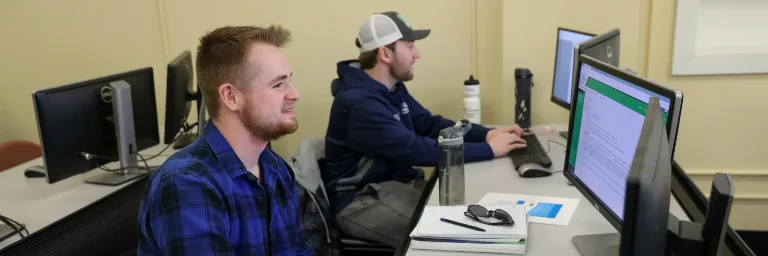
Data Science vs. Data Analytics: What’s the Difference?
Author: University of North Dakota May 22, 2024
The origins of data science are deeply rooted in the convergence of computer science and statistics.
Request Information
In the early days of computing, statisticians quickly recognized the profound potential of computers to enhance data analysis. Then, as data science continued to evolve, a complementary discipline began to take shape—data analytics.
While both fields deal with data, they exhibit distinctive characteristics and cater to different objectives, ultimately leading to divergent career paths. Continue reading to explore the key distinctions between data science vs. data analytics and determine which path aligns with your career aspirations.
What is Data Science?
Data science is an interdisciplinary field that focuses on extracting knowledge and insights from structured and unstructured data. Using various techniques, from statistics, machine learning and data mining, data scientists collect, clean and analyze data to make predictions and inform strategic decision-making. The process typically includes data collection, rigorous data cleaning, deep analysis and the interpretation of the results.
This field applies to virtually every industry, including finance, healthcare and technology, showcasing its wide-ranging utility and importance in solving complex problems.
What is Data Analytics?
Data analytics is the systematic examination of datasets to uncover valuable insights and informed conclusions, crucial for strategic decision-making in organizations.
The discipline includes several distinct approaches. Descriptive analytics summarizes past data to clarify what has occurred, setting the stage for diagnostic analytics, which probes into the causes behind these events. Building on this understanding, predictive analytics uses historical data to anticipate future outcomes. Finally, prescriptive analytics offers targeted strategies based on these forecasts, helping organizations achieve optimal results.
This progression from understanding what has happened to shaping future outcomes is vital for enhancing performance and sparking innovation across various industries.
Education Requirements
Pursuing a data science or analytics career requires a solid educational foundation in relevant fields. Each area has its specific educational paths that help aspirants acquire the necessary skills and knowledge.
Data Science Education
The journey into data science typically begins with an undergraduate degree in Data Science , Computer Science , statistics, Mathematics or a related field. These programs lay the groundwork by equipping students with essential mathematical and computational skills crucial for handling and analyzing complex data sets.
To deepen their knowledge and specialize further, many individuals pursue graduate degrees, such as a master's degreee in Data Science or related fields. These programs often focus on advanced topics like machine learning, big data technologies, artificial intelligence and sophisticated statistical methods.
Those looking to push the boundaries of data science may even opt for Ph.D. programs, which are research-focused and allow for specialization in cutting-edge areas such as deep learning, natural language processing or cognitive computing.
Data Analytics Education
Aspiring data analysts often start with undergraduate degrees in Business Analytics, Information Systems , Statistics or similar fields. These degrees provide a solid foundation for understanding how to collect, process and analyze data as well as apply it in a business context to solve problems and support decision-making.
Advancing in data analytics might involve pursuing a master's degree in Data Analytics, Business Intelligence or Applied Statistics . These programs typically focus on more strategic aspects of data analytics, including advanced statistical methods, predictive analytics and the use of analytics in business strategy. Post-graduate certifications in tools and technologies such as SQL, Tableau, SAS or Power BI are also highly valued in the industry.
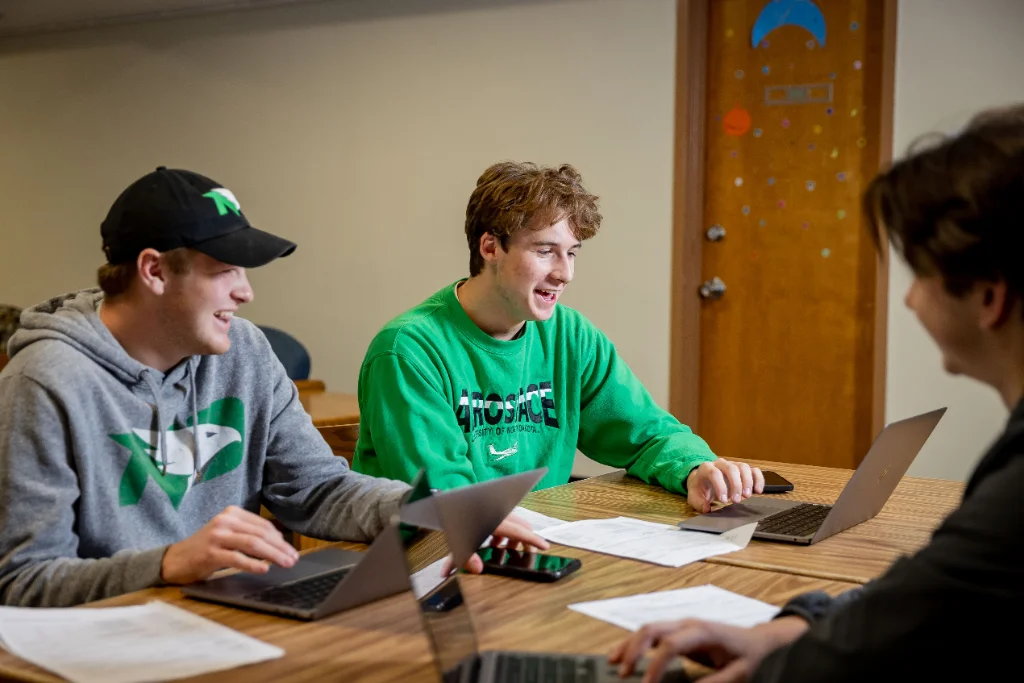
Skill Set Requirements
Both data science and data analytics professionals require a specific set of skills; however, some of these skills overlap, reflecting the integrated nature of these disciplines.
Data Science Skills
Data scientists are expected to possess a wide array of skills, each critical for various aspects of their role:
- Programming languages: Python and R for coding and data manipulation
- Machine learning: Application of algorithms and models for complex problem-solving
- Data visualization: Creation of visual representations to clarify and highlight data trends
- Domain expertise: Deep industry knowledge to effectively apply data insights
- Data analysis: Interpretation of complex datasets to extract actionable information
- Big data tools: Proficiency with platforms like Hadoop and Spark for handling large data volumes
- Deep learning frameworks: Familiarity with sophisticated models for advanced data analysis
- Statistical analysis: Application of statistical methods for meaningful data interpretation
Data Analytics Skills
Data analysts require several core competencies to effectively handle data:
- Data manipulation: Proficiency in cleaning, sorting, and organizing data
- Database management: Skilled in using SQL for efficient data retrieval and management
- Data visualization: Ability to create clear and impactful visual representations of data
- Data interpretation: Competence in analyzing large datasets to extract meaningful insights
- Analytical thinking: Strong critical thinking skills to evaluate data and assess implications
- Communication: Capability to translate technical data findings into understandable and actionable business insights
- Statistical analysis: Solid understanding of statistical methods to conduct accurate data evaluations
Career Opportunities
The fields of data science and data analytics offer varied career opportunities, some overlapping while others are unique to each field.
Data Science Careers
Data science offers a range of career paths, including:
- Data scientist
- Machine learning engineer
- Artificial intelligence specialist
- Deep learning engineer
- Big data engineer
- Algorithm developer
These roles typically focus on the development of new algorithms, predictive models and systems for data analysis and interpretation, pushing the boundaries of what organizations can achieve with data.
Data Analytics Careers
In data analytics, the roles are often more directly linked to business applications and include:
- Data analyst
- Business intelligence analyst
- Data engineer
- Marketing analyst
- Financial analyst
These professionals are crucial in analyzing data to inform business strategies, improve operational efficiency and enhance market understanding across various industries, such as finance, marketing and healthcare.
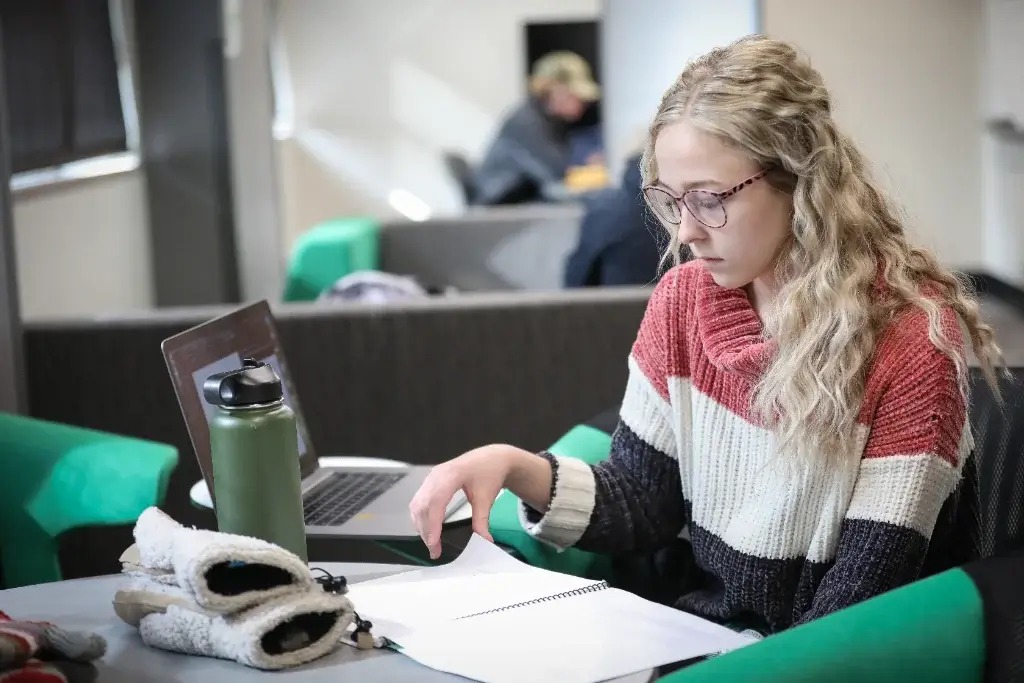
Salary and Job Outlook
The growing emphasis on data has propelled the fields of data science and data analytics into the spotlight, predicting a promising future for both domains. Not only are these roles expected to remain in high demand, but they also offer attractive salaries and substantial career growth opportunities.
Data Dcience Jobs
The role of a data scientist is not only intellectually rewarding but also financially lucrative, with an average salary of approximately $108,020 per year . The job market for data scientists is robust, boasting about 168,900 professionals engaged in this dynamic field. Looking ahead, the outlook for data science is exceptionally bright, with employment projected to grow at a rate of 35% from 2022 to 2032, a pace much faster than the average for all occupations.
Data Analytics Jobs
Similarly, data analytics professionals enjoy competitive salaries, with an average annual income of around $77,445 . The salary can vary depending on factors such as experience, location and the specific industry in which one works.
The job market for data analysts is also experiencing a significant upswing and is projected to grow by 25% by 2030. This expansion is a testament to the growing reliance on data analytics to inform and shape business decisions.
Challenges and Future Trends
Professionals in data science and analytics face numerous challenges, including maintaining data quality, managing privacy concerns and overcoming scalability issues. As data volumes continue to grow, keeping data accurate and clean becomes even more challenging.
Privacy concerns are also significant as both fields handle sensitive information, requiring compliance with increasingly stringent regulations. Scalability is another hurdle, as systems must be designed to handle large-scale data analysis.
Several trends and technologies are shaping the future of data science and data analytics. For instance, the advancement of AI and machine learning is expanding the capabilities and efficiency of data analysis. Big data analytics is also becoming more sophisticated, providing deeper insights and predictive capabilities. Additionally, there is a growing focus on the ethical aspects of data usage, pushing for transparency and fairness in data-driven decision-making.
Data Science vs. Data Analytics: Which One Should You Choose?
Choosing between a career in data science and analytics depends largely on your interests, strengths and aspirations. Data science might be the right path if you are drawn to complex mathematical models, deep statistical analysis and building algorithms.
On the other hand, if you are interested in using data to solve business problems, improve operational efficiencies and support decision-making, then a career in data analytics could be more suitable.
To make an informed decision, assess your skills in programming, mathematical analysis and interest in business applications. Reflect on whether you thrive in abstract mathematical concepts or prefer the tangible impact of data-driven solutions in business contexts. Also, consider your long-term career aspirations and how each path aligns with your goals.
Keep in mind that the fields of data science and data analytics are not mutually exclusive, and there is often overlap between the two. Ultimately, choose the path that resonates most with your passion, strengths and professional aspirations.
All in all, while both data science and data analytics revolve around the use of data, they differ significantly in scope and application. But, regardless of which path you choose, each offers a wealth of opportunities and the potential for a rewarding career in a field that is only expected to grow in relevance and demand.
Remember, in a world increasingly driven by data, your ability to analyze and interpret this vital resource can set you apart and propel you to success. Let UND help you unlock the full potential of data in your professional journey. Explore our cutting-edge programs to gain the expertise and skills necessary to thrive in these fields.
What tools and technologies are commonly used in data science compared to data analytics? ( Open this section)
Data science often utilizes tools such as Python, R, TensorFlow and Apache Hadoop, focusing on machine learning, deep learning and big data technologies. In contrast, data analytics frequently employs SQL, Tableau, Power BI and Excel, emphasizing data manipulation, visualization and reporting.
Are there any specific industries where data science is more prevalent compared to data analytics and vice versa? ( Open this section)
Data science is particularly prevalent in industries like technology, healthcare and finance, where predictive modeling and deep learning are crucial. Data analytics is more widespread in business sectors such as retail, e-commerce and marketing, where operational decisions and consumer behavior analysis are key.
How do data science and data analytics teams collaborate with other departments such as marketing, finance and operations? ( Open this section)
Data science and analytics teams work closely with marketing, finance and operations to provide insights that inform strategies and improve efficiency. They help these departments by analyzing trends, forecasting outcomes and optimizing processes based on data-driven evidence.
By clicking any link on this page you are giving your consent for us to set cookies, Privacy Information .
Methods for Integrative Analysis of Multi-Omics Data
Add to collection, downloadable content.
- Affiliation: Gillings School of Global Public Health, Department of Biostatistics
- Integrating multi-omics data has been a meaningful step in gaining a better understanding of diseases. Currently, simple correlation-based analyses are typically used in analyzing multi-omics data. However, such methods are not suitable for some data types, such as count data with overdispersion and excess zeros, without removing samples or features or applying transformations that make interpretation difficult. In our first aim, we utilize model-based correlations to reveal true relationships between metabolites and microbial species and to compare correlation patterns between ECC disease groups in the ZOE 2.0 study. Based on simulations, BZINB model-based correlation is, on average, more accurate than Spearman’s rank correlation and Pearson correlation for positively correlated vector pairs typical in microbiome and metabolome data. We utilize the correlation in identifying co-abundant modules in microbiome data using similarity-based clustering, and we identify biologically-relevant differentially correlated metabolites and species in the two ECC disease groups. In our second aim, we develop a clustering method for multi-omics features across multiple layers (metagenome, metatranscriptome, and metabolome) to identify co-abundance and co-regulation modules while assuming the directions of regulatory effect. To construct the affinity matrix, we used a rank-based similarity measure to account for zero-inflation. We predict one layer from another where there is prior knowledge of regulatory effects, using penalized zero-inflated negative binomial regression, to infer directed sparse regulatory effects. This, combined with the within-layer affinity matrix, results in a single affinity matrix that has a block structure that represents the similarities between and within each layer, to which we apply an asymmetrical normalized cut-based clustering algorithm. We evaluate the accuracy of the method in comparison to popular existing methods through simulation of zero-inflated negative binomial and rounded lognormal count data to represent multiple omics layers. In our third aim, we predict the abundances of commensal microbial genera based on DNA methylation in TCGA tissue samples. Methylation CpG sites are selected using regularized regression methods. Further development of this framework can potentially lead to multi-tissue prediction models for microbial taxa that may then be used to test association with clinical traits of interest as more data become available in the future.
- Biostatistics
- https://doi.org/10.17615/b7n5-vf53
- Dissertation
- In Copyright - Educational Use Permitted
- Franceschini, Nora
- Doctor of Philosophy
- University of North Carolina at Chapel Hill Graduate School
This work has no parents.
Select type of work
Master's papers.
Deposit your masters paper, project or other capstone work. Theses will be sent to the CDR automatically via ProQuest and do not need to be deposited.
Scholarly Articles and Book Chapters
Deposit a peer-reviewed article or book chapter. If you would like to deposit a poster, presentation, conference paper or white paper, use the “Scholarly Works” deposit form.
Undergraduate Honors Theses
Deposit your senior honors thesis.
Scholarly Journal, Newsletter or Book
Deposit a complete issue of a scholarly journal, newsletter or book. If you would like to deposit an article or book chapter, use the “Scholarly Articles and Book Chapters” deposit option.
Deposit your dataset. Datasets may be associated with an article or deposited separately.
Deposit your 3D objects, audio, images or video.
Poster, Presentation, Protocol or Paper
Deposit scholarly works such as posters, presentations, research protocols, conference papers or white papers. If you would like to deposit a peer-reviewed article or book chapter, use the “Scholarly Articles and Book Chapters” deposit option.
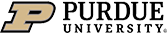
Thesis Middle High German Intensifiers
Reason: Publishing.
until file(s) become available
TTHE SYSTEM OF GERMAN INTENSIFIERS IN A CORPUS OF MIDDLE HIGH GERMAN
The present thesis presents a study conducted using corpus linguistics and variationist methods in order to explore the system of intensifiers in a corpus of Middle High German. The data consisted of a sample of adjectives from different texts from the Middle High German period (1050-1350). The study of intensifiers (e.g. sehr ‘ very ’, wirkick ‘ really ’, ganz ‘ quite ’ ) has been a topic of much discussion, mainly in English. On the contrary, there is little research on the system of German intensifiers (e.g. sehr, wirklich, ganz ). The analysis of data resulted in amplifiers being the most frequent type of intensifiers. Besides the thesis drew the distribution of the most frequent variants found in the sample, and the overall occurrence of intensifiers among different text types. Furthermore, the results suggest that the rate of intensification is relatively low and remains low throughout most of the Middle High German period, but is higher in courtly texts and religious legends than in other types of texts. These findings were comparable to the previous research done, suggesting that intensification of adjectives in German behaved similarly to what have been observed in English.
Degree Type
- Master of Arts
- Linguistics
Campus location
- West Lafayette
Advisor/Supervisor/Committee Chair
Additional committee member 2, additional committee member 3, usage metrics.
- Corpus linguistics
- Historical, comparative and typological linguistics

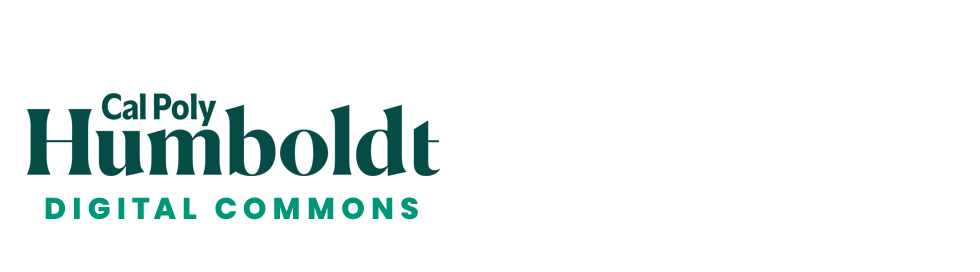
- < Previous
Home > Theses > 765
Cal Poly Humboldt theses and projects
The "upside-down" river: trends in total nitrogen, total phosphorus, and microcystin concentration in the klamath basin, 2010-2021.
Kayla M. Fitzpatrick , Humboldt State University Follow
Graduation Date
Spring 2024
Document Type
Master of Science degree with a major in Natural Resources, option Forestry, Watershed, & Wildland Sciences
Committee Chair Name
Andrew Stubblefield
Committee Chair Affiliation
HSU Faculty or Staff
Second Committee Member Name
Alison O'Dowd
Second Committee Member Affiliation
Third committee member name.
Margarita Otero-Diaz
Klamath River, Water quality, Northern California, Southern Oregon, Microcystin, Total nitrogen, Total phosphorus, Harmful algal bloom, Cyanobacteria, Cyanotoxin, River, Salmonid
Subject Categories
Natural Resources
The Klamath River tends to experience impaired upstream water quality, which improves downstream. Although this consensus is accepted, an updated spatiotemporal analysis of certain water quality parameters has not been conducted since 2017. With four of the Klamath River’s dams slated for removal by the end of 2024, it is critical to have an updated assessment of current water quality conditions from which to compare conditions following dam removal. In this study, total nitrogen (TN), total phosphorus (TP), and microcystin data from 2010-2021 were analyzed to determine concentration trends from Link Dam to the Klamath River Estuary.
Concentrations of TN and TP generally decrease with river mile, but nutrient levels in tributaries and reservoirs are more variable. Microcystin concentrations are generally highest in reservoirs. TN is generally increasing over time, while TP trends vary by site. Microcystin is generally decreasing or experiencing no trend, though some sites did not have enough data to complete microcystin temporal analysis. TN and TP concentrations consistently exceeded target level, and microcystin exceedances in reservoirs are often the most frequent and severe.
Based on this analysis, the mainstem Klamath River does tend to experience elevated nutrient concentrations upstream which improves downstream. The river, then, is “upside-down” in terms of nutrient concentration. The results of this study can be compared against future water quality monitoring and analysis efforts following dam removal, as evidence of the impacts of dam removal on water quality. This thesis is a small contribution toward the collective goal of understanding water quality in the Klamath Basin.
Recommended Citation
Fitzpatrick, Kayla M., "The "upside-down" river: Trends in total nitrogen, total phosphorus, and microcystin concentration in the Klamath Basin, 2010-2021" (2024). Cal Poly Humboldt theses and projects . 765. https://digitalcommons.humboldt.edu/etd/765
Included in
Water Resource Management Commons
Thesis/Project Location
To view the content in your browser, please download Adobe Reader or, alternately, you may Download the file to your hard drive.
NOTE: The latest versions of Adobe Reader do not support viewing PDF files within Firefox on Mac OS and if you are using a modern (Intel) Mac, there is no official plugin for viewing PDF files within the browser window.
Advanced Search
- Notify me via email or RSS
- Collections
Author Corner
- Submission Guidelines
- Submit Thesis
- Accessibility Resources Center
- HSU Library
- Graduate Studies
- HDS Non-exclusive Distribution License
- Submission Process
Thesis/Project Locations
- View theses/projects on map
- View theses/projects in Google Earth
Home | About | FAQ | My Account | Accessibility Statement
Privacy Copyright
- Get 7 Days Free
Zebra Technologies Corp Class A
Zebra: positive innovation day aligns with our moat thesis.
/s3.amazonaws.com/arc-authors/morningstar/ca129aad-9614-4bf0-993b-3df5f6bfa41e.jpg)
We maintain our $320 fair value estimate for Zebra Technologies after its 2024 Innovation Day showcase aligned with our positive view of the company and our long-term thesis. Zebra highlighted its product portfolio, customer relationships, and strategy for digital transformation, all of which we liked but weren’t new. We appreciate Zebra’s sticky ecosystem of solutions, comprising integrated hardware, software, and services. We believe these embed deeply in customers and contribute to the firm’s economic moat. Management also reiterated its existing financial targets, which align with our model. We see shares as fairly valued today.
Free Trial of Morningstar Investor
Get our analysts’ objective, in-depth, and continuous investment coverage of ZBRA so you can make buy / sell decisions free of market noise.
Sponsor Center

IMAGES
VIDEO
COMMENTS
And place questionnaires, copies of focus groups and interviews, and data sheets in the appendix. On the other hand, one must put the statistical analysis and sayings quoted by interviewees within the dissertation. 8. Thoroughness of Data. It is a common misconception that the data presented is self-explanatory.
This article is a practical guide to conducting data analysis in general literature reviews. The general literature review is a synthesis and analysis of published research on a relevant clinical issue, and is a common format for academic theses at the bachelor's and master's levels in nursing, physiotherapy, occupational therapy, public health and other related fields.
The results chapter (also referred to as the findings or analysis chapter) is one of the most important chapters of your dissertation or thesis because it shows the reader what you've found in terms of the quantitative data you've collected. It presents the data using a clear text narrative, supported by tables, graphs and charts.
An understanding of the data analysis that you will carry out on your data can also be an expected component of the Research Strategy chapter of your dissertation write-up (i.e., usually Chapter Three: Research Strategy). Therefore, it is a good time to think about the data analysis process if you plan to start writing up this chapter at this ...
This guide discusses the application of quantitative data analysis to your thesis statement. Writing a Strong Thesis Statement. In a relatively short essay of 10 to 15 pages, the thesis statement is generally found in the introductory paragraph. This kind of thesis statement is also typically rather short and straightforward.
Quantitative data analysis is one of those things that often strikes fear in students. It's totally understandable - quantitative analysis is a complex topic, full of daunting lingo, like medians, modes, correlation and regression.Suddenly we're all wishing we'd paid a little more attention in math class…. The good news is that while quantitative data analysis is a mammoth topic ...
QDA Method #3: Discourse Analysis. Discourse is simply a fancy word for written or spoken language or debate. So, discourse analysis is all about analysing language within its social context. In other words, analysing language - such as a conversation, a speech, etc - within the culture and society it takes place.
A data analysis dissertation is a complex and challenging project requiring significant time, effort, and expertise. Fortunately, it is possible to successfully complete a data analysis dissertation with careful planning and execution. ... c. Develop a Strong Thesis Statement. After you've done your research, it's time to start developing ...
A Data Analysis Plan (DAP) is about putting thoughts into a plan of action. Research questions are often framed broadly and need to be clarified and funnelled down into testable hypotheses and action steps. The DAP provides an opportunity for input from collaborators and provides a platform for training. Having a clear plan of action is also ...
The method you choose will depend on your research objectives and questions. These are the most common qualitative data analysis methods to help you complete your dissertation: 2. Content analysis: This method is used to analyze documented information from texts, email, media and tangible items.
A results section is where you report the main findings of the data collection and analysis you conducted for your thesis or dissertation. You should report all relevant results concisely and objectively, in a logical order. ... you can structure your results section around key themes or topics that emerged from your analysis of the data. For ...
Dissertation methodologies require a data analysis plan. Your dissertation data analysis plan should clearly state the statistical tests and assumptions of these tests to examine each of the research questions, how scores are cleaned and created, and the desired sample size for that test. The selection of statistical tests depend on two factors ...
Table of contents. Step 1: Write your hypotheses and plan your research design. Step 2: Collect data from a sample. Step 3: Summarize your data with descriptive statistics. Step 4: Test hypotheses or make estimates with inferential statistics.
5 Tips for Handling your Thesis Data Analysis. When writing your thesis, the process of analyzing data and working with statistics can be pretty hard at first. This is true whether you're using specialized data analysis software, like SPSS, or a more descriptive approach. But there are a few guidelines you can follow to make things simpler.
According to Belotto (2018), data analysis refers to the process of data management, description, evaluation, and interpretation. Thematic analysis is a method of data analysis in qualitative ...
4.1 INTRODUCTION. In this chapter, I describe the qualitative analysis of the data, including the practical steps involved in the analysis. A quantitative analysis of the data follows in Chapter 5. In the qualitative phase, I analyzed the data into generative themes, which will be described individually. I describe how the themes overlap.
In this article, we take up this open question as a point of departure and offer thematic analysis, an analytic method commonly used to identify patterns across language-based data (Braun & Clarke, 2006), as a useful starting point for learning about the qualitative analysis process.In doing so, we do not advocate for only learning the nuances of thematic analysis, but rather see it as a ...
Data analysis methods in the absence of primary data collection can involve discussing common patterns, as well as, controversies within secondary data directly related to the research area. My e-book, The Ultimate Guide to Writing a Dissertation in Business Studies: a step by step assistance offers practical assistance to complete a ...
interpretation of qualitative data collected for this thesis. 6.2.1 Analysis of qualitative data Qualitative data analysis can be described as the process of making sense from research participants‟ views and opinions of situations, corresponding patterns, themes, categories and ... data analysis well, when he provides the following ...
Fast-Track Your Data Analysis, Today. Enter your details below, pop us an email, or book an introductory consultation. If you are a human seeing this field, please leave it empty. Get 1-on-1 help analysing and interpreting your qualitative or quantitative dissertation or thesis data from the experts at Grad Coach. Book online now.
Score 94% Score 94%. 4.4 Writing the Data Analysis Chapter (s): Results and Evidence. Unlike the introduction, literature review and methodology chapter (s), your results chapter (s) will need to be written for the first time as you draft your thesis even if you submitted a proposal, though this part of your thesis will certainly build upon the ...
A data analysis is an evaluation of formal data to gain knowledge for the bachelor's, master's or doctoral thesis. The aim is to identify patterns in the data, i.e. regularities, irregularities or at least anomalies. Data can come in many forms, from numbers to the extensive descriptions of objects. As a rule, this data is always in ...
The main aim of this contribution is to present some possibilities and tools of data analysis with regards to availability of final users. Star schema Snowflake schema-in this schema are data ...
Open. Data science often utilizes tools such as Python, R, TensorFlow and Apache Hadoop, focusing on machine learning, deep learning and big data technologies. In contrast, data analytics frequently employs SQL, Tableau, Power BI and Excel, emphasizing data manipulation, visualization and reporting.
Integrating multi-omics data has been a meaningful step in gaining a better understanding of diseases. Currently, simple correlation-based analyses are typically used in analyzing multi-omics data. However, such methods are not suitable for some data types, such as count data with overdispersion and excess zeros, without removing samples or ...
The present thesis presents a study conducted using corpus linguistics and variationist methods in order to explore the system of intensifiers in a corpus of Middle High German. The data consisted of a sample of adjectives from different texts from the Middle High German period (1050-1350). The study of intensifiers (e.g. sehr 'very', wirkick 'really', ganz 'quite') has been a ...
Data is threatened during transmission and storage, which can lead to the leakage of sensitive information, while manual operations can lead to errors and low efficiency. ... Patent-Thesis Analysis, Global Trend, Technical Strengths and Weaknesses of each country and company. Read More. Artificial Intelligence based Hardware: Patent-Thesis ...
The Klamath River tends to experience impaired upstream water quality, which improves downstream. Although this consensus is accepted, an updated spatiotemporal analysis of certain water quality parameters has not been conducted since 2017. With four of the Klamath River's dams slated for removal by the end of 2024, it is critical to have an updated assessment of current water quality ...
May 14, 2024. We maintain our $320 fair value estimate for Zebra Technologies after its 2024 Innovation Day showcase aligned with our positive view of the company and our long-term thesis. Zebra ...