
An official website of the United States government
The .gov means it’s official. Federal government websites often end in .gov or .mil. Before sharing sensitive information, make sure you’re on a federal government site.
The site is secure. The https:// ensures that you are connecting to the official website and that any information you provide is encrypted and transmitted securely.
- Publications
- Account settings
Preview improvements coming to the PMC website in October 2024. Learn More or Try it out now .
- Advanced Search
- Journal List
- J Korean Med Sci
- v.34(45); 2019 Nov 25


Scientific Hypotheses: Writing, Promoting, and Predicting Implications
Armen yuri gasparyan.
1 Departments of Rheumatology and Research and Development, Dudley Group NHS Foundation Trust (Teaching Trust of the University of Birmingham, UK), Russells Hall Hospital, Dudley, West Midlands, UK.
Lilit Ayvazyan
2 Department of Medical Chemistry, Yerevan State Medical University, Yerevan, Armenia.
Ulzhan Mukanova
3 Department of Surgical Disciplines, South Kazakhstan Medical Academy, Shymkent, Kazakhstan.
Marlen Yessirkepov
4 Department of Biology and Biochemistry, South Kazakhstan Medical Academy, Shymkent, Kazakhstan.
George D. Kitas
5 Arthritis Research UK Epidemiology Unit, University of Manchester, Manchester, UK.
Scientific hypotheses are essential for progress in rapidly developing academic disciplines. Proposing new ideas and hypotheses require thorough analyses of evidence-based data and predictions of the implications. One of the main concerns relates to the ethical implications of the generated hypotheses. The authors may need to outline potential benefits and limitations of their suggestions and target widely visible publication outlets to ignite discussion by experts and start testing the hypotheses. Not many publication outlets are currently welcoming hypotheses and unconventional ideas that may open gates to criticism and conservative remarks. A few scholarly journals guide the authors on how to structure hypotheses. Reflecting on general and specific issues around the subject matter is often recommended for drafting a well-structured hypothesis article. An analysis of influential hypotheses, presented in this article, particularly Strachan's hygiene hypothesis with global implications in the field of immunology and allergy, points to the need for properly interpreting and testing new suggestions. Envisaging the ethical implications of the hypotheses should be considered both by authors and journal editors during the writing and publishing process.
INTRODUCTION
We live in times of digitization that radically changes scientific research, reporting, and publishing strategies. Researchers all over the world are overwhelmed with processing large volumes of information and searching through numerous online platforms, all of which make the whole process of scholarly analysis and synthesis complex and sophisticated.
Current research activities are diversifying to combine scientific observations with analysis of facts recorded by scholars from various professional backgrounds. 1 Citation analyses and networking on social media are also becoming essential for shaping research and publishing strategies globally. 2 Learning specifics of increasingly interdisciplinary research studies and acquiring information facilitation skills aid researchers in formulating innovative ideas and predicting developments in interrelated scientific fields.
Arguably, researchers are currently offered more opportunities than in the past for generating new ideas by performing their routine laboratory activities, observing individual cases and unusual developments, and critically analyzing published scientific facts. What they need at the start of their research is to formulate a scientific hypothesis that revisits conventional theories, real-world processes, and related evidence to propose new studies and test ideas in an ethical way. 3 Such a hypothesis can be of most benefit if published in an ethical journal with wide visibility and exposure to relevant online databases and promotion platforms.
Although hypotheses are crucially important for the scientific progress, only few highly skilled researchers formulate and eventually publish their innovative ideas per se . Understandably, in an increasingly competitive research environment, most authors would prefer to prioritize their ideas by discussing and conducting tests in their own laboratories or clinical departments, and publishing research reports afterwards. However, there are instances when simple observations and research studies in a single center are not capable of explaining and testing new groundbreaking ideas. Formulating hypothesis articles first and calling for multicenter and interdisciplinary research can be a solution in such instances, potentially launching influential scientific directions, if not academic disciplines.
The aim of this article is to overview the importance and implications of infrequently published scientific hypotheses that may open new avenues of thinking and research.
Despite the seemingly established views on innovative ideas and hypotheses as essential research tools, no structured definition exists to tag the term and systematically track related articles. In 1973, the Medical Subject Heading (MeSH) of the U.S. National Library of Medicine introduced “Research Design” as a structured keyword that referred to the importance of collecting data and properly testing hypotheses, and indirectly linked the term to ethics, methods and standards, among many other subheadings.
One of the experts in the field defines “hypothesis” as a well-argued analysis of available evidence to provide a realistic (scientific) explanation of existing facts, fill gaps in public understanding of sophisticated processes, and propose a new theory or a test. 4 A hypothesis can be proven wrong partially or entirely. However, even such an erroneous hypothesis may influence progress in science by initiating professional debates that help generate more realistic ideas. The main ethical requirement for hypothesis authors is to be honest about the limitations of their suggestions. 5
EXAMPLES OF INFLUENTIAL SCIENTIFIC HYPOTHESES
Daily routine in a research laboratory may lead to groundbreaking discoveries provided the daily accounts are comprehensively analyzed and reproduced by peers. The discovery of penicillin by Sir Alexander Fleming (1928) can be viewed as a prime example of such discoveries that introduced therapies to treat staphylococcal and streptococcal infections and modulate blood coagulation. 6 , 7 Penicillin got worldwide recognition due to the inventor's seminal works published by highly prestigious and widely visible British journals, effective ‘real-world’ antibiotic therapy of pneumonia and wounds during World War II, and euphoric media coverage. 8 In 1945, Fleming, Florey and Chain got a much deserved Nobel Prize in Physiology or Medicine for the discovery that led to the mass production of the wonder drug in the U.S. and ‘real-world practice’ that tested the use of penicillin. What remained globally unnoticed is that Zinaida Yermolyeva, the outstanding Soviet microbiologist, created the Soviet penicillin, which turned out to be more effective than the Anglo-American penicillin and entered mass production in 1943; that year marked the turning of the tide of the Great Patriotic War. 9 One of the reasons of the widely unnoticed discovery of Zinaida Yermolyeva is that her works were published exclusively by local Russian (Soviet) journals.
The past decades have been marked by an unprecedented growth of multicenter and global research studies involving hundreds and thousands of human subjects. This trend is shaped by an increasing number of reports on clinical trials and large cohort studies that create a strong evidence base for practice recommendations. Mega-studies may help generate and test large-scale hypotheses aiming to solve health issues globally. Properly designed epidemiological studies, for example, may introduce clarity to the hygiene hypothesis that was originally proposed by David Strachan in 1989. 10 David Strachan studied the epidemiology of hay fever in a cohort of 17,414 British children and concluded that declining family size and improved personal hygiene had reduced the chances of cross infections in families, resulting in epidemics of atopic disease in post-industrial Britain. Over the past four decades, several related hypotheses have been proposed to expand the potential role of symbiotic microorganisms and parasites in the development of human physiological immune responses early in life and protection from allergic and autoimmune diseases later on. 11 , 12 Given the popularity and the scientific importance of the hygiene hypothesis, it was introduced as a MeSH term in 2012. 13
Hypotheses can be proposed based on an analysis of recorded historic events that resulted in mass migrations and spreading of certain genetic diseases. As a prime example, familial Mediterranean fever (FMF), the prototype periodic fever syndrome, is believed to spread from Mesopotamia to the Mediterranean region and all over Europe due to migrations and religious prosecutions millennia ago. 14 Genetic mutations spearing mild clinical forms of FMF are hypothesized to emerge and persist in the Mediterranean region as protective factors against more serious infectious diseases, particularly tuberculosis, historically common in that part of the world. 15 The speculations over the advantages of carrying the MEditerranean FeVer (MEFV) gene are further strengthened by recorded low mortality rates from tuberculosis among FMF patients of different nationalities living in Tunisia in the first half of the 20th century. 16
Diagnostic hypotheses shedding light on peculiarities of diseases throughout the history of mankind can be formulated using artefacts, particularly historic paintings. 17 Such paintings may reveal joint deformities and disfigurements due to rheumatic diseases in individual subjects. A series of paintings with similar signs of pathological conditions interpreted in a historic context may uncover mysteries of epidemics of certain diseases, which is the case with Ruben's paintings depicting signs of rheumatic hands and making some doctors to believe that rheumatoid arthritis was common in Europe in the 16th and 17th century. 18
WRITING SCIENTIFIC HYPOTHESES
There are author instructions of a few journals that specifically guide how to structure, format, and make submissions categorized as hypotheses attractive. One of the examples is presented by Med Hypotheses , the flagship journal in its field with more than four decades of publishing and influencing hypothesis authors globally. However, such guidance is not based on widely discussed, implemented, and approved reporting standards, which are becoming mandatory for all scholarly journals.
Generating new ideas and scientific hypotheses is a sophisticated task since not all researchers and authors are skilled to plan, conduct, and interpret various research studies. Some experience with formulating focused research questions and strong working hypotheses of original research studies is definitely helpful for advancing critical appraisal skills. However, aspiring authors of scientific hypotheses may need something different, which is more related to discerning scientific facts, pooling homogenous data from primary research works, and synthesizing new information in a systematic way by analyzing similar sets of articles. To some extent, this activity is reminiscent of writing narrative and systematic reviews. As in the case of reviews, scientific hypotheses need to be formulated on the basis of comprehensive search strategies to retrieve all available studies on the topics of interest and then synthesize new information selectively referring to the most relevant items. One of the main differences between scientific hypothesis and review articles relates to the volume of supportive literature sources ( Table 1 ). In fact, hypothesis is usually formulated by referring to a few scientific facts or compelling evidence derived from a handful of literature sources. 19 By contrast, reviews require analyses of a large number of published documents retrieved from several well-organized and evidence-based databases in accordance with predefined search strategies. 20 , 21 , 22
The format of hypotheses, especially the implications part, may vary widely across disciplines. Clinicians may limit their suggestions to the clinical manifestations of diseases, outcomes, and management strategies. Basic and laboratory scientists analysing genetic, molecular, and biochemical mechanisms may need to view beyond the frames of their narrow fields and predict social and population-based implications of the proposed ideas. 23
Advanced writing skills are essential for presenting an interesting theoretical article which appeals to the global readership. Merely listing opposing facts and ideas, without proper interpretation and analysis, may distract the experienced readers. The essence of a great hypothesis is a story behind the scientific facts and evidence-based data.
ETHICAL IMPLICATIONS
The authors of hypotheses substantiate their arguments by referring to and discerning rational points from published articles that might be overlooked by others. Their arguments may contradict the established theories and practices, and pose global ethical issues, particularly when more or less efficient medical technologies and public health interventions are devalued. The ethical issues may arise primarily because of the careless references to articles with low priorities, inadequate and apparently unethical methodologies, and concealed reporting of negative results. 24 , 25
Misinterpretation and misunderstanding of the published ideas and scientific hypotheses may complicate the issue further. For example, Alexander Fleming, whose innovative ideas of penicillin use to kill susceptible bacteria saved millions of lives, warned of the consequences of uncontrolled prescription of the drug. The issue of antibiotic resistance had emerged within the first ten years of penicillin use on a global scale due to the overprescription that affected the efficacy of antibiotic therapies, with undesirable consequences for millions. 26
The misunderstanding of the hygiene hypothesis that primarily aimed to shed light on the role of the microbiome in allergic and autoimmune diseases resulted in decline of public confidence in hygiene with dire societal implications, forcing some experts to abandon the original idea. 27 , 28 Although that hypothesis is unrelated to the issue of vaccinations, the public misunderstanding has resulted in decline of vaccinations at a time of upsurge of old and new infections.
A number of ethical issues are posed by the denial of the viral (human immunodeficiency viruses; HIV) hypothesis of acquired Immune deficiency Syndrome (AIDS) by Peter Duesberg, who overviewed the links between illicit recreational drugs and antiretroviral therapies with AIDS and refuted the etiological role of HIV. 29 That controversial hypothesis was rejected by several journals, but was eventually published without external peer review at Med Hypotheses in 2010. The publication itself raised concerns of the unconventional editorial policy of the journal, causing major perturbations and more scrutinized publishing policies by journals processing hypotheses.
WHERE TO PUBLISH HYPOTHESES
Although scientific authors are currently well informed and equipped with search tools to draft evidence-based hypotheses, there are still limited quality publication outlets calling for related articles. The journal editors may be hesitant to publish articles that do not adhere to any research reporting guidelines and open gates for harsh criticism of unconventional and untested ideas. Occasionally, the editors opting for open-access publishing and upgrading their ethics regulations launch a section to selectively publish scientific hypotheses attractive to the experienced readers. 30 However, the absence of approved standards for this article type, particularly no mandate for outlining potential ethical implications, may lead to publication of potentially harmful ideas in an attractive format.
A suggestion of simultaneously publishing multiple or alternative hypotheses to balance the reader views and feedback is a potential solution for the mainstream scholarly journals. 31 However, that option alone is hardly applicable to emerging journals with unconventional quality checks and peer review, accumulating papers with multiple rejections by established journals.
A large group of experts view hypotheses with improbable and controversial ideas publishable after formal editorial (in-house) checks to preserve the authors' genuine ideas and avoid conservative amendments imposed by external peer reviewers. 32 That approach may be acceptable for established publishers with large teams of experienced editors. However, the same approach can lead to dire consequences if employed by nonselective start-up, open-access journals processing all types of articles and primarily accepting those with charged publication fees. 33 In fact, pseudoscientific ideas arguing Newton's and Einstein's seminal works or those denying climate change that are hardly testable have already found their niche in substandard electronic journals with soft or nonexistent peer review. 34
CITATIONS AND SOCIAL MEDIA ATTENTION
The available preliminary evidence points to the attractiveness of hypothesis articles for readers, particularly those from research-intensive countries who actively download related documents. 35 However, citations of such articles are disproportionately low. Only a small proportion of top-downloaded hypotheses (13%) in the highly prestigious Med Hypotheses receive on average 5 citations per article within a two-year window. 36
With the exception of a few historic papers, the vast majority of hypotheses attract relatively small number of citations in a long term. 36 Plausible explanations are that these articles often contain a single or only a few citable points and that suggested research studies to test hypotheses are rarely conducted and reported, limiting chances of citing and crediting authors of genuine research ideas.
A snapshot analysis of citation activity of hypothesis articles may reveal interest of the global scientific community towards their implications across various disciplines and countries. As a prime example, Strachan's hygiene hypothesis, published in 1989, 10 is still attracting numerous citations on Scopus, the largest bibliographic database. As of August 28, 2019, the number of the linked citations in the database is 3,201. Of the citing articles, 160 are cited at least 160 times ( h -index of this research topic = 160). The first three citations are recorded in 1992 and followed by a rapid annual increase in citation activity and a peak of 212 in 2015 ( Fig. 1 ). The top 5 sources of the citations are Clin Exp Allergy (n = 136), J Allergy Clin Immunol (n = 119), Allergy (n = 81), Pediatr Allergy Immunol (n = 69), and PLOS One (n = 44). The top 5 citing authors are leading experts in pediatrics and allergology Erika von Mutius (Munich, Germany, number of publications with the index citation = 30), Erika Isolauri (Turku, Finland, n = 27), Patrick G Holt (Subiaco, Australia, n = 25), David P. Strachan (London, UK, n = 23), and Bengt Björksten (Stockholm, Sweden, n = 22). The U.S. is the leading country in terms of citation activity with 809 related documents, followed by the UK (n = 494), Germany (n = 314), Australia (n = 211), and the Netherlands (n = 177). The largest proportion of citing documents are articles (n = 1,726, 54%), followed by reviews (n = 950, 29.7%), and book chapters (n = 213, 6.7%). The main subject areas of the citing items are medicine (n = 2,581, 51.7%), immunology and microbiology (n = 1,179, 23.6%), and biochemistry, genetics and molecular biology (n = 415, 8.3%).

Interestingly, a recent analysis of 111 publications related to Strachan's hygiene hypothesis, stating that the lack of exposure to infections in early life increases the risk of rhinitis, revealed a selection bias of 5,551 citations on Web of Science. 37 The articles supportive of the hypothesis were cited more than nonsupportive ones (odds ratio adjusted for study design, 2.2; 95% confidence interval, 1.6–3.1). A similar conclusion pointing to a citation bias distorting bibliometrics of hypotheses was reached by an earlier analysis of a citation network linked to the idea that β-amyloid, which is involved in the pathogenesis of Alzheimer disease, is produced by skeletal muscle of patients with inclusion body myositis. 38 The results of both studies are in line with the notion that ‘positive’ citations are more frequent in the field of biomedicine than ‘negative’ ones, and that citations to articles with proven hypotheses are too common. 39
Social media channels are playing an increasingly active role in the generation and evaluation of scientific hypotheses. In fact, publicly discussing research questions on platforms of news outlets, such as Reddit, may shape hypotheses on health-related issues of global importance, such as obesity. 40 Analyzing Twitter comments, researchers may reveal both potentially valuable ideas and unfounded claims that surround groundbreaking research ideas. 41 Social media activities, however, are unevenly distributed across different research topics, journals and countries, and these are not always objective professional reflections of the breakthroughs in science. 2 , 42
Scientific hypotheses are essential for progress in science and advances in healthcare. Innovative ideas should be based on a critical overview of related scientific facts and evidence-based data, often overlooked by others. To generate realistic hypothetical theories, the authors should comprehensively analyze the literature and suggest relevant and ethically sound design for future studies. They should also consider their hypotheses in the context of research and publication ethics norms acceptable for their target journals. The journal editors aiming to diversify their portfolio by maintaining and introducing hypotheses section are in a position to upgrade guidelines for related articles by pointing to general and specific analyses of the subject, preferred study designs to test hypotheses, and ethical implications. The latter is closely related to specifics of hypotheses. For example, editorial recommendations to outline benefits and risks of a new laboratory test or therapy may result in a more balanced article and minimize associated risks afterwards.
Not all scientific hypotheses have immediate positive effects. Some, if not most, are never tested in properly designed research studies and never cited in credible and indexed publication outlets. Hypotheses in specialized scientific fields, particularly those hardly understandable for nonexperts, lose their attractiveness for increasingly interdisciplinary audience. The authors' honest analysis of the benefits and limitations of their hypotheses and concerted efforts of all stakeholders in science communication to initiate public discussion on widely visible platforms and social media may reveal rational points and caveats of the new ideas.
Disclosure: The authors have no potential conflicts of interest to disclose.
Author Contributions:
- Conceptualization: Gasparyan AY, Yessirkepov M, Kitas GD.
- Methodology: Gasparyan AY, Mukanova U, Ayvazyan L.
- Writing - original draft: Gasparyan AY, Ayvazyan L, Yessirkepov M.
- Writing - review & editing: Gasparyan AY, Yessirkepov M, Mukanova U, Kitas GD.

What Is A Research (Scientific) Hypothesis? A plain-language explainer + examples
By: Derek Jansen (MBA) | Reviewed By: Dr Eunice Rautenbach | June 2020
If you’re new to the world of research, or it’s your first time writing a dissertation or thesis, you’re probably noticing that the words “research hypothesis” and “scientific hypothesis” are used quite a bit, and you’re wondering what they mean in a research context .
“Hypothesis” is one of those words that people use loosely, thinking they understand what it means. However, it has a very specific meaning within academic research. So, it’s important to understand the exact meaning before you start hypothesizing.
Research Hypothesis 101
- What is a hypothesis ?
- What is a research hypothesis (scientific hypothesis)?
- Requirements for a research hypothesis
- Definition of a research hypothesis
- The null hypothesis
What is a hypothesis?
Let’s start with the general definition of a hypothesis (not a research hypothesis or scientific hypothesis), according to the Cambridge Dictionary:
Hypothesis: an idea or explanation for something that is based on known facts but has not yet been proved.
In other words, it’s a statement that provides an explanation for why or how something works, based on facts (or some reasonable assumptions), but that has not yet been specifically tested . For example, a hypothesis might look something like this:
Hypothesis: sleep impacts academic performance.
This statement predicts that academic performance will be influenced by the amount and/or quality of sleep a student engages in – sounds reasonable, right? It’s based on reasonable assumptions , underpinned by what we currently know about sleep and health (from the existing literature). So, loosely speaking, we could call it a hypothesis, at least by the dictionary definition.
But that’s not good enough…
Unfortunately, that’s not quite sophisticated enough to describe a research hypothesis (also sometimes called a scientific hypothesis), and it wouldn’t be acceptable in a dissertation, thesis or research paper . In the world of academic research, a statement needs a few more criteria to constitute a true research hypothesis .
What is a research hypothesis?
A research hypothesis (also called a scientific hypothesis) is a statement about the expected outcome of a study (for example, a dissertation or thesis). To constitute a quality hypothesis, the statement needs to have three attributes – specificity , clarity and testability .
Let’s take a look at these more closely.
Need a helping hand?
Hypothesis Essential #1: Specificity & Clarity
A good research hypothesis needs to be extremely clear and articulate about both what’ s being assessed (who or what variables are involved ) and the expected outcome (for example, a difference between groups, a relationship between variables, etc.).
Let’s stick with our sleepy students example and look at how this statement could be more specific and clear.
Hypothesis: Students who sleep at least 8 hours per night will, on average, achieve higher grades in standardised tests than students who sleep less than 8 hours a night.
As you can see, the statement is very specific as it identifies the variables involved (sleep hours and test grades), the parties involved (two groups of students), as well as the predicted relationship type (a positive relationship). There’s no ambiguity or uncertainty about who or what is involved in the statement, and the expected outcome is clear.
Contrast that to the original hypothesis we looked at – “Sleep impacts academic performance” – and you can see the difference. “Sleep” and “academic performance” are both comparatively vague , and there’s no indication of what the expected relationship direction is (more sleep or less sleep). As you can see, specificity and clarity are key.
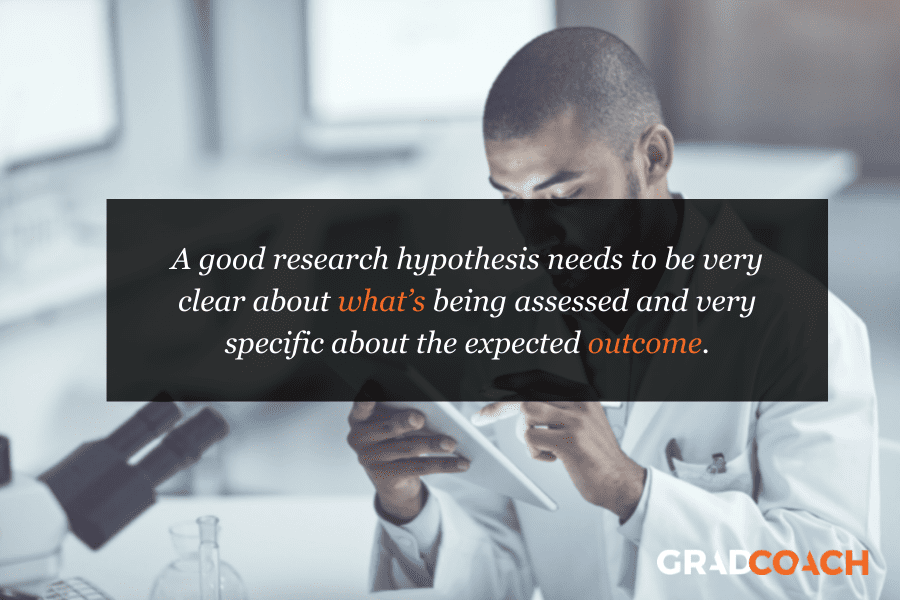
Hypothesis Essential #2: Testability (Provability)
A statement must be testable to qualify as a research hypothesis. In other words, there needs to be a way to prove (or disprove) the statement. If it’s not testable, it’s not a hypothesis – simple as that.
For example, consider the hypothesis we mentioned earlier:
Hypothesis: Students who sleep at least 8 hours per night will, on average, achieve higher grades in standardised tests than students who sleep less than 8 hours a night.
We could test this statement by undertaking a quantitative study involving two groups of students, one that gets 8 or more hours of sleep per night for a fixed period, and one that gets less. We could then compare the standardised test results for both groups to see if there’s a statistically significant difference.
Again, if you compare this to the original hypothesis we looked at – “Sleep impacts academic performance” – you can see that it would be quite difficult to test that statement, primarily because it isn’t specific enough. How much sleep? By who? What type of academic performance?
So, remember the mantra – if you can’t test it, it’s not a hypothesis 🙂
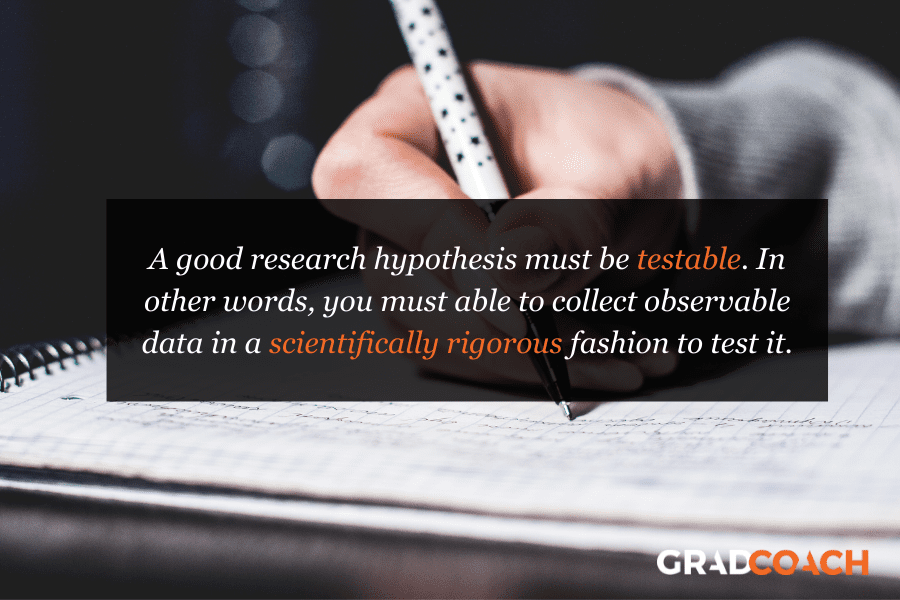
Defining A Research Hypothesis
You’re still with us? Great! Let’s recap and pin down a clear definition of a hypothesis.
A research hypothesis (or scientific hypothesis) is a statement about an expected relationship between variables, or explanation of an occurrence, that is clear, specific and testable.
So, when you write up hypotheses for your dissertation or thesis, make sure that they meet all these criteria. If you do, you’ll not only have rock-solid hypotheses but you’ll also ensure a clear focus for your entire research project.
What about the null hypothesis?
You may have also heard the terms null hypothesis , alternative hypothesis, or H-zero thrown around. At a simple level, the null hypothesis is the counter-proposal to the original hypothesis.
For example, if the hypothesis predicts that there is a relationship between two variables (for example, sleep and academic performance), the null hypothesis would predict that there is no relationship between those variables.
At a more technical level, the null hypothesis proposes that no statistical significance exists in a set of given observations and that any differences are due to chance alone.
And there you have it – hypotheses in a nutshell.
If you have any questions, be sure to leave a comment below and we’ll do our best to help you. If you need hands-on help developing and testing your hypotheses, consider our private coaching service , where we hold your hand through the research journey.
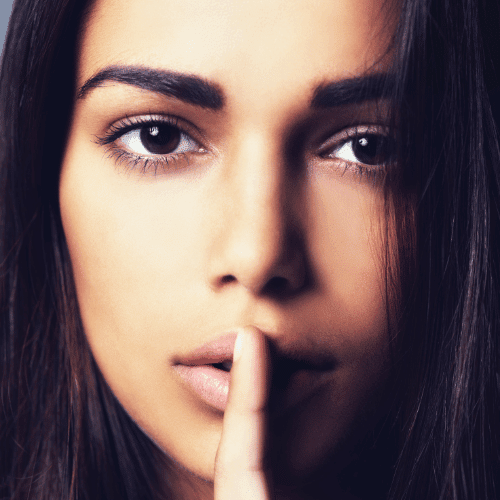
Psst... there’s more!
This post was based on one of our popular Research Bootcamps . If you're working on a research project, you'll definitely want to check this out ...
You Might Also Like:
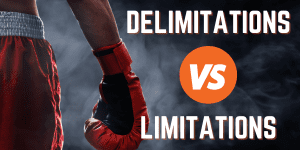
16 Comments
Very useful information. I benefit more from getting more information in this regard.
Very great insight,educative and informative. Please give meet deep critics on many research data of public international Law like human rights, environment, natural resources, law of the sea etc
In a book I read a distinction is made between null, research, and alternative hypothesis. As far as I understand, alternative and research hypotheses are the same. Can you please elaborate? Best Afshin
This is a self explanatory, easy going site. I will recommend this to my friends and colleagues.
Very good definition. How can I cite your definition in my thesis? Thank you. Is nul hypothesis compulsory in a research?
It’s a counter-proposal to be proven as a rejection
Please what is the difference between alternate hypothesis and research hypothesis?
It is a very good explanation. However, it limits hypotheses to statistically tasteable ideas. What about for qualitative researches or other researches that involve quantitative data that don’t need statistical tests?
In qualitative research, one typically uses propositions, not hypotheses.
could you please elaborate it more
I’ve benefited greatly from these notes, thank you.
This is very helpful
well articulated ideas are presented here, thank you for being reliable sources of information
Excellent. Thanks for being clear and sound about the research methodology and hypothesis (quantitative research)
I have only a simple question regarding the null hypothesis. – Is the null hypothesis (Ho) known as the reversible hypothesis of the alternative hypothesis (H1? – How to test it in academic research?
this is very important note help me much more
Trackbacks/Pingbacks
- What Is Research Methodology? Simple Definition (With Examples) - Grad Coach - […] Contrasted to this, a quantitative methodology is typically used when the research aims and objectives are confirmatory in nature. For example,…
Submit a Comment Cancel reply
Your email address will not be published. Required fields are marked *
Save my name, email, and website in this browser for the next time I comment.
- Print Friendly
- Skip to main content
- Skip to primary sidebar
- Skip to footer
- QuestionPro

- Solutions Industries Gaming Automotive Sports and events Education Government Travel & Hospitality Financial Services Healthcare Cannabis Technology Use Case NPS+ Communities Audience Contactless surveys Mobile LivePolls Member Experience GDPR Positive People Science 360 Feedback Surveys
- Resources Blog eBooks Survey Templates Case Studies Training Help center

Home Market Research
Research Hypothesis: What It Is, Types + How to Develop?
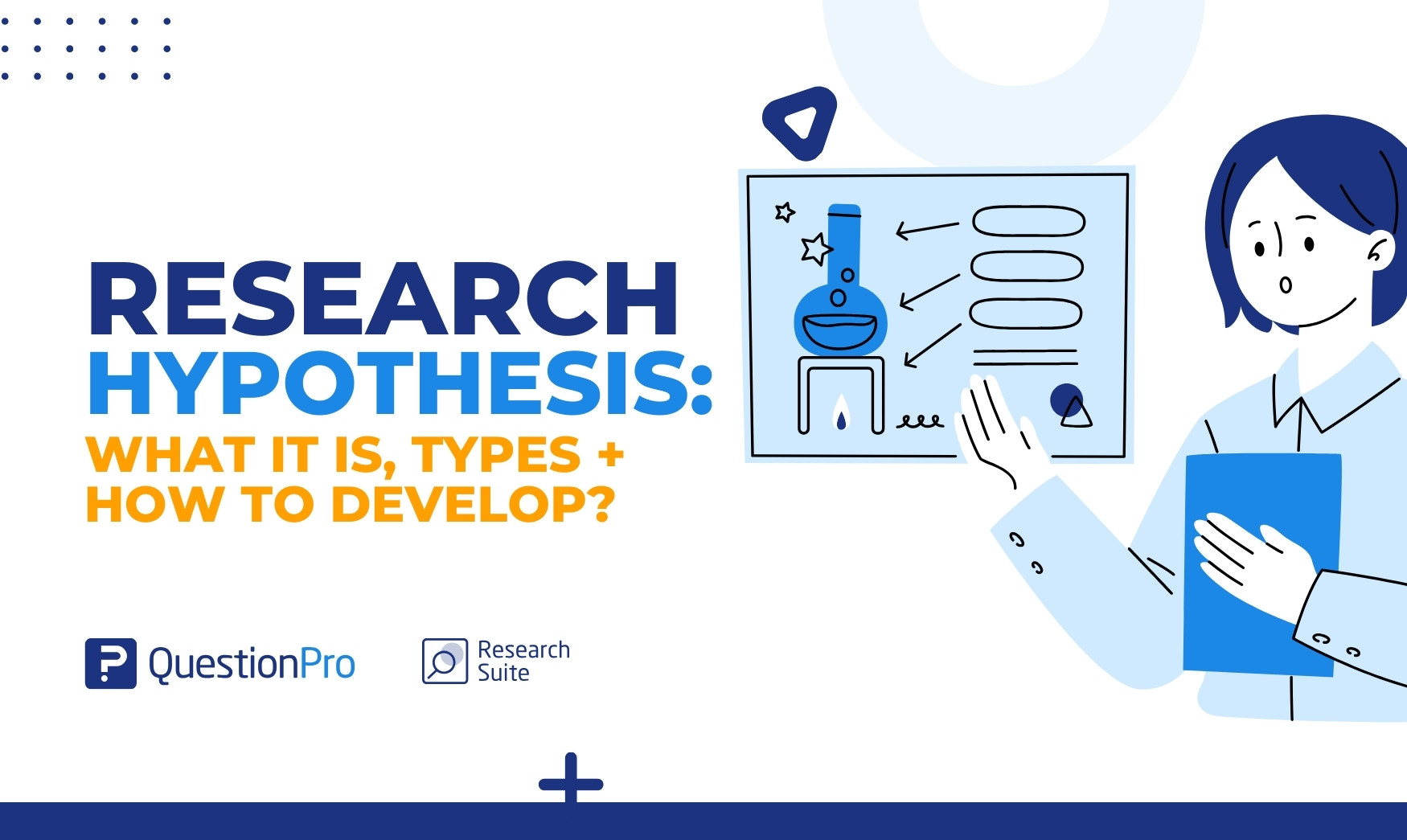
A research study starts with a question. Researchers worldwide ask questions and create research hypotheses. The effectiveness of research relies on developing a good research hypothesis. Examples of research hypotheses can guide researchers in writing effective ones.
In this blog, we’ll learn what a research hypothesis is, why it’s important in research, and the different types used in science. We’ll also guide you through creating your research hypothesis and discussing ways to test and evaluate it.
What is a Research Hypothesis?
A hypothesis is like a guess or idea that you suggest to check if it’s true. A research hypothesis is a statement that brings up a question and predicts what might happen.
It’s really important in the scientific method and is used in experiments to figure things out. Essentially, it’s an educated guess about how things are connected in the research.
A research hypothesis usually includes pointing out the independent variable (the thing they’re changing or studying) and the dependent variable (the result they’re measuring or watching). It helps plan how to gather and analyze data to see if there’s evidence to support or deny the expected connection between these variables.
Importance of Hypothesis in Research
Hypotheses are really important in research. They help design studies, allow for practical testing, and add to our scientific knowledge. Their main role is to organize research projects, making them purposeful, focused, and valuable to the scientific community. Let’s look at some key reasons why they matter:
- A research hypothesis helps test theories.
A hypothesis plays a pivotal role in the scientific method by providing a basis for testing existing theories. For example, a hypothesis might test the predictive power of a psychological theory on human behavior.
- It serves as a great platform for investigation activities.
It serves as a launching pad for investigation activities, which offers researchers a clear starting point. A research hypothesis can explore the relationship between exercise and stress reduction.
- Hypothesis guides the research work or study.
A well-formulated hypothesis guides the entire research process. It ensures that the study remains focused and purposeful. For instance, a hypothesis about the impact of social media on interpersonal relationships provides clear guidance for a study.
- Hypothesis sometimes suggests theories.
In some cases, a hypothesis can suggest new theories or modifications to existing ones. For example, a hypothesis testing the effectiveness of a new drug might prompt a reconsideration of current medical theories.
- It helps in knowing the data needs.
A hypothesis clarifies the data requirements for a study, ensuring that researchers collect the necessary information—a hypothesis guiding the collection of demographic data to analyze the influence of age on a particular phenomenon.
- The hypothesis explains social phenomena.
Hypotheses are instrumental in explaining complex social phenomena. For instance, a hypothesis might explore the relationship between economic factors and crime rates in a given community.
- Hypothesis provides a relationship between phenomena for empirical Testing.
Hypotheses establish clear relationships between phenomena, paving the way for empirical testing. An example could be a hypothesis exploring the correlation between sleep patterns and academic performance.
- It helps in knowing the most suitable analysis technique.
A hypothesis guides researchers in selecting the most appropriate analysis techniques for their data. For example, a hypothesis focusing on the effectiveness of a teaching method may lead to the choice of statistical analyses best suited for educational research.
Characteristics of a Good Research Hypothesis
A hypothesis is a specific idea that you can test in a study. It often comes from looking at past research and theories. A good hypothesis usually starts with a research question that you can explore through background research. For it to be effective, consider these key characteristics:
- Clear and Focused Language: A good hypothesis uses clear and focused language to avoid confusion and ensure everyone understands it.
- Related to the Research Topic: The hypothesis should directly relate to the research topic, acting as a bridge between the specific question and the broader study.
- Testable: An effective hypothesis can be tested, meaning its prediction can be checked with real data to support or challenge the proposed relationship.
- Potential for Exploration: A good hypothesis often comes from a research question that invites further exploration. Doing background research helps find gaps and potential areas to investigate.
- Includes Variables: The hypothesis should clearly state both the independent and dependent variables, specifying the factors being studied and the expected outcomes.
- Ethical Considerations: Check if variables can be manipulated without breaking ethical standards. It’s crucial to maintain ethical research practices.
- Predicts Outcomes: The hypothesis should predict the expected relationship and outcome, acting as a roadmap for the study and guiding data collection and analysis.
- Simple and Concise: A good hypothesis avoids unnecessary complexity and is simple and concise, expressing the essence of the proposed relationship clearly.
- Clear and Assumption-Free: The hypothesis should be clear and free from assumptions about the reader’s prior knowledge, ensuring universal understanding.
- Observable and Testable Results: A strong hypothesis implies research that produces observable and testable results, making sure the study’s outcomes can be effectively measured and analyzed.
When you use these characteristics as a checklist, it can help you create a good research hypothesis. It’ll guide improving and strengthening the hypothesis, identifying any weaknesses, and making necessary changes. Crafting a hypothesis with these features helps you conduct a thorough and insightful research study.
Types of Research Hypotheses
The research hypothesis comes in various types, each serving a specific purpose in guiding the scientific investigation. Knowing the differences will make it easier for you to create your own hypothesis. Here’s an overview of the common types:
01. Null Hypothesis
The null hypothesis states that there is no connection between two considered variables or that two groups are unrelated. As discussed earlier, a hypothesis is an unproven assumption lacking sufficient supporting data. It serves as the statement researchers aim to disprove. It is testable, verifiable, and can be rejected.
For example, if you’re studying the relationship between Project A and Project B, assuming both projects are of equal standard is your null hypothesis. It needs to be specific for your study.
02. Alternative Hypothesis
The alternative hypothesis is basically another option to the null hypothesis. It involves looking for a significant change or alternative that could lead you to reject the null hypothesis. It’s a different idea compared to the null hypothesis.
When you create a null hypothesis, you’re making an educated guess about whether something is true or if there’s a connection between that thing and another variable. If the null view suggests something is correct, the alternative hypothesis says it’s incorrect.
For instance, if your null hypothesis is “I’m going to be $1000 richer,” the alternative hypothesis would be “I’m not going to get $1000 or be richer.”
03. Directional Hypothesis
The directional hypothesis predicts the direction of the relationship between independent and dependent variables. They specify whether the effect will be positive or negative.
If you increase your study hours, you will experience a positive association with your exam scores. This hypothesis suggests that as you increase the independent variable (study hours), there will also be an increase in the dependent variable (exam scores).
04. Non-directional Hypothesis
The non-directional hypothesis predicts the existence of a relationship between variables but does not specify the direction of the effect. It suggests that there will be a significant difference or relationship, but it does not predict the nature of that difference.
For example, you will find no notable difference in test scores between students who receive the educational intervention and those who do not. However, once you compare the test scores of the two groups, you will notice an important difference.
05. Simple Hypothesis
A simple hypothesis predicts a relationship between one dependent variable and one independent variable without specifying the nature of that relationship. It’s simple and usually used when we don’t know much about how the two things are connected.
For example, if you adopt effective study habits, you will achieve higher exam scores than those with poor study habits.
06. Complex Hypothesis
A complex hypothesis is an idea that specifies a relationship between multiple independent and dependent variables. It is a more detailed idea than a simple hypothesis.
While a simple view suggests a straightforward cause-and-effect relationship between two things, a complex hypothesis involves many factors and how they’re connected to each other.
For example, when you increase your study time, you tend to achieve higher exam scores. The connection between your study time and exam performance is affected by various factors, including the quality of your sleep, your motivation levels, and the effectiveness of your study techniques.
If you sleep well, stay highly motivated, and use effective study strategies, you may observe a more robust positive correlation between the time you spend studying and your exam scores, unlike those who may lack these factors.
07. Associative Hypothesis
An associative hypothesis proposes a connection between two things without saying that one causes the other. Basically, it suggests that when one thing changes, the other changes too, but it doesn’t claim that one thing is causing the change in the other.
For example, you will likely notice higher exam scores when you increase your study time. You can recognize an association between your study time and exam scores in this scenario.
Your hypothesis acknowledges a relationship between the two variables—your study time and exam scores—without asserting that increased study time directly causes higher exam scores. You need to consider that other factors, like motivation or learning style, could affect the observed association.
08. Causal Hypothesis
A causal hypothesis proposes a cause-and-effect relationship between two variables. It suggests that changes in one variable directly cause changes in another variable.
For example, when you increase your study time, you experience higher exam scores. This hypothesis suggests a direct cause-and-effect relationship, indicating that the more time you spend studying, the higher your exam scores. It assumes that changes in your study time directly influence changes in your exam performance.
09. Empirical Hypothesis
An empirical hypothesis is a statement based on things we can see and measure. It comes from direct observation or experiments and can be tested with real-world evidence. If an experiment proves a theory, it supports the idea and shows it’s not just a guess. This makes the statement more reliable than a wild guess.
For example, if you increase the dosage of a certain medication, you might observe a quicker recovery time for patients. Imagine you’re in charge of a clinical trial. In this trial, patients are given varying dosages of the medication, and you measure and compare their recovery times. This allows you to directly see the effects of different dosages on how fast patients recover.
This way, you can create a research hypothesis: “Increasing the dosage of a certain medication will lead to a faster recovery time for patients.”
10. Statistical Hypothesis
A statistical hypothesis is a statement or assumption about a population parameter that is the subject of an investigation. It serves as the basis for statistical analysis and testing. It is often tested using statistical methods to draw inferences about the larger population.
In a hypothesis test, statistical evidence is collected to either reject the null hypothesis in favor of the alternative hypothesis or fail to reject the null hypothesis due to insufficient evidence.
For example, let’s say you’re testing a new medicine. Your hypothesis could be that the medicine doesn’t really help patients get better. So, you collect data and use statistics to see if your guess is right or if the medicine actually makes a difference.
If the data strongly shows that the medicine does help, you say your guess was wrong, and the medicine does make a difference. But if the proof isn’t strong enough, you can stick with your original guess because you didn’t get enough evidence to change your mind.
How to Develop a Research Hypotheses?
Step 1: identify your research problem or topic..
Define the area of interest or the problem you want to investigate. Make sure it’s clear and well-defined.
Start by asking a question about your chosen topic. Consider the limitations of your research and create a straightforward problem related to your topic. Once you’ve done that, you can develop and test a hypothesis with evidence.
Step 2: Conduct a literature review
Review existing literature related to your research problem. This will help you understand the current state of knowledge in the field, identify gaps, and build a foundation for your hypothesis. Consider the following questions:
- What existing research has been conducted on your chosen topic?
- Are there any gaps or unanswered questions in the current literature?
- How will the existing literature contribute to the foundation of your research?
Step 3: Formulate your research question
Based on your literature review, create a specific and concise research question that addresses your identified problem. Your research question should be clear, focused, and relevant to your field of study.
Step 4: Identify variables
Determine the key variables involved in your research question. Variables are the factors or phenomena that you will study and manipulate to test your hypothesis.
- Independent Variable: The variable you manipulate or control.
- Dependent Variable: The variable you measure to observe the effect of the independent variable.
Step 5: State the Null hypothesis
The null hypothesis is a statement that there is no significant difference or effect. It serves as a baseline for comparison with the alternative hypothesis.
Step 6: Select appropriate methods for testing the hypothesis
Choose research methods that align with your study objectives, such as experiments, surveys, or observational studies. The selected methods enable you to test your research hypothesis effectively.
Creating a research hypothesis usually takes more than one try. Expect to make changes as you collect data. It’s normal to test and say no to a few hypotheses before you find the right answer to your research question.
Testing and Evaluating Hypotheses
Testing hypotheses is a really important part of research. It’s like the practical side of things. Here, real-world evidence will help you determine how different things are connected. Let’s explore the main steps in hypothesis testing:
- State your research hypothesis.
Before testing, clearly articulate your research hypothesis. This involves framing both a null hypothesis, suggesting no significant effect or relationship, and an alternative hypothesis, proposing the expected outcome.
- Collect data strategically.
Plan how you will gather information in a way that fits your study. Make sure your data collection method matches the things you’re studying.
Whether through surveys, observations, or experiments, this step demands precision and adherence to the established methodology. The quality of data collected directly influences the credibility of study outcomes.
- Perform an appropriate statistical test.
Choose a statistical test that aligns with the nature of your data and the hypotheses being tested. Whether it’s a t-test, chi-square test, ANOVA, or regression analysis, selecting the right statistical tool is paramount for accurate and reliable results.
- Decide if your idea was right or wrong.
Following the statistical analysis, evaluate the results in the context of your null hypothesis. You need to decide if you should reject your null hypothesis or not.
- Share what you found.
When discussing what you found in your research, be clear and organized. Say whether your idea was supported or not, and talk about what your results mean. Also, mention any limits to your study and suggest ideas for future research.
The Role of QuestionPro to Develop a Good Research Hypothesis
QuestionPro is a survey and research platform that provides tools for creating, distributing, and analyzing surveys. It plays a crucial role in the research process, especially when you’re in the initial stages of hypothesis development. Here’s how QuestionPro can help you to develop a good research hypothesis:
- Survey design and data collection: You can use the platform to create targeted questions that help you gather relevant data.
- Exploratory research: Through surveys and feedback mechanisms on QuestionPro, you can conduct exploratory research to understand the landscape of a particular subject.
- Literature review and background research: QuestionPro surveys can collect sample population opinions, experiences, and preferences. This data and a thorough literature evaluation can help you generate a well-grounded hypothesis by improving your research knowledge.
- Identifying variables: Using targeted survey questions, you can identify relevant variables related to their research topic.
- Testing assumptions: You can use surveys to informally test certain assumptions or hypotheses before formalizing a research hypothesis.
- Data analysis tools: QuestionPro provides tools for analyzing survey data. You can use these tools to identify the collected data’s patterns, correlations, or trends.
- Refining your hypotheses: As you collect data through QuestionPro, you can adjust your hypotheses based on the real-world responses you receive.
A research hypothesis is like a guide for researchers in science. It’s a well-thought-out idea that has been thoroughly tested. This idea is crucial as researchers can explore different fields, such as medicine, social sciences, and natural sciences. The research hypothesis links theories to real-world evidence and gives researchers a clear path to explore and make discoveries.
QuestionPro Research Suite is a helpful tool for researchers. It makes creating surveys, collecting data, and analyzing information easily. It supports all kinds of research, from exploring new ideas to forming hypotheses. With a focus on using data, it helps researchers do their best work.
Are you interested in learning more about QuestionPro Research Suite? Take advantage of QuestionPro’s free trial to get an initial look at its capabilities and realize the full potential of your research efforts.
LEARN MORE FREE TRIAL
MORE LIKE THIS
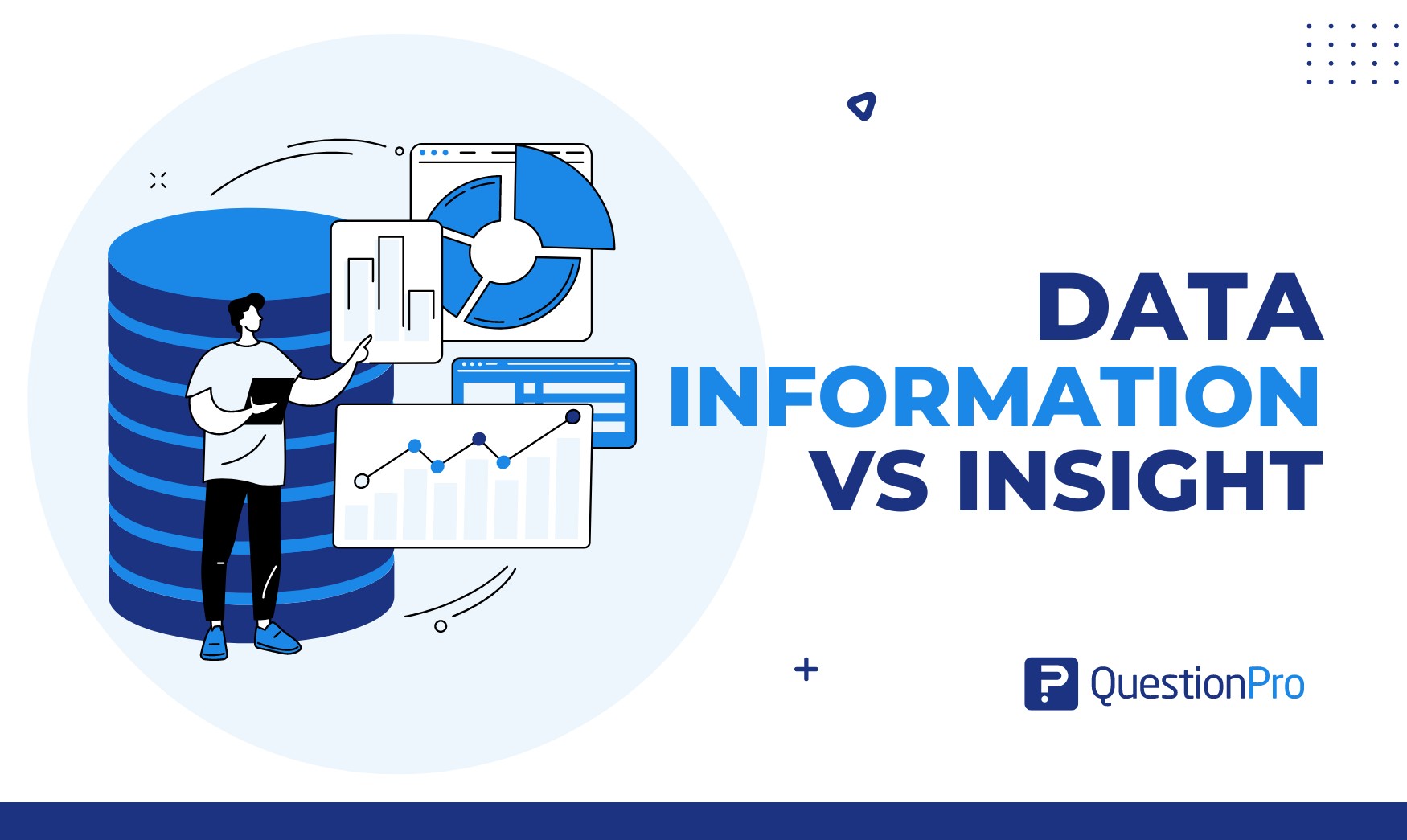
Data Information vs Insight: Essential differences
May 14, 2024
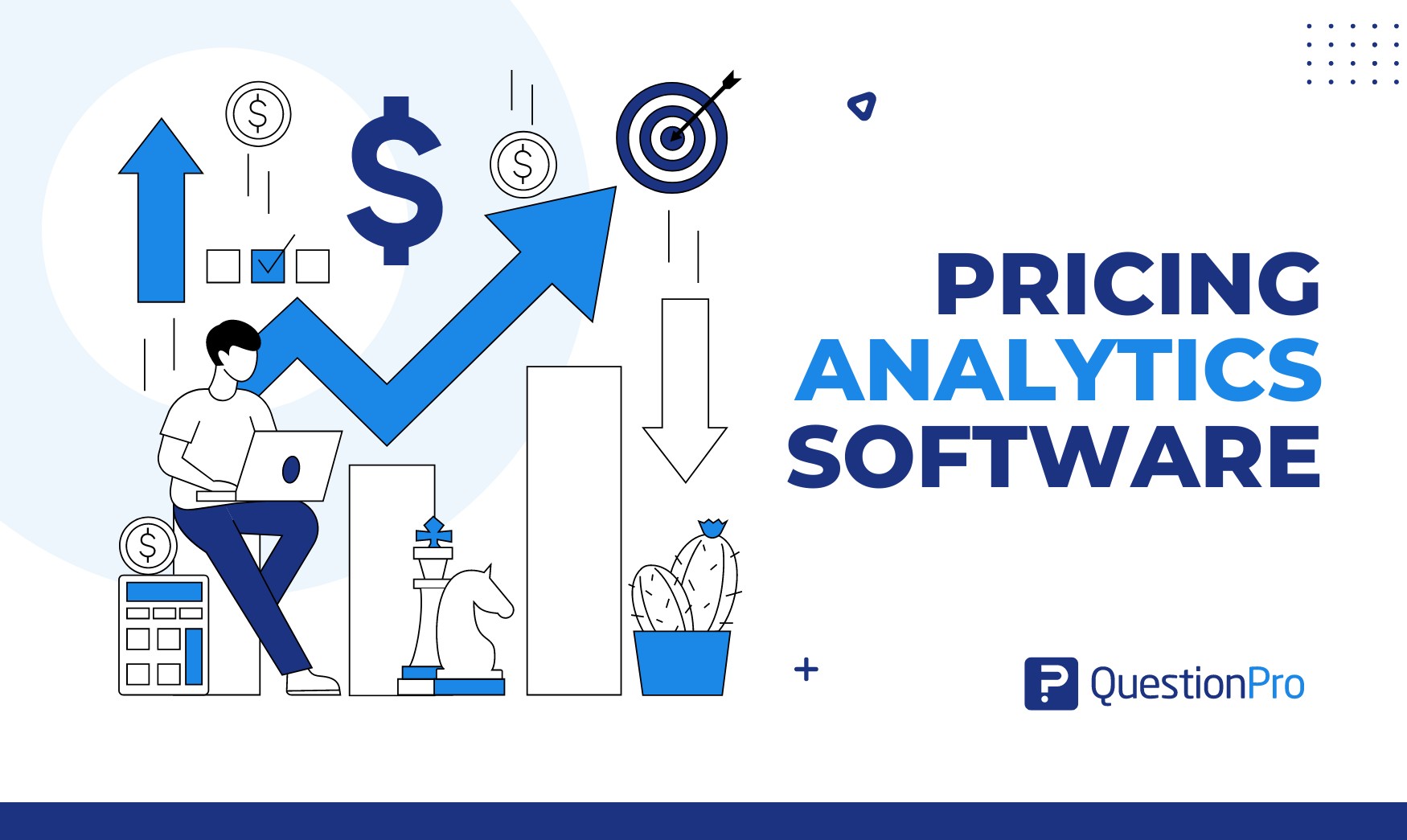
Pricing Analytics Software: Optimize Your Pricing Strategy
May 13, 2024
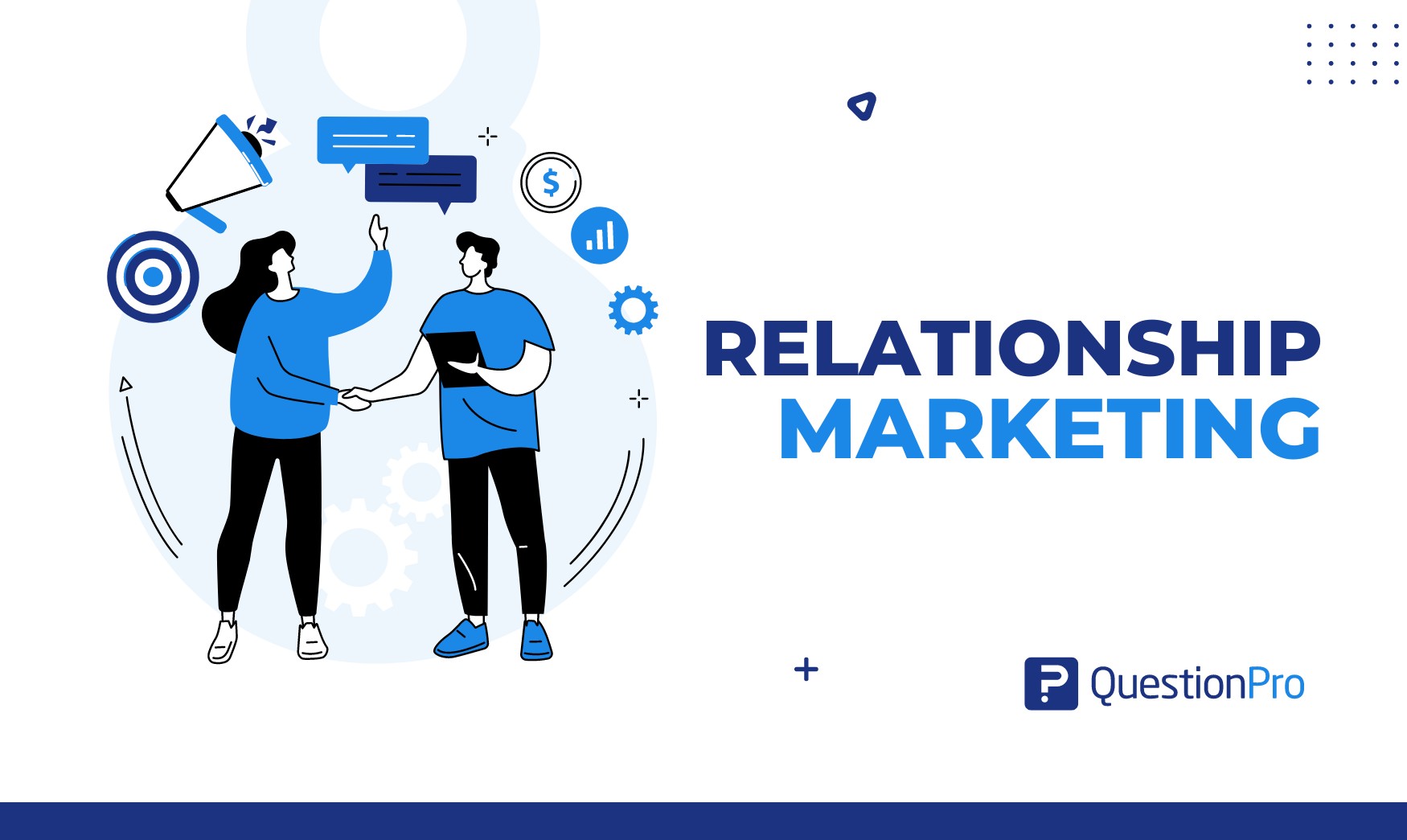
Relationship Marketing: What It Is, Examples & Top 7 Benefits
May 8, 2024
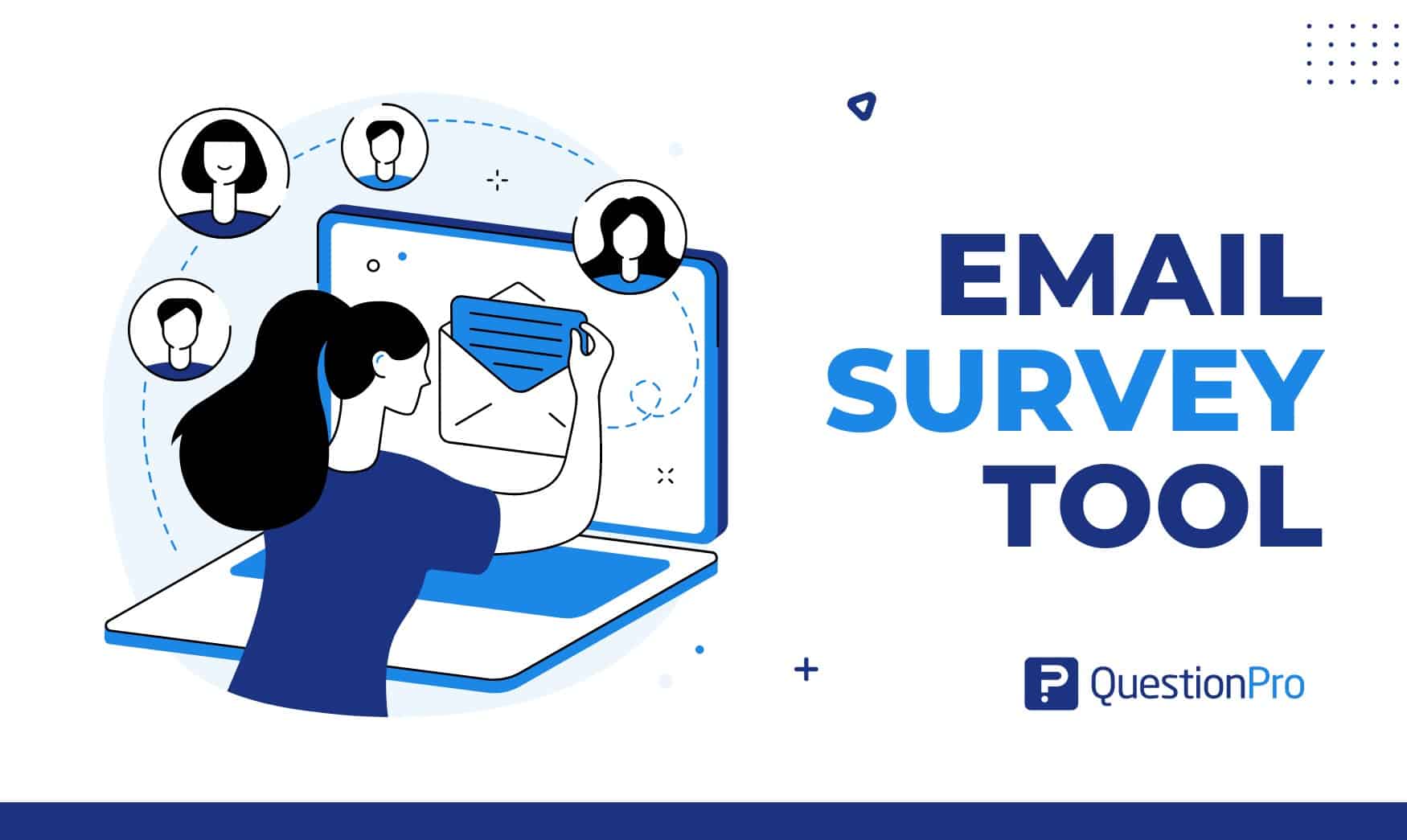
The Best Email Survey Tool to Boost Your Feedback Game
May 7, 2024
Other categories
- Academic Research
- Artificial Intelligence
- Assessments
- Brand Awareness
- Case Studies
- Communities
- Consumer Insights
- Customer effort score
- Customer Engagement
- Customer Experience
- Customer Loyalty
- Customer Research
- Customer Satisfaction
- Employee Benefits
- Employee Engagement
- Employee Retention
- Friday Five
- General Data Protection Regulation
- Insights Hub
- Life@QuestionPro
- Market Research
- Mobile diaries
- Mobile Surveys
- New Features
- Online Communities
- Question Types
- Questionnaire
- QuestionPro Products
- Release Notes
- Research Tools and Apps
- Revenue at Risk
- Survey Templates
- Training Tips
- Uncategorized
- Video Learning Series
- What’s Coming Up
- Workforce Intelligence
How Do You Formulate (Important) Hypotheses?
- Open Access
- First Online: 03 December 2022
Cite this chapter
You have full access to this open access chapter
- James Hiebert 6 ,
- Jinfa Cai 7 ,
- Stephen Hwang 7 ,
- Anne K Morris 6 &
- Charles Hohensee 6
Part of the book series: Research in Mathematics Education ((RME))
11k Accesses
Building on the ideas in Chap. 1, we describe formulating, testing, and revising hypotheses as a continuing cycle of clarifying what you want to study, making predictions about what you might find together with developing your reasons for these predictions, imagining tests of these predictions, revising your predictions and rationales, and so on. Many resources feed this process, including reading what others have found about similar phenomena, talking with colleagues, conducting pilot studies, and writing drafts as you revise your thinking. Although you might think you cannot predict what you will find, it is always possible—with enough reading and conversations and pilot studies—to make some good guesses. And, once you guess what you will find and write out the reasons for these guesses you are on your way to scientific inquiry. As you refine your hypotheses, you can assess their research importance by asking how connected they are to problems your research community really wants to solve.
You have full access to this open access chapter, Download chapter PDF
Part I. Getting Started
We want to begin by addressing a question you might have had as you read the title of this chapter. You are likely to hear, or read in other sources, that the research process begins by asking research questions . For reasons we gave in Chap. 1 , and more we will describe in this and later chapters, we emphasize formulating, testing, and revising hypotheses. However, it is important to know that asking and answering research questions involve many of the same activities, so we are not describing a completely different process.
We acknowledge that many researchers do not actually begin by formulating hypotheses. In other words, researchers rarely get a researchable idea by writing out a well-formulated hypothesis. Instead, their initial ideas for what they study come from a variety of sources. Then, after they have the idea for a study, they do lots of background reading and thinking and talking before they are ready to formulate a hypothesis. So, for readers who are at the very beginning and do not yet have an idea for a study, let’s back up. Where do research ideas come from?
There are no formulas or algorithms that spawn a researchable idea. But as you begin the process, you can ask yourself some questions. Your answers to these questions can help you move forward.
What are you curious about? What are you passionate about? What have you wondered about as an educator? These are questions that look inward, questions about yourself.
What do you think are the most pressing educational problems? Which problems are you in the best position to address? What change(s) do you think would help all students learn more productively? These are questions that look outward, questions about phenomena you have observed.
What are the main areas of research in the field? What are the big questions that are being asked? These are questions about the general landscape of the field.
What have you read about in the research literature that caught your attention? What have you read that prompted you to think about extending the profession’s knowledge about this? What have you read that made you ask, “I wonder why this is true?” These are questions about how you can build on what is known in the field.
What are some research questions or testable hypotheses that have been identified by other researchers for future research? This, too, is a question about how you can build on what is known in the field. Taking up such questions or hypotheses can help by providing some existing scaffolding that others have constructed.
What research is being done by your immediate colleagues or your advisor that is of interest to you? These are questions about topics for which you will likely receive local support.
Exercise 2.1
Brainstorm some answers for each set of questions. Record them. Then step back and look at the places of intersection. Did you have similar answers across several questions? Write out, as clearly as you can, the topic that captures your primary interest, at least at this point. We will give you a chance to update your responses as you study this book.
Part II. Paths from a General Interest to an Informed Hypothesis
There are many different paths you might take from conceiving an idea for a study, maybe even a vague idea, to formulating a prediction that leads to an informed hypothesis that can be tested. We will explore some of the paths we recommend.
We will assume you have completed Exercise 2.1 in Part I and have some written answers to the six questions that preceded it as well as a statement that describes your topic of interest. This very first statement could take several different forms: a description of a problem you want to study, a question you want to address, or a hypothesis you want to test. We recommend that you begin with one of these three forms, the one that makes most sense to you. There is an advantage to using all three and flexibly choosing the one that is most meaningful at the time and for a particular study. You can then move from one to the other as you think more about your research study and you develop your initial idea. To get a sense of how the process might unfold, consider the following alternative paths.
Beginning with a Prediction If You Have One
Sometimes, when you notice an educational problem or have a question about an educational situation or phenomenon, you quickly have an idea that might help solve the problem or answer the question. Here are three examples.
You are a teacher, and you noticed a problem with the way the textbook presented two related concepts in two consecutive lessons. Almost as soon as you noticed the problem, it occurred to you that the two lessons could be taught more effectively in the reverse order. You predicted better outcomes if the order was reversed, and you even had a preliminary rationale for why this would be true.
You are a graduate student and you read that students often misunderstand a particular aspect of graphing linear functions. You predicted that, by listening to small groups of students working together, you could hear new details that would help you understand this misconception.
You are a curriculum supervisor and you observed sixth-grade classrooms where students were learning about decimal fractions. After talking with several experienced teachers, you predicted that beginning with percentages might be a good way to introduce students to decimal fractions.
We begin with the path of making predictions because we see the other two paths as leading into this one at some point in the process (see Fig. 2.1 ). Starting with this path does not mean you did not sense a problem you wanted to solve or a question you wanted to answer.
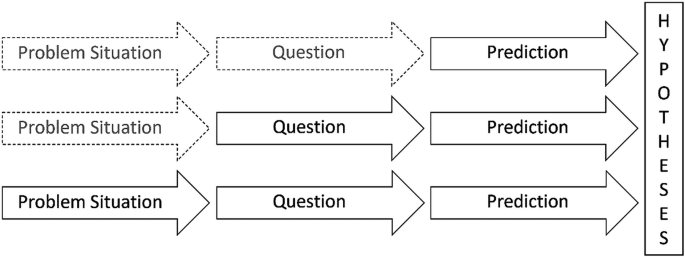
Three Pathways to Formulating Informed Hypotheses
Notice that your predictions can come from a variety of sources—your own experience, reading, and talking with colleagues. Most likely, as you write out your predictions you also think about the educational problem for which your prediction is a potential solution. Writing a clear description of the problem will be useful as you proceed. Notice also that it is easy to change each of your predictions into a question. When you formulate a prediction, you are actually answering a question, even though the question might be implicit. Making that implicit question explicit can generate a first draft of the research question that accompanies your prediction. For example, suppose you are the curriculum supervisor who predicts that teaching percentages first would be a good way to introduce decimal fractions. In an obvious shift in form, you could ask, “In what ways would teaching percentages benefit students’ initial learning of decimal fractions?”
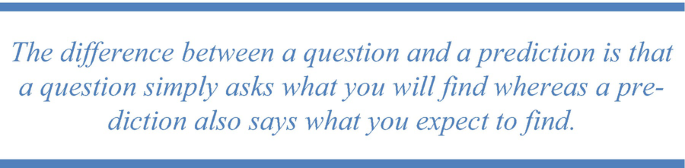
There are advantages to starting with the prediction form if you can make an educated guess about what you will find. Making a prediction forces you to think now about several things you will need to think about at some point anyway. It is better to think about them earlier rather than later. If you state your prediction clearly and explicitly, you can begin to ask yourself three questions about your prediction: Why do I expect to observe what I am predicting? Why did I make that prediction? (These two questions essentially ask what your rationale is for your prediction.) And, how can I test to see if it’s right? This is where the benefits of making predictions begin.
Asking yourself why you predicted what you did, and then asking yourself why you answered the first “why” question as you did, can be a powerful chain of thought that lays the groundwork for an increasingly accurate prediction and an increasingly well-reasoned rationale. For example, suppose you are the curriculum supervisor above who predicted that beginning by teaching percentages would be a good way to introduce students to decimal fractions. Why did you make this prediction? Maybe because students are familiar with percentages in everyday life so they could use what they know to anchor their thinking about hundredths. Why would that be helpful? Because if students could connect hundredths in percentage form with hundredths in decimal fraction form, they could bring their meaning of percentages into decimal fractions. But how would that help? If students understood that a decimal fraction like 0.35 meant 35 of 100, then they could use their understanding of hundredths to explore the meaning of tenths, thousandths, and so on. Why would that be useful? By continuing to ask yourself why you gave the previous answer, you can begin building your rationale and, as you build your rationale, you will find yourself revisiting your prediction, often making it more precise and explicit. If you were the curriculum supervisor and continued the reasoning in the previous sentences, you might elaborate your prediction by specifying the way in which percentages should be taught in order to have a positive effect on particular aspects of students’ understanding of decimal fractions.
Developing a Rationale for Your Predictions
Keeping your initial predictions in mind, you can read what others already know about the phenomenon. Your reading can now become targeted with a clear purpose.
By reading and talking with colleagues, you can develop more complete reasons for your predictions. It is likely that you will also decide to revise your predictions based on what you learn from your reading. As you develop sound reasons for your predictions, you are creating your rationales, and your predictions together with your rationales become your hypotheses. The more you learn about what is already known about your research topic, the more refined will be your predictions and the clearer and more complete your rationales. We will use the term more informed hypotheses to describe this evolution of your hypotheses.
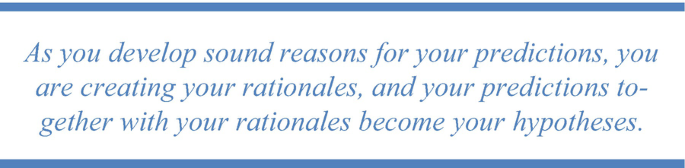
Developing more informed hypotheses is a good thing because it means: (1) you understand the reasons for your predictions; (2) you will be able to imagine how you can test your hypotheses; (3) you can more easily convince your colleagues that they are important hypotheses—they are hypotheses worth testing; and (4) at the end of your study, you will be able to more easily interpret the results of your test and to revise your hypotheses to demonstrate what you have learned by conducting the study.
Imagining Testing Your Hypotheses
Because we have tied together predictions and rationales to constitute hypotheses, testing hypotheses means testing predictions and rationales. Testing predictions means comparing empirical observations, or findings, with the predictions. Testing rationales means using these comparisons to evaluate the adequacy or soundness of the rationales.
Imagining how you might test your hypotheses does not mean working out the details for exactly how you would test them. Rather, it means thinking ahead about how you could do this. Recall the descriptor of scientific inquiry: “experience carefully planned in advance” (Fisher, 1935). Asking whether predictions are testable and whether rationales can be evaluated is simply planning in advance.
You might read that testing hypotheses means simply assessing whether predictions are correct or incorrect. In our view, it is more useful to think of testing as a means of gathering enough information to compare your findings with your predictions, revise your rationales, and propose more accurate predictions. So, asking yourself whether hypotheses can be tested means asking whether information could be collected to assess the accuracy of your predictions and whether the information will show you how to revise your rationales to sharpen your predictions.
Cycles of Building Rationales and Planning to Test Your Predictions
Scientific reasoning is a dialogue between the possible and the actual, an interplay between hypotheses and the logical expectations they give rise to: there is a restless to-and-fro motion of thought, the formulation and rectification of hypotheses (Medawar, 1982 , p.72).
As you ask yourself about how you could test your predictions, you will inevitably revise your rationales and sharpen your predictions. Your hypotheses will become more informed, more targeted, and more explicit. They will make clearer to you and others what, exactly, you plan to study.
When will you know that your hypotheses are clear and precise enough? Because of the way we define hypotheses, this question asks about both rationales and predictions. If a rationale you are building lets you make a number of quite different predictions that are equally plausible rather than a single, primary prediction, then your hypothesis needs further refinement by building a more complete and precise rationale. Also, if you cannot briefly describe to your colleagues a believable way to test your prediction, then you need to phrase it more clearly and precisely.
Each time you strengthen your rationales, you might need to adjust your predictions. And, each time you clarify your predictions, you might need to adjust your rationales. The cycle of going back and forth to keep your predictions and rationales tightly aligned has many payoffs down the road. Every decision you make from this point on will be in the interests of providing a transparent and convincing test of your hypotheses and explaining how the results of your test dictate specific revisions to your hypotheses. As you make these decisions (described in the succeeding chapters), you will probably return to clarify your hypotheses even further. But, you will be in a much better position, at each point, if you begin with well-informed hypotheses.
Beginning by Asking Questions to Clarify Your Interests
Instead of starting with predictions, a second path you might take devotes more time at the beginning to asking questions as you zero in on what you want to study. Some researchers suggest you start this way (e.g., Gournelos et al., 2019 ). Specifically, with this second path, the first statement you write to express your research interest would be a question. For example, you might ask, “Why do ninth-grade students change the way they think about linear equations after studying quadratic equations?” or “How do first graders solve simple arithmetic problems before they have been taught to add and subtract?”
The first phrasing of your question might be quite general or vague. As you think about your question and what you really want to know, you are likely to ask follow-up questions. These questions will almost always be more specific than your first question. The questions will also express more clearly what you want to know. So, the question “How do first graders solve simple arithmetic problems before they have been taught to add and subtract” might evolve into “Before first graders have been taught to solve arithmetic problems, what strategies do they use to solve arithmetic problems with sums and products below 20?” As you read and learn about what others already know about your questions, you will continually revise your questions toward clearer and more explicit and more precise versions that zero in on what you really want to know. The question above might become, “Before they are taught to solve arithmetic problems, what strategies do beginning first graders use to solve arithmetic problems with sums and products below 20 if they are read story problems and given physical counters to help them keep track of the quantities?”
Imagining Answers to Your Questions
If you monitor your own thinking as you ask questions, you are likely to begin forming some guesses about answers, even to the early versions of the questions. What do students learn about quadratic functions that influences changes in their proportional reasoning when dealing with linear functions? It could be that if you analyze the moments during instruction on quadratic equations that are extensions of the proportional reasoning involved in solving linear equations, there are times when students receive further experience reasoning proportionally. You might predict that these are the experiences that have a “backward transfer” effect (Hohensee, 2014 ).
These initial guesses about answers to your questions are your first predictions. The first predicted answers are likely to be hunches or fuzzy, vague guesses. This simply means you do not know very much yet about the question you are asking. Your first predictions, no matter how unfocused or tentative, represent the most you know at the time about the question you are asking. They help you gauge where you are in your thinking.
Shifting to the Hypothesis Formulation and Testing Path
Research questions can play an important role in the research process. They provide a succinct way of capturing your research interests and communicating them to others. When colleagues want to know about your work, they will often ask “What are your research questions?” It is good to have a ready answer.
However, research questions have limitations. They do not capture the three images of scientific inquiry presented in Chap. 1 . Due, in part, to this less expansive depiction of the process, research questions do not take you very far. They do not provide a guide that leads you through the phases of conducting a study.
Consequently, when you can imagine an answer to your research question, we recommend that you move onto the hypothesis formulation and testing path. Imagining an answer to your question means you can make plausible predictions. You can now begin clarifying the reasons for your predictions and transform your early predictions into hypotheses (predictions along with rationales). We recommend you do this as soon as you have guesses about the answers to your questions because formulating, testing, and revising hypotheses offers a tool that puts you squarely on the path of scientific inquiry. It is a tool that can guide you through the entire process of conducting a research study.
This does not mean you are finished asking questions. Predictions are often created as answers to questions. So, we encourage you to continue asking questions to clarify what you want to know. But your target shifts from only asking questions to also proposing predictions for the answers and developing reasons the answers will be accurate predictions. It is by predicting answers, and explaining why you made those predictions, that you become engaged in scientific inquiry.
Cycles of Refining Questions and Predicting Answers
An example might provide a sense of how this process plays out. Suppose you are reading about Vygotsky’s ( 1987 ) zone of proximal development (ZPD), and you realize this concept might help you understand why your high school students had trouble learning exponential functions. Maybe they were outside this zone when you tried to teach exponential functions. In order to recognize students who would benefit from instruction, you might ask, “How can I identify students who are within the ZPD around exponential functions?” What would you predict? Maybe students in this ZPD are those who already had knowledge of related functions. You could write out some reasons for this prediction, like “students who understand linear and quadratic functions are more likely to extend their knowledge to exponential functions.” But what kind of data would you need to test this? What would count as “understanding”? Are linear and quadratic the functions you should assess? Even if they are, how could you tell whether students who scored well on tests of linear and quadratic functions were within the ZPD of exponential functions? How, in the end, would you measure what it means to be in this ZPD? So, asking a series of reasonable questions raised some red flags about the way your initial question was phrased, and you decide to revise it.
You set the stage for revising your question by defining ZPD as the zone within which students can solve an exponential function problem by making only one additional conceptual connection between what they already know and exponential functions. Your revised question is, “Based on students’ knowledge of linear and quadratic functions, which students are within the ZPD of exponential functions?” This time you know what kind of data you need: the number of conceptual connections students need to bridge from their knowledge of related functions to exponential functions. How can you collect these data? Would you need to see into the minds of the students? Or, are there ways to test the number of conceptual connections someone makes to move from one topic to another? Do methods exist for gathering these data? You decide this is not realistic, so you now have a choice: revise the question further or move your research in a different direction.
Notice that we do not use the term research question for all these early versions of questions that begin clarifying for yourself what you want to study. These early versions are too vague and general to be called research questions. In this book, we save the term research question for a question that comes near the end of the work and captures exactly what you want to study . By the time you are ready to specify a research question, you will be thinking about your study in terms of hypotheses and tests. When your hypotheses are in final form and include clear predictions about what you will find, it will be easy to state the research questions that accompany your predictions.
To reiterate one of the key points of this chapter: hypotheses carry much more information than research questions. Using our definition, hypotheses include predictions about what the answer might be to the question plus reasons for why you think so. Unlike research questions, hypotheses capture all three images of scientific inquiry presented in Chap. 1 (planning, observing and explaining, and revising one’s thinking). Your hypotheses represent the most you know, at the moment, about your research topic. The same cannot be said for research questions.
Beginning with a Research Problem
When you wrote answers to the six questions at the end of Part I of this chapter, you might have identified a research interest by stating it as a problem. This is the third path you might take to begin your research. Perhaps your description of your problem might look something like this: “When I tried to teach my middle school students by presenting them with a challenging problem without showing them how to solve similar problems, they didn’t exert much effort trying to find a solution but instead waited for me to show them how to solve the problem.” You do not have a specific question in mind, and you do not have an idea for why the problem exists, so you do not have a prediction about how to solve it. Writing a statement of this problem as clearly as possible could be the first step in your research journey.
As you think more about this problem, it will feel natural to ask questions about it. For example, why did some students show more initiative than others? What could I have done to get them started? How could I have encouraged the students to keep trying without giving away the solution? You are now on the path of asking questions—not research questions yet, but questions that are helping you focus your interest.
As you continue to think about these questions, reflect on your own experience, and read what others know about this problem, you will likely develop some guesses about the answers to the questions. They might be somewhat vague answers, and you might not have lots of confidence they are correct, but they are guesses that you can turn into predictions. Now you are on the hypothesis-formulation-and-testing path. This means you are on the path of asking yourself why you believe the predictions are correct, developing rationales for the predictions, asking what kinds of empirical observations would test your predictions, and refining your rationales and predictions as you read the literature and talk with colleagues.
A simple diagram that summarizes the three paths we have described is shown in Fig. 2.1 . Each row of arrows represents one pathway for formulating an informed hypothesis. The dotted arrows in the first two rows represent parts of the pathways that a researcher may have implicitly travelled through already (without an intent to form a prediction) but that ultimately inform the researcher’s development of a question or prediction.
Part III. One Researcher’s Experience Launching a Scientific Inquiry
Martha was in her third year of her doctoral program and beginning to identify a topic for her dissertation. Based on (a) her experience as a high school mathematics teacher and a curriculum supervisor, (b) the reading she has done to this point, and (c) her conversations with her colleagues, she has developed an interest in what kinds of professional development experiences (let’s call them learning opportunities [LOs] for teachers) are most effective. Where does she go from here?
Exercise 2.2
Before you continue reading, please write down some suggestions for Martha about where she should start.
A natural thing for Martha to do at this point is to ask herself some additional questions, questions that specify further what she wants to learn: What kinds of LOs do most teachers experience? How do these experiences change teachers’ practices and beliefs? Are some LOs more effective than others? What makes them more effective?
To focus her questions and decide what she really wants to know, she continues reading but now targets her reading toward everything she can find that suggests possible answers to these questions. She also talks with her colleagues to get more ideas about possible answers to these or related questions. Over several weeks or months, she finds herself being drawn to questions about what makes LOs effective, especially for helping teachers teach more conceptually. She zeroes in on the question, “What makes LOs for teachers effective for improving their teaching for conceptual understanding?”
This question is more focused than her first questions, but it is still too general for Martha to define a research study. How does she know it is too general? She uses two criteria. First, she notices that the predictions she makes about the answers to the question are all over the place; they are not constrained by the reasons she has assembled for her predictions. One prediction is that LOs are more effective when they help teachers learn content. Martha makes this guess because previous research suggests that effective LOs for teachers include attention to content. But this rationale allows lots of different predictions. For example, LOs are more effective when they focus on the content teachers will teach; LOs are more effective when they focus on content beyond what teachers will teach so teachers see how their instruction fits with what their students will encounter later; and LOs are more effective when they are tailored to the level of content knowledge participants have when they begin the LOs. The rationale she can provide at this point does not point to a particular prediction.
A second measure Martha uses to decide her question is too general is that the predictions she can make regarding the answers seem very difficult to test. How could she test, for example, whether LOs should focus on content beyond what teachers will teach? What does “content beyond what teachers teach” mean? How could you tell whether teachers use their new knowledge of later content to inform their teaching?
Before anticipating what Martha’s next question might be, it is important to pause and recognize how predicting the answers to her questions moved Martha into a new phase in the research process. As she makes predictions, works out the reasons for them, and imagines how she might test them, she is immersed in scientific inquiry. This intellectual work is the main engine that drives the research process. Also notice that revisions in the questions asked, the predictions made, and the rationales built represent the updated thinking (Chap. 1 ) that occurs as Martha continues to define her study.
Based on all these considerations and her continued reading, Martha revises the question again. The question now reads, “Do LOs that engage middle school mathematics teachers in studying mathematics content help teachers teach this same content with more of a conceptual emphasis?” Although she feels like the question is more specific, she realizes that the answer to the question is either “yes” or “no.” This, by itself, is a red flag. Answers of “yes” or “no” would not contribute much to understanding the relationships between these LOs for teachers and changes in their teaching. Recall from Chap. 1 that understanding how things work, explaining why things work, is the goal of scientific inquiry.
Martha continues by trying to understand why she believes the answer is “yes.” When she tries to write out reasons for predicting “yes,” she realizes that her prediction depends on a variety of factors. If teachers already have deep knowledge of the content, the LOs might not affect them as much as other teachers. If the LOs do not help teachers develop their own conceptual understanding, they are not likely to change their teaching. By trying to build the rationale for her prediction—thus formulating a hypothesis—Martha realizes that the question still is not precise and clear enough.
Martha uses what she learned when developing the rationale and rephrases the question as follows: “ Under what conditions do LOs that engage middle school mathematics teachers in studying mathematics content help teachers teach this same content with more of a conceptual emphasis?” Through several additional cycles of thinking through the rationale for her predictions and how she might test them, Martha specifies her question even further: “Under what conditions do middle school teachers who lack conceptual knowledge of linear functions benefit from LOs that engage them in conceptual learning of linear functions as assessed by changes in their teaching toward a more conceptual emphasis on linear functions?”
Each version of Martha’s question has become more specific. This has occurred as she has (a) identified a starting condition for the teachers—they lack conceptual knowledge of linear functions, (b) specified the mathematics content as linear functions, and (c) included a condition or purpose of the LO—it is aimed at conceptual learning.
Because of the way Martha’s question is now phrased, her predictions will require thinking about the conditions that could influence what teachers learn from the LOs and how this learning could affect their teaching. She might predict that if teachers engaged in LOs that extended over multiple sessions, they would develop deeper understanding which would, in turn, prompt changes in their teaching. Or she might predict that if the LOs included examples of how their conceptual learning could translate into different instructional activities for their students, teachers would be more likely to change their teaching. Reasons for these predictions would likely come from research about the effects of professional development on teachers’ practice.
As Martha thinks about testing her predictions, she realizes it will probably be easier to measure the conditions under which teachers are learning than the changes in the conceptual emphasis in their instruction. She makes a note to continue searching the literature for ways to measure the “conceptualness” of teaching.
As she refines her predictions and expresses her reasons for the predictions, she formulates a hypothesis (in this case several hypotheses) that will guide her research. As she makes predictions and develops the rationales for these predictions, she will probably continue revising her question. She might decide, for example, that she is not interested in studying the condition of different numbers of LO sessions and so decides to remove this condition from consideration by including in her question something like “. . . over five 2-hour sessions . . .”
At this point, Martha has developed a research question, articulated a number of predictions, and developed rationales for them. Her current question is: “Under what conditions do middle school teachers who lack conceptual knowledge of linear functions benefit from five 2-hour LO sessions that engage them in conceptual learning of linear functions as assessed by changes in their teaching toward a more conceptual emphasis on linear functions?” Her hypothesis is:
Prediction: Participating teachers will show changes in their teaching with a greater emphasis on conceptual understanding, with larger changes on linear function topics directly addressed in the LOs than on other topics.
Brief Description of Rationale: (1) Past research has shown correlations between teachers’ specific mathematics knowledge of a topic and the quality of their teaching of that topic. This does not mean an increase in knowledge causes higher quality teaching but it allows for that possibility. (2) Transfer is usually difficult for teachers, but the examples developed during the LO sessions will help them use what they learned to teach for conceptual understanding. This is because the examples developed during the LO sessions are much like those that will be used by the teachers. So larger changes will be found when teachers are teaching the linear function topics addressed in the LOs.
Notice it is more straightforward to imagine how Martha could test this prediction because it is more precise than previous predictions. Notice also that by asking how to test a particular prediction, Martha will be faced with a decision about whether testing this prediction will tell her something she wants to learn. If not, she can return to the research question and consider how to specify it further and, perhaps, constrain further the conditions that could affect the data.
As Martha formulates her hypotheses and goes through multiple cycles of refining her question(s), articulating her predictions, and developing her rationales, she is constantly building the theoretical framework for her study. Because the theoretical framework is the topic for Chap. 3 , we will pause here and pick up Martha’s story in the next chapter. Spoiler alert: Martha’s experience contains some surprising twists and turns.
Before leaving Martha, however, we point out two aspects of the process in which she has been engaged. First, it can be useful to think about the process as identifying (1) the variables targeted in her predictions, (2) the mechanisms she believes explain the relationships among the variables, and (3) the definitions of all the terms that are special to her educational problem. By variables, we mean things that can be measured and, when measured, can take on different values. In Martha’s case, the variables are the conceptualness of teaching and the content topics addressed in the LOs. The mechanisms are cognitive processes that enable teachers to see the relevance of what they learn in PD to their own teaching and that enable the transfer of learning from one setting to another. Definitions are the precise descriptions of how the important ideas relevant to the research are conceptualized. In Martha’s case, definitions must be provided for terms like conceptual understanding, linear functions, LOs, each of the topics related to linear functions, instructional setting, and knowledge transfer.
A second aspect of the process is a practice that Martha acquired as part of her graduate program, a practice that can go unnoticed. Martha writes out, in full sentences, her thinking as she wrestles with her research question, her predictions of the answers, and the rationales for her predictions. Writing is a tool for organizing thinking and we recommend you use it throughout the scientific inquiry process. We say more about this at the end of the chapter.
Here are the questions Martha wrote as she developed a clearer sense of what question she wanted to answer and what answer she predicted. The list shows the increasing refinement that occurred as she continued to read, think, talk, and write.
Early questions: What kinds of LOs do most teachers experience? How do these experiences change teachers’ practices and beliefs? Are some LOs more effective than others? What makes them more effective?
First focused question: What makes LOs for teachers effective for improving their teaching for conceptual understanding?
Question after trying to predict the answer and imagining how to test the prediction: Do LOs that engage middle school mathematics teachers in studying mathematics content help teachers teach this same content with more of a conceptual emphasis?
Question after developing an initial rationale for her prediction: Under what conditions do LOs that engage middle school mathematics teachers in studying mathematics content help teachers teach this same content with more of a conceptual emphasis?
Question after developing a more precise prediction and richer rationale: Under what conditions do middle school teachers who lack conceptual knowledge of linear functions benefit from five 2-hour LO sessions that engage them in conceptual learning of linear functions as assessed by changes in their teaching toward a more conceptual emphasis on linear functions?
Part IV. An Illustrative Dialogue
The story of Martha described the major steps she took to refine her thinking. However, there is a lot of work that went on behind the scenes that wasn’t part of the story. For example, Martha had conversations with fellow students and professors that sharpened her thinking. What do these conversations look like? Because they are such an important part of the inquiry process, it will be helpful to “listen in” on the kinds of conversations that students might have with their advisors.
Here is a dialogue between a beginning student, Sam (S), and their advisor, Dr. Avery (A). They are meeting to discuss data Sam collected for a course project. The dialogue below is happening very early on in Sam’s conceptualization of the study, prior even to systematic reading of the literature.
Thanks for meeting with me today. As you know, I was able to collect some data for a course project a few weeks ago, but I’m having trouble analyzing the data, so I need your help. Let me try to explain the problem. As you know, I wanted to understand what middle-school teachers do to promote girls’ achievement in a mathematics class. I conducted four observations in each of three teachers’ classrooms. I also interviewed each teacher once about the four lessons I observed, and I interviewed two girls from each of the teachers’ classes. Obviously, I have a ton of data. But when I look at all these data, I don’t really know what I learned about my topic. When I was observing the teachers, I thought I might have observed some ways the teachers were promoting girls’ achievement, but then I wasn’t sure how to interpret my data. I didn’t know if the things I was observing were actually promoting girls’ achievement.
What were some of your observations?
Well, in a couple of my classroom observations, teachers called on girls to give an answer, even when the girls didn’t have their hands up. I thought that this might be a way that teachers were promoting the girls’ achievement. But then the girls didn’t say anything about that when I interviewed them and also the teachers didn’t do it in every class. So, it’s hard to know what effect, if any, this might have had on their learning or their motivation to learn. I didn’t want to ask the girls during the interview specifically about the teacher calling on them, and without the girls bringing it up themselves, I didn’t know if it had any effect.
Well, why didn’t you want to ask the girls about being called on?
Because I wanted to leave it as open as possible; I didn’t want to influence what they were going to say. I didn’t want to put words in their mouths. I wanted to know what they thought the teacher was doing that promoted their mathematical achievement and so I only asked the girls general questions, like “Do you think the teacher does things to promote girls’ mathematical achievement?” and “Can you describe specific experiences you have had that you believe do and do not promote your mathematical achievement?”
So then, how did they answer those general questions?
Well, with very general answers, such as that the teacher knows their names, offers review sessions, grades their homework fairly, gives them opportunities to earn extra credit, lets them ask questions, and always answers their questions. Nothing specific that helps me know what teaching actions specifically target girls’ mathematics achievement.
OK. Any ideas about what you might do next?
Well, I remember that when I was planning this data collection for my course, you suggested I might want to be more targeted and specific about what I was looking for. I can see now that more targeted questions would have made my data more interpretable in terms of connecting teaching actions to the mathematical achievement of girls. But I just didn’t want to influence what the girls would say.
Yes, I remember when you were planning your course project, you wanted to keep it open. You didn’t want to miss out on discovering something new and interesting. What do you think now about this issue?
Well, I still don’t want to put words in their mouths. I want to know what they think. But I see that if I ask really open questions, I have no guarantee they will talk about what I want them to talk about. I guess I still like the idea of an open study, but I see that it’s a risky approach. Leaving the questions too open meant I didn’t constrain their responses and there were too many ways they could interpret and answer the questions. And there are too many ways I could interpret their responses.
By this point in the dialogue, Sam has realized that open data (i.e., data not testing a specific prediction) is difficult to interpret. In the next part, Dr. Avery explains why collecting open data was not helping Sam achieve goals for her study that had motivated collecting open data in the first place.
Yes, I totally agree. Even for an experienced researcher, it can be difficult to make sense of this kind of open, messy data. However, if you design a study with a more specific focus, you can create questions for participants that are more targeted because you will be interested in their answers to these specific questions. Let’s reflect back on your data collection. What can you learn from it for the future?
When I think about it now, I realize that I didn’t think about the distinction between all the different constructs at play in my study, and I didn’t choose which one I was focusing on. One construct was the teaching moves that teachers think could be promoting achievement. Another is what teachers deliberately do to promote girls’ mathematics achievement, if anything. Another was the teaching moves that actually do support girls’ mathematics achievement. Another was what teachers were doing that supported girls’ mathematics achievement versus the mathematics achievement of all students. Another was students’ perception of what their teacher was doing to promote girls’ mathematics achievement. I now see that any one of these constructs could have been the focus of a study and that I didn’t really decide which of these was the focus of my course project prior to collecting data.
So, since you told me that the topic of this course project is probably what you’ll eventually want to study for your dissertation, which of these constructs are you most interested in?
I think I’m more interested in the teacher moves that teachers deliberately do to promote girls’ achievement. But I’m still worried about asking teachers directly and getting too specific about what they do because I don’t want to bias what they will say. And I chose qualitative methods and an exploratory design because I thought it would allow for a more open approach, an approach that helps me see what’s going on and that doesn’t bias or predetermine the results.
Well, it seems to me you are conflating three issues. One issue is how to conduct an unbiased study. Another issue is how specific to make your study. And the third issue is whether or not to choose an exploratory or qualitative study design. Those three issues are not the same. For example, designing a study that’s more open or more exploratory is not how researchers make studies fair and unbiased. In fact, it would be quite easy to create an open study that is biased. For example, you could ask very open questions and then interpret the responses in a way that unintentionally, and even unknowingly, aligns with what you were hoping the findings would say. Actually, you could argue that by adding more specificity and narrowing your focus, you’re creating constraints that prevent bias. The same goes for an exploratory or qualitative study; they can be biased or unbiased. So, let’s talk about what is meant by getting more specific. Within your new focus on what teachers deliberately do, there are many things that would be interesting to look at, such as teacher moves that address math anxiety, moves that allow girls to answer questions more frequently, moves that are specifically fitted to student thinking about specific mathematical content, and so on. What are one or two things that are most interesting to you? One way to answer this question is by thinking back to where your interest in this topic began.
In the preceding part of the dialogue, Dr. Avery explained how the goals Sam had for their study were not being met with open data. In the next part, Sam begins to articulate a prediction, which Sam and Dr. Avery then sharpen.
Actually, I became interested in this topic because of an experience I had in college when I was in a class of mostly girls. During whole class discussions, we were supposed to critically evaluate each other’s mathematical thinking, but we were too polite to do that. Instead, we just praised each other’s work. But it was so different in our small groups. It seemed easier to critique each other’s thinking and to push each other to better solutions in small groups. I began wondering how to get girls to be more critical of each other’s thinking in a whole class discussion in order to push everyone’s thinking.
Okay, this is great information. Why not use this idea to zoom-in on a more manageable and interpretable study? You could look specifically at how teachers support girls in critically evaluating each other’s thinking during whole class discussions. That would be a much more targeted and specific topic. Do you have predictions about what teachers could do in that situation, keeping in mind that you are looking specifically at girls’ mathematical achievement, not students in general?
Well, what I noticed was that small groups provided more social and emotional support for girls, whereas the whole class discussion did not provide that same support. The girls felt more comfortable critiquing each other’s thinking in small groups. So, I guess I predict that when the social and emotional supports that are present in small groups are extended to the whole class discussion, girls would be more willing to evaluate each other’s mathematical thinking critically during whole class discussion . I guess ultimately, I’d like to know how the whole class discussion could be used to enhance, rather than undermine, the social and emotional support that is present in the small groups.
Okay, then where would you start? Would you start with a study of what the teachers say they will do during whole class discussion and then observe if that happens during whole class discussion?
But part of my prediction also involves the small groups. So, I’d also like to include small groups in my study if possible. If I focus on whole groups, I won’t be exploring what I am interested in. My interest is broader than just the whole class discussion.
That makes sense, but there are many different things you could look at as part of your prediction, more than you can do in one study. For instance, if your prediction is that when the social and emotional supports that are present in small groups are extended to whole class discussions, girls would be more willing to evaluate each other’s mathematical thinking critically during whole class discussions , then you could ask the following questions: What are the social and emotional supports that are present in small groups?; In which small groups do they exist?; Is it groups that are made up only of girls?; Does every small group do this, and for groups that do this, when do these supports get created?; What kinds of small group activities that teachers ask them to work on are associated with these supports?; Do the same social and emotional supports that apply to small groups even apply to whole group discussion?
All your questions make me realize that my prediction about extending social and emotional supports to whole class discussions first requires me to have a better understanding of the social and emotional supports that exist in small groups. In fact, I first need to find out whether those supports commonly exist in small groups or is that just my experience working in small groups. So, I think I will first have to figure out what small groups do to support each other and then, in a later study, I could ask a teacher to implement those supports during whole class discussions and find out how you can do that. Yeah, now I’m seeing that.
The previous part of the dialogue illustrates how continuing to ask questions about one’s initial prediction is a good way to make it more and more precise (and researchable). In the next part, we see how developing a precise prediction has the added benefit of setting the researcher up for future studies.
Yes, I agree that for your first study, you should probably look at small groups. In other words, you should focus on only a part of your prediction for now, namely the part that says there are social and emotional supports in small groups that support girls in critiquing each other’s thinking . That begins to sharpen the focus of your prediction, but you’ll want to continue to refine it. For example, right now, the question that this prediction leads to is a question with a yes or no answer, but what you’ve said so far suggests to me that you are looking for more than that.
Yes, I want to know more than just whether there are supports. I’d like to know what kinds. That’s why I wanted to do a qualitative study.
Okay, this aligns more with my thinking about research as being prediction driven. It’s about collecting data that would help you revise your existing predictions into better ones. What I mean is that you would focus on collecting data that would allow you to refine your prediction, make it more nuanced, and go beyond what is already known. Does that make sense, and if so, what would that look like for your prediction?
Oh yes, I like that. I guess that would mean that, based on the data I collect for this next study, I could develop a more refined prediction that, for example, more specifically identifies and differentiates between different kinds of social and emotional supports that are present in small groups, or maybe that identifies the kinds of small groups that they occur in, or that predicts when and how frequently or infrequently they occur, or about the features of the small group tasks in which they occur, etc. I now realize that, although I chose qualitative research to make my study be more open, really the reason qualitative research fits my purposes is because it will allow me to explore fine-grained aspects of social and emotional supports that may exist for girls in small groups.
Yes, exactly! And then, based on the data you collect, you can include in your revised prediction those new fine-grained aspects. Furthermore, you will have a story to tell about your study in your written report, namely the story about your evolving prediction. In other words, your written report can largely tell how you filled out and refined your prediction as you learned more from carrying out the study. And even though you might not use them right away, you are also going to be able to develop new predictions that you would not have even thought of about social and emotional supports in small groups and your aim of extending them to whole-class discussions, had you not done this study. That will set you up to follow up on those new predictions in future studies. For example, you might have more refined ideas after you collect the data about the goals for critiquing student thinking in small groups versus the goals for critiquing student thinking during whole class discussion. You might even begin to think that some of the social and emotional supports you observe are not even replicable or even applicable to or appropriate for whole-class discussions, because the supports play different roles in different contexts. So, to summarize what I’m saying, what you look at in this study, even though it will be very focused, sets you up for a research program that will allow you to more fully investigate your broader interest in this topic, where each new study builds on your prior body of work. That’s why it is so important to be explicit about the best place to start this research, so that you can build on it.
I see what you are saying. We started this conversation talking about my course project data. What I think I should have done was figure out explicitly what I needed to learn with that study with the intention of then taking what I learned and using it as the basis for the next study. I didn’t do that, and so I didn’t collect data that pushed forward my thinking in ways that would guide my next study. It would be as if I was starting over with my next study.
Sam and Dr. Avery have just explored how specifying a prediction reveals additional complexities that could become fodder for developing a systematic research program. Next, we watch Sam beginning to recognize the level of specificity required for a prediction to be testable.
One thing that would have really helped would have been if you had had a specific prediction going into your data collection for your course project.
Well, I didn’t really have much of an explicit prediction in mind when I designed my methods.
Think back, you must have had some kind of prediction, even if it was implicit.
Well, yes, I guess I was predicting that teachers would enact moves that supported girls’ mathematical achievement. And I observed classrooms to identify those teacher moves, I interviewed teachers to ask them about the moves I observed, and I interviewed students to see if they mentioned those moves as promoting their mathematical achievement. The goal of my course project was to identify teacher moves that support girls’ mathematical achievement. And my specific research question was: What teacher moves support girls’ mathematical achievement?
So, really you were asking the teacher and students to show and tell you what those moves are and the effects of those moves, as a result putting the onus on your participants to provide the answers to your research question for you. I have an idea, let’s try a thought experiment. You come up with data collection methods for testing the prediction that there are social and emotional supports in small groups that support girls in critiquing each other’s thinking that still puts the onus on the participants. And then I’ll see if I can think of data collection methods that would not put the onus on the participants.
Hmm, well. .. I guess I could simply interview girls who participated in small groups and ask them “are there social and emotional supports that you use in small groups that support your group in critiquing each other’s thinking and if so, what are they?” In that case, I would be putting the onus on them to be aware of the social dynamics of small groups and to have thought about these constructs as much as I have. Okay now can you continue the thought experiment? What might the data collection methods look like if I didn’t put the onus on the participants?
First, I would pick a setting in which it was only girls at this point to reduce the number of variables. Then, personally I would want to observe a lot of groups of girls interacting in groups around tasks. I would be looking for instances when the conversation about students’ ideas was shut down and instances when the conversation about students’ ideas involved critiquing of ideas and building on each other’s thinking. I would also look at what happened just before and during those instances, such as: did the student continue to talk after their thinking was critiqued, did other students do anything to encourage the student to build on their own thinking (i.e., constructive criticism) or how did they support or shut down continued participation. In fact, now that I think about it, “critiquing each other’s thinking” can be defined in a number of different ways. I could mean just commenting on someone’s thinking, judging correctness and incorrectness, constructive criticism that moves the thinking forward, etc. If you put the onus on the participants to answer your research question, you are stuck with their definition, and they won’t have thought about this very much, if at all.
I think that what you are also saying is that my definitions would affect my data collection. If I think that critiquing each other’s thinking means that the group moves their thinking forward toward more valid and complete mathematical solutions, then I’m going to focus on different moves than if I define it another way, such as just making a comment on each other’s thinking and making each other feel comfortable enough to keep participating. In fact, am I going to look at individual instances of critiquing or look at entire sequences in which the critiquing leads to a goal? This seems like a unit of analysis question, and I would need to develop a more nuanced prediction that would make explicit what that unit of analysis is.
I agree, your definition of “critiquing each other’s thinking” could entirely change what you are predicting. One prediction could be based on defining critiquing as a one-shot event in which someone makes one comment on another person’s thinking. In this case the prediction would be that there are social and emotional supports in small groups that support girls in making an evaluative comment on another student’s thinking. Another prediction could be based on defining critiquing as a back-and-forth process in which the thinking gets built on and refined. In that case, the prediction would be something like that there are social and emotional supports in small groups that support girls in critiquing each other’s thinking in ways that do not shut down the conversation but that lead to sustained conversations that move each other toward more valid and complete solutions.
Well, I think I am more interested in the second prediction because it is more compatible with my long-term interests, which are that I’m interested in extending small group supports to whole class discussions. The second prediction is more appropriate for eventually looking at girls in whole class discussion. During whole class discussion, the teacher tries to get a sustained conversation going that moves the students’ thinking forward. So, if I learn about small group supports that lead to sustained conversations that move each other toward more valid and complete solutions , those supports might transfer to whole class discussions.
In the previous part of the dialogue, Dr. Avery and Sam showed how narrowing down a prediction to one that is testable requires making numerous important decisions, including how to define the constructs referred to in the prediction. In the final part of the dialogue, Dr. Avery and Sam begin to outline the reading Sam will have to do to develop a rationale for the specific prediction.
Do you see how your prediction and definitions are getting more and more specific? You now need to read extensively to further refine your prediction.
Well, I should probably read about micro dynamics of small group interactions, anything about interactions in small groups, and what is already known about small group interactions that support sustained conversations that move students’ thinking toward more valid and complete solutions. I guess I could also look at research on whole-class discussion methods that support sustained conversations that move the class to more mathematically valid and complete solutions, because it might give me ideas for what to look for in the small groups. I might also need to focus on research about how learners develop understandings about a particular subject matter so that I know what “more valid and complete solutions” look like. I also need to read about social and emotional supports but focus on how they support students cognitively, rather than in other ways.
Sounds good, let’s get together after you have processed some of this literature and we can talk about refining your prediction based on what you read and also the methods that will best suit testing that prediction.
Great! Thanks for meeting with me. I feel like I have a much better set of tools that push my own thinking forward and allow me to target something specific that will lead to more interpretable data.
Part V. Is It Always Possible to Formulate Hypotheses?
In Chap. 1 , we noted you are likely to read that research does not require formulating hypotheses. Some sources describe doing research without making predictions and developing rationales for these predictions. Some researchers say you cannot always make predictions—you do not know enough about the situation. In fact, some argue for the value of not making predictions (e.g., Glaser & Holton, 2004 ; Merton, 1968 ; Nemirovsky, 2011 ). These are important points of view, so we will devote this section to discussing them.
Can You Always Predict What You Will Find?
One reason some researchers say you do not need to make predictions is that it can be difficult to imagine what you will find. This argument comes up most often for descriptive studies. Suppose you want to describe the nature of a situation you do not know much about. Can you still make a prediction about what you will find? We believe that, although you do not know exactly what you will find, you probably have a hunch or, at a minimum, a very fuzzy idea. It would be unusual to ask a question about a situation you want to know about without at least a fuzzy inkling of what you might find. The original question just would not occur to you. We acknowledge you might have only a vague idea of what you will find and you might not have much confidence in your prediction. However, we expect if you monitor your own thinking you will discover you have developed a suspicion along the way, regardless how vague the suspicion might be. Through the cyclic process we discussed above, that suspicion or hunch gradually evolves and turns into a prediction.
The Benefits of Making Predictions Even When They Are Wrong: An Example from the 1970s
One of us was a graduate student at the University of Wisconsin in the late 1970s, assigned as a research assistant to a project that was investigating young children’s thinking about simple arithmetic. A new curriculum was being written, and the developers wanted to know how to introduce the earliest concepts and skills to kindergarten and first-grade children. The directors of the project did not know what to expect because, at the time, there was little research on five- and six-year-olds’ pre-instruction strategies for adding and subtracting.
After consulting what literature was available, talking with teachers, analyzing the nature of different types of addition and subtraction problems, and debating with each other, the research team formulated some hypotheses about children’s performance. Following the usual assumptions at the time and recognizing the new curriculum would introduce the concepts, the researchers predicted that, before instruction, most children would not be able to solve the problems. Based on the rationale that some young children did not yet recognize the simple form for written problems (e.g., 5 + 3 = ___), the researchers predicted that the best chance for success would be to read problems as stories (e.g., Jesse had 5 apples and then found 3 more. How many does she have now?). They reasoned that, even though children would have difficulty on all the problems, some story problems would be easier because the semantic structure is easier to follow. For example, they predicted the above story about adding 3 apples to 5 would be easier than a problem like, “Jesse had some apples in the refrigerator. She put in 2 more and now has 6. How many were in the refrigerator at the beginning?” Based on the rationale that children would need to count to solve the problems and that it can be difficult to keep track of the numbers, they predicted children would be more successful if they were given counters. Finally, accepting the common reasoning that larger numbers are more difficult than smaller numbers, they predicted children would be more successful if all the numbers in a problem were below 10.
Although these predictions were not very precise and the rationales were not strongly convincing, these hypotheses prompted the researchers to design the study to test their predictions. This meant they would collect data by presenting a variety of problems under a variety of conditions. Because the goal was to describe children’s thinking, problems were presented to students in individual interviews. Problems with different semantic structures were included, counters were available for some problems but not others, and some problems had sums to 9 whereas others had sums to 20 or more.
The punchline of this story is that gathering data under these conditions, prompted by the predictions, made all the difference in what the researchers learned. Contrary to predictions, children could solve addition and subtraction problems before instruction. Counters were important because almost all the solution strategies were based on counting which meant that memory was an issue because many strategies require counting in two ways simultaneously. For example, subtracting 4 from 7 was usually solved by counting down from 7 while counting up from 1 to 4 to keep track of counting down. Because children acted out the stories with their counters, the semantic structure of the story was also important. Stories that were easier to read and write were also easier to solve.
To make a very long story very short, other researchers were, at about the same time, reporting similar results about children’s pre-instruction arithmetic capabilities. A clear pattern emerged regarding the relative difficulty of different problem types (semantic structures) and the strategies children used to solve each type. As the data were replicated, the researchers recognized that kindergarten and first-grade teachers could make good use of this information when they introduced simple arithmetic. This is how Cognitively Guided Instruction (CGI) was born (Carpenter et al., 1989 ; Fennema et al., 1996 ).
To reiterate, the point of this example is that the study conducted to describe children’s thinking would have looked quite different if the researchers had made no predictions. They would have had no reason to choose the particular problems and present them under different conditions. The fact that some of the predictions were completely wrong is not the point. The predictions created the conditions under which the predictions were tested which, in turn, created learning opportunities for the researchers that would not have existed without the predictions. The lesson is that even research that aims to simply describe a phenomenon can benefit from hypotheses. As signaled in Chap. 1 , this also serves as another example of “failing productively.”
Suggestions for What to Do When You Do Not Have Predictions
There likely are exceptions to our claim about being able to make a prediction about what you will find. For example, there could be rare cases where researchers truly have no idea what they will find and can come up with no predictions and even no hunches. And, no research has been reported on related phenomena that would offer some guidance. If you find yourself in this position, we suggest one of three approaches: revise your question, conduct a pilot study, or choose another question.
Because there are many advantages to making predictions explicit and then writing out the reasons for these predictions, one approach is to adjust your question just enough to allow you to make a prediction. Perhaps you can build on descriptions that other researchers have provided for related situations and consider how you can extend this work. Building on previous descriptions will enable you to make predictions about the situation you want to describe.
A second approach is to conduct a small pilot study or, better, a series of small pilot studies to develop some preliminary ideas of what you might find. If you can identify a small sample of participants who are similar to those in your study, you can try out at least some of your research plans to help make and refine your predictions. As we detail later, you can also use pilot studies to check whether key aspects of your methods (e.g., tasks, interview questions, data collection methods) work as you expect.
A third approach is to return to your list of interests and choose one that has been studied previously. Sometimes this is the wisest choice. It is very difficult for beginning researchers to conduct research in brand-new areas where no hunches or predictions are possible. In addition, the contributions of this research can be limited. Recall the earlier story about one of us “failing productively” by completing a dissertation in a somewhat new area. If, after an exhaustive search, you find that no one has investigated the phenomenon in which you are interested or even related phenomena, it can be best to move in a different direction. You will read recommendations in other sources to find a “gap” in the research and develop a study to “fill the gap.” This can be helpful advice if the gap is very small. However, if the gap is large, too large to predict what you might find, the study will present severe challenges. It will be more productive to extend work that has already been done than to launch into an entirely new area.
Should You Always Try to Predict What You Will Find?
In short, our answer to the question in the heading is “yes.” But this calls for further explanation.
Suppose you want to observe a second-grade classroom in order to investigate how students talk about adding and subtracting whole numbers. You might think, “I don’t want to bias my thinking; I want to be completely open to what I see in the classroom.” Sam shared a similar point of view at the beginning of the dialogue: “I wanted to leave it as open as possible; I didn’t want to influence what they were going to say.” Some researchers say that beginning your research study by making predictions is inappropriate precisely because it will bias your observations and results. The argument is that by bringing a set of preconceptions, you will confirm what you expected to find and be blind to other observations and outcomes. The following quote illustrates this view: “The first step in gaining theoretical sensitivity is to enter the research setting with as few predetermined ideas as possible—especially logically deducted, a priori hypotheses. In this posture, the analyst is able to remain sensitive to the data by being able to record events and detect happenings without first having them filtered through and squared with pre-existing hypotheses and biases” (Glaser, 1978, pp. 2–3).
We take a different point of view. In fact, we believe there are several compelling reasons for making your predictions explicit.
Making Your Predictions Explicit Increases Your Chances of Productive Observations
Because your predictions are an extension of what is already known, they prepare you to identify more nuanced relationships that can advance our understanding of a phenomenon. For example, rather than simply noticing, in a general sense, that students talking about addition and subtraction leads them to better understandings, you might, based on your prediction, make the specific observation that talking about addition and subtraction in a particular way helps students to think more deeply about a particular concept related to addition and subtraction. Going into a study without predictions can bring less sensitivity rather than more to the study of a phenomenon. Drawing on knowledge about related phenomena by reading the literature and conducting pilot studies allows you to be much more sensitive and your observations to be more productive.
Making Your Predictions Explicit Allows You to Guard Against Biases
Some genres and methods of educational research are, in fact, rooted in philosophical traditions (e.g., Husserl, 1929/ 1973 ) that explicitly call for researchers to temporarily “bracket” or set aside existing theory as well as their prior knowledge and experience to better enter into the experience of the participants in the research. However, this does not mean ignoring one’s own knowledge and experience or turning a blind eye to what has been learned by others. Much more than the simplistic image of emptying one’s mind of preconceptions and implicit biases (arguably an impossible feat to begin with), the goal is to be as reflective as possible about one’s prior knowledge and conceptions and as transparent as possible about how they may guide observations and shape interpretations (Levitt et al., 2018 ).
We believe it is better to be honest about the predictions you are almost sure to have because then you can deliberately plan to minimize the chances they will influence what you find and how you interpret your results. For starters, it is important to recognize that acknowledging you have some guesses about what you will find does not make them more influential. Because you are likely to have them anyway, we recommend being explicit about what they are. It is easier to deal with biases that are explicit than those that lurk in the background and are not acknowledged.
What do we mean by “deal with biases”? Some journals require you to include a statement about your “positionality” with respect to the participants in your study and the observations you are making to gather data. Formulating clear hypotheses is, in our view, a direct response to this request. The reasons for your predictions are your explicit statements about your positionality. Often there are methodological strategies you can use to protect the study from undue influences of bias. In other words, making your vague predictions explicit can help you design your study so you minimize the bias of your findings.
Making Your Predictions Explicit Can Help You See What You Did Not Predict
Making your predictions explicit does not need to blind you to what is different than expected. It does not need to force you to see only what you want to see. Instead, it can actually increase your sensitivity to noticing features of the situation that are surprising, features you did not predict. Results can stand out when you did not expect to see them.
In contrast, not bringing your biases to consciousness might subtly shift your attention away from these unexpected results in ways that you are not aware of. This path can lead to claiming no biases and no unexpected findings without being conscious of them. You cannot observe everything, and some things inevitably will be overlooked. If you have predicted what you will see, you can design your study so that the unexpected results become more salient rather than less.
Returning to the example of observing a second-grade classroom, we note that the field already knows a great deal about how students talk about addition and subtraction. Being cognizant of what others have observed allows you to enter the classroom with some clear predictions about what will happen. The rationales for these predictions are based on all the related knowledge you have before stepping into the classroom, and the predictions and rationales help you to better deal with what you see. This is partly because you are likely to be surprised by the things you did not anticipate. There is almost always something that will surprise you because your predictions will almost always be incomplete or too general. This sensitivity to the unanticipated—the sense of surprise that sparks your curiosity—is an indication of your openness to the phenomenon you are studying.
Making Your Predictions Explicit Allows You to Plan in Advance
Recall from Chap. 1 the descriptor of scientific inquiry: “Experience carefully planned in advance.” If you make no predictions about what might happen, it is very difficult, if not impossible, to plan your study in advance. Again, you cannot observe everything, so you must make decisions about what you will observe. What kind of data will you plan to collect? Why would you collect these data instead of others? If you have no idea what to expect, on what basis will you make these consequential decisions? Even if your predictions are vague and your rationales for the predictions are a bit shaky, at least they provide a direction for your plan. They allow you to explain why you are planning this study and collecting these data. They allow you to “carefully plan in advance.”
Making Your Predictions Explicit Allows You to Put Your Rationales in Harm’s Way
Rationales are developed to justify the predictions. Rationales represent your best reasoning about the research problem you are studying. How can you tell whether your reasoning is sound? You can try it out with colleagues. However, the best way to test it is to put it in “harm’s way” (Cobb, Confrey, diSessa, Lehrer, & Schauble, 2003 p. 10). And the best approach to putting your reasoning in harm’s way is to test the predictions it generates. Regardless if you are conducting a qualitative or quantitative study, rationales can be improved only if they generate testable predictions. This is possible only if predictions are explicit and precise. As we described earlier, rationales are evaluated for their soundness and refined in light of the specific differences between predictions and empirical observations.
Making Your Predictions Explicit Forces You to Organize and Extend Your (and the Field’s) Thinking
By writing out your predictions (even hunches or fuzzy guesses) and by reflecting on why you have these predictions and making these reasons explicit for yourself, you are advancing your thinking about the questions you really want to answer. This means you are making progress toward formulating your research questions and your final hypotheses. Making more progress in your own thinking before you conduct your study increases the chances your study will be of higher quality and will be exactly the study you intended. Making predictions, developing rationales, and imagining tests are tools you can use to push your thinking forward before you even collect data.
Suppose you wonder how preservice teachers in your university’s teacher preparation program will solve particular kinds of math problems. You are interested in this question because you have noticed several PSTs solve them in unexpected ways. As you ask the question you want to answer, you make predictions about what you expect to see. When you reflect on why you made these predictions, you realize that some PSTs might use particular solution strategies because they were taught to use some of them in an earlier course, and they might believe you expect them to solve the problems in these ways. By being explicit about why you are making particular predictions, you realize that you might be answering a different question than you intend (“How much do PSTs remember from previous courses?” or even “To what extent do PSTs believe different instructors have similar expectations?”). Now you can either change your question or change the design of your study (i.e., the sample of students you will use) or both. You are advancing your thinking by being explicit about your predictions and why you are making them.
The Costs of Not Making Predictions
Avoiding making predictions, for whatever reason, comes with significant costs. It prevents you from learning very much about your research topic. It would require not reading related research, not talking with your colleagues, and not conducting pilot studies because, if you do, you are likely to find a prediction creeping into your thinking. Not doing these things would forego the benefits of advancing your thinking before you collect data. It would amount to conducting the study with as little forethought as possible.
Part VI. How Do You Formulate Important Hypotheses?
We provided a partial answer in Chap. 1 to the question of a hypothesis’ importance when we encouraged considering the ultimate goal to which a study’s findings might contribute. You might want to reread Part III of Chap. 1 where we offered our opinions about the purposes of doing research. We also recommend reading the March 2019 editorial in the Journal for Research in Mathematics Education (Cai et al., 2019b ) in which we address what constitutes important educational research.
As we argued in Chap. 1 and in the March 2019 editorial, a worthy ultimate goal for educational research is to improve the learning opportunities for all students. However, arguments can be made for other ultimate goals as well. To gauge the importance of your hypotheses, think about how clearly you can connect them to a goal the educational community considers important. In addition, given the descriptors of scientific inquiry proposed in Chap. 1 , think about how testing your hypotheses will help you (and the community) understand what you are studying. Will you have a better explanation for the phenomenon after your study than before?
Although we address the question of importance again, and in more detail, in Chap. 5 , it is useful to know here that you can determine the significance or importance of your hypotheses when you formulate them. The importance need not depend on the data you collect or the results you report. The importance can come from the fact that, based on the results of your study, you will be able to offer revised hypotheses that help the field better understand an important issue. In large part, it is these revised hypotheses rather than the data that determine a study’s importance.
A critical caveat to this discussion is that few hypotheses are self-evidently important. They are important only if you make the case for their importance. Even if you follow closely the guidelines we suggest for formulating an important hypothesis, you must develop an argument that convinces others. This argument will be presented in the research paper you write.

Consider Martha’s hypothesis presented earlier. When we left Martha, she predicted that “Participating teachers will show changes in their teaching with a greater emphasis on conceptual understanding with larger changes on linear function topics directly addressed in the LOs than on other topics.” For researchers and educators not intimately familiar with this area of research, it is not apparent why someone should spend a year or more conducting a dissertation to test this prediction. Her rationale, summarized earlier, begins to describe why this could be an important hypothesis. But it is by writing a clear argument that explains her rationale to readers that she will convince them of its importance.
How Martha fills in her rationale so she can create a clear written argument for its importance is taken up in Chap. 3 . As we indicated, Martha’s work in this regard led her to make some interesting decisions, in part due to her own assessment of what was important.
Part VII. Beginning to Write the Research Paper for Your Study
It is common to think that researchers conduct a study and then, after the data are collected and analyzed, begin writing the paper about the study. We recommend an alternative, especially for beginning researchers. We believe it is better to write drafts of the paper at the same time you are planning and conducting your study. The paper will gradually evolve as you work through successive phases of the scientific inquiry process. Consequently, we will call this paper your evolving research paper .

You will use your evolving research paper to communicate your study, but you can also use writing as a tool for thinking and organizing your thinking while planning and conducting the study. Used as a tool for thinking, you can write drafts of your ideas to check on the clarity of your thinking, and then you can step back and reflect on how to clarify it further. Be sure to avoid jargon and general terms that are not well defined. Ask yourself whether someone not in your field, maybe a sibling, a parent, or a friend, would be able to understand what you mean. You are likely to write multiple drafts with lots of scribbling, crossing out, and revising.
Used as a tool for communicating, writing the best version of what you know before moving to the next phase will help you record your decisions and the reasons for them before you forget important details. This best-version-for-now paper also provides the basis for your thinking about the next phase of your scientific inquiry.
At this point in the process, you will be writing your (research) questions, the answers you predict, and the rationales for your predictions. The predictions you make should be direct answers to your research questions and should flow logically from (or be directly supported by) the rationales you present. In addition, you will have a written statement of the study’s purpose or, said another way, an argument for the importance of the hypotheses you will be testing. It is in the early sections of your paper that you will convince your audience about the importance of your hypotheses.
In our experience, presenting research questions is a more common form of stating the goal of a research study than presenting well-formulated hypotheses. Authors sometimes present a hypothesis, often as a simple prediction of what they might find. The hypothesis is then forgotten and not used to guide the analysis or interpretations of the findings. In other words, authors seldom use hypotheses to do the kind of work we describe. This means that many research articles you read will not treat hypotheses as we suggest. We believe these are missed opportunities to present research in a more compelling and informative way. We intend to provide enough guidance in the remaining chapters for you to feel comfortable organizing your evolving research paper around formulating, testing, and revising hypotheses.
While we were editing one of the leading research journals in mathematics education ( JRME ), we conducted a study of reviewers’ critiques of papers submitted to the journal. Two of the five most common concerns were: (1) the research questions were unclear, and (2) the answers to the questions did not make a substantial contribution to the field. These are likely to be major concerns for the reviewers of all research journals. We hope the knowledge and skills you have acquired working through this chapter will allow you to write the opening to your evolving research paper in a way that addresses these concerns. Much of the chapter should help make your research questions clear, and the prior section on formulating “important hypotheses” will help you convey the contribution of your study.
Exercise 2.3
Look back at your answers to the sets of questions before part II of this chapter.
Think about how you would argue for the importance of your current interest.
Write your interest in the form of (1) a research problem, (2) a research question, and (3) a prediction with the beginnings of a rationale. You will update these as you read the remaining chapters.
Part VIII. The Heart of Scientific Inquiry
In this chapter, we have described the process of formulating hypotheses. This process is at the heart of scientific inquiry. It is where doing research begins. Conducting research always involves formulating, testing, and revising hypotheses. This is true regardless of your research questions and whether you are using qualitative, quantitative, or mixed methods. Without engaging in this process in a deliberate, intense, relentless way, your study will reveal less than it could. By engaging in this process, you are maximizing what you, and others, can learn from conducting your study.
In the next chapter, we build on the ideas we have developed in the first two chapters to describe the purpose and nature of theoretical frameworks . The term theoretical framework, along with closely related terms like conceptual framework, can be somewhat mysterious for beginning researchers and can seem like a requirement for writing a paper rather than an aid for conducting research. We will show how theoretical frameworks grow from formulating hypotheses—from developing rationales for the predicted answers to your research questions. We will propose some practical suggestions for building theoretical frameworks and show how useful they can be. In addition, we will continue Martha’s story from the point at which we paused earlier—developing her theoretical framework.
Cai, J., Morris, A., Hohensee, C., Hwang, S., Robison, V., Cirillo, M., Kramer, S. L., & Hiebert, J. (2019b). Posing significant research questions. Journal for Research in Mathematics Education, 50 (2), 114–120. https://doi.org/10.5951/jresematheduc.50.2.0114
Article Google Scholar
Carpenter, T. P., Fennema, E., Peterson, P. L., Chiang, C. P., & Loef, M. (1989). Using knowledge of children’s mathematics thinking in classroom teaching: An experimental study. American Educational Research Journal, 26 (4), 385–531.
Fennema, E., Carpenter, T. P., Franke, M. L., Levi, L., Jacobs, V. R., & Empson, S. B. (1996). A longitudinal study of learning to use children’s thinking in mathematics instruction. Journal for Research in Mathematics Education, 27 (4), 403–434.
Glaser, B. G., & Holton, J. (2004). Remodeling grounded theory. Forum: Qualitative Social Research, 5(2). https://www.qualitative-research.net/index.php/fqs/article/view/607/1316
Gournelos, T., Hammonds, J. R., & Wilson, M. A. (2019). Doing academic research: A practical guide to research methods and analysis . Routledge.
Book Google Scholar
Hohensee, C. (2014). Backward transfer: An investigation of the influence of quadratic functions instruction on students’ prior ways of reasoning about linear functions. Mathematical Thinking and Learning, 16 (2), 135–174.
Husserl, E. (1973). Cartesian meditations: An introduction to phenomenology (D. Cairns, Trans.). Martinus Nijhoff. (Original work published 1929).
Google Scholar
Levitt, H. M., Bamberg, M., Creswell, J. W., Frost, D. M., Josselson, R., & Suárez-Orozco, C. (2018). Journal article reporting standards for qualitative primary, qualitative meta-analytic, and mixed methods research in psychology: The APA Publications and Communications Board Task Force report. American Psychologist, 73 (1), 26–46.
Medawar, P. (1982). Pluto’s republic [no typo]. Oxford University Press.
Merton, R. K. (1968). Social theory and social structure (Enlarged edition). Free Press.
Nemirovsky, R. (2011). Episodic feelings and transfer of learning. Journal of the Learning Sciences, 20 (2), 308–337. https://doi.org/10.1080/10508406.2011.528316
Vygotsky, L. (1987). The development of scientific concepts in childhood: The design of a working hypothesis. In A. Kozulin (Ed.), Thought and language (pp. 146–209). The MIT Press.
Download references
Author information
Authors and affiliations.
School of Education, University of Delaware, Newark, DE, USA
James Hiebert, Anne K Morris & Charles Hohensee
Department of Mathematical Sciences, University of Delaware, Newark, DE, USA
Jinfa Cai & Stephen Hwang
You can also search for this author in PubMed Google Scholar
Rights and permissions
Open Access This chapter is licensed under the terms of the Creative Commons Attribution 4.0 International License ( http://creativecommons.org/licenses/by/4.0/ ), which permits use, sharing, adaptation, distribution and reproduction in any medium or format, as long as you give appropriate credit to the original author(s) and the source, provide a link to the Creative Commons license and indicate if changes were made.
The images or other third party material in this chapter are included in the chapter's Creative Commons license, unless indicated otherwise in a credit line to the material. If material is not included in the chapter's Creative Commons license and your intended use is not permitted by statutory regulation or exceeds the permitted use, you will need to obtain permission directly from the copyright holder.
Reprints and permissions
Copyright information
© 2023 The Author(s)
About this chapter
Hiebert, J., Cai, J., Hwang, S., Morris, A.K., Hohensee, C. (2023). How Do You Formulate (Important) Hypotheses?. In: Doing Research: A New Researcher’s Guide. Research in Mathematics Education. Springer, Cham. https://doi.org/10.1007/978-3-031-19078-0_2
Download citation
DOI : https://doi.org/10.1007/978-3-031-19078-0_2
Published : 03 December 2022
Publisher Name : Springer, Cham
Print ISBN : 978-3-031-19077-3
Online ISBN : 978-3-031-19078-0
eBook Packages : Education Education (R0)
Share this chapter
Anyone you share the following link with will be able to read this content:
Sorry, a shareable link is not currently available for this article.
Provided by the Springer Nature SharedIt content-sharing initiative
- Publish with us
Policies and ethics
- Find a journal
- Track your research
- Bipolar Disorder
- Therapy Center
- When To See a Therapist
- Types of Therapy
- Best Online Therapy
- Best Couples Therapy
- Best Family Therapy
- Managing Stress
- Sleep and Dreaming
- Understanding Emotions
- Self-Improvement
- Healthy Relationships
- Student Resources
- Personality Types
- Guided Meditations
- Verywell Mind Insights
- 2024 Verywell Mind 25
- Mental Health in the Classroom
- Editorial Process
- Meet Our Review Board
- Crisis Support
How to Write a Great Hypothesis
Hypothesis Definition, Format, Examples, and Tips
Kendra Cherry, MS, is a psychosocial rehabilitation specialist, psychology educator, and author of the "Everything Psychology Book."
:max_bytes(150000):strip_icc():format(webp)/IMG_9791-89504ab694d54b66bbd72cb84ffb860e.jpg)
Amy Morin, LCSW, is a psychotherapist and international bestselling author. Her books, including "13 Things Mentally Strong People Don't Do," have been translated into more than 40 languages. Her TEDx talk, "The Secret of Becoming Mentally Strong," is one of the most viewed talks of all time.
:max_bytes(150000):strip_icc():format(webp)/VW-MIND-Amy-2b338105f1ee493f94d7e333e410fa76.jpg)
Verywell / Alex Dos Diaz
- The Scientific Method
Hypothesis Format
Falsifiability of a hypothesis.
- Operationalization
Hypothesis Types
Hypotheses examples.
- Collecting Data
A hypothesis is a tentative statement about the relationship between two or more variables. It is a specific, testable prediction about what you expect to happen in a study. It is a preliminary answer to your question that helps guide the research process.
Consider a study designed to examine the relationship between sleep deprivation and test performance. The hypothesis might be: "This study is designed to assess the hypothesis that sleep-deprived people will perform worse on a test than individuals who are not sleep-deprived."
At a Glance
A hypothesis is crucial to scientific research because it offers a clear direction for what the researchers are looking to find. This allows them to design experiments to test their predictions and add to our scientific knowledge about the world. This article explores how a hypothesis is used in psychology research, how to write a good hypothesis, and the different types of hypotheses you might use.
The Hypothesis in the Scientific Method
In the scientific method , whether it involves research in psychology, biology, or some other area, a hypothesis represents what the researchers think will happen in an experiment. The scientific method involves the following steps:
- Forming a question
- Performing background research
- Creating a hypothesis
- Designing an experiment
- Collecting data
- Analyzing the results
- Drawing conclusions
- Communicating the results
The hypothesis is a prediction, but it involves more than a guess. Most of the time, the hypothesis begins with a question which is then explored through background research. At this point, researchers then begin to develop a testable hypothesis.
Unless you are creating an exploratory study, your hypothesis should always explain what you expect to happen.
In a study exploring the effects of a particular drug, the hypothesis might be that researchers expect the drug to have some type of effect on the symptoms of a specific illness. In psychology, the hypothesis might focus on how a certain aspect of the environment might influence a particular behavior.
Remember, a hypothesis does not have to be correct. While the hypothesis predicts what the researchers expect to see, the goal of the research is to determine whether this guess is right or wrong. When conducting an experiment, researchers might explore numerous factors to determine which ones might contribute to the ultimate outcome.
In many cases, researchers may find that the results of an experiment do not support the original hypothesis. When writing up these results, the researchers might suggest other options that should be explored in future studies.
In many cases, researchers might draw a hypothesis from a specific theory or build on previous research. For example, prior research has shown that stress can impact the immune system. So a researcher might hypothesize: "People with high-stress levels will be more likely to contract a common cold after being exposed to the virus than people who have low-stress levels."
In other instances, researchers might look at commonly held beliefs or folk wisdom. "Birds of a feather flock together" is one example of folk adage that a psychologist might try to investigate. The researcher might pose a specific hypothesis that "People tend to select romantic partners who are similar to them in interests and educational level."
Elements of a Good Hypothesis
So how do you write a good hypothesis? When trying to come up with a hypothesis for your research or experiments, ask yourself the following questions:
- Is your hypothesis based on your research on a topic?
- Can your hypothesis be tested?
- Does your hypothesis include independent and dependent variables?
Before you come up with a specific hypothesis, spend some time doing background research. Once you have completed a literature review, start thinking about potential questions you still have. Pay attention to the discussion section in the journal articles you read . Many authors will suggest questions that still need to be explored.
How to Formulate a Good Hypothesis
To form a hypothesis, you should take these steps:
- Collect as many observations about a topic or problem as you can.
- Evaluate these observations and look for possible causes of the problem.
- Create a list of possible explanations that you might want to explore.
- After you have developed some possible hypotheses, think of ways that you could confirm or disprove each hypothesis through experimentation. This is known as falsifiability.
In the scientific method , falsifiability is an important part of any valid hypothesis. In order to test a claim scientifically, it must be possible that the claim could be proven false.
Students sometimes confuse the idea of falsifiability with the idea that it means that something is false, which is not the case. What falsifiability means is that if something was false, then it is possible to demonstrate that it is false.
One of the hallmarks of pseudoscience is that it makes claims that cannot be refuted or proven false.
The Importance of Operational Definitions
A variable is a factor or element that can be changed and manipulated in ways that are observable and measurable. However, the researcher must also define how the variable will be manipulated and measured in the study.
Operational definitions are specific definitions for all relevant factors in a study. This process helps make vague or ambiguous concepts detailed and measurable.
For example, a researcher might operationally define the variable " test anxiety " as the results of a self-report measure of anxiety experienced during an exam. A "study habits" variable might be defined by the amount of studying that actually occurs as measured by time.
These precise descriptions are important because many things can be measured in various ways. Clearly defining these variables and how they are measured helps ensure that other researchers can replicate your results.
Replicability
One of the basic principles of any type of scientific research is that the results must be replicable.
Replication means repeating an experiment in the same way to produce the same results. By clearly detailing the specifics of how the variables were measured and manipulated, other researchers can better understand the results and repeat the study if needed.
Some variables are more difficult than others to define. For example, how would you operationally define a variable such as aggression ? For obvious ethical reasons, researchers cannot create a situation in which a person behaves aggressively toward others.
To measure this variable, the researcher must devise a measurement that assesses aggressive behavior without harming others. The researcher might utilize a simulated task to measure aggressiveness in this situation.
Hypothesis Checklist
- Does your hypothesis focus on something that you can actually test?
- Does your hypothesis include both an independent and dependent variable?
- Can you manipulate the variables?
- Can your hypothesis be tested without violating ethical standards?
The hypothesis you use will depend on what you are investigating and hoping to find. Some of the main types of hypotheses that you might use include:
- Simple hypothesis : This type of hypothesis suggests there is a relationship between one independent variable and one dependent variable.
- Complex hypothesis : This type suggests a relationship between three or more variables, such as two independent and dependent variables.
- Null hypothesis : This hypothesis suggests no relationship exists between two or more variables.
- Alternative hypothesis : This hypothesis states the opposite of the null hypothesis.
- Statistical hypothesis : This hypothesis uses statistical analysis to evaluate a representative population sample and then generalizes the findings to the larger group.
- Logical hypothesis : This hypothesis assumes a relationship between variables without collecting data or evidence.
A hypothesis often follows a basic format of "If {this happens} then {this will happen}." One way to structure your hypothesis is to describe what will happen to the dependent variable if you change the independent variable .
The basic format might be: "If {these changes are made to a certain independent variable}, then we will observe {a change in a specific dependent variable}."
A few examples of simple hypotheses:
- "Students who eat breakfast will perform better on a math exam than students who do not eat breakfast."
- "Students who experience test anxiety before an English exam will get lower scores than students who do not experience test anxiety."
- "Motorists who talk on the phone while driving will be more likely to make errors on a driving course than those who do not talk on the phone."
- "Children who receive a new reading intervention will have higher reading scores than students who do not receive the intervention."
Examples of a complex hypothesis include:
- "People with high-sugar diets and sedentary activity levels are more likely to develop depression."
- "Younger people who are regularly exposed to green, outdoor areas have better subjective well-being than older adults who have limited exposure to green spaces."
Examples of a null hypothesis include:
- "There is no difference in anxiety levels between people who take St. John's wort supplements and those who do not."
- "There is no difference in scores on a memory recall task between children and adults."
- "There is no difference in aggression levels between children who play first-person shooter games and those who do not."
Examples of an alternative hypothesis:
- "People who take St. John's wort supplements will have less anxiety than those who do not."
- "Adults will perform better on a memory task than children."
- "Children who play first-person shooter games will show higher levels of aggression than children who do not."
Collecting Data on Your Hypothesis
Once a researcher has formed a testable hypothesis, the next step is to select a research design and start collecting data. The research method depends largely on exactly what they are studying. There are two basic types of research methods: descriptive research and experimental research.
Descriptive Research Methods
Descriptive research such as case studies , naturalistic observations , and surveys are often used when conducting an experiment is difficult or impossible. These methods are best used to describe different aspects of a behavior or psychological phenomenon.
Once a researcher has collected data using descriptive methods, a correlational study can examine how the variables are related. This research method might be used to investigate a hypothesis that is difficult to test experimentally.

Experimental Research Methods
Experimental methods are used to demonstrate causal relationships between variables. In an experiment, the researcher systematically manipulates a variable of interest (known as the independent variable) and measures the effect on another variable (known as the dependent variable).
Unlike correlational studies, which can only be used to determine if there is a relationship between two variables, experimental methods can be used to determine the actual nature of the relationship—whether changes in one variable actually cause another to change.
The hypothesis is a critical part of any scientific exploration. It represents what researchers expect to find in a study or experiment. In situations where the hypothesis is unsupported by the research, the research still has value. Such research helps us better understand how different aspects of the natural world relate to one another. It also helps us develop new hypotheses that can then be tested in the future.
Thompson WH, Skau S. On the scope of scientific hypotheses . R Soc Open Sci . 2023;10(8):230607. doi:10.1098/rsos.230607
Taran S, Adhikari NKJ, Fan E. Falsifiability in medicine: what clinicians can learn from Karl Popper [published correction appears in Intensive Care Med. 2021 Jun 17;:]. Intensive Care Med . 2021;47(9):1054-1056. doi:10.1007/s00134-021-06432-z
Eyler AA. Research Methods for Public Health . 1st ed. Springer Publishing Company; 2020. doi:10.1891/9780826182067.0004
Nosek BA, Errington TM. What is replication ? PLoS Biol . 2020;18(3):e3000691. doi:10.1371/journal.pbio.3000691
Aggarwal R, Ranganathan P. Study designs: Part 2 - Descriptive studies . Perspect Clin Res . 2019;10(1):34-36. doi:10.4103/picr.PICR_154_18
Nevid J. Psychology: Concepts and Applications. Wadworth, 2013.
By Kendra Cherry, MSEd Kendra Cherry, MS, is a psychosocial rehabilitation specialist, psychology educator, and author of the "Everything Psychology Book."
Research Hypothesis In Psychology: Types, & Examples
Saul Mcleod, PhD
Editor-in-Chief for Simply Psychology
BSc (Hons) Psychology, MRes, PhD, University of Manchester
Saul Mcleod, PhD., is a qualified psychology teacher with over 18 years of experience in further and higher education. He has been published in peer-reviewed journals, including the Journal of Clinical Psychology.
Learn about our Editorial Process
Olivia Guy-Evans, MSc
Associate Editor for Simply Psychology
BSc (Hons) Psychology, MSc Psychology of Education
Olivia Guy-Evans is a writer and associate editor for Simply Psychology. She has previously worked in healthcare and educational sectors.
On This Page:
A research hypothesis, in its plural form “hypotheses,” is a specific, testable prediction about the anticipated results of a study, established at its outset. It is a key component of the scientific method .
Hypotheses connect theory to data and guide the research process towards expanding scientific understanding
Some key points about hypotheses:
- A hypothesis expresses an expected pattern or relationship. It connects the variables under investigation.
- It is stated in clear, precise terms before any data collection or analysis occurs. This makes the hypothesis testable.
- A hypothesis must be falsifiable. It should be possible, even if unlikely in practice, to collect data that disconfirms rather than supports the hypothesis.
- Hypotheses guide research. Scientists design studies to explicitly evaluate hypotheses about how nature works.
- For a hypothesis to be valid, it must be testable against empirical evidence. The evidence can then confirm or disprove the testable predictions.
- Hypotheses are informed by background knowledge and observation, but go beyond what is already known to propose an explanation of how or why something occurs.
Predictions typically arise from a thorough knowledge of the research literature, curiosity about real-world problems or implications, and integrating this to advance theory. They build on existing literature while providing new insight.
Types of Research Hypotheses
Alternative hypothesis.
The research hypothesis is often called the alternative or experimental hypothesis in experimental research.
It typically suggests a potential relationship between two key variables: the independent variable, which the researcher manipulates, and the dependent variable, which is measured based on those changes.
The alternative hypothesis states a relationship exists between the two variables being studied (one variable affects the other).
A hypothesis is a testable statement or prediction about the relationship between two or more variables. It is a key component of the scientific method. Some key points about hypotheses:
- Important hypotheses lead to predictions that can be tested empirically. The evidence can then confirm or disprove the testable predictions.
In summary, a hypothesis is a precise, testable statement of what researchers expect to happen in a study and why. Hypotheses connect theory to data and guide the research process towards expanding scientific understanding.
An experimental hypothesis predicts what change(s) will occur in the dependent variable when the independent variable is manipulated.
It states that the results are not due to chance and are significant in supporting the theory being investigated.
The alternative hypothesis can be directional, indicating a specific direction of the effect, or non-directional, suggesting a difference without specifying its nature. It’s what researchers aim to support or demonstrate through their study.
Null Hypothesis
The null hypothesis states no relationship exists between the two variables being studied (one variable does not affect the other). There will be no changes in the dependent variable due to manipulating the independent variable.
It states results are due to chance and are not significant in supporting the idea being investigated.
The null hypothesis, positing no effect or relationship, is a foundational contrast to the research hypothesis in scientific inquiry. It establishes a baseline for statistical testing, promoting objectivity by initiating research from a neutral stance.
Many statistical methods are tailored to test the null hypothesis, determining the likelihood of observed results if no true effect exists.
This dual-hypothesis approach provides clarity, ensuring that research intentions are explicit, and fosters consistency across scientific studies, enhancing the standardization and interpretability of research outcomes.
Nondirectional Hypothesis
A non-directional hypothesis, also known as a two-tailed hypothesis, predicts that there is a difference or relationship between two variables but does not specify the direction of this relationship.
It merely indicates that a change or effect will occur without predicting which group will have higher or lower values.
For example, “There is a difference in performance between Group A and Group B” is a non-directional hypothesis.
Directional Hypothesis
A directional (one-tailed) hypothesis predicts the nature of the effect of the independent variable on the dependent variable. It predicts in which direction the change will take place. (i.e., greater, smaller, less, more)
It specifies whether one variable is greater, lesser, or different from another, rather than just indicating that there’s a difference without specifying its nature.
For example, “Exercise increases weight loss” is a directional hypothesis.
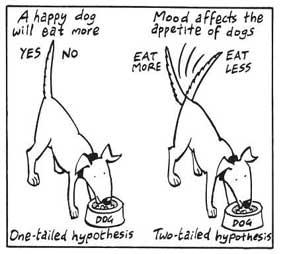
Falsifiability
The Falsification Principle, proposed by Karl Popper , is a way of demarcating science from non-science. It suggests that for a theory or hypothesis to be considered scientific, it must be testable and irrefutable.
Falsifiability emphasizes that scientific claims shouldn’t just be confirmable but should also have the potential to be proven wrong.
It means that there should exist some potential evidence or experiment that could prove the proposition false.
However many confirming instances exist for a theory, it only takes one counter observation to falsify it. For example, the hypothesis that “all swans are white,” can be falsified by observing a black swan.
For Popper, science should attempt to disprove a theory rather than attempt to continually provide evidence to support a research hypothesis.
Can a Hypothesis be Proven?
Hypotheses make probabilistic predictions. They state the expected outcome if a particular relationship exists. However, a study result supporting a hypothesis does not definitively prove it is true.
All studies have limitations. There may be unknown confounding factors or issues that limit the certainty of conclusions. Additional studies may yield different results.
In science, hypotheses can realistically only be supported with some degree of confidence, not proven. The process of science is to incrementally accumulate evidence for and against hypothesized relationships in an ongoing pursuit of better models and explanations that best fit the empirical data. But hypotheses remain open to revision and rejection if that is where the evidence leads.
- Disproving a hypothesis is definitive. Solid disconfirmatory evidence will falsify a hypothesis and require altering or discarding it based on the evidence.
- However, confirming evidence is always open to revision. Other explanations may account for the same results, and additional or contradictory evidence may emerge over time.
We can never 100% prove the alternative hypothesis. Instead, we see if we can disprove, or reject the null hypothesis.
If we reject the null hypothesis, this doesn’t mean that our alternative hypothesis is correct but does support the alternative/experimental hypothesis.
Upon analysis of the results, an alternative hypothesis can be rejected or supported, but it can never be proven to be correct. We must avoid any reference to results proving a theory as this implies 100% certainty, and there is always a chance that evidence may exist which could refute a theory.
How to Write a Hypothesis
- Identify variables . The researcher manipulates the independent variable and the dependent variable is the measured outcome.
- Operationalized the variables being investigated . Operationalization of a hypothesis refers to the process of making the variables physically measurable or testable, e.g. if you are about to study aggression, you might count the number of punches given by participants.
- Decide on a direction for your prediction . If there is evidence in the literature to support a specific effect of the independent variable on the dependent variable, write a directional (one-tailed) hypothesis. If there are limited or ambiguous findings in the literature regarding the effect of the independent variable on the dependent variable, write a non-directional (two-tailed) hypothesis.
- Make it Testable : Ensure your hypothesis can be tested through experimentation or observation. It should be possible to prove it false (principle of falsifiability).
- Clear & concise language . A strong hypothesis is concise (typically one to two sentences long), and formulated using clear and straightforward language, ensuring it’s easily understood and testable.
Consider a hypothesis many teachers might subscribe to: students work better on Monday morning than on Friday afternoon (IV=Day, DV= Standard of work).
Now, if we decide to study this by giving the same group of students a lesson on a Monday morning and a Friday afternoon and then measuring their immediate recall of the material covered in each session, we would end up with the following:
- The alternative hypothesis states that students will recall significantly more information on a Monday morning than on a Friday afternoon.
- The null hypothesis states that there will be no significant difference in the amount recalled on a Monday morning compared to a Friday afternoon. Any difference will be due to chance or confounding factors.
More Examples
- Memory : Participants exposed to classical music during study sessions will recall more items from a list than those who studied in silence.
- Social Psychology : Individuals who frequently engage in social media use will report higher levels of perceived social isolation compared to those who use it infrequently.
- Developmental Psychology : Children who engage in regular imaginative play have better problem-solving skills than those who don’t.
- Clinical Psychology : Cognitive-behavioral therapy will be more effective in reducing symptoms of anxiety over a 6-month period compared to traditional talk therapy.
- Cognitive Psychology : Individuals who multitask between various electronic devices will have shorter attention spans on focused tasks than those who single-task.
- Health Psychology : Patients who practice mindfulness meditation will experience lower levels of chronic pain compared to those who don’t meditate.
- Organizational Psychology : Employees in open-plan offices will report higher levels of stress than those in private offices.
- Behavioral Psychology : Rats rewarded with food after pressing a lever will press it more frequently than rats who receive no reward.

Related Articles
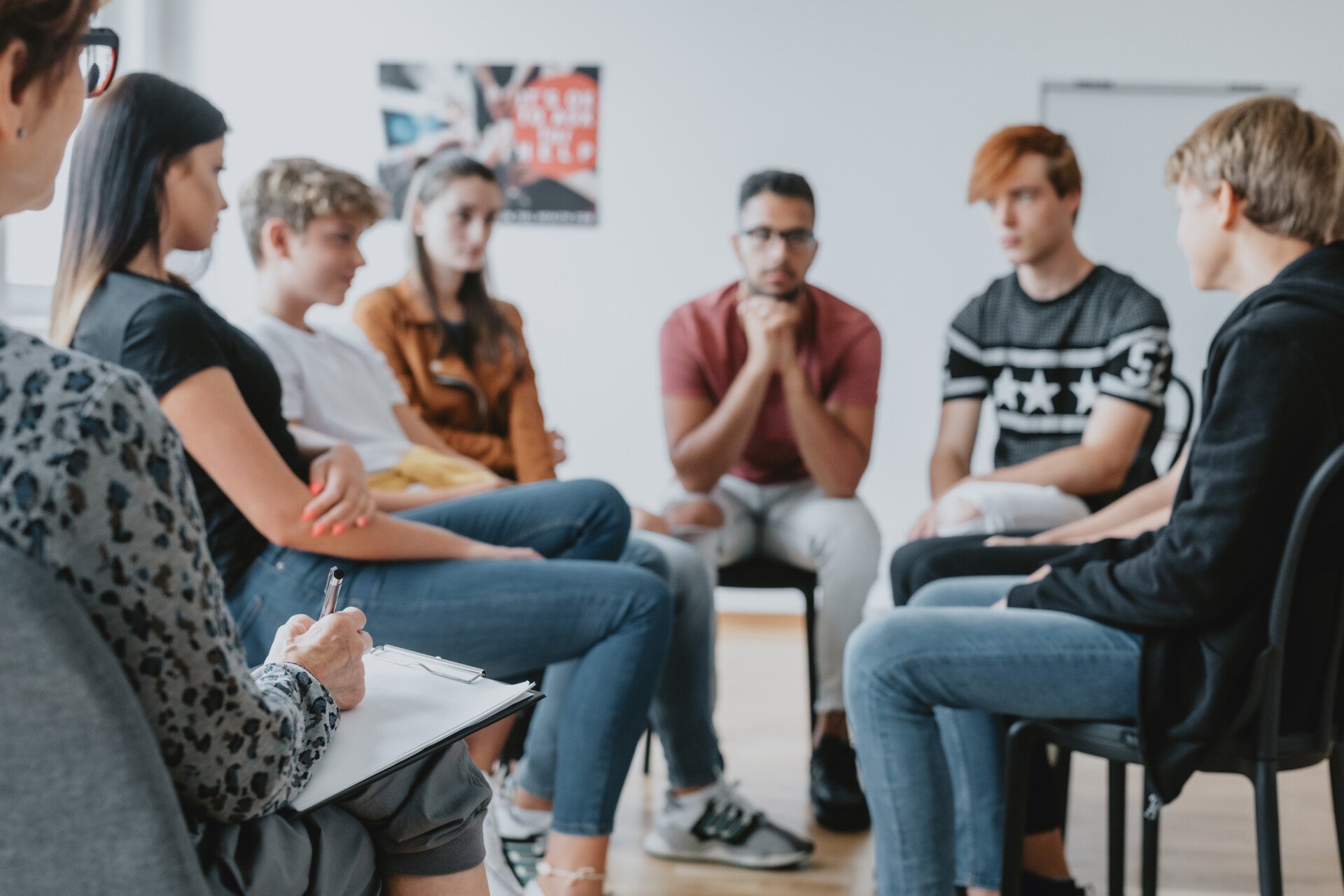
Research Methodology
What Is a Focus Group?
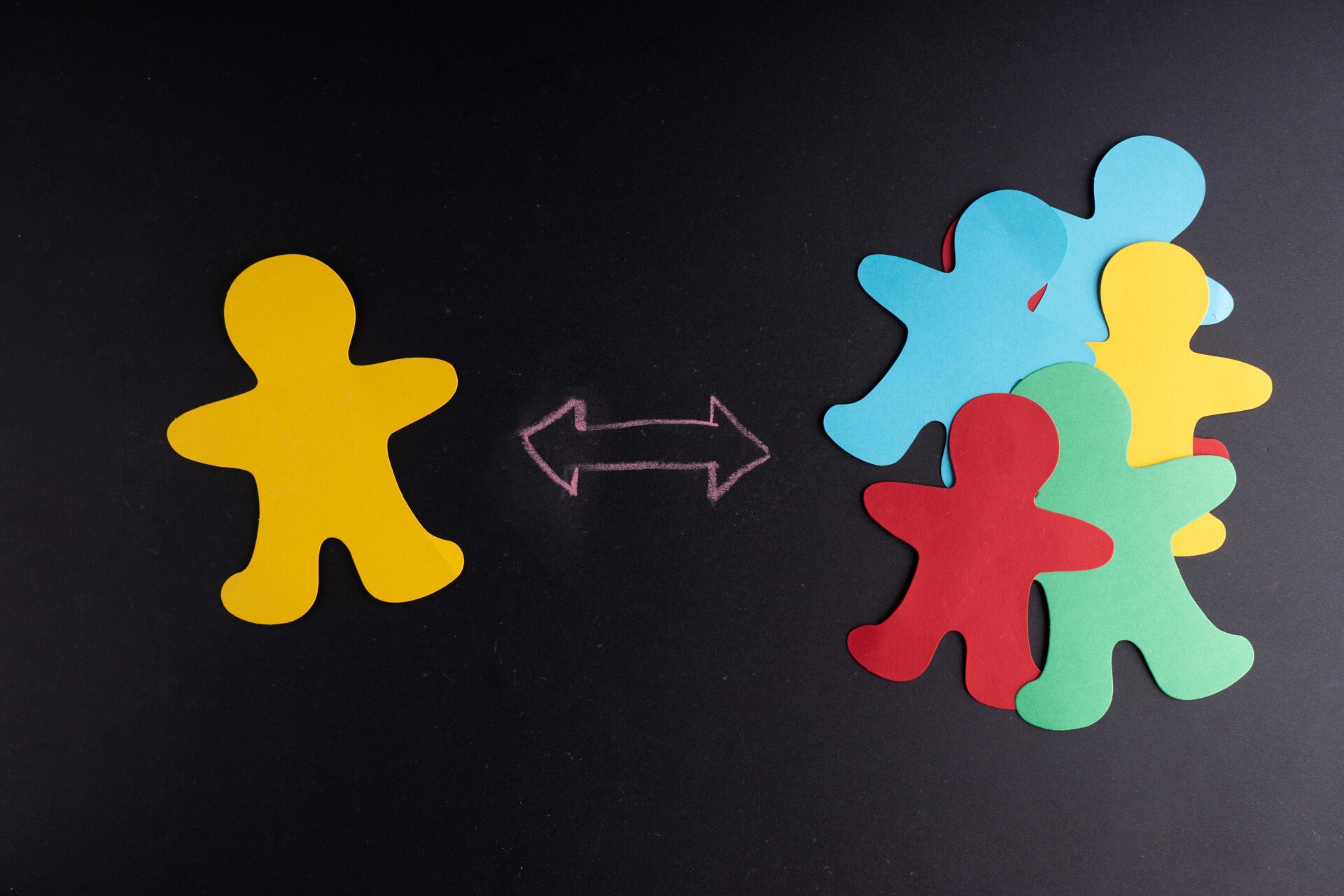
Cross-Cultural Research Methodology In Psychology
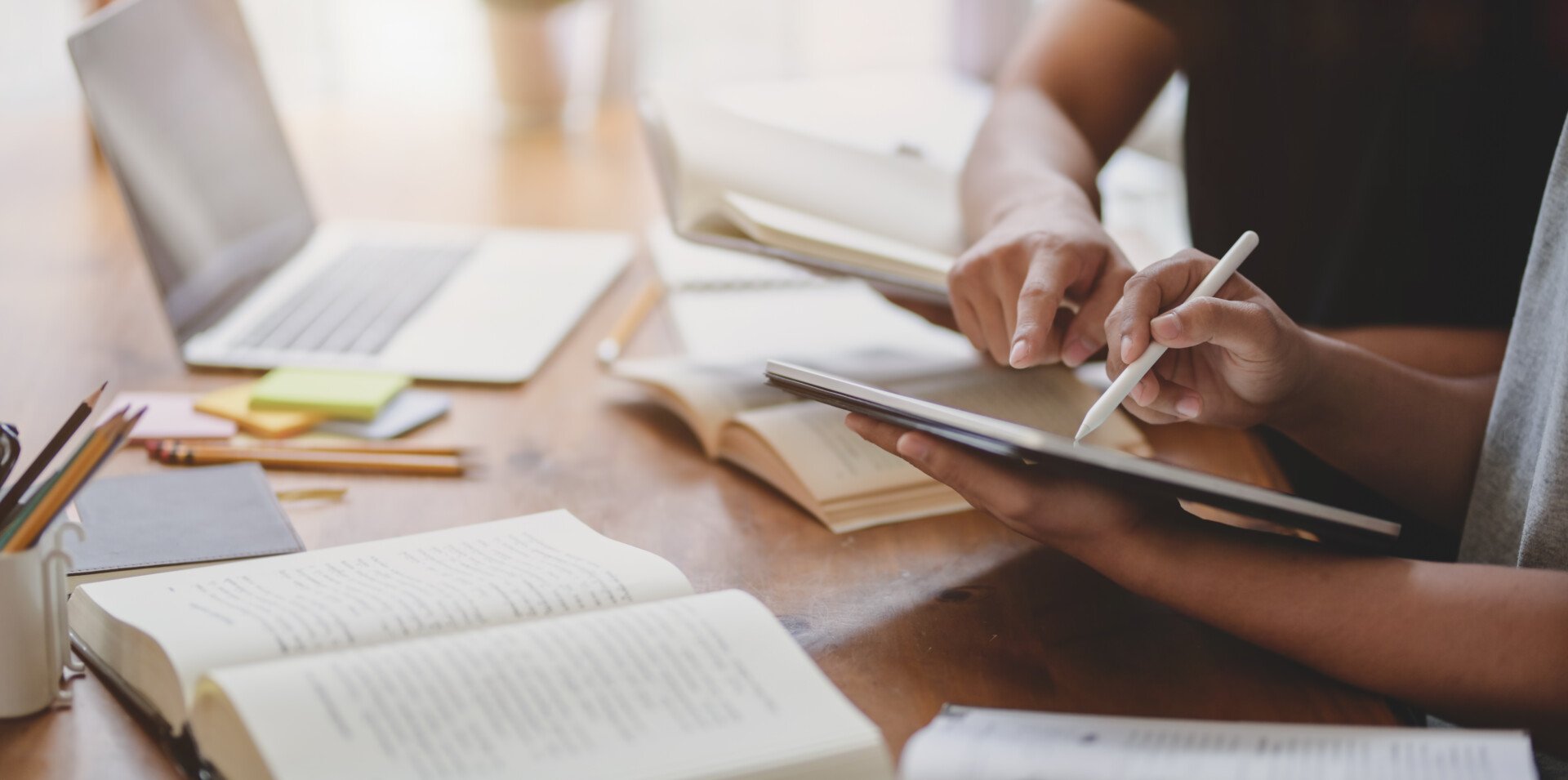
What Is Internal Validity In Research?
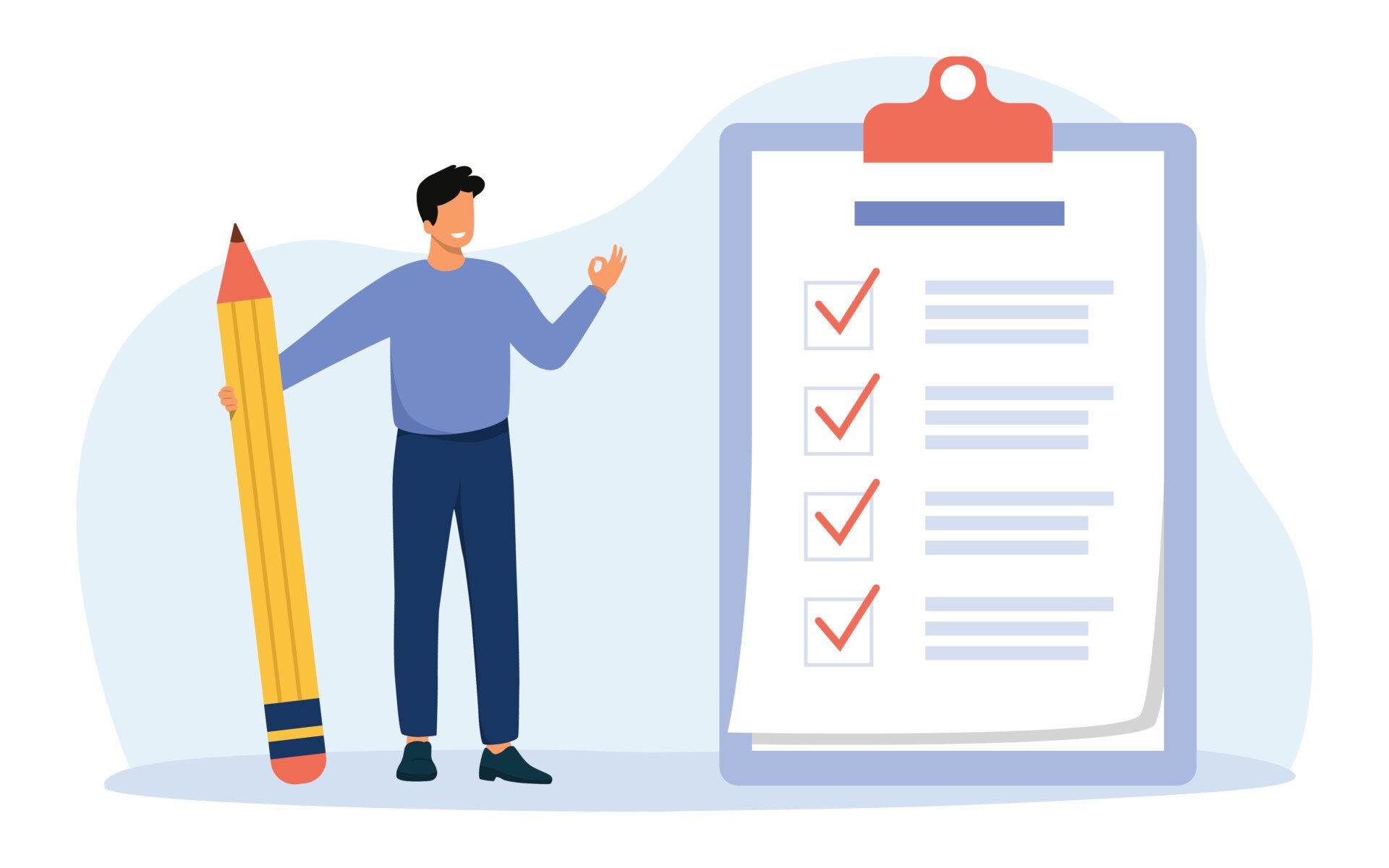
Research Methodology , Statistics
What Is Face Validity In Research? Importance & How To Measure
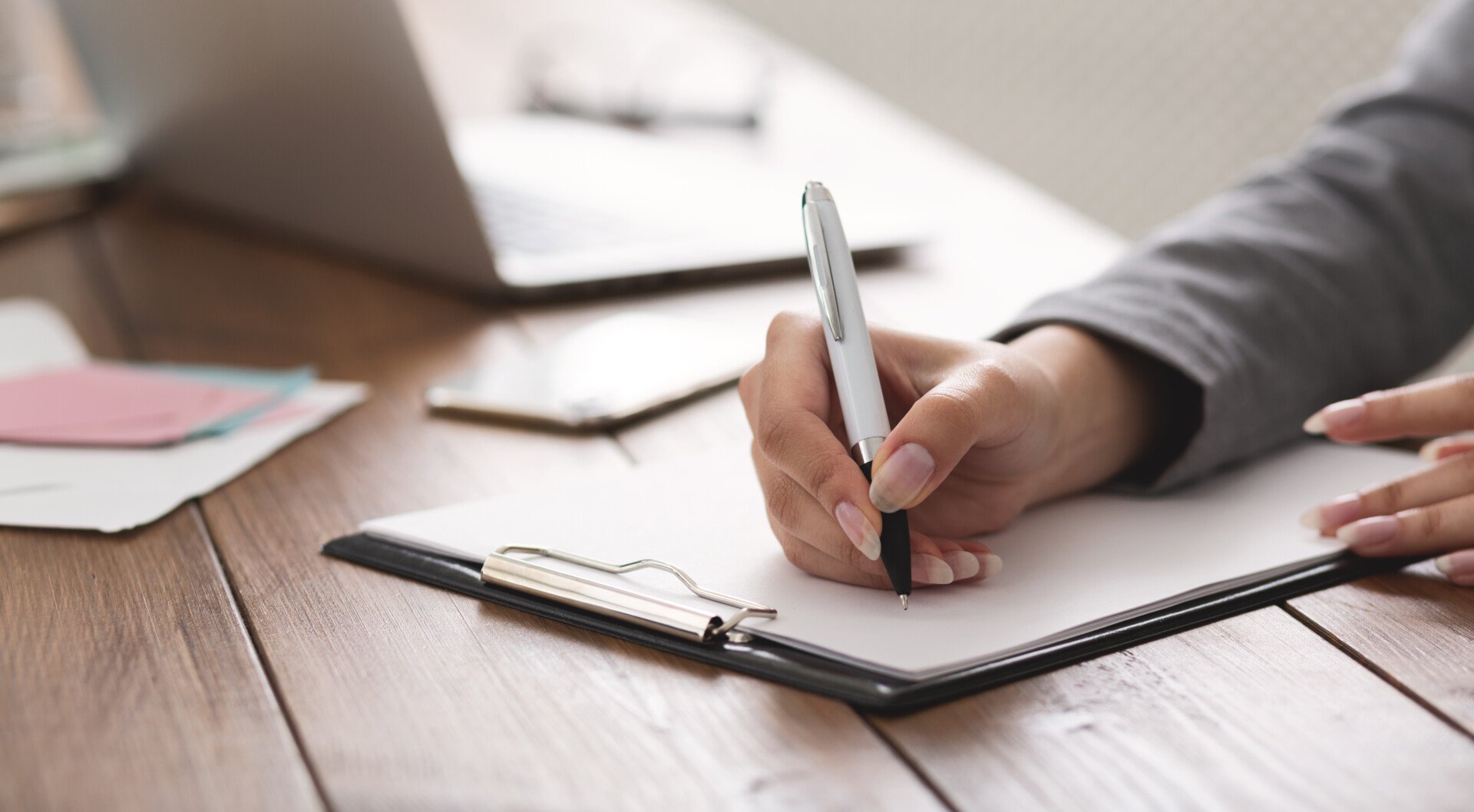
Criterion Validity: Definition & Examples
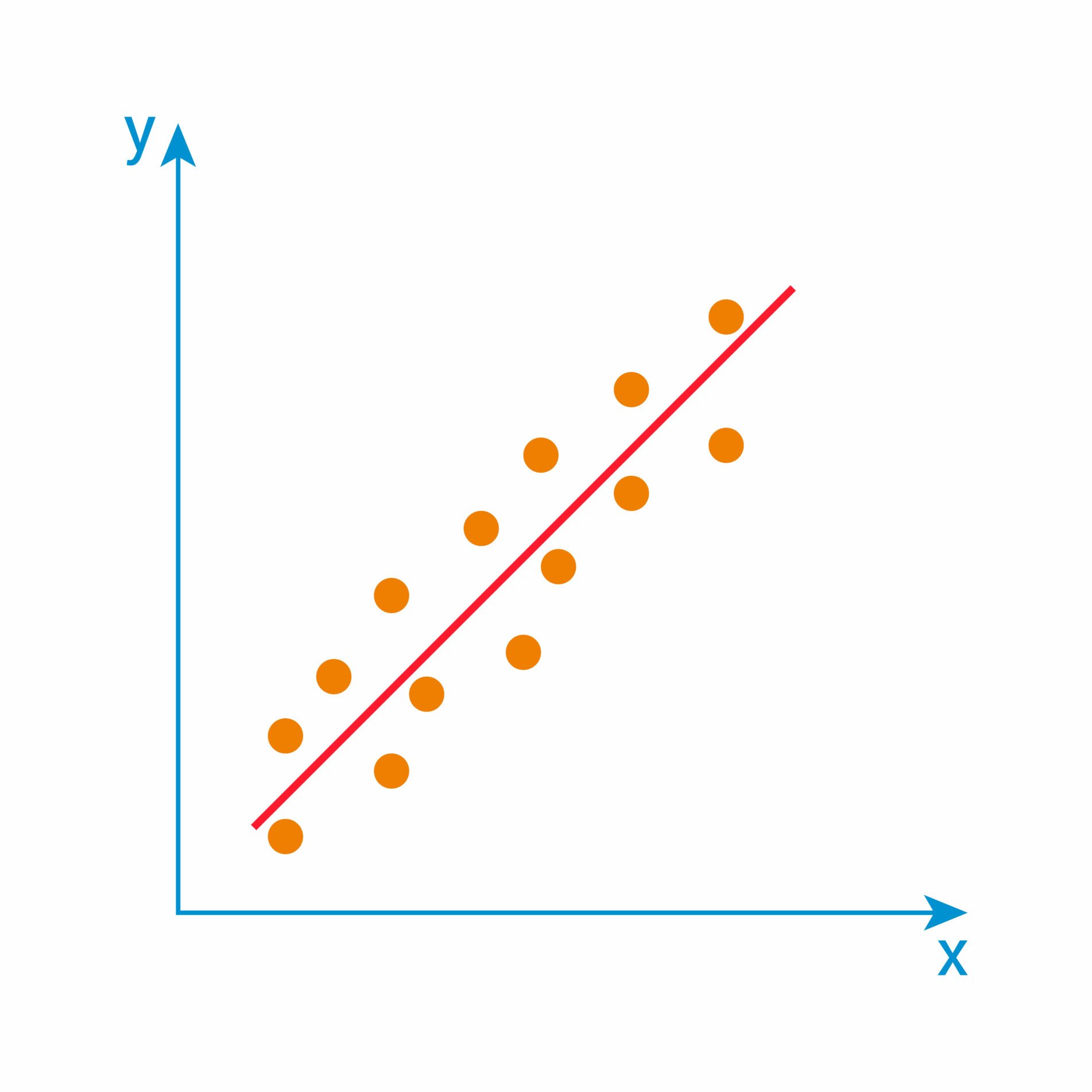
Convergent Validity: Definition and Examples
- Privacy Policy

Home » What is a Hypothesis – Types, Examples and Writing Guide
What is a Hypothesis – Types, Examples and Writing Guide
Table of Contents

Definition:
Hypothesis is an educated guess or proposed explanation for a phenomenon, based on some initial observations or data. It is a tentative statement that can be tested and potentially proven or disproven through further investigation and experimentation.
Hypothesis is often used in scientific research to guide the design of experiments and the collection and analysis of data. It is an essential element of the scientific method, as it allows researchers to make predictions about the outcome of their experiments and to test those predictions to determine their accuracy.
Types of Hypothesis
Types of Hypothesis are as follows:
Research Hypothesis
A research hypothesis is a statement that predicts a relationship between variables. It is usually formulated as a specific statement that can be tested through research, and it is often used in scientific research to guide the design of experiments.
Null Hypothesis
The null hypothesis is a statement that assumes there is no significant difference or relationship between variables. It is often used as a starting point for testing the research hypothesis, and if the results of the study reject the null hypothesis, it suggests that there is a significant difference or relationship between variables.
Alternative Hypothesis
An alternative hypothesis is a statement that assumes there is a significant difference or relationship between variables. It is often used as an alternative to the null hypothesis and is tested against the null hypothesis to determine which statement is more accurate.
Directional Hypothesis
A directional hypothesis is a statement that predicts the direction of the relationship between variables. For example, a researcher might predict that increasing the amount of exercise will result in a decrease in body weight.
Non-directional Hypothesis
A non-directional hypothesis is a statement that predicts the relationship between variables but does not specify the direction. For example, a researcher might predict that there is a relationship between the amount of exercise and body weight, but they do not specify whether increasing or decreasing exercise will affect body weight.
Statistical Hypothesis
A statistical hypothesis is a statement that assumes a particular statistical model or distribution for the data. It is often used in statistical analysis to test the significance of a particular result.
Composite Hypothesis
A composite hypothesis is a statement that assumes more than one condition or outcome. It can be divided into several sub-hypotheses, each of which represents a different possible outcome.
Empirical Hypothesis
An empirical hypothesis is a statement that is based on observed phenomena or data. It is often used in scientific research to develop theories or models that explain the observed phenomena.
Simple Hypothesis
A simple hypothesis is a statement that assumes only one outcome or condition. It is often used in scientific research to test a single variable or factor.
Complex Hypothesis
A complex hypothesis is a statement that assumes multiple outcomes or conditions. It is often used in scientific research to test the effects of multiple variables or factors on a particular outcome.
Applications of Hypothesis
Hypotheses are used in various fields to guide research and make predictions about the outcomes of experiments or observations. Here are some examples of how hypotheses are applied in different fields:
- Science : In scientific research, hypotheses are used to test the validity of theories and models that explain natural phenomena. For example, a hypothesis might be formulated to test the effects of a particular variable on a natural system, such as the effects of climate change on an ecosystem.
- Medicine : In medical research, hypotheses are used to test the effectiveness of treatments and therapies for specific conditions. For example, a hypothesis might be formulated to test the effects of a new drug on a particular disease.
- Psychology : In psychology, hypotheses are used to test theories and models of human behavior and cognition. For example, a hypothesis might be formulated to test the effects of a particular stimulus on the brain or behavior.
- Sociology : In sociology, hypotheses are used to test theories and models of social phenomena, such as the effects of social structures or institutions on human behavior. For example, a hypothesis might be formulated to test the effects of income inequality on crime rates.
- Business : In business research, hypotheses are used to test the validity of theories and models that explain business phenomena, such as consumer behavior or market trends. For example, a hypothesis might be formulated to test the effects of a new marketing campaign on consumer buying behavior.
- Engineering : In engineering, hypotheses are used to test the effectiveness of new technologies or designs. For example, a hypothesis might be formulated to test the efficiency of a new solar panel design.
How to write a Hypothesis
Here are the steps to follow when writing a hypothesis:
Identify the Research Question
The first step is to identify the research question that you want to answer through your study. This question should be clear, specific, and focused. It should be something that can be investigated empirically and that has some relevance or significance in the field.
Conduct a Literature Review
Before writing your hypothesis, it’s essential to conduct a thorough literature review to understand what is already known about the topic. This will help you to identify the research gap and formulate a hypothesis that builds on existing knowledge.
Determine the Variables
The next step is to identify the variables involved in the research question. A variable is any characteristic or factor that can vary or change. There are two types of variables: independent and dependent. The independent variable is the one that is manipulated or changed by the researcher, while the dependent variable is the one that is measured or observed as a result of the independent variable.
Formulate the Hypothesis
Based on the research question and the variables involved, you can now formulate your hypothesis. A hypothesis should be a clear and concise statement that predicts the relationship between the variables. It should be testable through empirical research and based on existing theory or evidence.
Write the Null Hypothesis
The null hypothesis is the opposite of the alternative hypothesis, which is the hypothesis that you are testing. The null hypothesis states that there is no significant difference or relationship between the variables. It is important to write the null hypothesis because it allows you to compare your results with what would be expected by chance.
Refine the Hypothesis
After formulating the hypothesis, it’s important to refine it and make it more precise. This may involve clarifying the variables, specifying the direction of the relationship, or making the hypothesis more testable.
Examples of Hypothesis
Here are a few examples of hypotheses in different fields:
- Psychology : “Increased exposure to violent video games leads to increased aggressive behavior in adolescents.”
- Biology : “Higher levels of carbon dioxide in the atmosphere will lead to increased plant growth.”
- Sociology : “Individuals who grow up in households with higher socioeconomic status will have higher levels of education and income as adults.”
- Education : “Implementing a new teaching method will result in higher student achievement scores.”
- Marketing : “Customers who receive a personalized email will be more likely to make a purchase than those who receive a generic email.”
- Physics : “An increase in temperature will cause an increase in the volume of a gas, assuming all other variables remain constant.”
- Medicine : “Consuming a diet high in saturated fats will increase the risk of developing heart disease.”
Purpose of Hypothesis
The purpose of a hypothesis is to provide a testable explanation for an observed phenomenon or a prediction of a future outcome based on existing knowledge or theories. A hypothesis is an essential part of the scientific method and helps to guide the research process by providing a clear focus for investigation. It enables scientists to design experiments or studies to gather evidence and data that can support or refute the proposed explanation or prediction.
The formulation of a hypothesis is based on existing knowledge, observations, and theories, and it should be specific, testable, and falsifiable. A specific hypothesis helps to define the research question, which is important in the research process as it guides the selection of an appropriate research design and methodology. Testability of the hypothesis means that it can be proven or disproven through empirical data collection and analysis. Falsifiability means that the hypothesis should be formulated in such a way that it can be proven wrong if it is incorrect.
In addition to guiding the research process, the testing of hypotheses can lead to new discoveries and advancements in scientific knowledge. When a hypothesis is supported by the data, it can be used to develop new theories or models to explain the observed phenomenon. When a hypothesis is not supported by the data, it can help to refine existing theories or prompt the development of new hypotheses to explain the phenomenon.
When to use Hypothesis
Here are some common situations in which hypotheses are used:
- In scientific research , hypotheses are used to guide the design of experiments and to help researchers make predictions about the outcomes of those experiments.
- In social science research , hypotheses are used to test theories about human behavior, social relationships, and other phenomena.
- I n business , hypotheses can be used to guide decisions about marketing, product development, and other areas. For example, a hypothesis might be that a new product will sell well in a particular market, and this hypothesis can be tested through market research.
Characteristics of Hypothesis
Here are some common characteristics of a hypothesis:
- Testable : A hypothesis must be able to be tested through observation or experimentation. This means that it must be possible to collect data that will either support or refute the hypothesis.
- Falsifiable : A hypothesis must be able to be proven false if it is not supported by the data. If a hypothesis cannot be falsified, then it is not a scientific hypothesis.
- Clear and concise : A hypothesis should be stated in a clear and concise manner so that it can be easily understood and tested.
- Based on existing knowledge : A hypothesis should be based on existing knowledge and research in the field. It should not be based on personal beliefs or opinions.
- Specific : A hypothesis should be specific in terms of the variables being tested and the predicted outcome. This will help to ensure that the research is focused and well-designed.
- Tentative: A hypothesis is a tentative statement or assumption that requires further testing and evidence to be confirmed or refuted. It is not a final conclusion or assertion.
- Relevant : A hypothesis should be relevant to the research question or problem being studied. It should address a gap in knowledge or provide a new perspective on the issue.
Advantages of Hypothesis
Hypotheses have several advantages in scientific research and experimentation:
- Guides research: A hypothesis provides a clear and specific direction for research. It helps to focus the research question, select appropriate methods and variables, and interpret the results.
- Predictive powe r: A hypothesis makes predictions about the outcome of research, which can be tested through experimentation. This allows researchers to evaluate the validity of the hypothesis and make new discoveries.
- Facilitates communication: A hypothesis provides a common language and framework for scientists to communicate with one another about their research. This helps to facilitate the exchange of ideas and promotes collaboration.
- Efficient use of resources: A hypothesis helps researchers to use their time, resources, and funding efficiently by directing them towards specific research questions and methods that are most likely to yield results.
- Provides a basis for further research: A hypothesis that is supported by data provides a basis for further research and exploration. It can lead to new hypotheses, theories, and discoveries.
- Increases objectivity: A hypothesis can help to increase objectivity in research by providing a clear and specific framework for testing and interpreting results. This can reduce bias and increase the reliability of research findings.
Limitations of Hypothesis
Some Limitations of the Hypothesis are as follows:
- Limited to observable phenomena: Hypotheses are limited to observable phenomena and cannot account for unobservable or intangible factors. This means that some research questions may not be amenable to hypothesis testing.
- May be inaccurate or incomplete: Hypotheses are based on existing knowledge and research, which may be incomplete or inaccurate. This can lead to flawed hypotheses and erroneous conclusions.
- May be biased: Hypotheses may be biased by the researcher’s own beliefs, values, or assumptions. This can lead to selective interpretation of data and a lack of objectivity in research.
- Cannot prove causation: A hypothesis can only show a correlation between variables, but it cannot prove causation. This requires further experimentation and analysis.
- Limited to specific contexts: Hypotheses are limited to specific contexts and may not be generalizable to other situations or populations. This means that results may not be applicable in other contexts or may require further testing.
- May be affected by chance : Hypotheses may be affected by chance or random variation, which can obscure or distort the true relationship between variables.
About the author
Muhammad Hassan
Researcher, Academic Writer, Web developer
You may also like

Data Collection – Methods Types and Examples

Delimitations in Research – Types, Examples and...

Research Process – Steps, Examples and Tips

Research Design – Types, Methods and Examples

Institutional Review Board – Application Sample...

Evaluating Research – Process, Examples and...
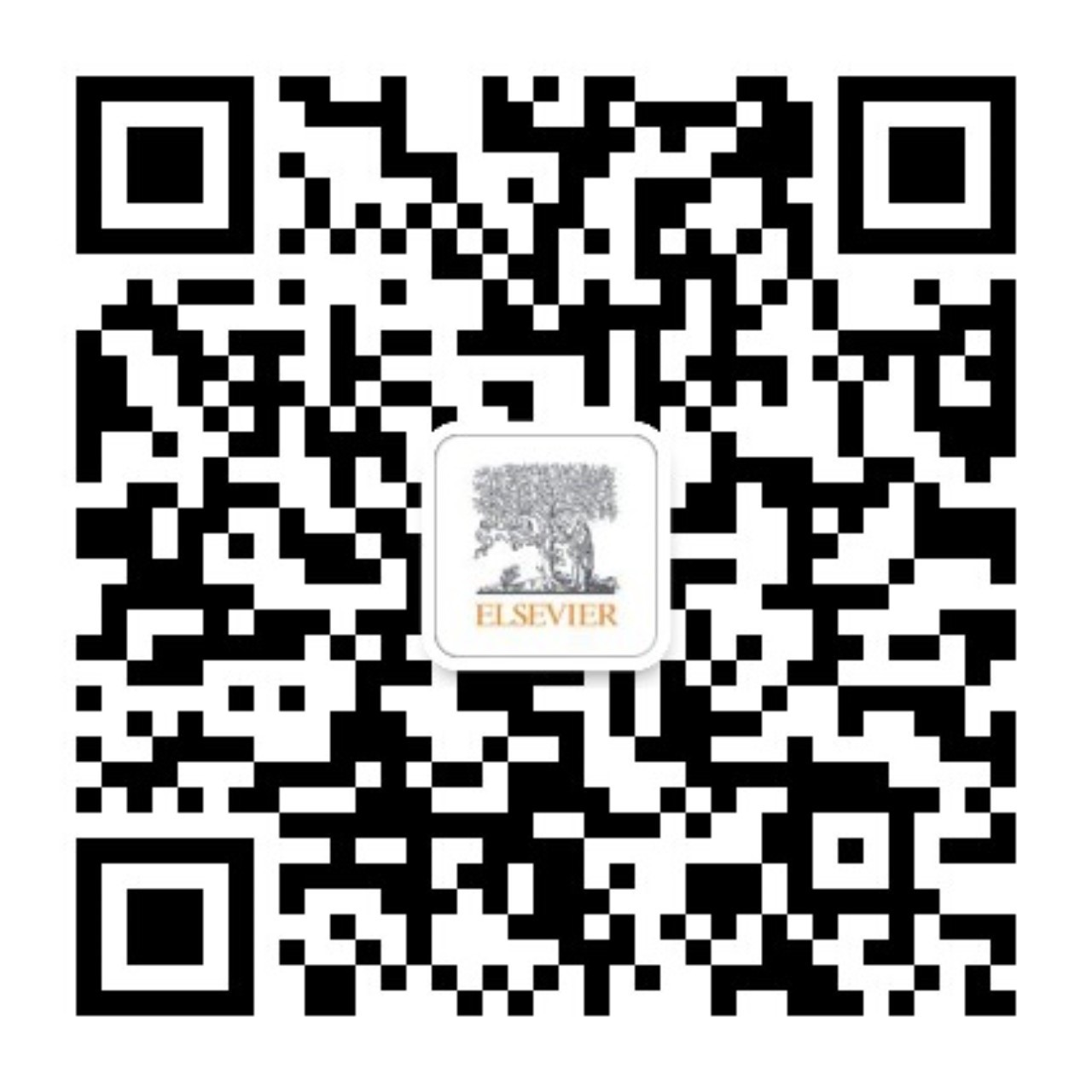
- Manuscript Preparation
What is and How to Write a Good Hypothesis in Research?
- 4 minute read
- 312.8K views
Table of Contents
One of the most important aspects of conducting research is constructing a strong hypothesis. But what makes a hypothesis in research effective? In this article, we’ll look at the difference between a hypothesis and a research question, as well as the elements of a good hypothesis in research. We’ll also include some examples of effective hypotheses, and what pitfalls to avoid.
What is a Hypothesis in Research?
Simply put, a hypothesis is a research question that also includes the predicted or expected result of the research. Without a hypothesis, there can be no basis for a scientific or research experiment. As such, it is critical that you carefully construct your hypothesis by being deliberate and thorough, even before you set pen to paper. Unless your hypothesis is clearly and carefully constructed, any flaw can have an adverse, and even grave, effect on the quality of your experiment and its subsequent results.
Research Question vs Hypothesis
It’s easy to confuse research questions with hypotheses, and vice versa. While they’re both critical to the Scientific Method, they have very specific differences. Primarily, a research question, just like a hypothesis, is focused and concise. But a hypothesis includes a prediction based on the proposed research, and is designed to forecast the relationship of and between two (or more) variables. Research questions are open-ended, and invite debate and discussion, while hypotheses are closed, e.g. “The relationship between A and B will be C.”
A hypothesis is generally used if your research topic is fairly well established, and you are relatively certain about the relationship between the variables that will be presented in your research. Since a hypothesis is ideally suited for experimental studies, it will, by its very existence, affect the design of your experiment. The research question is typically used for new topics that have not yet been researched extensively. Here, the relationship between different variables is less known. There is no prediction made, but there may be variables explored. The research question can be casual in nature, simply trying to understand if a relationship even exists, descriptive or comparative.
How to Write Hypothesis in Research
Writing an effective hypothesis starts before you even begin to type. Like any task, preparation is key, so you start first by conducting research yourself, and reading all you can about the topic that you plan to research. From there, you’ll gain the knowledge you need to understand where your focus within the topic will lie.
Remember that a hypothesis is a prediction of the relationship that exists between two or more variables. Your job is to write a hypothesis, and design the research, to “prove” whether or not your prediction is correct. A common pitfall is to use judgments that are subjective and inappropriate for the construction of a hypothesis. It’s important to keep the focus and language of your hypothesis objective.
An effective hypothesis in research is clearly and concisely written, and any terms or definitions clarified and defined. Specific language must also be used to avoid any generalities or assumptions.
Use the following points as a checklist to evaluate the effectiveness of your research hypothesis:
- Predicts the relationship and outcome
- Simple and concise – avoid wordiness
- Clear with no ambiguity or assumptions about the readers’ knowledge
- Observable and testable results
- Relevant and specific to the research question or problem
Research Hypothesis Example
Perhaps the best way to evaluate whether or not your hypothesis is effective is to compare it to those of your colleagues in the field. There is no need to reinvent the wheel when it comes to writing a powerful research hypothesis. As you’re reading and preparing your hypothesis, you’ll also read other hypotheses. These can help guide you on what works, and what doesn’t, when it comes to writing a strong research hypothesis.
Here are a few generic examples to get you started.
Eating an apple each day, after the age of 60, will result in a reduction of frequency of physician visits.
Budget airlines are more likely to receive more customer complaints. A budget airline is defined as an airline that offers lower fares and fewer amenities than a traditional full-service airline. (Note that the term “budget airline” is included in the hypothesis.
Workplaces that offer flexible working hours report higher levels of employee job satisfaction than workplaces with fixed hours.
Each of the above examples are specific, observable and measurable, and the statement of prediction can be verified or shown to be false by utilizing standard experimental practices. It should be noted, however, that often your hypothesis will change as your research progresses.
Language Editing Plus
Elsevier’s Language Editing Plus service can help ensure that your research hypothesis is well-designed, and articulates your research and conclusions. Our most comprehensive editing package, you can count on a thorough language review by native-English speakers who are PhDs or PhD candidates. We’ll check for effective logic and flow of your manuscript, as well as document formatting for your chosen journal, reference checks, and much more.
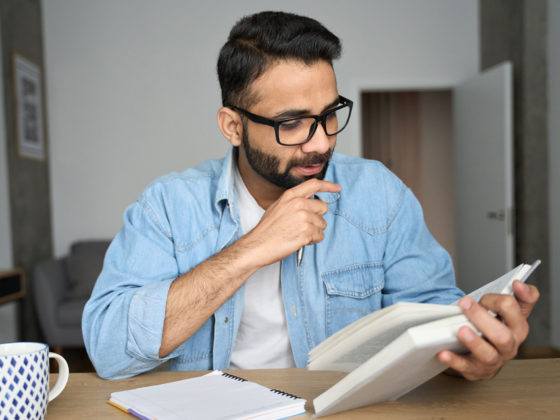
- Research Process
Systematic Literature Review or Literature Review?
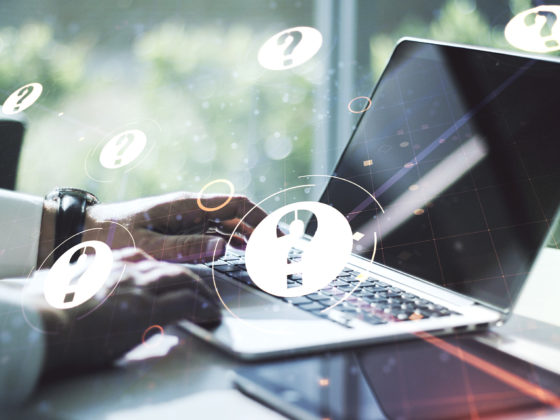
What is a Problem Statement? [with examples]
You may also like.
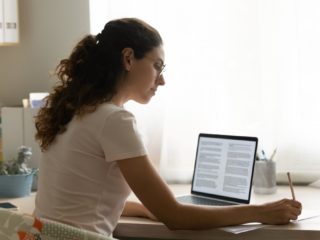
Make Hook, Line, and Sinker: The Art of Crafting Engaging Introductions
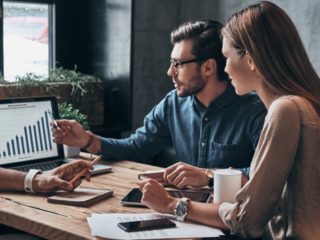
Can Describing Study Limitations Improve the Quality of Your Paper?
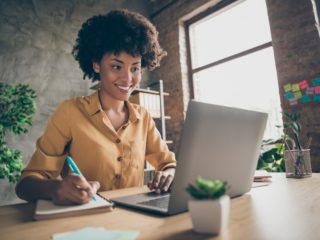
A Guide to Crafting Shorter, Impactful Sentences in Academic Writing
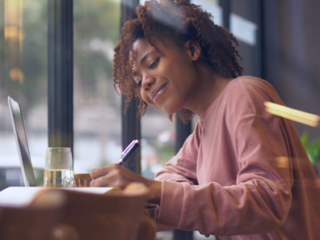
6 Steps to Write an Excellent Discussion in Your Manuscript
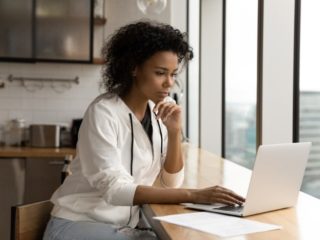
How to Write Clear and Crisp Civil Engineering Papers? Here are 5 Key Tips to Consider
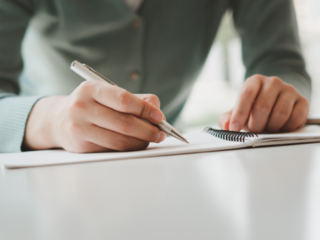
The Clear Path to An Impactful Paper: ②
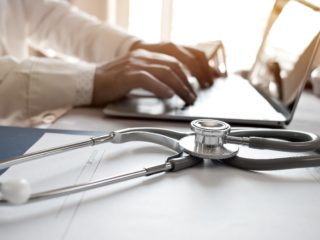
The Essentials of Writing to Communicate Research in Medicine
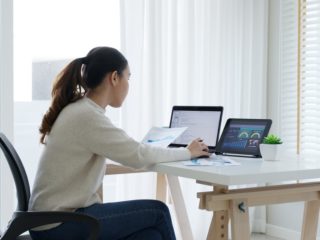
Changing Lines: Sentence Patterns in Academic Writing
Input your search keywords and press Enter.

How to Develop a Good Research Hypothesis
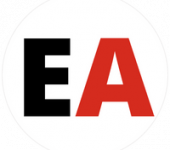
The story of a research study begins by asking a question. Researchers all around the globe are asking curious questions and formulating research hypothesis. However, whether the research study provides an effective conclusion depends on how well one develops a good research hypothesis. Research hypothesis examples could help researchers get an idea as to how to write a good research hypothesis.
This blog will help you understand what is a research hypothesis, its characteristics and, how to formulate a research hypothesis
Table of Contents
What is Hypothesis?
Hypothesis is an assumption or an idea proposed for the sake of argument so that it can be tested. It is a precise, testable statement of what the researchers predict will be outcome of the study. Hypothesis usually involves proposing a relationship between two variables: the independent variable (what the researchers change) and the dependent variable (what the research measures).
What is a Research Hypothesis?
Research hypothesis is a statement that introduces a research question and proposes an expected result. It is an integral part of the scientific method that forms the basis of scientific experiments. Therefore, you need to be careful and thorough when building your research hypothesis. A minor flaw in the construction of your hypothesis could have an adverse effect on your experiment. In research, there is a convention that the hypothesis is written in two forms, the null hypothesis, and the alternative hypothesis (called the experimental hypothesis when the method of investigation is an experiment).
Characteristics of a Good Research Hypothesis
As the hypothesis is specific, there is a testable prediction about what you expect to happen in a study. You may consider drawing hypothesis from previously published research based on the theory.
A good research hypothesis involves more effort than just a guess. In particular, your hypothesis may begin with a question that could be further explored through background research.
To help you formulate a promising research hypothesis, you should ask yourself the following questions:
- Is the language clear and focused?
- What is the relationship between your hypothesis and your research topic?
- Is your hypothesis testable? If yes, then how?
- What are the possible explanations that you might want to explore?
- Does your hypothesis include both an independent and dependent variable?
- Can you manipulate your variables without hampering the ethical standards?
- Does your research predict the relationship and outcome?
- Is your research simple and concise (avoids wordiness)?
- Is it clear with no ambiguity or assumptions about the readers’ knowledge
- Is your research observable and testable results?
- Is it relevant and specific to the research question or problem?
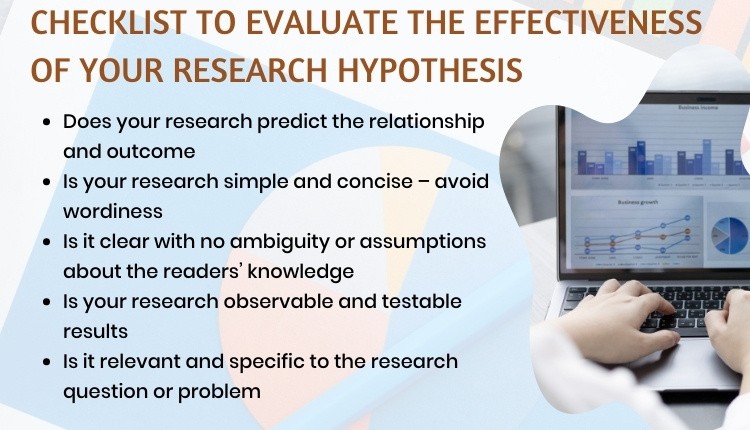
The questions listed above can be used as a checklist to make sure your hypothesis is based on a solid foundation. Furthermore, it can help you identify weaknesses in your hypothesis and revise it if necessary.
Source: Educational Hub
How to formulate a research hypothesis.
A testable hypothesis is not a simple statement. It is rather an intricate statement that needs to offer a clear introduction to a scientific experiment, its intentions, and the possible outcomes. However, there are some important things to consider when building a compelling hypothesis.
1. State the problem that you are trying to solve.
Make sure that the hypothesis clearly defines the topic and the focus of the experiment.
2. Try to write the hypothesis as an if-then statement.
Follow this template: If a specific action is taken, then a certain outcome is expected.
3. Define the variables
Independent variables are the ones that are manipulated, controlled, or changed. Independent variables are isolated from other factors of the study.
Dependent variables , as the name suggests are dependent on other factors of the study. They are influenced by the change in independent variable.
4. Scrutinize the hypothesis
Evaluate assumptions, predictions, and evidence rigorously to refine your understanding.
Types of Research Hypothesis
The types of research hypothesis are stated below:
1. Simple Hypothesis
It predicts the relationship between a single dependent variable and a single independent variable.
2. Complex Hypothesis
It predicts the relationship between two or more independent and dependent variables.
3. Directional Hypothesis
It specifies the expected direction to be followed to determine the relationship between variables and is derived from theory. Furthermore, it implies the researcher’s intellectual commitment to a particular outcome.
4. Non-directional Hypothesis
It does not predict the exact direction or nature of the relationship between the two variables. The non-directional hypothesis is used when there is no theory involved or when findings contradict previous research.
5. Associative and Causal Hypothesis
The associative hypothesis defines interdependency between variables. A change in one variable results in the change of the other variable. On the other hand, the causal hypothesis proposes an effect on the dependent due to manipulation of the independent variable.
6. Null Hypothesis
Null hypothesis states a negative statement to support the researcher’s findings that there is no relationship between two variables. There will be no changes in the dependent variable due the manipulation of the independent variable. Furthermore, it states results are due to chance and are not significant in terms of supporting the idea being investigated.
7. Alternative Hypothesis
It states that there is a relationship between the two variables of the study and that the results are significant to the research topic. An experimental hypothesis predicts what changes will take place in the dependent variable when the independent variable is manipulated. Also, it states that the results are not due to chance and that they are significant in terms of supporting the theory being investigated.
Research Hypothesis Examples of Independent and Dependent Variables
Research Hypothesis Example 1 The greater number of coal plants in a region (independent variable) increases water pollution (dependent variable). If you change the independent variable (building more coal factories), it will change the dependent variable (amount of water pollution).
Research Hypothesis Example 2 What is the effect of diet or regular soda (independent variable) on blood sugar levels (dependent variable)? If you change the independent variable (the type of soda you consume), it will change the dependent variable (blood sugar levels)
You should not ignore the importance of the above steps. The validity of your experiment and its results rely on a robust testable hypothesis. Developing a strong testable hypothesis has few advantages, it compels us to think intensely and specifically about the outcomes of a study. Consequently, it enables us to understand the implication of the question and the different variables involved in the study. Furthermore, it helps us to make precise predictions based on prior research. Hence, forming a hypothesis would be of great value to the research. Here are some good examples of testable hypotheses.
More importantly, you need to build a robust testable research hypothesis for your scientific experiments. A testable hypothesis is a hypothesis that can be proved or disproved as a result of experimentation.
Importance of a Testable Hypothesis
To devise and perform an experiment using scientific method, you need to make sure that your hypothesis is testable. To be considered testable, some essential criteria must be met:
- There must be a possibility to prove that the hypothesis is true.
- There must be a possibility to prove that the hypothesis is false.
- The results of the hypothesis must be reproducible.
Without these criteria, the hypothesis and the results will be vague. As a result, the experiment will not prove or disprove anything significant.
What are your experiences with building hypotheses for scientific experiments? What challenges did you face? How did you overcome these challenges? Please share your thoughts with us in the comments section.
Frequently Asked Questions
The steps to write a research hypothesis are: 1. Stating the problem: Ensure that the hypothesis defines the research problem 2. Writing a hypothesis as an 'if-then' statement: Include the action and the expected outcome of your study by following a ‘if-then’ structure. 3. Defining the variables: Define the variables as Dependent or Independent based on their dependency to other factors. 4. Scrutinizing the hypothesis: Identify the type of your hypothesis
Hypothesis testing is a statistical tool which is used to make inferences about a population data to draw conclusions for a particular hypothesis.
Hypothesis in statistics is a formal statement about the nature of a population within a structured framework of a statistical model. It is used to test an existing hypothesis by studying a population.
Research hypothesis is a statement that introduces a research question and proposes an expected result. It forms the basis of scientific experiments.
The different types of hypothesis in research are: • Null hypothesis: Null hypothesis is a negative statement to support the researcher’s findings that there is no relationship between two variables. • Alternate hypothesis: Alternate hypothesis predicts the relationship between the two variables of the study. • Directional hypothesis: Directional hypothesis specifies the expected direction to be followed to determine the relationship between variables. • Non-directional hypothesis: Non-directional hypothesis does not predict the exact direction or nature of the relationship between the two variables. • Simple hypothesis: Simple hypothesis predicts the relationship between a single dependent variable and a single independent variable. • Complex hypothesis: Complex hypothesis predicts the relationship between two or more independent and dependent variables. • Associative and casual hypothesis: Associative and casual hypothesis predicts the relationship between two or more independent and dependent variables. • Empirical hypothesis: Empirical hypothesis can be tested via experiments and observation. • Statistical hypothesis: A statistical hypothesis utilizes statistical models to draw conclusions about broader populations.

Wow! You really simplified your explanation that even dummies would find it easy to comprehend. Thank you so much.
Thanks a lot for your valuable guidance.
I enjoy reading the post. Hypotheses are actually an intrinsic part in a study. It bridges the research question and the methodology of the study.
Useful piece!
This is awesome.Wow.
It very interesting to read the topic, can you guide me any specific example of hypothesis process establish throw the Demand and supply of the specific product in market
Nicely explained
It is really a useful for me Kindly give some examples of hypothesis
It was a well explained content ,can you please give me an example with the null and alternative hypothesis illustrated
clear and concise. thanks.
So Good so Amazing
Good to learn
Thanks a lot for explaining to my level of understanding
Explained well and in simple terms. Quick read! Thank you
It awesome. It has really positioned me in my research project
Rate this article Cancel Reply
Your email address will not be published.
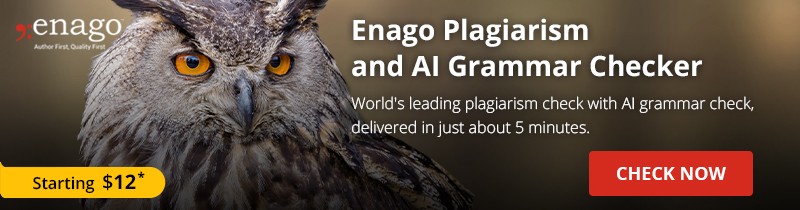
Enago Academy's Most Popular Articles
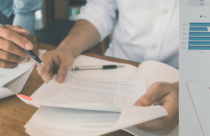
- Reporting Research
Choosing the Right Analytical Approach: Thematic analysis vs. content analysis for data interpretation
In research, choosing the right approach to understand data is crucial for deriving meaningful insights.…
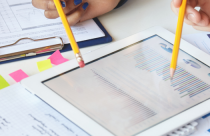
Comparing Cross Sectional and Longitudinal Studies: 5 steps for choosing the right approach
The process of choosing the right research design can put ourselves at the crossroads of…
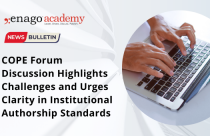
- Industry News
COPE Forum Discussion Highlights Challenges and Urges Clarity in Institutional Authorship Standards
The COPE forum discussion held in December 2023 initiated with a fundamental question — is…
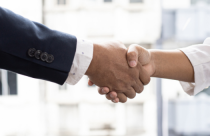
- Career Corner
Unlocking the Power of Networking in Academic Conferences
Embarking on your first academic conference experience? Fear not, we got you covered! Academic conferences…
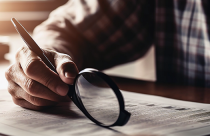
Research Recommendations – Guiding policy-makers for evidence-based decision making
Research recommendations play a crucial role in guiding scholars and researchers toward fruitful avenues of…
Choosing the Right Analytical Approach: Thematic analysis vs. content analysis for…
Comparing Cross Sectional and Longitudinal Studies: 5 steps for choosing the right…
How to Design Effective Research Questionnaires for Robust Findings

Sign-up to read more
Subscribe for free to get unrestricted access to all our resources on research writing and academic publishing including:
- 2000+ blog articles
- 50+ Webinars
- 10+ Expert podcasts
- 50+ Infographics
- 10+ Checklists
- Research Guides
We hate spam too. We promise to protect your privacy and never spam you.
I am looking for Editing/ Proofreading services for my manuscript Tentative date of next journal submission:
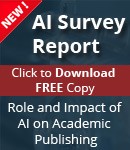
As a researcher, what do you consider most when choosing an image manipulation detector?
2.1 Why Is Research Important?
Learning objectives.
By the end of this section, you will be able to:
- Explain how scientific research addresses questions about behavior
- Discuss how scientific research guides public policy
- Appreciate how scientific research can be important in making personal decisions
Scientific research is a critical tool for successfully navigating our complex world. Without it, we would be forced to rely solely on intuition, other people’s authority, and blind luck. While many of us feel confident in our abilities to decipher and interact with the world around us, history is filled with examples of how very wrong we can be when we fail to recognize the need for evidence in supporting claims. At various times in history, we would have been certain that the sun revolved around a flat earth, that the earth’s continents did not move, and that mental illness was caused by possession ( Figure 2.2 ). It is through systematic scientific research that we divest ourselves of our preconceived notions and superstitions and gain an objective understanding of ourselves and our world.
The goal of all scientists is to better understand the world around them. Psychologists focus their attention on understanding behavior, as well as the cognitive (mental) and physiological (body) processes that underlie behavior. In contrast to other methods that people use to understand the behavior of others, such as intuition and personal experience, the hallmark of scientific research is that there is evidence to support a claim. Scientific knowledge is empirical : It is grounded in objective, tangible evidence that can be observed time and time again, regardless of who is observing.
While behavior is observable, the mind is not. If someone is crying, we can see behavior. However, the reason for the behavior is more difficult to determine. Is the person crying due to being sad, in pain, or happy? Sometimes we can learn the reason for someone’s behavior by simply asking a question, like “Why are you crying?” However, there are situations in which an individual is either uncomfortable or unwilling to answer the question honestly, or is incapable of answering. For example, infants would not be able to explain why they are crying. In such circumstances, the psychologist must be creative in finding ways to better understand behavior. This chapter explores how scientific knowledge is generated, and how important that knowledge is in forming decisions in our personal lives and in the public domain.
Use of Research Information
Trying to determine which theories are and are not accepted by the scientific community can be difficult, especially in an area of research as broad as psychology. More than ever before, we have an incredible amount of information at our fingertips, and a simple internet search on any given research topic might result in a number of contradictory studies. In these cases, we are witnessing the scientific community going through the process of reaching a consensus, and it could be quite some time before a consensus emerges. For example, the explosion in our use of technology has led researchers to question whether this ultimately helps or hinders us. The use and implementation of technology in educational settings has become widespread over the last few decades. Researchers are coming to different conclusions regarding the use of technology. To illustrate this point, a study investigating a smartphone app targeting surgery residents (graduate students in surgery training) found that the use of this app can increase student engagement and raise test scores (Shaw & Tan, 2015). Conversely, another study found that the use of technology in undergraduate student populations had negative impacts on sleep, communication, and time management skills (Massimini & Peterson, 2009). Until sufficient amounts of research have been conducted, there will be no clear consensus on the effects that technology has on a student's acquisition of knowledge, study skills, and mental health.
In the meantime, we should strive to think critically about the information we encounter by exercising a degree of healthy skepticism. When someone makes a claim, we should examine the claim from a number of different perspectives: what is the expertise of the person making the claim, what might they gain if the claim is valid, does the claim seem justified given the evidence, and what do other researchers think of the claim? This is especially important when we consider how much information in advertising campaigns and on the internet claims to be based on “scientific evidence” when in actuality it is a belief or perspective of just a few individuals trying to sell a product or draw attention to their perspectives.
We should be informed consumers of the information made available to us because decisions based on this information have significant consequences. One such consequence can be seen in politics and public policy. Imagine that you have been elected as the governor of your state. One of your responsibilities is to manage the state budget and determine how to best spend your constituents’ tax dollars. As the new governor, you need to decide whether to continue funding early intervention programs. These programs are designed to help children who come from low-income backgrounds, have special needs, or face other disadvantages. These programs may involve providing a wide variety of services to maximize the children's development and position them for optimal levels of success in school and later in life (Blann, 2005). While such programs sound appealing, you would want to be sure that they also proved effective before investing additional money in these programs. Fortunately, psychologists and other scientists have conducted vast amounts of research on such programs and, in general, the programs are found to be effective (Neil & Christensen, 2009; Peters-Scheffer, Didden, Korzilius, & Sturmey, 2011). While not all programs are equally effective, and the short-term effects of many such programs are more pronounced, there is reason to believe that many of these programs produce long-term benefits for participants (Barnett, 2011). If you are committed to being a good steward of taxpayer money, you would want to look at research. Which programs are most effective? What characteristics of these programs make them effective? Which programs promote the best outcomes? After examining the research, you would be best equipped to make decisions about which programs to fund.
Link to Learning
Watch this video about early childhood program effectiveness to learn how scientists evaluate effectiveness and how best to invest money into programs that are most effective.
Ultimately, it is not just politicians who can benefit from using research in guiding their decisions. We all might look to research from time to time when making decisions in our lives. Imagine that your sister, Maria, expresses concern about her two-year-old child, Umberto. Umberto does not speak as much or as clearly as the other children in his daycare or others in the family. Umberto's pediatrician undertakes some screening and recommends an evaluation by a speech pathologist, but does not refer Maria to any other specialists. Maria is concerned that Umberto's speech delays are signs of a developmental disorder, but Umberto's pediatrician does not; she sees indications of differences in Umberto's jaw and facial muscles. Hearing this, you do some internet searches, but you are overwhelmed by the breadth of information and the wide array of sources. You see blog posts, top-ten lists, advertisements from healthcare providers, and recommendations from several advocacy organizations. Why are there so many sites? Which are based in research, and which are not?
In the end, research is what makes the difference between facts and opinions. Facts are observable realities, and opinions are personal judgments, conclusions, or attitudes that may or may not be accurate. In the scientific community, facts can be established only using evidence collected through empirical research.
NOTABLE RESEARCHERS
Psychological research has a long history involving important figures from diverse backgrounds. While the introductory chapter discussed several researchers who made significant contributions to the discipline, there are many more individuals who deserve attention in considering how psychology has advanced as a science through their work ( Figure 2.3 ). For instance, Margaret Floy Washburn (1871–1939) was the first woman to earn a PhD in psychology. Her research focused on animal behavior and cognition (Margaret Floy Washburn, PhD, n.d.). Mary Whiton Calkins (1863–1930) was a preeminent first-generation American psychologist who opposed the behaviorist movement, conducted significant research into memory, and established one of the earliest experimental psychology labs in the United States (Mary Whiton Calkins, n.d.).
Francis Sumner (1895–1954) was the first African American to receive a PhD in psychology in 1920. His dissertation focused on issues related to psychoanalysis. Sumner also had research interests in racial bias and educational justice. Sumner was one of the founders of Howard University’s department of psychology, and because of his accomplishments, he is sometimes referred to as the “Father of Black Psychology.” Thirteen years later, Inez Beverly Prosser (1895–1934) became the first African American woman to receive a PhD in psychology. Prosser’s research highlighted issues related to education in segregated versus integrated schools, and ultimately, her work was very influential in the hallmark Brown v. Board of Education Supreme Court ruling that segregation of public schools was unconstitutional (Ethnicity and Health in America Series: Featured Psychologists, n.d.).
Although the establishment of psychology’s scientific roots occurred first in Europe and the United States, it did not take much time until researchers from around the world began to establish their own laboratories and research programs. For example, some of the first experimental psychology laboratories in South America were founded by Horatio Piñero (1869–1919) at two institutions in Buenos Aires, Argentina (Godoy & Brussino, 2010). In India, Gunamudian David Boaz (1908–1965) and Narendra Nath Sen Gupta (1889–1944) established the first independent departments of psychology at the University of Madras and the University of Calcutta, respectively. These developments provided an opportunity for Indian researchers to make important contributions to the field (Gunamudian David Boaz, n.d.; Narendra Nath Sen Gupta, n.d.).
When the American Psychological Association (APA) was first founded in 1892, all of the members were White males (Women and Minorities in Psychology, n.d.). However, by 1905, Mary Whiton Calkins was elected as the first female president of the APA, and by 1946, nearly one-quarter of American psychologists were female. Psychology became a popular degree option for students enrolled in the nation’s historically Black higher education institutions, increasing the number of Black Americans who went on to become psychologists. Given demographic shifts occurring in the United States and increased access to higher educational opportunities among historically underrepresented populations, there is reason to hope that the diversity of the field will increasingly match the larger population, and that the research contributions made by the psychologists of the future will better serve people of all backgrounds (Women and Minorities in Psychology, n.d.).
The Process of Scientific Research
Scientific knowledge is advanced through a process known as the scientific method . Basically, ideas (in the form of theories and hypotheses) are tested against the real world (in the form of empirical observations), and those empirical observations lead to more ideas that are tested against the real world, and so on. In this sense, the scientific process is circular. The types of reasoning within the circle are called deductive and inductive. In deductive reasoning , ideas are tested in the real world; in inductive reasoning , real-world observations lead to new ideas ( Figure 2.4 ). These processes are inseparable, like inhaling and exhaling, but different research approaches place different emphasis on the deductive and inductive aspects.
In the scientific context, deductive reasoning begins with a generalization—one hypothesis—that is then used to reach logical conclusions about the real world. If the hypothesis is correct, then the logical conclusions reached through deductive reasoning should also be correct. A deductive reasoning argument might go something like this: All living things require energy to survive (this would be your hypothesis). Ducks are living things. Therefore, ducks require energy to survive (logical conclusion). In this example, the hypothesis is correct; therefore, the conclusion is correct as well. Sometimes, however, an incorrect hypothesis may lead to a logical but incorrect conclusion. Consider this argument: all ducks are born with the ability to see. Quackers is a duck. Therefore, Quackers was born with the ability to see. Scientists use deductive reasoning to empirically test their hypotheses. Returning to the example of the ducks, researchers might design a study to test the hypothesis that if all living things require energy to survive, then ducks will be found to require energy to survive.
Deductive reasoning starts with a generalization that is tested against real-world observations; however, inductive reasoning moves in the opposite direction. Inductive reasoning uses empirical observations to construct broad generalizations. Unlike deductive reasoning, conclusions drawn from inductive reasoning may or may not be correct, regardless of the observations on which they are based. For instance, you may notice that your favorite fruits—apples, bananas, and oranges—all grow on trees; therefore, you assume that all fruit must grow on trees. This would be an example of inductive reasoning, and, clearly, the existence of strawberries, blueberries, and kiwi demonstrate that this generalization is not correct despite it being based on a number of direct observations. Scientists use inductive reasoning to formulate theories, which in turn generate hypotheses that are tested with deductive reasoning. In the end, science involves both deductive and inductive processes.
For example, case studies, which you will read about in the next section, are heavily weighted on the side of empirical observations. Thus, case studies are closely associated with inductive processes as researchers gather massive amounts of observations and seek interesting patterns (new ideas) in the data. Experimental research, on the other hand, puts great emphasis on deductive reasoning.
We’ve stated that theories and hypotheses are ideas, but what sort of ideas are they, exactly? A theory is a well-developed set of ideas that propose an explanation for observed phenomena. Theories are repeatedly checked against the world, but they tend to be too complex to be tested all at once; instead, researchers create hypotheses to test specific aspects of a theory.
A hypothesis is a testable prediction about how the world will behave if our idea is correct, and it is often worded as an if-then statement (e.g., if I study all night, I will get a passing grade on the test). The hypothesis is extremely important because it bridges the gap between the realm of ideas and the real world. As specific hypotheses are tested, theories are modified and refined to reflect and incorporate the result of these tests Figure 2.5 .
To see how this process works, let’s consider a specific theory and a hypothesis that might be generated from that theory. As you’ll learn in a later chapter, the James-Lange theory of emotion asserts that emotional experience relies on the physiological arousal associated with the emotional state. If you walked out of your home and discovered a very aggressive snake waiting on your doorstep, your heart would begin to race and your stomach churn. According to the James-Lange theory, these physiological changes would result in your feeling of fear. A hypothesis that could be derived from this theory might be that a person who is unaware of the physiological arousal that the sight of the snake elicits will not feel fear.
A scientific hypothesis is also falsifiable , or capable of being shown to be incorrect. Recall from the introductory chapter that Sigmund Freud had lots of interesting ideas to explain various human behaviors ( Figure 2.6 ). However, a major criticism of Freud’s theories is that many of his ideas are not falsifiable; for example, it is impossible to imagine empirical observations that would disprove the existence of the id, the ego, and the superego—the three elements of personality described in Freud’s theories. Despite this, Freud’s theories are widely taught in introductory psychology texts because of their historical significance for personality psychology and psychotherapy, and these remain the root of all modern forms of therapy.
In contrast, the James-Lange theory does generate falsifiable hypotheses, such as the one described above. Some individuals who suffer significant injuries to their spinal columns are unable to feel the bodily changes that often accompany emotional experiences. Therefore, we could test the hypothesis by determining how emotional experiences differ between individuals who have the ability to detect these changes in their physiological arousal and those who do not. In fact, this research has been conducted and while the emotional experiences of people deprived of an awareness of their physiological arousal may be less intense, they still experience emotion (Chwalisz, Diener, & Gallagher, 1988).
Scientific research’s dependence on falsifiability allows for great confidence in the information that it produces. Typically, by the time information is accepted by the scientific community, it has been tested repeatedly.
As an Amazon Associate we earn from qualifying purchases.
This book may not be used in the training of large language models or otherwise be ingested into large language models or generative AI offerings without OpenStax's permission.
Want to cite, share, or modify this book? This book uses the Creative Commons Attribution License and you must attribute OpenStax.
Access for free at https://openstax.org/books/psychology-2e/pages/1-introduction
- Authors: Rose M. Spielman, William J. Jenkins, Marilyn D. Lovett
- Publisher/website: OpenStax
- Book title: Psychology 2e
- Publication date: Apr 22, 2020
- Location: Houston, Texas
- Book URL: https://openstax.org/books/psychology-2e/pages/1-introduction
- Section URL: https://openstax.org/books/psychology-2e/pages/2-1-why-is-research-important
© Jan 6, 2024 OpenStax. Textbook content produced by OpenStax is licensed under a Creative Commons Attribution License . The OpenStax name, OpenStax logo, OpenStax book covers, OpenStax CNX name, and OpenStax CNX logo are not subject to the Creative Commons license and may not be reproduced without the prior and express written consent of Rice University.
Public Health Notes
Your partner for better health, hypothesis in research: definition, types and importance .
April 21, 2020 Kusum Wagle Epidemiology 0
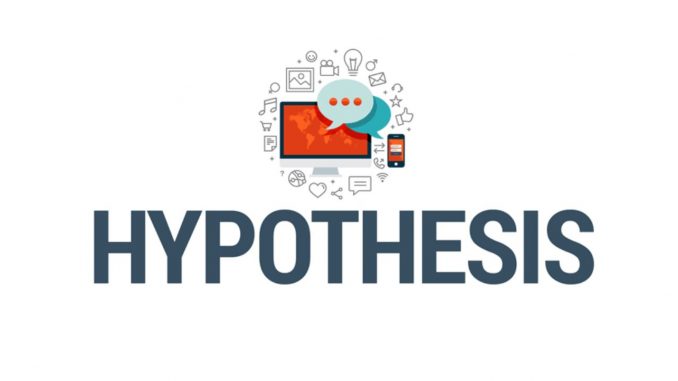
Table of Contents
What is Hypothesis?
- Hypothesis is a logical prediction of certain occurrences without the support of empirical confirmation or evidence.
- In scientific terms, it is a tentative theory or testable statement about the relationship between two or more variables i.e. independent and dependent variable.
Different Types of Hypothesis:
1. Simple Hypothesis:
- A Simple hypothesis is also known as composite hypothesis.
- In simple hypothesis all parameters of the distribution are specified.
- It predicts relationship between two variables i.e. the dependent and the independent variable
2. Complex Hypothesis:
- A Complex hypothesis examines relationship between two or more independent variables and two or more dependent variables.
3. Working or Research Hypothesis:
- A research hypothesis is a specific, clear prediction about the possible outcome of a scientific research study based on specific factors of the population.
4. Null Hypothesis:
- A null hypothesis is a general statement which states no relationship between two variables or two phenomena. It is usually denoted by H 0 .
5. Alternative Hypothesis:
- An alternative hypothesis is a statement which states some statistical significance between two phenomena. It is usually denoted by H 1 or H A .
6. Logical Hypothesis:
- A logical hypothesis is a planned explanation holding limited evidence.
7. Statistical Hypothesis:
- A statistical hypothesis, sometimes called confirmatory data analysis, is an assumption about a population parameter.
Although there are different types of hypothesis, the most commonly and used hypothesis are Null hypothesis and alternate hypothesis . So, what is the difference between null hypothesis and alternate hypothesis? Let’s have a look:
Major Differences Between Null Hypothesis and Alternative Hypothesis:
Importance of hypothesis:.
- It ensures the entire research methodologies are scientific and valid.
- It helps to assume the probability of research failure and progress.
- It helps to provide link to the underlying theory and specific research question.
- It helps in data analysis and measure the validity and reliability of the research.
- It provides a basis or evidence to prove the validity of the research.
- It helps to describe research study in concrete terms rather than theoretical terms.
Characteristics of Good Hypothesis:
- Should be simple.
- Should be specific.
- Should be stated in advance.
References and For More Information:
https://ocw.jhsph.edu/courses/StatisticalReasoning1/PDFs/2009/BiostatisticsLecture4.pdf
https://keydifferences.com/difference-between-type-i-and-type-ii-errors.html
https://www.khanacademy.org/math/ap-statistics/tests-significance-ap/error-probabilities-power/a/consequences-errors-significance
https://stattrek.com/hypothesis-test/hypothesis-testing.aspx
http://davidmlane.com/hyperstat/A2917.html
https://study.com/academy/lesson/what-is-a-hypothesis-definition-lesson-quiz.html
https://keydifferences.com/difference-between-null-and-alternative-hypothesis.html
https://blog.minitab.com/blog/adventures-in-statistics-2/understanding-hypothesis-tests-why-we-need-to-use-hypothesis-tests-in-statistics
- Characteristics of Good Hypothesis
- complex hypothesis
- example of alternative hypothesis
- example of null hypothesis
- how is null hypothesis different to alternative hypothesis
- Importance of Hypothesis
- null hypothesis vs alternate hypothesis
- simple hypothesis
- Types of Hypotheses
- what is alternate hypothesis
- what is alternative hypothesis
- what is hypothesis?
- what is logical hypothesis
- what is null hypothesis
- what is research hypothesis
- what is statistical hypothesis
- why is hypothesis necessary
Copyright © 2024 | WordPress Theme by MH Themes
HYPOTHESIS AND THEORY article
Artificial intelligence, human cognition, and conscious supremacy.
- 1 Sony Computer Science Laboratories, Shinagawa, Japan
- 2 Collective Intelligence Research Laboratory, The University of Tokyo, Meguro, Japan
The computational significance of consciousness is an important and potentially more tractable research theme than the hard problem of consciousness, as one could look at the correlation of consciousness and computational capacities through, e.g., algorithmic or complexity analyses. In the literature, consciousness is defined as what it is like to be an agent (i.e., a human or a bat), with phenomenal properties, such as qualia, intentionality, and self-awareness. The absence of these properties would be termed “unconscious.” The recent success of large language models (LLMs), such as ChatGPT, has raised new questions about the computational significance of human conscious processing. Although instances from biological systems would typically suggest a robust correlation between intelligence and consciousness, certain states of consciousness seem to exist without manifest existence of intelligence. On the other hand, AI systems seem to exhibit intelligence without consciousness. These instances seem to suggest possible dissociations between consciousness and intelligence in natural and artificial systems. Here, I review some salient ideas about the computational significance of human conscious processes and identify several cognitive domains potentially unique to consciousness, such as flexible attention modulation, robust handling of new contexts, choice and decision making, cognition reflecting a wide spectrum of sensory information in an integrated manner, and finally embodied cognition, which might involve unconscious processes as well. Compared to such cognitive tasks, characterized by flexible and ad hoc judgments and choices, adequately acquired knowledge and skills are typically processed unconsciously in humans, consistent with the view that computation exhibited by LLMs, which are pretrained on a large dataset, could in principle be processed without consciousness, although conversations in humans are typically done consciously, with awareness of auditory qualia as well as the semantics of what are being said. I discuss the theoretically and practically important issue of separating computations, which need to be conducted consciously from those which could be done unconsciously, in areas, such as perception, language, and driving. I propose conscious supremacy as a concept analogous to quantum supremacy, which would help identify computations possibly unique to consciousness in biologically practical time and resource limits. I explore possible mechanisms supporting the hypothetical conscious supremacy. Finally, I discuss the relevance of issues covered here for AI alignment, where computations of AI and humans need to be aligned.
1 Introduction
Recently, large language models (LLMs) have made rapid progress based on the transformer ( Vaswani et al., 2017 ) architecture, exhibiting many skills emulating but perhaps not matching human cognition, which were nonetheless once considered to be beyond the reach of machine intelligence, such as appropriate text generation based on a context, summarizing, searching under instructions, and optimization. With the advent of advanced AI systems such as ChatGPT ( Sanderson, 2023 ), questions are arising regarding the computational significance, if any, of consciousness. Despite some claims that LLMs are either already or soon becoming conscious ( Long, 2023 ), many regard these generative AI systems as doing computation unconsciously, thus forgoing possible ethical issues involved in AI abuse ( Blauth et al., 2022 ). Generic models of consciousness would also suggest the LLMs to be unconscious as a default hypothesis, unless otherwise demonstrated, e.g., by convincing behavior suggesting the presence of consciousness to an external observer or a theoretical reasoning supported by an academic consensus. If LLMs can or come close to pass human-level cognition tests such as the false belief task in the theory of mind ( Charman and Baron-Cohen, 1992 ; Baron-Cohen, 2000 ), the Turing test ( Turing, 1950 ), and Winograd schema challenge ( Sakaguchi et al., 2021 ) with their unconscious processing, what, if any, is the computational significance of consciousness?
Here, these abilities would not be necessary conditions for consciousness, as newborns are conscious without manifesting these abilities. The existence of these abilities would certainly be regarded as sufficient conditions for consciousness, in the generally accepted view of the human mind.
The theory of mind is related to the function of consciousness in the reportability and social context. The Turing test is tightly coupled with language, semantics in particular, and therefore closely related to consciousness. The Winograd schema challenge is crucial in understanding natural language, which is concerned with the nature of language here and now, locally, independent of the statistical properties dealt with in LLMs. The relation between functions exhibited by LLMs and consciousness is an interesting and timely question, especially when considering that natural language is typically processed when a human subject is conscious, except in the anecdotal and infrequent case of conversation in unconscious states, such as somniloquy ( Reimão and Lefévre, 1980 ), hypnosis ( Sarbin, 1997 ), and in a dream ( Kilroe, 2016 ), which is a state distinctive from typical conscious or unconscious states. In an apparent contradiction to the conventional assumption about the necessity of consciousness in typical natural language exchanges, computations demonstrated by LLMs are considered to be done unconsciously. If conversations involving texts partially or totally generated by LLMs virtually pass the Turing test, without computations involving consciousness, what, if any, does consciousness do computationally?
Velmans (1991) analyzed the function of consciousness in cortical information processing, taking into account the role of focus of attention, concluding that it was not clear if consciousness was necessary for cognitive processes, such as perception, learning, and creativity. Velmans elaborated on the complexity of speech production, where the tongue may make as many as 12 adjustments of shape per second, so that “within 1 min of discourse as many as 10–15 thousand neuromuscular events occur” ( Lenneberg, 1967 ). Based on these observations, Velmans suggested that speech production does not necessarily require consciousness. Such observations would necessitate a more nuanced consideration of the role of conscious and unconscious processes in language.
Apart from the conscious/unconscious divide, language occupies a central position in our understanding of consciousness. Velmans (2012) streamlined the foundations of consciousness studies, pointing out that the default position would be to reduce subjective experiences to objectively observable phenomena, such as brain function. On a more fundamental level, Velmans argued that language is associated with the dual-aspect nature of the psychophysical element of human experience, where language models the physical world only in incomplete ways, limited by the capacities of our senses. The central role of language in our understanding of the world, including consciousness, should be kept in mind when discussing artificial reproductions of language, including, but not limited to, the LLMs.
Many regard the problem of consciousness as primarily in the phenomenological domain, concerned with what is experienced by a subject when he or she is conscious, e.g., properties such as qualia, intentionality, and self-awareness as opposed to physical or functional descriptions of the brain function. There are experimental and theoretical approaches tackling the cognitive implications of consciousness based on ideas, such as neural correlates of consciousness (NCC, Crick and Koch, 1998 ; Koch et al., 2016 ), global workspace theory ( Baars, 1997 , 2005 ), integrated information theory ( Tononi et al., 2016 ), and free-energy principle ( Friston, 2010 ).
Wiese and Friston (2021) discussed the relevance of the free-energy principle as a constraint for the computational correlates of consciousness (CCC), stressing the importance of neural dynamics, not states. In their framework, trajectories rather than states are mapped to conscious experiences. They propose CCC as a more general concept than the neural correlates of consciousness (NCC), discussing the nature of the correlates as necessary, sufficient, or both conditions for consciousness.
Some, somewhat controversially, consider quantum effects as essential in explaining the nature of consciousness ( Hameroff, 1998 ; Woolf and Hameroff, 2001 ). Although there have been significant advances made, explaining the hard problem of consciousness ( Chalmers, 1995 ) from such theoretical approaches remains hypothetical at best, even if not cognitively closed ( McGinn, 1994 ), and a scientific consensus has not been reached yet. There are also arguments that hold that the hard problem is not necessarily essential for the study of consciousness. Seth (2021) argued that if we pursue the real problem of accounting for properties of consciousness in terms of biological mechanisms, the hard problem will turn out to be less important.
Given the difficulty in studying the phenomenological aspects of consciousness, with the advancement in artificial intelligence (AI), there is now a unique opportunity to study the nature of consciousness by approaching it from its computational significance. As artificial intelligence systems, such as LLMs, are reproducing and even surpassing human information processing capabilities, the identification of computational elements possibly unique to consciousness is coming under more focused analysis.
At present, it is difficult to give a precise definition of what computations unique to consciousness are. What follows are tentative descriptions adopted in this paper. From the objective point of view, neural computation correlating with consciousness would typically involve large areas of the brain processing information in coherent and integrated parallel manners, while sensory qualia represent the result of complex processing in compressed forms, as in color constancy ( Foster, 2011 ). Unconscious computation, on the other hand, does not meet these criteria. From the subjective point of view, conscious computation would be accompanied by such properties as qualia, intentionality, and self-consciousness. Unconscious computations do not cause these aspects of experience to emerge.
Artificial intelligence is an umbrella term, and its specific capabilities depend on parameters and configurations of system makeup and dynamics. For now, we would assume that AI systems referred to here are realized on classical computers. AI systems constructed on quantum computers might exhibit broader ranges of computational capabilities, possibly exhibiting quantum supremacy ( Arute et al., 2019 ), which describes the abilities of quantum computers to solve problems any classical computer could not solve in any practical time. Quantum supremacy is not a claim that quantum computers would be able to execute computations beyond what universal Turing machines ( Turing, 1936 ) are capable of. It is rather a claim that quantum computers can, under the circumstances, execute computations that could, in principle, be done by classical computers, but not within any practical period considering the physical time typically available to humans.
Similarly, conscious supremacy can be defined as domains of computation that can be conducted by conscious processes but cannot be executed by systems lacking consciousness in any practical time. Since the science of consciousness has not yet developed to reach the same level as quantum mechanics, it is difficult to give a precise definition of what conscious supremacy is at present. What follows is a tentative definition adopted in this article. Out of all the computations done in the neural networks in the brain, conscious supremacy refers to those areas of computation accompanied by consciousness, which are done in efficient and integrated ways compared to unconscious computation. Given the limits of resources available in the brain, computations executed in conscious supremacy would be, in a practical sense, impossible to execute by unconscious computation in any meaningful biological time. However, in principle, they could be done. Thus, there are no distinctions between computations belonging to conscious supremacy and other domains in terms of computability in principle. The practical impossibility of non-conscious systems to execute computations belonging to conscious supremacy would have been one of the adaptive values of consciousness in evolution.
The relationship between quantum supremacy and conscious supremacy will be discussed later.
As of now, quantum supremacy remains controversial ( McCormick, 2022 ). The merit of introducing the perhaps equally debatable concept of conscious supremacy is that we can hope to streamline aspects of computation conducted by conscious and unconscious processes.
Abilities to play board games, such as chess, shogi, and go, are no longer considered to be unique to human cognition after AI systems, such as Deep Blue ( Campbell et al., 2002 ) and AlphaZero ( Schrittwieser et al., 2020 ), defeated human champions. After the success of LLMs in executing a large part of natural language tasks, cognitive abilities once considered unique to humans, e.g., the theory of mind, Turing test, and Winograd schema challenge, might not be considered to be verifications of the ability of artificial intelligence systems to perform cognitive tasks on par with humans. It should be noted that the attribution of the theory of mind to LLMs remains controversial ( Aru et al., 2023 ), and the exact nature of cognitive functions related to natural language, if any, in LLMs is an open question. However, it does seem legitimate to start considering the exclusion of certain computations from the set of those unique to consciousness based on computational evidence. While such exclusion might reflect cognitive biases on the part of humans to raise the bar unfavorably for AI systems, in an effort to solve cognitive dissonance ( Aronson, 1969 ) about the relative superiorities of AI and humans, such considerations could serve as a filter to fine-tune domains of cognitive tasks uniquely executed by human cognition, conscious, and unconscious.
As artificial intelligence systems based on deep learning and other approaches advance in their abilities, tasks considered to be uniquely human would gradually diminish in the spectrum of functionalities. Specifically, the set X of computations considered unique to humans would be the complement of the union of the set of computations executed by artificial intelligence systems A 1 , A 2 , …, A N under consideration. Namely, X = A c , where A = A 1 UA 2 U… UA N ( Figure 1 ), where the whole set represents the space of possible computations conducted by humans. As the number of artificial intelligence systems increases, the uniquely human domain of computation would ultimately become X ∞ = A ∞ c , where A ∞ = lim N- > ∞ A 1 UA 2 U… UA N .
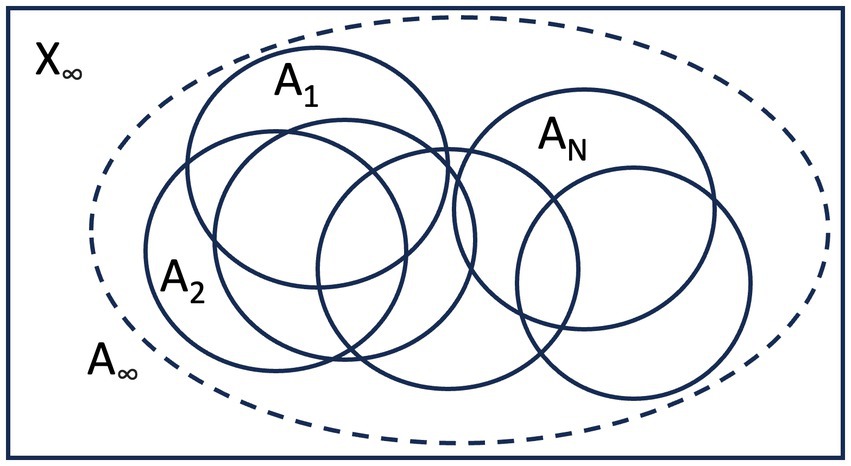
Figure 1 . The analysis of AI capabilities would help focus the computational domain unique to consciousness (X), which can be defined in terms of instances of AI systems. As the number of AI systems increases, computations unique to consciousness will be more finely defined.
Needless to say, such an argument is conceptual in nature, as it is difficult to draw a clear line between what could and could not be done by artificial intelligence systems at present. Among computations unique to humans, some would be executed consciously, while some might be a combination of conscious and unconscious computation, involving processes which lie either inside or outside the neural correlates of consciousness ( Crick and Koch, 1998 ; Koch et al., 2016 ). Theoretically, there could also be computations unique to humans executed unconsciously, although not of central interest in the context adopted here.
Penrose suggested that consciousness is correlated with the quantum mechanical effect, possibly involving quantum gravity ( Penrose, 1996 ). Penrose went on to collaborate with Stuart Hameroff. Penrose and Hameroff together suggested, in a series of papers ( Hameroff and Penrose, 1996 ; Hameroff and Penrose, 2014 ), that quantum mechanical processes in microtubules were involved in conscious processes, which went beyond the algorithmic capabilities of computability for the classical computer. Specifically, it was postulated that a process named “Orchestrated objective reduction” (Orch OR) was responsible for the generation of proto-consciousness in microtubules, a hypothesis independent from conventional arguments on quantum computing. One of the criticisms directed to such quantum models of consciousness was based on the fact that temperatures in biological systems are typically too high for quantum coherence or entanglement to be effective ( Tegmark, 2000 ).
2 Possibilities and limits of artificial intelligence systems
Artificial General Intelligence (AGI; Goertzel, 2014 ) is purported to execute all tasks carried out by a typical human brain and beyond. Proposed tasks to be executed by AGI include the Turing test, coffee making or Wozniak test ( Adams et al., 2012 ), college enrollment test ( Goertzel, 2014 ), employment test ( Scott et al., 2022 ), and the discovery of new scientific knowledge ( Kitano, 2016 ).
In identifying possible areas for uniquely human cognition and potential candidates for conscious supremacy, it is useful to discuss systemic potentials and limits of artificial intelligence, which are currently apparent.
Some LLMs have started to show sparks of general intelligence ( Bubeck et al., 2023 ) beyond abilities for linguistic processing. Such a potential might be explained by the inherent functions of language. The lexical hypothesis ( Crowne, 2007 ) states that important concepts in fields, such as personality study and general philosophy, would be expressible by everyday language. The ability of natural language to represent and analyze a wide range of information in the environment is consistent with the perceived general ability of LLMs to represent various truths about this world, without necessarily being conscious, thus suggesting the central importance of representation in the analysis of intelligence.
What is meant by representation is a potentially controversial issue. In the conventional sense of psychology and philosophy of mind, a representation refers to the internal state that corresponds to an external reality ( Marr, 1982 ). In the constructivist approach, representation would be an active construct of an agent’s knowledge, not necessarily requiring an external reality as a prior ( Von Glasersfeld, 1987 ). Representations in artificial intelligence systems would be somewhere in between, taking inspiration from various lines of theoretical approaches.
One of the problems with LLMs, such as ChatGPT, is the occurrence of hallucination ( Ji et al., 2023 ) and the tendency to produce sentences inconsistent with accepted facts, a term criticized by some researchers as an instance of anthropomorphism. Although humans also suffer from similar misconceptions, subjects typically are able to make confident judgments about their own statements ( Yeung and Summerfield, 2012 ), while methods for establishing similar capabilities in artificial intelligence systems have not been established. Regarding consciousness, metacognitive processes associated with consciousness ( Nelson, 1996 ) might help rectify potential errors in human cognition.
Behaviorist ways of thinking ( Araiba, 2019 ) suggest that human thoughts are ultimately represented in terms of bodily movements. No matter how well developed an intelligent agent might be, manifestations of its functionality would ultimately be found in its objective courses of action in the physical space. From this perspective, the intelligence of an agent would be judged in terms of its external behavior, an idea in AI research sometimes called instrumental convergence ( Bostrom, 2012 ).
The possibilities and limits of artificial intelligence systems would be tangibly assessed through analysis of behavior. In voluntary movement, evidence suggests that consciousness is involved in vetoing a particular action (free won’t) when it is judged to be inappropriate within a particular context ( Libet, 1999 ).
Thus, from robust handling of linguistic information to streamlining of external behavior, metacognitive monitoring and control would be central in identifying and rectifying limits of artificial intelligence systems, a view consistent with the idea that metacognition plays an essential role in consciousness ( Nelson, 1996 ).
3 Computations possibly unique to conscious processing
As of now, the eventual range of computational capabilities of artificial intelligence is unclear. Employing cognitive arguments based on the observation of what subset of computation is typically done consciously, in addition to insights on the limits of artificial intelligence, would help narrow down possible consciousness-specific tasks. In that process, the division of labor between conscious and unconscious processes could be made, as we thus outline heterogeneous aspects of cognition.
Acquiring new skills or making decisions in novel contexts would typically require the involvement of conscious processing, while the execution of acquired skills would proceed largely unconsciously ( Solomon, 1911 ; Lisman and Sternberg, 2013 ) in terms of the accompanying phenomenological properties, such as qualia, intentionality, and attention. Any cognitive task, when it needs to integrate information analyzed across many different regions in the brain, typically requires consciousness, reflecting the global nature of consciousness in terms of cortical regions involved ( Baars, 2005 ). The autonomous execution of familiar tasks would involve a different set of neural networks compared to the minimum set of neural activities (neural correlates, Koch et al., 2016 ) required for the sustaining of consciousness.
It is interesting to note here that some self-learning unsupervised artificial intelligence systems seem to possess abilities to acquire new skills and make decisions in novel contexts ( Silver et al., 2017 ; Schrittwieser et al., 2020 ). As the ability of artificial intelligence systems approaches the level purported for AGI ( Goertzel, 2014 ), the possibility of the emergence of consciousness might have to be considered.
The global neural workspace (GNW) theory ( Dehaene et al., 1998 ; Mashour et al., 2020 ) addresses how the neural networks in the brain support a dynamic network where relevant information can be assessed by local networks, eventually giving rise to consciousness. The multimodal nature of the GNW theory has inspired various theoretical works, including those related to deep learning networks ( LeCun et al., 2015 ; Bengio, 2017 ).
In evolution, one of the advantages of information processing involving consciousness might have been decision-making reflecting a multitude of sensory inputs. Multimodal perception typically subserves such a decision-making process. Since the science of decision-making is an integral part of AI alignment ( Yudkowsky, 2015 ), the difference between conscious and unconscious, as well as human and AI decision-making processes, would shed much light on the parameters of systems supporting the nature of conscious computation.
Technological issues surrounding self-driving cars ( Badue et al., 2021 ) have emerged as one of the most important research themes today, both from theoretical and practical standpoints. Driving cars involves a series of judgments, choices, and actions based on multimodal sensory information. Judgments on how to drive a vehicle often must be done within limited time windows in ad hoc situations, affected by the unpredictability of other human drivers, if any, and there are still challenges toward realizing fully self-driving vehicles ( Kosuru and Venkitaraman, 2023 ). Moral dilemmas involved in driving judgments require sorting out situations concerned with conflicting choices for safety, known collectively as the trolley problem ( Thomson, 1985 ), which is often intractable even when presented with clear alternative schemes ( Awad et al., 2018 ). In real-life situations, there would be perceptual and cognitive ambiguities about, for example, whether you can really save five people by sacrificing one. In the face of such difficulties, fully self-driving cars without conscious human interventions might turn out to be impossible ( Shladover, 2016 ).
The language is a series of micro-decisions, in that words must be selected, depending on the context, as follow-up sequences on what has been already expressed. The apparent success of LLMs in reproducing salient features of embedded knowledge in the language ( Singhal et al., 2023 ) is impressive. However, it might still fall short of executing situated or embodied choice of words, as required, for example, in the college enrollment and employment tests. A linguistic generative AI might nominally pass the Turing test in artificial and limited situations. However, when an AI system implemented in a robot interacts with a human in real-life situations, there might be a perceived uncanny valley ( Mori, 2012 ) linguistically, where negative emotions, such as uneasiness and repulsion, might be hypothetically induced in a human subject as the performance comes nearer to the human level.
4 Possible mechanisms for conscious supremacy
It is possible that there are computations uniquely executed by conscious processes, and there could be some similarities between conscious and quantum computations, independent of whether consciousness actually involves quantum processes in the brain. There could be similarities between postulated quantum supremacy and conscious supremacy, without underlying common mechanisms being necessarily implicated. It is worth noting here that just as it is in principle possible to simulate quantum computing on classical computers, it might be possible to simulate conscious computing, regardless of its nature, on classical computers, e.g., in terms of connectionist models representing neural networks in the brain.
There are several algorithms that demonstrate the superiority of quantum computing. For example, Schor’s algorithm ( Shor, 1994 ) can find prime factors of large numbers efficiently. Given a large number N, Shor’s algorithm for finding prime factors can run in polynomial time in terms of N, compared to sub-exponential time on optimal algorithms for a classical computer.
In conscious visual perception, the binding problem ( Feldman, 2012 ) questions how the brain integrates visual features, such as colors and forms, into coherent conscious percepts. The challenge of combinatorial explosion ( Treisman, 1999 ), in which all possible combinations of features, such as the yellow (color) Volkswagen Beetle car (form), must be dealt with, becomes essential there. Given the fact that forms ( Logothetis et al., 1995 ) and colors ( Zeki and Marini, 1998 ) are represented by distributed circuits in the brain, sorting through the possible combinations of forms and colors has similarities with the factoring problem addressed by Shor’s algorithm ( Figure 2 ).
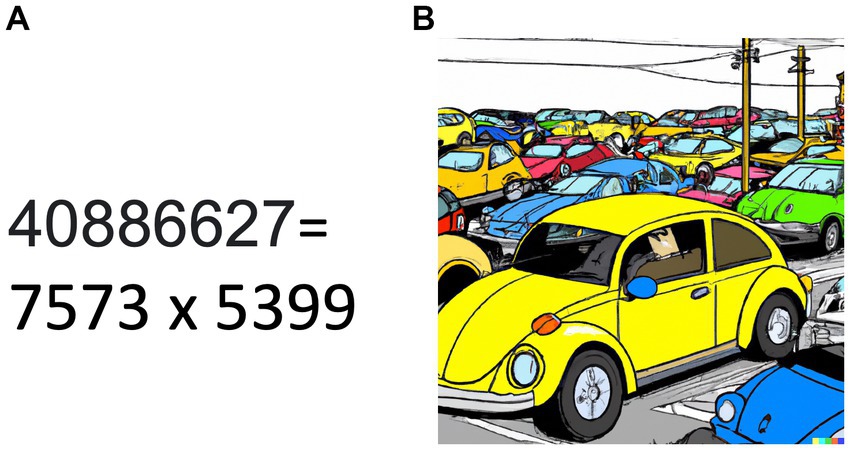
Figure 2 . Analogy between finding prime factors and integration of visual features. (A) Finding prime factors for a large number becomes increasingly difficult for classical computers. Quantum computing employing Shor’s algorithm provides an efficient method for factoring large natural numbers. (B) Sorting out combinatorial explosion in the integration of visual features represented in distributed neural networks in the brain is a still unresolved challenge known as the binding problem. The picture was generated by Dall-E (Open AI) with the prompt: A yellow Volkswagen Beetle car surrounded by cars of different shapes and colors seen from a distance in manga style.
In quantum computing ( Deutsch, 1985 ; Feynman, 1985 ), quantum superposition and entanglement are ingeniously employed to conduct algorithms effectively impossible for classical computers to execute in realistic time frames. In a quantum computing process, decoherence would introduce noise, and in order to execute on a large scale, a process called quantum error correction (QEC; Cai and Ma, 2021 ) is essential.
In conscious computing discussed here, similar mechanisms might be at play. For example, the contrast between the noisy neural firings and the apparently Platonic phenomenology of qualia suggests a process in which the variabilities due to noise in neural firings are rectified, named here conscious error correction (CEC). At present, the plausibility or the details of such an error-rectifying scheme is not clear. The possible relationships (if any) between QEC and CEC remain speculative at best at the moment. Despite these reservations, the involvement of error-correcting mechanisms in consciously conducted computation would be a line of thought worth investigating.
5 Implications for AI alignment
As artificial intelligence systems make progress, it is becoming important to align them with humans, an area called AI alignment ( Russell and Norvig, 2021 ).
The elucidation of computations uniquely executed by consciousness and the possible existence of conscious supremacy, i.e., computations specifically and uniquely executed by neural processes correlating with consciousness, would put a constraint on AI alignment schemes.
Specifically, it would be an efficient alignment strategy to develop AI systems with capabilities other than uniquely conscious computations, while leaving computation involving conscious supremacy to humans.
It is interesting to consider the implications of such divisions of labor between AIs and humans for AI safety ( Zhang et al., 2021 ). It would be impractical to require AI systems to carry out tasks better left to humans. Expecting AIs to execute tasks belonging to conscious supremacy would significantly disrupt AI safety.
Eliezer Yudkowsky’s conceptualization of Friendly AI ( Yudkowsky, 2008 ) is based on the importance of updating the system in accordance with humans ( Russell and Norvig, 2021 ). Reinforcement learning from human feedback (RLHF; Stiennon et al., 2020 ), a technique often used in the development of artificial intelligence systems, can be considered to be an instance of developing Friendly AI and an attempt at the division of labor between conscious (human) and unconscious (AI) computations.
Alignment of AIs with humans, in the context of AI safety in particular, would depend on an effective division of labor between cognition unique to humans centered on conscious supremacy and computation conducted by computers, in a way similar to the interaction between conscious and unconscious processes in the human brain. In this context, artificial intelligence systems can be regarded as extensions of unconscious processes in the brain. Insights on cortical plasticities from tool use ( Iriki et al., 1996 ) could provide relevant frameworks for discussion. It is important to note that limiting the functions of artificial intelligence systems to non-conscious operations does not necessarily guarantee robust alignment. Alignment would also depend on parameters that are dependent on the developers and stakeholders in the ecosystem of artificial intelligence. It would be important to discuss various aspects concerning alignment, including those put forward here.
Finally, the development of artificial consciousness ( Chrisley, 2008 ), whether theoretically or practically feasible or not, might not be an effective strategy for AI alignment. From the point of view of the division of labor, computational domains belonging to conscious supremacy would be better left to humans. Artificial intelligence systems would do a better job of alignment by trying to augment computations unique to consciousness, which are to be reasonably executed by humans, rather than by replacing them from scratch.
6 Discussion
I have addressed here the possibility of characterizing conscious processes from a computational point of view. The development of artificial intelligence systems provides unique opportunities to explore and focus more deeply on computational processes unique to consciousness.
At present, it is not clear whether consciousness would eventually emerge from present lines of research and development in artificial intelligence. It would be useful to start from the null hypothesis of the non-existence of consciousness in artificial intelligence systems. We would then be able to narrow down what consciousness uniquely computes.
I have proposed the concept of conscious supremacy. Although this is speculative at present, it would be useful to think in terms of computational contexts apart from the hard problem of the phenomenology of consciousness. The presence of conscious supremacy would be connected to the advantages the emergence of consciousness has provided in the history of evolution. Elucidating the nature of conscious supremacy would help decipher elements involved in consciousness, whether it is ultimately coupled with quantum processes or not.
The value of arguments presented in this paper is limited, as it has not yet specifically identified computations unique to consciousness. The efforts to characterize computations unique to consciousness in terms of conscious supremacy presented here would hopefully help streamline discussions on this issue, although, needless to say, much work remains to be done.
Data availability statement
The original contributions presented in the study are included in the article/supplementary material, further inquiries can be directed to the corresponding author.
Author contributions
KM: Conceptualization, Data curation, Formal analysis, Funding acquisition, Investigation, Methodology, Project administration, Resources, Software, Supervision, Validation, Visualization, Writing – original draft, Writing – review & editing.
The author declares that no financial support was received for the research, authorship, and/or publication of this article.
Conflict of interest
Author KM was employed by Sony Computer Science Laboratories.
Publisher’s note
All claims expressed in this article are solely those of the authors and do not necessarily represent those of their affiliated organizations, or those of the publisher, the editors and the reviewers. Any product that may be evaluated in this article, or claim that may be made by its manufacturer, is not guaranteed or endorsed by the publisher.
Adams, S., Arel, I., Bach, J., Coop, R., Furlan, R., Goertzel, B., et al. (2012). Mapping the landscape of human-level artificial general intelligence. AI Mag. 33, 25–41. doi: 10.1609/aimag.v33i1.2322
Crossref Full Text | Google Scholar
Araiba, S. (2019). Current diversification of behaviorism. Perspect. Beha. Sci. 43, 157–175. doi: 10.1007/s40614-019-00207-0
PubMed Abstract | Crossref Full Text | Google Scholar
Aronson, E. (1969). “The theory of cognitive dissonance: a current perspective” in Advances in experimental social psychology , vol. 4 (Academic Press), 1–34.
Google Scholar
Aru, J., Labash, A., Corcoll, O., and Vicente, R. (2023). Mind the gap: challenges of deep learning approaches to theory of mind. Artif. Intell. Rev. 56, 9141–9156. doi: 10.1007/s10462-023-10401-x
Arute, F., Arya, K., Babbush, R., Bacon, D., Bardin, J. C., Barends, R., et al. (2019). Quantum supremacy using a programmable superconducting processor. Nature 574, 505–510. doi: 10.1038/s41586-019-1666-5
Awad, E., Dsouza, S., Kim, R., Schulz, J., Henrich, J., Shariff, A., et al. (2018). The moral machine experiment. Nature 563, 59–64. doi: 10.1038/s41586-018-0637-6
Baars, B. J. (1997). In the theatre of consciousness. Global workspace theory, a rigorous scientific theory of consciousness. J. Conscious. Stud. 4, 292–309.
Baars, B. J. (2005). Global workspace theory of consciousness: toward a cognitive neuroscience of human experience. Prog. Brain Res. 150, 45–53. doi: 10.1016/S0079-6123(05)50004-9
Badue, C., Guidolini, R., Carneiro, R. V., Azevedo, P., Cardoso, V. B., Forechi, A., et al. (2021). Self-driving cars: a survey. Expert Syst. Appl. 165:113816. doi: 10.1016/j.eswa.2020.113816
Baron-Cohen, S. (2000). Theory of mind and autism: a review. Int. Rev. Res. Mental Retardat. 23, 169–184. doi: 10.1016/S0074-7750(00)80010-5
Beckman, D., Chari, A. N., Devabhaktuni, S., and Preskill, J. (1996). “Efficient networks for quantum factoring” (PDF). Phys. Rev. A 54, 1034–1063. doi: 10.1103/PhysRevA.54.1034
Bengio, Y. (2017). The consciousness prior. arXiv:1709.08568 . doi: 10.48550/arXiv.1709.08568
Benioff, P. (1980). The computer as a physical system: a microscopic quantum mechanical Hamiltonian model of computers as represented by Turing machines. J. Stat. Phys. 22, 563–591. doi: 10.1007/BF01011339
Blauth, T. F., Gstrein, O. J., and Zwitter, A. (2022). Artificial intelligence crime: an overview of malicious use and abuse of AI. IEEE Access 10, 77110–77122. doi: 10.1109/ACCESS.2022.3191790
Bostrom, N. (2012). The superintelligent will: motivation and instrumental rationality in advanced artificial agents. Mind. Mach. 22, 71–85. doi: 10.1007/s11023-012-9281-3
Bray, D. (1995). Protein molecules as computational elements in living cells. Nature 376, 307–312. doi: 10.1038/376307a0
Bubeck, S., Chandrasekaran, V., Eldan, R., Gehrke, J., Horvitz, E., Kamar, E., et al. (2023). Sparks of artificial general intelligence: early experiments with gpt-4. arXiv preprint 2303.12712 . doi: 10.48550/arXiv.2303.12712
Cai, W., and Ma, Y. (2021). Bosonic quantum error correction codes in superconducting quantum circuits. Fundamental Res. 1, 50–67. doi: 10.1016/j.fmre.2020.12.006
Campbell, M., Hoane, A. J. Jr., and Hsu, F. H. (2002). Deep Blue. Artif. Intell. 134, 57–83. doi: 10.1016/S0004-3702(01)00129-1
Chalmers, D. (1995). Facing up to the problem of consciousness. J. Conscious. 2, 200–219.
Charman, T., and Baron-Cohen, S. (1992). Understanding drawings and beliefs: a further test of the metarepresentation theory of autism: a research note. J. Child Psychol. Psychiatry 33, 1105–1112. doi: 10.1111/j.1469-7610.1992.tb00929.x
Chrisley, R. (2008). Philosophical foundations of artificial consciousness. Artif. Intell. Med. 44, 119–137. doi: 10.1016/j.artmed.2008.07.011
Crick, F., and Koch, C. (1998). Consciousness and neuroscience. Cereb. Cortex 8, 97–107. doi: 10.1093/cercor/8.2.97
Crowne, D. P. (2007). Personality theory . Oxford: Oxford University Press.
Dehaene, S., Kerszberg, M., and Changeux, J. P. (1998). A neuronal model of a global workspace in effortful cognitive tasks. Proc. Natl. Acad. Sci. 95, 14529–14534. doi: 10.1073/pnas.95.24.14529
Deutsch, D. (1985). Quantum theory, the church–Turing principle and the universal quantum computer. Proceedings of the Royal Society of London. A. Math. Phys. Sci. 400, 97–117.
Feldman, J. (2012). The neural binding problem. Cogn. Neurodyn. 7, 1–11. doi: 10.1007/s11571-012-9219-8
Feynman, R. P. (1985). Quantum mechanical computers. Optics News 11, 11–20. doi: 10.1364/ON.11.2.000011
Foster, D. H. (2011). Color constancy. Vis. Res. 51, 674–700.
Friston, K. (2010). The free-energy principle: a unified brain theory? Nat. Rev. Neurosci. 11, 127–138. doi: 10.1038/nrn2787
Goertzel, B. (2014). Artificial general intelligence: concept, state of the art, and future prospects. J. Artif. Gen. Intell. 5, 1–48. doi: 10.2478/jagi-2014-0001
Hameroff, S. (1998). Quantum computation in brain microtubules? The Penrose–Hameroff ‘Orch OR ‘model of consciousness. Philos. Trans. R. Soc. London, Ser. A 356, 1869–1896
Hameroff, S. R., and Penrose, R. (1996). Conscious events as orchestrated space-time selections. J. Conscious. Stud. 3, 36–53.
Hameroff, S., and Penrose, R. (2014). Consciousness in the universe: a review of the ‘Orch OR’theory. Phys Life Rev 11, 39–78. doi: 10.1016/j.plrev.2013.08.002
Iriki, A., Tanaka, M., and Iwamura, Y. (1996). Coding of modified body schema during tool use by macaque postcentral neurones. Neuroreport 7, 2325–2330. doi: 10.1097/00001756-199610020-00010
Ji, Z., Lee, N., Frieske, R., Yu, T., Su, D., Xu, Y., et al. (2023). Survey of hallucination in natural language generation. ACM Comput. Surv. 55, 1–38. doi: 10.1145/3571730
Kilroe, P. A. (2016). Reflections on the study of dream speech. Dreaming 26, 142–157. doi: 10.1037/drm0000016
Kitano, H. (2016). Artificial intelligence to win the nobel prize and beyond: creating the engine for scientific discovery. AI Mag. 37, 39–49. doi: 10.1609/aimag.v37i1.2642
Koch, C., Massimini, M., Boly, M., and Tononi, G. (2016). Neural correlates of consciousness: progress and problems. Nat. Rev. Neurosci. 17, 307–321. doi: 10.1038/nrn.2016.22
Kosuru, V. S. R., and Venkitaraman, A. K. (2023). Advancements and challenges in achieving fully autonomous self-driving vehicles. World J. Adv. Res. Rev. 18, 161–167. doi: 10.30574/wjarr.2023.18.1.0568
Lau, H., and Rosenthal, D. (2011). Empirical support for higher-order theories of conscious awareness. Trends Cogn. Sci. 15, 365–373. doi: 10.1016/j.tics.2011.05.009
LeCun, Y., Bengio, Y., and Hinton, G. (2015). Deep learning. Nature 521, 436–444. doi: 10.1038/nature14539
Lenneberg, E. H. (1967). Biological foundations of language , vol. 2. New York: Wiley, 59–67.
Libet, B. (1999). Do we have free will? J. Conscious. Stud. 6, 47–57.
Lisman, J., and Sternberg, E. J. (2013). Habit and nonhabit systems for unconscious and conscious behavior: implications for multitasking. J. Cogn. Neurosci. 25, 273–283. doi: 10.1162/jocn_a_00319
Logothetis, N. K., Pauls, J., and Poggio, T. (1995). Shape representation in the inferior temporal cortex of monkeys. Curr. Biol. 5, 552–563. doi: 10.1016/S0960-9822(95)00108-4
Long, R. (2023). Introspective capabilities in large language models. J. Conscious. Stud. 30, 143–153. doi: 10.53765/20512201.30.9.143
Marr, D. (1982). Vision: A computational investigation into the human representation and processing of visual information . New York: W. H. Freeman and Company.
Mashour, G. A., Roelfsema, P., Changeux, J. P., and Dehaene, S. (2020). Conscious processing and the global neuronal workspace hypothesis. Neuron 105, 776–798. doi: 10.1016/j.neuron.2020.01.026
McCormick, K. (2022). Race not over between classical and quantum computers. Physics 15:19. doi: 10.1103/Physics.15.19
McGinn, C. (1994). The problem of philosophy. Philos. Stud. 76, 133–156. doi: 10.1007/BF00989821
Mori, M. (2012). The uncanny valley. IEEE Robot. Automat. 19, 98–100. doi: 10.1109/MRA.2012.2192811
Nelson, T. O. (1996). Consciousness and metacognition. Am. Psychol. 51, 102–116. doi: 10.1037/0003-066X.51.2.102
Penrose, R. (1996). On gravity’s role in quantum state reduction. Gen. Relativ. Gravit. 28, 581–600. doi: 10.1007/BF02105068
Reimão, R. N., and Lefévre, A. B. (1980). Prevalence of sleep-talking in childhood. Brain Dev. 2, 353–357. doi: 10.1016/S0387-7604(80)80047-7
Russell, S. J., and Norvig, P. (2021). Artificial intelligence: A modern approach . 4th Edn. London: Pearson.
Sakaguchi, K., Bras, R. L., Bhagavatula, C., and Choi, Y. (2021). Winogrande: an adversarial winograd schema challenge at scale. Commun. ACM 64, 99–106. doi: 10.1145/3474381
Sanderson, K. (2023). GPT-4 is here: what scientists think. Nature 615:773. doi: 10.1038/d41586-023-00816-5
Sarbin, T. R. (1997). Hypnosis as a conversation:‘believed-in imaginings’ revisited. Contemp. Hypn. 14, 203–215. doi: 10.1002/ch.105
Schrittwieser, J., Antonoglou, I., Hubert, T., Simonyan, K., Sifre, L., Schmitt, S., et al. (2020). Mastering atari, go, chess and shogi by planning with a learned model. Nature 588, 604–609. doi: 10.1038/s41586-020-03051-4
Scott, A. C., Solórzano, J. R., Moyer, J. D., and Hughes, B. B. (2022). The future of artificial intelligence. Int. J. Artif. Intell. Mach. Learn. 2, 1–37. doi: 10.51483/IJAIML.2.1.2022.1-37
Seth, A. (2021). Being you: A new science of consciousness . New York: Penguin.
Shladover, S. E. (2016). The truth about “self-driving” cars. Sci. Am. 314, 52–57. doi: 10.1038/scientificamerican0616-52
Shor, P. W. (1994). “Algorithms for quantum computation: discrete logarithms and factoring” in Proceedings 35th annual symposium on foundations of computer science (Washington, DC: IEEE Computer Society Press), 124–134.
Silver, D., Schrittwieser, J., Simonyan, K., Antonoglou, I., Huang, A., Guez, A., et al. (2017). Mastering the game of go without human knowledge. Nature 550, 354–359. doi: 10.1038/nature24270
Singhal, K., Azizi, S., Tu, T., Mahdavi, S. S., Wei, J., Chung, H. W., et al. (2023). Large language models encode clinical knowledge. Nature 620, 172–180. doi: 10.1038/s41586-023-06291-2
Solomon, J. (1911). The philosophy of Bergson. Mind XX, 15–40. doi: 10.1093/mind/XX.77.15
Stiennon, N., Ouyang, L., Wu, J., Ziegler, D., Lowe, R., Voss, C., et al. (2020). Learning to summarize with human feedback. Adv. Neural Inf. Proces. Syst. 33, 3008–3021. doi: 10.48550/arXiv.2009.01325
Tegmark, M. (2000). Importance of quantum decoherence in brain processes. Phys. Rev. E 61, 4194–4206. doi: 10.1103/PhysRevE.61.4194
Thomson, J. J. (1985). The trolley problem. Yale Law J. 94, 1395–1415. doi: 10.2307/796133
Tononi, G., Boly, M., Massimini, M., and Koch, C. (2016). Integrated information theory: from consciousness to its physical substrate. Nat. Rev. Neurosci. 17, 450–461. doi: 10.1038/nrn.2016.44
Treisman, A. (1999). Solutions to the binding problem: progress through controversy and convergence. Neuron 24, 105–125. doi: 10.1016/S0896-6273(00)80826-0
Turing, A. (1936). On computable numbers, with an application to the Entscheidungsproblem. J. Math 58, 345–363.
Turing, A. (1950). Computing machinery and intelligence, mind. LIX LIX, 433–460. doi: 10.1093/mind/LIX.236.433
Vaswani, A., Shazeer, N., Parmar, N., Uszkoreit, J., Jones, L., Gomez, A. N., et al. (2017). Attention is all you need. Adv. Neural Inf. Proces. Syst. 30, 6000–6010. doi: 10.48550/arXiv.1706.03762
Velmans, M. (1991). Is human information processing conscious? Behav. Brain Sci. 14, 651–669. doi: 10.1017/S0140525X00071776
Velmans, M. (2012). Reflexive monism psychophysical relations among mind, matter, and consciousness. J. Conscious. Stud. 19, 143–165.
Von Glasersfeld, E. (1987). “Learning as a constructive activity” in Problems of representation in the teaching and learning of mathematics (Mahwah, NJ, USA: Lawrence Erlbaum Associates), 3–17.
Wiese, W., and Friston, K. J. (2021). The neural correlates of consciousness under the free energy principle: from computational correlates to computational explanation. Philos. Mind Sci. 2:9. doi: 10.33735/phimisci.2021.81
Woolf, N. J., and Hameroff, S. R. (2001). A quantum approach to visual consciousness. Trends Cogn. Sci. 5, 472–478. doi: 10.1016/S1364-6613(00)01774-5
Yeung, N., and Summerfield, C. (2012). Metacognition in human decision-making: confidence and error monitoring. Philos. Trans. R. Soc. B Biol. Sci. 367, 1310–1321. doi: 10.1098/rstb.2011.0416
Yudkowsky, E. (2008). “Artificial intelligence as a positive and negative factor in global risk” in Global Catastrophic Risks . eds. N. Bostrom and M. M. Ćirkovic, 308–345.
Yudkowsky, E. (2015). Rationality-from AI to zombies . Berkeley, CA, USA: Machine Intelligence Research Institute.
Zeki, S., and Marini, L. (1998). Three cortical stages of colour processing in the human brain. Brain J. Neurol. 121, 1669–1685. doi: 10.1093/brain/121.9.1669
Zhang, B., Anderljung, M., Kahn, L., Dreksler, N., Horowitz, M. C., and Dafoe, A. (2021). Ethics and governance of artificial intelligence: evidence from a survey of machine learning researchers. J. Artif. Intell. Res. 71, 591–666. doi: 10.1613/jair.1.12895
Keywords: conscious supremacy, artificial intelligence, consciousness, large language model, computation
Citation: Mogi K (2024) Artificial intelligence, human cognition, and conscious supremacy. Front. Psychol . 15:1364714. doi: 10.3389/fpsyg.2024.1364714
Received: 02 January 2024; Accepted: 26 April 2024; Published: 13 May 2024.
Reviewed by:
Copyright © 2024 Mogi. This is an open-access article distributed under the terms of the Creative Commons Attribution License (CC BY) . The use, distribution or reproduction in other forums is permitted, provided the original author(s) and the copyright owner(s) are credited and that the original publication in this journal is cited, in accordance with accepted academic practice. No use, distribution or reproduction is permitted which does not comply with these terms.
*Correspondence: Ken Mogi, [email protected]
Disclaimer: All claims expressed in this article are solely those of the authors and do not necessarily represent those of their affiliated organizations, or those of the publisher, the editors and the reviewers. Any product that may be evaluated in this article or claim that may be made by its manufacturer is not guaranteed or endorsed by the publisher.

IMAGES
VIDEO
COMMENTS
The aim of this article is to overview the importance and implications of infrequently published scientific hypotheses that may open new avenues of thinking and research. ... research design to test the hypothesis, and its ethical implications: Sections are chosen by the authors, depending on the topic: Introduction, Methods, Results and ...
A research hypothesis (also called a scientific hypothesis) is a statement about the expected outcome of a study (for example, a dissertation or thesis). To constitute a quality hypothesis, the statement needs to have three attributes - specificity, clarity and testability. Let's take a look at these more closely.
A research hypothesis is a statement that brings up a question and predicts what might happen. It's really important in the scientific method and is used in experiments to figure things out. Essentially, it's an educated guess about how things are connected in the research.
A research hypothesis is an assumption or a tentative explanation for a specific process observed during research. Unlike a guess, research hypothesis is a calculated, educated guess proven or disproven through research methods. ... Thus, knowing how to write a hypothesis is very important. Now that you have a firmer grasp on what a good ...
It seeks to explore and understand a particular aspect of the research subject. In contrast, a research hypothesis is a specific statement or prediction that suggests an expected relationship between variables. It is formulated based on existing knowledge or theories and guides the research design and data analysis. 7.
5. Phrase your hypothesis in three ways. To identify the variables, you can write a simple prediction in if…then form. The first part of the sentence states the independent variable and the second part states the dependent variable. If a first-year student starts attending more lectures, then their exam scores will improve.
A hypothesis (from the Greek, foundation) is a logical construct, interposed between a problem and its solution, which represents a proposed answer to a research question. It gives direction to the investigator's thinking about the problem and, therefore, facilitates a solution. Unlike facts and assumptions (presumed true and, therefore, not ...
As you refine your hypotheses, you can assess their research importance by asking how connected they are to problems your research community really wants to solve. ... We provided a partial answer in Chap. 1 to the question of a hypothesis' importance when we encouraged considering the ultimate goal to which a study's findings might contribute.
A hypothesis is a tentative statement about the relationship between two or more variables. It is a specific, testable prediction about what you expect to happen in a study. It is a preliminary answer to your question that helps guide the research process. Consider a study designed to examine the relationship between sleep deprivation and test ...
A research hypothesis, in its plural form "hypotheses," is a specific, testable prediction about the anticipated results of a study, established at its outset. It is a key component of the scientific method. Hypotheses connect theory to data and guide the research process towards expanding scientific understanding.
A research hypothesis is a specification of a testable prediction about what a researcher expects as the outcome of the study. It comprises certain aspects such as the population, variables, and the relationship between the variables. It states the specific role of the position of individual elements through empirical verification.
Introduction. In the interesting world of research studies, one important thing is the hypothesis. It is like a clever guess that helps researchers understand how variables interact and are connected.
The formulation of a hypothesis is based on existing knowledge, observations, and theories, and it should be specific, testable, and falsifiable. A specific hypothesis helps to define the research question, which is important in the research process as it guides the selection of an appropriate research design and methodology.
It's important to keep the focus and language of your hypothesis objective. An effective hypothesis in research is clearly and concisely written, and any terms or definitions clarified and defined. Specific language must also be used to avoid any generalities or assumptions. Use the following points as a checklist to evaluate the ...
Research problems and hypotheses are important means for attaining valuable knowledge. They are pointers or guides to such knowledge, or as formulated by Kerlinger ( 1986, p. 19): " … they direct investigation.". There are many kinds of problems and hypotheses, and they may play various roles in knowledge construction.
The steps to write a research hypothesis are: 1. Stating the problem: Ensure that the hypothesis defines the research problem. 2. Writing a hypothesis as an 'if-then' statement: Include the action and the expected outcome of your study by following a 'if-then' structure. 3.
Step 1: State your null and alternate hypothesis. After developing your initial research hypothesis (the prediction that you want to investigate), it is important to restate it as a null (H o) and alternate (H a) hypothesis so that you can test it mathematically.. The alternate hypothesis is usually your initial hypothesis that predicts a relationship between variables.
A hypothesis is a testable prediction about how the world will behave if our idea is correct, and it is often worded as an if-then statement (e.g., if I study all night, I will get a passing grade on the test). The hypothesis is extremely important because it bridges the gap between the realm of ideas and the real world.
A research hypothesis is a specific, clear prediction about the possible outcome of a scientific research study based on specific factors of the population. 4. Null Hypothesis: ... An alternative hypothesis is the opposite of the null hypothesis where we can find some statistical importance or relationship between two variables. In case of null ...
rela onship between variables. When formula ng a hypothesis deduc ve. reasoning is u lized as it aims in tes ng a theory or rela onships. Finally, hypothesis helps in discussion of ndings and ...
1 Sony Computer Science Laboratories, Shinagawa, Japan; 2 Collective Intelligence Research Laboratory, The University of Tokyo, Meguro, Japan; The computational significance of consciousness is an important and potentially more tractable research theme than the hard problem of consciousness, as one could look at the correlation of consciousness and computational capacities through, e.g ...
This research contributes to the overarching objectives of achieving carbon neutrality and enhancing environmental governance by examining the role of artificial intelligence-enhanced multi-energy optimization in rural energy planning within the broader context of a sustainable energy economy. By proposing an innovative planning framework that accounts for geographical and economic disparities ...
Background: Adequate bowel preparation is one of the most important prerequisite during the colonoscopy. It is conductive to detect the polypus, adenoma and early colorectal carcinoma and decrease the rate of overuse of medical resource. BianShi Moxibustion(BSM) is one of the most important Traditional Chinese Medicine(TCM) treatment options, which is called TCM proper technology. BSM is ...